- 1Department of Biotechnology, University of Verona, Verona, Italy
- 2Department of Biological Sciences, University of Calgary, Calgary, AB, Canada
- 3Department of Biological, Chemical and Pharmaceutical Sciences and Technologies (STEBICEF), University of Palermo, Palermo, Italy
Bacillus mycoides SeITE01 is an environmental isolate that transforms the oxyanion selenite (
Introduction
Bacillus mycoides SeITE01 is an aerobic rod-shaped endospore-forming Gram-positive bacterium belonging to the Firmicutes phylum that was isolated from the rhizosphere of the Se-hyperaccumulator plant Astragalus bisulcatus grown in seleniferous soils (Vallini et al., 2005). SeITE01 strain shows the ability to withstand high concentrations of (up to 25mM), reducing this oxyanion into the insoluble and less bioavailable elemental selenium (Se0) with the generation of biogenic selenium nanoparticles (Bio-SeNPs) (Lampis et al., 2014; Piacenza et al., 2019; Bulgarini et al., 2020). In recent years, SeNPs have been the subject of great interest due to their attractive characteristics. These nanostructures can be used in both the technological and industrial fields thank to their special physical features, such as semiconducting, photoelectric, and X-ray-sensing properties (Wadhwani et al., 2016). At the same time, it was shown that Bio-SeNPs can exert an efficient and high antibacterial activity against human pathogens, such as Escherichia coli, Pseudomonas aeruginosa, and Staphylococcus aureus (Zonaro et al., 2015; Cremonini et al., 2016; Piacenza et al., 2017) and inhibit the formation of bacterial biofilms on medical-hospital devices (Sonkusre and Cameotra, 2015).
While there has been progress in establishing mechanisms of metal ion toxicity to bacteria (Lemire et al., 2013), a complete understanding of all the biochemical processes from various metal(loid) ion exposures is far from complete. Various “-omics” approaches can be employed to help fill the knowledge gaps. The use of metabolomics to elucidate cell responses to metal exposure began about a decade ago (Booth et al., 2011a). Metabolomics are a relatively recent addition to the “-omics” toolbox with the advent of improvements in technologies, allowing it to be a growing discipline in the field of biological systems (Dettmer and Hammock, 2004). Complementing other “-omics”, this powerful tool studies the turnover of biochemicals in living cells (Villas-Bôas et al., 2007; Baidoo et al., 2012). Since metabolites are subjected to continuous turnover, their levels and distributions can have enormous spatial and temporal variability. Some metabolites can accumulate within cells and be highly abundant (in the range of mM), while others may be quickly transformed and/or consumed and be present only in small traces (in the order of pM; Warwick and Ellis, 2005; Baidoo et al., 2012). Consequently, the metabolite concentration and flux through various biochemical networks can provide integrative information on the physiological state and response to stress of a living organism. Several studies have been conducted to examine the metabolic responses of different bacterial strains exposed to metals, such as cadmium (Cd), copper (Cu), aluminum (Al), gallium (Ga) (Beriault et al., 2006; Lemire et al., 2008; Booth et al., 2011a; Zhai et al., 2018), and the metalloid oxyanion tellurite () (Tremaroli et al., 2009). These investigations analyzed the relationship between the metal stress and the bacterial behavior comparing free swimming planktonic populations with surface-attached biofilms (Booth et al., 2011b) or wild-type cells with mutants (Tremaroli et al., 2009). These studies have revealed multiple effects exerted by metals into bacterial cells in terms of biochemical changes and reconfiguration of cell metabolism (Beriault et al., 2006; Lemire et al., 2008; Tremaroli et al., 2009; Booth et al., 2011b; Zhai et al., 2018). Although the results obtained have demonstrated the ability to distinguish and describe the diverse strain phenotypes in response to the exposure of different metals, these metabolomics analyses used an end-point approach. In fact, most attention has been given to the quantitative end-point study of metal(loid) resistance and tolerance of different microbial strains (Beriault et al., 2006; Lemire et al., 2008; Tremaroli et al., 2009; Booth et al., 2011b; Zhai et al., 2018) and less work has asked how the different metal(loid) ions exert their toxic effects (Lemire et al., 2013).
In the present study, SeITE01 cells growing in the presence of were evaluated at different stages of growth. Liquid Chromatography Mass Spectrometry (LC-MS) (Haggarty and Burgess, 2017; Kamphorst and Lewis, 2017; Kamal and Sharad, 2018) was used to find metabolite changes in B. mycoides SeITE01 cultures treated or not with Na2SeO3 in order to evaluate the effect of oxyanion on bacterial cells while they are reducing it with the generation of Bio-SeNPs along a 48-h time course.
Materials and Methods
Culture Media, Chemicals, and Solutions
Oxoid™ Nutrient Broth (NB) and Oxoid™ Agar Bacteriological were provided by Thermo Fisher Scientific™ (Ontario, Canada). Chemicals at the analytical grade were purchased from Merck KGaA (Ontario, Canada). Na2SeO3 was prepared as a 500mM stock solution in deionized water and sterilized by filtration (0.2μm; Sarstedt Inc., Fisher Scientific™). Phosphate-buffered saline (PBS) solution was prepared at the final concentration of 100mM and pH=7.4, while methanol and double distilled water (MetOH-ddH2O) have been mixed in a v/v ratio 1:1.
Bacterial Strain and Growth Conditions
Bacillus mycoides SeITE01 was aerobically pre-cultured for 24h at 27°C on an orbital shaker (150rpm; G10 Gyrotory Shaker, New Brunswick Scientific CO., Inc.) in 50-ml Erlenmeyer flasks containing 20ml of the rich medium NB. Na2SeO3 stock solution (500mM) was added to the culture media at the final concentration of 2.0mM. Bacterial growth was carried out in 250-ml Erlenmeyer flasks containing 100ml of NB supplied or not with 2.0mM (namely, -treated and untreated, respectively) and inoculated with pre-cultured cells at an optical density (OD600; Hitachi U-2000 Spectrophotometer) of 0.01. All microbiological experiments were conducted in biological triplicates (n=3).
Evaluation of Bacterial Growth and Depletion
Growth profiles of SeITE01 cultured in presence or absence of Na2SeO3 were evaluated at different time points, namely, after 3, 6, 9, 12, 18, 24, and 48h of incubation. Growth was monitored by Colony Forming Units (CFU) counting on Nutrient Agar plates and reported as the logarithm of the CFU per milliliter (Log10 CFU/ml) of culture with standard deviation (SD). depletion in the medium was determined spectrophotometrically (Varian Cary® 50 Bio UV–Vis) as previously described (Kessi et al., 1999; Lampis et al., 2014). concentration was evaluated by measuring the absorbance of the organic phase at 377nm of the Se-2, 3-diaminonaphthalene complex in cyclohexane, using a 1-cm path length quartz cuvette (Hellma® Analytics) against a calibration curve (R2=0.9876) calculated as average value (n=3) and constructed by using 0, 50, 100, 150, and 200nmol of dissolved in liquid NB medium.
TEM Analysis
The imaging of SeITE01 cells was performed using a Hitachi H-7650 120kV transmission electron microscope (TEM) as described elsewhere (Piacenza et al., 2018). Aliquots (500μl) of bacterial cultures either supplied or not with were recovered at the same incubation times chosen for the metabolomics analysis (3, 12, 18, 24, and 48h) and centrifuged at 16,000g for 10min at 4°C. The obtained cell pellets were diluted in 10μl of ddH2O to reach a final CFU/ml value of 4×104, deposited on CF300-Cu-Carbon Film Copper grids, and air dried for 24-h prior to their observation.
