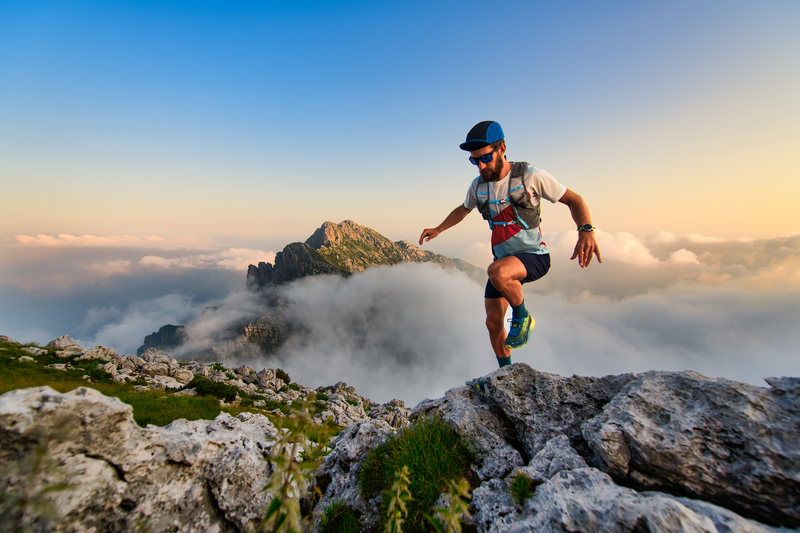
95% of researchers rate our articles as excellent or good
Learn more about the work of our research integrity team to safeguard the quality of each article we publish.
Find out more
REVIEW article
Front. Microbiol. , 22 June 2021
Sec. Systems Microbiology
Volume 12 - 2021 | https://doi.org/10.3389/fmicb.2021.685935
A growing number of experimental and computational approaches are illuminating the “microbial dark matter” and uncovering the integral role of commensal microbes in human health. Through this work, it is now clear that the human microbiome presents great potential as a therapeutic target for a plethora of diseases, including inflammatory bowel disease, diabetes and obesity. The development of more efficacious and targeted treatments relies on identification of causal links between the microbiome and disease; with future progress dependent on effective links between state-of-the-art sequencing approaches, computational analyses and experimental assays. We argue determining causation is essential, which can be attained by generating hypotheses using multi-omic functional analyses and validating these hypotheses in complex, biologically relevant experimental models. In this review we discuss existing analysis and validation methods, and propose best-practice approaches required to enable the next phase of microbiome research.
The human microbiome has now been implicated in several pathologies, including inflammatory bowel disease (IBD; Ott et al., 2004), diabetes (Vatanen et al., 2018), and obesity (Cox et al., 2014; de la Cuesta-Zuluaga et al., 2018) and therefore represents a broad-range potential therapeutic target. Evidence suggests microbiome-based interventions such as probiotics, may reduce symptoms of irritable bowel syndrome (IBS; Ford et al., 2014), antibiotic- associated diarrhea (Evans et al., 2016; Blaabjerg et al., 2017), preterm infant mortality rates and necrotizing enterocolitis (Sekhon et al., 2019; Underwood, 2019; Liu D. et al., 2020). Proposed mechanisms of action include: increases in intestinal barrier function (Burger-van Paassen et al., 2009), production of antimicrobials (Jones and Versalovic, 2009), and interaction with the immune system (Veckman et al., 2004; Smits et al., 2005; Kwon et al., 2010). Hence, further studies provide the opportunity to determine mechanistic links between the current generation probiotics and disease, and can inform the production of more targeted therapeutics for a wider range of conditions.
Sequencing and experimental analyses of the microbiome continue to advance substantially. Coupling multi-omics technologies, statistical and computational analyses, and more advanced disease models, these approaches promisingly provide the opportunity to establish disease causality and subsequently inform therapeutic development. We propose a workflow constituent of stages: (i) compositional and functional characterization of the microbiome, (ii) data-driven hypotheses generation, and (iii) experimental validation of hypotheses (Figure 1).
Figure 1. Overview of microbiome analysis workflow for the purpose of determining causality and enabling therapeutic development. (i) Compositional and functional characterization of a microbial community through identification of taxa and functions present. (ii) Data-driven hypothesis generation through application of computational methods including mathematical and statistical modeling. (iii) Experimental validation of hypotheses using in vivo and in vitro models such as animal models, cell lines, organoids and organ-on-a-chip.
As we emerge from the “unculturable” era of microbiome research, it is clear that the application of culture-independent sequencing methods has delivered substantial understandings of the taxonomic composition within bacterial communities. Studies involve experimentally extracting genomic DNA from samples of interest and grouping strains and species based on their genetic similarity in small genomic regions. Reads may be binned based on similarity thresholds, as operational taxonomic units (Westcott and Schloss, 2015), or biological sequences may be statistically distinguished from sequencing errors, as amplicon sequence variants (Eren et al., 2013; Tikhonov et al., 2015; Callahan et al., 2017). The output may be used to describe the community based on taxonomy or phylogeny. These methods have focused on correlating disease with microbial community composition and population structure, allowing the identification of key microbial groups that may mediate disease, yet all have inherent advantages and disadvantages (Table 1).
Table 1. Advantages and disadvantages of available taxonomic and functional characterization technologies.
Following sample collection, one approach to identify bacterial and archaeal taxonomy is amplification and sequencing of the 16S rRNA gene. Culture-independent, high-throughput, short read sequencing of genetic hypervariable regions, such as with 454 pyrosequencing, Ion Torrent® and Illumina® platforms, provide insight into microbial community composition, and has been beneficial in many fields, such as soil (Schöler et al., 2017) and marine (Wilson et al., 2010) environmental microbiology. The cost-effective nature of 16S rRNA sequencing facilitates studies with extremely large sample sizes (Minich et al., 2018), enabling monitoring of large communities over time (Poretsky et al., 2014), as well as the identification of specific taxa through targeted amplification (Berry and Gutierrez, 2017), or pathogen screening (Watanabe et al., 2018; Numberger et al., 2019). More recently, the application of long-read 16S rRNA gene sequencing has facilitated greater taxonomic resolution than available with amplicon-based 16S rRNA profiling (Johnson et al., 2019; Numberger et al., 2019).
In the gastrointestinal microbiome specifically, 16S rRNA profiling has also been widely applied (Eckburg et al., 2005; Turnbaugh et al., 2007; Gomez-Arango et al., 2016; Li et al., 2020; Ryan et al., 2020). The Human Microbiome Project (HMP) has applied this technology to characterize the complexity of the microbiome at different body sites in over 300 individuals (Turnbaugh et al., 2007), while diet and nutritional associated taxonomic changes to the microbiome have also been described (Zackular et al., 2016; Raman et al., 2019). In disease pathogenesis, substantial progress has also been made in identifying key microbial groups. Escherichia and Faecalibacterium genera have been associated with IBD disease phenotypes (Frank et al., 2011; Lopez-Siles et al., 2018), while a depletion in butyrate-producing Coprococcus spp. has been associated with depression (Valles-Colomer et al., 2019).
Despite the success of 16S rRNA profiling, it may not capture sufficient genomic variation to distinguish between closely related and yet functionally different species (Neville et al., 2018; Johnson et al., 2019). In addition, the reliance on “universal” primers for DNA amplification may introduce biases whereby some species are amplified more than others, with taxonomic coverage reported from 11% to 93% depending on primer choice (Thomas et al., 2012). Other sequencing artifacts, such as polymerase errors (Cline et al., 1996), chimeras (Haas et al., 2011; Eloe-Fadrosh et al., 2016), 16S rRNA copy number variation (Louca et al., 2018), and laboratory contamination (de Goffau et al., 2019; Han et al., 2020) are all exacerbated during PCR amplification.
As it does not rely on amplification of specific genetic markers, metagenomic shotgun sequencing provides a capacity to measure all genes in the community, thus overcoming many of the limitations of amplicon sequencing. With appropriate sequencing depth and analysis, this approach provides the potential to achieve species and strain level resolution and the foundations for functional characterization (i.e., the metabolic capacity of the microbiome).
Where extensive databases of complete genome sequences exist, reference-based methods of analysis may provide an ability to achieve high-resolution taxonomic classification through direct comparison (Lloyd-Price et al., 2017; Forster et al., 2019). Several programs exist for aligning millions of sequenced reads (or k-mers) to reference databases for taxonomic assignment, for example Kraken2 (Wood et al., 2019), MetaPhlAn2 (Truong et al., 2015), Metacache (Müller et al., 2017), CCMetagen (Marcelino et al., 2020a), and Centrifuge (Kim D. et al., 2016). While available metagenomic classifiers each have advantages and limitations that impact accuracy and resolution of classification (McIntyre et al., 2017), reference-guided analysis is also fundamentally limited by availability and selection of the reference genome database. Recent evidence suggests that databases with limited taxonomic diversity also cause misclassification of reads, as they align to evolutionarily conserved regions (Marcelino et al., 2020b). Large scale efforts, such as the Genome Taxonomy Database, which currently contains over 190,000 bacterial reference genomes (Parks et al., 2020), ensure that a comprehensive mix of references are available. Not only does this mitigate misassignment of reads, but it improves taxonomic resolution. In the context of the gut microbiome, Forster et al., improved taxonomic classification by 61% and achieved a subspecies level resolution for 50% of reads by adding 737 bacterial genomes into a reference database of genomes from the HMP collection (Forster et al., 2019).
Where the databases to support reference-based metagenomics are not available, de novo assembly of metagenomic sequence reads can be used to obtain metagenome assembled genomes (MAGs; Hugerth et al., 2015; Kang et al., 2015). This can be incredibly effective to identify new species and their phylogenies, as well as to provide catalogs of reference genomes (Parks et al., 2017; Almeida et al., 2019). However, this method can encounter difficulty reassembling repetitive sequence regions (Kingsford et al., 2010) and has problems with incorrect genome reconstructions in the presence of high complexity datasets with genetically similar community members (Sczyrba et al., 2017). Increased sequencing depth and inclusion of hybrid assemblies containing short and long reads or using single cell genomics (Zhang et al., 2006; Chijiiwa et al., 2020) aid in overcoming these issues (Bertrand et al., 2019; Xie et al., 2020); however, this remains cost prohibitive in many circumstances.
Accurate taxonomic classification of metagenomic sequences, relies on quality, diverse and well- populated reference databases. This calls for coordinated cross-disciplinary efforts to build these references using large, diverse datasets such as was undertaken recently for the human gastrointestinal microbiome (Forster et al., 2019; Almeida et al., 2021).
The phenotypic profile of the microbiome community is determined by the functional gene products from the microbiome. Microbial gene products perform several essential processes for human health, including synthesis of vitamins (Hill, 1997), breakdown of non-digestible carbohydrates (Flint et al., 2008), and aid in host immune development (Imaoka et al., 1996; Ivanov et al., 2009). Conversely, microbial gene products also confer virulence in microorganisms, activate inflammatory signaling pathways (Schirmer et al., 2016; Halfvarson et al., 2017) and trigger autoimmune diseases (Wu et al., 2010; Azad et al., 2013). Hence, deleting, altering or introducing various functional genes by manipulating the microbiome may be the key to producing microbiome-based therapies and managing related diseases. There are several techniques to perform functional assessment of the gastrointestinal microbiome, including functional inference from 16S rRNA sequencing, functional prediction from metagenomic sequencing and direct measurement through multi-omics studies (Table 1).
