- 1Stomatological Hospital, Southern Medical University and Guangdong Provincial Stomatological Hospital, Guangzhou, China
- 2School of Dentistry and Jonsson Comprehensive Cancer Center, University of California, Los Angeles, Los Angeles, CA, United States
The oral microbiome is one of the most complex microbial communities in the human body and is closely related to oral and systemic health. Dental plaque biofilms are the primary etiologic factor of periodontitis, which is a common chronic oral infectious disease. The interdependencies that exist among the resident microbiota constituents in dental biofilms and the interaction between pathogenic microorganisms and the host lead to the occurrence and progression of periodontitis. Therefore, accurately and comprehensively detecting periodontal organisms and dissecting their corresponding functional activity characteristics are crucial for revealing periodontitis pathogenesis. With the development of metagenomics and metatranscriptomics, the composition and structure of microbial communities as well as the overall functional characteristics of the flora can be fully profiled and revealed. In this review, we will critically examine the currently available metagenomic and metatranscriptomic evidence to bridge the gap between microbial dysbiosis and periodontitis and related systemic diseases.
Introduction
Periodontitis, triggered by the highly pathogenic biofilm accumulated on teeth, is a chronic inflammatory disease that progressively destroys the supporting periodontal tissues. If left untreated, this oral infectious disease can eventually lead to tooth loss. In addition, accumulating evidence has demonstrated that periodontitis is a potential risk factor for many systemic diseases, such as diabetes, cardiovascular diseases, and rheumatoid arthritis (RA) (Teles et al., 2021). Pathogenic microbes and their products in dental plaque can cause systemic inflammation and immune responses. The prevalence of periodontal diseases is high both in developed and developing countries, ranging from 20 to 50% (Nazir, 2017). In addition, severe periodontitis affects approximately 10% of the global population (Frencken et al., 2017). A survey reported that more than 40% of the U.S. population aged ≥30 years had periodontitis, and 7.8% of these individuals suffered from severe periodontitis (Eke et al., 2020). According to the 4th National Oral Health Epidemiological Survey, periodontitis is highly prevalent in Chinese adults. This issue is especially prevalent in elderly individuals aged 55–74, as 70% of them have detectable periodontal attachment loss, and approximately 15% of them have detectable deep periodontal pockets (≥6 mm). Even among adults aged 35–44, the detection rate of periodontal attachment loss reaches 33%, which demonstrates that periodontitis has a relatively high prevalence even in young adults (Jiao et al., 2021). According to the Global Burden of Disease Study, periodontal disease is a component of the global burden of chronic diseases and therefore represents a significant public health problem (Vos et al., 2017).
It has been well established that polymicrobial biofilms play an essential role in the initiation and progression of periodontitis. However, a number of questions remain to be addressed. For instance, what are the detailed differences in genomic architecture between microbial biofilms from patients with periodontitis and those from healthy volunteers? How do changes in microbial composition drive the pathogenesis of periodontitis? Are there any special microbial features that link to systemic diseases? In recent years, the development of metagenomics and metatranscriptomics has allowed the high-throughput study of microbial communities, which might shed light on the above questions. In this review, we will critically review the currently available evidence to gain a better understanding of the links between microbial dysbiosis and periodontitis and related systemic diseases.
Overview of Metagenomics and Metatranscriptomics
Metagenomics, the genomic analysis of all microorganisms in the environment, has utilized sequencing analysis to investigate microbial diversity, population structure, evolutionary relationships, functional activity, mutual collaboration, and the association between microorganisms and the environment (Handelsman, 2004). Via high-throughput sequencing, metagenomics is able to produce a significant quantity of sequence data, with between 120 gigabases and 1.5 terabases per run (Quince et al., 2017). The traditional microbial detection and identification approaches are mainly based on traditional culture methods, including sample preparation, broth culture, plate count, isolation of single bacterial colonies and microbial identification, and are not able to detect uncultivated microbial species. The cultivation-independent property and high sequencing efficiency of metagenomic technology make it possible to analyze the functional diversity of microbial communities, interpret the metabolic pathway compositions, explore the interaction patterns between microbes and the environment, and discover featured genes or species with specific functions (Wang and Jia, 2016).
Metatranscriptomics, emerging after metagenomics, mainly studies the gene expression profile and the in situ function of microbial communities (Yu et al., 2019), which also avoids the isolation and cultivation of microorganisms and effectively explores biological diversity at deeper and more intricate levels. Metatranscriptomics provides a broader perspective than metagenomics, as it can reveal details about transcriptionally active populations and contribute to exploring the gene expression pattern and functional characteristics of complex microbial communities (Stavros et al., 2016). Through combining metatranscriptomics and metagenomics, the taxonomy and function of the biological community under particular conditions can be linked together, and sophisticated in-depth related functions of the microbial community can be investigated as a whole (Aguiar-Pulido et al., 2016).
The analysis process of metagenomics and metatranscriptomics can be divided into the following four steps (shown in Figure 1): ➀ Sample collection and extraction: according to the purpose and scheme of the experiment, microbial samples are collected from the oral cavity, and DNA/RNA are extracted. ➁ Data acquisition: After extracting the DNA/RNA, the data are obtained by constructing a sequencing library and performing high-throughput sequencing. ➂ Data processing: Quality control should be carried out on the acquired data to remove artificially added primers and low-quality sequences during the process of library construction and sequencing. Simultaneously, it is necessary to compare with the human genome sequence to filter possible human gene contaminants (Davis et al., 2018). Finally, the clean data are compared with multiple databases to determine the species composition and functional composition (Tsute et al., 2010; Escapa et al., 2018). ➃ Statistical analysis: Statistical analysis is conducted based on the sample metadata to explore the potential links between oral microbiota and diseases.
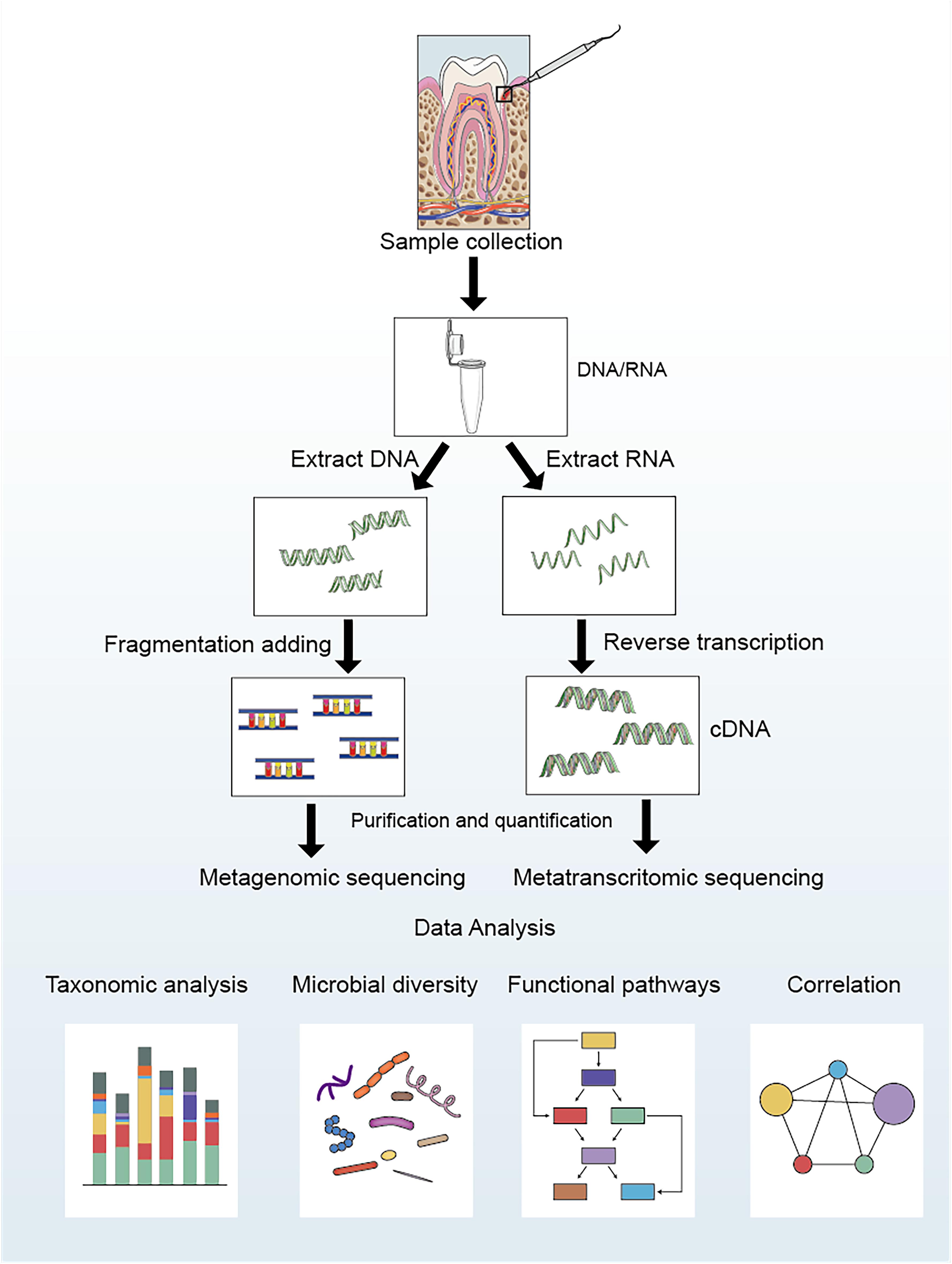
Figure 1. Laboratory flow and bioinformatic analysis for metagenomic and metatranscriptomic studies of oral microbial communities.