Metabolite Extraction
Samples from untreated and -treated cultures were collected at 3, 12, 18, 24, and 48h of bacterial growth. Preparation of SeITE01 intracellular samples for both experimental conditions was always started from the same number of CFU equal to 2×106/ml. Cell pellets were centrifuged at 16,000g for 10min at 4°C, washed once with cold PBS solution, and immediately stored at −80°C until use. The metabolite extraction protocol involved taking and re-suspending the cell pellets in 100μl of a pre-cooled (−20°C) mixture of MetOH-ddH2O, followed by 1min of vortexing and 10min of centrifugation at 16,000g at 4°C. 80μl of the suspensions was then transferred into clean glass vials and analyzed. A different approach was adopted for the extracellular samples. 500μl of each sample was collected, centrifuged at 16,000g for 10min at 4°C to remove residual bacterial cells, transferred in new and clean tubes, and stored at −80°C until use. 50μl of the chilled supernatants was then added to 950μl of the cold MetOH-ddH2O in order to reach a dilution equal to 1:20. The suspensions were vortexed for 1min, centrifuged again at 16,000g for 10min at 4°C, and ultimately, 800μl was analyzed by LC-MS.
LC-MS Acquisition
Metabolites present in the extracts were separated using ultra high-performance liquid chromatography on a Thermo Scientific Vanquish Horizon UHPLC system. A binary mixture of 20mM ammonium formate at pH 3.0 in water (Solvent A) and 0.1% (v/v) formic acid in acetonitrile (Solvent B) was used in conjunction with a Syncronis™ HILIC LC column (100mm×2.1mm×2.1μm; Thermo Scientific). The following analytical gradient was used (with respect to percentage of solvent B) to achieve chromatographic separation: 100% from 0 to 2min; 100 to 80% from 2 to 7min; 80 to 5% from 7 to 10min; 5% from 10 to 12min; 5 to 100% from 12 to 13min; and 100% from 13 to 15min. High-resolution mass spectral data were obtained on a Thermo Scientific Q-Exactive™ HF Hybrid Quadrupole-Orbitrap mass spectrometer coupled to a Thermo Scientific Ion Max-S API Source. Data were acquired in negative ion full-scan mode from 50 to 750 mass to charge ratio (m/z) at 240,000 resolution with automatic gain control target of 3×106 ions and a maximum injection time of 200ms. Identification and relative quantification of both intracellular and extracellular metabolites were carried out with the open source software Metabolomic Analysis and Visualization ENgine (MAVEN; Melamud et al., 2010). Metabolite peak assignments were determined by matching the previously established m/z and retention time (RT) of authentic standards with observed metabolite signals.
Statistical Analysis
Graphic representation of the clustered heat maps of raw data for the intracellular and extracellular dataset was obtained with R-3.3.3 software.1
Identification of metabolites whose concentration varied significantly between treatment conditions from the analysis of their temporal changes was performed with two advanced statistical approaches. They were carried out with the open source platform for statistical computing and graphics R (version 3.6.0) run under the free integrated development environment RStudio (version 1.0.153).2 The first method exploited multivariate empirical Bayes statistics to test the null hypothesis that the two expected profiles were the same. A T2 statistics equivalent to the two-sample Hotelling T2 statistics have been derived by considering a degree of moderation of the variance-covariance matrices toward a common matrix which retained the temporal correlation structure of the data (Tai and Speed, 2006). A ranking of metabolites’ profiles that varied at most in time between the cultures supplemented and not with was then computed. The top 10% of compounds in this ranking were considered for further analyses. The full algorithms are available in the time-course R package (Tai, 2019). The second approach was instead developed in-house. Data analysis started by considering each metabolite a multidimensional vector of time-course data samples, exploiting Principal Component Analysis (PCA) to identify the temporal dimensions where the samples varied at most. The vectors deriving from the Euclidean distances between untreated and -treated samples were expected to follow a Rayleigh distribution that therefore defined the null hypothesis for statistical comparisons (fitdistrplus package, Delignette-Muller and Dutang, 2015). A conservative significance threshold was set at p<10−4 for the rejection of the null hypothesis. Ultimately, metabolites identified by these statistical approaches were compared using Venn diagrams and used for the reconstruction of the final clustered heat maps. One further concern was that the raw data showed narrow peaked distributions with very long tails. To prevent a few metabolites from dominating the statistical comparisons because of high leverage, bestNormalize package was used to search for the best normalization procedure (Peterson, 2019). Intracellular metabolites were then normalized applying the BoxCox parametric transform (Zar, 2010) implemented in the geoR package (Ribeiro and Diggle, 2018), whereas for extracellular metabolites were used the Ordered Quantile normalization transformation (Bartlett, 1947) provided by the orderNorm function implemented in the bestNormalize package.
Results
Growth Under Exposure
Evaluation of the growth of B. mycoides SeITE01 exposed or not to 2.0mM Na2SeO3 is shown in Figure 1A. Bacterial cultures in absence of displayed a short lag phase of 3h, while stationary phase was reached after about 18h. On the other hand, the growth dynamics and final cells yield were negatively affected by the presence of . An extended lag phase between 6h and 12h was observed, and both exponential and stationary phases were delayed to around 18h and 24h, respectively. Moreover, in the exposed samples, the death phase was reached after 48h, while in the untreated ones, an extended stationary phase up to 96h was observed. Data description is congruent with that previously presented in Vallini et al. (2005) and Lampis et al. (2014).
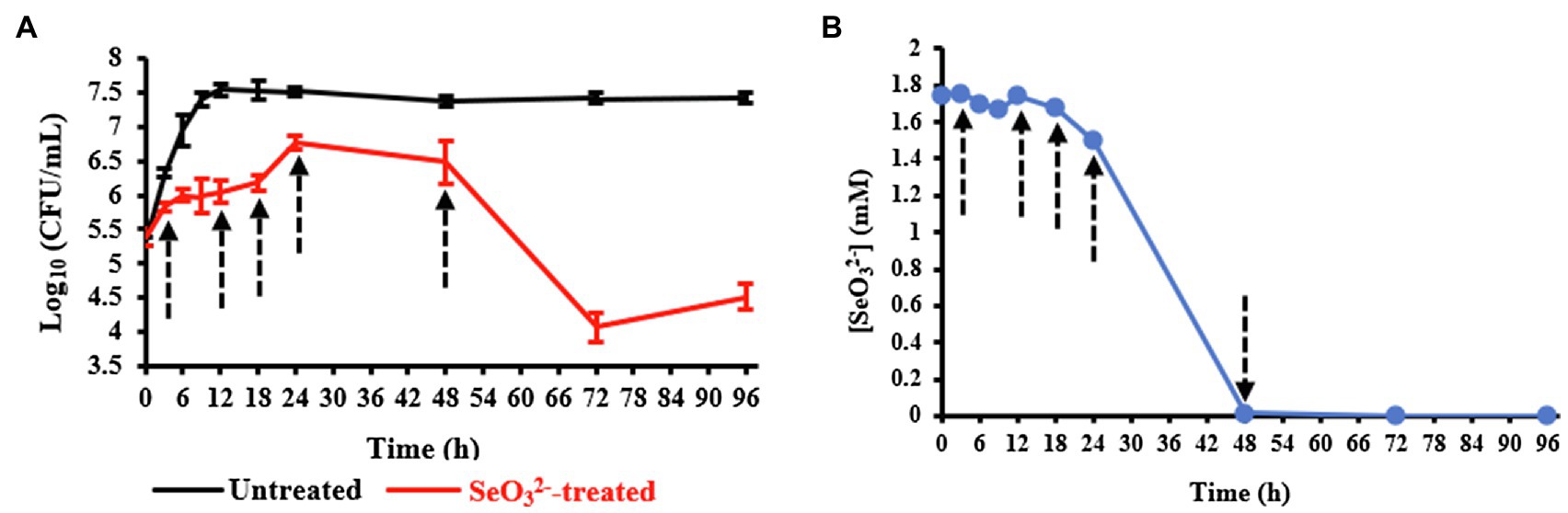
Figure 1. Panel (A) shows the growth profiles of Bacillus mycoides SeITE01 supplied or not with 2.0mM Na2SeO3. Panel (B) displays consumption by B. mycoides SeITE01 culture over time. Dotted arrows indicate the time points chosen for metabolomics investigation.