Functional capacity may be inferred based on known functions of the closely-related microorganisms identified by 16S rRNA amplicon sequencing (Langille et al., 2013). For example, Stevens et al. (2020), used PICRUSt2, a tool to predict function from 16S sequences (Douglas et al., 2020), to identify butyrate degradation and Gamma-aminobutyric acid degradation pathways in the gut microbiome of individuals with a depression phenotype, contrasted with a prevalence of butyrate producing species in healthy subjects. Predictions from 16S rRNA have also been successful in identifying metabolic shifts between microbiome data sets, as Noecker et al. (2016), identified that metabolites involved in bacterial vaginosis tended to be well predicted by their 16S rRNA prediction model. Functional prediction tools based on marker genes are better at predicting function in relation to different categories. When comparing metagenome prediction tools and metagenomic sequencing results, Sun et al. (2020), highlight that metagenome prediction tools, PICRUSt (Langille et al., 2013), PICRUSt2 (Douglas et al., 2020), and Tax4Fun (Aßhauer et al., 2015) are better at inferring function for “housekeeping” genes involved in translation and transcription, and metabolism-related functions, than for functions related to signaling molecules and signal transduction.
Microbes which exhibit identical 16S rRNA genes show functional similarities, however, there are also important differences which is a limitation of this approach. For example, in Escherichia coli approximately 20% of genes are core genes found in all strains. The remaining 80% are accessory genes that are not found in every strain and therefore contribute to the functional diversity within the species (Lukjancenko et al., 2010). As taxonomic resolution of 16S rRNA amplicon sequencing is largely limited to the genus level, inferring function based on taxonomic relatedness at this level is insufficient for comprehensive assessment of functional capacity. Additionally, where reference genomes are not available, no functional inference can be made.
Shotgun metagenomic sequencing provides a direct measurement of all genes present, which can be used to determine all potential functional gene products in the community. To assess functional capacity, sequenced shotgun metagenomic reads are mapped directly to reference gene or genome databases (Kanehisa and Goto, 2000; Consortium, 2020), or assembled into MAGs. Functions can be inferred through conventional gene prediction and annotation workflows such as Prokka (Seemann, 2014), DRAM (Shaffer et al., 2020), and KoFamScan (Aramaki et al., 2020).
Several studies highlight the power of functional prediction in ascertaining the phenotype of a microbial community using metagenomic sequencing. Perturbations of essential microbial functions, including short-chain fatty acid production and L-arginine synthesis, both involved in intestinal barrier function, have been identified in IBD (Vila et al., 2018; Parada Venegas et al., 2019). Additionally, Qin et al. (2012), have characterized microbial functional differences in type 2 diabetes, indicating enrichment in branched-chain amino acid transport, methane metabolism, sulfate reduction, and butyrate synthesis. While the exact roles of these functional changes may be unknown, these studies allow the postulation of potential disease mechanisms that now require statistical analyses and experimental validation.
Unfortunately, a substantial part of the functional capacity within the human microbiome remains unknown. Despite application of advanced computational approaches (Rifaioglu et al., 2019), with an increasing number of hypothetical protein sequences (Nauman et al., 2019), and recent identification of over 4000 small proteins with unknown function in the human microbiome, (Sberro et al., 2019) these limitations remain significant.
The requirement for analysis of functional state is not limited to assessing complete genomic capacity, as all genes present within a microbial community may not all be expressed (Turnbaugh et al., 2010; Franzosa et al., 2014, 2018). Therefore, attaining a holistic compositional and functional assessment of the microbiome requires an integrated -omic approach, with metagenomics, metatranscriptomics, metaproteomics, and metabolomics. Metatranscriptomics involves sequencing RNA transcripts from viable microorganisms for insight into gene expression profiles within the community (Moran, 2009), while protein profiles can be identified using metaproteomics (Wilmes and Bond, 2006) and metabolomics can enable the identification of metabolites (Fiehn, 2002). The multi-omics approach provides greater accuracy in determining the role of bacterial strains in disease pathogenesis by capturing the spectrum of genetic potential to phenotype (Heintz-Buschart and Wilmes, 2018).
As with metagenomic approaches, multi-omic data analysis is also largely limited by reference catalogs, generating bias toward previously characterized pathways. Multi-omics datasets generate a multitude of data types (gene content, expression, and microbial species, etc.), and this inherent complexity is challenging to integrate. The different sequencing outputs, such as sequence reads and metabolite counts, are not readily comparable (Palsson and Zengler, 2010; Jiang et al., 2019; Pinu et al., 2019). Differences in rates of transcription, translation and metabolization between different species within a sample call for extensive temporal sampling, which is often not feasible. Additionally, it is important to note that an increase in the number of samples and data types results in high data dimensionality as the number of variables to consider increases (Gloor et al., 2017). Without appropriate statistical correction, biases are introduced into results and conclusions can be drawn from false positives (Thorsen et al., 2016; Gloor et al., 2017). Furthermore, sampling the gut microbiome incurs additional difficulties, as it contains distinct communities and spatial heterogeneity between gastrointestinal regions (Zhang et al., 2014; Lavelle et al., 2015), which are not represented in fecal sampling. Even with extensive sampling, the high dimensionality of multi-omics datasets makes it challenging to determine mechanisms of action. Extensive work is being done in an attempt to overcome these issues in silico [see (Meng et al., 2016)].
Despite these challenges, integration of coupled multi-omic datasets from gastrointestinal microbiome samples has been successful in identifying potential links between microbiome functions and disease. Heintz-Buschart et al. (2016), used metaproteomics and metatranscriptomics to correlate the presence of human pancreatic enzymes in type 1 diabetes, with the expression of several microbial pathways, including glycolysis and thiamine synthesis, postulating their role in disease pathogenesis. Similarly, metabolites associated with Subdoligranulum species have been correlated with IBD (Lloyd-Price et al., 2019). Hence, the use of multiple dataset types provides the opportunity to link compositional microbial taxa to disease through potential mechanisms of interaction.
Taxonomic and functional information are the baseline to derive hypotheses and guide the experiments that can ultimately elucidate causal links between the microbiome and disease and inform therapeutic development. Data- driven hypotheses generation is therefore a key step to achieve translation of microbiome knowledge. Here we outline the most common challenges and emerging approaches in this field.
Measurements of microbiome datasets are frequently compositional whereby they are proportional and dependent on the analysis approach and sequencing depth (Gloor et al., 2017). As compositionality can strongly influence analysis and conclusions, normalization processes that treat microbiome data as ratios, such as centered log-ratio transformations, must be used before applying standard statistical analysis (Gloor et al., 2016, 2017; Lê Cao et al., 2016).
Multivariate statistics have been widely used to identify specific bacterial taxa, potentially associated with disease. Discriminatory methods, such as discriminant analyses, aim to define the taxa (or functions) that maximize differences between groups, such as healthy and diseased cohorts. By comparing the gut microbiota of individuals with atherosclerotic cardiovascular disease and healthy controls, Jie et al. (2017), reported a depletion in Bacteroides and Prevotella and an increase in abundance of Enterobacteriaceae and Streptococcus spp., identifying potential targets for microbiome modulation. These methods have also been used to identify potential biomarkers, or non-invasive early markers of disease, such as for colorectal cancer including Fusobacterium nucleatum and Parvimonas micra (Yu et al., 2017), and secondary colorectal metachronous adenoma including Escherichia and Acinetobacter (Liu Y. et al., 2020). Statistical algorithms that specifically account for compositional microbiome data, such as selbal (Rivera-Pinto et al., 2018), can be used to identify groups of taxa that may explain a variable of interest or disease phenotype.
Machine learning has been broadly applied in the context of human microbiome research, in antibiotic resistance prediction and modeling (Arango-Argoty et al., 2018; Rahman et al., 2018), taxonomic classification of metagenomic and 16S rRNA sequences (Vervier et al., 2016; Fiannaca et al., 2018; Desai et al., 2020), and gene function prediction (Cai et al., 2020). However, recent use in identifying microbial signatures and potential therapeutic targets in disease, may inform experimental validation and focus hypothesis generation for disease treatments. These methods have been able to predict incidence of IBD (Hacılar et al., 2018), IBS (Fukui et al., 2020), and colorectal cancer (Thomas et al., 2019) based on microbiome signatures. Notably, these methods can also identify potential mechanisms of disease, for example Thomas et al., have leveraged taxonomic and functional metagenomic information from healthy controls and patients with colorectal cancer, using a random forest classifier to identify reproducible biomarkers. They also reported a potential mechanism of action for colorectal cancer pathogenesis, as gene variants involved in synthesis of trimethylamine from choline in Hungatella hathewayi and Clostridium asparagiforme were significantly associated with colorectal cancer samples (Thomas et al., 2019). Similarly, Cai et al. (2017), identified microbial pathways, or reaction groups, potentially involved in IBD when analyzing data from Qin et al. (2010), using Non-Negative Matrix Factorization. Both ascorbate and aldarate metabolism, and amino sugar, nucleotide sugar metabolism, fructose and other metabolic pathways were identified to have a greater contribution to IBD samples. While machine learning techniques are promising for microbiome hypothesis generation, there are several challenges including: interpretability (Ghannam and Techtmann, 2021), incomplete data labels (Ten Hoopen et al., 2017), and choosing a model appropriate for the characteristics of the data (Namkung, 2020). Collaborative efforts in machine learning for microbiome analysis, such as that of the European Cooperation in Science and Technology network “ML4Mirobiome” aim to standardize and synergize different fields, and combat these challenges.
Genome-scale metabolic reconstructions are based on genomic, biochemical, multi-omics and published experimental data, and can be used to model the response of individual microbial species to changing environmental or dietary conditions and to infer microbial interactions (Thiele and Palsson, 2010; Magnúsdóttir and Thiele, 2018). For example, El-Semman et al. (2014), identified that when Bifidobacterium adolescentis was present, F. praunitzii changed its metabolism by increasing butyrate production, a short chain fatty acid associated with health. Similarly, Steinway et al. (2015), employed metabolic models to study the ability of different commensal microbes to decrease the abundance of Clostridioides difficile, finding that Barnesiella intestihominis was able to impede C. difficile growth through metabolic interactions. Although experimental validation is required, this knowledge may be used to develop B. intestinihominis as a therapeutic target. Metabolic models can also be used to infer the response of microorganisms to potential therapeutic strategies. Promising drug targets for the pathogen Klebsiella pneumoniae have been recently identified using metabolic modeling (Cesur et al., 2020), highlighting the potential of computational models to generate testable hypotheses.