The emergence of next-generation sequencing methods like 454 pyrosequencing, Illumina sequencing technology, ABI SOLiDsequencing technology, and Ion torrent technology as well as third-generation sequencing technology represented by Pacific Biosciences (PacBio) and Oxford Nanopore Technologies (ONT) have put the rapid genome sequencing on the fast track (Slatko et al., 2018). Metagenomics and metatranscriptomics have gradually opened a new chapter in the field of oral microbiology. In 2007, the National Institutes of Health (NIH) launched the Human Microbiome Project (HMP), which sequenced and analyzed microbes in 9 parts of the oral cavity of 242 healthy adults (Huttenhower et al., 2012; Methé et al., 2012). In 2008, the Forsyth Institute and King’s College London Dental Institute jointly established the Human Oral Microbiome Database (HOMD), and subsequent studies have continuously enriched the database resources, which offer a significant reference for studying the role of oral microbes in health and diseases (Dewhirst et al., 2010).
The primary goal of oral microbial metagenomics is to reveal the diversity and screen the functional genes of microbial communities. Belda-Ferre et al. (2012) employed metagenomics sequencing to analyze 8 dental plaque samples from a group of individuals with good oral health, a low-activity caries group (less than 4 cavities) and a high-activity caries group (more than 8 cavities). Significant differences were observed in the microbial communities between healthy and diseased individuals at the taxonomic and functional levels. In addition, dominant bacteria such as Streptococcus oralis, Streptococcus mitis, and Streptococcus sanguis isolated from healthy individuals might be used as probiotics to prevent caries. By metagenomic analysis of dental plaques from individuals with periodontitis and healthy individuals, the microbial community structure was found to be closely related to disease manifestations. Moreover, several functional genes and metabolic pathways related to bacterial chemotaxis and biosynthesis of polysaccharides were overexpressed in periodontitis, suggesting that the formation and alteration of the microbial community in the oral cavity significantly affected the pathogenesis of periodontitis (Wang et al., 2013). Similarly, dramatic difference was observed for the functional potential of the subgingival microbiome from the deep sites in generalized aggressive periodontitis, chronic periodontitis and healthy controls (Altabtbaei, 2016). Faust et al. analyzed the co-occurrence of microbial communities in dental plaque, vagina, gut, and other body site samples from the HMP. The results showed that a small portion of microbes, such as Firmicutes, built connections across multiple microbial communities and coordinated microbiota relationships, which verified the potential links between oral diseases and systemic diseases (Faust et al., 2012).
Metatranscriptomics can provide information on the interaction between the functional characteristics of the whole microbial community and diseases, reveal the relevant microbial attributes, and elucidate potential intervention targets. For example, metatranscriptomic analysis of dental caries in children revealed that the alteration of specific enzyme activities might be closely associated with the initiation and progression of caries. The expression level of alcohol dehydrogenase has been found to be increased considerably in a caries-free group, while arginine deiminase and urease levels have been shown to be higher in a dentin caries group (Kressirer et al., 2018). Belstrøm et al. (2017) compared the composition and functional activities of microbial communities in stimulated saliva samples from healthy, periodontitis, and caries individuals with metagenomics and metatranscriptomics. The results showed that the relative abundances of traditional periodontal pathogens such as Filifactor alocis and Porphyromonas gingivalis and caries-related bacteria such as Streptococcus mutans and Lactobacillus fermentum were higher in the saliva samples from the periodontitis and caries groups, respectively. In addition, lipid metabolism was found to be suppressed in the caries group, and abnormal carbohydrate metabolism was found in both the periodontitis and caries groups. In brief, the integration of multiomics might be a powerful tool to unravel the complex network of connections between microbial communities and host responses (Manoil et al., 2020).
Metagenomics and Metatranscriptomics Studies in Periodontitis
Relationship Between Periodontitis and Oral Microorganisms
Saliva and dental plaque can be easily obtained, and thus, they are optimal for investigating the initiation and development of periodontitis (Lasserre et al., 2018). The dynamic balance between the flora and the host is vital for maintaining oral health. Qualitative or quantitative shifts of the oral microbiome lead to microecological dysbiosis, an imbalance responsible for the development of periodontitis (Dewhirst et al., 2010). Under periodontal health circumstances, gram-positive bacteria such as Actinomyces and Streptococcus dominate the plaque biofilm, while poor oral hygiene results in a shift toward gram-negative bacteria and motile microorganisms (Ezzo and Cutler, 2003). When the microenvironment fluctuates, dysbiosis occurs in the microbial community, resulting in a reduction in species richness and loss of biodiversity (Kumar P. S., 2017). A more dysbiotic microbial community has been observed in well-maintained patients with chronic periodontitis than in healthy individuals (Lu et al., 2020). There is a bilateral impact between the microbial community and the host. An imbalanced microbial community affects the host response, resulting in an inappropriate and uncontrolled level of inflammation, which further damages periodontal tissues (Kilian et al., 2016). The inflammatory response leads to changes in the microbiome and the expression of bacterial virulence factors (Curtis et al., 2020). Metagenomics and metatranscriptomics have provided novel insights into the oral microbiome in periodontitis and related systemic diseases (Figure 2).
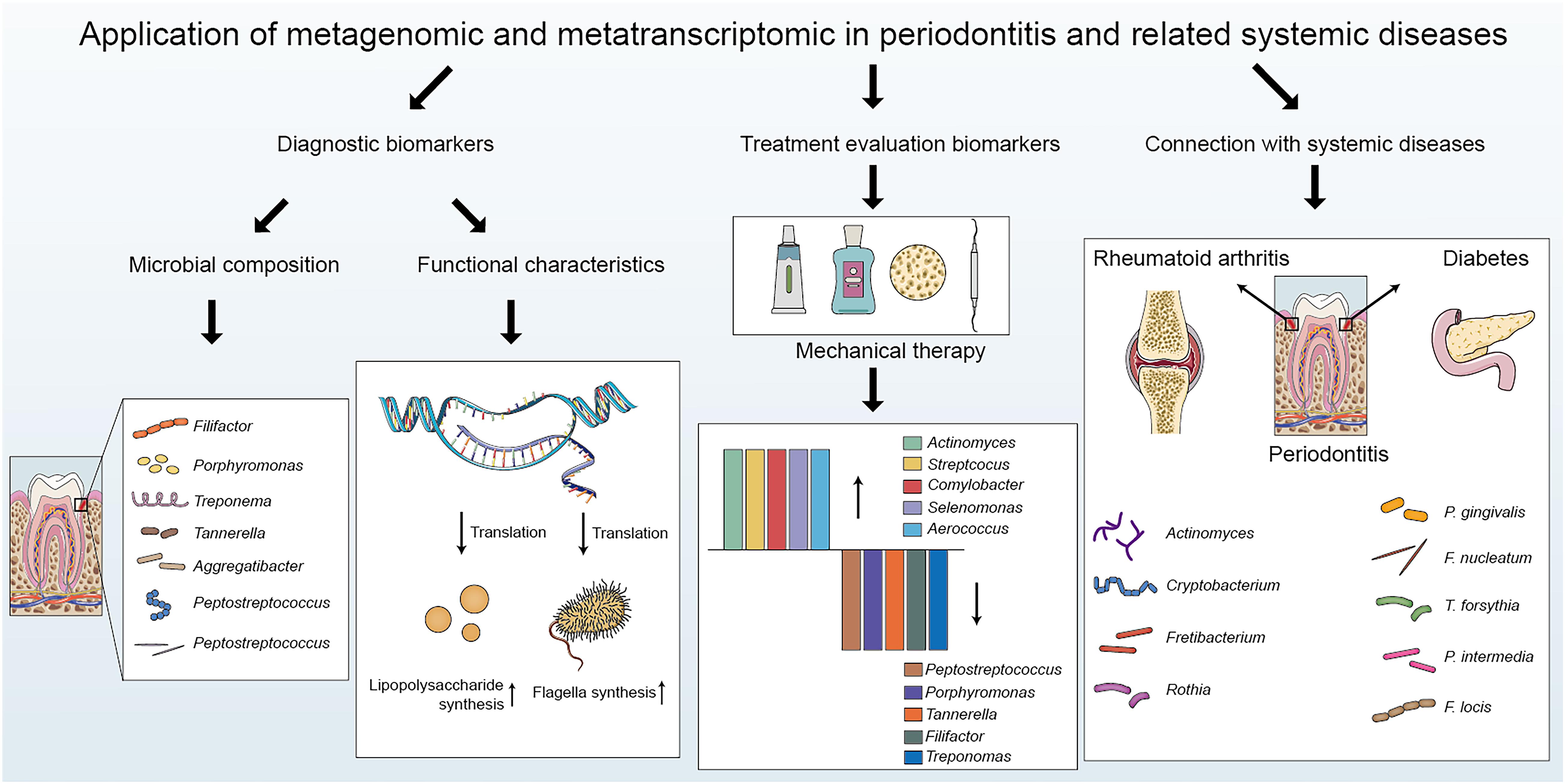
Figure 2. The applications of metagenomics and metatranscriptomics in periodontitis: biomarker detection, treatment evaluation and connection with periodontitis-related systemic diseases.