Upon evaluating the levels in the spent medium with time, oxyanion initially added was primarily depleted by cells after the stationary phase was reached (24-h time point) as shown in Figure 1B. Data obtained from both the growth curves and consumption allowed us to identify specific time points to be used for metabolomics investigation in order to capture relevant metabolic changes experienced by SeITE01 cells in -treated conditions and during the oxyanion bio-reduction process.
Cells Morphology Analysis in Presence of and Bio-SeNPs Detection
Temporal evolution of the morphology of untreated andtreated SeITE01 cells was investigated by TEM microscopy (Supplementary Figures 1 and 2). Untreated cells revealed expected development during the entire time course (Supplementary Figures 1A–E), exhibiting a rod-shaped morphology, which is typical of Bacillus sp. (Lampis et al., 2014). Growth in presence of (Supplementary Figure 2) induced, instead, some changes in cell morphology, especially in the early stages of growth (Supplementary Figures 2A–C). The typical rod-shaped morphology was reached by stationary phase (24h). At this time point, it was possible to observe nanostructures (spherical black or dark gray spots due to their electron dense nature) in both intracellular and extracellular space (Supplementary Figure 2D), which were not detected in untreated cell samples collected at the same time point, and that can be ascribed to SeNPs. By 48h, a considerable increase in the number of nanoparticles was found outside the bacterial cells (Supplementary Figure 2E). The appearance of Bio-SeNPs correlated with the depletion of observed between 24 and 48h as shown in Figure 1B.
Metabolomics Investigation
Our experiments were outlined to study the change of the biochemical state of SeITE01 cells at different points along the growth curve, namely, at beginning (3h) and end (12h) of lag phase, at early (18h) and late (24h) exponential phase and, finally, once they had reached stationary phase (48h) (Figure 1). Following metabolomic analysis, 125 compounds associated with the bacterial cell pellets (intracellular metabolites; >Supplementary Figure 3A) and 124 recurring in the cell free spent culture medium (extracellular metabolites; Supplementary Figure 3B) were initially identified. Graphical representations of the clustered heat maps underlined the complexity of the data. To identify significant variations in bacterial strain SeITE01 metabolism associated with -exposure during the time course, data were analyzed by two robust statistical approaches: the combination of PCA with the Squared Euclidean Distance (Supplementary Figure 4, Supplementary Table 1) and the integration of Bayesian Inference with the T2 statistics (Supplementary Table 2). These analyses provided two lists of 16 and 19 statistically relevant metabolites from the intracellular and extracellular samples, respectively. Data were then compared through Venn diagrams to graphically identify metabolites in common with the two statistical approaches (Supplementary Figure 5) and used for the reconstruction of the final clustered heat maps (Figure 2). The two categories of samples showed distinct differences in both their trends during the time course and the metabolic pathways they are involved in.
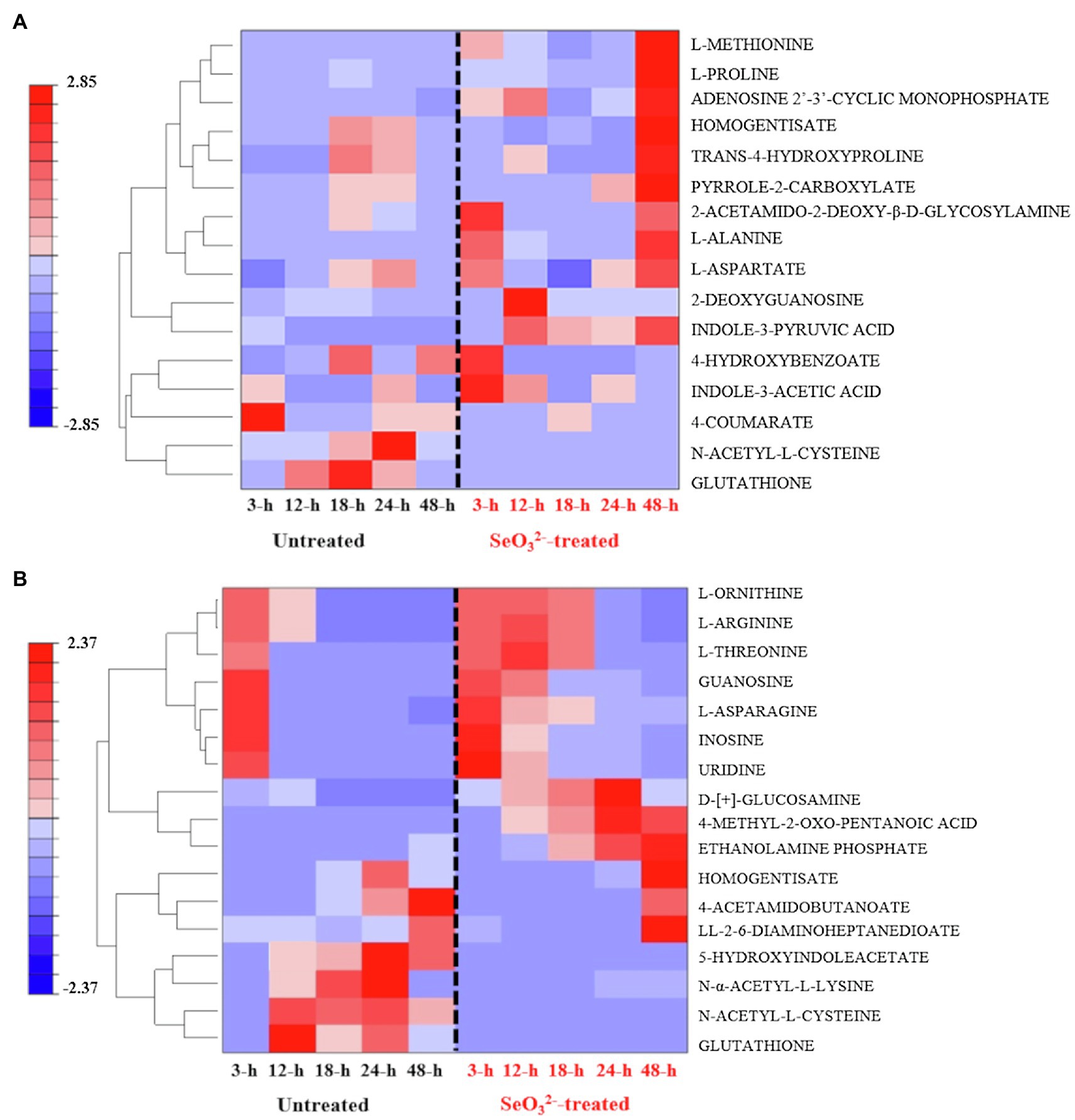
Figure 2. Clustered heat maps of the most statistically relevant intracellular (A) and extracellular (B) metabolites of B. mycoides SeITE01 identified by the two statistical approaches.
