- 1Department of Molecular Biology and Biochemistry, University of California, Irvine, Irvine, CA, United States
- 2Laboratory of Virology, Division of Intramural Research, National Institute of Allergy and Infectious Diseases, NIH, Rocky Mountain Laboratories, Hamilton, MT, United States
- 3Center for Virus Research, University of California, Irvine, Irvine, CA, United States
- 4Institute for Immunology, University of California, Irvine, Irvine, CA, United States
The 2013–2016 West Africa Ebola virus (EBOV) epidemic caused by the EBOV-Makona isolate is the largest and longest recorded to date. It incurred over 28,000 infections and ∼11,000 deaths. Early in this epidemic, several mutations in viral glycoprotein (A82V), nucleoprotein (R111C), and polymerase L (D759G) emerged and stabilized. In vitro studies of these new EBOV-Makona isolates showed enhanced fitness and viral replication capacity. However, in vivo studies in mice and rhesus macaques did not provide any evidence of enhanced viral fitness or shedding. Infection with late isolates carrying or early isolates lacking (early) these mutations resulted in uniformly lethal disease in nonhuman primates (NHPs), albeit with slightly delayed kinetics with late isolates. The recent report of a possible reemergence of EBOV from a persistent infection in a survivor of the epidemic highlights the urgency for understanding the impact of genetic variation on EBOV pathogenesis. However, potential molecular differences in host responses remain unknown. To address this gap in knowledge, we conducted the first comparative analysis of the host responses to lethal infection with EBOV-Mayinga and EBOV-Makona isolates using bivariate, longitudinal, regression, and discrimination transcriptomic analyses. Our analysis shows a conserved core of differentially expressed genes (DEGs) involved in antiviral defense, immune cell activation, and inflammatory processes in response to EBOV-Makona and EBOV-Mayinga infections. Additionally, EBOV-Makona and EBOV-Mayinga infections could be discriminated based on the expression pattern of a small subset of genes. Transcriptional responses to EBOV-Makona isolates that emerged later during the epidemic, specifically those from Mali and Liberia, lacked signatures of profound lymphopenia and excessive inflammation seen following infection with EBOV-Mayinga and early EBOV-Makona isolate C07. Overall, these findings provide novel insight into the mechanisms underlying the lower case fatality rate (CFR) observed with EBOV-Makona compared to EBOV-Mayinga.
Introduction
Zaire Ebola virus (EBOV) is a single-stranded, negative-sense RNA virus and a member of the Filoviridae family that is responsible for Ebola virus disease (EVD; Sanchez et al., 1993; Bell et al., 2015). EVD is characterized by lymphopenia, excessive inflammation, thrombocytopenia, and disseminated intravascular coagulation, ultimately leading to multi-organ failure and a high case fatality rate (CFR, 40–90%) (Geisbert et al., 2003b; Feldmann and Geisbert, 2011). EBOV preferentially infects antigen presenting cells (APCs), notably monocytes, macrophages, and dendritic cells (DCs), that play a critical role in viral dissemination (Geisbert et al., 2003a; Menicucci et al., 2017). Moreover, infected monocytes are a major source of pro-inflammatory cytokines, which precipitate the development of coagulopathy and organ injury (Geisbert et al., 2000, 2003b; Gupta et al., 2001). In contrast, EBOV infection of DCs interferes with their maturation and ability to mobilize T cells, thwarting the development of cellular and humoral immunity (Marzi et al., 2013; Barrenas et al., 2015; Iampietro et al., 2017; Pleet et al., 2017, 2018).
Different strains of EBOV show a considerable diversity in pathogenicity, lethality, and disease progression rate despite sharing ∼97% genome similarity (Bell et al., 2015; Marzi et al., 2015, 2018; Wong et al., 2016; Menicucci et al., 2017; Versteeg et al., 2017, 2019; Madelain et al., 2018; McMullan et al., 2019; Pinski and Messaoudi, 2020). The 1976 outbreak of EBOV-Mayinga and the 1995 outbreak of EBOV-Kikwit resulted in ∼300 cases each and CFRs of ∼90% (Centers for Disease Control and Prevention, 1995a, b; Muyembe-Tamfum et al., 1999; Kuhn et al., 2013; Kaner and Schaack, 2016). In contrast, the 2013–2016 West African epidemic caused by the EBOV-Makona isolate resulted in over 28,000 reported cases and a CFR of ∼39% (Kaner and Schaack, 2016; Shoman et al., 2017). Furthermore, EBOV-Makona accumulated nonsynonymous mutations in the nucleoprotein (NP, R111C), glycoprotein (GP, A82V), and polymerase (L, D759G) that were associated with increased replication capacity and enhanced viral fitness in vitro that may explain accelerated transmission (Dietzel et al., 2017; Hoffmann et al., 2017; Ruedas et al., 2017, 2018; Ueda et al., 2017; Marzi et al., 2018; Wong et al., 2019). Specifically, mutations in GP, the viral receptor for entry into host cells, could enhance infectivity. However, studies in nonhuman primates (NHP), the gold standard animal model to study EBOV pathogenesis, showed comparable clinical disease, although infection with EBOV-Makona isolates that emerged late in the epidemic, as a group (Mali and Liberia), resulted in delayed time to euthanasia (Geisbert et al., 2003a, 2015; Marzi et al., 2018; Longet et al., 2020).
Recent studies have reported distinct transcriptional responses in NHPs infected with EBOV-Makona Guinea C07 (early isolate) or EBOV-Kikwit (Rivera and Messaoudi, 2016; Versteeg et al., 2019; Pinski et al., 2021a). However, comparison of the host transcriptional responses to early and late EBOV-Makona isolates and to the historical and highly lethal EBOV-Mayinga strain are absent. Understanding similarities and differences in host responses to EBOV isolates is essential for connecting changes in pathogenicity to viral evolution, and aiding the development of vaccines and antivirals to prevent and address future outbreaks. For instance, a 2021 outbreak is believed to be caused by the reemergence of persistent EBOV in a recovered survivor of the 2013–2016 epidemic (Centers for Disease Control and Prevention, 2021a). Transcriptional studies have proven instrumental in understanding the mechanisms of pathogenesis of filoviruses (Eisfeld et al., 2017; Menicucci et al., 2017, 2019; Versteeg et al., 2017; Kotliar et al., 2020; Baillet et al., 2021; Pinski et al., 2021a, b). Therefore, in this study, we leveraged access to historical RNA samples from a previous study (Marzi et al., 2018) that compared clinical parameters following infection with early and late EBOV-Makona isolates to address this question. Specifically, we compared the transcriptional response following infection with early and late EBOV-Makona isolates as well as EBOV-Mayinga in rhesus macaques. We report that infection with the different EBOV isolates induces a transcriptional response characterized by a shared core of genes that play a role in antiviral defense, inflammation, and cell activation, which reflects EVD pathology. Additionally, we identified 300 genes involved in host defense and the stress responses that were sufficient to distinguish EBOV infections from each other during peak disease. Finally, infection with late EBOV-Makona isolates lacked transcriptional indicators of lymphopenia and robust upregulation of genes that play a role in inflammation, which may in part explain the lower CFR observed during the 2013–2016 West African epidemic.
Materials and Methods
Cohorts and Study Design
Historical RNA samples collected from our previous study were used in this study (Marzi et al., 2018). In this previous study, four cohorts of rhesus macaques were infected intramuscularly with 1 × 103 focus forming units (FFUs) of EBOV-Mayinga (n = 5) or EBOV-Makona isolates: Guinea C07, Mali, or Liberia (n = 3 each). Whole blood (WB) samples were collected at 0, 2, 4, and 6 days post infection (DPI), and RNA was isolated from the WB of EBOV-Mayinga- and EBOV-Makona-infected NHPs using the QIAmp Viral RNA Kit (Qiagen) as previously described (Marzi et al., 2018). This prior work was performed in the maximum containment laboratory at the Rocky Mountain Laboratories (RML), Division of Intramural Research, National Institute of Allergy and Infectious Diseases, National Institutes of Health. RML is an AAALAC accredited institution. All procedures followed standard operating procedures (SOPs) approved by the RML Institutional Biosafety Committee (IBC). Animal work was performed in strict accordance with the recommendations described in the Guide for the Care and Use of Laboratory Animals of the National Institute of Health, the Office of Animal Welfare and the Animal Welfare Act, United States Department of Agriculture. The study was approved by the RML Animal Care and Use Committee (ACUC). Procedures were conducted in animals anesthetized by trained personnel under the supervision of veterinary staff. The humane endpoint criteria for euthanasia were specified and approved by the RML ACUC. All efforts were made to ameliorate animal welfare and minimize animal suffering in accordance with the Weatherall report on the use of NHPs in research1.
Library Preparation and Sequencing
RNA samples from DPI 0, 4, and 6 were used in the current study. Integrity and concentration of historical RNA samples were validated on the Agilent 2100 Bioanalyzer prior to library construction. rRNA was depleted from the samples using the NEBNext rRNA Depletion kit before cDNA library construction with the NEBNext Ultra II Directional RNA Library Prep Kit (Illumina). cDNA library quality and concentration were confirmed on the Agilent 2100 Bioanalyzer before sequencing on the Illumina HiSeq2500 or NovaSeq platforms.
Downstream Analysis and Bioinformatics
Raw sequences were trimmed to a minimum length of 75 bp and average Phred score of 30 using Trim Galore before alignment to the Macaca mulatta genome “Macaca_mulatta.Mmul_8.0.1.dna.toplevel.fa” using tophat. Genes were annotated with the Ensembl annotation files for M. mulatta (Macaca_mulatta.Mmul_8.0.1.97.gtf). Preliminary processing of RNA-Seq data was performed using the systemPipeR package available from Bioconductor (Tw and Girke, 2016).
Three approaches were used to identify differentially expressed genes (DEGs): (1) EdgeR, (2) STEM, and (3) MaSigPro (Ernst and Bar-Joseph, 2006; Robinson et al., 2010; Nueda et al., 2014). EdgeR package, which uses the Trimmed means of M-values (TMM), was used to identify DEGs, and counts were normalized using the reads per kilobase of exon per million (rpkm) (Mortazavi et al., 2008; Menicucci et al., 2017; Versteeg et al., 2019). DEGs were then filtered for only those encoding human protein-coding homologs with an average rpkm ≥ 5, FDR ≤ 0.05, and fold change ≤ −1 or ≥ 1. To identify longitudinal patterns of gene expression changes throughout infection, we used Short Time Series Expression Miner (STEM) software, which clusters genes both by significance and expression patterns (Ernst and Bar-Joseph, 2006). MaSigPro was used for regression analysis with a two-way forward regression strategy comparing the EBOV-Makona Mali, Liberia, and EBOV-Mayinga isolates to EBOV-Makona Guinea C07 (Conesa et al., 2006). Genes that contributed significantly to the most degree of variance in at least 16 different comparisons were fit into the model and were then clustered by temporal expression pattern. Sparse partial least squares discrimination analysis (sPLS-DA) was performed with the mixOmics R package for validation and classification (Rohart et al., 2017). Models were initially built with a minimum of 10 components and validated with five-fold cross-validation and 10 repetitions.
Functional enrichment was carried out using Metascape to identify gene ontology (GO) terms representing biological processes (Zhou et al., 2019). GO network plot was created with Cytoscape visualization software (Otasek et al., 2019; Zhou et al., 2019). All heatmaps, bubbleplots, and Venn diagrams were made and analyzed through R packages (R Core Team, 2015; Wickham, 2009). Digital cell quantification (DCQ) was performed using ImmQuant, a platform that uses a deconvolution algorithm to compare transcriptomic sequencing data to reference datasets in order to predict changes in cell frequencies (Abbas et al., 2005; Novershtern et al., 2011; Frishberg et al., 2016). Both DMAP and IRIS datasets were used.
Statistical Analysis
All statistical analyses were performed in GraphPad Prism (version 7). Genes with similar temporal expression profiles were determined using time course data (analyzed through STEM) and were grouped into clusters (analysis of clusters shown in Figures 2, 4). All clusters for each isolate were regenerated on one plot with multiple biological replicates of the DEGs found through STEM (Figures 2A, 4A). ImmQuant deconvolution data was analyzed using a repeated measure mixed effect model statistical design against 0 DPI, where numbers of samples were not equal between timepoints. Specifically, multiple comparison analyses were performed between subsequent days post infection (4 DPI and 6 DPI) and baseline (0 DPI) and indicated with asterisks (Figures 3, 6 and Supplementary Figure 3). For EBOV-Makona Mali and Liberia datasets, significance was additionally tested using a two-tailed T test for population changes between 0 DPI and both 4 + 6 DPI.