Profiling Periodontitis-Related Pathogens With Metagenomics and Metatranscriptomics
Metagenomic and metatranscriptomic approaches enable the identification of dynamic changes in the composition and structure of the periodontal microflora (Table 1). Detecting the specific and robust bacterial species that cause periodontal microbiota dysbiosis is important not only for understanding the molecular mechanisms of periodontitis initiation and progression but also for providing novel targeted therapy for periodontitis. With the metagenomic method, new microbial species related to periodontitis can be discovered, and the periodontal microbial community can be comprehensively classified. Moreover, metagenomics can clarify the differences in the microbiota between healthy and diseased periodontal tissues, helping confirm dominant periodontal bacteria. By using the 16S rRNA metagenomic approach, six genera, Filifactor, Porphyromonas, Treponema, Tannerella, Aggregatibacter, and Peptostreptococcus, have been found to be significantly enriched in the subgingival plaque samples from severe chronic periodontitis patients compared to those from healthy subjects, indicating that microbial transition might play a crucial role in periodontitis pathogenesis. The metagenomic sequencing targets the entirety of the microbial genetic information, which might help gain a better understanding of the complex microbial communities in periodontal diseases (Tsai et al., 2018). A novel periodontal disease-associated bacterium named Candidatus Bacteroides Periocalifornicus (CPB) has been mainly detected in the deep periodontal pockets through metagenomics. It might become a candidate member of the red complex, as its relative abundance has been shown to be significantly associated with that of red complex bacteria and was involved in periodontitis-associated virulence (Torres et al., 2019). Subgingival plaque samples from healthy controls, patients with stable periodontitis and patients with progressing periodontitis have been analyzed by a shotgun metagenomic approach. The study revealed a strong correlation between the loss of oral microbial diversity and periodontitis. In addition, the abundances of Lactobacillus gasseri and Olsenella uli were increased, while Campylobacter was decreased in progressing periodontitis compared to those in stable periodontitis, which indicated that the altered microbial profile might be a potential marker for distinguishing the different periodontitis states (Ai et al., 2017). Shotgun metagenomic analysis of subgingival plaque from chronic periodontitis (CP), localized aggressive periodontitis (LAP) and generalized aggressive periodontitis (GAP) has revealed that genes that regulate acetate-scavenging lifestyle, utilization of alternative nutritional sources, oxidative and nitrosative stress responses, and siderophore production were unique to LAP. In addition, the virulence-associated functionalities and stress response were gradually increased from CP to GAP to LAP (Altabtbaei et al., 2021). Using the whole genome shotgun sequencing method, several overrepresented genes encoding proteins involved in fermentation, antibiotic resistance, adhesion, invasion and intracellular resistance were observed, suggesting a more effective gene-centric methodology to identify biomarkers and periodontitis predictors (Dabdoub et al., 2016). Striking differences in the composition of the subgingival microbiome have also been observed during peri-implantitis development. A total of seven discriminative predominant bacteria, Porphyromonas gingivalis, Tannerella forsythia, Porphyromonas endodontalis, Fretibacterium fastidiosum, Prevotella intermedia, Fusobacterium nucleatum, and Treponema denticola, have been identified in peri-implantitis by metagenomic sequencing (Ghensi et al., 2020).
Metatranscriptomics is important for determining the ecosystem function of microbial communities. In addition to clarifying the active microbial community in the disease state, metatranscriptomics also provides information on the functional characteristics of the overall microbial community to explore different functional activities in health or disease conditions. Metatranscriptomic analysis of subgingival plaque samples from patients with aggressive periodontitis has demonstrated that the composition of disease-associated communities varied considerably among patients, whereas the metabolic profiles and virulence gene expression were conserved. For example, the expression levels of genes controlling butyrate production, which promoted the development of periodontitis, increased in microbial communities from different patients, providing more profound insights into the relationship between microbe functional activities and periodontitis (Jorth et al., 2014). Through metatranscriptomic methodology, metabolic activities such as iron acquisition, lipopolysaccharide synthesis, and flagella synthesis have been found to be increased in periodontitis. Surprisingly, most virulence factors whose expression was upregulated in patients with periodontitis were from non-dominant periodontitis pathogens. These findings indicate that metatranscriptomics contributes to enhance the understanding of the core activities that represent the characteristics of periodontitis and the role of individual subgingival organisms in periodontitis (Duran-Pinedo et al., 2014). Cobalamin biosynthesis, proteolysis, and potassium transport have been found to be different in subgingival plaque samples from progressing and stable sites of periodontitis, indicating that changes in microbiome function were closely associated with periodontitis development (Yost et al., 2015).
Most of the studies described above are cross-sectional studies that focused on detecting changes in the composition of microbial communities under different conditions and revealed that microbial dysbiosis is directly related to periodontitis occurrence and development. However, additional longitudinal studies are needed to better understand the correlation between the structural composition/metabolic activities of the periodontitis-associated microbiome and disease progression. In addition, it is necessary to combine the expression profile of the host with microbial community structure, which might contribute to an in-depth understanding of the interaction between the host and microbiome (Solbiati and Frias-Lopez, 2018). Furthermore, proteomics and metabolomics are promising tools for clearly reflecting the functional proteins and final metabolites produced by the microbiome under different conditions, which can accurately reveal the host responses and aid the discovery of potential robust markers at the initial stage of periodontitis.
Detecting the Alteration of Periodontitis-Related Pathogens With Metagenomics Before and After Treatment
The purpose of initial periodontal therapy is to eliminate pathogenic factors, control inflammation, and curb disease development. Multiomic analysis comparing the changes in periodontal microorganisms before and after initial periodontal therapy is conducive to evaluating therapeutic efficacy (Table 2). Through the metagenomic shotgun sequencing approach, Shi et al. (2015) compared the dynamic changes in the subgingival microbiota in patients with periodontitis pre- and post-periodontal treatment at the same tooth sites. The relative abundance of periodontitis pathogens and disease-associated pathways involving flagella assembly and bacterial chemotaxis were notably changed after periodontal treatment, indicating that the subgingival microbiome composition and functional activities might be potential indicators for disease diagnosis and prognosis prediction. 16S rRNA metagenomic research has been conducted for comparative analysis of subgingival plaque samples from periodontitis patients using antiadhesive hydroxyapatite toothpaste or antimicrobial and antiadhesive fluoride toothpaste following mechanical periodontal therapy. Interestingly, different kinds of toothpaste did not cause significant changes in the microbial composition, suggesting that the toothpaste ingredients had similar effects on the oral microbiota (Hagenfeld et al., 2019). Pyrosequencing of subgingival plaque samples from patients with mandibular class II buccal furcation defects following furcation therapy with enamel matrix derivative (EMD) showed that EMD treatment selectively changed the subgingival microbial composition by reducing the abundance of pathogens and increasing symbiotic flora richness (Queiroz et al., 2017). Califf et al. (2017) simultaneously used 16S rRNA sequencing and shotgun metagenomic sequencing methodologies to perform multiomic analysis on subgingival and supragingival plaque samples from participants who were requested to rinse their mouths with 0.25% sodium hypochlorite or water as controls to compare the differences in the microbial communities in the periodontal pockets. Significant changes in bacterial genera, species, and metabolites were observed before and after treatment, and metabolite richness might be a more sensitive marker for reflecting the changed periodontitis status. Multiomic approaches, including 16S rRNA sequencing, shotgun metagenomics, and metabolomics, have shown that the relative abundance of Porphyromonas, Treponema, and Tannerella, as well as the levels of many metabolites, were decreased following periodontal therapy. Periodontal intervention not only affected the taxonomic composition of subgingival microbial communities but also allowed species benefiting periodontal health to recolonize the oral cavity (Zhang et al., 2020). To the best of our knowledge, very little information is currently available for the metatranscriptomic changes in the oral microbiome following periodontal treatment.