Intracellular Metabolites
Two key temporal responses can be observed within intracellular compounds of -treated cells: an early (3h) and a late response (48h). Metabolites associated with the first response were 4-hydroxybenzoate (4-HB) and indole-3-acetic acid (IAA), while L-proline and trans-4-hydroxyproline belong to the second one. In the case of untreated bacterial cells, it was not possible to identify families of metabolites showing a definitive temporal trend. However, attention must mostly be paid to glutathione (GSH) and N-acetyl-L-cysteine (NAC), which are lacking in -treated cells (Figure 2A).
Extracellular Metabolites
Distribution of metabolites belonging to the extracellular dataset showed a characteristic behavior pattern of metabolites consumed or produced (Figure 2B). Compounds in the lower part of the clustered heat map displayed an identical temporal evolution between the two treatments (i.e., L-serine, L-aspartate, and 5-oxo-D-proline), while in the upper portion, differences are recognizable with some metabolites present only in the untreated samples (i.e., N-α-acetyl-L-lysine and succinate), while others are observed only in -treated cultures (i.e., mono methyl glutarate, 3-dehydroshikimate, and D-ribose). Analysis of the most significant metabolites detected under exposure underlined the presence of four main classes of macromolecules: thiol redox and signaling molecules [e.g., GSH, NAC, and 5-hydroxyindoleacetate (5-HIAA)]; purine derivative, such as guanosine; amino acids (e.g., L-threonine and L-ornithine) and amino compounds (e.g., ethanolamine phosphate and D-[+]-glucosamine); and α-keto acid as 4 methyl-2-oxo pentanoic acid.
Discussion
Metabolomics can be considered a powerful tool for understanding and providing clues toward hypothesis for describing a given phenotype. Nevertheless, it is very sensitive to experimental design, sample preparation, statistical data analysis, and interpretation. Here, our statistical evaluation of both intracellular and extracellular datasets was complex. Cell culturing in rich NB medium would lead to a variety of metabolic pathways active, while the use of three different variables (untreated and -treated cultures, and time) prevented an ease use of classic statistical approaches so far applied for metabolomics studies. The challenge was to evaluate not only a possible difference in metabolites between -treated and untreated cultures, but also to see how this difference varies over the time course, possibly recognizing metabolites involved in bio-reduction process. The analysis of clustered heat maps deriving from the statistical processing of datasets (Figure 2) together with the graphic representation of the statistically relevant metabolites corresponding to different growth states allowed for the identification of the biomolecules that changed in the cell pellets (intracellular metabolites; Figure 3) and cell free spent medium (extracellular metabolites; Figure 4) samples. It is important to mention that interpretation of extracellular metabolites and identification of a possible relationship between these compounds and -oxyanion effect requires considerably more caution than the elucidation of the intracellular pool. Extracellular compounds, in fact, can be the result of selective nutrient import, active efflux of metabolites from the cytoplasm, cell membrane leakage due to osmotic stress, or cell death leading to complete release of all cell constituents. Below follows the time course of the experiment of growth of SelTE01 under selenite exposure.
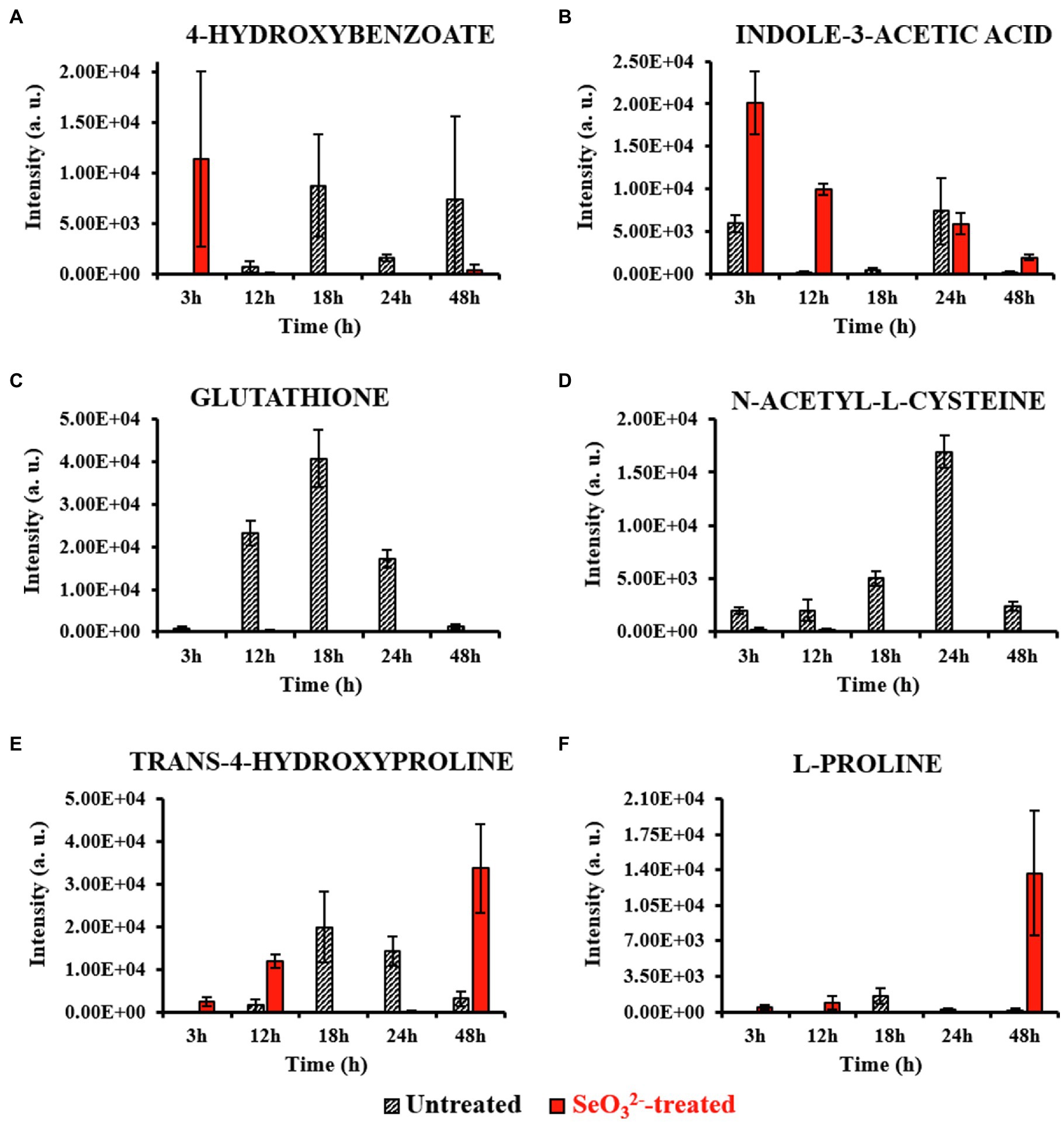
Figure 3. Most relevant intracellular metabolites. This figure shows the intensity trends of metabolites from both untreated and -treated cells during the time course. Panels (A,B) show metabolites present in the earliest stage of growth (3h). Panels (C,D) show metabolites accumulated during the end of lag (12h) and beginning of exponential (18h) phases. Panels (E,F) show metabolites present late in stationary phase (48h) and during Bio-SeNPs release.