Members of the microbiome function as an ecological community, and leveraging microbial interactions to develop biotherapeutics is a logically promising avenue. Biological interactions can be represented through networks, where systems’ elements, such as microbes and their metabolites, are represented by nodes, and their interactions depicted as edges. These networks may be based on simple correlation analyses such as standard Spearman correlations, or on more sophisticated alternatives such as Bayesian analyses (Jiang et al., 2019). In the human gut microbiome, Dai et al. (2018), used a correlation network analysis to identify the interactions between microbes enriched in colorectal cancer, whereby Clostridium species had the highest centralities, or more co-occurrence interactions than is expected by chance, suggesting that they may play a pivotal role in the disease. Additionally, Zhang et al. (2014), provide evidence that F. praunitzii and Bacteroides coprophilus co-occur less than is expected by chance, suggesting competition between these two species. Interestingly, competitive exclusion of F. praunitzii is associated with inflammation in patients with Crohn’s disease, and therefore B. coprophilus has been identified as a potential therapeutic candidate to be targeted for removal from the community (Zhang et al., 2014). Network theory can also be applied to integrated multi-omic data to identify the functional mechanisms behind microbial crosstalk (Lloyd-Price et al., 2019), providing targeted avenues for downstream characterization and validation in experimental models.
The marked influence of the individual genetic background on their response to drugs has been well established in the pharmacogenetics field. More recently, the emerging pharmacomicrobiomics field addresses the many ways in which the microbiome can modulate the host metabolic response to improve the efficacy of therapeutic strategies. For example, it has been observed that cardiac drugs may be inactivated by Eggerthella lenta in the gastrointestinal tract (Haiser et al., 2013). Additionally, in several forms of cancer, studies have shown that the microbiome can influence patient responsiveness to chemotherapy and immunotherapy [see (Helmink et al., 2019) for a review]. The exact mechanisms by which the microbiota influence therapeutic responses are still poorly characterized, but we expect that the ecological interactions among members of the microbiome and their interactions with the host immune system play a fundamental role in various microenvironments. Microbial community assembly and metabolism is known to be context-dependent, with particular pathways switching on and off depending on their biotic and abiotic surroundings. Therefore, a systems-biology approach that considers how the diverse microbiome influences host key pro-inflammatory and anti-inflammatory mediators is likely to reveal the most promising personalized therapies.
While statistical and computational analyses are critical in the identification of patterns in microbiome function and interactions, it is important to test these hypotheses experimentally in order to demonstrate causation and translate such findings for clinical application. Research findings that are both computationally and experimentally supported are more likely to render positive results at the clinal trial stage. Various tools and technologies have emerged for experimental hypotheses validation which encompasses both in vitro and in vivo approaches.
Mouse models can provide insights into host-microbial interactions, including how the microbiota shapes host immunity (Maier and Hentges, 1972; Bouskra et al., 2008), physiology, and metabolism (Cash et al., 2006). There are four main mouse models used in microbiome studies; specific pathogen-free (SPF) mice, bred for the absence of murine disease-causing pathogens (e.g., Helicobacter pylori); antibiotic treated (abx) mice, which are depleted of all or specific microbial groups; germ free (GF) mice are absent of any microbes; and gnotobiotic mice, which are selectively recolonized with either single microbes or defined communities. Germ free and gnotobiotic mice have the advantage of a controlled microbiome composition; however, require specific maintenance and breeding strategies to ensure appropriate development of lymphoid tissue (Bouskra et al., 2008) and mediation of immune response (Levy et al., 2015). In contrast, SPF and abx mice undergo normal immune development but lack a defined microbial community.
Specific pathogen-free mice have been utilized to elucidate the potential impact of the microbiome composition on host immune regulation and disease pathogenesis (Vijay-Kumar et al., 2007; Letran et al., 2011). In contrast to SPF mice, abx mice are administered with narrow spectrum antibiotics, hence rendering them more susceptible to infection due to the absence of the gut microbiome. Various studies have thus utilized the abx model to affirm the necessity of the gut microbiome in pathogen colonization resistance and immune response to infection (Abt et al., 2012; Deshmukh et al., 2014).
Germ free mice can be crucial to demonstrate the transmissibility of the microbiome and associated phenotype through fecal microbiome transplantation from donor to recipient animals (De Palma et al., 2017; Zhou et al., 2017; Le Bastard et al., 2018), and this model has led to the development of humanized gnotobiotic mice (Hazenberg et al., 1981; Turnbaugh et al., 2009; Chung et al., 2012). Generation of gnotobiotic mice from “diseased” or “healthy” human samples, with optimized diet and environmental exposures, may better reflect a human system (Park and Im, 2020).
Despite these important insights, the highly co-evolved nature of microbe and host (Ochman et al., 2010; Goodrich et al., 2014) introduce difficulties for human translation. Microbe species differ greatly between humans and mice, with many human-derived microorganisms failing to colonize mice (Kibe et al., 2005; Chung et al., 2012). For example, Lactobacillus reuteri has been identified in both human and mouse gastrointestinal tracts (Frese et al., 2011). However, strains present in rodents have been found to contain rodent specific genes that impact the colonization and function of the species in the murine model (Frese et al., 2011). Additionally, it has been shown that commensal (Lasaro et al., 2014) and enterohaemorrhagic strains (Roxas et al., 2010) of human E. coli are unable to permanently colonize conventionally reared mice. Therefore, despite the invaluable immunological insights obtained from animal in vivo models, their lack of congruency with the human microbial community structure and immune function (Chung et al., 2012; Eun et al., 2014), suggests the need for human-specific models in microbiome disease research.
Human-derived immortalized cell lines are a common tool to gain a better understanding of host-microbial functions due to several advantages (Bahrami et al., 2011; Sadabad et al., 2015). Immortalized cell lines are cost-effective, can continuously divide and proliferate, providing a means for efficient high-throughput and reproducible analyses. Human-derived cell lines widely utilized in the gastrointestinal field include intestinal epithelial-derived IEC-6 cells and the colonic adenocarcinoma-derived T84, HT-29, LS513, and Caco2 cell lines, which have been used to demonstrate the beneficial impact of probiotics, including strains of Lactobacilli and Bifidobacteria in enteroinvasive E. coli invasion (Khodaii et al., 2017). However, epithelial cell lines vary in gene expression compared to the normal intestinal epithelium within the human body (Bourgine et al., 2012) and, being comprised of a single cell type, are absent of endothelial and immune cell populations, which limits the ability to biologically represent human gut physiology and morphology (Pearce et al., 2018). Additionally, a monolayer of cells grown in a flask or petri dish cannot recapitulate the anaerobic conditions and epithelial structure present in the intestinal lumen which is the ecological niche of a large proportion of the microbiota. Therefore, it is difficult to effectively capture the native state of microbe and host using immortalized cell lines. While these models provide fundamental information on host cellular response to microbes, there remains a need for models capable of capturing the cellular diversity and anaerobic intestinal environment in order to appropriately assess host-microbe interactions.
In vitro intestinal organoids, referred to as “mini-guts” or enteroids/colonoids, are derived from intestinal stem cells and are self-organized into an enclosed, three-dimensional structure with a heterogeneous array of organ specific cell types (Sato et al., 2009). Derivation of organoid tissue models and co-culture methods have been well established and utilized in drug-screening, investigations of gastrointestinal infections, host-pathogen and host-microbiome interactions (Finkbeiner et al., 2012; Forbester et al., 2015; Hou et al., 2018; Driehuis et al., 2020). Additionally, ex vivo patient-derived organoid cultures (primary cells from human tissues) may be used as a tool for personalized therapeutic treatments (van de Wetering et al., 2015). Microinjection allows the delivery of microbes and substances into the organoid lumen, which has been particularly useful for studies of the microbiome (Williamson et al., 2018). For example, Leslie et al. (2015), used microinjection to demonstrate the loss of epithelial barrier integrity, through injection of viable C. difficile, as well as the C. difficile exotoxin, toxin A (TcdA). Furthermore, human fecal microbiota can be transplanted and maintained in culture within the intestinal lumen (Williamson et al., 2018). Although organoids can offer insight into disease pathogenesis and host-microbial relationships, organoid systems lack the critical physiological processes (peristalsis, oxygen gradient) and immune-microbiome functions (vasculature, intestinal immune cells) that are necessary to advance our understanding of the microbiome. As these are important factors when investigating human gut physiology and function, the limitations of organoid models may reduce the ability to decipher the complexity of host-microbiome crosstalk. Thus, it is essential to consider alternative experimental models that are more biologically accurate.
Organ-on-a-chip technology has revolutionized the ability to recapitulate organ-level physiology. The lung-on-a-chip was the pioneering platform that successfully micro-engineered the lung-alveoli vasculature and function, including controlled breathing motions by applying fluid shear stress on the device (Huh et al., 2010). Similarly, flow-induced motions have been reproduced in gut-chip systems to mimic intestinal peristalsis (Beaurivage et al., 2019). The gut-on-a-chip device models the structure, function, immune capability and other physiological processes in the human gut, all controlled by microfluidics, which allows fine-tuning of fluids at microscale levels (Vickerman et al., 2008; Bhatia and Ingber, 2014). This emerging technology provides a means of hypotheses validation and demonstration of causation in microbiome studies. The gut-on-a-chip device consists of upper and lower microfluidic channels separated by a polymeric porous membrane which allow continued perfusion, mimicking the dynamics of the gastrointestinal system, unlike other existing in vitro model systems (Bhatia and Ingber, 2014). The upper channel or apical membrane allows intestinal epithelial cells to be cultured, while media containing cellular growth factors can be perfused through the channel. Endothelial and immune cells (e.g., myeloid cells, leukocytes) can be compartmentalized in the lower channel or basolateral membrane to mimic the intestinal microvasculature, allowing for epithelial-endothelial interactions to occur. For comprehensive analyses of host-microbial pathophysiology, innate and adaptive immune responses can be selectively modeled by differentiating patient-derived primary cells into various immune cell subsets (e.g., macrophages, dendritic cells; Maurer et al., 2019). In addition, these systems can maintain an oxygen gradient from the aerobic microvasculature channels to the anaerobic epithelial layer, allowing for co-culture of a diverse array of aerobic and anaerobic gastrointestinal microbes (Jalili-Firoozinezhad et al., 2019). A microbiome can therefore be established at a tissue-interface, and host-microbe responses can be measured in real-time.