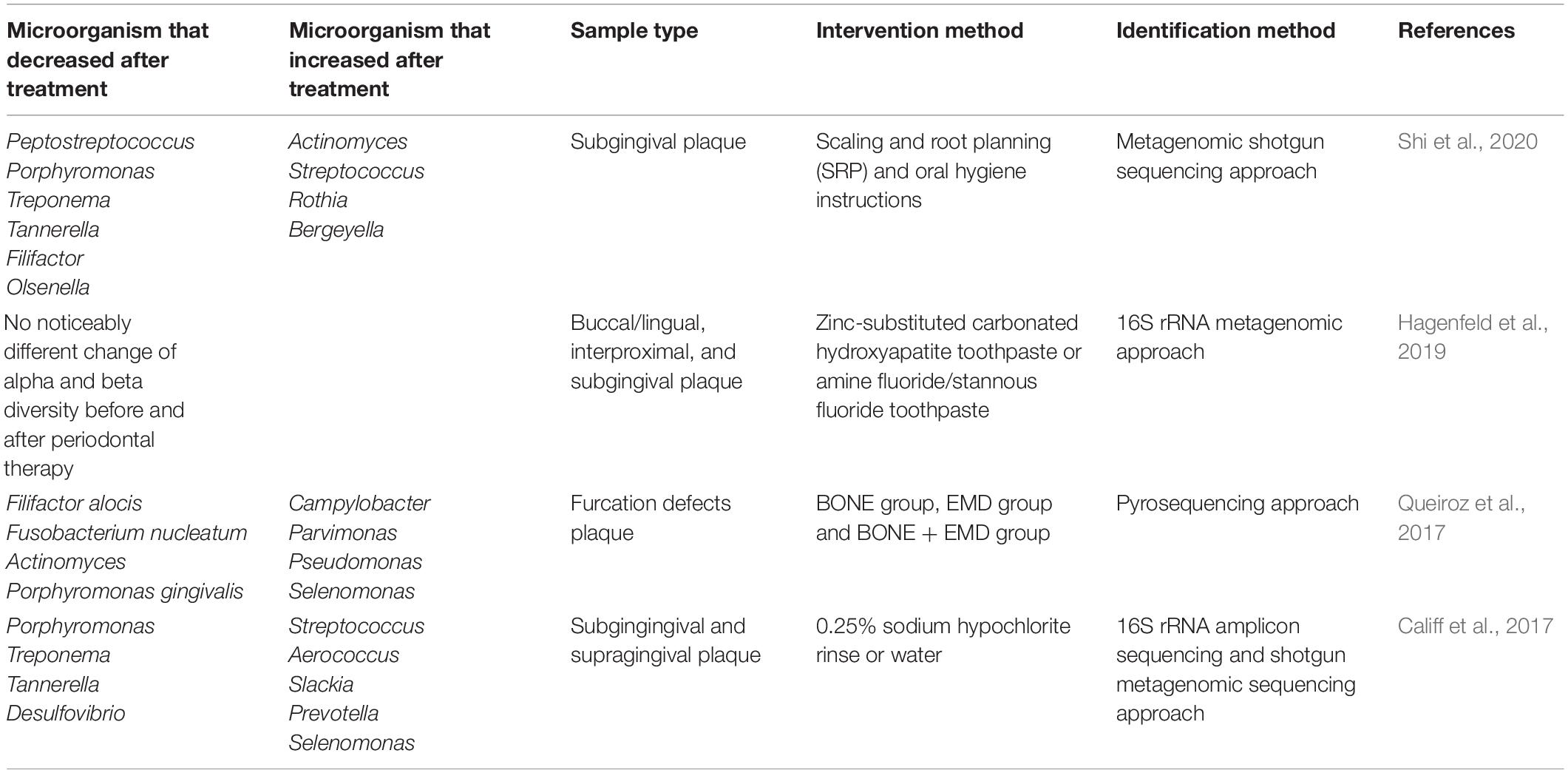
Table 2. Detecting the alteration of periodontitis related pathogens before and after treatment with metagenomics and metatranscriptomics.
Evaluation of Periodontitis-Related Systemic Diseases With Metagenomics
In recent years, a growing number of studies have suggested that periodontal pathogens are closely related to the occurrence and development of various systemic diseases, including cardiovascular diseases, diabetes, RA, Alzheimer’s disease, respiratory tract infection, and cancer (Bui et al., 2019). The association between periodontal microbial communities and periodontitis-related systemic diseases detected with metagenomics and metatranscriptomics is summarized in Table 3. The whole metagenomic shotgun sequencing approach has been used to analyze subgingival plaque samples from 4 groups based on the presence/absence of type 2 diabetes and moderate-to-severe periodontitis. The existence of diabetes and/or periodontitis markedly reduced the abundance and diversity of subgingival microorganisms, and an increase in Anaerolineaceae bacterium abundance was observed in the patients with both type 2 diabetes and periodontitis (Farina et al., 2019). Saeb et al. (2019) compared the microbial diversity and composition in normoglycemic, impaired glucose tolerance, and diabetes conditions by an ion 16STM metagenomic sequencing approach. The biological and phylogenetic diversity of oral microbial communities in patients with diabetes and prediabetes was significantly reduced compared with those in patients with normoglycemia. In addition, there was a rise in pathogenic components in the hyperglycemic microbiota. The application of the metagenomic sequencing approach to gingival sulcus biofilms from patients affected by periodontitis and type 2 diabetes revealed that the amount of Sphingobacteriaceae bacteria in the biofilm was significantly reduced during periodontitis, and Sphingobacteriaceae plays an important role in regulating the sensitivity of cells to insulin, suggesting that periodontitis might significantly affect type 2 diabetes development (Babaev et al., 2017). The metagenomic shotgun sequencing approach was performed for a longitudinal analysis of the subgingival microbiome from patients with periodontitis with/without type 2 diabetes. The results showed that patients with type 2 diabetes were more susceptible to a transformation of the subgingival microbiota to a state of dysbiosis, which might be caused by impaired host metabolism and immune regulation. In addition, a set of microbial genes were found to be enriched in the pathways associated with periodontitis and in the pathways that might connect T2DM and periodontitis. These findings might contribute to a better understanding of the two-way relationship between periodontitis and diabetes (Shi et al., 2020). Metagenomics has also revealed a specific correlation between periodontitis and RA. Significant differences have been found in the subgingival microbial structure in periodontally healthy individuals with or without RA. The relative abundance of gram-negative anaerobes in the subgingival microbial community has been found to be increased in RA, manifesting a state of biological disorder. Arachidonic acid and ester lipid metabolism pathways were enriched in the dental plaque biofilm of RA patients. In addition, the periodontal pathogen Cryptobacterium curtum identified in RA generates large amounts of citrulline and subsequently promotes the generation of citrullinated RA autoantigens, which confirms a link between periodontitis and RA (Lopez-Oliva et al., 2018). A longitudinal study investigated fecal, tooth, and salivary samples from individuals with RA and healthy controls by using metagenomic shotgun sequencing. The results revealed that the intestinal and oral microbiomes were consistent, which indicated an overlap of species abundance and function at different body parts. Moreover, the dysbiosis of the oral flora in RA patients was partially reversed after RA treatment. Functionally, the transport and metabolism of iron, sulfur, zinc, and arginine were altered in the microbiota of RA patients. These findings provide novel insights into specific alterations in the intestinal and oral microbiomes of RA patients and suggest a promising method for diagnosis and prognosis prediction based on oral microbiome composition (Zhang et al., 2015). Metagenomic sequencing of supra-gingival dental plaque from patients with Alzheimer’s disease (AD) and healthy controls demonstrated that the microbial diversity was lower in AD. In addition, the numbers of Lactobacillales, Streptococcaceae, and Firmicutes/Bacteroidetes were significantly increased, whereas Fusobacterium and Proteobacteria were significantly decreased in patients with AD, indicating that detecting the altered oral microbiota might contribute to the diagnosis of AD in the early stages (Wu et al., 2021). Periodontitis induced-systemic inflammation is a risk factor of cardiovascular disease. Metagenomic shotgun sequencing revealed that the composition of subgingival microbiome varied significantly between severe and mild periodontitis. In addition, the bacteria and metabolic pathways more abundant in severe PD was strongly associated with systemic inflammation, supporting the indirect inflammatory mechanism that the oral microbiome produces inflammatory mediators in the systemic circulation (Plachokova et al., 2021). Metagenomic sequencing of bacterial biofilms revealed that periodontal pathogens Porphyromonas gingivalis, Fusobacterium nucleatum, Tannerella forsythia, and Treponema denticola were highly correlated with cytokine IL-1β and vascular flow post sodium nitroprusside treatment, indicating the direct link between the abundance of specific periodontal pathogens and CVD (Kumar P. K. V., 2017). Metagenomic sequencing was performed to compare the microbial communities between HPV+ and HPV− CP granulation-samples. The abundance of Capnocytophaga ochracea was increased in HPV + CP samples, while Porphyromonas endodontalis, Macellibacteroides fermentas, Treponema phagedenis, and Campylo-bacter rectus species were overexpressed in HPV- CP samples. In addition, the changes in species richness led to activate pathways favoring carcinogenesis, indicating that these species might serve as novel biomarkers for oral cancer (Chowdhry et al., 2019). There is currently little information available on evaluating the connection between oral microbiome and periodontitis related systemic diseases with metatranscriptomics.