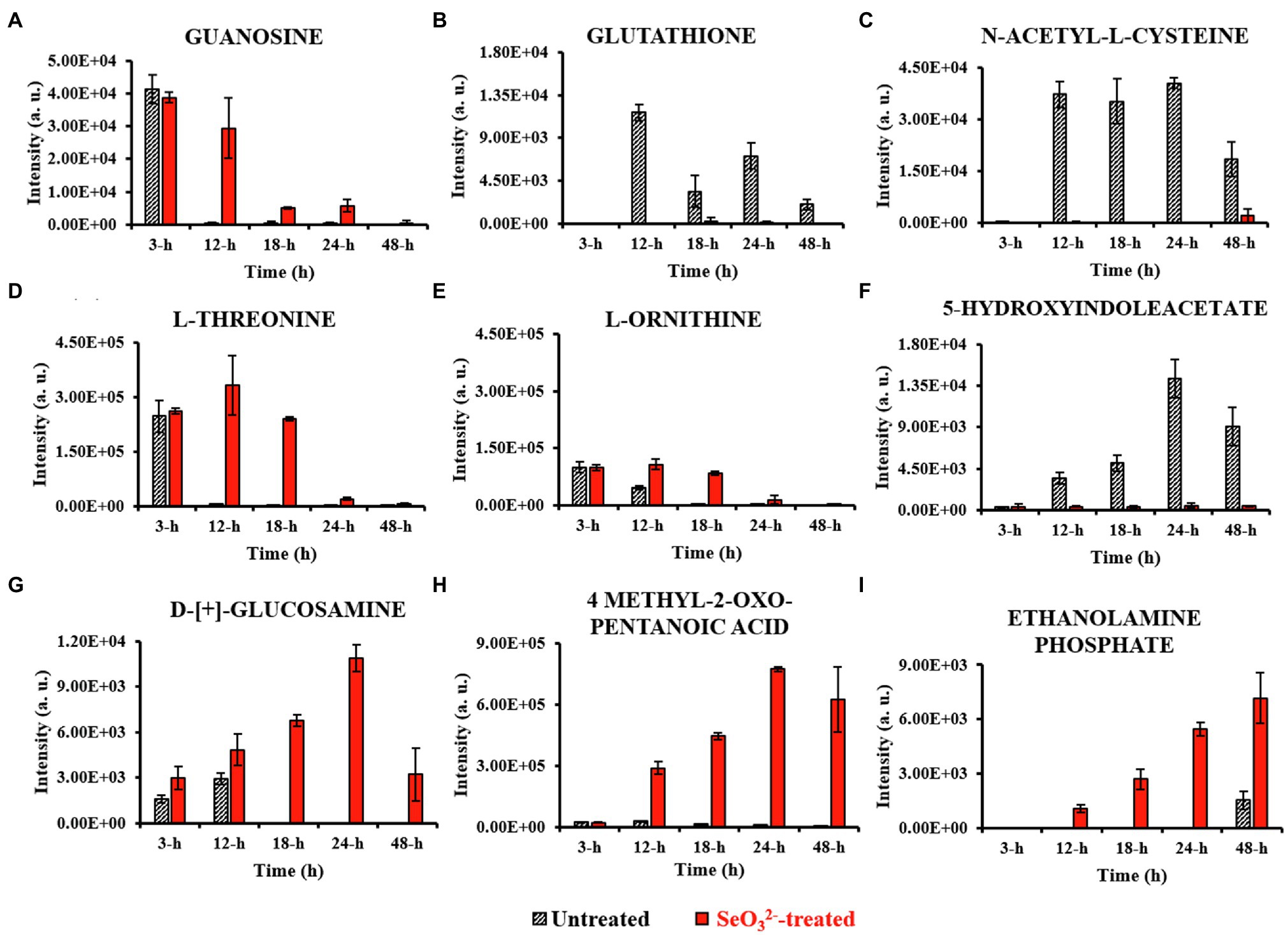
Figure 4. Most relevant extracellular metabolites. This figure shows the intensity trends of metabolites from both untreated and -treated cells during the time course. Panel (A) shows metabolite present in the earliest stage of growth (3h). Panels (B–E) show metabolites extruded during lag (12h) and exponential (18h) stages. Panels (F–H) represent metabolites detected during stationary stage (24h), while panel (I) describes metabolite secreted in late stationary phase (48h).
Lag phase of growth shows the initial uptake of by SeITE01 cells can be traced back to the earliest stage of growth, the 3-h time point. The oxyanion’s transport into cellular compartments is accompanied by a change in cell morphology (Supplementary Figure 2A), an increase in intracellular levels of 4-HB and IAA (Figures 3A,B), and the extracellular accumulation of guanosine (Figure 4A). 4-HB is a precursor of the primary electron transport chain carrier ubiquinone (Q) also involved in gene regulation and oxygen radical scavenging (Søballe and Poole, 1999). Its accumulation in -treated cells suggests an oxidative stress role induced by , acting as antioxidant molecule and ROS scavenger. Moreover, Sévin and Sauer (2014) have demonstrated that the intracellular accumulation of ubiquinone in E. coli can elicit osmotic-stress tolerance through the modification of cell membrane composition. IAA is an ubiquitous signaling molecule responsive to different stress conditions (Somers et al., 2005; Bianco et al., 2006; Zarkan et al., 2020). Finally, we see guanosine, which can play regulatory roles in stress response, biofilm formation, and cellular damage protection (Cornforth and Foster, 2013; Rowlett et al., 2017; Bange and Bedrunka, 2020). Thus, the observations at this growth phase suggest the cells elucidating general stress response adapting to the loaded environment.
From the end of the lag (12h) and exponential (18h) growth phases under selenite exposure, we see uptake but no conversion to Se0 (Figure 1B), and cells continue to present structural malformation (Supplementary Figures 2B,C). However, the detection of reductive thiol (RSH) compounds only in untreated samples and their total absence in the exposed ones allows us to hypothesize that has started reactions with these types of molecules, as expected. GSH (Figures 3C, 4B) and NAC (Figures 3D, 4C) were found at high levels both intracellularly and extracellularly for untreated samples, yet essentially absent from exposed ones. It is recognized that GSH and related thiol compounds are notoriously challenging to accurately quantify (Lu et al., 2017). Besides, it is known that RSH molecules are important for chalcogen chemistry through involvement in Painter reduction reactions of to Se0. In this way, the total absence of GSH and NAC in -treated samples throughout the entire time course supports the literature observations that these metabolites are rapidly consumed (Painter, 1941; Ganther, 1971; Kessi et al., 1999; Kessi and Hanselmann, 2004; Kessi, 2006) and they may be involved in proactive toxicity. Furthermore, they act as a substrate or reducing sources to scavenge ROS produced as a consequence of reactions and cell damage, playing a defensive role (Turner et al., 1998). Extracellularly, we see the amino acids L-threonine (Thr) and ornithine (Orn) changing (Figures 4D,E). Orn is of interest as it plays numerous roles in cells as a biosynthetic precursor of arginine linked to the urea cycle (Stalon et al., 1987; Reitzer, 2009). It is also a key to the biosynthesis of polyamines, a class of compounds involved in a variety of cellular processes, such as gene expression, cell growth, survival, and stress response (Gevrekci, 2017). Additionally, Orn can be used for the synthesis of phosphorus-free ornithine lipids, which are alternative membrane lipids activated under stress and widespread among eubacteria (Sohlenkamp and Geiger, 2016; Sohlenkamp, 2019). Combining these growth phase observations, we can postulate that is reacting with RSH molecules, and subsequent stress response may be in part dealt with changes in membrane lipids.