Proof of concept studies have demonstrated the efficacy of generating a diverse microbiome in situ (Jalili-Firoozinezhad et al., 2019). Specifically, Kim H.J. et al. (2016), demonstrated the role of intestinal probiotic bacterial strains in modulating inflammation by enhancing intestinal barrier function. Similarly, this technology has been used to model the attachment, invasion and propagation of Shigella in the intestinal epithelium (Grassart et al., 2019) and the involvement of commensal microbial metabolites in colonic epithelium injury during enterohemorrhagic E. coli infection (Tovaglieri et al., 2019). In addition to modeling host-bacterial interactions, organ-on-a-chip models can also be used for identification and testing of therapeutic bacterial candidates. To target specific health and disease states, patient-derived primary cells can be isolated from biopsies and then cultured in gut-chip devices to fine-tune analysis of host response toward drugs, probiotics or pathogens (Kasendra et al., 2018; Van Den Berg et al., 2019). The organ-on-a-chip is an invaluable tool for studying the role of the microbiome in health and disease in various tissue types of the human body. The scalable nature of this technology provides a means to assemble different organ-chips to produce a human-chip system for investigations on an inter-systemic level (Maschmeyer et al., 2015; Picollet-D’hahan et al., 2021). It will also provide significant insight of the interconnectivity between the gut microbiome with other tissue or organ systems. While further research and development is underway, an established organ-on-a-chip platform could enhance the ability to validate hypotheses in a high-throughput manner and pave way for the development of therapeutics toward efficacious personalized precision medicine.
Human gastrointestinal microbiome research has the potential to deliver critical clinical and therapeutic development if it shifts toward mechanistic studies. We proposed that a suitable avenue to translate microbiome research is a workflow that includes (i) compositional and functional characterization of the microbiome, (ii) data-driven hypotheses generation, and (iii) experimental validation of hypotheses. By integrating recent advances in computational, statistical and experimental methods through this workflow, the ability to identify disease causation and propose logical microbiome therapeutics in disease treatment is possible.
The next step toward implementation of microbiome-based treatments relies on a deeper biological understanding of how microbial communities respond to the introduction of new strains or microbial mimetics. As such, research into ecological interactions and pharmacomicrobiomics will be key to understand the complex ways in which the microbiome influence host health and response to drugs. We expect that a systems-biology approach targeting the microbiome and host-microbiome interactions will guide the successful implementation of microbiome therapeutics.
RBY and SCF conceptualized the idea. RBY wrote the manuscript and made the figure. VRM, MC, ELG, and SCF contributed to various sections and edited the review. All authors contributed to the article and approved the submitted version.
This work was supported by the Australian National Health and Medical Research Council (1141564, 1181105, and 1186371 to SCF) and the Victorian Government’s Operational Infrastructure Support Program. RBY is supported by an Australian Government Research Training Program (RTP) scholarship and SCF is supported by the Australian National Health and Medical Research Council (1159239).
SCF is a consultant to BiomeBank, Australia.
The remaining authors declare that the research was conducted in the absence of any commercial or financial relationships that could be construed as a potential conflict of interest.
The authors acknowledge the support of the Australian National Research Council, the Victorian Government’s Operational Infrastructure Support Program, and the Australian Government Research Training Program (RTP).
Abt, M. C., Osborne, L. C., Monticelli, L. A., Doering, T. A., Alenghat, T., Sonnenberg, G. F., et al. (2012). Commensal bacteria calibrate the activation threshold of innate antiviral immunity. Immunity 37, 158–170. doi: 10.1016/j.immuni.2012.04.011
Almeida, A., Mitchell, A. L., Boland, M., Forster, S. C., Gloor, G. B., Tarkowska, A., et al. (2019). A new genomic blueprint of the human gut microbiota. Nature 568, 499–504. doi: 10.1038/s41586-019-0965-1
Almeida, A., Nayfach, S., Boland, M., Strozzi, F., Beracochea, M., Shi, Z. J., et al. (2021). A unified catalog of 204,938 reference genomes from the human gut microbiome. Nat. Biotechnol. 39, 105–114. doi: 10.1038/s41587-020-0603-3
Aramaki, T., Blanc-Mathieu, R., Endo, H., Ohkubo, K., Kanehisa, M., Goto, S., et al. (2020). KofamKOALA: KEGG ortholog assignment based on profile HMM and adaptive score threshold. Bioinformatics 36, 2251–2252. doi: 10.1093/bioinformatics/btz859
Arango-Argoty, G., Garner, E., Pruden, A., Heath, L. S., Vikesland, P., and Zhang, L. (2018). DeepARG: a deep learning approach for predicting antibiotic resistance genes from metagenomic data. Microbiome 6:23.
Azad, M. B., Konya, T., Maughan, H., Guttman, D. S., Field, C. J., Sears, M. R., et al. (2013). Infant gut microbiota and the hygiene hypothesis of allergic disease: impact of household pets and siblings on microbiota composition and diversity. Allergy Asthma Clin. Immunol. 9:15. doi: 10.1186/1710-1492-9-15
Aßhauer, K. P., Wemheuer, B., Daniel, R., and Meinicke, P. (2015). Tax4Fun: predicting functional profiles from metagenomic 16S rRNA data. Bioinformatics 31, 2882–2884. doi: 10.1093/bioinformatics/btv287
Bahrami, B., Child, M. W., Macfarlane, S., and Macfarlane, G. T. (2011). Adherence and cytokine induction in Caco-2 cells by bacterial populations from a three-stage continuous-culture model of the large intestine. Appl. Environ. Microbiol. 77, 2934–2942. doi: 10.1128/aem.02244-10
Beaurivage, C., Naumovska, E., Chang, Y. X., Elstak, E. D., Nicolas, A., Wouters, H., et al. (2019). Development of a gut-on-a-chip model for high throughput disease modeling and drug discovery. Int. J. Mol. Sci. 20:5661. doi: 10.3390/ijms20225661
Berry, D., and Gutierrez, T. (2017). Evaluating the detection of hydrocarbon-degrading bacteria in 16S rRNA gene sequencing surveys. Front. Microbiol. 8:896. doi: 10.3389/fmicb.2017.00896
Bertrand, D., Shaw, J., Kalathiyappan, M., Ng, A. H. Q., Kumar, M. S., Li, C., et al. (2019). Hybrid metagenomic assembly enables high-resolution analysis of resistance determinants and mobile elements in human microbiomes. Nat. Biotechnol. 37, 937–944. doi: 10.1038/s41587-019-0191-2
Bhatia, S. N., and Ingber, D. E. (2014). Microfluidic organs-on-chips. Nat. Biotechnol. 32, 760–772. doi: 10.1038/nbt.2989
Blaabjerg, S., Artzi, D. M., and Aabenhus, R. (2017). Probiotics for the prevention of antibiotic-associated diarrhea in outpatients—a systematic review and meta-analysis. Antibiotics 6:21. doi: 10.3390/antibiotics6040021
Bourgine, J., Billaut-Laden, I., Happillon, M., Lo-Guidice, J.-M., Maunoury, V., Imbenotte, M., et al. (2012). Gene expression profiling of systems involved in the metabolism and the disposition of xenobiotics: comparison between human intestinal biopsy samples and colon cell lines. Drug Metab. Dispos. 40, 694–705. doi: 10.1124/dmd.111.042465
Bouskra, D., Brézillon, C., Bérard, M., Werts, C., Varona, R., Boneca, I. G., et al. (2008). Lymphoid tissue genesis induced by commensals through NOD1 regulates intestinal homeostasis. Nature 456, 507–510. doi: 10.1038/nature07450
Burger-van Paassen, N., Vincent, A., Puiman, P. J., Van Der Sluis, M., Bouma, J., Boehm, G., et al. (2009). The regulation of intestinal mucin MUC2 expression by short-chain fatty acids: implications for epithelial protection. Biochem. J. 420, 211–219. doi: 10.1042/bj20082222
Cai, Y., Gu, H., and Kenney, T. (2017). Learning microbial community structures with supervised and unsupervised non-negative matrix factorization. Microbiome 5:110.
Cai, Y., Wang, J., and Deng, L. (2020). SDN2GO: an integrated deep learning model for protein function prediction. Front. Bioeng. Biotechnol. 8:391. doi: 10.3389/fbioe.2020.00391
Callahan, B. J., Mcmurdie, P. J., and Holmes, S. P. (2017). Exact sequence variants should replace operational taxonomic units in marker-gene data analysis. ISME J. 11, 2639–2643. doi: 10.1038/ismej.2017.119
Cash, H. L., Whitham, C. V., Behrendt, C. L., and Hooper, L. V. (2006). Symbiotic bacteria direct expression of an intestinal bactericidal lectin. Science 313, 1126–1130. doi: 10.1126/science.1127119
Cesur, M. F., Siraj, B., Uddin, R., Durmuş, S., and Çakır, T. (2020). Network-based metabolism-centered screening of potential drug targets in Klebsiella pneumoniae at genome scale. Front. Cell. Infect. Microbiol. 9:447. doi: 10.3389/fcimb.2019.00447
Chijiiwa, R., Hosokawa, M., Kogawa, M., Nishikawa, Y., Ide, K., Sakanashi, C., et al. (2020). Single-cell genomics of uncultured bacteria reveals dietary fiber responders in the mouse gut microbiota. Microbiome 8:5.
Chung, H., Pamp, S. J., Hill, J. A., Surana, N. K., Edelman, S. M., Troy, E. B., et al. (2012). Gut immune maturation depends on colonization with a host-specific microbiota. Cell 149, 1578–1593. doi: 10.1016/j.cell.2012.04.037
Cline, J., Braman, J. C., and Hogrefe, H. H. (1996). PCR fidelity of pfu DNA polymerase and other thermostable DNA polymerases. Nucleic Acids Res. 24, 3546–3551. doi: 10.1093/nar/24.18.3546
Consortium, T. U. (2020). UniProt: the universal protein knowledgebase in 2021. Nucleic Acids Res. 49, D480–D489.
Cox, L. M., Yamanishi, S., Sohn, J., Alekseyenko, A. V., Leung, J. M., Cho, I., et al. (2014). Altering the intestinal microbiota during a critical developmental window has lasting metabolic consequences. Cell 158, 705–721. doi: 10.1016/j.cell.2014.05.052
Dai, Z., Coker, O. O., Nakatsu, G., Wu, W. K., Zhao, L., Chen, Z., et al. (2018). Multi-cohort analysis of colorectal cancer metagenome identified altered bacteria across populations and universal bacterial markers. Microbiome 6:70.
de Goffau, M. C., Lager, S., Sovio, U., Gaccioli, F., Cook, E., Peacock, S. J., et al. (2019). Human placenta has no microbiome but can contain potential pathogens. Nature 572, 329–334. doi: 10.1038/s41586-019-1451-5
de la Cuesta-Zuluaga, J., Corrales-Agudelo, V., Velásquez-Mejía, E. P., Carmona, J. A., Abad, J. M., and Escobar, J. S. (2018). Gut microbiota is associated with obesity and cardiometabolic disease in a population in the midst of Westernization. Sci. Rep. 8:11356.
De Palma, G., Lynch, M. D., Lu, J., Dang, V. T., Deng, Y., Jury, J., et al. (2017). Transplantation of fecal microbiota from patients with irritable bowel syndrome alters gut function and behavior in recipient mice. Sci. Transl. Med. 9:eaaf6397.
