- 1Climate and Ecosystem Sciences Division, Lawrence Berkeley National Laboratory, Berkeley, CA, United States
- 2Department of Civil and Environmental Engineering, University of California, Los Angeles, Los Angeles, CA, United States
- 3Department of Environmental Science, Policy, and Management, University of California, Berkeley, Berkeley, CA, United States
- 4Department of Molecular and Cell Biology, University of California, Berkeley, Berkeley, CA, United States
- 5Department of Plant and Microbial Biology, University of California, Berkeley, Berkeley, CA, United States
- 6Energy and Resources Group, University of California, Berkeley, Berkeley, CA, United States
Current knowledge of the mechanisms driving soil organic matter (SOM) turnover and responses to warming is mainly limited to surface soils, although over 50% of global soil carbon is contained in subsoils. Deep soils have different physicochemical properties, nutrient inputs, and microbiomes, which may harbor distinct functional traits and lead to different SOM dynamics and temperature responses. We hypothesized that kinetic and thermal properties of soil exoenzymes, which mediate SOM depolymerization, vary with soil depth, reflecting microbial adaptation to distinct substrate and temperature regimes. We determined the Michaelis-Menten (MM) kinetics of three ubiquitous enzymes involved in carbon (C), nitrogen (N) and phosphorus (P) acquisition at six soil depths down to 90 cm at a temperate forest, and their temperature sensitivity based on Arrhenius/Q10 and Macromolecular Rate Theory (MMRT) models over six temperatures between 4–50°C. Maximal enzyme velocity (Vmax) decreased strongly with depth for all enzymes, both on a dry soil mass and a microbial biomass C basis, whereas their affinities increased, indicating adaptation to lower substrate availability. Surprisingly, microbial biomass-specific catalytic efficiencies also decreased with depth, except for the P-acquiring enzyme, indicating distinct nutrient demands at depth relative to microbial abundance. These results suggested that deep soil microbiomes encode enzymes with intrinsically lower turnover and/or produce less enzymes per cell, reflecting distinct life strategies. The relative kinetics between different enzymes also varied with depth, suggesting an increase in relative P demand with depth, or that phosphatases may be involved in C acquisition. Vmax and catalytic efficiency increased consistently with temperature for all enzymes, leading to overall higher SOM-decomposition potential, but enzyme temperature sensitivity was similar at all depths and between enzymes, based on both Arrhenius/Q10 and MMRT models. In a few cases, however, temperature affected differently the kinetic properties of distinct enzymes at discrete depths, suggesting that it may alter the relative depolymerization of different compounds. We show that soil exoenzyme kinetics may reflect intrinsic traits of microbiomes adapted to distinct soil depths, although their temperature sensitivity is remarkably uniform. These results improve our understanding of critical mechanisms underlying SOM dynamics and responses to changing temperatures through the soil profile.
Introduction
Soils are estimated to contain ∼3,000 Gt carbon (C), which is more than all C in the atmosphere and in living biomass combined (Köchy et al., 2015). The dynamics of the large soil C reservoir is sensitive to climate change, and C losses as carbon dioxide (CO2) are expected to become a major positive feedback to global warming through increased soil organic matter (SOM) decomposition (Crowther et al., 2016; Van Gestel et al., 2018). An estimated 55 ± 50 Gt C may be lost globally from just 1°C warming of the upper 10 cm of soil alone (Crowther et al., 2016). While current model predictions of C dynamics and responses to climate change are largely based on surface soils (Trumbore, 2009; Crowther et al., 2016; Van Gestel et al., 2018), soils below 20 cm contain up to 50% of the global soil C budget within the top 1 m of soil (Jobbágy and Jackson, 2000; Balesdent et al., 2018). These subsoils are predicted to warm at rates similar to those of surface soils (Soong et al., 2020), and recent in situ deep soil warming experiments have shown uniform warming responses down to 100–120 cm depth leading to soil C losses at least three times higher than those estimated based on surface soils alone (Hicks Pries et al., 2017; Hanson et al., 2020; Nottingham et al., 2020; Soong et al., 2021). Despite these observations, relatively little is known about the microbial mechanisms and interactions mediating SOM turnover and CO2 emissions, and their responses to environmental changes in subsoils (Rumpel and Kögel-Knabner, 2011; Gross and Harrison, 2019), which are essential to improve predictions of SOM dynamics in response to warming.
Soil physicochemical properties and environmental conditions, such as nutrient inputs, temperature, moisture, mineralogy, and organic matter composition vary markedly with depth, creating distinct environments for the microbial processes that mediate SOM transformations (Trumbore, 2000; Blume et al., 2002; Fierer et al., 2003a; Salomé et al., 2010; Rumpel and Kögel-Knabner, 2011; Jones et al., 2018). The rate-limiting steps in SOM decomposition are primarily catalyzed by microbial exoenzymes, which depolymerize plant and microbial residues into lower molecular weight compounds that are assimilated by both plants and microbes (Davidson and Janssens, 2006; Burns et al., 2013). The kinetic and thermal properties of exoenzymes are therefore fundamental determinants of SOM turnover, nutrient availability, soil C stability, and greenhouse gas emissions, as well as their responses to environmental changes (Davidson and Janssens, 2006; Wallenstein et al., 2011; Sinsabaugh and Shah, 2012; Chen et al., 2018). In addition to the large diversity of exoenzymes targeting different organic compounds, evolutionarily distinct exoenzymes that catalyze the same reactions (i.e., isozymes) can vary widely in their kinetic properties, namely their catalytic rate constant, or turnover number (kcat), and related maximal reaction velocity (Vmax), their Michaelis constant (Km), which is inversely proportional to their affinity for the substrate, and their catalytic efficiency (kcat/Km) (Khalili et al., 2011; Nannipieri et al., 2012; Sinsabaugh and Shah, 2012; Tischer et al., 2015). These properties constitute microbial evolutionary adaptations and trade-offs related to resource supply and demand, as well as other environmental constraints, such as temperature and pH, associated with distinct ecological niches (Allison et al., 2011; Sinsabaugh and Shah, 2012; Ho et al., 2017; Malik et al., 2020). In soils, for example, exoenzyme kinetics have been shown to reflect variation in nutrient availability, pH, climate, and plant root proximity (Baker and Allison, 2017; Zhang et al., 2018; Puissant et al., 2019; Tian et al., 2020). Temperature is also a major factor controlling microbial community assembly, growth and functionality (Allison and Treseder, 2008; Bradford, 2013; Cavicchioli et al., 2019; Lax et al., 2020), and warming has been shown to change the abundance of diverse taxa and functional groups through the soil profile (Jiang et al., 2020; Dove et al., 2021). Moreover, temperatures of optimal enzyme activity are broadly correlated with the optimal growth temperatures of their organisms, as well as with the frequency of specific metabolic pathways, reflecting a concerted evolutionary adaptation to temperature and associated selective pressures (Somero, 2004; Engqvist, 2018). Therefore, variation in substrate and temperature regimes through the soil profile is likely to select for microbiomes producing enzymes with distinct kinetic and thermal properties, which may impose depth-dependent constraints on SOM turnover and responses to warming (Allison and Treseder, 2008; Carrillo et al., 2018; Cavicchioli et al., 2019; Isobe et al., 2019; Nunan et al., 2020; Xu et al., 2021). Microbial community composition and functional potential have indeed been shown to vary strongly with soil depth, reflecting selective adaptation to distinct niches (Blume et al., 2002; Fierer et al., 2003b; Hansel et al., 2008; Hartmann et al., 2009; Eilers et al., 2012; Turner et al., 2017; Jiao et al., 2018; Brewer et al., 2019; Diamond et al., 2019; Liu et al., 2019; Yan et al., 2019; Dove et al., 2021; Zosso et al., 2021). At the same time, exoenzyme activities in nature are dependent on multiple factors that can directly or indirectly modulate their kinetics, thermodynamics, and expression, beyond the intrinsic traits of the microbiome and the enzymes they encode. In particular, microbe-plant interactions, soil properties, and environmental conditions all affect enzyme expression, turnover, mobility, and substrate accessibility (Davidson and Janssens, 2006; Wallenstein et al., 2011; Bradford, 2013; Burns et al., 2013; Tang and Riley, 2019). Consequently, the effective kinetics of mixed exoenzyme pools in complex environments are emergent properties that reflect not only the summation of traits from distinct isozymes and organisms, but also direct and indirect interactions between enzymes and the environment (Davidson and Janssens, 2006; Sinsabaugh and Shah, 2012; Burns et al., 2013).
Given the critical role of exoenzymes in soil C stability and CO2 emissions, their activities and environmental controls have been extensively studied in the context of warming and other environmental changes, as indicators of SOM decomposition activity and nutrient availability (Allison et al., 2011; Sinsabaugh and Shah, 2012; Burns et al., 2013). Despite efforts to also integrate kinetic and thermal properties of exoenzymes to better understand the mechanisms of SOM turnover in response to warming (Wallenstein et al., 2011; German et al., 2012; Burns et al., 2013; Razavi et al., 2015, 2016; Alster et al., 2016a,2020; Loeppmann et al., 2016a; Allison et al., 2018), most studies have focused on one kinetic property (i.e., Vmax) and/or on surface soils. Several studies have investigated exoenzyme activities through the soil profile (Taylor et al., 2002; Venkatesan and Senthurpandian, 2006; Gelsomino and Azzellino, 2011; Kramer et al., 2013; Schnecker et al., 2014, 2015; Stone et al., 2014; Loeppmann et al., 2016a; Jing et al., 2017; Darby et al., 2020; Dove et al., 2020). However, nearly all of these studies, possibly with just one exception (Loeppmann et al., 2016a), have relied on enzyme activity assays based on single substrate concentrations and have not experimentally determined the Michaelis-Menten (MM) kinetics required to accurately estimate Vmax, as well as Km and catalytic efficiency, which cannot be otherwise inferred. While both approaches share the same technical limitations and must be interpreted in the context of complex enzyme pools and environmental samples, assays using single substrate concentrations are also prone to underestimate the full enzyme activity potential (i.e., Vmax), as substrate may be below the enzyme saturation point, or exceed it to the point of inhibition (Wallenstein et al., 2011). Soil temperature regimes are well-known to vary with depth, as heat diffusion is dampened through the soil profile, leading to narrower temperature ranges in deeper soils and preventing them from reaching the same temperature extremes as those at the surface (Al-Kaisi et al., 2017). However, the temperature sensitivity of soil exoenzymes produced by microbes potentially adapted to these distinct depth-dependent temperature regimes has rarely been characterized (Steinweg et al., 2013). Moreover, studies that determined both the MM kinetics of exoenzymes and their direct temperature sensitivity are scarce, even for surface soils (Razavi et al., 2015, 2016; Allison et al., 2018).
The temperature sensitivity of soil exoenzymes and other biogeochemical processes has been typically determined based on the linear Arrhenius model and related Q10 coefficient, which represents a simple empirical metric expressing variation in activity rates at every 10°C change in temperature (Alster et al., 2020). However, it has been argued that the Q10 coefficient may not reliably represent soil biological processes, as it lacks a biological and mechanistic basis, and does not capture the unimodality of typical enzyme reactions (Hobbs et al., 2013; Alster et al., 2020). These caveats possibly explain the frequent inability of Q10-values to describe observed temperature responses of soil biological processes, and lack of comparability between studies (Alster et al., 2020). Macromolecular Rate Theory (MMRT) has been recently proposed as a more realistic model of enzyme temperature sensitivity based on thermodynamics and the change in heat capacity associated with enzyme catalysis, which accounts for declines in enzyme activity below thermal denaturation temperatures (Hobbs et al., 2013). MMRT can thus appropriately capture the unimodal behavior of enzyme response to temperature, and describes temperature sensitivity as comprising three fundamental components: temperature optimum (Topt), the temperature at which reaction rates are maximal; point of maximum temperature sensitivity (TSmax), the temperature at which reaction rates change the most; and change in heat capacity (ΔCp‡), which describes the degree of curvature of the parabolic response of reaction rates to temperature (Alster et al., 2020). A limited number of studies have applied MMRT to soil biological activities, including exoenzymes in soils and cultures of soil microbes, where it could describe temperature responses more coherently than Arrhenius models and provide more realistic interpretations of temperature sensitivity (Schipper et al., 2014; Alster et al., 2016a,b, 2018; Robinson et al., 2017). However, to our knowledge, MMRT has never been used to investigate the temperature sensitivity of exoenzymes over the whole soil profile.
Different soil models have been developed to represent exoenzyme kinetics, thermodynamics, ecological stoichiometry, enzyme diffusion, and interactions with environmental factors (German et al., 2012; Sinsabaugh and Shah, 2012; Sulman et al., 2014; Wieder et al., 2014; Tang and Riley, 2015, 2019; Wang et al., 2015; Wang and Allison, 2019). However, these processes have only recently started to be incorporated into depth-resolved soil biogeochemical models (Dwivedi et al., 2019; Wang et al., 2021), are rarely considered in fully coupled ecosystem scale models (Grant, 2013; Pasut et al., 2021), and are entirely unrepresented in current Earth system models. Moreover, exoenzyme kinetics, when included in depth-resolved models, are represented as a function of microbial biomass, and not as explicit properties that may vary independently due to differences in microbial life strategies or microbe-substrate interactions.
We investigated how kinetic properties and temperature sensitivity of soil exoenzymes vary with soil depth, possibly representing depth-dependent traits associated with microbiomes adapted to distinct soil environments. Given the role of forests as globally critical C reservoirs (Griscom et al., 2017), we investigated exoenzymes in soils from a temperate coniferous forest site, which has been shown to have lost substantial subsoil C in response to experimental warming (Hicks Pries et al., 2017; Soong et al., 2021). The soil profile at this site is also known to reflect typical gradients in decreasing soil C and temperature range (Hicks Pries et al., 2017; Soong et al., 2021). We hypothesized that: (i) enzyme Vmax declines with depth, in concert with declines in substrate concentrations and overall nutrient demand; (ii) enzyme affinities and catalytic efficiencies increase with depth to maximize resource acquisition under low substrate concentrations; (iii) variation of kinetic properties with depth differs between C-, N- and P-acquiring enzymes, reflecting differences in relative substrate availability and demand; (iv) temperature sensitivity of exoenzymes increases with depth, reflecting selection of enzymes adapted to lower and narrower temperature ranges in deeper soils. We determined the MM kinetics and catalytic efficiencies of the hydrolytic enzymes β-glucosidase (BG), leucine aminopeptidase (LAP) and acid phosphatase (AP) (involved in C, N and P acquisition, respectively), as a function of both soil dry mass and microbial biomass C, in soils collected at six depths down to 90 cm. Furthermore, we investigated enzyme temperature sensitivity based on the Arrhenius model and Q10 coefficients, and on the MMRT model over six temperatures between 4–50°C, following a fully factorial experimental design considering substrate type and concentration, soil depth and temperature.
Materials and Methods
Site Description and Sample Collection
Soil samples were collected at the University of California Blodgett Experimental Forest, Sierra Nevada, CA, United States (120°39′40″ W; 38°54′43″ N), described by Hicks Pries et al. (2018). Briefly, Blodgett forest is located in a Mediterranean climate with mean annual precipitation of 1,660 mm and a mean annual air temperature of 12.5°C. The soil was classified as Alfisol of granitic origin, and has a developed O horizon. The site is a mixed coniferous forest with ponderosa pine (Pinus ponderosa), sugar pine (Pinus lambertiana), incense cedar (Calodefrus decurrens), white fir (Abies concolor) and douglas fir (Pseudotsuga menziesii) as dominant tree species. The mean annual soil temperature ranges between 11.5 and 10.4°C at 5 and 100 cm depths, respectively, although soil temperatures vary annually between 0–29°C, 1–19°C and 2–16°C at 5, 30, and 100 cm depth, respectively. Three soil cores were collected in July 2019 using a 4.78 cm diameter soil corer with a 10 kg hand-held slide-hammer. The surface litter layer of the O horizon was removed prior to sampling, and mineral soil samples were recovered sequentially in 10 cm increments down to 90 cm depth. Samples were kept cold during transportation to the laboratory, where they were sieved to 2 mm and stored at 4°C. Samples were analyzed within approximately a week of collection. To ensure the accessibility and discoverability of the samples generated here, and to align with the National Science Foundation’s guidelines of effective data practices, all samples have been registered with IGSN Global Sample Numbers through the System for Earth Sample Registration (SESAR). SESAR is maintained by the GeoInformatics Research Group of the Lamont-Doherty Earth Observatory at https://www.geosamples.org/. Sample IGSNs are shown in Supplementary Table 1.
