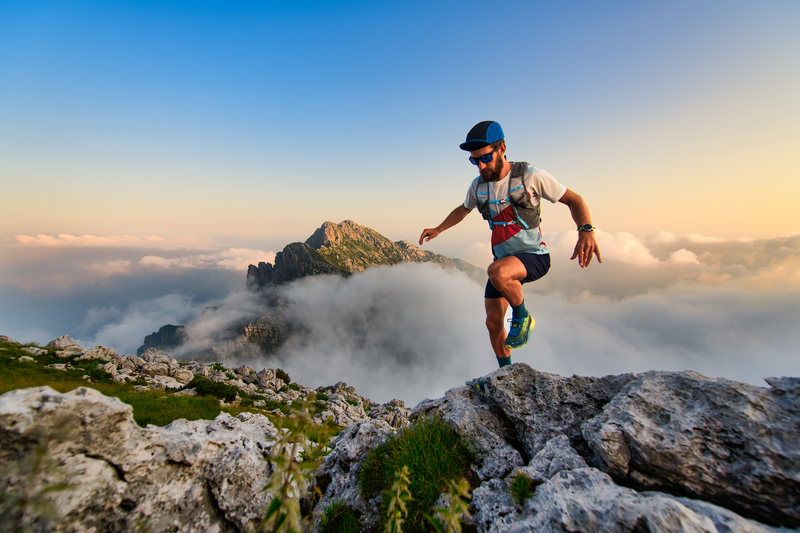
95% of researchers rate our articles as excellent or good
Learn more about the work of our research integrity team to safeguard the quality of each article we publish.
Find out more
ORIGINAL RESEARCH article
Front. Microbiol. , 04 October 2021
Sec. Microbiological Chemistry and Geomicrobiology
Volume 12 - 2021 | https://doi.org/10.3389/fmicb.2021.741523
This article is part of the Research Topic New Insights into the Microbial and Chemical Processes Controlling Mercury Biogeochemical Cycling View all 8 articles
Climate change dramatically impacts Arctic and subarctic regions, inducing shifts in wetland nutrient regimes as a consequence of thawing permafrost. Altered hydrological regimes may drive changes in the dynamics of microbial mercury (Hg) methylation and bioavailability. Important knowledge gaps remain on the contribution of specific microbial groups to methylmercury (MeHg) production in wetlands of various trophic status. Here, we measured aqueous chemistry, potential methylation rates (kmeth), volatile fatty acid (VFA) dynamics in peat-soil incubations, and genetic potential for Hg methylation across a groundwater-driven nutrient gradient in an interior Alaskan fen. We tested the hypotheses that (1) nutrient inputs will result in increased methylation potentials, and (2) syntrophic interactions contribute to methylation in subarctic wetlands. We observed that concentrations of nutrients, total Hg, and MeHg, abundance of hgcA genes, and rates of methylation in peat incubations (kmeth) were highest near the groundwater input and declined downgradient. hgcA sequences near the input were closely related to those from sulfate-reducing bacteria (SRB), methanogens, and syntrophs. Hg methylation in peat incubations collected near the input source (FPF2) were impacted by the addition of sulfate and some metabolic inhibitors while those down-gradient (FPF5) were not. Sulfate amendment to FPF2 incubations had higher kmeth relative to unamended controls despite no effect on kmeth from addition of the sulfate reduction inhibitor molybdate. The addition of the methanogenic inhibitor BES (25 mM) led to the accumulation of VFAs, but unlike molybdate, it did not affect Hg methylation rates. Rather, the concurrent additions of BES and molybdate significantly decreased kmeth, suggesting a role for interactions between SRB and methanogens in Hg methylation. The reduction in kmeth with combined addition of BES and molybdate, and accumulation of VFA in peat incubations containing BES, and a high abundance of syntroph-related hgcA sequences in peat metagenomes provide evidence for MeHg production by microorganisms growing in syntrophy. Collectively the results suggest that wetland nutrient regimes influence the activity of Hg methylating microorganisms and, consequently, Hg methylation rates. Our results provide key information about microbial Hg methylation and methylating communities under nutrient conditions that are expected to become more common as permafrost soils thaw.
Methylmercury (MeHg) is a potent neurotoxic compound that bioaccumulates and biomagnifies in aquatic food webs (USEPA, 1997). Northern freshwater wetlands are ecosystems that have been shown to be net sources of MeHg (Loseto et al., 2004; Tjerngren et al., 2012), and elevated MeHg concentrations in fish and mammals have been reported in the Arctic and subarctic regions (Dietz et al., 2013). Northern wetlands receive inorganic mercury primarily through atmospheric deposition of Hg(II) and direct uptake of gaseous elemental mercury by plants [and subsequent oxidation to Hg(II)] (Enrico et al., 2016; Jiskra et al., 2017; Obrist et al., 2017). Under anoxic conditions in wetlands, Hg(II) can be methylated to form MeHg (Loseto et al., 2004; Mitchell et al., 2008; Tjerngren et al., 2012; Gordon et al., 2016; Schaefer J. K. et al., 2020).
Mercury methylation is an anaerobic process carried out by bacteria and archaea. Formerly, the methylation of Hg has been largely attributed to sulfate- and iron-reducing bacteria (SRB and IRB, respectively) (Gilmour and Henry, 1992; Fleming et al., 2006). More recently, the discovery of the hgcAB genes encoding for the Hg methylase and associated ferredoxin (Parks et al., 2013) has revealed that several additional guilds of anaerobic microorganisms can methylate Hg, including methanogens (Gilmour et al., 2013, 2018; Yu et al., 2013, 2018), fermenters (Gilmour et al., 2013) and syntrophs (Gilmour et al., 2013; Yu et al., 2018). This advancement has provided an important gene marker for identifying environments and microbial taxa with a potential for Hg methylation (Bae et al., 2014; Schaefer J. K. et al., 2014; Christensen et al., 2016; Jones et al., 2020; McDaniel et al., 2020; Peterson et al., 2020). However, the contribution of newly identified methylating guilds to MeHg production in the environment remains to be demonstrated.
In peat-forming wetlands, primary production exceeds decomposition, resulting in the buildup of organic carbon stores (Gorham, 1991; Charman et al., 2013; Nichols and Peteet, 2019). Carbon degradation in the anoxic zone occurs through a combination of primary and secondary fermentation processes and anaerobic respiration. The relative contribution of these different pathways depends upon the availability of nutrients and suitable electron acceptors. When terminal electron acceptors are scarce, mineralization of fermentation products is driven primarily through syntrophy between secondary fermenters and methanogens (McInerney et al., 2008, 2009; Schmidt et al., 2016), whereby substrates are metabolized by two groups of organisms under conditions that are not thermodynamically favorable for either of them alone (Morris et al., 2013). Notably, many Hg methylating organisms are within metabolic guilds involved in anaerobic carbon degradation in peat-forming wetlands. The contribution of these metabolic guilds to MeHg formation and influence of nutrient availability on Hg methylating communities remain to be understood.
Permafrost loss in the Arctic and subarctic causes hydrological changes (Box et al., 2019) that can contribute nutrients in the form of terminal electron acceptors to wetland ecosystems (Reyes and Lougheed, 2015; Schadel et al., 2016). In addition, changes in wetland hydrology has the potential to contribute previously sequestered Hg to wetland active layers (Rydberg et al., 2010; Ci et al., 2020; Mu et al., 2020; Schaefer K. et al., 2020). Several studies have linked Hg methylation potential and MeHg accumulation to wetland trophic status (Tjerngren et al., 2012; Gordon et al., 2016; Poulin et al., 2019; Schaefer J. K. et al., 2020), but few have investigated the microbial processes driving these relationships, such as impacts of nutrient inputs on microbial community dynamics and corresponding Hg methylation potentials. Forecasting the implications of a warming climate on Hg risk to northern ecosystems necessitates an improved understanding of Hg methylating communities and methylation potential across nutrient regimes.
Here, we tested the hypothesis that nutrient inputs influence microbial community structures, increasing Hg methylation rates and MeHg accumulation in a fen wetland in interior Alaska. We characterized the biogeochemical conditions of the wetland and the active peat microbiome through 16S rRNA sequencing, hgcA amplicon sequencing, and metagenomics at six sites across a nutrient gradient. Potential rates of Hg methylation were measured along the gradient in incubations aimed at stimulating or inhibiting specific microbial guilds, to ultimately elucidate the metabolic pathways involved in MeHg formation. In this study, we show how inputs of nutrients can promote Hg methylation in subarctic peatlands by stimulating respiratory pathways when electron acceptors are present (e.g., sulfate) and through syntrophic interactions when these electron acceptors become depleted.
Six sites spanning a distance of 73 m within a fen wetland, referred to as Frozen Pond Fen (FPF) were identified in interior Alaska near Fairbanks (N64.91417, W147.83487). A strong geochemical gradient was observed across the fen, and Poulin et al. (2019) identified evidence for a groundwater input near FPF1. A site map is presented in Supplementary Figure 1, and site coordinates and pore water geochemistry are summarized in Table 1. Average monthly precipitation and daily temperature were collected from NOAA National Centers for Environmental Information station USC00503368 and are presented in SI. Average daily high temperature was similar between 2016 and 2018 (Supplementary Figure 2), however, average monthly rainfall prior to sampling dates was higher in 2016 (Supplementary Figure 3). Pore water and peat samples were collected in summers of 2016 (July 13) and 2018 (June 27). Samples for molecular characterization of the soil microbial communities were collected from 5 cm below the water table at each site. Approximately 1 g of soil was collected with 75% ethanol rinsed forceps and placed into 3 mL LifeGuard Soil Preservation Solution (Qiagen). Samples were stored on ice and transferred for temporary storage (−20°C) prior to shipping to Rutgers University, NJ, (within 5 days) where they were stored at −80°C until processing.