Desai, H. P., Parameshwaran, A. P., Sunderraman, R., and Weeks, M. (2020). Comparative study using neural networks for 16S ribosomal gene classification. J. Comput. Biol. 27, 248–258. doi: 10.1089/cmb.2019.0436
Deshmukh, H. S., Liu, Y., Menkiti, O. R., Mei, J., Dai, N., O’leary, C. E., et al. (2014). The microbiota regulates neutrophil homeostasis and host resistance to Escherichia coli K1 sepsis in neonatal mice. Nat. Med. 20, 524–530. doi: 10.1038/nm.3542
Douglas, G. M., Maffei, V. J., Zaneveld, J. R., Yurgel, S. N., Brown, J. R., Taylor, C. M., et al. (2020). PICRUSt2 for prediction of metagenome functions. Nat. Biotechnol. 38, 685–688. doi: 10.1038/s41587-020-0548-6
Driehuis, E., Kretzschmar, K., and Clevers, H. (2020). Establishment of patient-derived cancer organoids for drug-screening applications. Nat. Protoc. 15, 3380–3409. doi: 10.1038/s41596-020-0379-4
Eckburg, P. B., Bik, E. M., Bernstein, C. N., Purdom, E., Dethlefsen, L., Sargent, M., et al. (2005). Diversity of the human intestinal microbial flora. Science 308, 1635–1638. doi: 10.1126/science.1110591
Eloe-Fadrosh, E. A., Ivanova, N. N., Woyke, T., and Kyrpides, N. C. (2016). Metagenomics uncovers gaps in amplicon-based detection of microbial diversity. Nat. Microbiol. 1:15032.
El-Semman, I. E., Karlsson, F. H., Shoaie, S., Nookaew, I., Soliman, T. H., and Nielsen, J. (2014). Genome-scale metabolic reconstructions of Bifidobacterium adolescentis L2-32 and Faecalibacterium prausnitzii A2-165 and their interaction. BMC Syst. Biol. 8:41. doi: 10.1186/1752-0509-8-41
Eren, A. M., Maignien, L., Sul, W. J., Murphy, L. G., Grim, S. L., Morrison, H. G., et al. (2013). Oligotyping: differentiating between closely related microbial taxa using 16S rRNA gene data. Methods Ecol. Evol. 4, 1111–1119. doi: 10.1111/2041-210x.12114
Eun, C. S., Mishima, Y., Wohlgemuth, S., Liu, B., Bower, M., Carroll, I. M., et al. (2014). Induction of bacterial antigen-specific colitis by a simplified human microbiota consortium in gnotobiotic interleukin-10–/− mice. Infect. Immun. 82, 2239–2246. doi: 10.1128/iai.01513-13
Evans, M., Salewski, R. P., Christman, M. C., Girard, S.-A., and Tompkins, T. A. (2016). Effectiveness of Lactobacillus helveticus and Lactobacillus rhamnosus for the management of antibiotic-associated diarrhoea in healthy adults: a randomised, double-blind, placebo-controlled trial. Br. J. Nutr. 116, 94–103. doi: 10.1017/s0007114516001665
Fiannaca, A., La Paglia, L., La Rosa, M., Renda, G., Rizzo, R., Gaglio, S., et al. (2018). Deep learning models for bacteria taxonomic classification of metagenomic data. BMC Bioinformatics 19(Suppl. 7):198.
Fiehn, O. (2002). Metabolomics–the link between genotypes and phenotypes. Plant Mol. Biol. 48, 155–171. doi: 10.1007/978-94-010-0448-0_11
Finkbeiner, S. R., Zeng, X.-L., Utama, B., Atmar, R. L., Shroyer, N. F., and Estes, M. K. (2012). Stem cell-derived human intestinal organoids as an infection model for rotaviruses. mBio 3:e00159-12.
Flint, H. J., Bayer, E. A., Rincon, M. T., Lamed, R., and White, B. A. (2008). Polysaccharide utilization by gut bacteria: potential for new insights from genomic analysis. Nat. Rev. Microbiol. 6, 121–131. doi: 10.1038/nrmicro1817
Forbester, J. L., Goulding, D., Vallier, L., Hannan, N., Hale, C., Pickard, D., et al. (2015). Interaction of Salmonella enterica serovar Typhimurium with intestinal organoids derived from human induced pluripotent stem cells. Infect. Immun. 83, 2926–2934. doi: 10.1128/iai.00161-15
Ford, A. C., Quigley, E. M., Lacy, B. E., Lembo, A. J., Saito, Y. A., Schiller, L. R., et al. (2014). Efficacy of prebiotics, probiotics, and synbiotics in irritable bowel syndrome and chronic idiopathic constipation: systematic review and meta-analysis. Am. J. Gastroenterol. 109, 1547–1561. doi: 10.1038/ajg.2014.202
Forster, S. C., Kumar, N., Anonye, B. O., Almeida, A., Viciani, E., Stares, M. D., et al. (2019). A human gut bacterial genome and culture collection for improved metagenomic analyses. Nat. Biotechnol. 37, 186–192. doi: 10.1038/s41587-018-0009-7
Frank, D. N., Robertson, C. E., Hamm, C. M., Kpadeh, Z., Zhang, T., Chen, H., et al. (2011). Disease phenotype and genotype are associated with shifts in intestinal-associated microbiota in inflammatory bowel diseases. Inflamm. Bowel Dis. 17, 179–184. doi: 10.1002/ibd.21339
Franzosa, E. A., Mciver, L. J., Rahnavard, G., Thompson, L. R., Schirmer, M., Weingart, G., et al. (2018). Species-level functional profiling of metagenomes and metatranscriptomes. Nat. Methods 15, 962–968. doi: 10.1038/s41592-018-0176-y
Franzosa, E. A., Morgan, X. C., Segata, N., Waldron, L., Reyes, J., Earl, A. M., et al. (2014). Relating the metatranscriptome and metagenome of the human gut. Proc. Natl. Acad. Sci. U.S.A. 111, E2329–E2338.
Frese, S. A., Benson, A. K., Tannock, G. W., Loach, D. M., Kim, J., Zhang, M., et al. (2011). The evolution of host specialization in the vertebrate gut symbiont Lactobacillus reuteri. PLoS Genet. 7:e1001314. doi: 10.1371/journal.pgen.1001314
Fukui, H., Nishida, A., Matsuda, S., Kira, F., Watanabe, S., Kuriyama, M., et al. (2020). Usefulness of machine learning-based gut microbiome analysis for identifying patients with irritable bowels syndrome. J. Clin. Med. 9:2403. doi: 10.3390/jcm9082403
Ghannam, R. B., and Techtmann, S. M. (2021). Machine learning applications in microbial ecology, human microbiome studies, and environmental monitoring. Comput. Struct. Biotechnol. J. 19, 1092–1107. doi: 10.1016/j.csbj.2021.01.028
Gloor, G. B., Macklaim, J. M., Pawlowsky-Glahn, V., and Egozcue, J. J. (2017). Microbiome datasets are compositional: and this is not optional. Front. Microbiol. 8:2224. doi: 10.3389/fmicb.2017.02224
Gloor, G. B., Wu, J. R., Pawlowsky-Glahn, V., and Egozcue, J. J. (2016). It’s all relative: analyzing microbiome data as compositions. Ann. Epidemiol. 26, 322–329. doi: 10.1016/j.annepidem.2016.03.003
Gomez-Arango, L. F., Barrett, H. L., Mcintyre, H. D., Callaway, L. K., Morrison, M., and Dekker Nitert, M. (2016). Increased systolic and diastolic blood pressure is associated with altered gut microbiota composition and butyrate production in early pregnancy. Hypertension 68, 974–981. doi: 10.1161/hypertensionaha.116.07910
Goodrich, J. K., Waters, J. L., Poole, A. C., Sutter, J. L., Koren, O., Blekhman, R., et al. (2014). Human genetics shape the gut microbiome. Cell 159, 789–799.
Grassart, A., Malardé, V., Gobaa, S., Sartori-Rupp, A., Kerns, J., Karalis, K., et al. (2019). Bioengineered human organ-on-chip reveals intestinal microenvironment and mechanical forces impacting Shigella infection. Cell Host Microbe 26, 435–444.e4.
Haas, B. J., Gevers, D., Earl, A. M., Feldgarden, M., Ward, D. V., Giannoukos, G., et al. (2011). Chimeric 16S rRNA sequence formation and detection in Sanger and 454-pyrosequenced PCR amplicons. Genome Res. 21, 494–504. doi: 10.1101/gr.112730.110
Hacılar, H., Nalbantoğlu, O. U., and Bakir-Güngör, B. (2018). “Machine learning analysis of inflammatory bowel disease-associated metagenomics dataset,” in Proceedings of the 2018 3rd International Conference on Computer Science and Engineering (UBMK): IEEE), Antalya, 434–438.
Haiser, H. J., Gootenberg, D. B., Chatman, K., Sirasani, G., Balskus, E. P., and Turnbaugh, P. J. (2013). Predicting and manipulating cardiac drug inactivation by the human gut bacterium Eggerthella lenta. Science 341, 295–298. doi: 10.1126/science.1235872
Halfvarson, J., Brislawn, C. J., Lamendella, R., Vázquez-Baeza, Y., Walters, W. A., Bramer, L. M., et al. (2017). Dynamics of the human gut microbiome in inflammatory bowel disease. Nat. Microbiol. 2:17004.
Han, D., Gao, P., Li, R., Tan, P., Xie, J., Zhang, R., et al. (2020). Multicenter assessment of microbial community profiling using 16S rRNA gene sequencing and shotgun metagenomic sequencing. J. Adv. Res. 26, 111–121. doi: 10.1016/j.jare.2020.07.010
Hazenberg, M., Bakker, M., and Verschoor-Burggraaf, A. (1981). Effects of the human intestinal flora on germ-free mice. J. Appl. Bacteriol. 50, 95–106. doi: 10.1111/j.1365-2672.1981.tb00874.x
Heintz-Buschart, A., May, P., Laczny, C. C., Lebrun, L. A., Bellora, C., Krishna, A., et al. (2016). Integrated multi-omics of the human gut microbiome in a case study of familial type 1 diabetes. Nat. Microbiol. 2:16180.
Heintz-Buschart, A., and Wilmes, P. (2018). Human gut microbiome: function matters. Trends Microbiol. 26, 563–574. doi: 10.1016/j.tim.2017.11.002
Helmink, B. A., Khan, M. W., Hermann, A., Gopalakrishnan, V., and Wargo, J. A. (2019). The microbiome, cancer, and cancer therapy. Nat. Med. 25, 377–388.
Hill, M. (1997). Intestinal flora and endogenous vitamin synthesis. Eur. J. Cancer Prev. 6(Suppl. 1), S43–S45.
Hou, Q., Ye, L., Liu, H., Huang, L., Yang, Q., Turner, J., et al. (2018). Lactobacillus accelerates ISCs regeneration to protect the integrity of intestinal mucosa through activation of STAT3 signaling pathway induced by LPLs secretion of IL-22. Cell Death Differ. 25, 1657–1670. doi: 10.1038/s41418-018-0070-2
Hugerth, L. W., Larsson, J., Alneberg, J., Lindh, M. V., Legrand, C., Pinhassi, J., et al. (2015). Metagenome-assembled genomes uncover a global brackish microbiome. Genome Biol. 16:279.