Exoenzyme Activity Assays
Extracellular hydrolytic enzyme activities were determined fluorometrically according to standard assays (German et al., 2011b) at six depth intervals, following the experimental design in Table 1. Briefly, we used the methylumbelliferone (MUF)-linked substrates MUF-β-D-glucopyranoside and MUF-phosphate for determination of β-glucosidase (BG) and acid phosphatase (AP) activities, respectively. Leucine aminopeptidase (LAP) activity was determined using the substrate L-leucine-7-amido-4methylcoumarin (AMC). Assays were performed for each of six soil depths from each of three replicate soil cores, by combining 200 μL of soil homogenate with 50 μL of fluorogenic substrate solution in each microplate well. Soil homogenates were prepared with 1 g of fresh soil in 100 mL 50 mM acetate buffer with pH 5.5, by mixing with a regular blender. The same buffer was used to prepare all substrate solutions, soil homogenates, serial dilutions of standards in the absence or presence of soil homogenate (quenching controls), blank quenching controls without standards, and blank controls in the absence or presence of each of the eight substrate concentrations. Standards in the presence or absence of soil homogenates (quenched standards) were prepared over six 1:10 serial dilutions, from 0.625 to 20 μM for MUF, and 0.3125 to 10 μM for AMC. MUF and AMC standards without soil homogenates, blank controls with only substrates, and blank quenching controls with soil homogenates, but no MUF or AMC standards, were performed in duplicate. Each enzyme was assayed individually over a range of eight substrate concentrations, as follows: 10, 30, 60, 100, 150, 250, 450, and 800 μM for BG; 10, 20, 40, 70, 110, 190, 350, and 600 μM for LAP; and 10, 40, 80, 130, 200, 350, 700, and 1200 μM for AP (Table 1). Parallel assays for each sample, enzyme and substrate concentration were performed in black microplates individually covered with lids to avoid evaporation, and incubated in the dark at 4, 10, 16, 25, 35, or 50°C. Fluorescence was recorded (excitation: 365 nm, and emission: 450 nm) after approximately 1, 4, and 24 h to determine the optimal incubation time. Four analytical replicates were measured per sample for each combination of enzyme, substrate concentration and temperature. A set of standards, and blank, substrate and quenching controls was incubated together with each batch of assays at each temperature, to correct fluorescence measurements for temperature-specific effects in the assays. Incubation temperatures were selected in order to capture the unimodal response predicted by MMRT with Topt-values well above native temperatures, as observed by previous studies of exoenzymes from temperate environments (Alster et al., 2016b), while including the temperature range and approximate seasonal averages at our experimental site.
Microbial Biomass C and Dissolved C and N Pools
Microbial biomass C (MBC), dissolved organic C (DOC) and total dissolved N (TDN) were determined at every 10 cm depth interval between 0–90 cm depth. MBC was estimated using the chloroform-fumigation extraction method (Brookes et al., 1985). Five-gram soil samples were fumigated in 50 mL closed vials containing a jumbo cotton ball soaked with ethanol-free chloroform over, but not touching, the soil, for 7 days, with chloroform replenished on day 4. Fumigated and a non-fumigated soil samples were extracted with 25 mL of 0.5 M K2SO4 on an orbital shaker table for 60 min, then gravity filtered through pre-leached #42 Whatman filter paper, and frozen until further analysis. DOC and TDN in fumigated and non-fumigated samples were quantified using a Lotix Combustion TOC/TN Analyzer (Teledyne Tekmar, Mason, OH, United States). No correction factor (kEC) was applied to account for incomplete microbial biomass lysis during the fumigation.
Data Analyses
All data manipulations and analyses were performed in R versions 3.6.1-4.0.4 (R Core Team, 2020). All enzyme trait abbreviations and definitions are indicated in Table 2. Net fluorescence in the enzyme assays, including quenching corrections, were calculated following German et al. (2011b). Negative values due to analytical error were excluded from the dataset (0.8%); analytical outliers were further identified based on the Interquartile Range method, and a maximum of one value was excluded out of the four analytical replicates per sample. In total, these procedures excluded 1917 out of 26784 data-points (7.2%). Enzyme maximal velocity (Vmax) and Michaelis constants (Km) were computed by fitting a 2-parameter Michaelis-Menten (MM) model over all analytical replicates of each of eight substrate concentrations using the drm function in the drc package (Ritz et al., 2015), with a data-driven self-starter function specific to the model. Following preliminary analyses, and when necessary, we excluded data points corresponding to one of the eight individual substrate concentrations for which all analytical replicates consistently did not fit the distribution of the remaining data (i.e., due to inhibition at high concentrations or technical errors during assay preparation). In order to alleviate variance heterogeneity of analytical replicates between substrate concentrations, we applied a Box-Cox transformation to all models using the boxcox function in the drc package (Ritz et al., 2015). Comparison between the parameters Vmax and Km estimated based on transformed and non-transformed models showed that Box-Cox transformation improved the fit of models with substantial analytical variance, but had a marginal or no effect on parameters estimated by models with initial good fit. Individual models yielding nonsignificant Vmax or Km estimates (p > 0.05) after Box-Cox transformation were considered to have bad fit and were thus excluded from further analyses (excluded 11 out of 324 models). To determine the optimal assay incubation time at each temperature, we compared MM models fit to data collected after each of three sequential incubation periods (1, 4, and 24 h). We selected the minimum incubation period necessary to reach the highest Vmax-value, under the assumptions that lower Vmax-values reflected either insufficient incubation time for reactions to reach saturation, decrease in activity, or loss of fluorescence due to prolonged incubation after saturation had been reached. The same incubation period was consistently selected for each batch of assays performed at the same temperature. Vmax was expressed per mass of dry soil as Vmax/ds (nmol g–1 h–1) and per unit of microbial biomass C (MBC) as Vmax/MBC (nmol μg MBC–1 h–1). The apparent catalytic efficiency (CEds) was calculated as:
and the biomass-specific catalytic efficiency (CEMBC) as:
Q10 coefficients were calculated over the full experimental temperature range (six temperatures from 4 to 50°C) and over a realistic field range (five temperatures from 4 to 35°C) following the approach by Allison et al. (2018). Briefly, the degree of change in Vmax, Km or CE per °C was inferred based on linear regressions between the natural logarithm of each parameter and temperature, and converted to Q10-values based on the relationship:
The Arrhenius activation energy (Ea) was calculated based on the slope of the linear regression between ln(Vmax) and 1/T, and the relationship:
where T is the temperature in kelvin and R is the universal gas constant. Linear regression models were calculated using the lm function in the stats package native to R (R Core Team, 2020). The change in heat capacity (ΔCp‡), temperature optimum (Topt) and point of maximum temperature sensitivity (TSmax) were calculated by fitting ln(Vmax) over the six incubation temperatures between 4–50°C using the Macromolecular Rate Theory (MMRT) model, according to the equations and definitions described by Alster et al. (2020). The reference temperature T0 was set to 315 K to best match the measured data, following the recommendations by Alster et al. (2020). Model fit comparisons were based on the Akaike Information Criterion (AIC) and respective relative likelihoods, corrected AIC (AICc), and Bayesian Information Criterion (BIC), following the guidelines by Burnham and Anderson (2004). AIC, BIC, and adjusted R2-values of the linear models were extracted from the linear regression model computed with the lm function in the stats package (R Core Team, 2020). AICc of all models, and AIC and BIC of the nonlinear models were calculated using the R package AICcmodavg (Mazerolle, 2020). One-way and two-way Analyses of Variance (ANOVA) were performed with the aov function, followed by post hoc Tukey’s tests using the function TukeyHSD with p-values adjusted for multiple comparisons, using the stats package (R Core Team, 2020). Compact letter displays for the Tukey’s tests were computed with the function HSD.test in the package agricolae (de Mendiburu and Yaseen, 2020). Assumptions of ANOVA were tested based on Levene’s tests with the leveneTest function in the package car (Fox and Weisberg, 2019), Shapiro-Wilk tests with the shapiro.test function in the package stats (R Core Team, 2020), skewness of residuals with the skewness function in the package agricolae (de Mendiburu and Yaseen, 2020), and plots of homogeneity of residuals’ variance and normality of residuals (Q-Q plots). Data was ln-transformed as necessary, and all tests reported as significant were based on a p-value < 0.05. Figure displays were prepared with the package cowplot (Wilke, 2020). The maximum percentage of variation (i.e., decline) in kinetic parameters with depth, per temperature, was calculated as the percentage of difference between the highest and lowest values within the upper and lower depth intervals mentioned in the text, for example:
As the ANOVA showed that variation in kinetic parameters with depth was not dependent on temperature, the percentages of variation with depth are reported as the average decline among all temperatures, per enzyme and kinetic parameter. The inconsistently high mean Km-values only at 16°C was excluded from those calculations. All raw and processed data, as well as the code used to parse and analyze them are available as Supplementary Material (see “Supplementary_Materials_File_Descriptions.pdf”).
Results
Exoenzyme Kinetics Vary With Soil Depth
We determined the MM kinetics of the enzymes acid phosphatase (AP), β-glucosidase (BG), and leucine aminopeptidase (LAP) in soils collected at six depth intervals from triplicate soil cores down to 90 cm (0–10, 10–20, 30–40, 50–60, 60–70, and 80–90 cm), at six temperatures between 4 and 50°C (4, 10, 16, 25, 35, or 50°C) (Table 1). The activity of all enzymes showed typical MM behavior. Enzyme kinetic traits analyzed here and their definitions are indicated in Table 2.
The Vmax of all three enzymes, estimated on a dry soil mass basis (Vmax/ds), declined significantly with soil depth over all temperatures (p < 0.001), and differences among depths were not dependent on temperature (i.e., no significant depth × temperature interaction) (Figure 1A and Table 3). Mean Vmax/ds declined almost continuously from the soil surface (0–20 cm) down to 60–90 cm by up to 96.4 ± 0.4% (mean ± standard error; see Materials and Methods for details) across all enzymes and temperatures. This variation was only significant between three to four depth ranges, which differed between enzymes (Figure 1A, Supplementary Table 2): Vmax/ds of BG declined progressively down to 60 cm, but not below that depth; Vmax/ds of AP declined only over the mid-depth range, from 20 to 30 cm and from 40 to 60 cm; Vmax/ds of LAP also did not vary within the upper 20 cm, but declined gradually down to a lower depth than that of AP, namely from 20 to 30 cm, from 40 to 50 cm and from 60 to 80 cm.
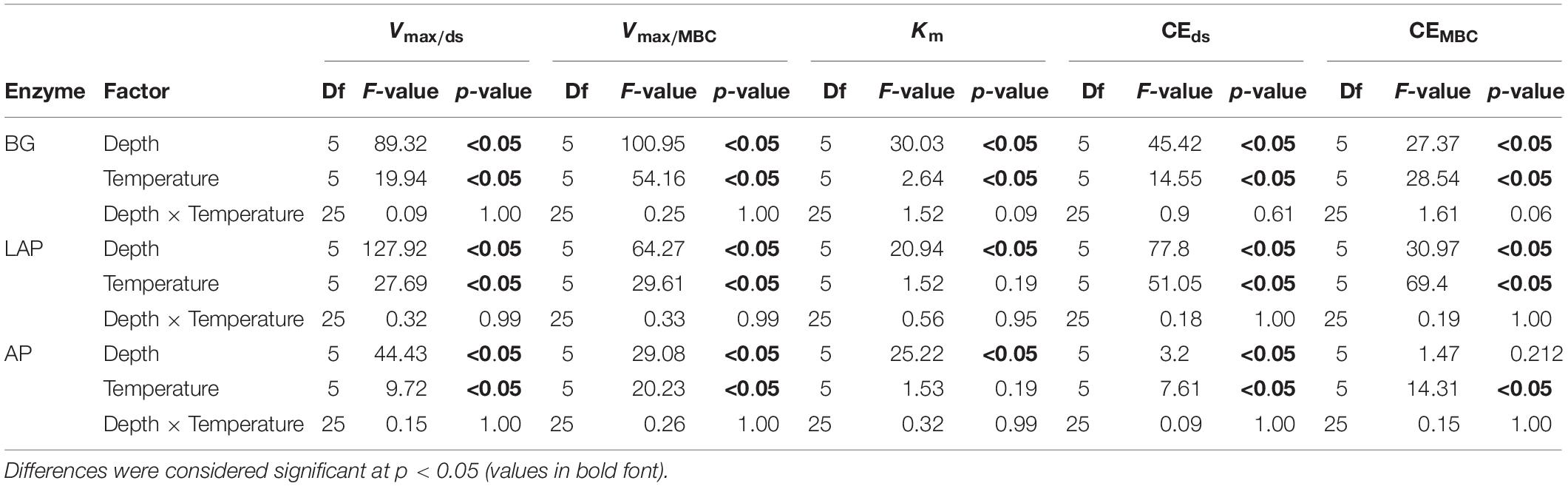
Table 3. Two-way fixed effects ANOVA of kinetic parameters with depth and temperature as independent factors, per enzyme.
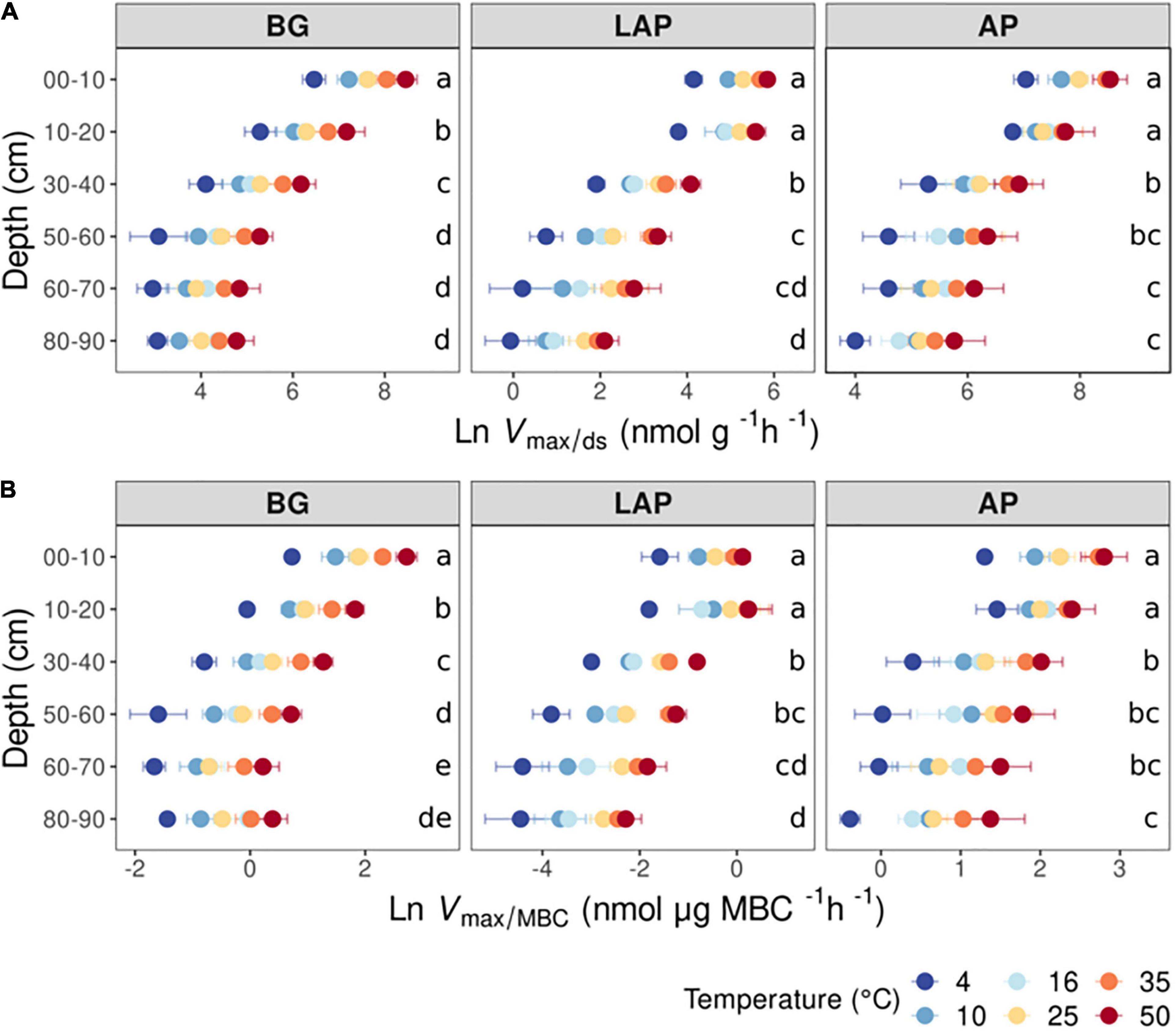
Figure 1. Enzyme maximum velocity (Vmax) at different depths and six temperatures from 4 to 50°C, expressed per (A) dry soil mass (Vmax/ds), or (B) microbial biomass C (Vmax/MBC). Two-way ANOVA with depth and temperature as interactive factors indicated that both depth and temperature had a significant effect on Vmax of all enzymes (p < 0.05), but without interaction between the two factors (Table 3). Colors indicate incubation temperatures and letters indicate significant differences (p < 0.05) between depths per enzyme, based on Tukey’s tests after ANOVA tests. Error bars represent the standard error of the mean (n = 3).
Since the concentration of microbial biomass carbon (MBC) declined strongly with soil depth, especially over the upper 30 cm (Supplementary Figure 1), much of the decline in Vmax/ds with depth may have been driven by lower microbial abundance. Therefore, we computed a biomass-specific Vmax, by expressing it per unit MBC (Vmax/MBC), under the assumption that cell lysis efficiency by chloroform fumigation was similar across samples, thus yielding comparable MBC estimates. Vmax/MBC of all enzymes declined significantly down the soil profile over all temperatures, following the same trends as those of Vmax/ds (p < 0.001) (Table 3, Supplementary Table 2, and Figure 1B). The overall decline in Vmax/MBC between 10–20 and 60–90 cm was only 8% lower (88.4 ± 1.7%) than that of Vmax/ds, indicating that variation in Vmax/ds did not depend primarily on microbial biomass concentration. Like Vmax/ds, Vmax/MBC did not show a significant interaction between depth and temperature. Also similar to Vmax/ds, Vmax/MBC of AP and LAP did not vary within the upper 20 cm and declined mostly from 20 to 30 cm (Figure 1B). Vmax/MBC did not decline significantly over the mid-depth range for either AP or LAP, but it was significantly lower at 80–90 cm than at 30–40 cm for AP, and lower between 60 and 90 cm than at 30–40 cm for LAP. Vmax/MBC of BG declined more consistently down to 70 cm over all temperatures, but did not vary further.