Data Availability
Sequencing data for rhesus macaques is available at BioProject PRJNA718880.
Results
Host Transcriptional Response to EBOV-Mayinga Highlights Dysregulation of Innate and Adaptive Immune Responses
No studies to date have examined the transcriptional response to EBOV-Mayinga, the first EBOV isolate identified in 1976 (CFR of ∼90%). Therefore, we leveraged access to historical WB RNA samples from our previous study to profile host responses at 0, 4, and 6 DPI (Marzi et al., 2018). Significant transcriptional changes were identified with a total of 985 and 1,721 DEGs detected 4 and 6 DPI, respectively, with a substantial overlap between the two time points (Figure 1A). Functional enrichment of these DEGs using Metascape showed that DEGs downregulated at 4 and 6 DPI enriched to GO terms related to adaptive immunity (Figure 1B) such as “thymus development” (e.g., AGER, IL7R, and ZAP70) and “T cell activation” (e.g., AKT1, BTLA, and TCF7) (Figure 1B and Supplementary Figure 1A) (Zhou et al., 2019). DEGs downregulated at 6 DPI enriched to “antigen processing and presentation of exogenous peptide antigen via MHC class II” (e.g., HLA-DMA, -DMB, and -DOB) and “B cell proliferation” (e.g., CD19, CD180, and PLCL2) (Figure 1B and Supplementary Figure 1A). In addition, DEGs downregulated at 6 DPI played a role in “DNA repair” (e.g., ATM, DNA2, and POLE2) and “cell cycle phase transition” (e.g., BUB1B, CDC27, and CKAP5) (Figure 1B).
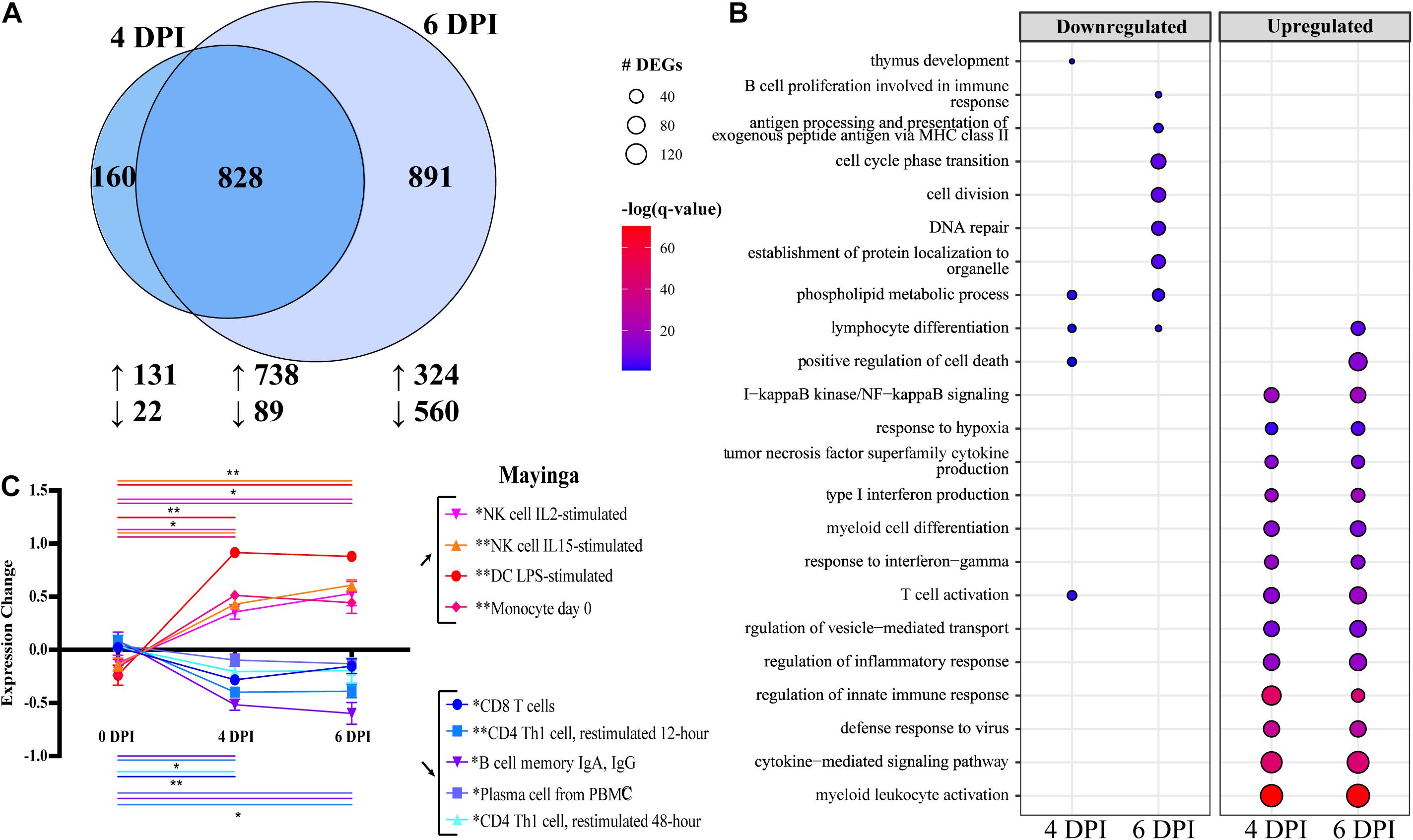
Figure 1. Differential gene expression analysis with EdgeR following EBOV-Mayinga infection in rhesus macaques shows robust immune activation and dysregulation. (A) Venn diagram of number of differentially expressed genes (DEGs) (FDR ≤ 0.05, fold change ≥ 1 or 1, rpkm 5; see section “Materials and Methods”) detected at 4 and 6 DPI following EBOV-Mayinga infection. The number of upregulated and downregulated DEGs is indicate with upward- and downward-pointing arrows, respectively. (B) Bubbleplot summarizing functional enrichment of DEGs up- and downregulated 4 and 6 DPI. Color intensity of each bubble represents the negative log of the FDR-adjusted p-value [–log(q-value)], and the relative size of each bubble represents the number of DEGs belonging to the specified gene ontology (GO) term. (C) Digital cell quantification analysis using DMAP and IRIS datasets. Lines are color-coded to match both the subset listed in the legend. Significance lines of which both ends denote the two time points of the two-way multiple comparisons significance testing. Asterisks in legend cell population denote overall significance (0–4 DPI, 4–6 DPI, and 0–6 DPI) through the mixed effects model test. *p < 0.05, **p < 0.01, ***p < 0.001.
In contrast to downregulated DEGs, upregulated DEGs detected at 4 and 6 DPI enriched predominantly to GO terms related to innate immunity and inflammation, notably “myeloid cell differentiation” (e.g., BATF2, MAFB, NFE2 < TLR2, TLR3, and RELB) (Figure 1B and Supplementary Figure 1B). DEGs enriching to “type I interferon (IFN) production” consisted primarily of IFN-stimulated genes (ISG; e.g., IFI16, IRF7, ISG15, and STAT1), as well as genes involved in detection and response to pathogenic nucleic acid (e.g., DHX58, DDX58, IRF7, and TBK1) (Supplementary Figure 1C). Finally, some upregulated DEGs at 4 and 6 DPI also enriched to “T cell activation” (e.g., CD274, IL2RA, LFNG, and TCIRG1) (Supplementary Figure 1D).
We next sought to correlate these transcriptional changes with alterations in immune cell frequencies and activation status. Since flow cytometry was not conducted in the earlier study and no PBMC were cryopreserved, we performed DCQ to predict changes in immune cell population frequencies based on our transcriptional data (Figure 1C). Using the IRIS immune cell database, transcriptional findings predicted sharp decreases in the frequencies of T and B cells (particularly antibody-secreting B cells), while the number of myeloid cells and natural killer (NK) cell populations was predicted to increase with disease progression (Figure 1C).
Given that bivariate analysis of the transcriptional changes at each DPI relative to baseline does not consider the dynamic, longitudinal patterns of gene expression, we next used Short Time-series Expression Miner (STEM) to identify clusters of genes, the expression of which changes in a similar manner over time (Figure 2) (Ernst and Bar-Joseph, 2006). We identified three clusters with genes in clusters 0 and 2 significantly upregulated throughout infection, while expression of genes in cluster 1 was slightly decreased 4 DPI before returning to baseline levels of expression at 6 DPI (cluster 1) (Figure 2A). Genes in cluster 0 (n = 2,303) enriched to GO terms related to the innate inflammatory response such as “myeloid leukocyte activation” (e.g., CD14, CD53, and CD55), “cytokine-mediated signaling pathway” (e.g., IFNAR2, IL15RA, JUNB, and LTB), “NIK/NF-kappaB signaling” (e.g., NFKB2, RELA, TRAF2, and TLR9), and “apoptotic signaling pathway” (e.g., CASP1, CASP4, and FAS) (Figure 2B). Genes also enriched to GO term “lymphocyte activation” and were related to both cellular (e.g., CD274, JAK3, TNFRSF14, and TREML2) and humoral (e.g., BCL6, LYN, and PRKCB) immunity (Figures 2B,C). Cluster 2 (n = 495) was comprised of genes that enriched to GO terms indicative of heightened inflammatory state such as “positive regulation of tumor necrosis factor production” (e.g., JAK2 and TNFRSF1A), “myeloid leukocyte activation” (e.g., FCER1G, NLRP3, and TLR4) (Figure 2D), and “defense response to virus” (e.g., BST2, AIM2, and HERC5) (Figure 2E). Lastly, the downregulated genes of cluster 1 (n = 495) were involved in metabolic and cellular processes such as “regulation of cell cycle process” (e.g., ANAPC2, CCND1, and CDC20) and “RNA catabolic process” (e.g., AGO1, LARP1, and PYM1) (Figures 2F,G).