Stationary phase (24h) marks the beginning of the SeNPs formation and extracellularly accumulation and the cells adapting a normal rod-shape morphology (Supplementary Figure 2D). 5-HIAA is an indole derivative with roles in virulence, cell cycle regulation, acid, pH and heat resistance, and a signaling molecule in biofilm formation (Hu et al., 2010; Lee and Lee, 2010; Zarkan et al., 2020). Cellular survival in this stage of growth is deeply linked to both energy metabolism and stress resistance (Wang et al., 2001; Hu et al., 2010; Gaimster et al., 2014; Zarkan et al., 2020). D-[+]-glucosamine (GlcN) and 4-methyl-2-oxo pentanoic acid are observed, increasing up to 24h. GlcN is a non-acetylated amino sugar whose acetylated form is one of the two components of peptidoglycan (Vollmer et al., 2008). In other species of Bacillus, the presence of this metabolite followed the activation of defense responses against external agents (Psylinakis et al., 2005; Vollmer, 2008). Under stressful conditions, bacterial cells can activate the peptidoglycan turnover, a phenomenon known as cell wall recycling (Reith and Mayer, 2011). In Bacillus species, the predominant fatty acid species of membrane lipids are represented by the branched-chain fatty acids (BCFAs) (de Mendoza et al., 2002; Tojo et al., 2005), where this metabolite represents a precursor (Lowe et al., 1983; Belitsky, 2015). Under adverse states, bacterial cells can adjust BCFA composition to regulate membrane fluidity, allowing survival in a wide range of physical and chemical environments (Singh et al., 2008). Zhu et al. (2014) demonstrated that both B. subtilis and S. aureus responded to carbon nanotubes (CNTs) toxic stress through changing their fatty acid composition. The modification helped to compensate for the fluidizing effect of nanostructures on the cytoplasmic membrane making it more “rigid” which conforms to the early theory of “homeoviscous adaptation” described by Sinensky (1974). These observations may reflect the signaling around the stress of release of Se atoms and SeNPs from the cytoplasm out of the cell where one can imagine would be disruptive toward cell wall and membrane envelope.
Well after stationary phase (48h), we see the full conversion of to Se0 and subsequent increase of SeNP sizes and quantity in the extracellular space (Supplementary Figure 2E). This is accompanied by intracellular accumulation of trans-4-hydroxyproline (Hyp) and L-proline (Pro) (Figures 3E,F), which show osmoprotectant activity (Kim et al., 2017), as well as extracellular accumulation of the amino compound ethanolamine phosphate (Figure 4I). Ethanolamine phosphate is in the phosphatidyl ethanolamine (PE) pathway for this key lipid head group (Kaval and Garsin, 2018). Both of these observations suggest further membrane adaptation and protection likely from the stress of releasing the SeNPs through the cell barrier.
Conclusion
In the present study, untargeted metabolomics analysis was adopted to explore the effects of the oxyanion on the cells of the Gram-positive bacterium Bacillus mycoides SeITE01. This study suggests that this strain faces the toxic effect of by activating several stress defense systems during the growth and through exposure, reduction, and SeNPs production. The identified metabolites were consistent with the hypothesis of intracellular accumulation of osmo-protective solutes and antioxidants, the activation of ROS scavengers, as well as compounds that participate in stabilizing the cytoplasmic membrane. Furthermore, in the cell free spent medium (extracellular), there was a change in metabolites related to oxidative stress, signaling, stress linked amino acids and metabolites involved in modifications of bacterial membranes lipids and cell walls.
Data Availability Statement
The original contributions presented in the study are included in the article/Supplementary Material, and further inquiries can be directed to the corresponding author.
Author Contributions
GB, SL, and RT designed the study. GB conducted all the experiments. IL made the metabolomics equipment available. RG analyzed the samples with LC-MS. RC performed the statistical analysis on the datasets. GB, EP, and AP collected the TEM images. GB and RT wrote the manuscript. GV provided his senior authorship by supervising the ultimate reading of the manuscript. All authors approved the submitted version.
Funding
We acknowledge financial support by the Internationalization award of University of Verona for travel to University of Calgary for data collection. SL was supported by JP2017 grant from the University of Verona. RT and IL recognize the Natural Sciences and Engineering Research council (NSERC) of Canada for Discovery grants supporting the research experiments.
Conflict of Interest
The authors declare that the research was conducted in the absence of any commercial or financial relationships that could be construed as a potential conflict of interest.
Publisher’s Note
All claims expressed in this article are solely those of the authors and do not necessarily represent those of their affiliated organizations, or those of the publisher, the editors and the reviewers. Any product that may be evaluated in this article, or claim that may be made by its manufacturer, is not guaranteed or endorsed by the publisher.
Acknowledgments
We thank Priyanka Mukherjee [Facility Coordinator of the Microscopy and Imaging Facility (MIF), University of Calgary] for her support in performing the TEM imaging.
Supplementary Material
The Supplementary Material for this article can be found online at: https://www.frontiersin.org/articles/10.3389/fmicb.2021.711000/full#supplementary-material
Footnotes
References
Baidoo, E. E. K., Benke, P. I., and Keasling, J. D. (2012). “Mass Spectrometry-Based Microbial Metabolomics,” in Microbial Systems Biology. Methods in Molecular Biology (Methods and Protocols). Vol 881. ed. A. Navid (Totowa, NJ: Humana Press).
Bange, G., and Bedrunka, P. (2020). Physiology of guanosine-based second messenger signaling in Bacillus subtilis. Biol. Chem. 401, 1307–1322. doi: 10.1515/hsz-2020-0241
Belitsky, B. R. (2015). Role of branched-chain amino acid transport in Bacillus subtilis CodY activity. J. Bacteriol. 197, 1330–1338. doi: 10.1128/JB.02563-14
Beriault, R., Hamel, R., Chenier, D., Mailloux, R., Joly, H., and Appanna, V. (2006). The overexpression of NADPH-producing enzymes counters the oxidative stress evoked by gallium, an iron mimetic. Biometals 20, 165–176. doi: 10.1007/s10534-006-9024-0
Bianco, C., Imperlini, E., Calogero, R., Senatore, B., Amoresano, A., Carpentieri, A., et al. (2006). Indole-3-acetic acid improves Escherichia coli’s defences to stress. Arch. Microbiol. 185, 373–382. doi: 10.1007/s00203-006-0103-y
Booth, S. C., Workentine, M. L., Weljie, A. M., and Turner, R. J. (2011a). Metabolomics and its application to studying metal toxicity. Metallomics 3, 1142–1152. doi: 10.1039/c1mt00070e
Booth, S. C., Workentine, M. L., Wen, J., Shaykhutdinov, R., Vogel, H. J., Ceri, H., et al. (2011b). Differences in metabolism between the biofilm and planktonic response to metal stress. J. Proteome Res. 10, 3190–3199. doi: 10.1021/pr2002353
Bulgarini, A., Lampis, S., Turner, R. J., and Giovanni, V. (2020). Biomolecular composition of capping-layer and stability of biogenic selenium nanoparticles synthesized by both Gram-positive and Gram-negative bacteria. Microb. Biotechnol. 14, 198–212. doi: 10.1111/1751-7915.13666
Cornforth, D. M., and Foster, K. R. (2013). Competition sensing: the social side of bacterial stress responses. Nat. Rev. Microbiol. 11, 285–293. doi: 10.1038/nrmicro2977
Cremonini, E., Zonaro, E., Donini, M., Lampis, S., Boaretti, M., Dusi, S., et al. (2016). Biogenic selenium nanoparticles: characterization, antimicrobial activity and effects on human dendritic cells and fibroblasts. Microb. Biotechnol. 9, 758–771. doi: 10.1111/1751-7915.12374
de Mendoza, D., Schujman, G. E., and Aguilar, P. S. (2002). “Biosynthesis and function of membrane lipids,” in Bacillus subtilis and Its Closest Relatives: From Genes to Cells. eds. A. L. Sonenshein, J. A. Hoch, and R. Losick (Washington, DC: American Society for Microbiolgy Press), 43–55.