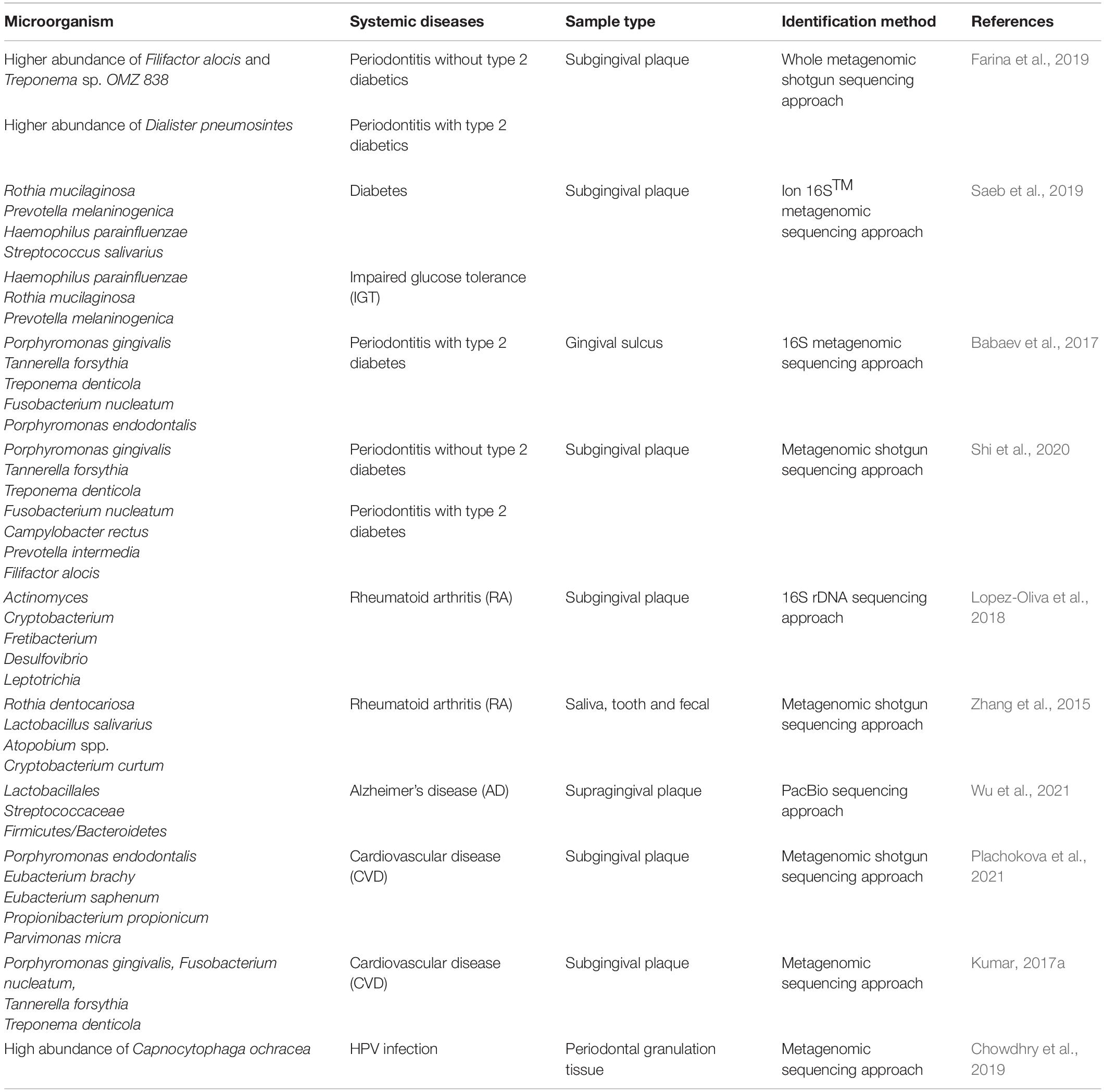
Table 3. Evaluation of periodontitis related systemic diseases with metagenomics and metatranscriptomics.
The Limitation of the Metagenomics and Metatranscriptomics
The consensus findings of metagenomic and metatranscriptomic studies in periodontitis and related systemic diseases have been summarized in Table 4. One major shortcoming of current studies is that the sample size is small, which might significantly affect the interpretation of the impact of periodontal treatment on the periodontal microbiome composition. In addition, the study design, research object, sampling method, therapeutic strategy, and duration of follow-up are very heterogeneous. Therefore, it is still unclear whether metagenomics and metatranscriptomics are more promising than traditional clinical indicators for evaluating the effectiveness of periodontal treatment (Feres et al., 2021). Additional well-designed randomized clinical trials with longer follow-up times, combined with multiomic analysis, are warranted to confirm whether metagenomic/metatranscriptomic changes can be used as outcome indicators and contribute to personalized periodontal therapy.
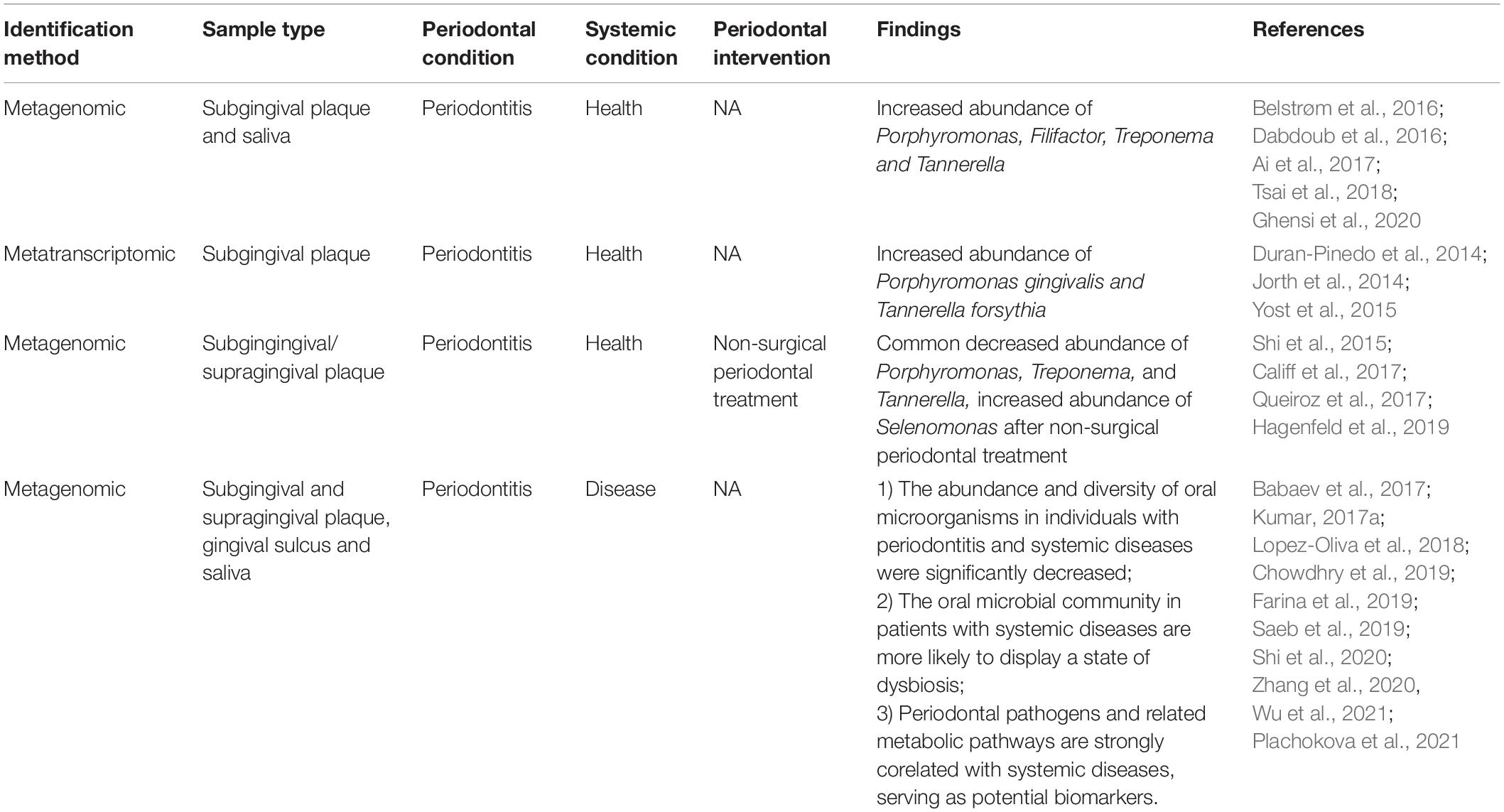
Table 4. Consensus findings of metagenomic and metatranscriptomic studies in periodontitis and related systemic diseases.