Km also declined (i.e., enzyme affinity increased) significantly with depth for all enzymes across temperatures (p < 0.001) with no significant interaction between depth and temperature (Table 3, Supplementary Table 2). However, Km declined less with depth than Vmax/ds or Vmax/MBC, and mainly between the upper 20 cm and lower depths, by up to 85.6 ± 1.3%, with some differences between enzymes. Km of AP and BG declined with depth following trends similar to those of their Vmax (Figure 2): Km of AP declined mainly from 20 to 30 cm and remained relatively constant down to 80 cm, although it was significantly lower at 80–90 cm than at 30–40 cm; Km of BG declined consistently down to 40 cm, without further variation (despite a spuriously high mean Km at 80–90 cm only at 16°C). Unlike its Vmax, the Km of LAP only declined from 20 to 30 cm, and did not vary significantly below that depth.
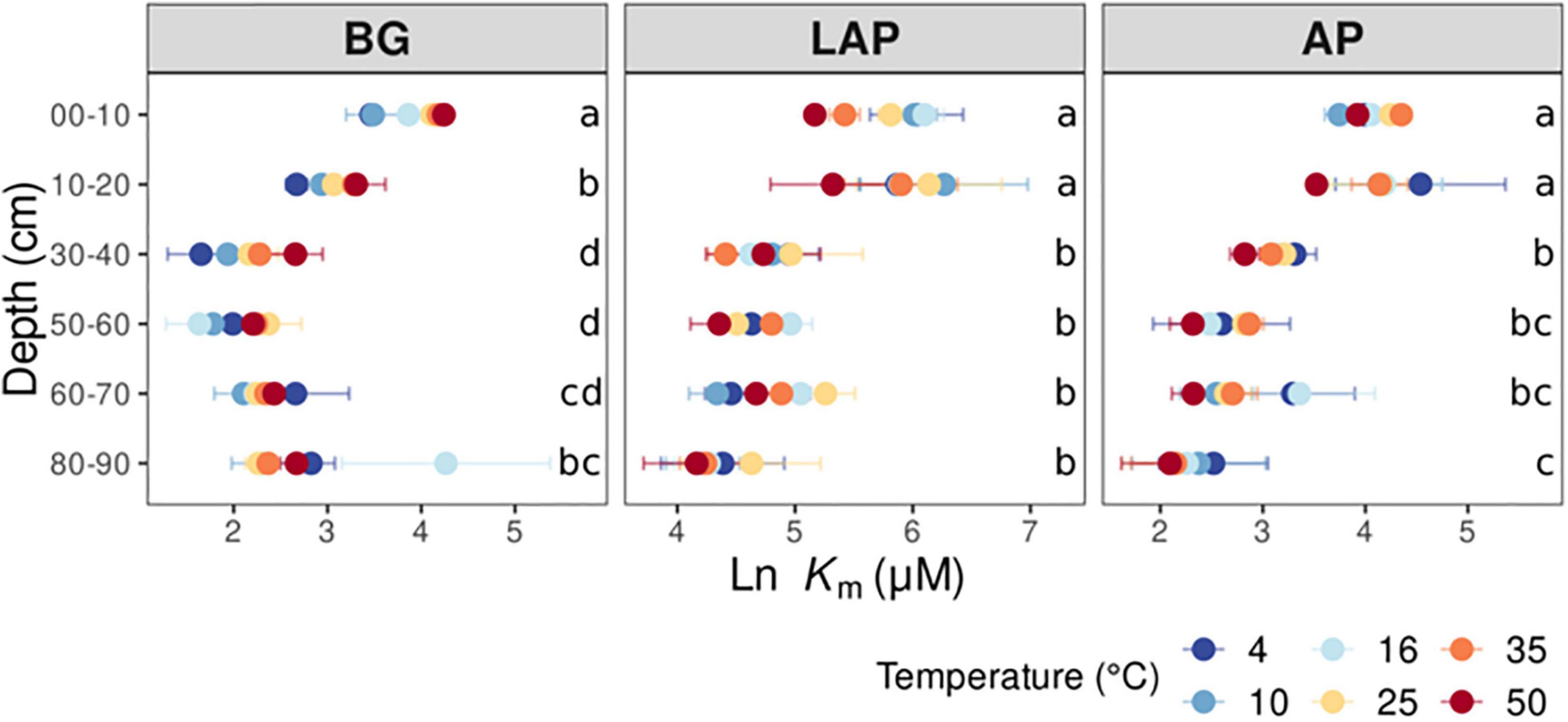
Figure 2. Enzyme Michaelis constant (Km) at different depths and six temperatures from 4 to 50°C. Two-way ANOVA with depth and temperature as interactive factors indicated that depth had a significant effect on Km of all enzymes (p < 0.05), whereas temperature only had a significant effect on Km of BG (Table 3). There was no depth × temperature effect. Colors indicate incubation temperatures and letters indicate significant differences (p < 0.05) between depths per enzyme, based on Tukey’s tests after ANOVA tests. Error bars represent the standard error of the mean (n = 3).
The apparent (i.e., observed) catalytic efficiency (CEds), estimated as the ratio between Vmax/ds and Km, reflects the catalytic efficiency of the enzyme pool present per mass of soil, regardless of microbial abundance or enzyme demand. CEds declined significantly across temperatures for all enzymes (p < 0.001), also without a significant interaction between depth and temperature (Table 3, Supplementary Table 2). The CEds of BG and LAP followed similar trends and declined mainly over the mid-depth range by up to 90.5 ± 1.1% over all temperatures, without significant variation in the upper 20 cm, or below 60 cm (Figure 3A). CEds of AP declined much less through the soil profile (58.1 ± 3.0%), and it was only significantly lower at 60–90 cm than in the upper 10 cm. Biomass-specific catalytic efficiency (CEMBC), estimated as the ratio between Vmax/MBC and Km, represents the inherent catalytic efficiency of the enzyme pool produced by the local microbiome, as a function of its specific enzyme production capacity and demand. CEMBC declined significantly with depth for BG and LAP (p < 0.001) across temperatures, but not for AP (Table 3, Supplementary Table 2, and Figure 3B). CEMBC of BG and LAP varied less with depth than other kinetic properties, and declined significant mainly below 60 cm by up to 71.8 ± 2.5% (Figure 3B). These declines in CEMBC reflected the decline in Vmax/MBC at lower depths, where Km remained relatively constant. Like for all other kinetic parameters, depth-dependent differences in CEMBC were not dependent on temperature (Table 3).
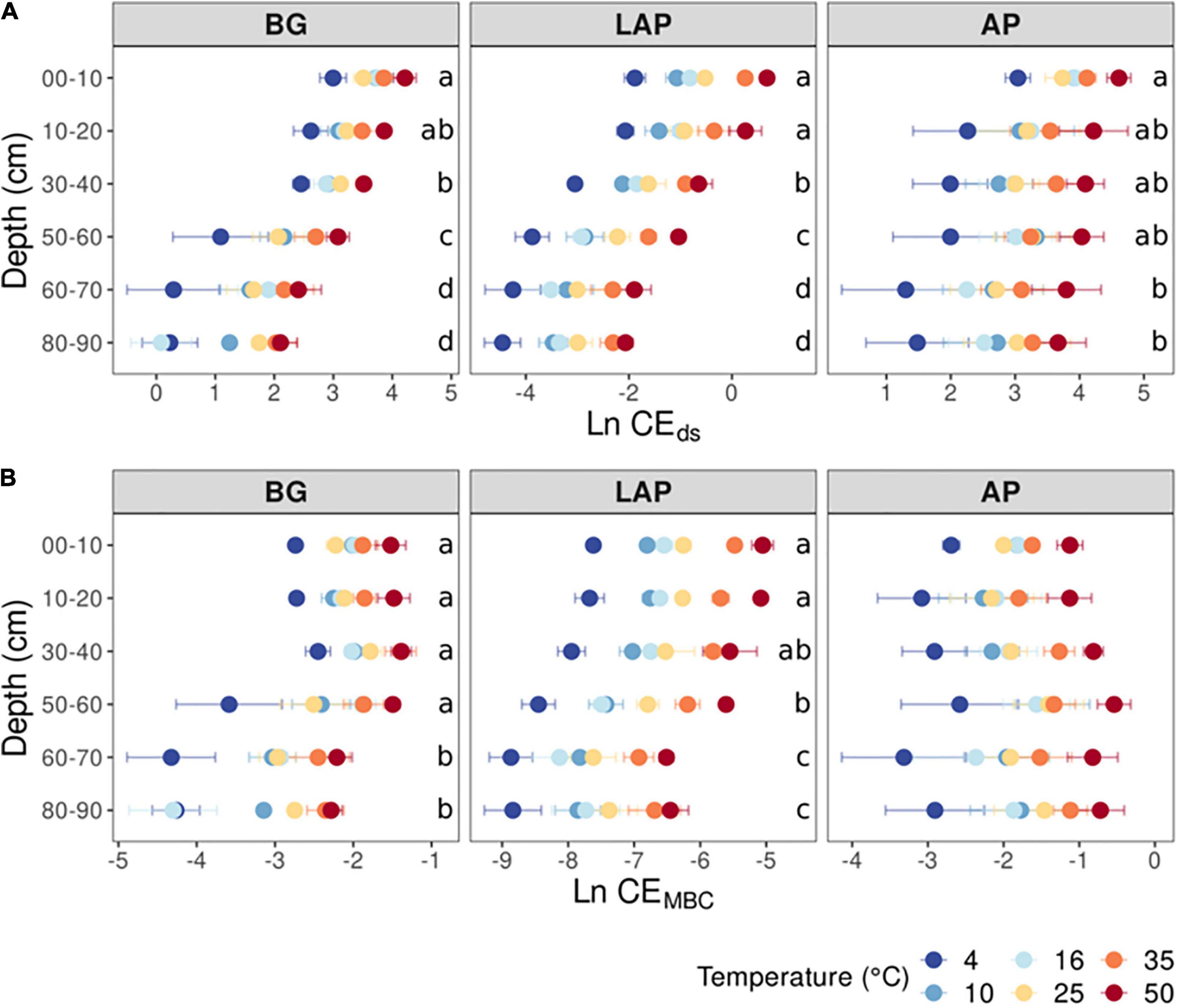
Figure 3. Enzyme catalytic efficiency at different depths and six temperatures from 4 to 50°C, calculated on a (A) dry soil mass basis (CEds), or a (B) microbial biomass C basis (CEMBC). Two-way ANOVA with depth and temperature as interactive factors indicated that both depth and temperature had a significant effect on CEds and CEMBC of all enzymes (p < 0.05), except for no effect of depth on CEMBC of AP (Table 3). There was no depth × temperature effect. Colors indicate incubation temperatures and letters indicate significant differences (p < 0.05) between depths per enzyme, based on Tukey’s tests after ANOVA tests. Error bars represent the standard error of the mean (n = 3).
As there was no significant interaction between depth and temperature for any kinetic parameter (Table 3), we performed a random effects ANOVA with depth as independent variable and temperature as block variable, to control for possible confounding effects of the latter on depth-dependent differences. This analysis yielded the same results as the fixed effects ANOVA reported above, with the single exception that Vmax/ds of AP declined significantly also between 50–60 cm and 80–90 cm (data not shown).
Exoenzyme Kinetics Vary Among Enzymes as a Function of Soil Depth
All kinetic properties varied significantly among enzymes at all depths and across temperatures (p < 0.001), but there was only a significant interaction between enzyme and temperature in the upper 10 cm for Km, CEds and CEMBC (p < 0.001) (Supplementary Table 3). Vmax differed significantly among all enzymes at all depths, with AP having consistently the highest values, followed by BG and then LAP (p < 0.05) (Supplementary Table 4 and Figures 1A,B). Km differed significantly among all enzymes between 10 and 60 cm (p < 0.05), but it did not differ between AP and BG in the upper 10 cm or below 60 cm (Supplementary Table 4 and Figure 2). LAP had always the highest Km (i.e., lowest affinity). In contrast, BG had always the lowest Km, at least at depths where it was significantly different than that of AP (i.e., between 10 and 60 cm). CEMBC differed significantly among all enzymes in the upper 10 cm and below 50 cm (p < 0.05), but not between AP and BG from 10 to 40 cm (Supplementary Table 4 and Figure 3B). Similar to Vmax, the CEMBC of LAP was always the lowest among enzymes, followed by those of BG and then AP at depths where it differed significantly (Supplementary Table 4 and Figure 3B). As CEMBC was calculated based on the same MBC value for all enzymes at each depth, CEds varied between enzymes similarly to CEMBC (Supplementary Table 4 and Figure 3A).
We investigated the ratios between kinetic properties (Vmax, Km, and CE) of the three different enzymes as indicators for variation in relative nutrient demand through the soil profile. Based on two-way fixed effects ANOVA with depth and temperature as independent factors, all kinetic ratios between enzymes varied significantly with depth over all temperatures, with the exception of ratios between Km of LAP and AP (KmLAP:AP) (p < 0.005) (Supplementary Table 5). The effects of temperature on kinetic ratios are described in a separate section below. VmaxBG:LAP declined significantly from 10 to 20 cm, followed by a suggestive continuous increase down to 90 cm, although it was only significantly higher at 80–90 cm than at 10–20 cm (Figure 4A). VmaxBG:AP declined with depth down to 70 cm, mainly from 10 to 20 cm and from 40 to 60 cm, followed by a significant increase from 70 to 90 cm that appeared to result partially from a spurious high value only at 16°C, among all six temperatures (Figure 4B). VmaxLAP:AP generally declined with depth below 20 cm, but this variation was mainly significant between the upper 20 cm and the lower 30 cm (i.e., from 60 to 90 cm) (Figure 4C). KmBG:LAP and KmBG:AP followed trends similar to those of their corresponding Vmax ratios: KmBG:LAP declined significantly from 10 to 20 cm and remained relatively invariant through the profile (Figure 4D), whereas KmBG:AP declined from 10 to 20 cm, followed by a suggestive but non-significant increase down to 70 cm (Figure 4E). The significant increases in KmBG:LAP and KmBG:AP at 80–90 cm were likely driven, at least partially, by the same spurious high Km-value of BG only at 16°C mentioned above. In contrast, KmLAP:AP did not vary significantly with depth nor show any apparent trends (Figure 4F). CEBG:LAP increased significantly from the upper 20 cm to a depth of 30–40 cm, although below that depth it did not vary significantly from any upper depths (Figure 4G). CEBG:AP did not vary within the upper 40 cm, but it was significantly lower below 50 cm (Figure 4H). CELAP:AP declined with depth below 20 cm, but this variation was only significant between the upper 40 cm and the lower 30 cm (from 60 to 90 cm) (Figure 4I). This variation in CELAP:AP with depth mirrored the general trend of VmaxLAP:AP, as KmLAP:AP was relatively invariant though the soil profile.
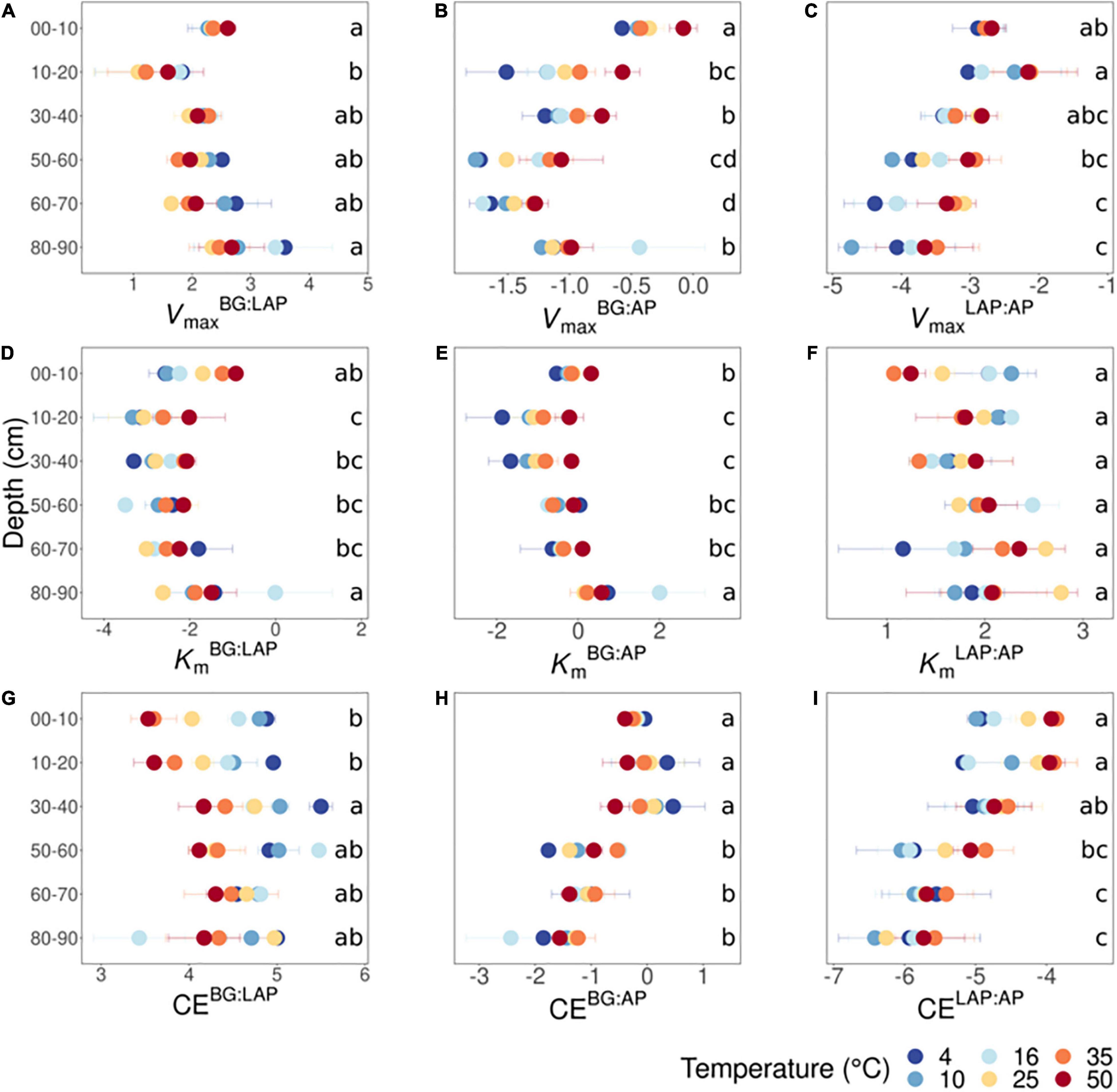
Figure 4. Ratios between kinetic parameters of BG, LAP and AP at different depths and six temperatures from 4 to 50°C. (A) VmaxBG:LAP, (B) VmaxBG:AP, (C) VmaxLAP:AP, (D) KmBG:LAP, (E) KmBG:AP, (F) KmLAP:AP, (G) CEBG:LAP, (H) CEBG:AP, and (I) CELAP:AP. Two-way ANOVA with depth and temperature as interactive factors indicated that depth had a significant effect on all ratios (p < 0.05), except on KmLAP:AP, whereas temperature had a significant effect only on VmaxBG:AP, VmaxLAP:AP and CEBG:LAP (Supplementary Table 5). There was a depth × temperature effect only on CEBG:LAP. Colors indicate incubation temperatures and letters indicate significant differences (p < 0.05) between depths per ratio, based on Tukey’s tests after ANOVA tests. Error bars represent the standard error of the mean (n = 3); dots without error bars represent data-points with n < 3 (data excluded due to Michaelis-Menten models with poor fit).