Table 1. Sampling locations in Frozen Pond Fen (2016 and 2018) and filtered pore water geochemical data from 10 cm below the water table (2016).
Peat soil samples for Hg methylation assays were collected from the saturated zone above the underlaying permafrost (∼5–10 cm below the water table) and placed in 0.95 L soda-lime glass Mason jars which were completely filled and immediately sealed. Peat soils were stored on ice for approximately 6 h prior to the initiation of methylation assays.
Sampling procedures, sample processing, storage, and analyses in 2016 were performed as previously described (Poulin et al., 2019) and included characterization of pore water pH, conductivity, anions, cations, DOC, specific UV absorbance at 254 nm (SUVA254), and Hg species including total Hg (THg), MeHg, and dissolved gaseous mercury [Hg(0)(aq)]. In 2018, pH was measured in situ. Pore water was collected from ∼5 cm below the water table into 15 mL Falcon tubes for cation and anion analysis, and into pre-muffled amber glass vials for DOC analysis. All samples were stored on ice for ∼6 h before being frozen (ions) or kept at 4°C (DOC) and shipped to Rutgers University for analysis.
DOC was measured in duplicate for each site using a Shimadzu TOC-VCSH. The lowest standard of 1 ppm represented the limit of detection. Ions were measured by ion chromatography (Thermo Scientific Dionex Aquion Ion Chromatograph) at the Dawson Lab in the Department of Environmental Sciences at Rutgers University. Anions were eluted on a Dionex IonPac AS11 4 × 50 mm guard column and 4 × 250 mm column coupled to a Dionex AERS 500 carbonate anion suppressor with a 9 mM NaCO3 eluent over 30 min. Cations were eluted on a Dionex IonPac CS12a 4 × 40 mm guard column and a 4 × 250 mm column coupled to a Dionex SC-CERS 500 salt converter cation suppressor with 20 mM methanesulfonic acid eluent over 20 min.
Detection limits are reported in Supplementary Table 2. Peat was homogenized and collected in silver foil for C:N analysis. Total organic carbon and total nitrogen were measured with a Carlo Erba NA1500 series 2 elemental analyzer in the Rutgers Department of Marine and Coastal Sciences.
Potential methylation assays were determined on the day of sampling using two different types of incubations. To account for variation in pore water chemistry and Hg speciation and the impact they may have on the availability of the substrate to the methylating communities (Gilmour et al., 1998), 198HgCl2 (for experiments performed in 2016) or 200HgCl2 (for experiments performed in 2018) was preequilibrated with pore water from FPF2 prior to its addition to incubations from both sites. Incubations were conducted in sites FPF2 and FPF5, as geochemical parameters in FPF1 were drastically higher than the rest of the gradient, and many parameters were considerably low or below detection in FPF4. Peat from FPF2 and FPF5 was initially homogenized by hand. Incubations were prepared in duplicate or triplicate with 5 g saturated peat added to 20 mL serum bottles under N2 flow. Individual samples were prepared for initial time point (T = 0 h) and final time point (T = 24 h) for each treatment. Each peat incubation was amended by pipette with the appropriate substrate/inhibitor (see below) and an enriched Hg(II) isotope (198Hg or 200Hg) pre-equilibrated with FPF2 site porewater for 4 h. Incubations were mixed following amendment addition with a borosilicate glass stir rod, sealed with Teflon stoppers, and flushed with N2. Time 0 incubations were immediately stored at −20°C and incubations were carried out at room temperature for 24 h, followed by storage at −20°C that stopped the experiment. Experimental treatments included a control (no amendment), sulfate addition (∼0.11 mM sodium sulfate), molybdate addition (∼0.2 mM sodium molybdate), 2-bromoethanesulfonic acid addition (∼25 mM BES), and a combination of both molybdate and BES (∼0.2 mM sodium molybdate, ∼25 mM BES). 198HgCl2 was incubated with pore water from site FPF2 for 4 h prior to spiking incubations. Molybdate, a structural analog to sulfate, has been shown to inhibit ATP sulfurylase (Peck, 1959; Taylor and Oremland, 1979) and is a specific inhibitor of dissimilatory sulfate reduction (Biswas et al., 2009; Sela-Adler et al., 2017). Molybdate concentrations used in this study (0.2 mM) have previously shown near complete inhibition of sulfate reduction in freshwater sediment with <0.1 mM sulfate (Fleming et al., 2006). BES is an analog of coenzyme M (Gunsalus et al., 1978) and specific inhibitor of methanogenesis (Oremland and Polcin, 1982; Compeau and Bartha, 1985).
Details regarding the analysis of enriched mercury stable isotopes in the incubations are presented in Supplementary Material. Peat was freeze-dried prior to Hg analysis. Potential Hg methylation rate constants (kmeth) in 2016 were calculated as [Me198Hg]/[T198Hg] × 100% (Note: T198Hg is total 198Hg detected in dried peat). Time 0 incubation kmeth averages were subtracted from time final incubation kmeth after 24 h. Due to limitations of sample material in 2018, T200Hg was not analyzed in each incubation and therefore, an average T200Hg was calculated for each site and used to calculate kmeth as [Me200Hg]/[T200Hg] × 100%.
In the laboratory, slurries from each site were prepared using previously established procedures (Duddleston et al., 2002; Zhang et al., 2020) in a glove bag filled with 100% N2 gas. Excess pore water was squeezed from the peat and woody plant roots and any remaining green vegetation were removed. About 100–300 g peat (wet weight) was homogenized with pore water in a blender at a ratio of ∼1:3 wet weight peat: total volume slurry. Slurries prepared in this manner contained 0.04–0.08 g dried peat per mL. In each of the 200 mL serum bottles, 50 mL of slurries were mixed with 100 mL MilliQ water and incubated for 50 days. On day 1, one set of serum bottle (n = 2) per treatment was amended with 1 mL of 500 mM BES to inhibit methanogenesis (Compeau and Bartha, 1985). Slurry samples (1.0 mL) for anion analysis were periodically collected from incubation bottles using a plastic 1.0 mL syringe. Samples were centrifuged (12,000 × g) for 5 min and the supernatant filtered through a 0.22 μm sterilized PES syringe filter (Celltreat). Anions (acetate, propionate, butyrate, SO42–, and NO3–) were measured by injecting 25 μL of filtered supernatant into an automated Dionex DX600 ion chromatograph (Thermo Scientific) fitted with an AS11HC ion exchange column (4 mm) with suppression and with a potassium hydroxide gradient eluent generator and conductivity detector.
Frozen peat samples were thawed at 4°C and centrifuged at 2500 × g for 5 min to remove the Lifeguard storage solution. RNeasy Powersoil Total RNA kit (Qiagen) and DNA extraction kit (Qiagen) were used to extract RNA and DNA, respectively, from the same original sample and as described by the manufacturer. RNA and DNA were quantified using both Qubit (ThermoFisher) and Nanodrop (ThermoFisher). RNA integrity was determined through gel electrophoresis and RNA and DNA extracts were stored at −80°C and −20°C, respectively. To prepare cDNA, RNA extracts were DNase treated using Turbo DNase Free Kit (Sigma) and Supercript III First Strand cDNA Kit (ThermoFisher). The V4 region of the 16S rRNA genes was amplified using primers 515F and 806R (Apprill et al., 2015; Parada et al., 2016) from DNA extracts and cDNA preparations, and amplicons were sequenced by Illumina MiSeq at MRDNA (Shallowater, TX) (2016 libraries) and at the Rutgers Genome Cooperative (2018 libraries).
hgcA amplicon libraries were prepared for DNA extracts from FPF1-6 (2016) with primers and protocol developed by Schaefer J. K. et al. (2014). Amplification products were separated by gel electrophoresis and bands corresponding to 650 bp were excised and purified (Wizard, Promega). Purified PCR products were shipped to MRDNA for sequencing by the PacBio Sequel platform according to manufacturer’s protocol, including the CCS2 algorithm which was used to generate a consensus sequence as secondary analysis.
DNA extracts from sites FPF1-6 (2016 and 2018) were used for shotgun metagenomic analysis by the Community Science Program of the Department of Energy Joint Genome Institute (CSP 504041). Details on rRNA removal, library construction, sequencing, and downstream analysis can be found on the JGI genome portal.1
Resulting raw sequence files were processed in QIIME2 (version 2018.6). Briefly, samples were imported, demultiplexed, pair-joined, trimmed of adapters, and denoised using the Deblur plugin (Amir et al., 2017). A phylogenetic tree was constructed with Fasttree (Price et al., 2010) and diversity metrics were calculated using the Diversity plugin. Samples were rarified to 8122, a depth which retained most samples and features. Taxonomic assignment was done using a Baysean-Naïve classifier trained on the V4 region of the Silva database (132–99%) (Quast et al., 2013; Bokulich et al., 2018). Biological replicates were averaged for all sites.
hgcA consensus amplicon sequences were demultiplexed and primer sequences were removed. Reference hgcA sequences based on ORNL predicted methylators list (Gionfriddo et al., 2019) were used to train and test a naïve-Bayesian classifier (Pedregosa et al., 2011) using the QIIME2 framework. Amplicon sequences were classified, and family level taxonomy is presented.