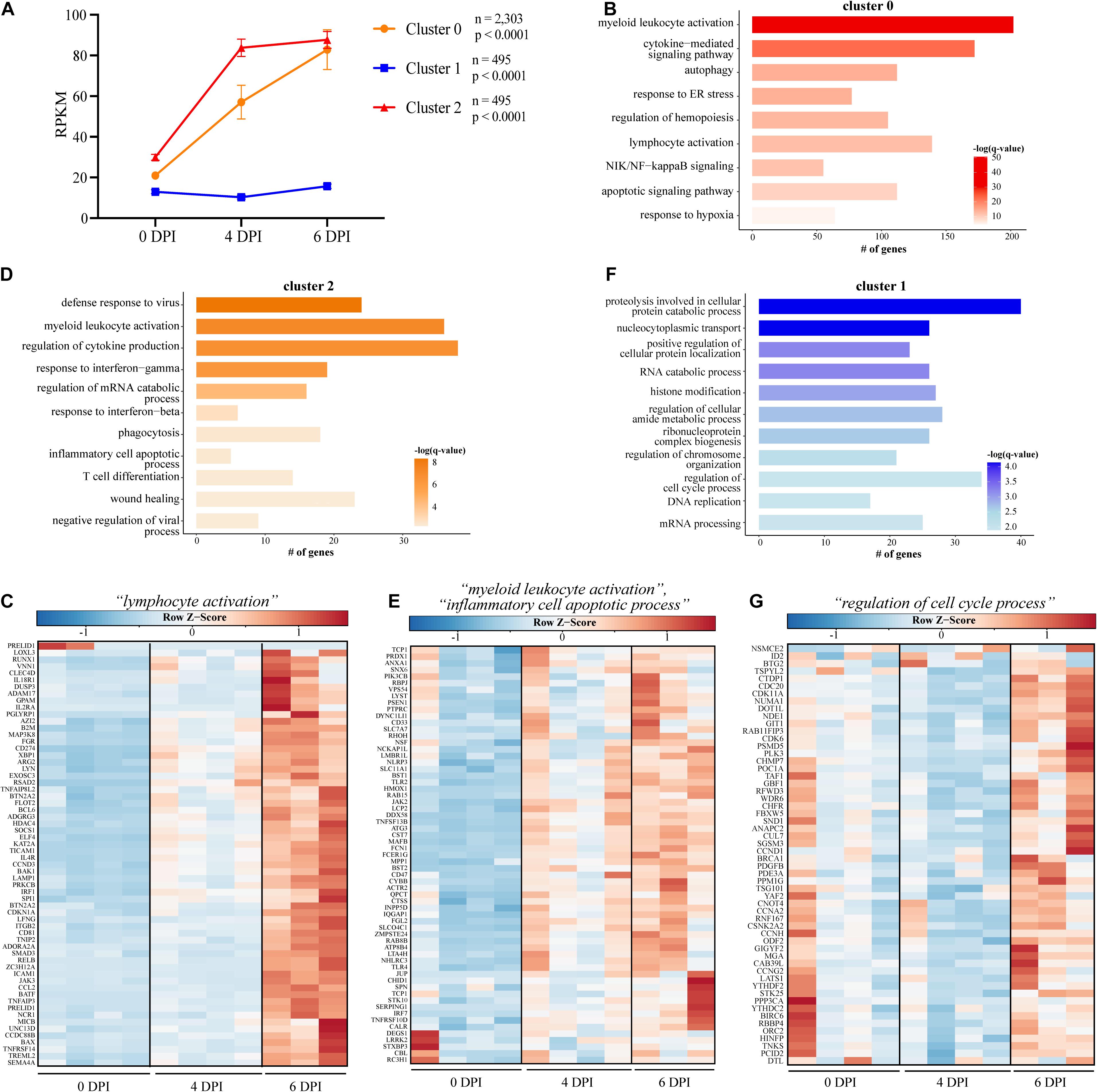
Figure 2. Short Time Series Expression Miner (STEM) analysis of gene expression data following EBOV-Mayinga infection. (A) Gene expression clusters identified by STEM with accompany number (n) of genes and p-value (p). GO term bar graphs representing functional enrichment of the genes from (B) cluster 0, (D) cluster 2, and (F) cluster 1. Horizontal bars represent the number of genes mapping to each GO term, while color intensity represents the –log(q-value) value of the corresponding GO term. Gene expression heatmaps depicting genes from GO term (C) “lymphocyte activation” in cluster 0, (E) “myeloid leukocyte activation” and “inflammatory cell apoptotic process” in cluster 2, and (G) “regulation of cell cycle process” from cluster 1. Each column represents one animal. Red represents upregulation; blue, downregulation. Range of colors is based on scaled and centered rpkm values of the represented DEGs.
Transcriptional Responses to Late EBOV-Makona Isolates Indicate Attenuated Inflammation and Lymphopenia
Next, we examined transcriptional changes following challenge with early (Guinea C07) and late (Liberia and Mali) EBOV-Makona isolates (Figure 3). Disease progression and clinical data for these animals were reported in our previous study and indicate uniform lethal infection, albeit slightly delayed following infection with late isolates in rhesus macaques (Marzi et al., 2018). Comparative bivariate transcriptional analysis revealed striking similarities in the overall magnitude and character of the transcriptional response to early and late isolates (Figures 3A–C). The majority of DEGs detected 4 DPI and 6 DPI following challenge with each isolate overlapped, with a greater number of DEGs detected 6 DPI compared to 4 DPI, and most DEGs were upregulated (Figures 3B–D). Liberia exhibited a particularly lower number of DEGs (∼500) at 4 DPI compared to other isolates (>1,000 DEGs) (Figure 3B). These downregulated DEGs enriched to GO terms related to gene expression, including “translation,” “mRNA processing,” and “protein targeting to ER” (Figure 3E). On the other hand, DEGs downregulated by 6 DPI played a role in cell cycle (C07, Mali), adaptive immunity (C07, Liberia, Mali), translation (Liberia), and apoptosis (C07) (Figure 3E). Functional enrichment of upregulated DEGs at 4 and 6 DPI is consistent with a heightened inflammatory response with over-representation of GO terms related to EVD pathology (e.g., “defense response to virus,” “positive regulation of cell death,” and “regulation of cytokine production”) (Figure 3E).
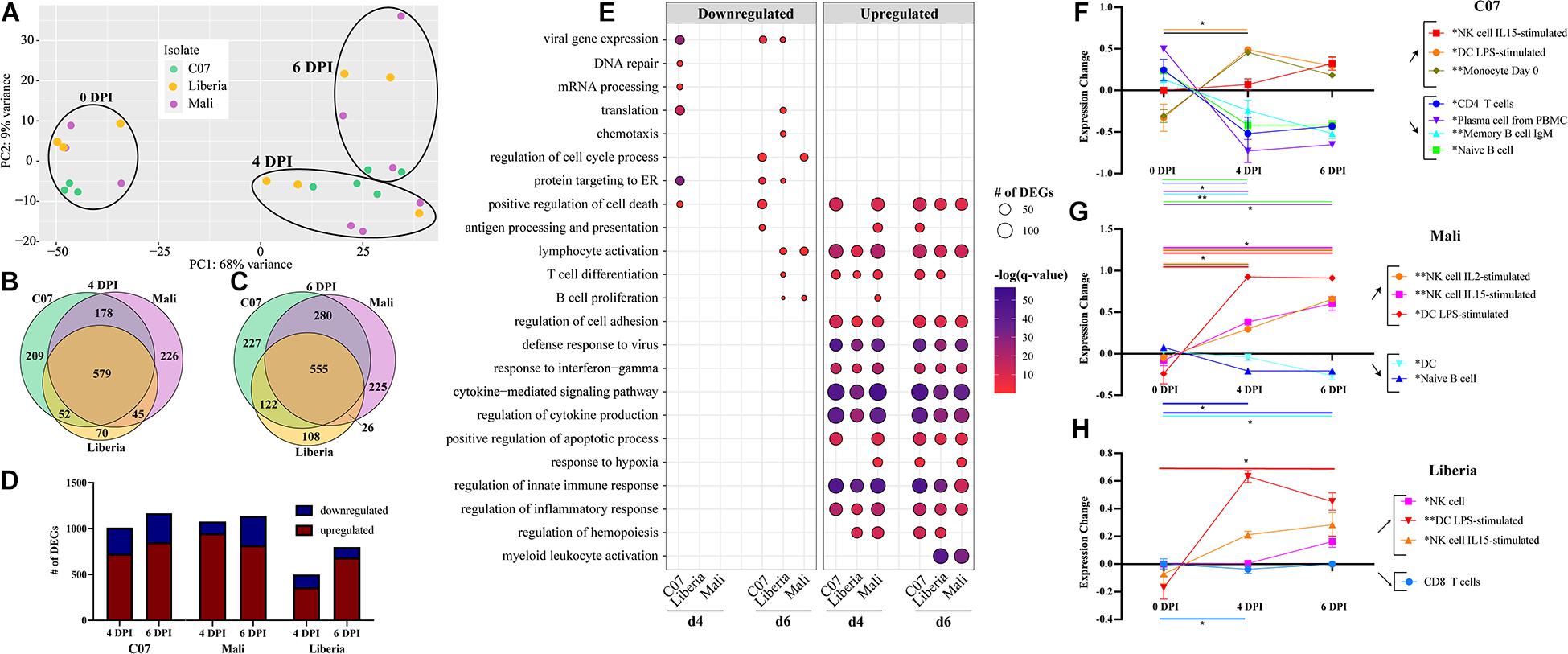
Figure 3. Host response comparison between early and late isolates of EBOV-Makona. (A) Principal component analysis of normalized transcript counts at 0, 4, and 6 DPI for EBOV-Makona isolates. Venn diagrams of number of differentially expressed genes (DEGs) (FDR 0.05, fold change ≥ 1 or 1, RPKM 5) identified through EdgeR following infection with either EBOV-Makona Guinea C07, Liberia, or Mali at (B) 4 DPI and (C) 6 DPI. (D) Bar graph of DEGs detected at 4 and 6 DPI for each isolate. (E) Bubbleplot presenting functional enrichment of upregulated and downregulated DEGs detected in each isolate at 4 and 6 DPI. The size of the bubble represents the number of DEGs, while the color represents the –log(q-value). ImmQuant analysis using DMAP and IRIS datasets for (F) C07, (G) Mali, and (H) Liberia. Lines are color-coded on the graph to match both the subset listed in the legend and the significance lines, of which both ends denote the two time points of the two-way multiple comparisons significance testing. A “*” over a legend denotes overall significance through the mixed effects model test. *p < 0.05, **p < 0.01, ***p < 0.001.
Given that DEGs detected 4 and 6 DPI following infection with all three isolates enriched to similar GO terms, we next examined the overlap between those sets of DEGs (Supplementary Figure 2). DEGs that enriched to GO term “positive regulation of cell death” were upregulated throughout infection and showed significant overlap (Supplementary Figure 2A). These genes played a role in apoptosis (e.g., CASP1, FAS, and TNFRSF1A) and inflammation (e.g., IL6, NLRP3, TLR4, and TNF) (Supplementary Figure 2A). Similarly, many upregulated DEGs enriching to “defense response to virus” were shared between the isolates and encoded nucleic acid sensors (e.g., DDX58, DDX60, and OAS1), components of the IFN signaling pathway (e.g., STAT1, STAT2, and TBK1), and ISGs (e.g., AIM2, IFI16, and ISG15) (Supplementary Figure 2B). Shared DEGS that enriched to “regulation of cytokine production” included a mixture of genes important for inflammation (e.g., IL18R1 and MYD88), chemotaxis (e.g., FLOT1 and ROCK2), and pathogen recognition (e.g., CLEC7A, CLEC4A, and TLR2) (Supplementary Figure 2C). DEGs upregulated at 4–6 DPI in all isolates and enriching to “lymphocyte activation” reflected both B and T cell-mediated immunity (e.g., ARG2, BCL6, LYN, and STAT3) (Supplementary Figure 2D). Similarly, DEGs belonging only to C07 and Liberia for this term also associated with lymphocyte-mediated immunity (e.g., CD81, IL6, and LCP1) as well as T cell regulation (e.g., IDO1, PDCD1LG2, and TCIRG1) (Supplementary Figure 2D).
Complete blood count analysis showed hallmarks of EVD in all four cohorts of animals including lymphopenia, neutrophilia, and thrombocytopenia (Supplementary Figure 3 and Supplementary Table 1). Furthermore, significant declines in platelets were noted as early as 2 DPI but only in Mayinga-infected animals (Supplementary Figure 3 and Supplementary Table 1). Declines in lymphocyte numbers were noted only in Mayinga and C07 infections at 4 DPI (Supplementary Figure 3 and Supplementary Table 1). However, lymphopenia was most pronounced in Mayinga-infected animals (Figure 3B), whereas neutrophilia was not evident in animals infected with the late EBOV-Makona variant Mali (Figure 3C). To gain a deeper understanding of the changes in immune cell frequencies following infection with EBOV-Makona isolates, we performed DCQ. Frequencies of activated NK and DC subsets were predicted to increase over the course of infection for all three isolates (Figures 3F–H). Higher frequencies of monocytes were only predicted following C07 infection (Figure 3F). Significant lymphopenia was predicted to occur following infection with C07 wherein levels of CD4 T cells and several B cell subsets decreased with infection (Figure 3F). In contrast, significant but modest changes in naïve B cells or CD8 T cells were predicted to occur following infection with Mali and Liberia variants, respectively (Figures 3G–H).
We next used STEM to identify groups of genes with similar patterns of longitudinal gene expression (Supplementary Figure 4). In all isolates, we detected a cluster (cluster 0) of genes whose expression progressively increased over the course of infection (Supplementary Figures 4A–C). Genes in cluster 0 from all three isolates enriched to similar GO terms involved in both innate (e.g., “myeloid leukocyte activation” and “regulation of innate immune response”) and adaptive (e.g., “lymphocyte activation” and “T cell differentiation”) immunity (Supplementary Figure 4D). A second cluster (cluster 1) featured genes whose expression levels were slightly downregulated at 4 DPI before returning to near baseline by 6 DPI and enriched to “translation” and “DNA repair” (Supplementary Figure 4E). However, a unique cluster (cluster 2) was identified following infection with the EBOV-Makona Guinea C07 isolate, which consisted of genes robustly upregulated at 4 and 6 DPI. Genes in this cluster played roles in innate immunity (e.g., “myeloid leukocyte activation”), adaptive immunity (e.g., “T cell receptor signaling pathway”), antiviral defense (e.g., “cellular response to type I IFN”), and EVD pathology (e.g., “blood coagulation”) (Supplementary Figure 4F).