Temperature Sensitivity of Exoenzymes Is Similar Through the Soil Profile
Temperature had an overall significant effect on Vmax, CEMBC and CEds of all enzymes, and on Km of only BG, over the whole soil profile (p < 0.001) (Table 3 and Figures 1–3). The temperature sensitivity of enzyme Vmax (Vmax/MBC and Vmax/ds scale identically with temperature at each depth) was further determined using a linear Arrhenius model and Q10 coefficient over the full temperature range tested (4–50°C, n = 6) (Supplementary Figure 2) and a realistic in situ soil range (4–35°C, n = 5) (Figure 5), as well as based on the temperature optimum (Topt), point of maximum temperature sensitivity (TSmax) and change in heat capacity (ΔCp‡) estimated using the non-linear MMRT model over the full temperature range (4–50°C, n = 6) (Figure 6). Enzyme thermal traits analyzed here and their definitions are indicated in Table 2. Although the fits of Arrhenius and MMRT models did not differ substantially, overall comparisons consistently favored MMRT, suggesting that it provided a more realistic representation of enzyme temperature response (see extended Results and Discussion in Supplementary Information).
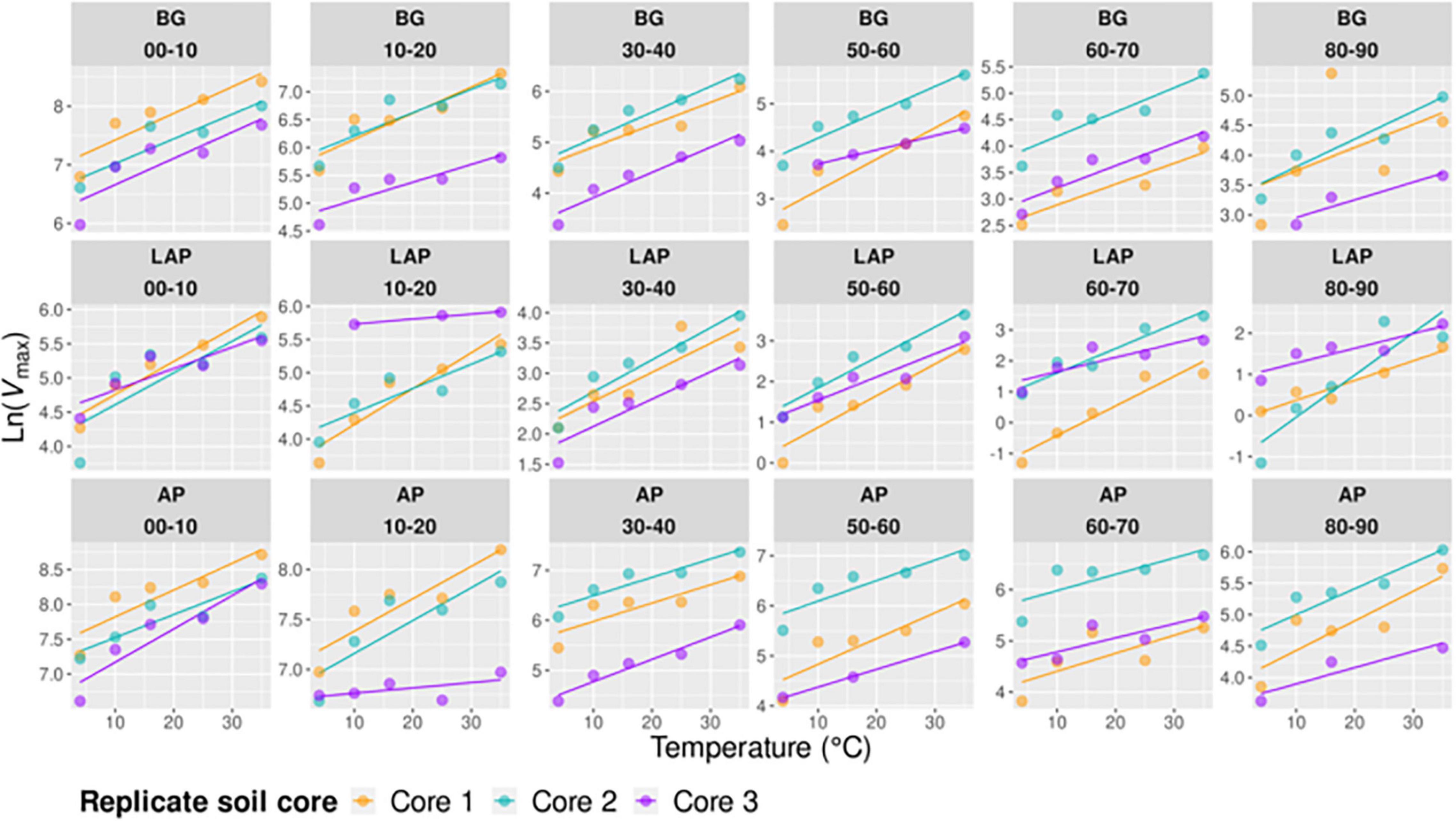
Figure 5. Arrhenius models of Vmax over five temperatures from 4 to 35°C per enzyme, depth, and replicate core sample.
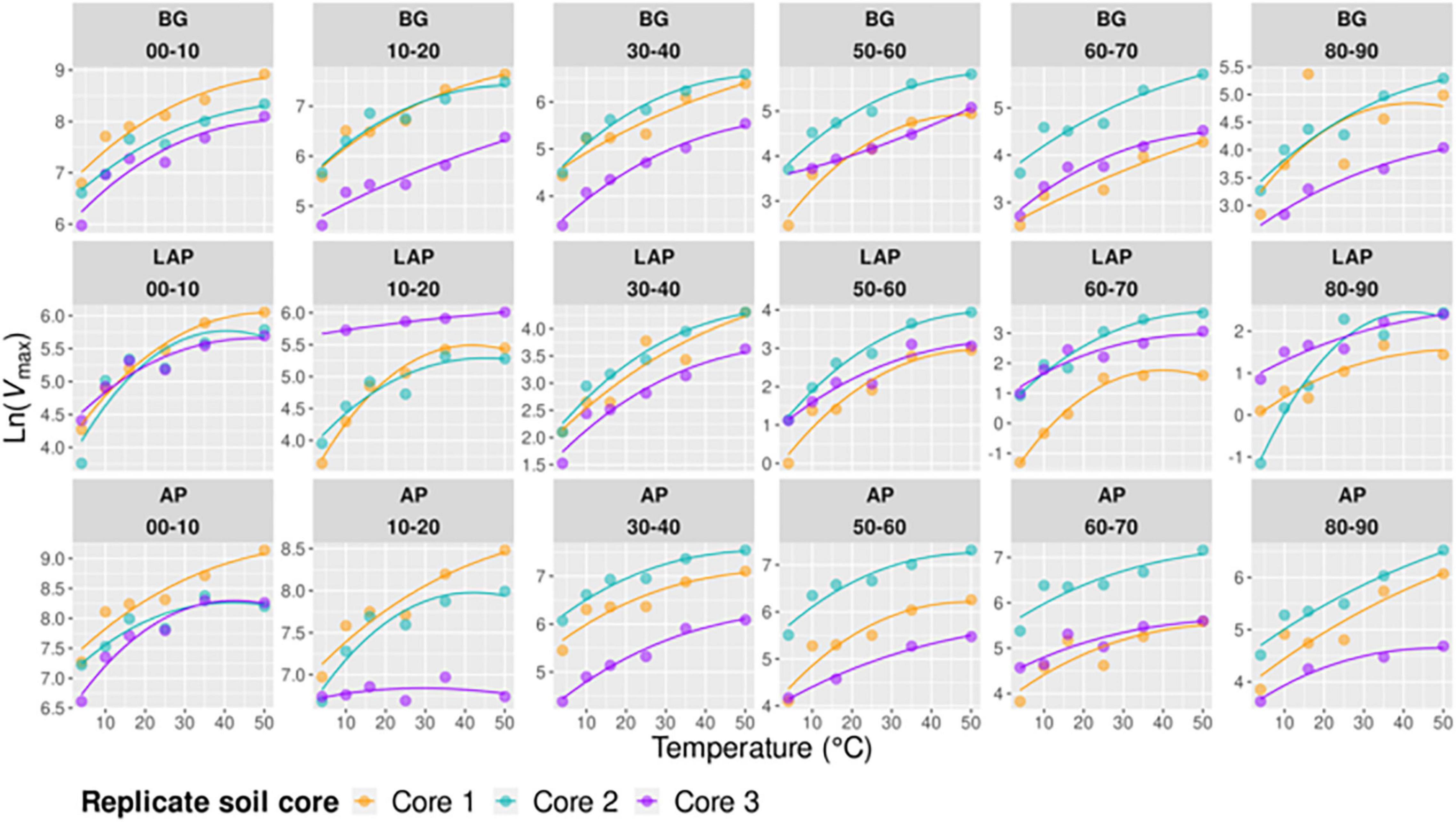
Figure 6. Macromolecular Rate Theory (MMRT) models of Vmax over six temperatures from 4 to 50°C per enzyme, depth, and replicate core sample.
Despite some suggestive, depth-dependent trends in the Q10-values of Vmax, Km, and CE, they did not vary significantly with depth for any enzyme (Table 4), whether estimated over a realistic in situ soil temperature range (4–35°C) (Figure 7), or the full experimental temperature range (4–50°C) (Supplementary Figure 3). Both temperature ranges yielded similar Q10-values that varied within a narrow range, although Q10-values over 4–50°C were slightly lower than those over 4–35°C due to a frequent decline in the response rate of Vmax between 35 and 50°C (Supplementary Table 8). Therefore, only Q10-values over the realistic in situ soil temperature range are henceforth presented. The Q10 of Vmax was consistently above 1, indicating a positive effect of temperature on Vmax across depths of 1.44 ± 0.03, 1.56 ± 0.03, and 1.78 ± 0.10 (mean ± se) for AP, BG and LAP, respectively (Figure 7A and Supplementary Table 8). Mean activation energies (Ea) estimated from the same linear relationships were 25.69 ± 1.74, 31.50 ± 1.45, and 39.33 ± 3.98 kJ mol–1 K–1 (mean ± se) across depths for AP, BG, and LAP respectively (Supplementary Table 8). The Q10 of Km varied between 0.83 and 1.30 across enzymes and depths, although Km was, on average, relatively insensitive to temperature compared to other parameters, with mean Q10-values across depths of 1.00 ± 0.06, 1.14 ± 0.05, and 0.99 ± 0.07 (mean ± se) for AP, BG and LAP, respectively (Figure 7B and Supplementary Table 8). This was consistent with the two-way ANOVA showing that temperature had generally no significant effect on Km. The significant effect of temperature on the Km of BG detected by the two-way ANOVA was likely due to the spurious high Km of BG only at 16°C at 80–90 cm (Table 3 and Figure 2), which was not reflected on the Q10 computed across temperatures. The Q10 of CE was consistently above 1, similar to that of Vmax, with similar overall mean values across enzymes: 1.51 ± 0.08, 1.42 ± 0.08, and 1.82 ± 0.05 (mean ± se) for AP, BG, and LAP respectively (Figure 7C and Supplementary Table 8).
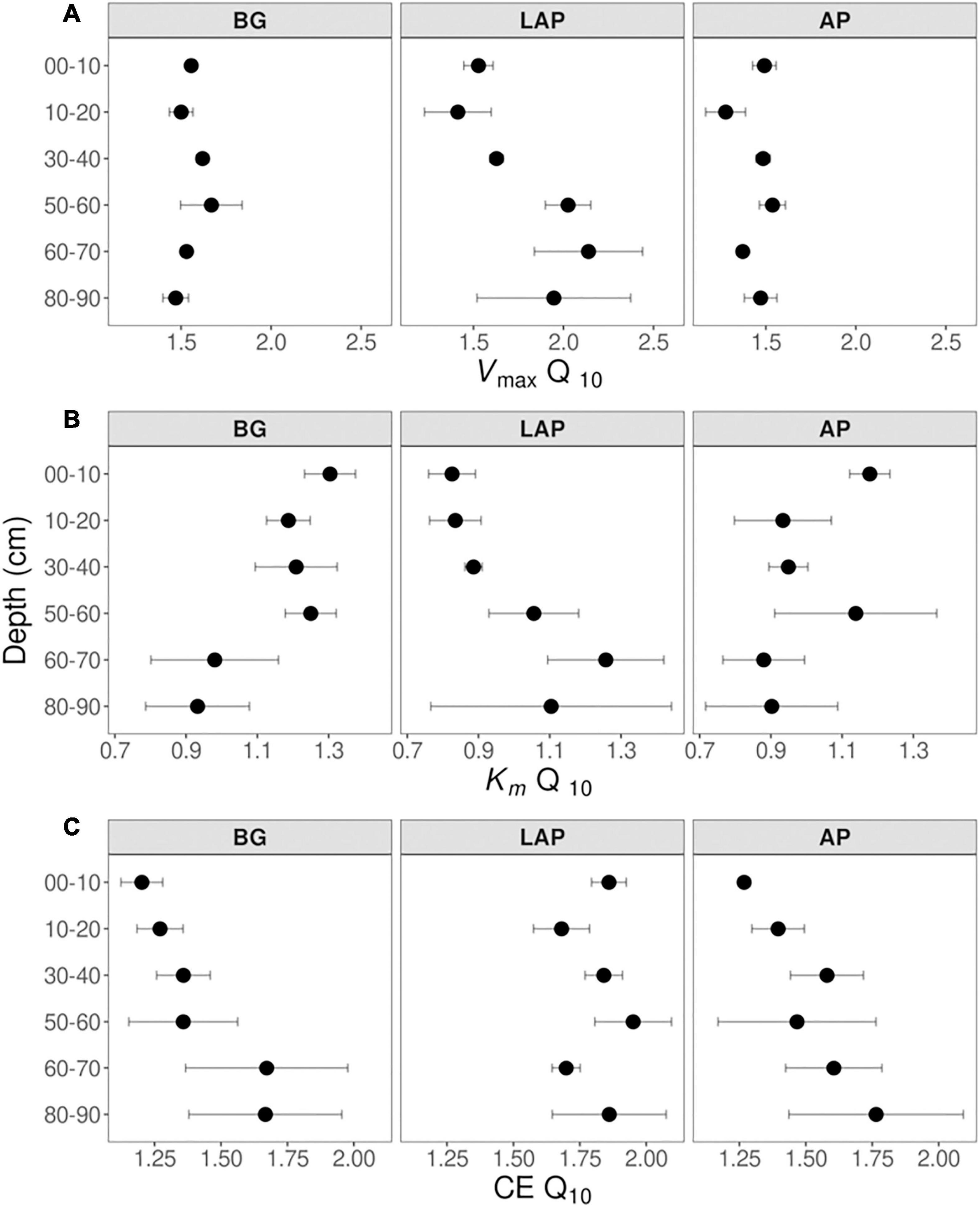
Figure 7. Q10 of Michaelis-Menten kinetic parameters over five temperatures from 4 to 35°C at different depths. (A) Vmax, (B) Km, and (C) CE. Differences between depths are not significant (p > 0.05), based on one-way ANOVA tests per enzyme (Table 4). Error bars represent the standard error of the mean (n = 3).
None of the temperature sensitivity parameters estimated by MMRT –Topt, TSmax and ΔCp‡– varied significantly with depth (with one exception; see below) (Figure 8 and Table 4). These parameter estimates showed considerable variability among replicates, and estimates from models with poor fit to MMRT’s predicted behavior (Topt or TSmax < 0°C, or > 200°C; four out of 54 total models) were excluded from the analysis, likely reducing the statistical power of few pairwise comparisons between depths and enzymes. Mean Topt and TSmax were consistent across depths and enzymes, with mean values of 65.19 ± 3.74°C and 31.63 ± 1.98°C (mean ± se), respectively (Figures 8A,B and Supplementary Table 8). TSmax of LAP was significantly different between the 0–10 and 30–40 cm depth intervals (p < 0.05), which was the only exception to otherwise non-significantly different parameter estimates across either enzymes or depths. Mean ΔCp‡-values were similar between AP and BG across depths, with a combined mean value of −0.79 ± 0.06 kJ mol–1 K–1 (mean ± se) (Figure 8C and Supplementary Table 8). ΔCp‡ of LAP spanned a broader range of values (−1.32 ± 0.21, mean ± se), mainly due to suggestive, albeit non-significant, lower values at depths below 60 cm.