Shotgun metagenomes were processed through the JGI IMG pipeline (v 5.0.0) (Chen et al., 2019). Translated protein sequences were downloaded from the JGI Genome portal and a Hidden Markov Model presented in Gionfriddo et al. (2020) was used to search metagenome assemblies for HgcA sequences with an inclusion threshold of e-value <8 × 10–10. To determine this e-value threshold for including a sequence in our analysis, we used 627 reference sequences for Pfam03599 which contains CO dehydrogenase/acetyl-CoA synthase delta subunit. We removed Pfam03599 sequences containing the HgcA cap region [N(V/I)WCAA] (Smith et al., 2015) and ran the remaining 535 sequences against an HgcA HMM model using hmmsearch in HMMER.2 All sequences with e-value <1 × 10–5 were manually checked for the HgcA-like cap region following an NCBI BLAST search. An e-value threshold of <8 × 1010 was determined to exclude non-HgcA CO dehydrogenase/acetyl-CoA synthase delta subunit sequences. The e-value <8 × 10–10 cutoff includes long sequences and sequences that were downstream of the NVWCAA cap with confidence that they were incomplete HgcA. Corresponding DNA contigs were selected and taxonomic annotation assigned through the JGI IMG 5.0.0 pipeline for hgcA validated sequences was obtained from the JGI Genome portal. Because taxonomic affiliation was assigned on the entire contig (not just hgcA), greater resolution was obtained when compared to a tree placement method (i.e., pplacer). Final relative abundance of hgcA from each site was calculated as sequences that passed HMM filtering times the scaffold/contig coverage. Abundances were normalized per 106 total genes. We report hgcA taxonomy at the class level for all homologs and at the family level taxonomy for hits >60% identity score.
Marker genes for microbial metabolism were selected from the annotated metagenomes on JGI IMG database. KEGG Orthology ID K00399 was used to identify mcrA genes, K11181 was used to identify dsrB genes, and K00520 for merA genes. Since the relative abundances of dsrA and dsrB, encoding the alpha and beta subunits of the dissimilatory sulfite reductase, respectively, were highly correlated across the wetland, we focus our results and discussion on the latter, dsrB (K11181).
Shotgun metagenomes are available on the DOE JGI Genome Portal (see text foonote 1) and IMG platform.3 16S rRNA sequences are deposited at the National Science Foundation Arctic Data Center (Barkay and Roth, 2019). Statistical analyses were conducted in Microsoft Excel and R using the vegan (Oksanen et al., 2020) and stats packages. Correlations were conducted in R using the stat_cor function in ggpubr package. Distance across the fen was used as the independent variable for correlation analyses unless otherwise noted. All data used in coorelation analyses were tested for normality using a Shapiro-Wilk test. Normal data were subjected to Pearson coorelation and Spearman correlations were calculated for non-normal data. Methylation incubation data were determined to be normally distributed through Shapiro-Wilk test. One-way ANOVA was used to determine significance followed by t-test to determine significance between treatments.
Six sites were chosen in Frozen Pond Fen (FPF) along a natural groundwater-driven geochemical gradient. Lateral gradients of all measured parameters were apparent between FPF1 (nearest the groundwater source) and FPF6 (73 m from FPF1) (Table 1 and Supplementary Figure 4). Conductivity and Ca2+ were highest at FPF1 and significantly declined across the gradient (Pearson’s r = -0.903, p = 0.008 and Pearson’s r = −0.899, p = 0.009, respectively) (Table 1 and Supplementary Figure 4), indicative of a groundwater source near FPF1. pH also significantly declined along the fen gradient from site 1 to site 6 (Pearson’s r = −0.966, p < 0.001) with a maxima of pH 6.8 at FPF1. Dissolved organic carbon (DOC) decreased significantly from FPF1 to FPF6 (Spearman’s ρ = −1, p = 0.003), with reversed trends in the DOM SUVA254 (Pearson’s r = 0.828, p = 0.042), suggesting a lower aromaticity of the DOC nearest the source. The concentrations of sulfate and sulfide were highest in FPF1 (38 and 1.6 μmol/L, respectively), both declining to <1 μmol/L at FPF2 (27 m from FPF1). Manganese and iron concentrations were highest nearest FPF1 (15.6 and 103 μmol/L) but declined rapidly to near or below detection with distance from the groundwater source. Calliergon sp., Scorpidium revolvens, and Carex aquatalis were the dominant plant species in the Frozen Pond Fen with little variation between sites (Poulin et al., 2019). No correlation was observed between C:N and distance from FPF1, indicating that no large-scale differences in wetland trophic status occur across the fen.
As for most other parameters, pore water concentrations of dissolved gaseous mercury [Hg(0)(aq)], dissolved total mercury, and dissolved MeHg declined significantly with increasing distance from FPF1 (THg Pearson’s r = −0.854, p = 0.02; MeHg Spearman’s ρ = −0.928, p = 0.008) (Table 1 and Supplementary Figure 5). A positive correlation between MeHg and total dissolved Hg concentrations was observed (Spearman’s ρ = 0.81, p = 0.049) across all six sites. These results correspond to a decrease in the percentage of Hg as MeHg (%MeHg) along the gradient, with MeHg accounting for 18% of total Hg at FPF1 and for only 2% in FPF5 and FPF6. Interestingly, all measured Hg species were elevated in site FPF3 relative to those in FPF2 with Hg(0)(aq) and MeHg more than 4-fold and 1.7-fold higher, respectively, despite a minimal increase in total Hg.
The FPF sites were resampled in 2018, 2 years after the initial analyses. Resampling was conducted to validate previous gradient trends and to determine how the fen changed over the course of 2 years. Significant negative trends in pore water pH, Ca2+, and DOC were observed across the fen again in 2018 (Supplementary Figure 4 and Supplementary Table 2). Average pH and sulfate (FPF1 only) was higher in 2018 when compared to 2016 (2018 pH = 6.25, 2016 pH = 5.95) (Supplementary Figure 4 and Supplementary Table 4). Rainfall was higher in 2016 than 2018 immediately preceding sampling which may have contributed to some of the differences in porewater chemistry between sampling years (Supplementary Figure 3). In situ Hg species were not measured in 2018.
The microbial community was assessed through (1) 16S rRNA gene and transcript metataxonomic analysis, (2) shotgun metagenomic analyses, and (3) high-throughput amplicon sequencing of hgcA genes (2016 only). This multifaceted approach allowed us to identify shifts in the microbial community composition between sites, identify potential methylators, and compare sequence-based approaches.
The microbial community as assessed by 16S rRNA sequencing of cDNA was dominated by Proteobacteria across all sites (Figure 1). Site FPF1 had the highest community diversity (Supplementary Table 3), and the relative abundance of Proteobacteria increased from the nutrient rich to nutrient poor locations of the fen. In 2018, the community composition and diversity were less variable across the gradient, but overall community alpha diversity was significantly higher when compared to 2016 (Kruskal-Wallis, p = 0.0039) (Supplementary Table 3). Several families with known methylating organisms were identified in the 16S rRNA sequencing libraries (Supplementary Table 4), including Syntrophaceae, Geobacteraceae, Desulfobulbaceae, Desulfobacteraceae, Ruminococcaceae, and Methanoregulaceae.
Figure 1. Microbial community structure based on 16S rRNA amplicon reads in Frozen Pond Fen in (A) 2016 and (B) 2018. Phylum level taxonomy is displayed, and the top 12 phyla are presented. Phyla with relative abundances <2% are grouped into “Other”.
hgcA homologs were extracted from shotgun metagenomes and taxonomically classified across the gradient to assess the genetic potential for mercury methylation. In 2016, hgcA relative abundance was significantly negatively correlated with distance from FPF1 (Pearson’s r = −0.83 p = 0.042) and showed similar trends of %MeHg along the gradient (ρ = −0.77, p = 0.1) (Figure 2A).
Figure 2. The abundance and taxonomic distribution of hgcA genes in Frozen Pond Fen in 2016 and their relationship with pore water %MeHg. (A) Normalized hgcA gene copies in shotgun metagenomes and the %MeHg decline with increased distance across the fen gradient. Pearson (R) and Spearman (ρ) correlation statistics are displayed. (B) Class level distribution of hgcA sequences from the shotgun metagenomes (left) and amplicon sequencing (right). (C) Family level distribution of hgcA reads from shotgun metagenomes and amplicon sequencing.
Bacterial hgcA sequences were more abundant than archaeal sequences in the shotgun metagenomes from all sites (average bacterial abundance = 97 genes per metagenome; average archaeal abundance = 19.7 genes per metagenome), with Desulfobacterota (formerly Deltaproteobacteria) being the dominant phylum of hgcA sequences classified (Figure 2B). Sequences that had amino acid identity scores >60% were classified further to family level. In sites FPF1, FPF2, FPF4, and FPF5, >38% of hgcA sequences belonged to families that can participate in obligate or facultative syntrophic interactions including Syntrophaceae, Desulfobacteraceae, Syntrophorhabdaceae, Syntrophomonadaceae, Methanoregulaceae, Methanospirillaceae, Methanocorpusculaceae, and Methanosarcinaceae (Figure 2C). hgcA gene-homolog families belonging to the class Clostridia were in higher relative abundance in sites FPF2, FPF4, and FPF5 when compared to the nutrient rich site, FPF1 (Figure 2C). Site FPF6 had no hgcA sequences with identity scores >60% in 2016, so family data is displayed as unclassified (Figure 2C). hgcA diversity was highest in FPF1, followed by decreasing diversity along the gradient with a low at site FPF5 (Supplementary Table 3).