Monitoring the Onset and Progression of Periodontitis With Proteomics and Metabolomics
Proteomics and metabolomics are powerful tools for discovering novel and robust disease markers. In addition, the disease-specific proteins or metabolites can be effortless detected in gingival crevicular fluid (GCF), saliva and dental plaque due to their easy accessibility and availability. Therefore, proteomics and metabolomics have already shown great promise for monitoring the onset and progression of periodontitis. Proteomics analysis of saliva samples from patients with periodontitis, patients with dental caries and healthy individuals revealed that the levels of complement proteins and inflammatory mediators were increased in periodontitis and dental caries (Belstrøm et al., 2016). Similarly, the proteomics analysis showed that beta-2-glycoprotein I, α-fibrinogen, hemopexin, and plasminogen were abnormally expressed in the saliva samples from patients with periodontitis compared to the healthy controls (Orti et al., 2018). Bostanci et al. (2018) compared the protein profiles from unstimulated saliva samples of periodontitis patients, gingivitis patients and healthy subjects. The results demonstrated that metalloproteinase-9, ras-related protein-1 and actin-related protein 2/3 complex subunit 5 were significantly upregulated, while clusterin and deleted in malignant brain tumors 1 were downregulated in the periodontitis group compared to the control group. Interestingly, by comparing the proteomic profiles of pre- and post-treatment GCF samples, a robust protein signature including azurocidin, lysozyme C, myosin 9, and smooth muscle actin was built up for determining the clinical endpoints for CP (Guzman et al., 2018). Similar to proteomics, metabolomics has also been widely used for the diagnosis and prognosis prediction of periodontitis. Metabolomics analysis of GCF samples of patients with general chronic periodontitis and healthy controls indicated that the levels of citramalic acid and N-carbamylglutamate showed good performance for diagnosis of general chronic periodontitis (Pei et al., 2020). The metabolomic profiles of serum and GCF samples varied significantly between the patients with aggressive periodontitis and healthy subjects. Specifically, the levels of urea and allo-inositol were increased, while glutathione, 2,5-dihydroxybenzaldehyde, adipic acid and 2-deoxyguanosine were decreased in the serum samples from patients with aggressive periodontitis. For the GCF samples, the levels of noradrenaline, uridine, α-tocopherol, dehydroascorbic acid, xanthine, galactose, glucose-1-phosphate, and ribulose-5-phosphate were higher in patients with aggressive periodontitis, while thymidine, glutathione and ribose-5-phosphate were lower in the disease group (Chen et al., 2018). By integrating 16S rRNA metagenomic sequencing and metabolomics, many abnormally expressed metabolites were found to be positively correlated with periodontal pathogens such as Porphyromonas, Prevotella, and Fusobacterium species (Na et al., 2021). Similarly, a strong correlation was found between lipid mediators and subgingival microbiome profile, suggesting that inflammation induced by lipid mediators significantly affected the microbial composition in periodontitis (Lee et al., 2021). Metabolomics might also be used for evaluating the therapeutic efficacy. The salivary metabolomic profile of patients with generalized periodontitis was markedly altered by non-surgical periodontal therapy. Interestingly, many important metabolites returned to normal levels following treatment (Citterio et al., 2020). Interestingly, a recent multiomics study showed that the levels of hosphatidylcholines, plasmenyl-phosphatidylcholines, ceramides, and host proteins related to actin filament rearrangement were increased in the dental plaques from patients with periodontal diseases compared to the healthy controls (Overmyer et al., 2021). Therefore, efficiently integrating proteomics/metabolomics with metagenomics/metatranscriptomics might provide a more comprehensive understanding of the interaction between oral microbiome and periodontitis.
Future Perspective and Conclusion
Collectively, the composition and structure of periodontal microbial communities based on different levels of periodontal microecological bacterial taxonomy as well as the functional activity characteristics can be determined with metagenomics and metatranscriptomics. In addition, exploring the potential correlation between the dynamic changes in the functional behaviors of the periodontal microbiome and the progression and outcome of periodontitis provides valuable information for developing effective prevention and treatment strategies. However, a high degree of host interference, low sensitivity in detecting low-abundance genes, and high detection cost are the limitations of metagenomics. Furthermore, metatranscriptomics is not able to detect the final metabolites of the microbial communities. Therefore, more powerful evidence-based experimental studies are needed. Combining proteomic and metabolomic analyses is vital to reflect the functional proteins and metabolites produced by the microbiome under different conditions. The integration of various microbial detection methodologies will contribute significantly to our understanding of the complex subgingival microbial community and periodontitis pathogenesis.
Author Contributions
SH and LC: conceptualization and funding acquisition. YH, XZ, SH, and LC: writing–original draft and editing. All authors contributed to the article and approved the submitted version.
Funding
This work was supported by the National Natural Science Foundation of China (No. 81901006).
Conflict of Interest
The authors declare that the research was conducted in the absence of any commercial or financial relationships that could be construed as a potential conflict of interest.
Publisher’s Note
All claims expressed in this article are solely those of the authors and do not necessarily represent those of their affiliated organizations, or those of the publisher, the editors and the reviewers. Any product that may be evaluated in this article, or claim that may be made by its manufacturer, is not guaranteed or endorsed by the publisher.
References
Aguiar-Pulido, V., Huang, W., Suarez-Ulloa, V., Cickovski, T., Mathee, K., and Narasimhan, G. (2016). Metagenomics, Metatranscriptomics, and Metabolomics Approaches for Microbiome Analysis. Evol Bioinform. 12, 5–16. doi: 10.4137/EBO.S36436
Ai, D., Huang, R., Wen, J., Li, C., Zhu, J., and Xia, L. C. (2017). Integrated metagenomic data analysis demonstrates that a loss of diversity in oral microbiota is associated with periodontitis. BMC Genomics 18:32545. doi: 10.1186/s12864-016-3254-5
Altabtbaei, K. (2016). Metagenomic Analysis of Periodontal Bacteria Associated With Generalized Aggressive Periodontitis. dissertation/master’s thesis. Ohio, IL: The Ohio State University..
Altabtbaei, K., Maney, P., Ganesan, S. M., Dabdoub, S. M., and Kumar, P. S. (2021). Anna Karenina and the subgingival microbiome associated with periodontitis. Microbiome 9:97. doi: 10.1186/s40168-021-01056-3
Babaev, E., Balmasova, I., Mkrtumyan, A., Kostryukova, S., Vakhitova, E., Ilina, E., et al. (2017). Metagenomic analysis of gingival sulcus microbiota and pathogenesis of periodontitis associated with Type 2 diabetes mellitus. Bull. Exp. Biol. Med. 163, 718–721. doi: 10.1007/s10517-017-3888-6
Belda-Ferre, P., Alcaraz, L. D., Cabrera-Rubio, R., Romero, H., Simon-Soro, A., Pignatelli, M., et al. (2012). The oral metagenome in health and disease. ISME J. 6, 46–56. doi: 10.1038/ismej.2011.85
Belstrøm, D., Constancias, F., Liu, Y., Yang, L., Drautz-Moses, D. I., Schuster, S. C., et al. (2017). Metagenomic and metatranscriptomic analysis of saliva reveals disease-associated microbiota in patients with periodontitis and dental caries. NPJ Biofilms Microbiom. 3, 1–8. doi: 10.1038/s41522-017-0031-4
Belstrøm, D., Jersie-Christensen, R. R., Lyon, D., Damgaard, C., Jensen, L. J., Holmstrup, P., et al. (2016). Metaproteomics of saliva identifies human protein markers specific for individuals with periodontitis and dental caries compared to orally healthy controls. PeerJ 4:e2433. doi: 10.7717/peerj.2433
Bostanci, N., Selevsek, N., Wolski, W., Grossmann, J., Bao, K., Wahlander, A., et al. (2018). Targeted proteomics guided by label-free quantitative proteome analysis in saliva reveal transition signatures from health to periodontal disease. Mol. Cell Proteomics 17, 1392–1409. doi: 10.1074/mcp.RA118.000718
Bui, F. Q., Almeida-Da-Silva, C. L. C., Huynh, B., Trinh, A., and Ojcius, D. M. (2019). Association between periodontal pathogens and systemic disease. Biomed. J. 42, 27–35. doi: 10.1016/j.bj.2018.12.001
Califf, K. J., Schwarzberg-Lipson, K., Garg, N., Gibbons, S. M., Caporaso, J. G., Slots, J. R., et al. (2017). Multi-omics Analysis of Periodontal Pocket Microbial Communities Pre- and Posttreatment. mSystems 2, 00016–00017.