Ebola Virus-Makona and EBOV-Mayinga Induce an Overlapping Core of Antiviral, Inflammatory, and Apoptotic Genes
We next compared transcriptional changes among animals infected with EBOV-Mayinga or EBOV-Makona isolates (Figure 4). PCA showed a significant overlap between all four infections at each DPI (Figure 4A). We identified a shared core of 704 genes that were involved in antiviral immunity (e.g., IFIH1, IRF7, OAS1, ISG15, and STAT1 – GO terms “defense response to virus,” “cellular response to type I IFN”), inflammation (e.g., NOD2, TICAM1, and TLR4 – GO terms “activation of innate immune response,” “myeloid cell differentiation”), cytokine signaling (e.g., CCL4, INPP5D, and IRAK2 – GO term “cytokine-mediated signaling pathway”), and immune cell activation (e.g., LYN, MAFB, PRKCB, and VAV1 – GO terms “lymphocyte activation”) (Figures 4B,C).
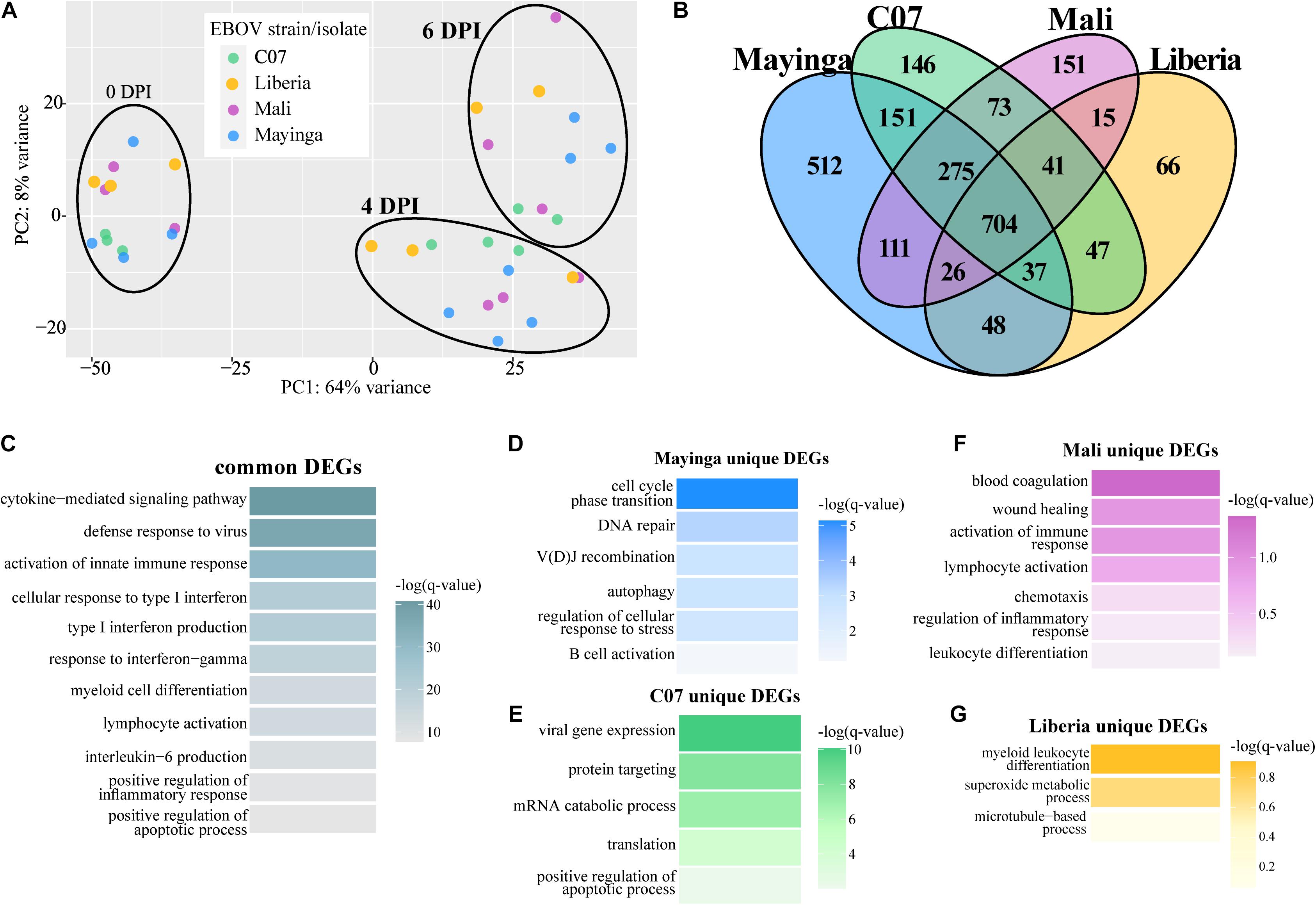
Figure 4. Ebola virus-Makona and EBOV-Mayinga isolates share a core of DEGs related to EVD pathology. (A) Principal component analysis of normalized transcript counts at 0, 4, and 6 DPI for EBOV-Makona isolates and EBOV-Mayinga. (B) Four-way Venn diagram depicting overlaps between all up- and downregulated DEGs identified 4 and 6 DPI for all isolates. Functional enrichments for DEGs (C) common to all isolates or unique to (D) EBOV-Mayinga, (E) Guinea C07, (F) Mali, and (G) Liberia. Color indicates the –log(q-value) of each GO term.
Additionally, there were DEGs unique to each infection. The largest group of unique DEGs was detected following EBOV-Mayinga infection. Those unique DEGs were associated with B cell-mediated immunity (e.g., ATM, CD27, PRKDC, and TCF3), stress responses (e.g., BAX, EYA3, and NUP155), and DNA repair (e.g., COPS2, LIG3, and PYHIN1) (Figure 4D). DEGs detected only following infection with the early EBOV-Makona Guinea C07 isolate played a role in nucleic acid metabolism (e.g., GO term “viral gene expression” and “translation”) and catabolic stress responses (e.g., GO term “positive regulation of apoptotic process”) (Figure 4E). Many DEGs unique to late EBOV-Makona Mali infection enriched to GO terms related to EVD associated processes, notably “blood coagulation,” “regulation of inflammatory response,” and “wound healing” (Figure 4F). The few DEGs unique to late EBOV-Makona Liberia infection played a role in myeloid cell differentiation (e.g., HCLS1 and RARA) and host metabolic processes (e.g., CCS and PN3) (Figure 4G).
To identify clusters of genes with similar patterns of gene expression across all EBOV-Makona and EBOV-Mayinga isolates, we applied a two-way forward regression model using MaSigPro (Figure 5) (Conesa et al., 2006). We retained only those genes that were considered significant in at least 16 comparisons and then clustered them based on temporal expression patterns, which results in four significant clusters (Figures 5A–D). Genes in clusters 1 and 2 exhibited modest upregulation 4 DPI followed by a sharp increase 6 DPI. These 495 genes played a role in the activation and regulation of innate (e.g., “regulation of innate immune response”) and adaptive (e.g., “lymphocyte activation”) immune responses (Figures 5A,E). Notable genes within this cluster are involved in leukocyte–leukocyte interactions (e.g., ICAM1 and ITGB2), myeloid cell signaling (e.g., CD14, RELA, NFKB1, and TRAF3), as well as lymphocyte activation (e.g., BATF, LAT2, and PRKCB) (Supplementary Figure 5A). The 301 genes in cluster 2 mainly played a role in translation (“mRNA processing”), antigen presentation (“autophagy” and “antigen processing and presentation”), and coagulopathy (“blood coagulation”; e.g., A2M, P2RX1, PRKAR2B, and SELP) (Figures 5B,E and Supplementary Figure 5B). Expression of genes in cluster 3 progressively increased from 0 to 6 DPI and enriched to similar GO processes described for clusters 1 and 2 in addition to “type I IFN production”/“response to virus” (e.g., DDX58, IFIH1, IRF1, STAT1/3, and ISG15) (Supplementary Figure 5C). Cluster 4 consisted of 20 genes associated with metabolism that were mostly downregulated over the course of infection (Figure 5D).
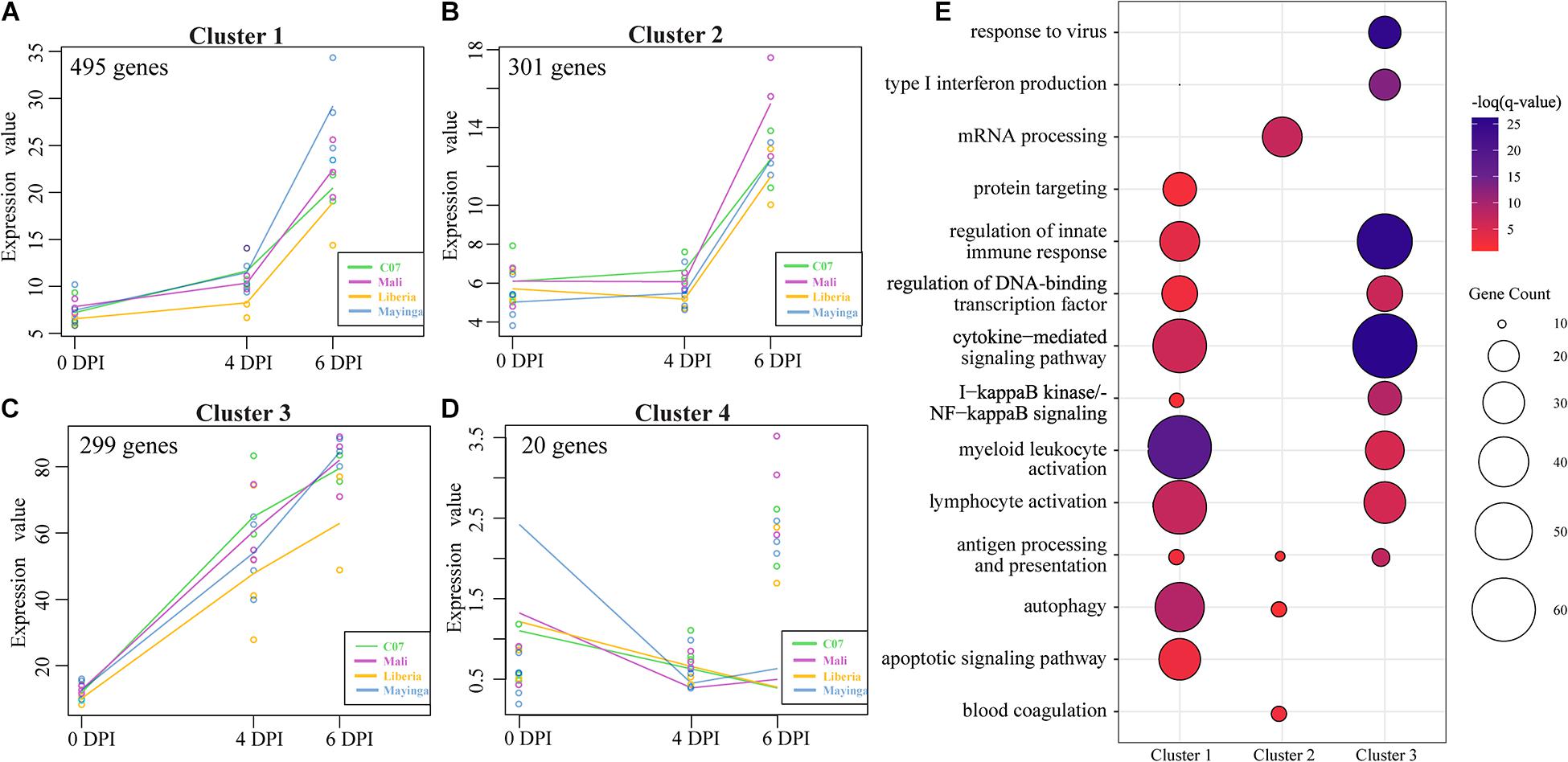
Figure 5. MaSigPro, two-way forward regression analysis of EBOV-Makona and EBOV-Mayinga isolates. (A–D) Gene clusters 1–4 identified by MaSigPro. (E) Bubbleplot depicting functional enrichment of genes belonging to clusters 1, 2, and 3. The size of the bubble represents the number of DEGs, while the color represents the –log(q-value).