In comparison to the shotgun metagenomes, hgcA sequences obtained with high-throughput, primer-based amplicon sequencing aligned with only two Hg methylating taxa, Desulfobacterota and Methanomicrobia (Figure 2B, Methanomicrobia reads <1%). This is not surprising given the PCR primer bias toward the Desulfobacterota (Schaefer J. K. et al., 2014) and the abundance of these classes in the metagenome libraries. This PCR approach had clear limitations for identifying alternative groups of potential Hg methylators (e.g., Clostridia) and assessing the relative distribution of families within the Desulfobacterota. Two families of SRB, Desulfobacteraceae and Desulfovibrionaceae, known for robust Hg methylation activities (Gilmour et al., 2011), dominated the hgcA amplicon sequences in FPF1 despite their low abundance in the metagenome libraries. Geobacteraceae hgcA amplicon reads were detected in all FPF sites, albeit at low levels with a maximum proportion of 6% in FPF6 (Figure 2C), in agreement with the metagenome libraries.
In 2018, hgcA copy number from shotgun metagenomes was not significantly correlated with distance from FPF1, and a more even distribution of hgcA genes was observed along the gradient (Pearson’s r = −0.46, p = 0.36) when compared to 2016 (Supplementary Figure 6A). Average hgcA diversity was higher than in 2016 with lower variability (Shannon’s H 2016 = 2.12 ± 1.01, 2018 = 2.52 ± 0.49), however, this difference was not significant (Kruskal-Wallis, p = 0.44) (Supplementary Table 3). Similar to 2016, site FPF1 had the highest diversity of hgcA sequences in 2018 (Supplementary Table 3). hgcA sequences that aligned with organisms with syntrophic metabolism were highest in sites FPF1 and FPF2, whereas sequences most similar to those of the class Clostridia were found in highest relative abundance in FPF5 (Supplementary Figure 6C). All FPF sites in 2018 had hgcA sequences that aligned with metabolically diverse microbial families (Supplementary Figure 6C).
Results from the mercury methylating community were further validated by investigation of marker genes for microbial metabolism. In both years (2016 and 2018), dsrB, a marker for sulfate reduction, was highest in FPF1, coincident with the highest sulfate porewater concentrations (Supplementary Table 5). dsrB abundance tended to decline along the gradient, although the trend was weaker in 2018 (Pearson’s r = −0.59, p = 0.22) than in 2016 (Pearson’s r = −0.734, p = 0.097). The average dsrB gene counts were higher in 2018 (mean = 96.5) compared to 2016 (mean = 64.9) when sulfate concentrations in the groundwater inflows were also higher (Supplementary Table 2). A significant correlation between hgcA and dsrB was seen across the gradient in 2016 (Pearson’s r = 0.98, p < 0.001), but not in 2018. The marker gene for methanogenesis, mcrA, was detected in all sites and had no clear trend in either year (Supplementary Table 5).
The gene encoding for the mercuric reductase, merA, was detected along the gradient in both years (Supplementary Table 5). No trend in normalized abundance was observed along the gradient in either year. While merA encodes for one potential mechanism for Hg(0)(aq) formation, no significant correlation was observed between merA abundance and Hg(0)(aq), suggesting other mechanisms (Wiatrowski et al., 2006; Gregoire et al., 2018) may be important for the elevated levels of Hg(0) detected in the fen (Table 1; Poulin et al., 2019).
To assess the impact of the groundwater input on Hg methylation, potential Hg methylation rates by peat slurries were measured in sites FPF2 and FPF5 representing the nutrient rich and nutrient poor portions of the gradient, respectively. Treatments, designed to elucidate the contribution of specific microbial guilds to Hg methylation, included (1) sulfate addition, which can stimulate SRB, (2) molybdate addition, which inhibits SRB, (3) BES addition, which inhibits methanogenesis (Oremland and Capone, 1988), and (4) molybdate and BES which inhibit both SRB and methanogens.
Potential methylation rate constants in unamended incubations were nearly 5-fold higher (p = 0.013) in FPF2 than in FPF5 (9.3 and 2.0 kmeth/day, respectively) (Figure 3) in 2016, corresponding to higher hgcA gene abundances and diversity in the former (Figure 2). Sulfate addition (∼0.11 mM) to the FPF2 slurry incubation increased kmeth 1.8-fold in 2016 (p = 0.09) when compared to the control slurry (Figure 3). This suggests that Hg methylation in the control slurries was potentially limited by sulfate availability and that the community was primed to utilize sulfate, consistent with the presence of SRB in 16S rRNA libraries (Figure 1) and dsrB genes in the shotgun metagenomes (Supplementary Table 5). However, kmeth in FPF2 was not significantly impacted by the addition of molybdate, suggesting SRB played a limited role in Hg methylation in the control incubation. While SRB were present and active (Figures 1, 2), the lack of significant reduction in kmeth by molybdate indicates that other microbial guilds substantially contributed to methylation to mask the impact of the inhibitor on kmeth (Figure 3). The specific inhibitor of methanogenesis, BES, had no significant effect on kmeth, likewise ruling out methanogens as the major Hg methylating group in FPF2 (Figure 3). However, when molybdate and BES were added concurrently, a 42% decrease in kmeth was observed (2016 p = 0.076; not significant) (Figure 3), supporting a role for both SRB and methanogens in the methylation of mercury that was not detected when inhibitors were added individually.
Figure 3. Mercury methylation rates (kmeth) at sites FPF2 (gray bars) and FPF5 (black bars) in 2016. X-axis labels indicate treatment with stimulator or inhibitors of specific microbial guilds. Error bars represent standard deviations. * means p < 0.10, ** means p < 0.05.
In contrast, incubations from site FPF5 were not significantly impacted by sulfate or inhibitor (molybdate/BES) amendment in 2016. This indicates that methylation of Hg was likely carried out by a metabolic guild that was not impacted by BES or molybdate (Figure 3) such as IRB or fermenters. In fact, Geobacteraceae hgcA genes were detected in highest relative abundance in sites FPF3–FPF6, suggesting a potential role for IRB in Hg methylation down-gradient (Figure 2 and Table 1).
In 2018, kmeth was higher in both sites compared to 2016, and statistically similar (p = 0.82) in the FPF2 and FPF5 control incubations (Supplementary Figure 7). Similar to 2016, kmeth was 1.7-fold higher when sulfate was added. When added independent of one another, molybdate and BES had no significant impact on Hg methylation rates in FPF2, but concurrent addition of both inhibitors resulted in a 45% decrease in kmeth as seen in 2016 (Figure 3).
Contrary to 2016, Hg methylation in incubations from FPF5 in 2018 was significantly stimulated by the addition of either molybdate and BES when added alone, but not when added concurrently (Supplementary Figure 7). Sulfate addition resulted in a 2-fold increase in kmeth, however, this was not significant (p = 0.25) (Supplementary Figure 7). These results suggest that SRB and methanogens were not the only methylating guilds in FPF5. Molybdate and BES may have stimulated guilds not impacted by the inhibitors by reducing competition for resources, one possible explanation for the increase in kmeth. As in 2016, metabolic guilds not tested for could be primary Hg methylators in FPF5, including IRB and fermenters.
The abundance of hgcA homologs closely related to syntrophs and the significant decrease in kmeth with concurrent addition of BES and molybdate indicated that syntrophic interactions may have contributed to Hg methylation in the fen. To further determine the role of syntrophy in the fen, we monitored VFAs in peat slurry incubations of 2016 samples with and without the methanogen inhibitor BES as an indication of syntrophic interactions between methanogens and VFA fermenters. After an initial pulse of VFAs from slurry preparation and organic matter decomposition, VFA concentrations declined in unamended control incubations (no BES) reaching below detection levels by day 43 (Figure 4 and Supplementary Figure 8). When methanogens were inhibited by the addition of BES, VFA concentrations (i.e., butyrate, propionate, and acetate) did not decline, but continued to accumulate until plateauing to a constant level after ∼36 days. By consuming the products of propionate and butyrate fermentation (acetate and H2), methanogens promote the fermentation of these VFAs. Propionate and butyrate are not directly utilized by methanogens (Evans et al., 2019), thus their accumulation in the presence of BES demonstrates the importance of syntrophic interactions between VFA oxidizers and methanogens in driving mineralization of organic matter when terminal electron acceptors are depleted.
Figure 4. Concentration of acetate, propionate, and butyrate over 50 days in peat slurry incubations from FPF2 (A) and FPF5 (B). Solid lines represent no-amendment controls and dashed lined represent incubations to which BES was added to inhibit methanogens. Acetate and propionate concentrations are displayed on the left y-axis and butyrate is displayed on the right. Note the difference in y-axis scales between subplots (A) and (B).
Production and consumption of VFAs was faster in FPF2 than in FPF5, and BES treatment resulted in 2–6-fold higher concentrations of individual VFAs in FPF2 when compared to FPF5. Known syntrophic taxa, such as the Syntrophaceae, were higher in relative abundance nearer the groundwater source (FPF1 and FPF2) than further downgradient (FPF5), coincident with higher VFA accumulations following BES treatment (Supplementary Table 4).
Similar responses to BES treatment were observed in the other FPF sites, except for site FPF4, where VFAs accumulated in both control and treatment incubations (Supplementary Figure 8). Methanogenic taxa and mcrA genes were detected in FPF4 at abundances similar to the surrounding sites (Supplementary Table 5), and initial acetate concentrations in the FPF4 slurry were higher than all other sites, possibly disrupting the system. Collectively, the results across the fen suggest syntrophy is a major mineralization pathway of secondary fermentation products (i.e., VFAs) and support results of the community analysis and Hg methylation incubations.