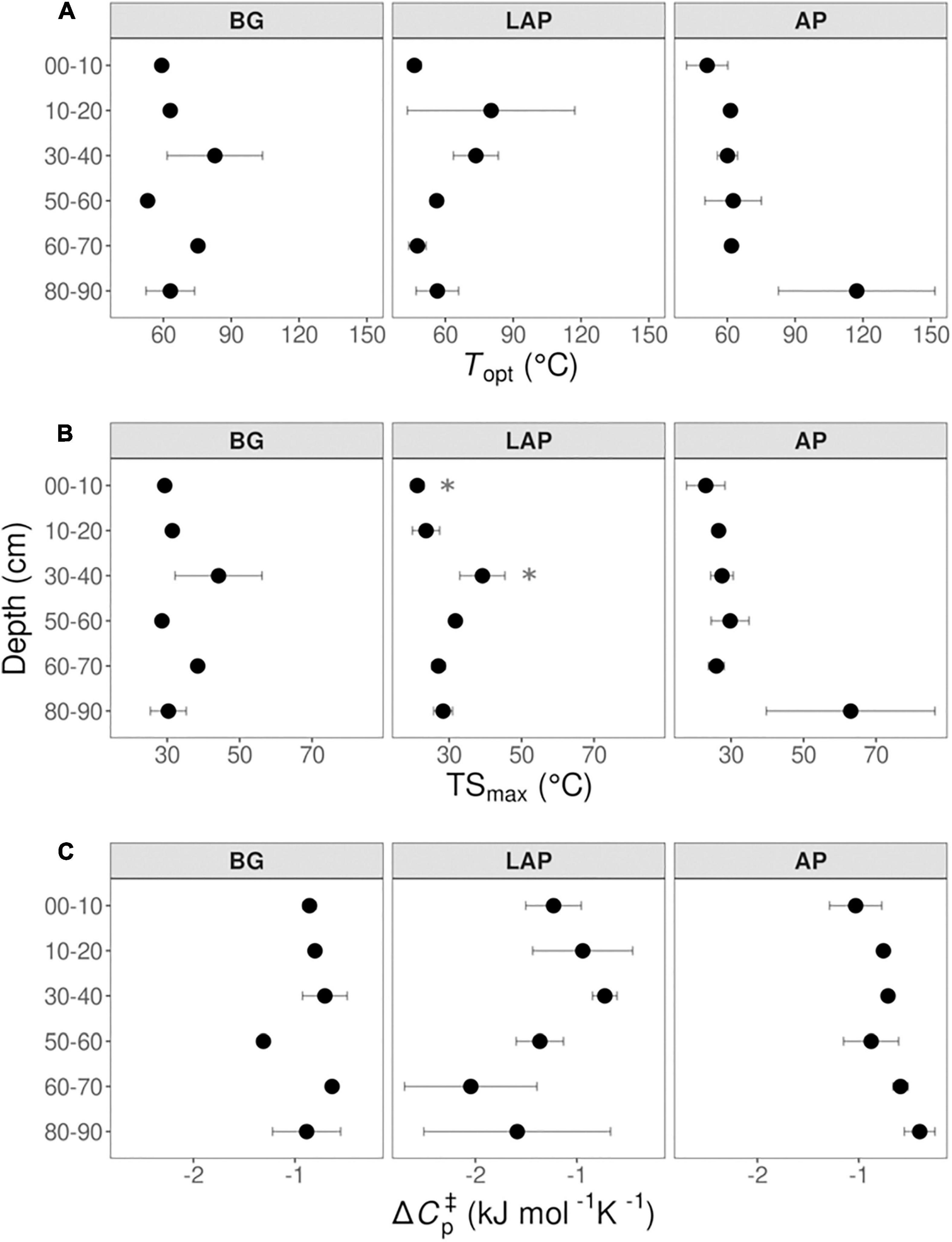
Figure 8. Macromolecular Rate Theory (MMRT) model estimates over six temperatures from 4 to 50°C at different depths. (A) Topt, (B) TSmax, and (C) ΔCp‡. Differences between depths are not significant (p > 0.05), based on one-way ANOVA tests per enzyme (Table 4), except for TSmax of LAP between the depths indicated by asterisks (*). Error bars represent the standard error of the mean (n = 3).
Temperature Sensitivity of Some Kinetic Properties Varies Between Exoenzymes but Only at Discrete Depths
Temperature had a significant, positive effect on the Vmax ratio of BG to AP (VmaxBG:AP), and LAP to AP (VmaxLAP:AP) across depths, and a significant, negative effect on ratios between catalytic efficiencies of BG and LAP (CEBG:LAP) (p < 0.05) (Supplementary Table 5 and Figures 4A–I). This indicated that Vmax and CE of those enzymes were differently affected by temperature. CEBG:LAP was also subject to a depth × temperature interaction (p < 0.05), suggesting that the different effects of temperature on CE of BG and LAP were depth-dependent. The Q10 of Vmax and CE, and MMRT’s ΔCp‡ varied significantly between enzymes across the soil profile (p < 0.05), but there was no enzyme × depth interaction in the two-way ANOVA (Table 5). One-way ANOVA between enzymes at each depth interval showed that Q10-values varied significantly only between some enzymes and at discrete depths (Supplementary Tables 9–10). While the Q10 of Vmax was only significantly different between AP and LAP at 60–70 cm (p < 0.05), this analysis also showed that the Q10 of Km was significantly lower for LAP in the upper 10 cm relative to the other two enzymes (p < 0.05). This difference suggested that the affinity of LAP might increase with temperature (i.e., lower Km) at this depth (Q10 = 0.83 ± 0.07, mean ± se), compared to those of AP (Q10 = 1.18 ± 0.06) or BG (Q10 = 1.30 ± 0.07). The Q10 of CE was significantly higher for LAP in the upper 10 cm (1.86 ± 0.07, mean ± se) relative to those of AP (1.27 ± 0.02) or BG (1.20 ± 0.08) (p < 0.05), reflecting the apparent negative effect of higher temperatures on the Km of LAP at that depth. Although overall ΔCp‡-values varied significantly between enzymes across depths (i.e., two-way ANOVA) (Table 5), one-way ANOVA did not detect significant differences at any specific depth for any MMRT parameter (Supplementary Table 9).

Table 5. Two-way fixed effects ANOVA of temperature sensitivity estimates with enzyme type and depth as independent factors.
Discussion
We show that kinetic properties of the enzymes BG, LAP, and AP varied markedly through the soil profile at a temperate forest, even when accounting for the large variation in microbial biomass. Moreover, this variation in enzyme kinetics was independent from temperature, as kinetic properties varied similarly between soil depths over temperatures between 4 and 50°C. We also show that the temperature sensitivity of each enzyme is similar through the soil profile, based on both linear Arrhenius and non-linear MMRT models, although temperature can directly affect the relative kinetics between enzyme types at discrete depths. To our knowledge, this is the first study to investigate the MM kinetic properties of soil enzymes and their direct temperature sensitivity through the soil profile, in this case to 90 cm.
Higher Exoenzyme Affinity, but Lower Vmax and Catalytic Efficiency, Indicate Adaptation to Lower Substrate Availability and Distinct Microbial Life Strategies in Deeper Soils
As hypothesized, Vmax/ds and Km declined strongly with soil depth, but followed distinct trends that depended on enzyme type. The decline in Vmax/ds of 96.4 ± 0.4% across enzymes and temperatures down to 90 cm indicated a drastic decline in enzyme production capacity, product demand, or substrate availability. This was consistent with the lower microbial biomass concentrations in deeper soils observed here, as generally reported across studies (Blume et al., 2002; Fierer et al., 2003a; Schnecker et al., 2014; Loeppmann et al., 2016a; Jones et al., 2018). Higher density of plant roots in surface soils may have also contributed to higher near-surface Vmax/ds, as shown particularly for BG in rooted soils when compared to fallow soils (Loeppmann et al., 2016b), and in rhizosphere hotspots when compared to bulk soil (Tian et al., 2020). Plants may induce higher enzyme Vmax by promoting microbial growth through C-rich exudates, competing with microbes for N and P, or directly stimulating microbial enzyme production to enhance availability of assimilable products (Burns et al., 2013). Declines in activity of hydrolytic enzymes have been consistently observed down to depths between 50 and 420 cm in diverse soils, including from temperate, taiga and tropical forests, arctic tundra, grasslands and croplands (Taylor et al., 2002; Venkatesan and Senthurpandian, 2006; Gelsomino and Azzellino, 2011; Kramer et al., 2013; Schnecker et al., 2014, 2015; Stone et al., 2014; Loeppmann et al., 2016a; Jing et al., 2017; Darby et al., 2020; Dove et al., 2020). However, among the five studies from which we could retrieve at least approximate Vmax/ds-values, only Loeppmann et al. (2016a) observed mean declines in Vmax/ds of BG, LAP and AP down to 70 cm similar to those observed here down to 90 cm (91.0 ± 2.2%), whereas others observed substantially smaller mean declines of approximately 67.5 ± 6.3% for AP and BG at depths between 55 and 110 cm (Venkatesan and Senthurpandian, 2006; Gelsomino and Azzellino, 2011; Stone et al., 2014). Consistent with this, only Loeppmann et al. (2016a) determined Vmax based on a MM model over a series of enzyme substrate concentrations, as needed to estimate MM kinetics accurately. Potential enzyme activities have been frequently shown to correlate positively with MBC concentration (Perucci, 1992; Gelsomino and Azzellino, 2011; Stone et al., 2014; Ren et al., 2018), as Vmax is linearly dependent on enzyme concentration, which in turn is largely dependent on microbial abundance. However, this is not always the case (Waring et al., 2014), as exoenzyme production may also be induced or repressed depending on substrate availability and product demand, following the evolutionary-economic mechanisms that regulate allocation of cellular resources (Allison et al., 2011; German et al., 2011a). Moreover, enzyme production varies between organisms and is subject to variable levels of regulation (Allison et al., 2011; Sinsabaugh and Shah, 2012; Burns et al., 2013). For example, some isozymes and enzyme types may be expressed at stable constitutional levels under specific conditions, as previously suggested for deep soils (Stone et al., 2014), whereas expression of others may be more strictly induced by cellular demands or environmental cues. Therefore, a biomass-specific Vmax (Vmax/MBC) can be interpreted as a catalytic rate constant independent of microbial abundance, which represents the collective effect of inherent enzyme properties, and specific enzyme production and demand of the microbiome. Surprisingly, Vmax/MBC of all enzymes declined nearly as much with depth as Vmax/ds, indicating that microbial abundance was not the primary driver of variation in Vmax. Loeppmann et al. (2016a) found similar trends only for BG and LAP, and only below 30–40 cm, as Vmax/MBC increased from the surface to that depth and only then declined consistently down to 70 cm. These differences might have resulted from a steeper decline in substrate availability and microbial biomass in our soils, which are covered by a thick litter layer and have a shallow rhizosphere, compared to a more extensive rhizosphere (i.e., maize) and less surface litter in the soils studied by Loeppmann et al. (2016a). The consistent decline in Vmax/MBC in deeper soils observed by us, and to some extent also Loeppmann et al. (2016a), contrasts with most other studies where Vmax/MBC of AP, BG and LAP either increased, or did not vary with depth (Gelsomino and Azzellino, 2011; Kramer et al., 2013; Stone et al., 2014; Schnecker et al., 2015; Dove et al., 2020). We could only identify one study that detected a decline in Vmax/MBC of BG (Taylor et al., 2002), although declines with depth have been more frequently observed for other enzymes (Taylor et al., 2002; Gelsomino and Azzellino, 2011; Schnecker et al., 2015). However, Vmax in those studies was inferred from a single concentration of substrate across depths that was often below the saturation point necessary to reach the Vmax-values we observed, particularly in surface soils. We suggest that the apparent increase, or lack of variation, in Vmax/MBC previously observed may have resulted from underestimating Vmax in surface soils. Our results indicate that exoenzyme pools in deeper soils have inherently low potential reaction rates due to lower expression levels, lower substrate turnover, and/or catalytic efficiency than those in surface soils, possibly reflecting differences in microbial life strategies and substrate preferences.
The consistent increase in affinity (i.e., decrease in Km) of all enzymes with depth by 85.6 ± 1.3%, mainly between 20 and 60 cm, indicated a major decline in substrate availability at mid-depths consistent with the decline in DOC, TDN (Supplementary Figures 4A,B) and total C and N in these soils (Hicks Pries et al., 2017, 2018). This supported our hypothesis that persistently low substrate concentrations in deep soils select for microbes encoding isozymes with lower Km in order to maintain relatively constant maximal catalytic rates (Sinsabaugh et al., 2014). Km-values largely above physiologic substrate concentrations would render enzyme activity entirely dependent on substrate availability, which, under deep soil conditions, would lead to suboptimal rates and provide limited return to the investment on enzymes. Conversely, higher substrate availability in surface soils through plant litter inputs likely alleviates the selective pressure on enzymes with high affinity. Inputs of readily assimilable compounds through root exudation may further alleviate this pressure by reducing the relative importance of continuously maintaining maximal depolymerization rates (Allison et al., 2011), similar to what has been observed for substrate induced respiration (Blagodatskaya et al., 2009). The variation in enzyme affinities with depth observed here indeed appeared to reflect the overall decline in root density –both fine and coarse roots– below 40 cm at this site (Hicks Pries et al., 2018). The Km of BG particularly mirrored the continuous steep decline in fine root density down to 40 cm (Hicks Pries et al., 2018), whereas those of AP and LAP did not vary within the upper 20 cm. This suggests that availability of easily metabolizable C-containing compounds exuded by fine roots may have a particular regulatory effect on depolymerization of cellulose (Allison and Vitousek, 2005; Allison et al., 2011) through selection of microbes encoding BG isozymes with distinct affinities. This hypothesis is further supported by the higher affinities of cellulose-degradation enzymes, including BG, observed in fallow soils relative to rooted soils (Loeppmann et al., 2016b), and in bulk soils relative to rhizosphere hotspots (Tian et al., 2020). Moreover, microbes producing BG in the rhizosphere have been shown to be distinct from those in the detritusphere (Nuccio et al., 2020). It should be noted that, like in all other studies of enzyme kinetics or activity in environmental samples based on these assays, both Vmax and Km estimates may be affected by the native substrate concentrations (Sinsabaugh et al., 2014). At the same time, it seems implausible that such effects might have contributed substantially to the extreme declines in Vmax and Km with depth observed, given their interdependency and the necessary requirement for low Km under the much lower substrate concentrations in deeper soils.
The catalytic efficiency (CE), also referred to as specificity constant (Gelsomino and Azzellino, 2011), can be determined as kcat/Km when Km exceeds the concentration of substrate present, which is typically the case under physiological conditions (Berg et al., 2002; Koshland, 2002). Therefore, CE not only represents a fundamental functional property under direct selective pressure, but also evolutionary tradeoffs between kcat and affinity, which are themselves subject to selection (Sinsabaugh et al., 2014). However, kcat expresses the maximum amount of substrate converted per unit of time, per enzyme unit (assuming a single catalytic center per enzyme), and thus it cannot be directly inferred from enzyme assays in complex environmental samples, such as soils, where specific enzyme concentrations are generally unknown and hard to quantify. In these cases, an apparent CE (CEds) has been estimated as Vmax/ds/Km (Moscatelli et al., 2012; Kujur and Kumar Patel, 2014; Triebwasser-Freese et al., 2015; Loeppmann et al., 2016a,b; Razavi et al., 2016), which represents the observed CE of the enzyme pool present per mass of soil, regardless of the specific production capacity and demand of the microbiome. In our soils, CEds of all enzymes declined significantly with depth, with CEds of BG and LAP declining consistently over the mid-depth (20 to 60 cm) by up to ∼90%, whereas that of AP varied much less. These results are consistent with those from the only other study that, to our knowledge, determined CEds in deep soils, where CEds of these same enzymes declined by 2- to 20-fold between the upper 40 cm and depths down to 70 cm (Loeppmann et al., 2016a). Tian et al. (2020) have also shown that CEds of BG and AP was higher in fertile soils than in nutrient-poor soils, consistent with higher CEds in surface soils with greater nutrient availability than deep soils. However, contrary to our initial expectations, CE based on biomass-specific Vmax (CEMBC) either declined by up to ∼70% (BG and LAP) or did not vary (AP) with depth. We initially hypothesized that CE would increase with depth to maximize return on the investment in enzymes, given the scarce substrates provided by lower plant litter inputs and lower compensation by root exudates. Microbial communities adapted to these conditions would be expected to encode isozymes with higher affinity (i.e., lower Km) and/or produce more exoenzymes per unit biomass (i.e., higher Vmax/MBC) in a proportion that favors higher Vmax/MBC/Km ratios (i.e., CEMBC). As the Km-values of BG and LAP were relatively invariable between 30 and 90 cm, their lower CEMBC at depths below 60 cm was mainly driven by a decline in Vmax/MBC, suggesting that it was constrained by lower production of enzymes per unit biomass below that depth rather than higher Km. On the other hand, enzymes may optimize kcat in adaptation to environmental pressures (e.g., temperature) at the expense of Km, leading to conformational adaptations that reduce active site binding, which result in higher Km and suboptimal catalytic efficiencies (Struvay and Feller, 2012). Therefore, a lower CEMBC in deeper soils driven by lower Vmax/MBC may also reflect enzymes with lower kcat, as a result of trade-offs with Km, rather than lower enzyme production. The contrasting lack of variation in CEMBC of AP with depth, regardless of individual variation in Vmax/MBC and Km, might have resulted from different factors and interactions related to variation in relative P availability and demand, and in regulation of AP expression. Alternatively, P may be primarily acquired from minerals rather than organic compounds (Alori et al., 2017), and thus the CEMBC of AP alone does not directly reflect P demand. It should also be noted that variation in enzyme and substrate diffusion through the soil matrix, or their stabilization through mineral-organic interactions, can lead to differences in substrate availability and enzyme accessibility. For example, lower diffusivity or higher adsorption of enzymes or substrates to soil particles could select for enzymes with higher affinities and prompt higher enzyme production per cell. Conversely, stabilization of active enzymes can increase their longevity and effectiveness over time (Burns et al., 2013), which could alleviate the selective pressure on their catalytic efficiency, as we observed in deeper soils.