Huh, D., Matthews, B. D., Mammoto, A., Montoya-Zavala, M., Hsin, H. Y., and Ingber, D. E. (2010). Reconstituting organ-level lung functions on a chip. Science 328, 1662–1668. doi: 10.1126/science.1188302
Imaoka, A., Matsumoto, S., Setoyama, H., Okada, Y., and Umesaki, Y. (1996). Proliferative recruitment of intestinal intraepithelial lymphocytes after microbial colonization of germ-free mice. Eur. J. Immunol. 26, 945–948. doi: 10.1002/eji.1830260434
Ivanov, I. I., Atarashi, K., Manel, N., Brodie, E. L., Shima, T., Karaoz, U., et al. (2009). Induction of intestinal Th17 cells by segmented filamentous bacteria. Cell 139, 485–498.
Jalili-Firoozinezhad, S., Gazzaniga, F. S., Calamari, E. L., Camacho, D. M., Fadel, C. W., Bein, A., et al. (2019). A complex human gut microbiome cultured in an anaerobic intestine-on-a-chip. Nat. Biomed. Eng. 3, 520–531. doi: 10.1038/s41551-019-0397-0
Jiang, D., Armour, C. R., Hu, C., Mei, M., Tian, C., Sharpton, T. J., et al. (2019). Microbiome multi-Omics network analysis: statistical considerations, limitations, and opportunities. Front. Genet. 10:995. doi: 10.3389/fgene.2019.00995
Jie, Z., Xia, H., Zhong, S.-L., Feng, Q., Li, S., Liang, S., et al. (2017). The gut microbiome in atherosclerotic cardiovascular disease. Nat. Commun. 8:845.
Johnson, J. S., Spakowicz, D. J., Hong, B.-Y., Petersen, L. M., Demkowicz, P., Chen, L., et al. (2019). Evaluation of 16S rRNA gene sequencing for species and strain-level microbiome analysis. Nat. Commun. 10:5029.
Jones, S. E., and Versalovic, J. (2009). Probiotic Lactobacillus reuteri biofilms produce antimicrobial and anti-inflammatory factors. BMC Microbiol. 9:35. doi: 10.1186/1471-2180-9-35
Kanehisa, M., and Goto, S. (2000). KEGG: kyoto encyclopedia of genes and genomes. Nucleic Acids Res. 28, 27–30.
Kang, D. D., Froula, J., Egan, R., and Wang, Z. (2015). MetaBAT, an efficient tool for accurately reconstructing single genomes from complex microbial communities. PeerJ 3:e1165. doi: 10.7717/peerj.1165
Kasendra, M., Tovaglieri, A., Sontheimer-Phelps, A., Jalili-Firoozinezhad, S., Bein, A., Chalkiadaki, A., et al. (2018). Development of a primary human Small Intestine-on-a-Chip using biopsy-derived organoids. Sci. Rep. 8:2871.
Khodaii, Z., Ghaderian, S. M. H., and Natanzi, M. M. (2017). Probiotic bacteria and their supernatants protect enterocyte cell lines from Enteroinvasive Escherichia coli (EIEC) invasion. Int. J. Mol. Cell. Med. 6, 183–189.
Kibe, R., Sakamoto, M., Yokota, H., Ishikawa, H., Aiba, Y., Koga, Y., et al. (2005). Movement and fixation of intestinal microbiota after administration of human feces to germfree mice. Appl. Environ. Microbiol. 71, 3171–3178. doi: 10.1128/aem.71.6.3171-3178.2005
Kim, D., Song, L., Breitwieser, F. P., and Salzberg, S. L. (2016). Centrifuge: rapid and sensitive classification of metagenomic sequences. Genome Res. 26, 1721–1729. doi: 10.1101/gr.210641.116
Kim, H. J., Li, H., Collins, J. J., and Ingber, D. E. (2016). Contributions of microbiome and mechanical deformation to intestinal bacterial overgrowth and inflammation in a human gut-on-a-chip. Proc. Natl. Acad. Sci. U.S.A. 113, E7–E15.
Kingsford, C., Schatz, M. C., and Pop, M. (2010). Assembly complexity of prokaryotic genomes using short reads. BMC Bioinformatics 11:21. doi: 10.1186/1471-2105-11-21
Kwon, H.-K., Lee, C.-G., So, J.-S., Chae, C.-S., Hwang, J.-S., Sahoo, A., et al. (2010). Generation of regulatory dendritic cells and CD4+ Foxp3+ T cells by probiotics administration suppresses immune disorders. Proc. Natl. Acad. Sci. U.S.A. 107, 2159–2164. doi: 10.1073/pnas.0904055107
Langille, M. G., Zaneveld, J., Caporaso, J. G., Mcdonald, D., Knights, D., Reyes, J. A., et al. (2013). Predictive functional profiling of microbial communities using 16S rRNA marker gene sequences. Nat. Biotechnol. 31, 814–821. doi: 10.1038/nbt.2676
Lasaro, M., Liu, Z., Bishar, R., Kelly, K., Chattopadhyay, S., Paul, S., et al. (2014). Escherichia coli isolate for studying colonization of the mouse intestine and its application to two-component signaling knockouts. J. Bacteriol. 196, 1723–1732. doi: 10.1128/jb.01296-13
Lavelle, A., Lennon, G., O’sullivan, O., Docherty, N., Balfe, A., Maguire, A., et al. (2015). Spatial variation of the colonic microbiota in patients with ulcerative colitis and control volunteers. Gut 64, 1553–1561. doi: 10.1136/gutjnl-2014-307873
Le Bastard, Q., Ward, T., Sidiropoulos, D., Hillmann, B. M., Chun, C. L., Sadowsky, M. J., et al. (2018). Fecal microbiota transplantation reverses antibiotic and chemotherapy-induced gut dysbiosis in mice. Sci. Rep. 8:6219.
Lê Cao, K.-A., Costello, M.-E., Lakis, V. A., Bartolo, F., Chua, X.-Y., Brazeilles, R., et al. (2016). MixMC: a multivariate statistical framework to gain insight into microbial communities. PLoS One 11:e0160169. doi: 10.1371/journal.pone.0160169
Leslie, J. L., Huang, S., Opp, J. S., Nagy, M. S., Kobayashi, M., Young, V. B., et al. (2015). Persistence and toxin production by Clostridium difficile within human intestinal organoids result in disruption of epithelial paracellular barrier function. Infect. Immun. 83, 138–145. doi: 10.1128/iai.02561-14
Letran, S. E., Lee, S.-J., Atif, S. M., Flores-Langarica, A., Uematsu, S., Akira, S., et al. (2011). TLR5-deficient mice lack basal inflammatory and metabolic defects but exhibit impaired CD4 T cell responses to a flagellated pathogen. J. Immunol. 186, 5406–5412. doi: 10.4049/jimmunol.1003576
Levy, M., Thaiss, C. A., Zeevi, D., Dohnalova, L., Zilberman-Schapira, G., Mahdi, J. A., et al. (2015). Microbiota-modulated metabolites shape the intestinal microenvironment by regulating NLRP6 inflammasome signaling. Cell 163, 1428–1443. doi: 10.1016/j.cell.2015.10.048
Li, Q., Chang, Y., Zhang, K., Chen, H., Tao, S., and Zhang, Z. (2020). Implication of the gut microbiome composition of type 2 diabetic patients from northern china. Sci. Rep. 10:5450.
Liu, D., Shao, L., Zhang, Y., and Kang, W. (2020). Safety and efficacy of Lactobacillus for preventing necrotizing enterocolitis in preterm infants: a systematic review and meta-analysis. Int. J. Surg. 76, 79–87. doi: 10.1016/j.ijsu.2020.02.031
Liu, Y., Geng, R., Liu, L., Jin, X., Yan, W., Zhao, F., et al. (2020). Gut microbiota-based algorithms in the prediction of metachronous adenoma in colorectal cancer patients following surgery. Front. Microbiol. 11:1106. doi: 10.3389/fmicb.2020.01106
Lloyd-Price, J., Arze, C., Ananthakrishnan, A. N., Schirmer, M., Avila-Pacheco, J., Poon, T. W., et al. (2019). Multi-omics of the gut microbial ecosystem in inflammatory bowel diseases. Nature 569, 655–662.
Lloyd-Price, J., Mahurkar, A., Rahnavard, G., Crabtree, J., Orvis, J., Hall, A. B., et al. (2017). Strains, functions and dynamics in the expanded Human Microbiome Project. Nature 550, 61–66. doi: 10.1038/nature23889
Lopez-Siles, M., Enrich-Capó, N., Aldeguer, X., Sabat-Mir, M., Duncan, S. H., Garcia-Gil, L. J., et al. (2018). Alterations in the abundance and co-occurrence of Akkermansia muciniphila and Faecalibacterium prausnitzii in the colonic mucosa of inflammatory bowel disease subjects. Front. Cell. Infect. Microbiol. 8:281. doi: 10.3389/fcimb.2018.00281
Louca, S., Doebeli, M., and Parfrey, L. W. (2018). Correcting for 16S rRNA gene copy numbers in microbiome surveys remains an unsolved problem. Microbiome 6:41.
Lukjancenko, O., Wassenaar, T. M., and Ussery, D. W. (2010). Comparison of 61 sequenced Escherichia coli genomes. Microb. Ecol. 60, 708–720. doi: 10.1007/s00248-010-9717-3
Magnúsdóttir, S., and Thiele, I. (2018). Modeling metabolism of the human gut microbiome. Curr. Opin. Biotechnol. 51, 90–96. doi: 10.1016/j.copbio.2017.12.005
Maier, B. R., and Hentges, D. J. (1972). Experimental Shigella infections in laboratory animals I. Antagonism by human normal flora components in gnotobiotic mice. Infect. Immun. 6, 168–173. doi: 10.1128/iai.6.2.168-173.1972
Marcelino, V. R., Clausen, P. T., Buchmann, J. P., Wille, M., Iredell, J. R., Meyer, W., et al. (2020a). CCMetagen: comprehensive and accurate identification of eukaryotes and prokaryotes in metagenomic data. Genome Biol. 21:103.
Marcelino, V. R., Holmes, E. C., and Sorrell, T. C. (2020b). The use of taxon-specific reference databases compromises metagenomic classification. BMC Genomics 21:184. doi: 10.1186/s12864-020-6592-2
Maschmeyer, I., Lorenz, A. K., Schimek, K., Hasenberg, T., Ramme, A. P., Hübner, J., et al. (2015). A four-organ-chip for interconnected long-term co-culture of human intestine, liver, skin and kidney equivalents. Lab Chip 15, 2688–2699. doi: 10.1039/c5lc00392j
Maurer, M., Gresnigt, M. S., Last, A., Wollny, T., Berlinghof, F., Pospich, R., et al. (2019). A three-dimensional immunocompetent intestine-on-chip model as in vitro platform for functional and microbial interaction studies. Biomaterials 220:119396. doi: 10.1016/j.biomaterials.2019.119396
McIntyre, A. B., Ounit, R., Afshinnekoo, E., Prill, R. J., Hénaff, E., Alexander, N., et al. (2017). Comprehensive benchmarking and ensemble approaches for metagenomic classifiers. Genome Biol. 18:182.