We examined an Alaskan fen to evaluate the effects of groundwater nutrient inputs on soil microbiome structure and microbial Hg methylation. Our results (1) connect nutrient inputs in northern peatlands to increased diversity and abundance of Hg methylating communities and increased Hg methylation potentials, and (2) document the role of syntrophic interactions in Hg methylation. To our knowledge, this is the first study to pair activity measurements with hgcA sequencing through shotgun metagenomics to reveal the role of syntrophy in Hg methylation in the environment. Importantly, our results provide evidence for the involvement of syntrophy in Hg methylation in an ecosystem that is critical to the global Hg cycle due to vast stores of Hg with connections to aquatic food webs in the Arctic and subarctic (Dietz et al., 2013).
Nutrient inputs in Frozen Pond Fen impacted microbial community structure and Hg methylation rates. Microbial communities clearly responded to nutrient inputs in the Frozen Pond Fen (Table 1 and Supplementary Figure 4) as evidenced by a corresponding decline in community diversity away from the source (Supplementary Table 3). More importantly, the abundance of hgcA gene homologs in peat metagenomes declined significantly along the gradient, closely reflecting the decline in %MeHg (Figure 2A). These negative correlations with distance from the groundwater source suggest that nutrient inputs provide conditions that promote activity of Hg methylating microorganisms in the fen. Groundwater brings electron donors and acceptors (Table 1), supporting anaerobic respiration such as that carried out by SRBs. This was reflected in the community composition as SRB were more abundant nearest the groundwater source at FPF1 and FPF2 (Figure 2 and Supplementary Table 5). Despite no decrease in kmeth with molybdate addition in FPF2, sulfate addition significantly enhanced Hg methylation in FPF2 incubations (Figure 3). These results suggest that SRB were not directly contributing to Hg methylation in FPF2 but that the community was primed to utilize sulfate and that subsequent releases of sulfate into the fen could promote MeHg production by directly stimulating SRB Hg methylators or by stimulating non-methylating SRB that support the growth of Hg methylating syntrophs. Sulfate-reducing bacteria are well-known, efficient Hg methylators (Gilmour and Henry, 1992; Gilmour et al., 2013), and our results indicate that increases in warming-associated nutrient inputs (Reyes and Lougheed, 2015) may stimulate SRB, resulting in increased MeHg accumulation in these wetlands. While SRB may be a minor component of the total microbial community, their activity can contribute significantly to organic matter breakdown in fen systems even at low micromolar sulfate concentrations (Pester et al., 2010).
Our conclusion is supported by previous research in wetlands that has shown sites of intermediate trophic status, such as fens, have high net MeHg production. These intermediate sites have higher kmeth compared to ombrotrophic bogs (Tjerngren et al., 2012; Poulin et al., 2019; Schaefer J. K. et al., 2020), while having lower rates of demethylation compared to eutrophic swamps (Kronberg et al., 2012). Fens generally have higher pH and sulfate concentrations than bogs, and a link between those pore water geochemical parameters and %MeHg has been observed (Gordon et al., 2016). Furthermore, Hg methylation potential (kmeth) in peatlands has been shown to be significantly correlated with a natural sulfur gradient and could be stimulated with experimental sulfur addition (Akerblom et al., 2020), in line with our results showing increased kmeth with sulfate addition in the Frozen Pond Fen. The results from the Frozen Pond Fen expand our understanding of Hg dynamics in peatlands by linking the genetic potential for Hg methylation with previously reported trends in Hg methylation potentials based on nutrient status.
When electron acceptors such as sulfate become depleted, syntrophy becomes an increasingly more important metabolic pathway. Here we show that that increase promotes Hg methylation. Molecular evidence and activity measurements in the Frozen Pond Fen incubations both provide evidence for Hg methylation through syntrophy. Many FPF metagenomic hgcA reads are closely related to those of organisms that can participate in facultative or obligate syntrophy in the nutrient rich sites, including methanogenic families as well as Syntrophaceae, Desulfobacteraceae, Syntrophorhabdaceae, Syntrophomonadaceae, and Peptococcaceae (Figure 2C). The 16S rRNA sequences validated these results, with a large relative abundance of Syntrophaceae and Syntrophobacteraceae in nutrient richer sites (Supplementary Table 4). The Syntrophorhabdaceae, Syntrophomonadaceae, as well as two genera in the Syntrophaceae, Syntrophus, and Smithella, are largely obligate syntrophs requiring an H2 scavenging partner (Kuever, 2014a, b). The hgcA amplicon sequencing showed high relative abundances of two SRB families that could participate in facultative syntrophic interactions; however, a clear discrepancy between sequencing methods was observed (Figure 2). Our results show that metagenomic sequencing may better describe Hg methylator diversity due to reduced bias compared to primer-based approaches as has been previously suggested (Christensen et al., 2019). Overwhelmingly, our results indicate that the genetic potential for Hg methylation by syntrophy is dominant in the Frozen Pond Fen, especially in nutrient rich sites (Figure 2).
The molecular results supporting syntrophy involvement in Hg methylation were validated by peat incubations in FPF2 and FPF5 of both Hg methylation potential and VFA dynamics (Figures 3, 4, respectively). The lack of inhibition of kmeth by molybdate suggests methylation by microbial guilds other than SRB (Compeau and Bartha, 1985), possibly stimulated by their inhibition. Several methanogen species have been shown to methylate (Yu et al., 2013; Gilmour et al., 2018), however, BES had no inhibitory effect on MeHg formation (Figure 3). Together, these observations indicate that several guilds, likely interacting with each other, contributed to methylation. When molybdate and BES were added concurrently, Hg methylation was significantly inhibited in FPF2 (Figure 3) suggesting that syntrophs, likely coupled to H2 and acetate utilizers, contribute to MeHg formation in the fen. Two genera in the Syntrophaceae, as well as the Desulfobacteraceae and some Peptococcaceae (Lee et al., 2009) are able to grow syntrophically or reduce sulfate when it becomes available (Muyzer and Stams, 2008), potentially explaining stimulation with sulfate while no inhibition with molybdate was observed. Likewise, our results also showed that the VFAs acetate, propionate, and butyrate built up in incubations treated with BES (Figure 4). Because propionate and butyrate cannot be utilized directly by methanogens (Evans et al., 2019), their accumulation after BES treatment suggests that methanogens were growing in syntrophy with VFA fermenters. Acetate accumulated in BES treated slurries by the first time point (t = 1 day), indicating that methanogenesis was inhibited (Figure 4) while propionate and butyrate accumulated after 15 days. Fermentation of VFAs will be greatly inhibited if the byproducts of fermentation, namely acetate and H2, are not consumed. The time lag in propionate and butyrate build up with BES treatment could therefore indicate that non-methanogenic H2 and acetate utilizing organisms support the growth of syntrophs in FPF under shorter time periods (>15 days).
Syntrophic metabolic niches have been studied in peat-forming wetlands previously (Schmidt et al., 2016). The contributions of syntrophic interactions to MeHg production by syntrophic interactions has been recently suggested (Bae et al., 2014; Hu et al., 2020; Schaefer J. K. et al., 2020), and hgcA genes and transcripts closely related to the syntrophic genus Smithella have been detected in permafrost impacted peatlands (McDaniel et al., 2020). In order to be active and contribute to MeHg production, syntrophs must metabolize with a partner microorganism that can utilize secondary fermentation end products such as H2 and acetate (Muyzer and Stams, 2008). Many methanogens and SRB can utilize acetate or H2, and SRB will outcompete methanogens when sulfate is available. In the absence of sulfate, SRB can also grow syntrophically with methanogens by fermenting volatile fatty acids (Muyzer and Stams, 2008). Yu et al. (2018) showed that these various syntrophic interactions may support methylation of Hg regardless of which syntrophic partner has methylating capabilities. Correspondingly, we observed significant reduction in kmeth in FPF peat only when both major H2 and acetate utilizing guilds were inhibited by BES and molybdate. As contemporary sequencing methods continue to identify the full extent of hgcA presence and diversity, it is important to consider the contribution of newly identified guilds to MeHg production in the environment. By pairing activity measurements with sequence-based approaches, our results robustly indicate Hg methylation by microorganisms growing in syntrophy in the environment.
We did not directly test the role of IRB or fermenters in Hg methylation through our inhibitor incubations. However, when both BES and molybdate were added to peat incubations, MeHg was still formed in both sites in both years (Figure 3). This MeHg formation is not attributed to SRB, methanogens, or major syntrophic pathways, suggesting a role for other microbial metabolic guilds. 16S rRNA sequencing and metagenomes hgcA gene homologs showed an abundance of sequences associated with the IRB family, Geobacteraceae, as well as the class Clostridia which includes Hg methylating strains that are metabolically diverse, including SRB, IRB, fermenters, dehalorespirers, and microorganisms that can participate in syntrophy (Figure 2). Species in Geobacteraceae have been shown to be strong methylators in pure culture (Kerin et al., 2006; Schaefer and Morel, 2009) and have been associated with environmental MeHg production (Bravo et al., 2018). Hg methylators within the class Clostridia grown in pure culture have lower methylation rates than the phylum Desulfobacterota, in general (Gilmour et al., 2013). Some hgcA sequences in the metagenomes were also related to Dehalococcoidia and Bacteroidia, two classes for which Hg methylation potentials are unknown.