To identify gene signatures that can differentiate among the groups, we next applied a sparse partial least-squares discrimination analysis (sPLS-DA) (Figure 6). Contrary to PCA, sPLS-DA permits identification of the minimum number of genes responsible for driving a given component of variation while preserving maximum covariance among defined groups. We identified three components that contributed to the majority of variation among EBOV strains (Figures 6A–C). The six genes that explained component 1 (16% variation) included immunoglobulin light chain IGKV2D-40, intracellular trafficking protein RAB30, and transcription factor TCF4 that regulates lymphoid and plasmacytoid DC development (Figures 6A–D). These six genes were sufficient to separate EBOV-Mayinga and EBOV-Makona isolates, but this separation was heavily influenced by Mali infection (Figures 6D,E). The six genes that explained component 2 (14% variation) separated Liberia from EBOV-Mayinga infections (Figures 6A–D). These six genes played a role in immune signaling (e.g., FCN1 and TYROBP) and ubiquitin-protein ligase complex component (RMND5A) and were upregulated with EBOV-Mayinga and Liberia infection, respectively (Figure 6E). Nearly 300 genes belonging to component 3 (6% variation) distinguished C07 from all other EBOV infections (Figures 6B,C). These genes played a role in host defense as well as cellular homeostasis, as indicated by enrichment to GO terms “myeloid leukocyte activation” (e.g., TLR3, TLR5, CR2, and CCR3), “lymphocyte activation” (e.g., IGHA2, IL7R, ITGA4, and LILRB2), “protein folding” (e.g., UBC and UBE2D1), and “positive regulation of cellular protein localization” (e.g., MAPK1 and TIMP2) (Figures 6F,G). This included a large number of chaperones and ubiquitin-conjugating enzymes (e.g., DNAJA1, HSPA1B, and UBE2D1) and genes modulating lymphocyte-mediated immunity (e.g., IGHA2, IL7R, ITGA4, and LILRB2) that were most prominently expressed in C07 infection (Figure 6G).
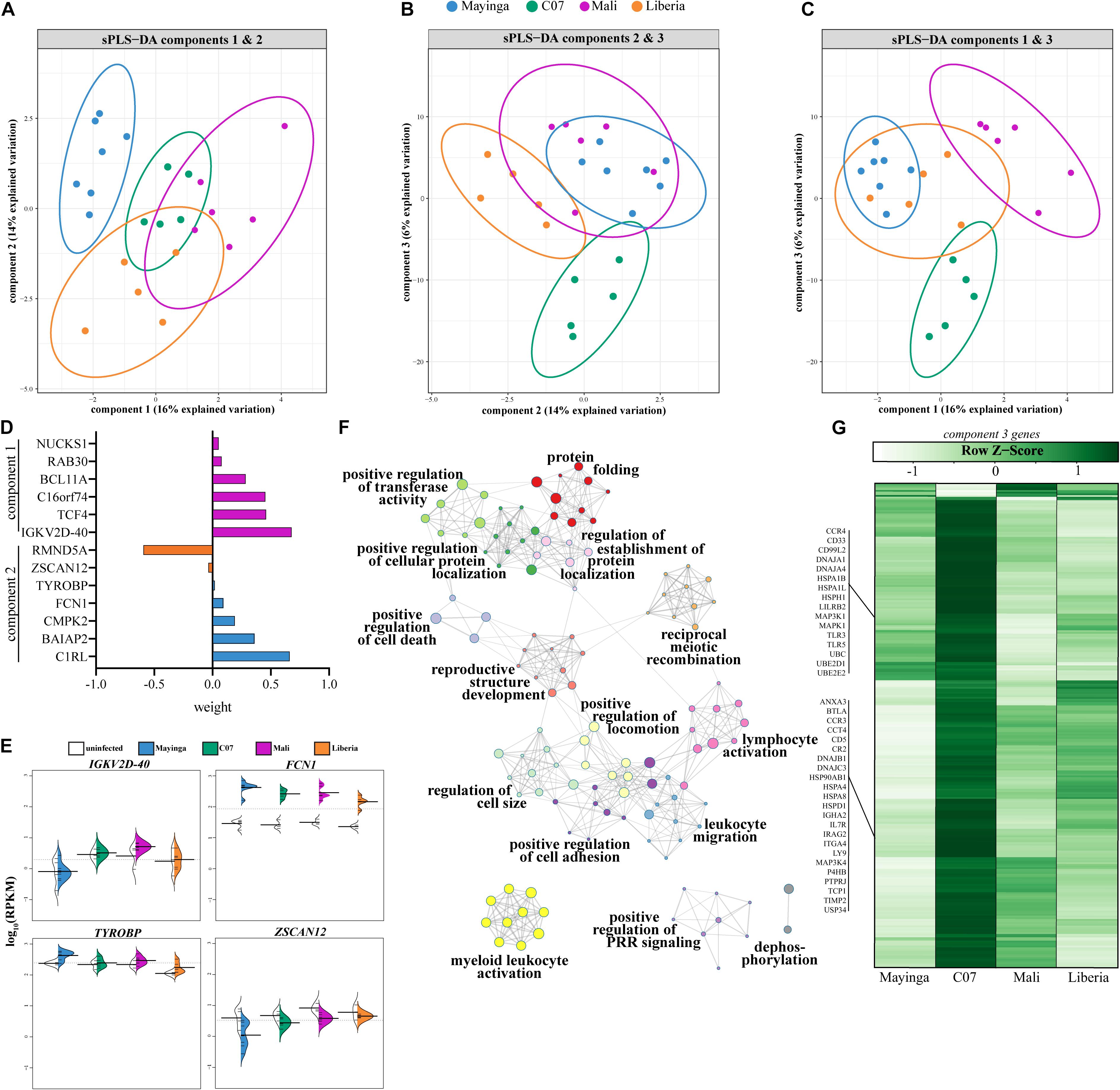
Figure 6. Genes regulating immune and stress responses are sufficient to distinguish EBOV infections. (A–C) Plots depicting the three components contributing the most to the variation amongst infected animals at 4 and 6 DPI as determined by sparse partial least squares discrimination analysis (sPLS-DA). (D) Weight loadings of genes belonging to components 1 and 2 as identified in panels (A–C). (E) Beanplots illustrating expression (log10RPKM) of genes from panel D. (F) Gene ontology (GO) network depicting functional enrichment of 290 genes of component 3. Clusters of closely related GO terms are labeled with the most significant GO term. Node size represents the number of genes associated with the GO term. Gray lines represent shared interactions between GO terms, with density and number indicating the strengths of connections between closely related GO terms. (G) Heatmap representing the 290 genes of component 3. Each column represents the average rpkm values of the indicated group across 4 and 6 DPI. Range of colors is based on scaled and centered rpkm values of the represented DEGs.
Discussion
Recent epidemiological studies suggest that different EBOV strains are associated with significant differences in CFRs despite sharing over 97% genetic similarity (Bell et al., 2015; Marzi et al., 2015, 2018; Wong et al., 2016; Menicucci et al., 2017; Versteeg et al., 2017, 2019; Madelain et al., 2018; McMullan et al., 2019). Specifically, historical strains EBOV-Mayinga and EBOV-Kikwit incur CFRs near 90%, while the EBOV-Makona strain from the 2013–2016 West Africa epidemic incurred a notably lower CFR of ∼40% (Centers for Disease Control and Prevention, 1995a, b; Muyembe-Tamfum et al., 1999; Kuhn et al., 2013; Zhang and Scheuermann, 2014; Kaner and Schaack, 2016; Shoman et al., 2017). Over the course of the 2013–2016 West Africa epidemic, several isolates of EBOV-Makona emerged late (Mali, Liberia) in 2014, which contained mutations in key genes (e.g., GP, NP, and the RNA dependent RNA polymerase) that were associated with changes in replication kinetics in vitro and were believed to facilitate widespread dissemination of the virus. However, in vivo studies in NHP did not show increased virulence of these variants, but rather a modest delay to euthanasia in a uniformly lethal challenge model (Marzi et al., 2018). Additionally, the CFR late in the epidemic was not higher than that observed earlier in the epidemic (Garske et al., 2017; Forna et al., 2020). It is likely that the lower CFR observed during the West Africa Epidemic is due to improved public health intervention strategies and mobilization. Indeed, CFRs for recent EBOV outbreaks in the DRC (2017–2020) are also lower than historical CFRs, ranging from ∼40 to 66% across 3,662 reported cases (Centers for Disease Control and Prevention, 2021b). However, the potential impact of these mutations on host immunity and defense has yet to be defined. These studies would provide key insight into the interaction between viral genetic evolution on host pathogenesis.
Therefore, in this study, we conducted the first comparative analysis of host molecular responses to infection with EBOV-Mayinga and EBOV-Mayinga infections using an NHP model (Marzi et al., 2018). We leveraged access to historical RNA samples and used RNA-Sequencing to compare transcriptional responses in the WB of rhesus macaques infected with a lethal dose of either EBOV-Mayinga or early (C07) or late (Mali, Liberia) EBOV-Makona isolates. Bivariate, longitudinal, regression, and discrimination analysis strategies allowed us to identify key to comprehensively identify differences and similarities among host responses.
Our analysis of the transcriptional response to EBOV-Mayinga revealed transcriptional changes reflecting canonical characteristics of EVD such as progressive upregulation of genes associated with inflammation, apoptosis, and antiviral defense, while genes associated with adaptive immunity were primarily suppressed. These observations are in line with the severe lymphopenia, myelopoiesis, and the cytokine storm that constitute the hallmarks of EVD (Geisbert et al., 2003a; Rivera and Messaoudi, 2016; Jacob et al., 2020). These results support previous analyses of host transcriptional responses to EBOV-Kikwit in cynomolgus macaques and fatal EBOV infection in humans (Barrenas et al., 2015; Eisfeld et al., 2017; Liu et al., 2017; Menicucci et al., 2017; Versteeg et al., 2017; Kotliar et al., 2020).
As described for EBOV-Mayinga infection, transcriptomes of NHPs infected with early or late EBOV-Makona isolates indicated progressive upregulation of genes related to innate immune activation, inflammation, and innate antiviral immunity. A core of ISGs was longitudinally upregulated following infection with all isolates and confirmed by regression analysis. This was paralleled by predicted increases in the frequencies of activated DCs and monocytes by DCQ analysis. Although our analysis could not resolve plasmacytoid (p)DCs, the major source of type I IFN, previous analysis showed that circulating IFN-alpha levels in the animals studied here were significantly elevated at 6 DPI for all EBOV-infected NHPs (Marzi et al., 2018). A sustained antiviral IFN and cytokine response has been shown to be detrimental to the host by suppressing adaptive immunity in acute and chronic viral infections and inducing bystander death of T and B cells, which is not seen in the bat reservoir of EBOV (Geisbert et al., 2000, 2003a; Bradfute et al., 2008; Stacey et al., 2009; O’Brien et al., 2011; Davidson et al., 2015). Recent transcriptional profiling of WB RNA samples from EBOV-infected human patients also identified a strong inflammatory ISG signature as a predictor of fatal outcome (Villinger et al., 1999; Baize et al., 2002; Wauquier et al., 2010; McElroy et al., 2014; Liu et al., 2017). Interestingly, the expression of TCF4, a transcription factor highly expressed in pDCs, was sufficient to distinguish EBOV-Makona (especially late isolate Mali) from EBOV-Mayinga infections (Forrest et al., 2014). This observation suggests that activation of pDCs may be associated with reduced mortality. The aberrant IFN production associated with fatal EBOV outcome could be primarily mediated by non-pDC cells that are infected by EBOB such as endothelial cells. All EBOV-Makona isolates also featured increases in activated NK cells and inflammatory DCs, which may contribute to EVD pathology (Warfield et al., 2004; Mohamadzadeh et al., 2006; Cimini et al., 2017; Menicucci et al., 2017; Fausther-Bovendo et al., 2019; Kotliar et al., 2020).
Our discrimination analysis did not identify inflammatory genes as main drivers of transcriptional distinction among EBOV infections, suggesting that the cytokine storm is a conserved outcome. Rather, genes involved in host defense, such as FCN1 and TYROBP expressed in EBOV-Mayinga infection, and numerous protein chaperones highly upregulated in EBOV-Makona C07 infection, were sufficient to separate infections. Protein folding in response to cellular stress can be either beneficial or harmful during viral infection since increases in the levels of protein chaperones may facilitate the folding of viral proteins or the expression of host antiviral defense proteins (Maruri-Avidal et al., 2008; Khachatoorian et al., 2016; Pujhari et al., 2019; Paladino et al., 2020; Wan et al., 2020). This finding complements our longitudinal (STEM) and bivariate analyses that identified sets of genes uniquely expressed during EBOV-Makona C07 infection that associate with protein localization and cellular stress. This distinction provides a potential explanation for the delayed disease progression following EBOV-Makona C07 infection relative to EBOV-Mayinga.