Chen, H., Zhou, W., Liao, Y., Hu, S., Chen, T., and Song, Z. (2018). Analysis of metabolic profiles of generalized aggressive periodontitis. J. Periodontal Res. 53, 894–901. doi: 10.1111/jre.12579
Chowdhry, R., Singh, N., Sahu, D. K., Tripathi, R. K., and Kant, R. (2019). Dysbiosis and variation in predicted functions of the granulation tissue microbiome in hpv positive and negative severe chronic Periodontitis. Biomed. Res. Int. 2019, 1–12. doi: 10.1155/2019/8163591
Citterio, F., Romano, F., Meoni, G., Iaderosa, G., Grossi, S., Sobrero, A., et al. (2020). Changes in the salivary metabolic profile of generalized periodontitis patients after non-surgical periodontal therapy: a metabolomic analysis using nuclear magnetic resonance spectroscopy. J. Clin. Med. 9:3977.
Curtis, M. A., Diaz, P. I., and Van Dyke, T. E. (2020). The role of the microbiota in periodontal disease. Periodontol 2000, 14–25. doi: 10.1111/prd.12296
Dabdoub, S. M., Ganesan, S. M., and Kumar, P. S. (2016). Comparative metagenomics reveals taxonomically idiosyncratic yet functionally congruent communities in periodontitis. Sci. Rep. 6:38993. doi: 10.1038/srep38993
Davis, N. M., Proctor, D. M., Holmes, S. P., Relman, D. A., and Callahan, B. J. (2018). Simple statistical identification and removal of contaminant sequences in marker-gene and metagenomics data. Microbiome 6, 1–14. doi: 10.1186/s40168-018-0605-2
Dewhirst, F. E., Chen, T., Izard, J., Paster, B. J., Tanner, A. C. R., Yu, W. H., et al. (2010). The Human Oral Microbiome. J. Bact. 192, 5002–5017.
Duran-Pinedo, A. E., Chen, T., Teles, R., Starr, J. R., Wang, X., Krishnan, K., et al. (2014). Community-wide transcriptome of the oral microbiome in subjects with and without periodontitis. ISME J. 8:1659. doi: 10.1038/ismej.2014.23
Eke, P. I., Borgnakke, W. S., and Genco, R. J. (2020). Recent epidemiologic trends in periodontitis in the USA. Periodontol 82, 257–267.
Escapa, I. F., Chen, T., Huang, Y., Gajare, P., Dewhirst, F. E., Lemon, K. P., et al. (2018). New Insights into Human Nostril Microbiome from the Expanded Human Oral Microbiome Database (eHOMD): a Resource for the Microbiome of the Human Aerodigestive Tract. mSystems 3, e118–e187. doi: 10.1128/mSystems.00187-18
Ezzo, P. J., and Cutler, C. W. (2003). Microorganisms as risk indicators for periodontal disease. Periodontol 2000, 24–35. doi: 10.1046/j.0906-6713.2003.03203.x
Farina, R., Severi, M., Carrieri, A., Miotto, E., and Scapoli, C. (2019). Whole metagenomic shotgun sequencing of the subgingival microbiome of diabetics and non-diabetics with different periodontal conditions. Arch. Oral Biol. 104, 13–23. doi: 10.1016/j.archoralbio.2019.05.025
Faust, K., Sathirapongsasuti, J. F., Izard, J., Segata, N., and Huttenhower, C. (2012). Microbial Co-occurrence Relationships in the Human Microbiome. PLoS Comput. Biol. 8:e1002606. doi: 10.1371/journal.pcbi.1002606
Feres, M., Retamal Valdes, B., Goncalves, C., Cristina Figueiredo, L., and Teles, F. (2021). Did Omics change periodontal therapy? Periodontol 85, 182–209. doi: 10.1111/prd.12358
Frencken, J. E., Sharma, P., Stenhouse, L., Green, D., Laverty, D., and Dietrich, T. (2017). Global epidemiology of dental caries and severe periodontitis - a comprehensive review. J. Clin. Periodontol 44, S94–S105.
Ghensi, P., Manghi, P., Zolfo, M., Armanini, F., Pasolli, E., Bolzan, M., et al. (2020). Strong oral plaque microbiome signatures for dental implant diseases identified by strain-resolution metagenomics. NPJ Biofilm Microbio. 6, 1–12. doi: 10.1038/s41522-020-00155-7
Guzman, Y. A., Sakellari, D., Papadimitriou, K., and Floudas, C. A. (2018). High-throughput proteomic analysis of candidate biomarker changes in gingival crevicular fluid after treatment of chronic periodontitis. J. Clin. Period. 53, 853–860. doi: 10.1111/jre.12575
Hagenfeld, D., Prior, K., Harks, I., JockelSchneider, Y., May, T. W., Harmsen, D., et al. (2019). No differences in microbiome changes between anti-adhesive and antibacterial ingredients in toothpastes during periodontal therapy. J. Periodon. Res. 54, 435–443. doi: 10.1111/jre.12645
Handelsman, J. (2004). Metagenomics: Application of Genomics to Uncultured Microorganisms. Microbiol. Mol. Biol. Rev. 68, 669–685.
Huttenhower, C., Gevers, D., Knight, R., Abubucker, S., Badger, J. H., Chinwalla, A. T., et al. (2012). Structure, function and diversity of the healthy human microbiome. Nature 486:207. doi: 10.1038/nature11234
Jiao, J., Jing, W., Si, Y., Feng, X., Tai, B., Hu, D., et al. (2021). The prevalence and severity of periodontal disease in mainland china: data from the fourth national oral health survey (2015–2016). J. Clin. Periodontol 48, 168–179. doi: 10.1111/jcpe.13396
Jorth, P., Turner, K. H., Gumus, P., Nizam, N., Buduneli, N., and Whiteley, M. (2014). Metatranscriptomics of the human oral microbiome during health and disease. mBio 5:e01012. doi: 10.1128/mBio.01012-14
Kilian, M., Chapple, I., Hannig, M., Marsh, P., Meuric, V., Pedersen, A., et al. (2016). The oral microbiome–an update for oral healthcare professionals. Br. Dent. J. 221, 657–666. doi: 10.1038/sj.bdj.2016.865
Kressirer, C. A., Tsute, C., Kristie, L. H., Jorge, F. L., Dewhirst, F. E., Tavares, M. A., et al. (2018). Functional profiles of coronal and dentin caries in children. J. Oral Microbiol. 10:1495976. doi: 10.1080/20002297.2018.1495976
Kumar, P. S. (2017). From focal sepsis to periodontal medicine: a century of exploring the role of the oral microbiome in systemic disease. J. Physiol. 595, 465–476. doi: 10.1113/JP272427
Kumar, P. K. V. (2017). Metagenomic Analysis Uncovers Strong Relationship between Periodontal Pathogens and Vascular Dysfunction in American Indian Population. dissertation/master’s thesis. San Diego, IL: San Diego State University.
Lasserre, J. F., Brecx, M. C., and Toma, S. (2018). Oral microbes, biofilms and their role in periodontal and peri-implant diseases. Materials 11:1802. doi: 10.3390/ma11101802
Lee, C. T., Li, R., Zhu, L., Tribble, G. D., and Dyke, T. (2021). Subgingival Microbiome and Specialized pro-resolving lipid mediator pathway profiles are correlated in periodontal inflammation. Front. Immunol. 12:691216.
Lopez-Oliva, I., Paropkari, A. D., Saraswat, S., Serban, S., Yonel, Z., Sharma, P., et al. (2018). Dysbiotic subgingival microbial communities in periodontally healthy patients with rheumatoid arthritis. Arthritis Rheumatol. 70, 1008–1013. doi: 10.1002/art.40485
Lu, H., He, L., Xu, J., Song, W., Feng, X., Zhao, Y., et al. (2020). Well-maintained patients with a history of periodontitis still harbor a more disbiotic microbiome than health. J. Periodontol 91, 1584–1594. doi: 10.1002/JPER.19-0498
Manoil, D., Al-Manei, K., and Belibasakis, G. N. (2020). A Systematic review of the root canal microbiota associated with apical periodontitis: lessons from next-generation sequencing. Proteomics Clin. Appl. 14, 1–17.