Comparing the results of our 2016 sampling with those obtained for the same locations in 2018 supports our conclusion. Trends in kmeth and community characteristics were less defined in 2018 (Supplementary Figure 7), corresponding to a dampened geochemical gradient (Supplementary Figure 4). Increased precipitation could have diluted nutrients in FPF in 2016 (Siegel and Glaser, 1987), and may have resulted in a higher water table which could inhibit microbial activity and impact MeHg concentrations (Haynes et al., 2017). Unlike in 2016, kmeth in FPF5 were similar to those observed in FPF2 (Supplementary Figure 7) and the decline in hgcA gene copy number along the gradient was much less pronounced (Supplementary Figure 6). Taxonomic classification of hgcA reads in 2018 revealed abundant SRB, syntrophic, and methanogenic families (Supplementary Figure 6), a finding that was further validated by the 16S rRNA results (Figure 1). Similar to results from site FPF2 in 2016, the concurrent addition of molybdate and BES to peat incubations in 2018 led to a significant decline in kmeth in both sites, indicating that syntrophy contributed to Hg methylation (Supplementary Figure 7).
In peatland ecosystems underlain by permafrost, rapid thawing as a result of increasing air temperatures is causing numerous changes including expansion of the active layer (Akerman and Johansson, 2008), release of previously sequestered nutrients into groundwater or surface water (Reyes and Lougheed, 2015), and changes in hydrology. While we did not determine the hydrology across FPF, pore water geochemical factors were significantly correlated with distance from the edge of the fen where a groundwater input was identified (Table 1). The impacts of warming-associated changes on microbial activities (Jansson and Tas, 2014) and supply of nutrients (Ma et al., 2019) are profound. Collectively, our results show that Frozen Pond Fen is an ecosystem in transition, biogeochemically responding to environmental changes. As the Arctic and subarctic are altered in response to climate change, conditions in ombrotrophic peatlands may shift to favor robust Hg methylating communities. Paired with potential Hg release from permafrost (Rydberg et al., 2010; Mu et al., 2020; Schaefer K. et al., 2020), these changes point to a potential for increased MeHg production and release from northern wetlands.
The datasets presented in this study can be found in online repositories. The names of the repository/repositories and accession number(s) can be found below: https://arcticdata.io/catalog/view/; doi: 10.18739/A2X921J5D; https://genome.jgi.doe.gov/portal/EffofApeatlands/EffofApeatlands.info.html, 504041.
TB, MH, JS, DK, BP, and SR: conception and design of experiments. SR, BP, ZB, XL, LZ, DK, MH, JS, and TB: collection, analysis, and interpretation of all data. SR: writing of the manuscript. BP, ZB, LZ, JS, and TB: revision of the manuscript. All authors contributed to the manuscript and have approved the submitted version.
This research was supported by the United States National Science Foundation grant PLR-1304773 to MH and TB, United States National Science Foundation ARC #1304804 and Macrosystem Biology 1241937 to MH. SR was supported by United States National Science Foundation Graduate Research Fellowship Program grant DGE 1842213. Metagenomic sequencing was provided by the United States Department of Energy Joint Genome Institute Community Science Project 504041. The work conducted by the United States Department of Energy Joint Genome Institute, a DOE Office of Science User Facility, was supported by the Office of Science of the United States Department of Energy under Contract No. DE-AC02-05CH11231.
The authors declare that the research was conducted in the absence of any commercial or financial relationships that could be construed as a potential conflict of interest.
All claims expressed in this article are solely those of the authors and do not necessarily represent those of their affiliated organizations, or those of the publisher, the editors and the reviewers. Any product that may be evaluated in this article, or claim that may be made by its manufacturer, is not guaranteed or endorsed by the publisher.
We extend our gratitude to Mary Beth Leigh and Eric Henderson of the University of Alaska, Fairbanks, who have provided logistic support to our field campaigns.
The Supplementary Material for this article can be found online at: https://www.frontiersin.org/articles/10.3389/fmicb.2021.741523/full#supplementary-material
Akerblom, S., Nilsson, M. B., Skyllberg, U., Bjorn, E., Jonsson, S., Ranneby, B., et al. (2020). Formation and mobilization of methylmercury across natural and experimental sulfur deposition gradients. Environ. Pollut. 263(Pt A), 114398. doi: 10.1016/j.envpol.2020.114398
Akerman, H. J., and Johansson, M. (2008). Thawing permafrost and thicker active layers in sub-arctic Sweden. Permafrost Periglac. Process. 19, 279–292. doi: 10.1002/ppp.626
Amir, A., McDonald, D., Navas-Molina, J. A., Kopylova, E., Morton, J. T., Xu, Z. Z., et al. (2017). Deblur rapidly resolves single-nucleotide community sequence patterns. mSystems 2:e00191-16. doi: 10.1128/mSystems.00191-16
Apprill, A., McNally, S., Parsons, R., and Weber, L. (2015). Minor revision to V4 region SSU rRNA 806R gene primer greatly increases detection of SAR11 bacterioplankton. Aquat. Microb. Ecol. 75, 129–137. doi: 10.3354/ame01753
Bae, H. S., Dierberg, F. E., and Ogram, A. (2014). Syntrophs dominate sequences associated with the mercury methylation-related gene hgcA in the water conservation areas of the Florida Everglades. Appl. Environ. Microbiol. 80, 6517–6526. doi: 10.1128/AEM.01666-14
Barkay, T., and Roth, S. (2019). Interior Alaskan Bog to fen Gradient Wetland Soil 16S rRNA (Ribosomal ribonucleic acid) Metagenomes, Alaska, 2013-2018. Available online at: https://search.dataone.org/view/doi%3A10.18739%2FA2X921J5D (accessed September 1, 2019).
Biswas, K., Woodards, N., Xu, H. F., and Barton, L. (2009). Reduction of molybdate by sulfate-reducing bacteria. Biometals 22, 131–139. doi: 10.1007/s10534-008-9198-8
Bokulich, N. A., Kaehler, B. D., Rideout, J. R., Dillon, M., Bolyen, E., Knight, R., et al. (2018). Optimizing taxonomic classification of marker-gene amplicon sequences with QIIME 2 ’ s q2-feature-classifier plugin. Microbiome 6:90. doi: 10.1186/s40168-018-0470-z
Box, J. E., Colgan, W. T., Christensen, T. R., Schmidt, N. M., Lund, M., Parmentier, F. J. W., et al. (2019). Key indicators of Arctic climate change: 1971-2017. Environ. Res. Lett. 14:045010. doi: 10.1088/1748-9326/aafc1b
Bravo, A. G., Zopfi, J., Buck, M., Xu, J. Y., Bertilsson, S., Schaefer, J. K., et al. (2018). Geobacteraceae are important members of mercury-methylating microbial communities of sediments impacted by waste water releases. ISME J. 12, 802–812. doi: 10.1038/s41396-017-0007-7
Charman, D. J., Beilman, D. W., Blaauw, M., Booth, R. K., Brewer, S., Chambers, F. M., et al. (2013). Climate-related changes in peatland carbon accumulation during the last millennium. Biogeosciences 10, 929–944. doi: 10.5194/bg-10-929-2013
Chen, I. M. A., Chu, K., Palaniappan, K., Pillay, M., Ratner, A., Huang, J. H., et al. (2019). IMG/M v.5.0: an integrated data management and comparative analysis system for microbial genomes and microbiomes. Nucleic Acids Res. 47, D666–D677. doi: 10.1093/nar/gky901
Christensen, G. A., Gionfriddo, C. M., King, A. J., Moberly, J. G., Miller, C. L., Somenahally, A. C., et al. (2019). Determining the Reliability of Measuring Mercury Cycling Gene Abundance with Correlations with Mercury and Methylmercury Concentrations. Environ. Sci. Technol. 53, 8649–8663. doi: 10.1021/acs.est.8b06389
Christensen, G. A., Wymore, A. M., King, A. J., Podar, M., Hurt, R. A., Santillan, E. U., et al. (2016). Development and validation of broad-range qualitative and clade-specific quantitative molecular probes for assessing mercury methylation in the environment. Appl. Environ. Microbiol. 82, 6068–6078. doi: 10.1128/AEM.01271-16
Ci, Z. J., Peng, F., Xue, X., and Zhang, X. S. (2020). Permafrost Thaw dominates mercury emission in Tibetan Thermokarst Ponds. Environ. Sci. Technol. 54, 5456–5466. doi: 10.1021/acs.est.9b06712
Compeau, G. C., and Bartha, R. (1985). Sulfate-Reducing Bacteria - Principal Methylators of Mercury in Anoxic Estuarine Sediment. Appl. Environ. Microbiol. 50, 498–502. doi: 10.1128/aem.50.2.498-502.1985
Dietz, R., Sonne, C., Basu, N., Braune, B., O’Hara, T., Letcher, R. J., et al. (2013). What are the toxicological effects of mercury in Arctic biota? Sci. Total Environ. 443, 775–790. doi: 10.1016/j.scitotenv.2012.11.046
Duddleston, K. N., Kinney, M. A., Kiene, R. P., and Hines, M. E. (2002). Anaerobic microbial biogeochemistry in a northern bog: acetate as a dominant metabolic end product. Glob. Biogeochem. Cycles 16:1063. doi: 10.1029/2001GB001402
Enrico, M., Le Roux, G., Marusczak, N., Heimburger, L. E., Claustres, A., Fu, X. W., et al. (2016). Atmospheric mercury transfer to peat bogs dominated by gaseous elemental mercury dry deposition. Environ. Sci. Technol. 50, 2405–2412. doi: 10.1021/acs.est.5b06058
Evans, P. N., Boyd, J. A., Leu, A. O., Woodcroft, B., Parks, D. H., Hugenholtz, P., et al. (2019). An evolving view of methane metabolism in the Archaea. Nat. Rev. Microbiol. 17, 219–232. doi: 10.1038/s41579-018-0136-7
Fleming, E. J., Mack, E. E., Green, P. G., and Nelson, D. C. (2006). Mercury methylation from unexpected sources: molybdate-inhibited freshwater sediments and an iron-reducing bacterium. Appl. Environ. Microbiol. 72, 457–464. doi: 10.1128/AEM.72.1.457-464.2006
Gilmour, C. C., Bullock, A. L., McBurney, A., Podar, M., and Elias, D. A. (2018). Robust Mercury Methylation across Diverse Methanogenic Archaea. mBio 9:e02403-17. doi: 10.1128/mBio.02403-17
Gilmour, C. C., Elias, D. A., Kucken, A. M., Brown, S. D., Palumbo, A. V., Schadt, C. W., et al. (2011). Sulfate-Reducing Bacterium Desulfovibrio desulfuricans ND132 as a Model for Understanding Bacterial Mercury Methylation. Appl. Environ. Microbiol. 77, 3938–3951. doi: 10.1128/AEM.02993-10
Gilmour, C. C., and Henry, E. A. (1992). Mercury Methylation by Sulfate-Reducing Bacteria - Biogeochemical and Pure Culture Studies. Abstracts of Papers of the American Chemical Society 203, 140-Geoc. Washington, DC: American Chemical Society.