Meng, C., Zeleznik, O. A., Thallinger, G. G., Kuster, B., Gholami, A. M., and Culhane, A. C. (2016). Dimension reduction techniques for the integrative analysis of multi-omics data. Brief. Bioinform. 17, 628–641. doi: 10.1093/bib/bbv108
Minich, J. J., Humphrey, G., Benitez, R. A., Sanders, J., Swafford, A., Allen, E. E., et al. (2018). High-throughput miniaturized 16S rRNA amplicon library preparation reduces costs while preserving microbiome integrity. mSystems 3:e00166-18.
Moran, M. A. (2009). Metatranscriptomics: eavesdropping on complex microbial communities. Microbe 4, 329–335. doi: 10.1128/microbe.4.329.1
Müller, A., Hundt, C., Hildebrandt, A., Hankeln, T., and Schmidt, B. (2017). MetaCache: context-aware classification of metagenomic reads using minhashing. Bioinformatics 33, 3740–3748. doi: 10.1093/bioinformatics/btx520
Namkung, J. (2020). Machine learning methods for microbiome studies. J. Microbiol. 58, 206–216. doi: 10.1007/s12275-020-0066-8
Nauman, M., Rehman, H. U., Politano, G., and Benso, A. (2019). Beyond homology transfer: deep learning for automated annotation of proteins. J. Grid Comput. 17, 225–237. doi: 10.1007/s10723-018-9450-6
Neville, B. A., Forster, S. C., and Lawley, T. D. (2018). Commensal Koch’s postulates: establishing causation in human microbiota research. Curr. Opin. Microbiol. 42, 47–52. doi: 10.1016/j.mib.2017.10.001
Noecker, C., Eng, A., Srinivasan, S., Theriot, C. M., Young, V. B., Jansson, J. K., et al. (2016). Metabolic model-based integration of microbiome taxonomic and metabolomic profiles elucidates mechanistic links between ecological and metabolic variation. mSystems 1:e00013-15.
Numberger, D., Ganzert, L., Zoccarato, L., Mühldorfer, K., Sauer, S., Grossart, H.-P., et al. (2019). Characterization of bacterial communities in wastewater with enhanced taxonomic resolution by full-length 16S rRNA sequencing. Sci. Rep. 9:9673.
Ochman, H., Worobey, M., Kuo, C.-H., Ndjango, J.-B. N., Peeters, M., Hahn, B. H., et al. (2010). Evolutionary relationships of wild hominids recapitulated by gut microbial communities. PLoS Biol. 8:e1000546. doi: 10.1371/journal.pbio.1000546
Ott, S., Musfeldt, M., Wenderoth, D., Hampe, J., Brant, O., Fölsch, U., et al. (2004). Reduction in diversity of the colonic mucosa associated bacterial microflora in patients with active inflammatory bowel disease. Gut 53, 685–693. doi: 10.1136/gut.2003.025403
Palsson, B., and Zengler, K. (2010). The challenges of integrating multi-omic data sets. Nat. Chem. Biol. 6, 787–789. doi: 10.1038/nchembio.462
Parada Venegas, D., De La Fuente, M. K., Landskron, G., González, M. J., Quera, R., Dijkstra, G., et al. (2019). Short chain fatty acids (SCFAs)-mediated gut epithelial and immune regulation and its relevance for inflammatory bowel diseases. Front. Immunol. 10:277. doi: 10.3389/fimmu.2019.00277
Park, J. C., and Im, S.-H. (2020). Of men in mice: the development and application of a humanized gnotobiotic mouse model for microbiome therapeutics. Exp. Mol. Med. 52, 1383–1396. doi: 10.1038/s12276-020-0473-2
Parks, D. H., Chuvochina, M., Chaumeil, P.-A., Rinke, C., Mussig, A. J., and Hugenholtz, P. (2020). A complete domain-to-species taxonomy for Bacteria and Archaea. Nat. Biotechnol. 38, 1079–1086. doi: 10.1038/s41587-020-0501-8
Parks, D. H., Rinke, C., Chuvochina, M., Chaumeil, P.-A., Woodcroft, B. J., Evans, P. N., et al. (2017). Recovery of nearly 8,000 metagenome-assembled genomes substantially expands the tree of life. Nat. Microbiol. 2, 1533–1542. doi: 10.1038/s41564-017-0012-7
Pearce, S. C., Coia, H. G., Karl, J. P., Pantoja-Feliciano, I. G., Zachos, N. C., and Racicot, K. (2018). Intestinal in vitro and ex vivo models to study host-microbiome interactions and acute stressors. Front. Physiol. 9:1584. doi: 10.3389/fphys.2018.01584
Picollet-D’hahan, N., Zuchowska, A., Lemeunier, I., and Le Gac, S. (2021). Multiorgan-on-a-chip: a systemic approach to model and decipher inter-organ communication. Trends Biotechnol. doi: 10.1016/j.tibtech.2020.11.014 [Epub ahead of print].
Pinu, F. R., Beale, D. J., Paten, A. M., Kouremenos, K., Swarup, S., Schirra, H. J., et al. (2019). Systems biology and multi-omics integration: viewpoints from the metabolomics research community. Metabolites 9:76. doi: 10.3390/metabo9040076
Poretsky, R., Rodriguez-R, L. M., Luo, C., Tsementzi, D., and Konstantinidis, K. T. (2014). Strengths and limitations of 16S rRNA gene amplicon sequencing in revealing temporal microbial community dynamics. PLoS One 9:e93827. doi: 10.1371/journal.pone.0093827
Qin, J., Li, R., Raes, J., Arumugam, M., Burgdorf, K. S., Manichanh, C., et al. (2010). A human gut microbial gene catalogue established by metagenomic sequencing. Nature 464, 59–65.
Qin, J., Li, Y., Cai, Z., Li, S., Zhu, J., Zhang, F., et al. (2012). A metagenome-wide association study of gut microbiota in type 2 diabetes. Nature 490, 55–60.
Rahman, S. F., Olm, M. R., Morowitz, M. J., and Banfield, J. F. (2018). Machine learning leveraging genomes from metagenomes identifies influential antibiotic resistance genes in the infant gut microbiome. mSystems 3:e00123-17.
Raman, A. S., Gehrig, J. L., Venkatesh, S., Chang, H.-W., Hibberd, M. C., Subramanian, S., et al. (2019). A sparse covarying unit that describes healthy and impaired human gut microbiota development. Science 365:eaau4735. doi: 10.1126/science.aau4735
Rifaioglu, A. S., Doðan, T., Martin, M. J., Cetin-Atalay, R., and Atalay, V. (2019). DEEPred: automated protein function prediction with multi-task feed-forward deep neural networks. Sci. Rep. 9:7344.
Rivera-Pinto, J., Egozcue, J. J., Pawlowsky-Glahn, V., Paredes, R., Noguera-Julian, M., and Calle, M. L. (2018). Balances: a new perspective for microbiome analysis. mSystems 3:e00053-18.
Roxas, J. L., Koutsouris, A., Bellmeyer, A., Tesfay, S., Royan, S., Falzari, K., et al. (2010). Enterohemorrhagic E. coli alters murine intestinal epithelial tight junction protein expression and barrier function in a Shiga toxin independent manner. Lab. Invest. 90, 1152–1168. doi: 10.1038/labinvest.2010.91
Ryan, F. J., Ahern, A., Fitzgerald, R., Laserna-Mendieta, E., Power, E., Clooney, A., et al. (2020). Colonic microbiota is associated with inflammation and host epigenomic alterations in inflammatory bowel disease. Nat. Commun. 11:1512.
Sadabad, M. S., Von Martels, J. Z., Khan, M. T., Blokzijl, T., Paglia, G., Dijkstra, G., et al. (2015). A simple coculture system shows mutualism between anaerobic faecalibacteria and epithelial Caco-2 cells. Sci. Rep. 5:17906.
Sato, T., Vries, R. G., Snippert, H. J., Van De Wetering, M., Barker, N., Stange, D. E., et al. (2009). Single Lgr5 stem cells build crypt-villus structures in vitro without a mesenchymal niche. Nature 459, 262–265. doi: 10.1038/nature07935
Sberro, H., Fremin, B. J., Zlitni, S., Edfors, F., Greenfield, N., Snyder, M. P., et al. (2019). Large-scale analyses of human microbiomes reveal thousands of small, novel genes. Cell 178, 1245–1259.e14.
Schirmer, M., Smeekens, S. P., Vlamakis, H., Jaeger, M., Oosting, M., Franzosa, E. A., et al. (2016). Linking the human gut microbiome to inflammatory cytokine production capacity. Cell 167, 1125–1136.e8.
Schöler, A., Jacquiod, S., Vestergaard, G., Schulz, S., and Schloter, M. (2017). Analysis of soil microbial communities based on amplicon sequencing of marker genes. Biol. Fertil. Soils 53, 485–489. doi: 10.1007/s00374-017-1205-1
Sczyrba, A., Hofmann, P., Belmann, P., Koslicki, D., Janssen, S., Dröge, J., et al. (2017). Critical assessment of metagenome interpretation—a benchmark of metagenomics software. Nat. Methods 14, 1063–1071.
Seemann, T. (2014). Prokka: rapid prokaryotic genome annotation. Bioinformatics 30, 2068–2069. doi: 10.1093/bioinformatics/btu153
Sekhon, M. K., Grubb, P. H., Newman, M., and Yoder, B. A. (2019). Implementation of a probiotic protocol to reduce rates of necrotizing enterocolitis. J. Perinatol. 39, 1315–1322. doi: 10.1038/s41372-019-0443-5
Shaffer, M., Borton, M. A., Mcgivern, B. B., Zayed, A. A., La Rosa, S. L., Solden, L. M., et al. (2020). DRAM for distilling microbial metabolism to automate the curation of microbiome function. Nucleic Acids Res. 48, 8883–8900. doi: 10.1093/nar/gkaa621
Smits, H. H., Engering, A., Van Der Kleij, D., De Jong, E. C., Schipper, K., Van Capel, T. M., et al. (2005). Selective probiotic bacteria induce IL-10–producing regulatory T cells in vitro by modulating dendritic cell function through dendritic cell–specific intercellular adhesion molecule 3–grabbing nonintegrin. J. Allergy Clin. Immunol. 115, 1260–1267. doi: 10.1016/j.jaci.2005.03.036
Steinway, S. N., Biggs, M. B., Loughran, T. P. Jr., Papin, J. A., and Albert, R. (2015). Inference of network dynamics and metabolic interactions in the gut microbiome. PLoS Comput. Biol. 11:e1004338. doi: 10.1371/journal.pcbi.1004338
Stevens, B. R., Roesch, L., Thiago, P., Russell, J. T., Pepine, C. J., Holbert, R. C., et al. (2020). Depression phenotype identified by using single nucleotide exact amplicon sequence variants of the human gut microbiome. Mol. Psychiatry. doi: 10.1038/s41380-020-0652-5
Sun, S., Jones, R. B., and Fodor, A. A. (2020). Inference-based accuracy of metagenome prediction tools varies across sample types and functional categories. Microbiome 8:46.