Our results suggest that microbial communities in deep subsoils encode exoenzymes with intrinsically lower kcat, and/or produce less enzymes per cell than those in surface soils, leading to a lower emergent CEMBC. The expectation that Vmax/MBC and CEMBC would increase with depth assumes that microbiomes have largely redundant metabolic and elemental demands, and thus that exoenzymes are optimized to provide nutrients in proportion to the size and demands of the community, as a function of nutrient availability (Allison et al., 2011). However, several studies have shown that microbiomes change markedly with soil depth (Fierer et al., 2003b; Hansel et al., 2008; Hartmann et al., 2009; Eilers et al., 2012; Jiao et al., 2018; Liu et al., 2019), with deep soils harboring less diverse and functionally distinct organisms (Brewer et al., 2019; Diamond et al., 2019; Yan et al., 2019; Dove et al., 2021). Life strategies that prioritize cellular maintenance over fast growth and maximal resource exploitation, and properties such as utilization of alternative substrates, storage compound production, and ability to sporulate or undergo dormancy may all affect exoenzyme properties, and possibly contribute to relax selective pressures on their kinetics (Ho et al., 2017; Ramin and Allison, 2019). Dove et al. (2021) have shown that deep soil microbes at our site have lower growth rates and lower carbon use efficiency than those at the surface, reflecting a lower nutrient demand and greater investment on cellular maintenance that may underlie the lower Vmax/MBC and CEMBC we observed. Moreover, the declining substrate availability with depth is expected to decrease the return on exoenzyme investment and favor alternative metabolic strategies that do not rely primarily on depolymerization of complex organic matter. Dove et al. (2021) have indeed shown that microbiomes in these deep soils have lower potential to degrade complex carbohydrates, similar to those in other soils (Diamond et al., 2019). Conversely, it has been shown that deep soil communities are enriched in organisms that can metabolize one-carbon (C1) and other low-molecular weight C compounds, and use inorganic N forms as energy sources (Brewer et al., 2019; Diamond et al., 2019), as well as in taxa that comprise mainly chemoautotrophs (Cao et al., 2012; Eilers et al., 2012; Turner et al., 2017; Brewer et al., 2019; Diamond et al., 2019). Therefore, a smaller fraction of organisms relying on exoenzymes in deep soils is also likely to contribute to a lower emergent CEMBC due to both lower overall biomass-specific enzyme production and lower competition for enzyme products within the community. Nevertheless, it should be noted that, while microbial biomass largely dominates living matter in mineral and usually dry soils with low root density, such as these, we cannot entirely exclude minor contributions of enzymes produced by plants and soil fauna to the activities measured.
Relative Kinetics Between Exoenzymes Vary With Depth, Suggesting Variation in Nutrient Demands
As expected, Vmax differed significantly between all three enzymes at every depth, reflecting fundamental differences in relative nutrient demand, as well as possible differences in enzyme properties and regulation. According to ecoenzymatic stoichiometry, ratios between Vmax of hydrolytic enzymes involved in acquisition of C, N or P reflect the relative demand in these elements in relation to their availability, and thus the equilibrium between microbial biomass and SOM stoichiometry (Sinsabaugh and Shah, 2012). Here, both Vmax and CE ratios between C- and N-acquiring enzymes (VmaxBG:LAP and CEBG:LAP, respectively) were relatively constant through the soil profile, despite suggestive increases at lower depths, which were consistent with the trend in soil C:N ratio (Hicks Pries et al., 2018) and ratios between dissolved C and N pools (Supplementary Figure 4C). At the same time, Vmax and CE ratios between BG:AP and LAP:AP decreased with depth, suggesting an increasing demand for P relative to either C or N, similar to previous observations of ratios between activities of C- and P-acquiring enzymes in both temperate and tropical soils (Stone et al., 2014; Loeppmann et al., 2016a). Alternatively, it has been shown that soil microbes can use phosphorylated compounds primarily as a C source (Heuck et al., 2015), and thus higher Vmax and CE of AP may rather indicate higher C demand in the absence of more favorable C sources in deep soils, as previous suggested (Stone et al., 2014). It should be noted, however, that depolymerization of organic matter and nutrient acquisition involves also other enzymes, and therefore the enzyme investigated here may not fully represent C, N or P demand and availability (Sinsabaugh and Shah, 2012).
Exoenzyme Temperature Sensitivity Is Similar Through the Soil Profile Across Enzyme Types
Our results confirmed the general expectation that activity of BG, LAP and AP is stimulated by increasing temperatures up to optimal temperatures above those typically observed in moderate environments, with Q10-values for Vmax and activation energies within the ranges typically observed for soil exoenzymes (Trasar-Cepeda et al., 2007; Brzostek and Finzi, 2012; German et al., 2012; Stone et al., 2012; Steinweg et al., 2013; Razavi et al., 2015, 2016; Nottingham et al., 2016). However, despite few suggestive trends, temperature sensitivity did not vary significantly with depth for any enzyme or kinetic property, based on either Q10, following a linear Arrhenius model, or Topt, TSmax and ΔCp‡ of Vmax estimated by the non-linear MMRT model. We could not determine unambiguously whether the response of Vmax to temperature was best explained by Arrhenius or MMRT models, although comparisons suggested that the latter generally fit the data better. Moreover, positive temperature response rates of Vmax declined at higher temperatures in most cases (i.e., above TSmax = 31.63 ± 1.98°C, up to Topt = 65.19 ± 3.74°C, followed by a negative response), as observed by other studies (Alster et al., 2016a,2018), further indicating that MMRT represented a more realistic temperature response behavior. On the other hand, this also indicated that the linear models captured the temperature response better under lower temperatures within the native temperature range, before response rates slowed towards Topt, similar to what has been observed by Alster et al. (2016a). The uniform temperature sensitivity observed here contradicted our initial hypothesis that exoenzymes in deeper soils are more sensitive to temperature changes as a result of microbial adaptation to lower and narrower temperature ranges (Schipper et al., 2014), as observed at our site. Previous studies have observed that exoenzymes from colder soil environments tend to be more sensitive to temperature (Koch et al., 2007; Brzostek and Finzi, 2012), as well as in some subsoils in relation to surface soils (Steinweg et al., 2013). Therefore, we expected that Q10-values and activation energies would increase with depth, whereas either Topt, ΔCp‡, or both, would decrease. Under MMRT’s Optimum-Driven hypothesis, the more frequent lower temperatures in deeper soils could select for enzymes with lower Topt, regardless of their ΔCp‡ (Alster et al., 2020). Conversely, the Thermal Breadth hypothesis postulates that enzymes subject to large temperature ranges have less negative ΔCp‡ (i.e., flatter temperature response curves) but not necessarily different Topt, and thus the narrower temperature ranges in deeper soils would lead to more negative ΔCp‡ (Alster et al., 2020). In turn, the Enzyme Rigidity hypothesis predicts that cold-adapted enzymes have more negative ΔCp‡ due to their lower rigidity, which would lead to a decline in ΔCp‡, and consequently Topt, with depth, following selection of enzymes adapted to lower temperatures (Alster et al., 2020). The uniform temperature sensitivity of all enzymes through the soil profile may instead reflect a convergence of enzyme Topt towards the similar MATs across depths at our site (10.4–11.5°C), despite different temperature ranges. On the other hand, the low MATs of our soils appear to contradict the relatively high ΔCp‡ observed, compared to values previously reported (Alster et al., 2016a,2018), which are expected to reflect a high enzyme rigidity typical of warm-adapted enzymes. It is possible that enzyme ΔCp‡ in our soils are mainly driven by their wide temperature ranges, despite their narrowing with depth, leading to selection of enzymes with less negative ΔCp‡, and thus able to maintain more constant activity rates under varying temperatures (Alster et al., 2020). Moreover, reactions potentially involving a diverse isozyme pool, such as those measured here, reflect the summation of the temperature response curves of those enzymes, and thus are also expected to have a less negative ΔCp‡ (Alster et al., 2018).
Our results are consistent with the uniform temperature sensitivity (apparent Q10) of in situ soil respiration over the top meter of soil previously observed at this site (Hicks Pries et al., 2017). This suggests that SOM depolymerization by exoenzymes may be closely linked to the response of total soil respiration to temperature, likely by modulating the contribution of microbial heterotrophic metabolism. In turn, Km was relatively insensitive to temperature, with mean Q10-values around 1 across depths and enzymes. This suggests that enzyme affinities may be biochemically constrained to prevent being affected by temperature fluctuations, as previously suggested (Allison et al., 2018). Topt of Vmax (65.19 ± 3.74°C), based on the MMRT model, was much higher than natural soil temperatures, whereas TSmax (31.63 ± 1.98°C) was just above the temperature maximum in surface soils (29°C at 5 cm), but substantially higher than those at lower depths (19°C at 30 cm and 16°C at 100 cm), or mean annual temperatures (MAT) over the upper meter of soil (10.4–11.5°C). Similar high Topt estimates based on MMRT have been generally observed for microbial exoenzyme activities and complex metabolic processes in soils (Schipper et al., 2014), and for soil bacterial isolates (Alster et al., 2016a). This is consistent with the fact that the thermal stability and optimal catalytic temperature of enzymes from mesophilic organisms tend to be higher than that of their native environment (Engqvist, 2018). While persistent warming is expected to generally induce higher enzyme activity through the whole soil profile, a uniform TSmax may, however, result in variable net annual temperature responses at different depths due to their different temperature ranges, duration of different temperature regimes, and seasonal variation, regardless of similar MATs. How these factors may interact in response to sustained long-term warming, and their outcomes, will depend on the degree of thermal adaptability of exoenzymes through changes in microbial community composition, and expression of isozymes with different properties (Wallenstein et al., 2011; Bradford, 2013). It should be noted, however, that we cannot completely rule out the possibility that the spatial variability of some temperature sensitivity estimates might have precluded detection of robust differences between depths. Such variability is a common limitation of measurements of emergent responses of complex biological systems, especially due to sample-specific variables that cannot be accounted for with the methods currently available. These challenges emphasize the need for novel approaches that allow a more reproducible assessment of such processes across spatiotemporal scales.
Different Exoenzymes Have Overall Similar Temperature Sensitivities but Temperature Can Affect Their Relative Kinetics at Discrete Depths
The magnitude of all temperature sensitivity parameters was remarkably similar between enzymes through the soil profile, although Q10-values of Vmax and Km have been frequently shown to vary between co-occurring soil enzymes (Wallenstein et al., 2011). Likewise, Topt, TSmax and ΔCp‡ can vary substantially between enzymes (Alster et al., 2016a). Nevertheless, we did observe a significantly lower Q10 of Km and consequently significantly higher Q10 of CE of LAP in the upper 10 cm, relative to the other enzymes. The fact that the mean Q10 of Km of LAP was below 1 (Q10 = 0.83), while those of BG and AP were not (Q10 = 1.30 and 1.18, respectively), suggested that the affinity of LAP was positively stimulated by higher temperatures at this depth (i.e., Km decreased), or that those of BG and AP were negatively affected. As the Q10 of Vmax did not differ significantly between any enzyme, this led to a significantly higher positive temperature response of the CE of LAP. This likely contributed to the significant negative effect of higher temperatures on the CEBG:LAP ratio, and shows that temperature can directly affect the relative catalytic efficiencies between C- and N-acquiring enzymes. This was consistent with previous observations suggesting that kinetic responses to temperature may vary among enzyme types, leading to changes in relative cycling of different nutrients (Allison et al., 2018). Moreover, the significant interaction between depth and temperature on CEBG:LAP ratios confirmed that their variation with depth was dependent on temperature, possibly reflecting the higher CE Q10 of LAP in the upper 10 cm. Higher temperatures also had a significant positive effect on VmaxBG:AP and VmaxLAP:AP ratios, indicating that AP was generally less stimulated by higher temperature than BG or LAP. As enzyme assays at different temperatures were performed with the same soil preparations per depth and incubated over short periods, these relative differences in Vmax likely reflected a direct effect of temperature on enzyme kcat, independently of enzyme concentration. Despite the uniform temperature sensitivity of all kinetic parameters of individual enzymes through the soil profile, these results show that temperature can affects differently the intrinsic kinetic properties (i.e., Km and kcat) of distinct enzymes in a depth-dependent manner, presumably without active microbial regulation.
Conclusion
Kinetic and thermal properties of exoenzymes are fundamental components of complex trait spaces that allow microbes to thrive under variable nutrient availability and temperature regimes, as well as other interacting selective pressures (Allison et al., 2011; Sinsabaugh and Shah, 2012; Sinsabaugh et al., 2014; Ho et al., 2017; Ramin and Allison, 2019; Malik et al., 2020). Our results indicate a strong variation in exoenzyme kinetics through the soil profile. We propose that this may reflect variation in substrate availability, differences in exoenzyme production, and/or expression of distinct isozymes. These possibilities, however, require further investigation. Moreover, we show that the temperature sensitivity of specific kinetic properties is remarkably similar through the soil profile and between enzymes, although it can, at least in some cases, differ between enzymes at discrete depths. This suggested that temperature may directly affect relative substrate depolymerization and nutrient acquisition potential, effectively decoupling enzyme relative activities from other regulatory factors, such as nutrient demand and substrate availability. Although microbial trait spaces are not static, as microbiomes adapt to changing conditions, they are likely to constrain both immediate responses and the trajectory of longer-term responses to environmental changes (Conant et al., 2011; Bradford, 2013; Xu et al., 2021). Therefore, it is essential to identify and validate key microbial traits and their environmental constraints in order to build a mechanistic understanding that can be generalized across spatiotemporal scales, and combine theory, measurements, and models to improve the representation of microbial processes in Earth system models (Wieder et al., 2015; Blankinship et al., 2018). Together, our results improve the mechanistic understanding of microbial processes driving SOM dynamics as a function of soil depth and temperature, and provide new directions towards improved representation of key microbial traits in depth-resolved biogeochemical models.
Data Availability Statement
The original contributions presented in the study are included in the article/Supplementary Material, further inquiries can be directed to the corresponding author/s.
Author Contributions
RA, MM, and EB conceived and designed the study. RA, IC, GM, and EB collected soil samples. RA, IC, HS, and BW performed soil chemical analyses and enzyme assays. RA analyzed and interpreted the data with support from IC, GM, MM, and EB. RA wrote the manuscript with input from MM, MT, and EB. All authors read and reviewed the manuscript.
Funding
This work was performed at Lawrence Berkeley National Laboratory and supported by the U.S. Department of Energy Office of Science, Office of Biological and Environmental Research under Contract No. DE-AC02-05CH11231 to LBNL as part of the Belowground Biogeochemistry Science Focus Area, through the Terrestrial Ecosystem Science Program. IC was supported by a fellowship from the National GEM Consortium. HS was supported by the University of California, Berkeley, CA, Sponsored Projects for Undergraduate Research Program. BW was supported by the California Alliance for Minority Participation program sponsored by the National Science Foundation.
Conflict of Interest
The authors declare that the research was conducted in the absence of any commercial or financial relationships that could be construed as a potential conflict of interest.
Publisher’s Note
All claims expressed in this article are solely those of the authors and do not necessarily represent those of their affiliated organizations, or those of the publisher, the editors and the reviewers. Any product that may be evaluated in this article, or claim that may be made by its manufacturer, is not guaranteed or endorsed by the publisher.
Acknowledgments
We are grateful to Xiaoqin Wu and Romy Chakraborty (Lawrence Berkeley National Laboratory) for technical support with DOC and TDN measurements. We also thank William Riley, Jinyun Tang, and the whole Belowground Biogeochemistry Scientific Focus Area team at Lawrence Berkeley National Laboratory for helpful discussions and suggestions.