Gilmour, C. C., Podar, M., Bullock, A. L., Graham, A. M., Brown, S. D., Somenahally, A. C., et al. (2013). Mercury Methylation by novel microorganisms from new environments. Environ. Sci. Technol. 47, 11810–11820. doi: 10.1021/es403075t
Gilmour, C. C., Riedel, G. S., Ederington, M. C., Bell, J. T., Benoit, J. M., Gill, G. A., et al. (1998). Methylmercury concentrations and production rates across a trophic gradient in the northern Everglades. Biogeochemistry 40, 327–345. doi: 10.1023/A:1005972708616
Gionfriddo, C. M., Wymore, A. M., Jones, D. S., Wilpiszeski, R. L., Lynes, M. M., Christensen, G. A., et al. (2020). An ImprovedhgcABPrimer Set and direct high-throughput sequencing expand Hg-Methylator diversity in nature. Front. Microbiol. 11:541554. doi: 10.3389/fmicb.2020.541554
Gionfriddo, C., Podar, M., Gilmour, C., Pierce, E., and Elias, D. (2019). ORNL Compiled Mercury Methylator Database [Online]. RNLCIFSFA (Critical Interfaces Science Focus Area). Oak Ridge, TN: Oak Ridge National Lab.
Gordon, J., Quinton, W., Branfireun, B. A., and Olefeldt, D. (2016). Mercury and methylmercury biogeochemistry in a thawing permafrost wetland complex, Northwest Territories, Canada. Hydrol. Process. 30, 3627–3638. doi: 10.1002/hyp.10911
Gorham, E. (1991). Northern Peatlands - role in the carbon-cycle and probable responses to climatic warming. Ecol. Appl. 1, 182–195. doi: 10.2307/1941811
Gregoire, D. S., Lavoie, N. C., and Poulain, A. J. (2018). Heliobacteria reveal fermentation as a key pathway for mercury reduction in anoxic environments. Environ. Sci. Technol. 52, 4145–4153. doi: 10.1021/acs.est.8b00320
Gunsalus, R. P., Romesser, J. A., and Wolfe, R. S. (1978). Preparation of Coenzyme-M analogs and their activity in Methyl Coenzyme-M Reductase System of Methanobacterium thermoautotrophicum. Biochemistry 17, 2374–2377. doi: 10.1021/bi00605a019
Haynes, K. M., Kane, E. S., Potvin, L., Lilleskov, E. A., Kolka, R. K., and Mitchell, C. P. J. (2017). Mobility and transport of mercury and methylmercury in peat as a function of changes in water table regime and plant functional groups. Glob. Biogeochem. Cycles 31, 233–244. doi: 10.1002/2016GB005471
Hu, H., Wang, B., Bravo, A. G., Björn, E., Skyllberg, U., Amouroux, D., et al. (2020). Shifts in mercury methylation across a peatland chronosequence: from sulfate reduction to methanogenesis and syntrophy. J. Hazard. Mater. 387:121967. doi: 10.1016/j.jhazmat.2019.121967
Jansson, J. K., and Tas, N. (2014). The microbial ecology of permafrost. Nat. Rev. Microbiol. 12, 414–425. doi: 10.1038/nrmicro3262
Jiskra, M., Wiederhold, J. G., Skyllberg, U., Kronberg, R. M., and Kretzschmar, R. (2017). Source tracing of natural organic matter bound mercury in boreal forest runoff with mercury stable isotopes. Environ. Sci. Process. Impacts 19, 1235–1248. doi: 10.1039/C7EM00245A
Jones, D. S., Johnson, N. W., Mitchell, C. P. J., Walker, G. M., Bailey, J. V., Pastor, J., et al. (2020). Diverse Communities of hgcAB(+) Microorganisms Methylate Mercury in Freshwater Sediments Subjected to Experimental Sulfate Loading. Environ. Sci. Technol. 54, 14265–14274. doi: 10.1021/acs.est.0c02513
Kerin, E. J., Gilmour, C. C., Roden, E., Suzuki, M. T., Coates, J. D., and Mason, R. P. (2006). Mercury methylation by dissimilatory iron-reducing bacteria. Appl. Environ. Microbiol. 72, 7919–7921. doi: 10.1128/AEM.01602-06
Kronberg, R. M., Tjerngren, I., Drott, A., Bjorn, E., and Skyllberg, U. (2012). Net degradation of methyl mercury in alder swamps. Environ. Sci. Technol. 46, 13144–13151. doi: 10.1021/es303543k
Kuever, J. (2014a). “The family Syntrophaceae,” in The Prokaryotes: Deltaproteobacteria and Epsilonproteobacteria, eds E. Rosenberg, E. F. DeLong, S. Lory, E. Stackebrandt, and F. Thompson (Berlin: Springer), 281–288.
Kuever, J. (2014b). “The family Syntrophorhabdaceae,” in The Prokaryotes: Deltaproteobacteria and Epsilonproteobacteria, eds E. Rosenberg, E. F. DeLong, S. Lory, E. Stackebrandt, and F. Thompson (Berlin: Springer), 301–303.
Lee, Y. J., Romanek, C. S., and Wiegel, J. (2009). Desulfosporosinus youngiae sp nov., a spore-forming, sulfate-reducing bacterium isolated from a constructed wetland treating acid mine drainage. Int. J. Syst. Evol. Microbiol. 59, 2743–2746. doi: 10.1099/ijs.0.007336-0
Loseto, L. L., Siciliano, S. D., and Lean, D. R. S. (2004). Methylmercury production in High Arctic wetlands. Environ. Toxicol. Chem. 23, 17–23. doi: 10.1897/02-644
Ma, Q., Jin, H. J., Yu, C. R., and Bense, V. F. (2019). Dissolved organic carbon in permafrost regions: a review. Sci. China Earth Sci. 62, 349–364. doi: 10.1007/s11430-018-9309-6
McDaniel, E. A., Peterson, B. D., Stevens, S. L. R., Tran, P. Q., Anantharaman, K., and McMahon, K. D. (2020). Expanded phylogenetic diversity and metabolic flexibility of mercury-methylating microorganisms. mSystems 5:e00299-20. doi: 10.1128/mSystems.00299-20
McInerney, M. J., Sieber, J. R., and Gunsalus, R. P. (2009). Syntrophy in anaerobic global carbon cycles. Curr. Opin. Biotechnol. 20, 623–632. doi: 10.1016/j.copbio.2009.10.001
McInerney, M. J., Struchtemeyer, C. G., Sieber, J., Mouttaki, H., Stams, A. J. M., Schink, B., et al. (2008). Physiology, ecology, phylogeny, and genomics of microorganisms capable of syntrophic metabolism. Ann. N. Y. Acad. Sci. 1125, 58–72. doi: 10.1196/annals.1419.005
Mitchell, C. P. J., Branfireun, B. A., and Kolka, R. K. (2008). Spatial characteristics of net methylmercury production hot spots in peatlands. Environ. Sci. Technol. 42, 1010–1016. doi: 10.1021/es0704986
Morris, B. E., Henneberger, R., Huber, H., and Moissl-Eichinger, C. (2013). Microbial syntrophy: interaction for the common good. FEMS Microbiol. Rev. 37, 384–406. doi: 10.1111/1574-6976.12019
Mu, C. C., Schuster, P. F., Abbott, B. W., Kang, S. C., Guo, J. M., Sun, S. W., et al. (2020). Permafrost degradation enhances the risk of mercury release on Qinghai-Tibetan Plateau. Sci. Total Environ. 708:135127. doi: 10.1016/j.scitotenv.2019.135127
Muyzer, G., and Stams, A. J. M. (2008). The ecology and biotechnology of sulphate-reducing bacteria. Nat. Rev. Microbiol. 6, 441–454. doi: 10.1038/nrmicro1892
Nichols, J. E., and Peteet, D. M. (2019). Rapid expansion of northern peatlands and doubled estimate of carbon storage. Nat. Geosci. 12, 917–921. doi: 10.1038/s41561-019-0454-z
Obrist, D., Agnan, Y., Jiskra, M., Olson, C. L., Colegrove, D. P., Hueber, J., et al. (2017). Tundra uptake of atmospheric elemental mercury drives Arctic mercury pollution. Nature 547, 201–204. doi: 10.1038/nature22997
Oksanen, J., Blanchet, F. G., Friendly, M., Kindt, R., Legendre, P., McGlinn, D., et al. (2020). vegan: Community Ecology Package. R package version 2.5-7. Available online at: https://CRAN.R-project.org/package=vegan (accessed January 1, 2020).