Ten Hoopen, P., Finn, R. D., Bongo, L. A., Corre, E., Fosso, B., Meyer, F., et al. (2017). The metagenomic data life-cycle: standards and best practices. GigaScience 6:gix047. doi: 10.1093/gigascience/gix047
Thiele, I., and Palsson, B. Ø. (2010). A protocol for generating a high-quality genome-scale metabolic reconstruction. Nat. Protoc. 5, 93–121. doi: 10.1038/nprot.2009.203
Thomas, A. M., Manghi, P., Asnicar, F., Pasolli, E., Armanini, F., Zolfo, M., et al. (2019). Metagenomic analysis of colorectal cancer datasets identifies cross-cohort microbial diagnostic signatures and a link with choline degradation. Nat. Med. 25, 667–678. doi: 10.1038/s41591-019-0405-7
Thomas, M. C., Thomas, D. K., Kalmokoff, M. L., Brooks, S. P., and Selinger, L. B. (2012). Molecular methods to measure intestinal bacteria: a review. J. AOAC Int. 95, 5–23. doi: 10.5740/jaoacint.sge_inglis
Thorsen, J., Brejnrod, A., Mortensen, M., Rasmussen, M. A., Stokholm, J., Al-Soud, W. A., et al. (2016). Large-scale benchmarking reveals false discoveries and count transformation sensitivity in 16S rRNA gene amplicon data analysis methods used in microbiome studies. Microbiome 4:62.
Tikhonov, M., Leach, R. W., and Wingreen, N. S. (2015). Interpreting 16S metagenomic data without clustering to achieve sub-OTU resolution. ISME J. 9, 68–80. doi: 10.1038/ismej.2014.117
Tovaglieri, A., Sontheimer-Phelps, A., Geirnaert, A., Prantil-Baun, R., Camacho, D. M., Chou, D. B., et al. (2019). Species-specific enhancement of enterohemorrhagic E. coli pathogenesis mediated by microbiome metabolites. Microbiome 7:43.
Truong, D. T., Franzosa, E. A., Tickle, T. L., Scholz, M., Weingart, G., Pasolli, E., et al. (2015). MetaPhlAn2 for enhanced metagenomic taxonomic profiling. Nat. Methods 12, 902–903. doi: 10.1038/nmeth.3589
Turnbaugh, P. J., Ley, R. E., Hamady, M., Fraser-Liggett, C. M., Knight, R., and Gordon, J. I. (2007). The human microbiome project. Nature 449, 804–810.
Turnbaugh, P. J., Quince, C., Faith, J. J., Mchardy, A. C., Yatsunenko, T., Niazi, F., et al. (2010). Organismal, genetic, and transcriptional variation in the deeply sequenced gut microbiomes of identical twins. Proc. Natl. Acad. Sci. U.S.A. 107, 7503–7508. doi: 10.1073/pnas.1002355107
Turnbaugh, P. J., Ridaura, V. K., Faith, J. J., Rey, F. E., Knight, R., and Gordon, J. I. (2009). The effect of diet on the human gut microbiome: a metagenomic analysis in humanized gnotobiotic mice. Sci. Transl. Med. 1:6ra14. doi: 10.1126/scitranslmed.3000322
Underwood, M. A. (2019). Probiotics and the prevention of necrotizing enterocolitis. J. Pediatr. Surg. 54, 405–412.
Valles-Colomer, M., Falony, G., Darzi, Y., Tigchelaar, E. F., Wang, J., Tito, R. Y., et al. (2019). The neuroactive potential of the human gut microbiota in quality of life and depression. Nat. Microbiol. 4, 623–632. doi: 10.1038/s41564-018-0337-x
van de Wetering, M., Francies, H. E., Francis, J. M., Bounova, G., Iorio, F., Pronk, A., et al. (2015). Prospective derivation of a living organoid biobank of colorectal cancer patients. Cell 161, 933–945. doi: 10.1016/j.cell.2015.03.053
Van Den Berg, A., Mummery, C. L., Passier, R., and Van Der Meer, A. D. (2019). Personalised organs-on-chips: functional testing for precision medicine. Lab Chip 19, 198–205. doi: 10.1039/c8lc00827b
Vatanen, T., Franzosa, E. A., Schwager, R., Tripathi, S., Arthur, T. D., Vehik, K., et al. (2018). The human gut microbiome in early-onset type 1 diabetes from the TEDDY study. Nature 562, 589–594. doi: 10.1038/s41586-018-0620-2
Veckman, V., Miettinen, M., Pirhonen, J., Sirén, J., Matikainen, S., and Julkunen, I. (2004). Streptococcus pyogenes and Lactobacillus rhamnosus differentially induce maturation and production of Th1-type cytokines and chemokines in human monocyte-derived dendritic cells. J. Leukoc. Biol. 75, 764–771. doi: 10.1189/jlb.1003461
Vervier, K., Mahé, P., Tournoud, M., Veyrieras, J.-B., and Vert, J.-P. (2016). Large-scale machine learning for metagenomics sequence classification. Bioinformatics 32, 1023–1032. doi: 10.1093/bioinformatics/btv683
Vickerman, V., Blundo, J., Chung, S., and Kamm, R. (2008). Design, fabrication and implementation of a novel multi-parameter control microfluidic platform for three-dimensional cell culture and real-time imaging. Lab Chip 8, 1468–1477. doi: 10.1039/b802395f
Vijay-Kumar, M., Sanders, C. J., Taylor, R. T., Kumar, A., Aitken, J. D., Sitaraman, S. V., et al. (2007). Deletion of TLR5 results in spontaneous colitis in mice. J. Clin. Invest. 117, 3909–3921.
Vila, A. V., Imhann, F., Collij, V., Jankipersadsing, S. A., Gurry, T., Mujagic, Z., et al. (2018). Gut microbiota composition and functional changes in inflammatory bowel disease and irritable bowel syndrome. Sci. Transl. Med. 10:eaa8914.
Watanabe, N., Kryukov, K., Nakagawa, S., Takeuchi, J. S., Takeshita, M., Kirimura, Y., et al. (2018). Detection of pathogenic bacteria in the blood from sepsis patients using 16S rRNA gene amplicon sequencing analysis. PLoS One 13:e0202049. doi: 10.1371/journal.pone.0202049
Westcott, S. L., and Schloss, P. D. (2015). De novo clustering methods outperform reference-based methods for assigning 16S rRNA gene sequences to operational taxonomic units. PeerJ 3:e1487. doi: 10.7717/peerj.1487
Williamson, I. A., Arnold, J. W., Samsa, L. A., Gaynor, L., Disalvo, M., Cocchiaro, J. L., et al. (2018). A high-throughput organoid microinjection platform to study gastrointestinal microbiota and luminal physiology. Cell. Mol. Gastroenterol. Hepatol. 6, 301–319. doi: 10.1016/j.jcmgh.2018.05.004
Wilmes, P., and Bond, P. L. (2006). Metaproteomics: studying functional gene expression in microbial ecosystems. Trends Microbiol. 14, 92–97. doi: 10.1016/j.tim.2005.12.006
Wilson, G. S., Raftos, D. A., Corrigan, S. L., and Nair, S. V. (2010). Diversity and antimicrobial activities of surface-attached marine bacteria from Sydney Harbour, Australia. Microbiol. Res. 165, 300–311. doi: 10.1016/j.micres.2009.05.007
Wood, D. E., Lu, J., and Langmead, B. (2019). Improved metagenomic analysis with Kraken 2. Genome Biol. 20:257.
Wu, H.-J., Ivanov, I. I., Darce, J., Hattori, K., Shima, T., Umesaki, Y., et al. (2010). Gut-residing segmented filamentous bacteria drive autoimmune arthritis via T helper 17 cells. Immunity 32, 815–827. doi: 10.1016/j.immuni.2010.06.001
Xie, H., Yang, C., Sun, Y., Igarashi, Y., Jin, T., and Luo, F. (2020). PacBio long reads improve metagenomic assemblies, gene catalogs, and genome binning. Front. Genet. 11:516269. doi: 10.3389/fgene.2020.516269
Yu, J., Feng, Q., Wong, S. H., Zhang, D., Yi Liang, Q., Qin, Y., et al. (2017). Metagenomic analysis of faecal microbiome as a tool towards targeted non-invasive biomarkers for colorectal cancer. Gut 66, 70–78. doi: 10.1136/gutjnl-2015-309800
Zackular, J. P., Moore, J. L., Jordan, A. T., Juttukonda, L. J., Noto, M. J., Nicholson, M. R., et al. (2016). Dietary zinc alters the microbiota and decreases resistance to Clostridium difficile infection. Nat. Med. 22, 1330–1334. doi: 10.1038/nm.4174
Zhang, K., Martiny, A. C., Reppas, N. B., Barry, K. W., Malek, J., Chisholm, S. W., et al. (2006). Sequencing genomes from single cells by polymerase cloning. Nat. Biotechnol. 24, 680–686. doi: 10.1038/nbt1214
Zhang, Z., Geng, J., Tang, X., Fan, H., Xu, J., Wen, X., et al. (2014). Spatial heterogeneity and co-occurrence patterns of human mucosal-associated intestinal microbiota. ISME J. 8, 881–893. doi: 10.1038/ismej.2013.185
Keywords: microbiome, faecal transplant, gastrointestinal disorder, 16S rRNA sequencing, metagenomic sequencing, microbial genomics, bacteriotherapy, live biotherapeutics
Citation: Young RB, Marcelino VR, Chonwerawong M, Gulliver EL and Forster SC (2021) Key Technologies for Progressing Discovery of Microbiome-Based Medicines. Front. Microbiol. 12:685935. doi: 10.3389/fmicb.2021.685935
Received: 26 March 2021; Accepted: 25 May 2021;
Published: 22 June 2021.
Edited by:
George Tsiamis, University of Patras, GreeceReviewed by:
Christopher L. Hemme, University of Rhode Island, United StatesCopyright © 2021 Young, Marcelino, Chonwerawong, Gulliver and Forster. This is an open-access article distributed under the terms of the Creative Commons Attribution License (CC BY). The use, distribution or reproduction in other forums is permitted, provided the original author(s) and the copyright owner(s) are credited and that the original publication in this journal is cited, in accordance with accepted academic practice. No use, distribution or reproduction is permitted which does not comply with these terms.
*Correspondence: Samuel C. Forster, c2FtLmZvcnN0ZXJAaHVkc29uLm9yZy5hdQ==
Disclaimer: All claims expressed in this article are solely those of the authors and do not necessarily represent those of their affiliated organizations, or those of the publisher, the editors and the reviewers. Any product that may be evaluated in this article or claim that may be made by its manufacturer is not guaranteed or endorsed by the publisher.
Research integrity at Frontiers
Learn more about the work of our research integrity team to safeguard the quality of each article we publish.