Methé, B. A., Nelson, K. E., Pop, M., Creasy, H. H., Giglio, M. G., Huttenhower, C., et al. (2012). A framework for human microbiome research. Nature 486:215. doi: 10.1038/nature11209
Na, H. S., Kim, S., Kim, S., Yu, Y., Kim, S. Y., Kim, H.-J., et al. (2021). Molecular subgroup of periodontitis revealed by integrated analysis of the microbiome and metabolome in a cross-sectional observational study. J. Oral Microbiol. 13:1902707. doi: 10.1080/20002297.2021.1902707
Nazir, M. A. (2017). Prevalence of periodontal disease, its association with systemic diseases and prevention. Int. J. Health Sci. 11:72.
Orti, V., Mertens, B., Vialaret, J., Gibert, P., Relaño-Ginés, A., Lehmann, S., et al. (2018). Data from a targeted proteomics approach to discover biomarkers in saliva for the clinical diagnosis of periodontitis. Data Brief 18, 294–299.
Overmyer, K. A., Rhoads, T. W., Merrill, A. E., Ye, Z., Westphall, M. S., Acharya, A., et al. (2021). Proteomics, lipidomics, metabolomics and 16S DNA sequencing of dental plaque from patients with diabetes and periodontal disease. Mol. Cell Proteomics 2021:100126. doi: 10.1016/j.mcpro.2021.100126
Pei, J., Li, F., Xie, Y., Liu, J., Yu, T., and Feng, X. (2020). Microbial and metabolomic analysis of gingival crevicular fluid in general chronic periodontitis patients: lessons for a predictive, preventive, and personalized medical approach. EPMA J. 11, 197–215. doi: 10.1007/s13167-020-00202-5
Plachokova, A. S., Andreu-Sánchez, S., Noz, M. P., Fu, J., and Riksen, N. P. (2021). Oral Microbiome in Relation to Periodontitis Severity and Systemic Inflammation. Int. J. Mol. Sci. 22:5876. doi: 10.3390/ijms22115876
Queiroz, L. A., Casarin, R. C., Dabdoub, S. M., Tatakis, D. N., Sallum, E. A., and Kumar, P. S. (2017). Furcation therapy with enamel matrix derivative: Effects on the subgingival microbiome. J. Periodontol 88, 617–625.
Quince, C., Walker, A. W., Simpson, J. T., Loman, N. J., and Segata, N. (2017). Shotgun metagenomics, from sampling to analysis. Nat. Biotechnol. 35, 833–844. doi: 10.1038/nbt.3935
Saeb, A. T., Al-Rubeaan, K. A., Aldosary, K., Raja, G. U., Mani, B., Abouelhoda, M., et al. (2019). Relative reduction of biological and phylogenetic diversity of the oral microbiota of diabetes and pre-diabetes patients. Microb. Pathog. 128, 215–229. doi: 10.1016/j.micpath.2019.01.009
Shi, B., Chang, M., Martin, J., Mitreva, M., Lux, R., Klokkevold, P., et al. (2015). Dynamic Changes in the subgingival microbiome and their potential for diagnosis and prognosis of periodontitis. mBio 6:e01926.
Shi, B., Lux, R., Klokkevold, P., Chang, M., Barnard, E., Haake, S., et al. (2020). The subgingival microbiome associated with periodontitis in type 2 diabetes mellitus. ISME J. 14, 519–530. doi: 10.1038/s41396-019-0544-3
Slatko, B. E., Gardner, A. F., and Ausubel, F. M. (2018). Overview of Next-Generation Sequencing Technologies. Curr. Protoc. Mol. Biol. 122:e59. doi: 10.1002/cpmb.59
Solbiati, J., and Frias-Lopez, J. (2018). Metatranscriptome of the oral microbiome in health and disease. J. Dent. Res. 97, 492–500. doi: 10.1177/0022034518761644
Stavros, B., Gili, Z.-S., and Eran, E. (2016). Use of Metatranscriptomics in Microbiome Research. Bioinform. Biol. Insights 10, 19–25. doi: 10.4137/BBI.S34610
Teles, F., Wang, Y., Hajishengallis, G., Hasturk, H., and Marchesan, J. T. (2021). Impact of systemic factors in shaping the periodontal microbiome. Periodontol 2021, 126–160. doi: 10.1111/prd.12356
Torres, P. J., Thompson, J., McLean, J. S., Kelley, S. T., and Edlund, A. (2019). Discovery of a novel periodontal disease-associated bacterium. Microb. Ecol. 77, 267–276. doi: 10.1007/s00248-018-1200-6
Tsai, C.-Y., Tang, C. Y., Tan, T.-S., Chen, K.-H., Liao, K.-H., and Liou, M.-L. (2018). Subgingival microbiota in individuals with severe chronic periodontitis. J. Microbiol. Immunol. Infect. 51, 226–234. doi: 10.1016/j.jmii.2016.04.007
Tsute, C., WenHan, Y., Jacques, I., V, B. O., Abirami, L., and E, D. F. (2010). The Human Oral Microbiome Database: a web accessible resource for investigating oral microbe taxonomic and genomic information. Database 2010, 1–10. doi: 10.1093/database/baq013
Vos, T., Abajobir, A. A., Abate, K. H., Abbafati, C., Abbas, K. M., Abd-Allah, F., et al. (2017). Global, regional, and national incidence, prevalence, and years lived with disability for 328 diseases and injuries for 195 countries, 1990–2016: a systematic analysis for the Global Burden of Disease Study 2016. Lancet 390, 1211–1259. doi: 10.1016/S0140-6736(17)32154-2
Wang, J., and Jia, H. (2016). Metagenome-wide association studies: fine-mining the microbiome. Nat. Rev. Microbiol. 14, 508–522. doi: 10.1038/nrmicro.2016.83
Wang, J., Qi, J., Zhao, H., He, S., Zhang, Y., Wei, S., et al. (2013). Metagenomic sequencing reveals microbiota and its functional potential associated with periodontal disease. Sci. Rep. 3:1843.
Wu, Y.-F., Lee, W.-F., Salamanca, E., Yao, W.-L., Su, J.-N., Wang, S.-Y., et al. (2021). Oral microbiota changes in elderly patients, an indicator of alzheimer’s disease. Int. J. Environ. Res. Public Health 18:4211. doi: 10.3390/ijerph18084211
Yost, S., Duran-Pinedo, A. E., Teles, R., Krishnan, K., and Frias-Lopez, J. (2015). Functional signatures of oral dysbiosis during periodontitis progression revealed by microbial metatranscriptome analysis. Genome Med. 7, 1–19.
Yu, K., Yi, S., Li, B., Guo, F., Peng, X., Wang, Z., et al. (2019). An integrated meta-omics approach reveals substrates involved in synergistic interactions in a bisphenol A (BPA)-degrading microbial community. Microbiome 7, 1–13. doi: 10.1186/s40168-019-0634-5
Zhang, X., Zhang, D., Jia, H., Feng, Q., Wang, D., Liang, D., et al. (2015). The oral and gut microbiomes are perturbed in rheumatoid arthritis and partly normalized after treatment. Nat. Med. 21, 895–905. doi: 10.1038/nm.3914
Keywords: metagenomics, metatranscriptomics, periodontitis, microbial community, systemic diseases
Citation: Huang Y, Zhao X, Cui L and Huang S (2021) Metagenomic and Metatranscriptomic Insight Into Oral Biofilms in Periodontitis and Related Systemic Diseases. Front. Microbiol. 12:728585. doi: 10.3389/fmicb.2021.728585
Received: 21 June 2021; Accepted: 21 September 2021;
Published: 13 October 2021.
Edited by:
Axel Cloeckaert, Institut National de Recherche pour l’Agriculture, l’Alimentation et l’Environnement (INRAE), FranceReviewed by:
Eija Könönen, University of Turku, FinlandGajender Aleti, University of California, San Diego, United States
Xuesong He, The Forsyth Institute, United States
Carina Maciel Silva-Boghossian, Federal University of Rio de Janeiro, Brazil
Copyright © 2021 Huang, Zhao, Cui and Huang. This is an open-access article distributed under the terms of the Creative Commons Attribution License (CC BY). The use, distribution or reproduction in other forums is permitted, provided the original author(s) and the copyright owner(s) are credited and that the original publication in this journal is cited, in accordance with accepted academic practice. No use, distribution or reproduction is permitted which does not comply with these terms.
*Correspondence: Li Cui, enN1Y2xsakB1Y2xhLmVkdQ==; Shaohong Huang, aHNoLmNoaW5hQHRvbS5jb20=
†These authors have contributed equally to this work