Oremland, R. S., and Capone, D. G. (1988). Use of specific inhibitors in biogeochemistry and microbial ecology. Adv. Microb. Ecol. 10, 285–383. doi: 10.1007/978-1-4684-5409-3_8
Oremland, R. S., and Polcin, S. (1982). Methanogenesis and sulfate reduction - competitive and noncompetitive substrates in estuarine sediments. Appl. Environ. Microbiol. 44, 1270–1276. doi: 10.1128/aem.44.6.1270-1276.1982
Parada, A., Walters, W., Hyde, E. R., Berg-Lyons, D., Ackermann, G., Humphrey, G., et al. (2016). Improved Bacterial 16S rRNA Gene (V4 and V4-5) and fungal internal transcribed spacer marker gene primers for microbial community surveys. mSystems 1:e00009-15. doi: 10.1128/mSystems.00009-15
Parks, J. M., Johs, A., Podar, M., Bridou, R., Hurt, R. A., Smith, S. D., et al. (2013). The genetic basis for bacterial mercury Methylation. Science 339, 1332–1335. doi: 10.1126/science.1230667
Peck, H. D. (1959). The Atp-Dependent Reduction of Sulfate with Hydrogen in Extracts of Desulfovibrio Desulfuricans. Proc. Natl. Acad. Sci. U.S.A. 45, 701–708. doi: 10.1073/pnas.45.5.701
Pedregosa, F., Varoquaux, G., Gramfort, A., Michel, V., Thirion, B., Grisel, O., et al. (2011). Scikit-learn: machine learning in python. J. Mach. Learn. Res. 12, 2825–2830.
Pester, M., Bittner, N., Deevong, P., Wagner, M., and Loy, A. (2010). A ‘rare biosphere’ microorganism contributes to sulfate reduction in a peatland. ISME J. 4, 1591–1602. doi: 10.1038/ismej.2010.75
Peterson, B. D., McDaniel, E. A., Schmidt, A. G., Lepak, R. F., Janssen, S. E., Tran, P. Q., et al. (2020). Mercury methylation genes identified across diverse anaerobic microbial guilds in a eutrophic sulfate-enriched lake. Environ. Sci. Technol. 54, 15840–15851. doi: 10.1021/acs.est.0c05435
Poulin, B. A., Ryan, J. N., Tate, M. T., Krabbenhoft, D. P., Hines, M. E., Barkay, T., et al. (2019). Geochemical factors controlling dissolved elemental mercury and methylmercury formation in alaskan wetlands of varying trophic status. Environ. Sci. Technol. 53, 6203–6213. doi: 10.1021/acs.est.8b06041
Price, M. N., Dehal, P. S., and Arkin, A. P. (2010). FastTree 2-approximately maximum-likelihood trees for large alignments. PLoS One 5:e9490. doi: 10.1371/journal.pone.0009490
Quast, C., Pruesse, E., Yilmaz, P., Gerken, J., Schweer, T., Yarza, P., et al. (2013). The SILVA ribosomal RNA gene database project: improved data processing and web-based tools. Nucleic Acids Res. 41, D590–D596. doi: 10.1093/nar/gks1219
Reyes, F. R., and Lougheed, V. L. (2015). Rapid nutrient release from permafrost thaw in arctic aquatic ecosystems. Arctic Antarct. Alp. Res. 47, 35–48. doi: 10.1657/AAAR0013-099
Rydberg, J., Klaminder, J., Rosen, P., and Bindler, R. (2010). Climate driven release of carbon and mercury from permafrost mires increases mercury loading to sub-arctic lakes. Sci. Total Environ. 408, 4778–4783. doi: 10.1016/j.scitotenv.2010.06.056
Schadel, C., Bader, M. K. F., Schuur, E. A. G., Biasi, C., Bracho, R., Capek, P., et al. (2016). Potential carbon emissions dominated by carbon dioxide from thawed permafrost soils. Nat. Clim. Change 6, 950–953. doi: 10.1038/nclimate3054
Schaefer, J. K., Kronberg, R. M., Bjorn, E., and Skyllberg, U. (2020). Anaerobic guilds responsible for mercury methylation in boreal wetlands of varied trophic status serving as either a methylmercury source or sink. Environ. Microbiol. 22, 3685–3699. doi: 10.1111/1462-2920.15134
Schaefer, J. K., Kronberg, R. M., Morel, F. M. M., and Skyllberg, U. (2014). Detection of a key Hg methylation gene, hgcA, in wetland soils. Environ. Microbiol. Rep. 6, 441–447. doi: 10.1111/1758-2229.12136
Schaefer, J. K., and Morel, F. M. M. (2009). High methylation rates of mercury bound to cysteine by Geobacter sulfurreducens. Nat. Geosci. 2, 123–126. doi: 10.1038/ngeo412
Schaefer, K., Elshorbany, Y., Jafarov, E., Schuster, P. F., Striegl, R. G., Wickland, K. P., et al. (2020). Potential impacts of mercury released from thawing permafrost. Nat. Commun. 11:4650. doi: 10.1038/s41467-020-18398-5
Schmidt, O., Hink, L., Horn, M. A., and Drake, H. L. (2016). Peat: home to novel syntrophic species that feed acetate- and hydrogen-scavenging methanogens. ISME J. 10, 1954–1966. doi: 10.1038/ismej.2015.256
Sela-Adler, M., Ronen, Z., Herut, B., Antler, G., Vigderovich, H., Eckert, W., et al. (2017). Co-existence of methanogenesis and sulfate reduction with common substrates in sulfate-rich estuarine sediments. Front. Microbiol. 8:766. doi: 10.3389/fmicb.2017.00766
Siegel, D. I., and Glaser, P. H. (1987). Groundwater-flow in a bog fen complex, lost River Peatland, Northern Minnesota. J. Ecol. 75, 743–754. doi: 10.2307/2260203
Smith, S. D., Bridou, R., Johs, A., Parks, J. M., Elias, D. A., Hurt, R. A., et al. (2015). Site-directed mutagenesis of HgcA and HgcB reveals amino acid residues important for mercury methylation. Appl. Environ. Microbiol. 81, 3205–3217. doi: 10.1128/AEM.00217-15
Taylor, B. F., and Oremland, R. S. (1979). Depletion of adenosine-triphosphate in desulfovibrio by Oxyanions of Group-Vi Elements. Curr. Microbiol. 3, 101–103. doi: 10.1007/BF02602440
Tjerngren, I., Karlsson, T., Bjorn, E., and Skyllberg, U. (2012). Potential Hg methylation and MeHg demethylation rates related to the nutrient status of different boreal wetlands. Biogeochemistry 108, 335–350. doi: 10.1007/s10533-011-9603-1
USEPA (1997). ”Mercury Study Report to Congress. Volume VII: Characterization of Human Health and Wildlife Risks from Mercury Exposure in the United States EPA-452/R-97-009. Washington, DC: US EPA.
Wiatrowski, H. A., Ward, P. M., and Barkay, T. (2006). Novel reduction of mercury(II) by mercury-sensitive dissimilatory metal reducing bacteria. Environ. Sci. Technol. 40, 6690–6696. doi: 10.1021/es061046g
Yu, R. Q., Reinfelder, J. R., Hines, M. E., and Barkay, T. (2013). Mercury Methylation by the Methanogen Methanospirillum hungatei. Appl. Environ. Microbiol. 79, 6325–6330. doi: 10.1128/AEM.01556-13
Yu, R. Q., Reinfelder, J. R., Hines, M. E., and Barkay, T. (2018). Syntrophic pathways for microbial mercury methylation. ISME J. 12, 1826–1835. doi: 10.1038/s41396-018-0106-0
Keywords: climate change, peatland, hgcA, syntrophy, mercury methylation
Citation: Roth S, Poulin BA, Baumann Z, Liu X, Zhang L, Krabbenhoft DP, Hines ME, Schaefer JK and Barkay T (2021) Nutrient Inputs Stimulate Mercury Methylation by Syntrophs in a Subarctic Peatland. Front. Microbiol. 12:741523. doi: 10.3389/fmicb.2021.741523
Received: 14 July 2021; Accepted: 13 September 2021;
Published: 04 October 2021.
Edited by:
Sukhwan Yoon, Korea Advanced Institute of Science and Technology, South KoreaReviewed by:
Marisol Goni-Urriza, Université de Pau et des Pays de l’Adour, FranceCopyright © 2021 Roth, Poulin, Baumann, Liu, Zhang, Krabbenhoft, Hines, Schaefer and Barkay. This is an open-access article distributed under the terms of the Creative Commons Attribution License (CC BY). The use, distribution or reproduction in other forums is permitted, provided the original author(s) and the copyright owner(s) are credited and that the original publication in this journal is cited, in accordance with accepted academic practice. No use, distribution or reproduction is permitted which does not comply with these terms.
*Correspondence: Jeffra K. Schaefer, c2NoYWVmamVAZW52c2NpLnJ1dGdlcnMuZWR1
†Deceased
Disclaimer: All claims expressed in this article are solely those of the authors and do not necessarily represent those of their affiliated organizations, or those of the publisher, the editors and the reviewers. Any product that may be evaluated in this article or claim that may be made by its manufacturer is not guaranteed or endorsed by the publisher.
Research integrity at Frontiers
Learn more about the work of our research integrity team to safeguard the quality of each article we publish.