Interestingly, genes related to type I IFN response, apoptosis, cell death, and inflammatory signaling pathways were less upregulated initially following infection with the EBOV-Makona Liberia isolate. These differences were in line with the lack of neutrophilia and reduced levels of serum IFN-alpha in this group of animals. A delay in these transcriptional changes may contribute to minor differences in disease pathology, such as prolonged time to death in some NHPs, and the lack of lymphopenia predicted using DCQ (Marzi et al., 2018). Additionally, genes related to hemopoiesis were upregulated early during infection, which may result in the reduced severity of lymphopenia observed in this group. Although macaques infected with the EBOV-Makona Liberia isolate ultimately succumb to disease, the delay in clinical disease progression may provide a larger window for clinical intervention (Marzi et al., 2018). Furthermore, subversion of the host response may also permit viral persistence, as seen by the reemergence of an EBOV isolate closely related to EBOV-Makona Liberia in a survivor from the 2013–2016 outbreak (Centers for Disease Control and Prevention, 2021a).
Genes associated with adaptive immunity were largely downregulated in all animals, albeit more pronounced following EBOV-Mayinga, followed by EBOV-Makona Guinea C07 infection compared to other EBOV-Makona isolates. Furthermore, we detected significant declines in CD4 Th1 and CD8 populations in only EBOV-Mayinga infection that paralleled robust apoptotic gene expression. The lymphopenia predicted based on gene expression agrees with our previous flow cytometry analysis of PBMC from EBOV-Makona infected cynomolgus macaques (Versteeg et al., 2017). Interestingly, discrimination analysis identified a number of T cell-related genes (e.g., IL7R, LY9, and TCP1) that were uniquely upregulated with Makona-C07 infection, which may reflect aberrant T cell activation associated with fatal outcomes in EVD patients (Baize et al., 1999; Agrati et al., 2016). The expression of these genes could potentially explain the reduced severity in lymphopenia and CFR compare to Mayinga infection. Nevertheless, minor dysregulation in T cell responses can have significant ramifications for disease progression. Dysregulated T cell and cytotoxic responses are associated with a poor EVD prognosis, which may be driven by excessive production of type I IFN interferon (Agrati et al., 2016; Ruibal et al., 2016; Speranza et al., 2018).
Humoral immunity is also critical for recovery from and long-term protection against EVD (Thom et al., 2020). Monoclonal antibodies targeting the EBOV GP, as well as antibodies formed following vaccination with the FDA-approved EBOV vaccine, are also known to be protective in both humans and NHPs (Maruyama et al., 1999; Corti et al., 2016; Holtsberg et al., 2016; Misasi et al., 2016; Gaudinski et al., 2019). EBOV-Makona and EBOV-Mayinga isolates induced upregulation of genes involved in humoral immunity, although these gene sets were largely distinct. The loss of lymphocytes and predicted decline in B cell populations in was most pronounced in EBOV-Mayinga, followed by EBOV-Makona Guinea C07 and Mali, but not EBOV-Makona Liberia.
The major caveat of our study is that lethal doses of EBOV were administered to each animal, resulting in rapid disease progression not seen in typical human infections. This prevents resolution of finer differences in disease progression and restricts longitudinal analysis, which should be addressed in future studies by using nonlethal doses. Another caveat is that mutations identified during a human outbreak may not elicit the same response from rhesus macaques’ immune system. Nevertheless, this is the first study to compare molecular host responses using transcriptomics within the WB of animals infected with the historical EBOV-Mayinga isolate or the EBOV-Makona isolates that arose during the recent 2013–2016 West Africa epidemic. Our data provide critical insight into the impact of genetic variation among and between strains of EBOV on the molecular host response to infection in NHPs. Our transcriptional analyses indicate similar patterns of gene expression related to immune activation, inflammation and cell death, and induction of a sustained core of innate antiviral genes following infection with all EBOV isolates. However, late EBOV-Makona isolates Mali and Liberia were associated with less severe lymphopenia and smaller transcriptional responses than earlier EBOV-Makona isolates that are not reflected as disease attenuation at the clinical level. Later EBOV-Makona isolates also lacked the sharp upregulation of pathology-associated genes/cytokine storm seen in early EBOV-Makona isolate infections. However, a small subset of genes (∼300) that play a role in innate and adaptive immunity was sufficient to distinguish one infection from another, suggesting potential differences in immune responses. While transcriptional differences exist, all infections result in lethal outcomes. Therefore, viral genetic variation is associated with distinct molecular EVD pathogenesis, but not outcome implying that this cannot be a major factor influencing the 2013–2016 epidemic transmission rate or reduced CFR.
Data Availability Statement
Sequencing data for rhesus macaques is available at BioProject PRJNA718880.
Ethics Statement
The animal study was reviewed and approved by the Rocky Mountain Laboratory Animal Care and Use Committee.
Author Contributions
KM, AP, IM, and AM designed the experiments. KM, AP, and AM conducted the experiments. KM, AP, and IM analyzed the data and wrote the manuscript. All authors approved the manuscript.
Funding
This work was supported by the National Center for Research Resources and the National Center for Advancing Translational Sciences, NIH, through grant UL1 TR001414 awarded to IM, and the Intramural Research Program NIAID, NIH.
Conflict of Interest
The authors declare that the research was conducted in the absence of any commercial or financial relationships that could be construed as a potential conflict of interest.
Publisher’s Note
All claims expressed in this article are solely those of the authors and do not necessarily represent those of their affiliated organizations, or those of the publisher, the editors and the reviewers. Any product that may be evaluated in this article, or claim that may be made by its manufacturer, is not guaranteed or endorsed by the publisher.
Supplementary Material
The Supplementary Material for this article can be found online at: https://www.frontiersin.org/articles/10.3389/fmicb.2021.714817/full#supplementary-material
Supplementary Figure 1 | Analysis of EBOV-Mayinga infection DEGs enriching to key GO terms. (A) Heatmap of downregulated genes enriching to “Thymus development,” “B cell proliferation involved in immune response,” “Antigen processing and presentation of exogenous peptide antigen via MHC class II,” “Lymphocyte differentiation,” and “T cell activation.” Membership of genes to gene ontology (GO) terms is denoted by matching colored marker. Heatmaps of 4 DPI unique, 6 DPI unique, or shared upregulated genes enriching to (B) “Myeloid cell differentiation,” (C) “T cell activation,” and (D) “Type I interferon production.” Each column represents one animal. Red presents upregulated; blue represents downregulated. Range of colors is based on scaled and centered rpkm values of the represented DEGs.
Supplementary Figure 2 | Comparison of upregulated DEGs enriching to GO terms shared by early and late EBOV-Makona isolates. Heatmaps of shared upregulated DEGs enriching to GO terms common to all isolates: (A) “positive regulation of cell death,” (B) “defense response to virus,” (C) “regulation of cytokine production,” and (D) “lymphocyte activation.” Accompanying Venn diagrams depict the DEGs enriching to each isolate per given the given GO term. Only DEGs shared by either all isolates [e.g., panel (A)] or by Guinea C07 and Mali [e.g., panel (D)] are depicted and are indicated in red in the Venn diagram. Each column represents one animal. Red presents upregulated; blue represents downregulated. Range of colors is based on scaled and centered rpkm values of the represented DEGs.
Supplementary Figure 3 | Ebola virus infection results in lymphopenia, neutrophilia, and thrombocytopenia. (A) Total number of white blood cells (thousand/μl), (B) lymphocytes (% total cells), (C) neutrophils (%) total cells, and (D) platelet count (thousand/μl) for EBOV-Mayinga and EBOV-Makona isolates from d0 to d6. Significant differences between isolates at a given time point were determined with one-way ANOVA with multiple comparisons. ∗Guinea C07, #Mali, $Liberia. p-value < 0.05∗, p-value < 0.01∗∗, p-value < 0.001∗∗∗, and p-value < 0.0001****.
Supplementary Figure 4 | STEM analysis of EBOV-Makona isolates. Gene expression graphs depicting the STEM clusters identified for EBOV-Makona (A) Guinea C07, (B) Mali, and (C) Liberia. GO term enrichment heatmaps for (D) cluster 0 and (E) cluster 1. Color intensity represents the statistical significance [shown as the −log(q-value)] with the range of colors based on GO terms with the lowest and highest significance statistic for the entire set of GO terms per cluster. The number of genes enriching to each GO term per column is represented in each box; white boxes indicate no statistical significance. (F) GO term bar graphs representing enrichment of genes in cluster 3. Horizontal bars represent the number of genes mapping to each GO term, while color intensity represents the −log(q-value) value of the corresponding GO term. Panels (D–F) follow the color key in plots A–C: cluster 0, red; cluster 1, blue; and cluster 2, orange.
Supplementary Figure 5 | Analysis of genes identified by MaSigPro, two-way forward regression analysis for EBOV-Makona and EBOV-Mayinga isolates. Heatmaps of genes identified by MaSigPro for (A) cluster 1, (B) cluster 2, (C) cluster 3, and (D) cluster 4. The latter was split into two sub-clusters: cluster 4A containing genes that were upregulated only in response to Liberia isolate and cluster 4B containing genes downregulated by all isolates. Select genes are indicated. Each column represents the median RPKM of all animals at a given day post infection. Red presents upregulated; blue represents downregulated. Range of colors is based on scaled and centered rpkm values of the represented DEGs.
Supplementary Table 1 | Statistical analysis (adjusted p-values) of longitudinal changes in cell and platelet populations. Adjusted p-values for data in Supplementary Figure 3. Two-way ANOVA with Geisser-Greenhouse correction to account for unequal variability of differences. Significant values highlighted in red. DPI, days post infection.
Footnotes
References
Abbas, A. R., Baldwin, D., Ma, Y., Ouyang, W., Gurney, A., Martin, F., et al. (2005). Immune response in silico (IRIS): immune-specific genes identified from a compendium of microarray expression data. Genes Immun. 6, 319–331. doi: 10.1038/sj.gene.6364173
Agrati, C., Castilletti, C., Casetti, R., Sacchi, A., Falasca, L., Turchi, F., et al. (2016). Longitudinal characterization of dysfunctional T cell-activation during human acute Ebola infection. Cell Death Dis. 7:e2164. doi: 10.1038/cddis.2016.55
Baillet, N., Reynard, S., Perthame, E., Hortion, J., Journeaux, A., Mateo, M., et al. (2021). Systemic viral spreading and defective host responses are associated with fatal Lassa fever in macaques. Commun. Biol. 4:27. doi: 10.1038/s42003-020-01543-7
Baize, S., Leroy, E. M., Georges, A. J., Georges-Courbot, M. C., Capron, M., Bedjabaga, I., et al. (2002). Inflammatory responses in Ebola virus-infected patients. Clin. Exp. Immunol. 128, 163–168. doi: 10.1046/j.1365-2249.2002.01800.x
Baize, S., Leroy, E. M., Georges-Courbot, M. C., Capron, M., Lansoud-Soukate, J., Debre, P., et al. (1999). Defective humoral responses and extensive intravascular apoptosis are associated with fatal outcome in Ebola virus-infected patients. Nat. Med. 5, 423–426. doi: 10.1038/7422
Barrenas, F., Green, R. R., Thomas, M. J., Law, G. L., Proll, S. C., Engelmann, F., et al. (2015). Next-generation sequencing reveals a controlled immune response to Zaire Ebola virus challenge in cynomolgus macaques immunized with vesicular stomatitis virus expressing Zaire Ebola virus glycoprotein (VSVΔG/EBOVgp). Clin. Vaccine Immunol. 22, 354–356. doi: 10.1128/CVI.00733-14
Bell, A., Lewandowski, K., Myers, R., Wooldridge, D., Aarons, E., Simpson, A., et al. (2015). Genome sequence analysis of Ebola virus in clinical samples from three British healthcare workers, August 2014 to March 2015. Euro Surveill. 20:21131. doi: 10.2807/1560-7917.es2015.20.20.21131
Bradfute, S. B., Warfield, K. L., and Bavari, S. (2008). Functional CD8+ T cell responses in lethal Ebola virus infection. J. Immunol. 180, 4058–4066. doi: 10.4049/jimmunol.180.6.4058
Centers for Disease Control and Prevention (1995a). Outbreak of Ebola viral hemorrhagic fever–Zaire, 1995. MMWR Morb. Mortal. Wkly. Rep. 44, 381–382.