Supplementary Material
The Supplementary Material for this article can be found online at: https://www.frontiersin.org/articles/10.3389/fmicb.2021.735282/full#supplementary-material
References
Al-Kaisi, M. M., Lal, R., Olson, K. R., and Lowery, B. (2017). “Fundamentals and functions of soil environment,” in Soil Health and Intensification of Agroecosytems, eds M. M. Al-Kaisi and B. Lowery (Amsterdam: Elsevier), 1–23. doi: 10.1016/B978-0-12-805317-1.00001-4
Allison, S. D., Romero-Olivares, A. L., Lu, Y., Taylor, J. W., and Treseder, K. K. (2018). Temperature sensitivities of extracellular enzyme Vmax and Km across thermal environments. Glob. Chang. Biol. 24, 2884–2897. doi: 10.1111/gcb.14045
Allison, S. D., and Treseder, K. K. (2008). Warming and drying suppress microbial activity and carbon cycling in boreal forest soils. Glob. Chang. Biol. 14, 2898–2909. doi: 10.1111/j.1365-2486.2008.01716.x
Allison, S. D., and Vitousek, P. M. (2005). Responses of extracellular enzymes to simple and complex nutrient inputs. Soil Biol. Biochem. 37, 937–944. doi: 10.1016/j.soilbio.2004.09.014
Allison, S. D., Weintraub, M. N., Gartner, T. B., and Waldrop, M. P. (2011). “Evolutionary-economic principles as regulators of soil enzyme production and ecosystem function,” in Soil Enzymology, eds G. Shukla and A. Varma (Berlin: Springer Berlin Heidelberg), 229–243. doi: 10.1007/978-3-642-14225-3_12
Alori, E. T., Glick, B. R., and Babalola, O. O. (2017). Microbial phosphorus solubilization and its potential for use in sustainable agriculture. Front. Microbiol. 8:971. doi: 10.3389/fmicb.2017.00971
Alster, C. J., Baas, P., Wallenstein, M. D., Johnson, N. G., and von Fischer, J. C. (2016a). Temperature sensitivity as a microbial trait using parameters from macromolecular rate theory. Front. Microbiol. 7:1821. doi: 10.3389/fmicb.2016.01821
Alster, C. J., Koyama, A., Johnson, N. G., Wallenstein, M. D., and von Fischer, J. C. (2016b). Temperature sensitivity of soil microbial communities: an application of macromolecular rate theory to microbial respiration. J. Geophys. Res. Biogeosci. 121, 1420–1433. doi: 10.1002/2016JG003343
Alster, C. J., von Fischer, J. C., Allison, S. D., and Treseder, K. K. (2020). Embracing a new paradigm for temperature sensitivity of soil microbes. Glob. Chang. Biol. 26, 3221–3229. doi: 10.1111/gcb.15053
Alster, C. J., Weller, Z. D., and von Fischer, J. C. (2018). A meta-analysis of temperature sensitivity as a microbial trait. Glob. Chang. Biol. 24, 4211–4224. doi: 10.1111/gcb.14342
Baker, N. R., and Allison, S. D. (2017). Extracellular enzyme kinetics and thermodynamics along a climate gradient in southern California. Soil Biol. Biochem. 114, 82–92. doi: 10.1016/j.soilbio.2017.07.005
Balesdent, J., Basile-Doelsch, I., Chadoeuf, J., Cornu, S., Derrien, D., Fekiacova, Z., et al. (2018). Atmosphere–soil carbon transfer as a function of soil depth. Nature 559, 599–602. doi: 10.1038/s41586-018-0328-3
Berg, J. M., Tymoczko, J. L., and Stryer, L. (2002). Biochemistry, 5th Edn. New York, NY: W.H. Freeman.
Blagodatskaya, E., Blagodatsky, S. A., Anderson, T. H., and Kuzyakov, Y. (2009). Contrasting effects of glucose, living roots and maize straw on microbial growth kinetics and substrate availability in soil. Eur. J. Soil Sci. 60, 186–197. doi: 10.1111/j.1365-2389.2008.01103.x
Blankinship, J. C., Berhe, A. A., Crow, S. E., Druhan, J. L., Heckman, K. A., Keiluweit, M., et al. (2018). Improving understanding of soil organic matter dynamics by triangulating theories, measurements, and models. Biogeochemistry 140, 1–13. doi: 10.1007/s10533-018-0478-2
Blume, E., Bischoff, M., Reichert, J. M., Moorman, T., Konopka, A., and Turco, R. F. (2002). Surface and subsurface microbial biomass, community structure and metabolic activity as a function of soil depth and season. Appl. Soil Ecol. 20, 171–181. doi: 10.1016/S0929-1393(02)00025-2
Bradford, M. A. (2013). Thermal adaptation of decomposer communities in warming soils. Front. Microbiol. 4:333. doi: 10.3389/fmicb.2013.00333
Brewer, T. E., Aronson, E. L., Arogyaswamy, K., Billings, S. A., Botthoff, J. K., Campbell, A. N., et al. (2019). Ecological and genomic attributes of novel bacterial taxa that thrive in subsurface soil horizons. mBio 10:e01318-19. doi: 10.1128/mBio.01318-19
Brookes, P. C., Landman, A., Pruden, G., and Jenkinson, D. S. (1985). Chloroform fumigation and the release of soil nitrogen: a rapid direct extraction method to measure microbial biomass nitrogen in soil. Soil Biol. Biochem. 17, 837–842. doi: 10.1016/0038-0717(85)90144-0
Brzostek, E. R., and Finzi, A. C. (2012). Seasonal variation in the temperature sensitivity of proteolytic enzyme activity in temperate forest soils. J. Geophys. Res. Biogeosci. 117, 1–10. doi: 10.1029/2011JG001688
Burnham, K. P., and Anderson, D. R. (2004). Multimodel inference: understanding AIC and BIC in model selection. Sociol. Methods Res. 33, 261–304. doi: 10.1177/0049124104268644
Burns, R. G., DeForest, J. L., Marxsen, J., Sinsabaugh, R. L., Stromberger, M. E., Wallenstein, M. D., et al. (2013). Soil enzymes in a changing environment: current knowledge and future directions. Soil Biol. Biochem. 58, 216–234. doi: 10.1016/j.soilbio.2012.11.009
Cao, P., Zhang, L. M., Shen, J. P., Zheng, Y. M., Di, H. J., and He, J. Z. (2012). Distribution and diversity of archaeal communities in selected Chinese soils. FEMS Microbiol. Ecol. 80, 146–158. doi: 10.1111/j.1574-6941.2011.01280.x
Carrillo, Y., Dijkstra, F. A., LeCain, D., Blumenthal, D., and Pendall, E. (2018). Elevated CO2 and warming cause interactive effects on soil carbon and shifts in carbon use by bacteria. Ecol. Lett. 21, 1639–1648. doi: 10.1111/ele.13140
Cavicchioli, R., Ripple, W. J., Timmis, K. N., Azam, F., Bakken, L. R., Baylis, M., et al. (2019). Scientists’ warning to humanity: microorganisms and climate change. Nat. Rev. Microbiol. 17, 569–586. doi: 10.1038/s41579-019-0222-5
Chen, H., Li, D., Zhao, J., Xiao, K., and Wang, K. (2018). Effects of nitrogen addition on activities of soil nitrogen acquisition enzymes: a meta-analysis. Agric. Ecosyst. Environ. 252, 126–131. doi: 10.1016/j.agee.2017.09.032
Conant, R. T., Ryan, M. G., Ågren, G. I., Birge, H. E., Davidson, E. A., Eliasson, P. E., et al. (2011). Temperature and soil organic matter decomposition rates–synthesis of current knowledge and a way forward. Glob. Chang. Biol. 17, 3392–3404. doi: 10.1111/j.1365-2486.2011.02496.x
Crowther, T. W., Todd-Brown, K. E. O., Rowe, C. W., Wieder, W. R., Carey, J. C., MacHmuller, M. B., et al. (2016). Quantifying global soil carbon losses in response to warming. Nature 540, 104–108. doi: 10.1038/nature20150
Darby, B. A., Goodale, C. L., Chin, N. A., Fuss, C. B., Lang, A. K., Ollinger, S. V., et al. (2020). Depth patterns and connections between gross nitrogen cycling and soil exoenzyme activities in three northern hardwood forests. Soil Biol. Biochem. 147:107836. doi: 10.1016/j.soilbio.2020.107836
Davidson, E. A., and Janssens, I. A. (2006). Temperature sensitivity of soil carbon decomposition and feedbacks to climate change. Nature 440, 165–173. doi: 10.1038/nature04514
de Mendiburu, F., and Yaseen, M. (2020). agricolae: Statistical Procedures for Agricultural Research. Available online at: https://cran.r-project.org/package=agricolae (accessed April, 2020).
Diamond, S., Andeer, P. F., Li, Z., Crits-Christoph, A., Burstein, D., Anantharaman, K., et al. (2019). Mediterranean grassland soil C–N compound turnover is dependent on rainfall and depth, and is mediated by genomically divergent microorganisms. Nat. Microbiol. 4, 1356–1367. doi: 10.1038/s41564-019-0449-y
Dove, N. C., Arogyaswamy, K., Billings, S. A., Botthoff, J. K., Carey, C. J., Cisco, C., et al. (2020). Continental-scale patterns of extracellular enzyme activity in the subsoil: an overlooked reservoir of microbial activity. Environ. Res. Lett. 15:1040a1. doi: 10.1088/1748-9326/abb0b3
Dove, N. C., Torn, M. S., Hart, S. C., and Tañ, N. (2021). Metabolic capabilities mute positive response to direct and indirect impacts of warming throughout the soil profile. Nat. Commun. 12:2089. doi: 10.1038/s41467-021-22408-5
Dwivedi, D., Tang, J., Bouskill, N., Georgiou, K., Chacon, S. S., and Riley, W. J. (2019). Abiotic and biotic controls on soil organo–mineral interactions: developing model structures to analyze why soil organic matter persists. Rev. Mineral. Geochem. 85, 329–348. doi: 10.2138/rmg.2019.85.11
Eilers, K. G., Debenport, S., Anderson, S., and Fierer, N. (2012). Digging deeper to find unique microbial communities: the strong effect of depth on the structure of bacterial and archaeal communities in soil. Soil Biol. Biochem. 50, 58–65. doi: 10.1016/j.soilbio.2012.03.011
Engqvist, M. K. M. (2018). Correlating enzyme annotations with a large set of microbial growth temperatures reveals metabolic adaptations to growth at diverse temperatures. BMC Microbiol. 18:177. doi: 10.1186/s12866-018-1320-7
Fierer, N., Allen, A. S., Schimel, J. P., and Holden, P. A. (2003a). Controls on microbial CO2 production: a comparison of surface and subsurface soil horizons. Glob. Chang. Biol. 9, 1322–1332. doi: 10.1046/j.1365-2486.2003.00663.x
Fierer, N., Schimel, J. P., and Holden, P. A. (2003b). Variations in microbial community composition through two soil depth profiles. Soil Biol. Biochem. 35, 167–176. doi: 10.1016/S0038-0717(02)00251-1
Fox, J., and Weisberg, S. (2019). An R Companion to Applied Regression, 3rd Edn. Thousand Oaks, CA: Sage.
Gelsomino, A., and Azzellino, A. (2011). Multivariate analysis of soils: microbial biomass, metabolic activity, and bacterial-community structure and their relationships with soil depth and type. J. Plant Nutr. Soil Sci. 174, 381–394. doi: 10.1002/jpln.200900267
German, D. P., Weintraub, M. N., Grandy, A. S., Lauber, C. L., Rinkes, Z. L., and Allison, S. D. (2011b). Optimization of hydrolytic and oxidative enzyme methods for ecosystem studies. Soil Biol. Biochem. 43, 1387–1397. doi: 10.1016/j.soilbio.2011.03.017
German, D. P., Chacon, S. S., and Allison, S. D. (2011a). Substrate concentration and enzyme allocation can affect rates of microbial decomposition. Ecology 92, 1471–1480. doi: 10.1890/10-2028.1
German, D. P., Marcelo, K. R. B., Stone, M. M., and Allison, S. D. (2012). The Michaelis-Menten kinetics of soil extracellular enzymes in response to temperature: a cross-latitudinal study. Glob. Chang. Biol. 18, 1468–1479. doi: 10.1111/j.1365-2486.2011.02615.x
Grant, R. F. (2013). Modelling changes in nitrogen cycling to sustain increases in forest productivity under elevated atmospheric CO2 and contrasting site conditions. Biogeosciences 10, 7703–7721. doi: 10.5194/bg-10-7703-2013
Griscom, B. W., Adams, J., Ellis, P. W., Houghton, R. A., Lomax, G., Miteva, D. A., et al. (2017). Natural climate solutions. Proc. Natl. Acad. Sci. U.S.A. 114, 11645–11650. doi: 10.1073/pnas.1710465114
Gross, C. D., and Harrison, R. B. (2019). The case for digging deeper: soil organic carbon storage, dynamics, and controls in our changing world. Soil Syst. 3, 1–24. doi: 10.3390/soilsystems3020028
Hansel, C. M., Fendorf, S., Jardine, P. M., and Francis, C. A. (2008). Changes in bacterial and archaeal community structure and functional diversity along a geochemically variable soil profile. Appl. Environ. Microbiol. 74, 1620–1633. doi: 10.1128/AEM.01787-07
Hanson, P. J., Griffiths, N. A., Iversen, C. M., Norby, R. J., Sebestyen, S. D., Phillips, J. R., et al. (2020). Rapid net carbon loss from a whole-ecosystem warmed peatland. AGU Adv. 1:e2020AV000163.
Hartmann, M., Lee, S., Hallam, S. J., and Mohn, W. W. (2009). Bacterial, archaeal and eukaryal community structures throughout soil horizons of harvested and naturally disturbed forest stands. Environ. Microbiol. 11, 3045–3062. doi: 10.1111/j.1462-2920.2009.02008.x
Heuck, C., Weig, A., and Spohn, M. (2015). Soil microbial biomass C: N: P stoichiometry and microbial use of organic phosphorus. Soil Biol. Biochem. 85, 119–129. doi: 10.1016/j.soilbio.2015.02.029
Hicks Pries, C. E., Castanha, C., Porras, R. C., and Torn, M. S. (2017). The whole-soil carbon flux in response to warming. Science 355, 1420–1423. doi: 10.1126/science.aal1319
Hicks Pries, C. E., Sulman, B. N., West, C., O’Neill, C., Poppleton, E., Porras, R. C., et al. (2018). Root litter decomposition slows with soil depth. Soil Biol. Biochem. 125, 103–114. doi: 10.1016/j.soilbio.2018.07.002
Ho, A., Di Lonardo, D. P., and Bodelier, P. L. E. (2017). Revisiting life strategy concepts in environmental microbial ecology. FEMS Microbiol. Ecol. 93:fix006. doi: 10.1093/femsec/fix006
Hobbs, J. K., Jiao, W., Easter, A. D., Parker, E. J., Schipper, L. A., and Arcus, V. L. (2013). Change in heat capacity for enzyme catalysis determines temperature dependence of enzyme catalyzed rates. ACS Chem. Biol. 8, 2388–2393. doi: 10.1021/cb4005029
Isobe, K., Allison, S. D., Khalili, B., Martiny, A. C., and Martiny, J. B. H. (2019). Phylogenetic conservation of bacterial responses to soil nitrogen addition across continents. Nat. Commun. 10:2499. doi: 10.1038/s41467-019-10390-y
Jiang, L., Song, Y., Sun, L., Song, C., Wang, X., Ma, X., et al. (2020). Effects of warming on carbon emission and microbial abundances across different soil depths of a peatland in the permafrost region under anaerobic condition. Appl. Soil Ecol. 156:103712. doi: 10.1016/j.apsoil.2020.103712
Jiao, S., Chen, W., Wang, J., Du, N., Li, Q., and Wei, G. (2018). Soil microbiomes with distinct assemblies through vertical soil profiles drive the cycling of multiple nutrients in reforested ecosystems. Microbiome 6:146. doi: 10.1186/s40168-018-0526-0
Jing, X., Chen, X., Tang, M., Ding, Z., Jiang, L., Li, P., et al. (2017). Nitrogen deposition has minor effect on soil extracellular enzyme activities in six Chinese forests. Sci. Total Environ. 607–608, 806–815. doi: 10.1016/j.scitotenv.2017.07.060
Jobbágy, E. G., and Jackson, R. B. (2000). The vertical distribution of soil organic carbon and its relation to climate and vegetation. Ecol. Appl. 10, 423–436.
Jones, D. L., Magthab, E. A., Gleeson, D. B., Hill, P. W., Sánchez-Rodríguez, A. R., Roberts, P., et al. (2018). Microbial competition for nitrogen and carbon is as intense in the subsoil as in the topsoil. Soil Biol. Biochem. 117, 72–82. doi: 10.1016/j.soilbio.2017.10.024
Khalili, B., Nourbakhsh, F., Nili, N., Khademi, H., and Sharifnabi, B. (2011). Diversity of soil cellulase isoenzymes is associated with soil cellulase kinetic and thermodynamic parameters. Soil Biol. Biochem. 43, 1639–1648. doi: 10.1016/j.soilbio.2011.03.019
Koch, O., Tscherko, D., and Kandeler, E. (2007). Temperature sensitivity of microbial respiration, nitrogen mineralization, and potential soil enzyme activities in organic alpine soils. Glob. Biogeochem. Cycles 21, 1–11. doi: 10.1029/2007GB002983
Köchy, M., Hiederer, R., and Freibauer, A. (2015). Global distribution of soil organic carbon–part 1: masses and frequency distributions of SOC stocks for the tropics, permafrost regions, wetlands, and the world. Soil 1, 351–365. doi: 10.5194/soil-1-351-2015
Koshland, D. E. (2002). The application and usefulness of the ratio kcat/KM. Bioorg. Chem. 30, 211–213. doi: 10.1006/bioo.2002.1246
Kramer, S., Marhan, S., Haslwimmer, H., Ruess, L., and Kandeler, E. (2013). Temporal variation in surface and subsoil abundance and function of the soil microbial community in an arable soil. Soil Biol. Biochem. 61, 76–85. doi: 10.1016/j.soilbio.2013.02.006
Kujur, M., and Kumar Patel, A. (2014). Kinetics of soil enzyme activities under different ecosystems: an index of soil quality. Chil. J. Agric. Res. 74, 96–104. doi: 10.4067/S0718-58392014000100015
Lax, S., Abreu, C. I., and Gore, J. (2020). Higher temperatures generically favour slower-growing bacterial species in multispecies communities. Nat. Ecol. Evol. 4, 560–567. doi: 10.1038/s41559-020-1126-5
Liu, N., Hu, H., Ma, W., Deng, Y., Liu, Y., Hao, B., et al. (2019). Contrasting biogeographic patterns of bacterial and archaeal diversity in the top- and subsoils of temperate grasslands. mSystems 4, 1–18. doi: 10.1128/msystems.00566-19
Loeppmann, S., Blagodatskaya, E., Pausch, J., and Kuzyakov, Y. (2016a). Enzyme properties down the soil profile–a matter of substrate quality in rhizosphere and detritusphere. Soil Biol. Biochem. 103, 274–283. doi: 10.1016/j.soilbio.2016.08.023
Loeppmann, S., Blagodatskaya, E., Pausch, J., and Kuzyakov, Y. (2016b). Substrate quality affects kinetics and catalytic efficiency of exo-enzymes in rhizosphere and detritusphere. Soil Biol. Biochem. 92, 111–118. doi: 10.1016/j.soilbio.2015.09.020
Malik, A. A., Martiny, J. B. H., Brodie, E. L., Martiny, A. C., Treseder, K. K., and Allison, S. D. (2020). Defining trait-based microbial strategies with consequences for soil carbon cycling under climate change. ISME J. 14, 1–9. doi: 10.1038/s41396-019-0510-0
Mazerolle, M. J. (2020). AICcmodavg: Model Selection and Multimodel Inference Based on (Q)AIC(c). Available online at: https://cran.r-project.org/package=AICcmodavg (accessed May, 2020).