Delignette-Muller, M. L., and Dutang, C. (2015). Fitdistrplus: an R package for fitting distributions. J. Stat. Softw. 64, 1–34. doi: 10.18637/jss.v064.i04
Dettmer, K., and Hammock, B. D. (2004). Metabolomics-A new exciting field within the “omics” sciences. Environ. Health Perspect. 112, A396–A397. doi: 10.1289/ehp.112-1241997
Gaimster, H., Cama, J., Hernández-Ainsa, S., Keyser, U. F., and Summers, D. K. (2014). The indole pulse: a new perspective on indole signalling in Escherichia coli. PLoS One 9:e93168. doi: 10.1371/journal.pone.0093168
Ganther, E. H. (1971). Reduction of the selenotrisulfide derivative of glutathione to a persulfide analog by glutathione reductase. Biochemistry 10, 4089–4098. doi: 10.1021/bi00798a013
Gevrekci, A. Ö. (2017). The roles of polyamines in microorganisms. World J. Microbiol. Biotechnol. 33:204. doi: 10.1007/s11274-017-2370-y
Haggarty, J., and Burgess, K. E. V. (2017). Recent advances in liquid and gas chromatography methodology for extending coverage of the metabolome. Curr. Opin. Biotechnol. 43, 77–85. doi: 10.1016/j.copbio.2016.09.006
Hu, M., Zhang, C., Mu, Y., Shen, Q., and Feng, Y. (2010). Indole affects biofilm formation in bacteria. Indian J. Microbiol. 50, 362–368. doi: 10.1007/s12088-011-0142-1
Kamal, S., and Sharad, W. (2018). Step-up in liquid chromatography from HPLC to UPLC: a comparative and comprehensive review. J. Pharm. Innov. 7, 342–347.
Kamphorst, J. J., and Lewis, I. A. (2017). Editorial overview: Recent innovations in the metabolomics revolution. Curr. Opin. Biotechnol. 43, iv–vii. doi: 10.1016/j.copbio.2017.01.005
Kaval, K. G., and Garsin, D. A. (2018). Ethanolamine utilization in bacteria. MBio 9:e00066-18. doi: 10.1128/mBio.00066-18
Kessi, J. (2006). Enzymic systems proposed to be involved in the dissimilatory reduction of selenite in the purple non-sulfur bacteria Rhodospirillum rubrum and Rhodobacter capsulatus. Microbiology 152, 731–743. doi: 10.1099/mic.0.28240-0
Kessi, J., and Hanselmann, K. W. (2004). Similarities between the abiotic reduction of selenite with glutathione and the dissimilatory reaction mediated by Rhodospirillum rubrum and Escherichia coli. J. Biol. Chem. 279, 50662–50669. doi: 10.1074/jbc.M405887200
Kessi, J., Ramuz, M., Wehrli, E., Spycher, M., and Bachofen, R. (1999). Reduction of selenite and detoxification of elemental selenium by the phototrophic bacterium Rhodospirillum rubrum. Appl. Environ. Microbiol. 65, 4734–4740. doi: 10.1128/AEM.65.11.4734-4740.1999
Kim, K. H., Jia, B., and Jeon, C. O. (2017). Identification of trans-4-hydroxy-l-proline as a compatible solute and its biosynthesis and molecular characterization in Halobacillus halophilus. Front. Microbiol. 8:2054. doi: 10.3389/fmicb.2017.02054
Lampis, S., Zonaro, E., Bertolini, C., Bernardi, P., Butler, C. S., and Vallini, G. (2014). Delayed formation of zero-valent selenium nanoparticles by Bacillus mycoides SeITE01 as a consequence of selenite reduction under aerobic conditions. Microb. Cell Factories 13:35. doi: 10.1186/1475-2859-13-35
Lee, J.-H., and Lee, J. (2010). Indole as an intercellular signal in microbial communities. FEMS Microbiol. Rev. 34, 426–444. doi: 10.1111/j.1574-6976.2009.00204.x
Lemire, J., Harrison, J. J., and Turner, R. J. (2013). Antimicrobial activity of metals: mechanisms, molecular targets and applications. Nat. Rev. Microbiol. 11, 371–384. doi: 10.1038/nrmicro3028
Lemire, J., Kumar, P., Mailloux, R., Cossar, K., and Appanna, V. D. (2008). Metabolic adaptation and oxaloacetate homeostasis in P. fluorescens exposed to aluminum toxicity. J. Basic Microbiol. 48, 252–259. doi: 10.1002/jobm.200800007
Lowe, P. N., Hodgson, J. A., and Perham, R. N. (1983). Dual role of a single multienzyme complex in the oxidative decarboxylation of pyruvate and branched-chain 2-oxo acids in Bacillus subtilis. Biochem. J. 215, 133–140. doi: 10.1042/bj2150133
Lu, W., Su, X., Klien, M. S., Lewis, I. A., Fiehn, O., and Rabinowitz, J. D. (2017). Metabolite measurement: pitfalls to avoid and practices to follow. Annu. Rev. Biochem. 86, 277–304. doi: 10.1146/annurev-biochem-061516-044952
Melamud, E., Vastag, L., and Rabinowitz, J. D. (2010). Metabolomic analysis and visualization engine for LC-MS data. Anal. Chem. 82, 9818–9826. doi: 10.1021/ac1021166
Painter, E. P. (1941). The chemistry and toxicity of selenium compounds with special reference to the selenium problem. Chem. Rev. 28, 179–213. doi: 10.1021/cr60090a001
Peterson, R. A. (2019). Ordered quantile normalization: a semiparametric transformation built for the cross-validation era. J. Appl. Stat. 47, 1–16. doi: 10.1080/02664763.2019.1630372
Piacenza, E., Presentato, A., Ambrosi, E., Speghini, A., Turner, R. J., Vallini, G., et al. (2018). Physical-chemical properties of biogenic selenium nanostructures produced by Stenotrophomonas maltophilia SeITE02 and Ochrobactrum sp. MPV1. Front. Microbiol. 9:3178. doi: 10.3389/fmicb.2018.03178
Piacenza, E., Presentato, A., Bardelli, M., Lampis, S., Vallini, G., and Turner, R. J. (2019). The influence of bacterial physiology on the processing of selenite, biogenesis of nanomaterials and their thermodynamic stability. Molecules 24:2532. doi: 10.3390/molecules24142532
Piacenza, E., Presentato, A., Zonaro, E., Lemire, J. A., Demeter, M., Vallini, G., et al. (2017). Antimicrobial activity of biogenically produced spherical Se-nanomaterials embedded in organic material against Pseudomonas aeruginosa and Staphylococcus aureus strains on hydroxyapatite-coated surfaces. Microb. Biotechnol. 10, 804–818. doi: 10.1111/1751-7915.12700
Psylinakis, E., Boneca, I. G., Mavromatis, K., Deli, A., Hayhurst, E., Foster, S. J., et al. (2005). Peptidoglycan N-acetylglucosamine deacetylases from Bacillus cereus, highly conserved proteins in Bacillus anthracis. J. Biol. Chem. 280, 30856–30863. doi: 10.1074/jbc.M407426200
Reith, J., and Mayer, C. (2011). Peptidoglycan turnover and recycling in Gram-positive bacteria. Appl. Microbiol. Biotechnol. 92, 1–11. doi: 10.1007/s00253-011-3486-x
Reitzer, L. (2009). “Amino Acid Synthesis,” in Encyclopedia of Microbiology. 3rd Edn. ed. M. Schaechter (Academic Press), 1–17.
Ribeiro, P. J., and Diggle, P. J. (2018). geoR: Analysis of geostatistical data. R package version 1.7-5.2.1. Available at: https://CRAN.R-project.org/package=geoR (Accessed January 2021).