Centers for Disease Control and Prevention (1995b). Update: outbreak of Ebola viral hemorrhagic fever–Zaire, 1995. MMWR Morb. Mortal. Wkly. Rep. 44, 468–469, 475.
Centers for Disease Control and Prevention (2021a). 2021 Democratic Republic of the Congo, North Kivu Province. CDC.
Centers for Disease Control and Prevention (2021b). History of Ebola Outbreaks: Cases and Outbreaks of EVD by Year. CDC.
Cimini, E., Viola, D., Cabeza-Cabrerizo, M., Romanelli, A., Tumino, N., Sacchi, A., et al. (2017). Different features of Vdelta2 T and NK cells in fatal and non-fatal human Ebola infections. PLoS Negl. Trop Dis. 11:e0005645. doi: 10.1371/journal.pntd.0005645
Conesa, A., Nueda, M. J., Ferrer, A., and Talon, M. (2006). maSigPro: a method to identify significantly differential expression profiles in time-course microarray experiments. Bioinformatics 22, 1096–1102. doi: 10.1093/bioinformatics/btl056
Corti, D., Misasi, J., Mulangu, S., Stanley, D. A., Kanekiyo, M., Wollen, S., et al. (2016). Protective monotherapy against lethal Ebola virus infection by a potently neutralizing antibody. Science 351, 1339–1342. doi: 10.1126/science.aad5224
Davidson, S., Maini, M. K., and Wack, A. (2015). Disease-promoting effects of type I interferons in viral, bacterial, and coinfections. J. Interferon Cytokine Res. 35, 252–264. doi: 10.1089/jir.2014.0227
Dietzel, E., Schudt, G., Krahling, V., Matrosovich, M., and Becker, S. (2017). Functional characterization of adaptive mutations during the West African Ebola virus outbreak. J. Virol. 91:e01913-16. doi: 10.1128/JVI.01913-16
Eisfeld, A. J., Halfmann, P. J., Wendler, J. P., Kyle, J. E., Burnum-Johnson, K. E., Peralta, Z., et al. (2017). Multi-platform ‘omics analysis of human Ebola virus disease pathogenesis. Cell Host Microbe 22, 817–829.e8. doi: 10.1016/j.chom.2017.10.011
Ernst, J., and Bar-Joseph, Z. (2006). STEM: a tool for the analysis of short time series gene expression data. BMC Bioinformatics 7:191. doi: 10.1186/1471-2105-7-191
Fausther-Bovendo, H., Qiu, X., He, S., Bello, A., Audet, J., Ippolito, G., et al. (2019). NK cells accumulate in infected tissues and contribute to pathogenicity of Ebola virus in mice. J. Virol. 93:e01703-18. doi: 10.1128/JVI.01703-18
Feldmann, H., and Geisbert, T. W. (2011). Ebola haemorrhagic fever. Lancet 377, 849–862. doi: 10.1016/S0140-6736(10)60667-8
Forna, A., Nouvellet, P., Dorigatti, I., and Donnelly, C. A. (2020). Case fatality ratio estimates for the 2013-2016 West African Ebola epidemic: application of boosted regression trees for imputation. Clin. Infect. Dis. 70, 2476–2483. doi: 10.1093/cid/ciz678
Forrest, M. P., Hill, M. J., Quantock, A. J., Martin-Rendon, E., and Blake, D. J. (2014). The emerging roles of TCF4 in disease and development. Trends Mol. Med. 20, 322–331. doi: 10.1016/j.molmed.2014.01.010
Frishberg, A., Brodt, A., Steuerman, Y., and Gat-Viks, I. (2016). ImmQuant: a user-friendly tool for inferring immune cell-type composition from gene-expression data. Bioinformatics 32, 3842–3843. doi: 10.1093/bioinformatics/btw535
Garske, T., Cori, A., Ariyarajah, A., Blake, I. M., Dorigatti, I., Eckmanns, T., et al. (2017). Heterogeneities in the case fatality ratio in the West African Ebola outbreak 2013-2016. Philos. Trans. R. Soc. Lond. B Biol. Sci. 372:20160308. doi: 10.1098/rstb.2016.0308
Gaudinski, M. R., Coates, E. E., Novik, L., Widge, A., Houser, K. V., Burch, E., et al. (2019). Safety, tolerability, pharmacokinetics, and immunogenicity of the therapeutic monoclonal antibody mAb114 targeting Ebola virus glycoprotein (VRC 608): an open-label phase 1 study. Lancet 393, 889–898. doi: 10.1016/S0140-6736(19)30036-4
Geisbert, T. W., Hensley, L. E., Gibb, T. R., Steele, K. E., Jaax, N. K., and Jahrling, P. B. (2000). Apoptosis induced in vitro and in vivo during infection by Ebola and Marburg viruses. Lab. Invest. 80, 171–186. doi: 10.1038/labinvest.3780021
Geisbert, T. W., Hensley, L. E., Larsen, T., Young, H. A., Reed, D. S., Geisbert, J. B., et al. (2003a). Pathogenesis of Ebola hemorrhagic fever in cynomolgus macaques: evidence that dendritic cells are early and sustained targets of infection. Am. J. Pathol. 163, 2347–2370. doi: 10.1016/S0002-9440(10)63591-2
Geisbert, T. W., Strong, J. E., and Feldmann, H. (2015). Considerations in the use of nonhuman primate models of Ebola virus and Marburg virus infection. J. Infect. Dis. 212(Suppl. 2), S91–S97. doi: 10.1093/infdis/jiv284
Geisbert, T. W., Young, H. A., Jahrling, P. B., Davis, K. J., Kagan, E., and Hensley, L. E. (2003b). Mechanisms underlying coagulation abnormalities in ebola hemorrhagic fever: overexpression of tissue factor in primate monocytes/macrophages is a key event. J. Infect. Dis. 188, 1618–1629. doi: 10.1086/379724
Gupta, M., Mahanty, S., Bray, M., Ahmed, R., and Rollin, P. E. (2001). Passive transfer of antibodies protects immunocompetent and imunodeficient mice against lethal Ebola virus infection without complete inhibition of viral replication. J. Virol. 75, 4649–4654. doi: 10.1128/JVI.75.10.4649-4654.2001
Hoffmann, M., Crone, L., Dietzel, E., Paijo, J., Gonzalez-Hernandez, M., Nehlmeier, I., et al. (2017). A polymorphism within the internal fusion loop of the Ebola virus glycoprotein modulates host cell entry. J. Virol. 91:e00177-17. doi: 10.1128/JVI.00177-17
Holtsberg, F. W., Shulenin, S., Vu, H., Howell, K. A., Patel, S. J., Gunn, B., et al. (2016). Pan-ebolavirus and Pan-filovirus mouse monoclonal antibodies: protection against Ebola and Sudan viruses. J. Virol. 90, 266–278. doi: 10.1128/JVI.02171-15
Iampietro, M., Younan, P., Nishida, A., Dutta, M., Lubaki, N. M., Santos, R. I., et al. (2017). Ebola virus glycoprotein directly triggers T lymphocyte death despite of the lack of infection. PLoS Pathog. 13:e1006397. doi: 10.1371/journal.ppat.1006397
Jacob, S. T., Crozier, I., Fischer, W. A. II, Hewlett, A., Kraft, C. S., Vega, M. A., et al. (2020). Ebola virus disease. Nat. Rev. Dis. Primers 6:13. doi: 10.1038/s41572-020-0147-3
Khachatoorian, R., Riahi, R., Ganapathy, E., Shao, H., Wheatley, N. M., Sundberg, C., et al. (2016). Allosteric heat shock protein 70 inhibitors block hepatitis C virus assembly. Int. J. Antimicrob. Agents 47, 289–296. doi: 10.1016/j.ijantimicag.2016.01.012
Kotliar, D., Lin, A. E., Logue, J., Hughes, T. K., Khoury, N. M., Raju, S. S., et al. (2020). Single-cell profiling of Ebola virus disease in vivo reveals viral and host dynamics. Cell 183, 1383–1401 e19. doi: 10.1016/j.cell.2020.10.002
Kuhn, J. H., Bao, Y., Bavari, S., Becker, S., Bradfute, S., Brister, J. R., et al. (2013). Virus nomenclature below the species level: a standardized nomenclature for laboratory animal-adapted strains and variants of viruses assigned to the family Filoviridae. Arch. Virol. 158, 1425–1432. doi: 10.1007/s00705-012-1594-2
Liu, X., Speranza, E., Munoz-Fontela, C., Haldenby, S., Rickett, N. Y., Garcia-Dorival, I., et al. (2017). Transcriptomic signatures differentiate survival from fatal outcomes in humans infected with Ebola virus. Genome Biol. 18:4. doi: 10.1186/s13059-016-1137-3
Longet, S., Mellors, J., Carroll, M. W., and Tipton, T. (2020). Ebolavirus: comparison of survivor immunology and animal models in the search for a correlate of protection. Front. Immunol. 11:599568. doi: 10.3389/fimmu.2020.599568
Madelain, V., Baize, S., Jacquot, F., Reynard, S., Fizet, A., Barron, S., et al. (2018). Ebola viral dynamics in nonhuman primates provides insights into virus immuno-pathogenesis and antiviral strategies. Nat. Commun. 9:4013. doi: 10.1038/s41467-018-06215-z
Maruri-Avidal, L., Lopez, S., and Arias, C. F. (2008). Endoplasmic reticulum chaperones are involved in the morphogenesis of rotavirus infectious particles. J. Virol. 82, 5368–5380. doi: 10.1128/JVI.02751-07
Maruyama, T., Rodriguez, L. L., Jahrling, P. B., Sanchez, A., Khan, A. S., Nichol, S. T., et al. (1999). Ebola virus can be effectively neutralized by antibody produced in natural human infection. J. Virol. 73, 6024–6030. doi: 10.1128/JVI.73.7.6024-6030.1999
Marzi, A., Chadinah, S., Haddock, E., Feldmann, F., Arndt, N., Martellaro, C., et al. (2018). Recently identified mutations in the Ebola virus-Makona genome do not alter pathogenicity in animal models. Cell Rep. 23, 1806–1816. doi: 10.1016/j.celrep.2018.04.027
Marzi, A., Engelmann, F., Feldmann, F., Haberthur, K., Shupert, W. L., Brining, D., et al. (2013). Antibodies are necessary for rVSV/ZEBOV-GP-mediated protection against lethal Ebola virus challenge in nonhuman primates. Proc. Natl. Acad. Sci. U. S. A. 110, 1893–1898. doi: 10.1073/pnas.1209591110
Marzi, A., Feldmann, F., Hanley, P. W., Scott, D. P., Gunther, S., and Feldmann, H. (2015). Delayed disease progression in cynomolgus macaques infected with Ebola virus Makona Strain. Emerg. Infect. Dis. 21, 1777–1783. doi: 10.3201/eid2110.150259
McElroy, A. K., Erickson, B. R., Flietstra, T. D., Rollin, P. E., Nichol, S. T., Towner, J. S., et al. (2014). Ebola hemorrhagic fever: novel biomarker correlates of clinical outcome. J. Infect. Dis. 210, 558–566. doi: 10.1093/infdis/jiu088
McMullan, L. K., Flint, M., Chakrabarti, A., Guerrero, L., Lo, M. K., Porter, D., et al. (2019). Characterisation of infectious Ebola virus from the ongoing outbreak to guide response activities in the democratic republic of the Congo: a phylogenetic and in vitro analysis. Lancet Infect. Dis. 19, 1023–1032. doi: 10.1016/S1473-3099(19)30291-9
Menicucci, A. R., Jankeel, A., Feldmann, H., Marzi, A., and Messaoudi, I. (2019). Antiviral innate responses induced by VSV-EBOV vaccination contribute to rapid protection. mBio 10:e00597-19. doi: 10.1128/mBio.00597-19
Menicucci, A. R., Versteeg, K., Woolsey, C., Mire, C. E., Geisbert, J. B., Cross, R. W., et al. (2017). Transcriptome analysis of circulating immune cell subsets highlight the role of monocytes in Zaire Ebola virus Makona pathogenesis. Front. Immunol. 8:1372. doi: 10.3389/fimmu.2017.01372
Misasi, J., Gilman, M. S., Kanekiyo, M., Gui, M., Cagigi, A., Mulangu, S., et al. (2016). Structural and molecular basis for Ebola virus neutralization by protective human antibodies. Science 351, 1343–1346. doi: 10.1126/science.aad6117