Moscatelli, M. C., Lagomarsino, A., Garzillo, A. M. V., Pignataro, A., and Grego, S. (2012). β-Glucosidase kinetic parameters as indicators of soil quality under conventional and organic cropping systems applying two analytical approaches. Ecol. Indic. 13, 322–327. doi: 10.1016/j.ecolind.2011.06.031
Nannipieri, P., Giagnoni, L., Renella, G., Puglisi, E., Ceccanti, B., Masciandaro, G., et al. (2012). Soil enzymology: classical and molecular approaches. Biol. Fertil. Soils 48, 743–762. doi: 10.1007/s00374-012-0723-0
Nottingham, A. T., Meir, P., Velasquez, E., and Turner, B. L. (2020). Soil carbon loss by experimental warming in a tropical forest. Nature 584, 234–237. doi: 10.1038/s41586-020-2566-4
Nottingham, A. T., Turner, B. L., Whitaker, J., Ostle, N., Bardgett, R. D., McNamara, N. P., et al. (2016). Temperature sensitivity of soil enzymes along an elevation gradient in the Peruvian Andes. Biogeochemistry 127, 217–230. doi: 10.1007/s10533-015-0176-2
Nuccio, E. E., Starr, E., Karaoz, U., Brodie, E. L., Zhou, J., Tringe, S. G., et al. (2020). Niche differentiation is spatially and temporally regulated in the rhizosphere. ISME J. 14, 999–1014. doi: 10.1038/s41396-019-0582-x
Nunan, N., Schmidt, H., and Raynaud, X. (2020). The ecology of heterogeneity: soil bacterial communities and C dynamics. Philos. Trans. R. Soc. B Biol. Sci. 375:20190249. doi: 10.1098/rstb.2019.0249
Pasut, C., Tang, F. H. M., Hamilton, D., Riley, W. J., and Maggi, F. (2021). Spatiotemporal assessment of GHG emissions and nutrient sequestration linked to agronutrient runoff in global wetlands. Glob. Biogeochem. Cycles 35:e2020GB006816. doi: 10.1029/2020GB006816
Perucci, P. (1992). Enzyme activity and microbial biomass in a field soil amended with municipal refuse. Biol. Fertil. Soils 14, 54–60. doi: 10.1007/BF00336303
Puissant, J., Jones, B., Goodall, T., Mang, D., Blaud, A., Gweon, H. S., et al. (2019). The pH optimum of soil exoenzymes adapt to long term changes in soil pH. Soil Biol. Biochem. 138:107601. doi: 10.1016/j.soilbio.2019.107601
R Core Team (2020). R: A Language and Environment for Statistical Computing. Vienna: R Foundation for Statistical Computing.
Ramin, K. I., and Allison, S. D. (2019). Bacterial tradeoffs in growth rate and extracellular enzymes. Front. Microbiol. 10:2956.
Razavi, B. S., Blagodatskaya, E., and Kuzyakov, Y. (2015). Nonlinear temperature sensitivity of enzyme kinetics explains canceling effect–a case study on loamy haplic Luvisol. Front. Microbiol. 6:1126. doi: 10.3389/fmicb.2015.01126
Razavi, B. S., Blagodatskaya, E., and Kuzyakov, Y. (2016). Temperature selects for static soil enzyme systems to maintain high catalytic efficiency. Soil Biol. Biochem. 97, 15–22. doi: 10.1016/j.soilbio.2016.02.018
Ren, Q., Song, H., Yuan, Z., Ni, X., and Li, C. (2018). Changes in soil enzyme activities and microbial biomass after revegetation in the Three Gorges Reservoir, China. Forests 9:249. doi: 10.3390/f9050249
Ritz, C., Baty, F., Streibig, J. C., and Gerhard, D. (2015). Dose-response analysis using R. PLoS One 10:e0146021. doi: 10.1371/journal.pone.0146021
Robinson, J. M., O’Neill, T. A., Ryburn, J., Liang, L. L., Arcus, V. L., and Schipper, L. A. (2017). Rapid laboratory measurement of the temperature dependence of soil respiration and application to changes in three diverse soils through the year. Biogeochemistry 133, 101–112. doi: 10.1007/s10533-017-0314-0
Rumpel, C., and Kögel-Knabner, I. (2011). Deep soil organic matter-a key but poorly understood component of terrestrial C cycle. Plant Soil 338, 143–158. doi: 10.1007/s11104-010-0391-5
Salomé, C., Nunan, N., Pouteau, V., Lerch, T. Z., and Chenu, C. (2010). Carbon dynamics in topsoil and in subsoil may be controlled by different regulatory mechanisms. Glob. Chang. Biol. 16, 416–426. doi: 10.1111/j.1365-2486.2009.01884.x
Schipper, L. A., Hobbs, J. K., Rutledge, S., and Arcus, V. L. (2014). Thermodynamic theory explains the temperature optima of soil microbial processes and high Q10 values at low temperatures. Glob. Chang. Biol. 20, 3578–3586. doi: 10.1111/gcb.12596
Schnecker, J., Wild, B., Hofhansl, F., Alves, R. J. E., Bárta, J., Èapek, P., et al. (2014). Effects of soil organic matter properties and microbial community composition on enzyme activities in cryoturbated arctic soils. PLoS One 9:e94076. doi: 10.1371/journal.pone.0094076
Schnecker, J., Wild, B., Takriti, M., Alves, R. J. E., Gentsch, N., Gittel, A., et al. (2015). Microbial community composition shapes enzyme patterns in topsoil and subsoil horizons along a latitudinal transect in Western Siberia. Soil Biol. Biochem. 83, 106–115. doi: 10.1016/j.soilbio.2015.01.016
Sinsabaugh, R. L., Belnap, J., Findlay, S. G., Shah, J. J. F., Hill, B. H., Kuehn, K. A., et al. (2014). Extracellular enzyme kinetics scale with resource availability. Biogeochemistry 121, 287–304. doi: 10.1007/s10533-014-0030-y
Sinsabaugh, R. L., and Shah, J. J. F. (2012). Ecoenzymatic stoichiometry and ecological theory. Annu. Rev. Ecol. Evol. Syst. 43, 313–343. doi: 10.1146/annurev-ecolsys-071112-124414
Somero, G. N. (2004). Adaptation of enzymes to temperature: searching for basic “strategies”. Comp. Biochem. Physiol. Part B Biochem. Mol. Biol. 139, 321–333. doi: 10.1016/j.cbpc.2004.05.003
Soong, J. L., Castanha, C., Hicks Pries, C. E., Ofiti, N., Porras, R. C., Riley, W. J., et al. (2021). Five years of whole-soil warming led to loss of subsoil carbon stocks and increased CO2 efflux. Sci. Adv. 7:eabd1343. doi: 10.1126/sciadv.abd1343
Soong, J. L., Phillips, C. L., Ledna, C., Koven, C. D., and Torn, M. S. (2020). CMIP5 models predict rapid and deep soil warming over the 21st century. J. Geophys. Res. Biogeosciences 125:e2019JG005266. doi: 10.1029/2019JG005266
Steinweg, J. M., Jagadamma, S., Frerichs, J., and Mayes, M. A. (2013). Activation energy of extracellular enzymes in soils from different biomes. PLoS One 8:e59943. doi: 10.1371/journal.pone.0059943
Stone, M. M., DeForest, J. L., and Plante, A. F. (2014). Changes in extracellular enzyme activity and microbial community structure with soil depth at the Luquillo Critical Zone Observatory. Soil Biol. Biochem. 75, 237–247. doi: 10.1016/j.soilbio.2014.04.017
Stone, M. M., Weiss, M. S., Goodale, C. L., Adams, M. B., Fernandez, I. J., German, D. P., et al. (2012). Temperature sensitivity of soil enzyme kinetics under N-fertilization in two temperate forests. Glob. Chang. Biol. 18, 1173–1184. doi: 10.1111/j.1365-2486.2011.02545.x
Struvay, C., and Feller, G. (2012). Optimization to low temperature activity in psychrophilic enzymes. Int. J. Mol. Sci. 13, 11643–11665. doi: 10.3390/ijms130911643
Sulman, B. N., Phillips, R. P., Oishi, A. C., Shevliakova, E., and Pacala, S. W. (2014). Microbe-driven turnover offsets mineral-mediated storage of soil carbon under elevated CO2. Nat. Clim. Chang. 4, 1099–1102. doi: 10.1038/nclimate2436
Tang, J., and Riley, W. J. (2015). Weaker soil carbon-climate feedbacks resulting from microbial and abiotic interactions. Nat. Clim. Chang. 5, 56–60. doi: 10.1038/nclimate2438
Tang, J., and Riley, W. J. (2019). Competitor and substrate sizes and diffusion together define enzymatic depolymerization and microbial substrate uptake rates. Soil Biol. Biochem. 139:107624. doi: 10.1016/j.soilbio.2019.107624
Taylor, J. P., Wilson, B., Mills, M. S., and Burns, R. G. (2002). Comparison of microbial numbers and enzymatic activities in surface soils and subsoils using various techniques. Soil Biol. Biochem. 34, 387–401. doi: 10.1016/S0038-0717(01)00199-7
Tian, P., Razavi, B. S., Zhang, X., Wang, Q., and Blagodatskaya, E. (2020). Microbial growth and enzyme kinetics in rhizosphere hotspots are modulated by soil organics and nutrient availability. Soil Biol. Biochem. 141:107662. doi: 10.1016/j.soilbio.2019.107662
Tischer, A., Blagodatskaya, E., and Hamer, U. (2015). Microbial community structure and resource availability drive the catalytic efficiency of soil enzymes under land-use change conditions. Soil Biol. Biochem. 89, 226–237. doi: 10.1016/j.soilbio.2015.07.011
Trasar-Cepeda, C., Gil-Sotres, F., and Leirós, M. C. (2007). Thermodynamic parameters of enzymes in grassland soils from Galicia, NW Spain. Soil Biol. Biochem. 39, 311–319. doi: 10.1016/j.soilbio.2006.08.002
Triebwasser-Freese, D. J., Tharayil, N., Preston, C. M., and Gerard, P. G. (2015). Catalytic kinetics and activation energy of soil peroxidases across ecosystems of differing lignin chemistries. Biogeochemistry 124, 113–129. doi: 10.1007/s10533-015-0086-3
Trumbore, S. (2000). Age of soil organic matter and soil respiration: radiocarbon constraints on belowground C dynamics. Ecol. Appl. 10, 399–411.
Trumbore, S. (2009). Radiocarbon and soil carbon dynamics. Annu. Rev. Earth Planet. Sci. 37, 47–66. doi: 10.1146/annurev.earth.36.031207.124300
Turner, S., Mikutta, R., Meyer-Stüve, S., Guggenberger, G., Schaarschmidt, F., Lazar, C. S., et al. (2017). Microbial community dynamics in soil depth profiles over 120,000 years of ecosystem development. Front. Microbiol. 8:874. doi: 10.3389/fmicb.2017.00874
Van Gestel, N., Shi, Z., Van Groenigen, K. J., Osenberg, C. W., Andresen, L. C., Dukes, J. S., et al. (2018). Predicting soil carbon loss with warming. Nature 554, E4–E5. doi: 10.1038/nature25745
Venkatesan, S., and Senthurpandian, V. K. (2006). Comparison of enzyme activity with depth under tea plantations and forested sites in south India. Geoderma 137, 212–216. doi: 10.1016/j.geoderma.2006.08.011
Wallenstein, M., Allison, S. D., Ernakovich, J., Steinweg, J. M., and Sinsabaugh, R. L. (2011). “Controls on the temperature sensitivity of soil enzymes: a key driver of in situ enzyme activity rates,” in Soil Enzymology, eds G. Shukla and A. Varma (Berlin: Springer Berlin Heidelberg), 245–258. doi: 10.1007/978-3-642-14225-3_13
Wang, B., and Allison, S. D. (2019). Emergent properties of organic matter decomposition by soil enzymes. Soil Biol. Biochem. 136:107522. doi: 10.1016/j.soilbio.2019.107522
Wang, G., Jagadamma, S., Mayes, M. A., Schadt, C. W., Steinweg, J. M., Gu, L., et al. (2015). Microbial dormancy improves development and experimental validation of ecosystem model. ISME J. 9, 226–237. doi: 10.1038/ismej.2014.120
Wang, Y., Zhang, H., Ciais, P., Goll, D., Huang, Y., Wood, J. D., et al. (2021). Microbial activity and root carbon inputs are more important than soil carbon diffusion in simulating soil carbon profiles. J. Geophys. Res. Biogeosci. 126, 1–19. doi: 10.1029/2020JG006205
Waring, B. G., Weintraub, S. R., and Sinsabaugh, R. L. (2014). Ecoenzymatic stoichiometry of microbial nutrient acquisition in tropical soils. Biogeochemistry 117, 101–113. doi: 10.1007/s10533-013-9849-x
Wieder, W. R., Allison, S. D., Davidson, E. A., Georgiou, K., Hararuk, O., He, Y., et al. (2015). Explicitly representing soil microbial processes in Earth system models. Glob. Biogeochem. Cycles 29, 1782–1800. doi: 10.1002/2015GB005188
Wieder, W. R., Grandy, A. S., Kallenbach, C. M., and Bonan, G. B. (2014). Integrating microbial physiology and physio-chemical principles in soils with the MIcrobial-MIneral Carbon Stabilization (MIMICS) model. Biogeosciences 11, 3899–3917. doi: 10.5194/bg-11-3899-2014
Wilke, C. O. (2020). cowplot: Streamlined Plot Theme and Plot Annotations for “ggplot2”. Available online at: https://cran.r-project.org/package=cowplot (accessed February, 2021).
Xu, M., Li, X., Kuyper, T. W., Xu, M., Li, X., and Zhang, J. (2021). High microbial diversity stabilizes the responses of soil organic carbon decomposition to warming in the subsoil on the Tibetan Plateau. Glob. Chang. Biol. 27, 2061–2075. doi: 10.1111/gcb.15553
Yan, H., Yang, F., Gao, J., Peng, Z., and Chen, W. (2019). Subsoil microbial community responses to air exposure and legume growth depend on soil properties across different depths. Sci. Rep. 9:18536. doi: 10.1038/s41598-019-55089-8
Zhang, X., Yang, Y., Zhang, C., Niu, S., Yang, H., Yu, G., et al. (2018). Contrasting responses of phosphatase kinetic parameters to nitrogen and phosphorus additions in forest soils. Funct. Ecol. 32, 106–116.
Keywords: extracellular enzymes, soil, subsoil, temperature sensitivity, Michaelis-Menten, Arrhenius, macromolecular rate theory, enzyme kinetics
Citation: Alves RJE, Callejas IA, Marschmann GL, Mooshammer M, Singh HW, Whitney B, Torn MS and Brodie EL (2021) Kinetic Properties of Microbial Exoenzymes Vary With Soil Depth but Have Similar Temperature Sensitivities Through the Soil Profile. Front. Microbiol. 12:735282. doi: 10.3389/fmicb.2021.735282
Received: 02 July 2021; Accepted: 03 November 2021;
Published: 30 November 2021.
Edited by:
Graeme W. Nicol, Université de Lyon, FranceReviewed by:
Jeremy Puissant, UK Centre for Ecology and Hydrology (UKCEH), United KingdomLiz Shaw, University of Reading, United Kingdom
Copyright © 2021 Alves, Callejas, Marschmann, Mooshammer, Singh, Whitney, Torn and Brodie. This is an open-access article distributed under the terms of the Creative Commons Attribution License (CC BY). The use, distribution or reproduction in other forums is permitted, provided the original author(s) and the copyright owner(s) are credited and that the original publication in this journal is cited, in accordance with accepted academic practice. No use, distribution or reproduction is permitted which does not comply with these terms.
*Correspondence: Ricardo J. Eloy Alves, rjealves@lbl.gov; Eoin L. Brodie, elbrodie@lbl.gov
†Present address: Hans W. Singh, Center for Marine Biotechnology and Biomedicine, Scripps Institution of Oceanography, University of California, San Diego, La Jolla, CA, United States; Bizuayehu Whitney, Khoury College of Computer Sciences, Northeastern University, Boston, MA, United States