Rowlett, V. W., Mallampalli, V. K. P. S., Karlstaedt, A., Dowhan, W., Taegtmeyer, H., Margolin, W., et al. (2017). Impact of membrane phospholipid alterations in Escherichia coli on cellular function and bacterial stress adaptation. J. Bacteriol. 199:e00849-16. doi: 10.1128/JB.00849-16
Sévin, D. C., and Sauer, U. (2014). Ubiquinone accumulation improves osmotic-stress tolerance in Escherichia coli. Nat. Chem. Biol. 10, 266–274. doi: 10.1038/nchembio.1437
Sinensky, M. (1974). Homeoviscous adaptation-A homeostatic process that regulates viscosity of membrane lipids in Escherichia coli. PNAS 71, 522–525. doi: 10.1073/pnas.71.2.522
Singh, V. K., Hattangady, D. S., Giotis, E. S., Singh, A. K., Chamberlain, N. R., Stuart, M. K., et al. (2008). Insertional inactivation of branched-chain -keto acid dehydrogenase in Staphylococcus aureus leads to decreased branched-chain membrane fatty acid content and increased susceptibility to certain stresses. Appl. Environ. Microbiol. 74, 5882–5890. doi: 10.1128/AEM.00882-08
Søballe, B., and Poole, R. K. (1999). Microbial ubiquinones: multiple roles in respiration, gene regulation and oxidative stress management. Microbiology 145, 1817–1830. doi: 10.1099/13500872-145-8-1817
Sohlenkamp, C. (2019). “Ornithine lipids and other amino acid-containing acyloxyacyl lipids,” in Biogenesis of Fatty Acids, Lipids and Membranes. Handbook of Hydrocarbon and Lipid Microbiology. ed. O. Geiger (Cham: Springer).
Sohlenkamp, C., and Geiger, O. (2016). Bacterial membrane lipids: diversity in structures and pathways. FEMS Microbiol. Rev. 40, 133–159. doi: 10.1093/femsre/fuv008
Somers, E., Ptacek, D., Gysegom, P., Srinivasan, M., and Vanderleyden, J. (2005). Azospirillum brasilense produces the auxin-like phenylacetic acid by using the key enzyme for indole-3-acetic acid biosynthesis. Appl. Environ. Microbiol. 71, 1803–1810. doi: 10.1128/AEM.71.4.1803-1810.2005
Sonkusre, P., and Cameotra, S. S. (2015). Biogenic selenium nanoparticles inhibit Staphylococcus aureus adherence on different surfaces. Colloids Surf. B. Biointerfaces 136, 1051–1057. doi: 10.1016/j.colsurfb.2015.10.052
Stalon, V., Vander Wauven, C., Momin, P., and Legrain, C. (1987). Catabolism of arginine, citrulline and ornithine by Pseudomonas and related bacteria. J. Gen. Microbiol. 133, 2487–2495. doi: 10.1099/00221287-133-9-2487
Tai, Y. C. (2019). Timecourse: statistical analysis for developmental microarray time course data. R package version 1.56.0. Available at: http://www.bioconductor.org (Accessed January 2021).
Tai, Y. C., and Speed, T. P. (2006). A multivariate empirical Bayes statistic for replicated microarray time course data. Ann. Stat. 34, 2387–2412. doi: 10.1214/009053606000000759
Tojo, S., Satomura, T., Morisaki, K., Deutscher, J., Hirooka, K., and Fujita, Y. (2005). Elaborate transcription regulation of the Bacillus subtilis ilv-leu operon involved in the biosynthesis of branched-chain amino acids through global regulators of CcpA, CodY and TnrA. Mol. Microbiol. 56, 1560–1573. doi: 10.1111/j.1365-2958.2005.04635.x
Tremaroli, V., Workentine, M. L., Weljie, A. M., Vogel, H. J., Ceri, H., Viti, C., et al. (2009). Metabolomic investigation of the bacterial response to a metal challenge. Appl. Environ. Microbiol. 75, 719–728. doi: 10.1128/AEM.01771-08
Turner, R. J., Weiner, J. H., and Taylor, D. E. (1998). Selenium metabolism in Escherichia coli. Biometals 11, 223–227. doi: 10.1023/A:1009290213301
Vallini, G., Di Gregorio, S., and Lampis, S. (2005). Rhizosphere-induced selenium precipitation for possible applications in phytoremediation of Se polluted effluents. Z. Naturforsch. 60, 349–356. doi: 10.1515/znc-2005-3-419
Villas-Bôas, S. G., Roessner, U., Hansen, M. A. E., Smedsgaard, J., and Nielsen, J. (2007). Metabolome Analysis: An Introduction. Hoboken: Wiley.
Vollmer, W. (2008). Structural variation in the glycan strands of bacterial peptidoglycan. FEMS Microbiol. Rev. 32, 287–306. doi: 10.1111/j.1574-6976.2007.00088.x
Vollmer, W., Blanot, D., and De Pedro, M. A. (2008). Peptidoglycan structure and architecture. FEMS Microbiol. Rev. 32, 149–167. doi: 10.1111/j.1574-6976.2007.00094.x
Wadhwani, S. A., Shedbalkar, U. U., Singh, R., and Chopade, B. A. (2016). Biogenic selenium nanoparticles: current status and future prospects. Appl. Microbiol. Biotechnol. 100, 2555–2566. doi: 10.1007/s00253-016-7300-7
Wang, D., Ding, X., and Rather, P. N. (2001). Indole can act as an extracellular signal in Escherichia coli. J. Bacteriol. 183, 4210–4216. doi: 10.1128/JB.183.14.4210-4216.2001
Warwick, B. D., and Ellis, D. I. (2005). Metabolomics: current analytical platforms and methodologies. Trends Anal. Chem. 24, 285–294. doi: 10.1016/j.trac.2004.11.021
Zarkan, A., Liu, J., Matuszewska, M., Gaimster, H., and Summers, D. K. (2020). Local and universal action: the paradoxes of indole signalling in bacteria. Trends Microbiol. 28, 566–577. doi: 10.1016/j.tim.2020.02.007
Zhai, Q., Xiao, Y., Narbad, A., and Chen, W. (2018). Comparative metabolomic analysis reveals global cadmium stress response of Lactobacillus plantarum strains. Metallomics 10, 1065–1077. doi: 10.1039/C8MT00095F
Zhu, B., Xia, X., Xia, N., Zhang, S., and Guo, X. (2014). Modification of fatty acids in membranes of bacteria: implication for an adaptive mechanism to the toxicity of carbon nanotubes. Environ. Sci. Technol. 48, 4086–4095. doi: 10.1021/es404359v
Keywords: Bacillus mycoides SeITE01, selenite, selenium nanoparticles, signaling molecules, time course, untargeted metabolomics
Citation: Baggio G, Groves RA, Chignola R, Piacenza E, Presentato A, Lewis IA, Lampis S, Vallini G and Turner RJ (2021) Untargeted Metabolomics Investigation on Selenite Reduction to Elemental Selenium by Bacillus mycoides SeITE01. Front. Microbiol. 12:711000. doi: 10.3389/fmicb.2021.711000
Edited by:
Nelson da Cruz Soares, University of Sharjah, United Arab EmiratesReviewed by:
Isao Yumoto, National Institute of Advanced Industrial Science and Technology (AIST), JapanSuereta Fortuin, Stellenbosch University, South Africa
Copyright © 2021 Baggio, Groves, Chignola, Piacenza, Presentato, Lewis, Lampis, Vallini and Turner. This is an open-access article distributed under the terms of the Creative Commons Attribution License (CC BY). The use, distribution or reproduction in other forums is permitted, provided the original author(s) and the copyright owner(s) are credited and that the original publication in this journal is cited, in accordance with accepted academic practice. No use, distribution or reproduction is permitted which does not comply with these terms.
*Correspondence: Silvia Lampis, c2lsdmlhLmxhbXBpc0B1bml2ci5pdA==