Mohamadzadeh, M., Coberley, S. S., Olinger, G. G., Kalina, W. V., Ruthel, G., Fuller, C. L., et al. (2006). Activation of triggering receptor expressed on myeloid cells-1 on human neutrophils by marburg and ebola viruses. J. Virol. 80, 7235–7244. doi: 10.1128/JVI.00543-06
Mortazavi, A., Williams, B. A., McCue, K., Schaeffer, L., and Wold, B. (2008). Mapping and quantifying mammalian transcriptomes by RNA-Seq. Nat. Methods 5, 621–628. doi: 10.1038/nmeth.1226
Muyembe-Tamfum, J. J., Kipasa, M., Kiyungu, C., and Colebunders, R. (1999). Ebola outbreak in Kikwit, democratic republic of the Congo: discovery and control measures. J. Infect. Dis. 179(Suppl. 1), S259–S262. doi: 10.1086/514302
Novershtern, N., Subramanian, A., Lawton, L. N., Mak, R. H., Haining, W. N., McConkey, M. E., et al. (2011). Densely interconnected transcriptional circuits control cell states in human hematopoiesis. Cell 144, 296–309. doi: 10.1016/j.cell.2011.01.004
Nueda, M. J., Tarazona, S., and Conesa, A. (2014). Next maSigPro: updating maSigPro bioconductor package for RNA-seq time series. Bioinformatics 30, 2598–2602. doi: 10.1093/bioinformatics/btu333
O’Brien, M., Manches, O., Sabado, R. L., Baranda, S. J., Wang, Y., Marie, I., et al. (2011). Spatiotemporal trafficking of HIV in human plasmacytoid dendritic cells defines a persistently IFN-alpha-producing and partially matured phenotype. J. Clin. Invest. 121, 1088–1101. doi: 10.1172/JCI44960
Otasek, D., Morris, J. H., Boucas, J., Pico, A. R., and Demchak, B. (2019). Cytoscape automation: empowering workflow-based network analysis. Genome Biol. 20:185. doi: 10.1186/s13059-019-1758-4
Paladino, L., Vitale, A. M., Caruso Bavisotto, C., Conway de Macario, E., Cappello, F., Macario, A. J. L., et al. (2020). The role of molecular chaperones in virus infection and implications for understanding and treating COVID-19. J. Clin. Med. 9:3518. doi: 10.3390/jcm9113518
Pinski, A., Woolsey, C., Jankeel, A., Cross, R., Basler, C. F., Geisbert, T., et al. (2021a). Transcriptional analysis of lymphoid tissues from infected nonhuman primates reveals the basis for attenuation and immunogenicity of an Ebola virus encoding a mutant VP35 protein. J. Virol. 95:e01995-20. doi: 10.1128/JVI.01995-20
Pinski, A. N., Maroney, K. J., Marzi, A., and Messaoudi, I. (2021b). Distinct transcriptional responses to fatal Ebola virus infection in cynomolgus and rhesus macaques suggest species-specific immune responses. Emerg. Microbes Infect. 10, 1320–1330. doi: 10.1080/22221751.2021.1942229
Pinski, A. N., and Messaoudi, I. (2020). To B or Not to B: mechanisms of protection conferred by rVSV-EBOV-GP and the roles of innate and adaptive immunity. Microorganisms 8:1473. doi: 10.3390/microorganisms8101473
Pleet, M. L., DeMarino, C., Lepene, B., Aman, M. J., and Kashanchi, F. (2017). The role of exosomal VP40 in Ebola virus disease. DNA Cell Biol. 36, 243–248. doi: 10.1089/dna.2017.3639
Pleet, M. L., Erickson, J., DeMarino, C., Barclay, R. A., Cowen, M., Lepene, B., et al. (2018). Ebola virus VP40 modulates cell cycle and biogenesis of extracellular vesicles. J. Infect. Dis. 218(suppl_5), S365–S387. doi: 10.1093/infdis/jiy472
Pujhari, S., Brustolin, M., Macias, V. M., Nissly, R. H., Nomura, M., Kuchipudi, S. V., et al. (2019). Heat shock protein 70 (Hsp70) mediates Zika virus entry, replication, and egress from host cells. Emerg. Microbes Infect. 8, 8–16. doi: 10.1080/22221751.2018.1557988
R Core Team (2015). R: A Language and Environment for Statistical Computing. Vienna: R Foundation for Statistical Computing.
Rivera, A., and Messaoudi, I. (2016). Molecular mechanisms of Ebola pathogenesis. J. Leukoc. Biol. 100, 889–904. doi: 10.1189/jlb.4RI0316-099RR
Robinson, M. D., McCarthy, D. J., and Smyth, G. K. (2010). edgeR: a bioconductor package for differential expression analysis of digital gene expression data. Bioinformatics 26, 139–140. doi: 10.1093/bioinformatics/btp616
Rohart, F., Gautier, B., Singh, A., and Le Cao, K. A. (2017). mixOmics: an R package for ‘omics feature selection and multiple data integration. PLoS Comput. Biol. 13:e1005752. doi: 10.1371/journal.pcbi.1005752
Ruedas, J. B., Arnold, C. E., Palacios, G., and Connor, J. H. (2018). Growth-adaptive mutations in the Ebola virus makona glycoprotein alter different steps in the virus entry pathway. J. Virol. 92:e00820-18. doi: 10.1128/JVI.00820-18
Ruedas, J. B., Ladner, J. T., Ettinger, C. R., Gummuluru, S., Palacios, G., and Connor, J. H. (2017). Spontaneous mutation at amino acid 544 of the Ebola virus glycoprotein potentiates virus entry and selection in tissue culture. J. Virol. 91:e00392-17. doi: 10.1128/JVI.00392-17
Ruibal, P., Oestereich, L., Ludtke, A., Becker-Ziaja, B., Wozniak, D. M., Kerber, R., et al. (2016). Unique human immune signature of Ebola virus disease in Guinea. Nature 533, 100–104. doi: 10.1038/nature17949
Sanchez, A., Kiley, M. P., Holloway, B. P., and Auperin, D. D. (1993). Sequence analysis of the Ebola virus genome: organization, genetic elements, and comparison with the genome of Marburg virus. Virus Res. 29, 215–240.
Shoman, H., Karafillakis, E., and Rawaf, S. (2017). The link between the West African Ebola outbreak and health systems in Guinea, Liberia and Sierra Leone: a systematic review. Global. Health 13:1. doi: 10.1186/s12992-016-0224-2
Speranza, E., Ruibal, P., Port, J. R., Feng, F., Burkhardt, L., Grundhoff, A., et al. (2018). T-cell receptor diversity and the control of T-cell homeostasis mark Ebola virus disease survival in humans. J. Infect. Dis. 218(suppl_5), S508–S518. doi: 10.1093/infdis/jiy352
Stacey, A. R., Norris, P. J., Qin, L., Haygreen, E. A., Taylor, E., Heitman, J., et al. (2009). Induction of a striking systemic cytokine cascade prior to peak viremia in acute human immunodeficiency virus type 1 infection, in contrast to more modest and delayed responses in acute hepatitis B and C virus infections. J. Virol. 83, 3719–3733. doi: 10.1128/JVI.01844-08
Thom, R., Tipton, T., Strecker, T., Hall, Y., Akoi Bore, J., Maes, P., et al. (2020). Longitudinal antibody and T cell responses in Ebola virus disease survivors and contacts: an observational cohort study. Lancet Infect. Dis. 21, 507–516. doi: 10.1016/S1473-3099(20)30736-2
Tw, H. B., and Girke, T. (2016). systemPipeR: NGS workflow and report generation environment. BMC Bioinformatics 17:388. doi: 10.1186/s12859-016-1241-0
Ueda, M. T., Kurosaki, Y., Izumi, T., Nakano, Y., Oloniniyi, O. K., Yasuda, J., et al. (2017). Functional mutations in spike glycoprotein of Zaire ebolavirus associated with an increase in infection efficiency. Genes Cells 22, 148–159. doi: 10.1111/gtc.12463
Versteeg, K., Menicucci, A. R., Woolsey, C., Mire, C. E., Geisbert, J. B., Cross, R. W., et al. (2017). Infection with the Makona variant results in a delayed and distinct host immune response compared to previous Ebola virus variants. Sci. Rep. 7:9730. doi: 10.1038/s41598-017-09963-y
Versteeg, K., Menicucci, A. R., Woolsey, C., Mire, C. E., Geisbert, J. B., Cross, R. W., et al. (2019). Infection with the Makona variant results in a delayed and distinct host immune response compared to previous Ebola virus variants (vol 7, 9730, 2017). Sci. Rep. 9:7329.
Villinger, F., Rollin, P. E., Brar, S. S., Chikkala, N. F., Winter, J., Sundstrom, J. B., et al. (1999). Markedly elevated levels of interferon (IFN)-gamma, IFN-alpha, interleukin (IL)-2, IL-10, and tumor necrosis factor-alpha associated with fatal Ebola virus infection. J. Infect. Dis. 179(Suppl. 1), S188–S191. doi: 10.1086/514283
Wan, Q., Song, D., Li, H., and He, M. L. (2020). Stress proteins: the biological functions in virus infection, present and challenges for target-based antiviral drug development. Signal Transduct. Target. Ther. 5:125. doi: 10.1038/s41392-020-00233-4
Warfield, K. L., Perkins, J. G., Swenson, D. L., Deal, E. M., Bosio, C. M., Aman, M. J., et al. (2004). Role of natural killer cells in innate protection against lethal ebola virus infection. J. Exp. Med. 200, 169–179. doi: 10.1084/jem.20032141
Wauquier, N., Becquart, P., Padilla, C., Baize, S., and Leroy, E. M. (2010). Human fatal zaire ebola virus infection is associated with an aberrant innate immunity and with massive lymphocyte apoptosis. PLoS Negl. Trop. Dis. 4:e837. doi: 10.1371/journal.pntd.0000837
Wong, G., He, S., Leung, A., Cao, W., Bi, Y., Zhang, Z., et al. (2019). Naturally occurring single mutations in Ebola virus observably impact infectivity. J. Virol. 93:e01098-18. doi: 10.1128/JVI.01098-18
Wong, G., Qiu, X., de La Vega, M. A., Fernando, L., Wei, H., Bello, A., et al. (2016). Pathogenicity comparison between the Kikwit and Makona Ebola virus variants in rhesus macaques. J. Infect. Dis. 214(Suppl. 3), S281–S289. doi: 10.1093/infdis/jiw267
Zhang, Y., and Scheuermann, R. H. (2014). 2014 Ebola Outbreak - Genome Sequence Variation Analysis. Virus Pathogen Resource (ViPR).
Keywords: Ebola virus, transcriptomic (RNA-Seq), viral epidemiology, nonhuman primate (macaque), Ebola virus disease
Citation: Maroney KJ, Pinski AN, Marzi A and Messaoudi I (2021) Transcriptional Analysis of Infection With Early or Late Isolates From the 2013–2016 West Africa Ebola Virus Epidemic Does Not Suggest Attenuated Pathogenicity as a Result of Genetic Variation. Front. Microbiol. 12:714817. doi: 10.3389/fmicb.2021.714817
Received: 25 May 2021; Accepted: 06 July 2021;
Published: 13 August 2021.
Edited by:
Leonard Peruski, Centers for Disease Control and Prevention, United StatesReviewed by:
Joel Mark Montgomery, Centers for Disease Control and Prevention, United StatesMark Nicholas Wass, University of Kent, United Kingdom
Copyright © 2021 Maroney, Pinski, Marzi and Messaoudi. This is an open-access article distributed under the terms of the Creative Commons Attribution License (CC BY). The use, distribution or reproduction in other forums is permitted, provided the original author(s) and the copyright owner(s) are credited and that the original publication in this journal is cited, in accordance with accepted academic practice. No use, distribution or reproduction is permitted which does not comply with these terms.
*Correspondence: Ilhem Messaoudi, imessaou@uci.edu
†These authors have contributed equally to this work and share first authorship