- 1College of Food Science, Fujian Agriculture and Forestry University, Fuzhou, China
- 2College of Life Science, Ningde Normal University, Ningde, China
- 3Fujian Higher Education Research Center for Local Biological Resources, Ningde, China
- 4Fujian Anjoy Food Co., Ltd., Xiamen, China
The objectives of this study were to isolate and identify the dominant microorganism in Flammulina velutipes fruiting bodies (FVFB) and to develop kinetic models for describing its growth. The native microflora community on FVFB was isolated and identified using morphological examination and high-throughput sequencing analysis. FVFB presented complex microbial communities with dominant microorganisms being Lactococcus lactis. Irradiated FVFB were inoculated with the isolated strain of L. lactis and cultivated at various temperatures (4, 10, 16, 20, 25, 32, and 37°C). Three primary models, namely the Huang, Baranyi and Roberts, and reparameterized Gompertz models, and three secondary models, namely the Huang square-root, Ratkowsky square-root, and Arrhenius-type models, were developed and evaluated. With the lowest values of mean square error (MSE, 0.023–0.161) and root mean square error (RMSE, 0.152–0.401) values, the reparameterized Gompertz model was more suitable to describe the growth of L. lactis on FVFB than both Huang and Baranyi and Roberts models. The Ratkowsky square-root model provided more accurate estimation for the effect of temperature on the specific growth rate of L. lactis. The minimum growth temperature predicted by the Ratkowsky square-root model was −7.1°C. The kinetic models developed in this study could be used to evaluate the growth behavior of L. lactis on FVFB and estimate the shelf-life of FVFB.
Introduction
Flammulina velutipes, known as golden needle mushroom, is one of the major edible mushrooms employed in factory cultivation in East Asia (Fang et al., 2015). F. velutipes had various distinct advantages, owing to its simple cultivation technique, ability of fast fruiting, high yield, desirable taste, and rich in nutrients (essential amino acids, dietary fiber, protein, vitamins, and low in fat). Since Flammulina velutipes fruiting bodies (FVFB) have a variety of bioactivities, such as hepatoprotective, antitumor, antihyperlipidemia, immune regulation, and so on (Zhao et al., 2019; Wang and Zhang, 2021), the consumption demands of FVFB were increasing rapidly. The shelf-life of postharvest FVFB is very short (1∼3 days) when stored under ambient condition. The deterioration of postharvest FVFB occurs easily during storage. The main reason might be microbial growth (Shi et al., 2018). Niu et al. (2020) reported that Pseudomonas spp. is spoilage organisms on postharvest FVFB. FVFB had a neutral pH value and high moisture content, which could be a suitable medium for the growth of pathogenic and spoilage bacteria.
Lactococcus lactis is a Gram-positive bacterium. It is one of the most important microorganisms used extensively in dairy products and other fermented foods (Odamaki et al., 2011). L. lactis could survive and grow well in many foods, which could utilize carbohydrates fermentatively and form lactic acid as an end-product. The increasing amount of L. lactis and lactic acid in FVFB could significantly reduce its quality by causing softening, browning, rotting, and deterioration. The texture and pH value of FVFB also could be significantly changed with the increasing population of L. lactis. Thus, the commercial value of postharvest FVFB could decrease during storage. Based on the beneficial use of L. lactis in food fermentation, L. lactis has generally been recognized as safe status (Wessels et al., 2004; Food and Drug Administration, 2012). However, some studies had also reported some species of Lactococcus to an opportunistic pathogen (Mannion and Rothburn, 1990; Aguirre and Collins, 1993). Rodrigues et al. (2016) suggested that the Lactococcus is a potential etiological agent in mastitis outbreak. Zhao et al. (2013) showed that the pathogen causing postharvest water-soaked and sunken lesions on the stipes and decay of Pleurotus eryngii was isolated and identified as L. lactis subsp. lactis. Therefore, L. lactis could be potential pathogenic microbes for the mushroom and food industry.
The predictive microbiology models were available to describe the microbial growth, survival, or inactivation in response to environmental conditions (Laurent, 2016; Akkermans et al., 2018). Primary models are used to describe microbial growth data as a function of time under constant environmental conditions (Whiting and Buchanan, 1993). The reparameterized Gompertz model (Zwietering et al., 1990; Buchanan et al., 1997), Huang model (Huang, 2013), Baranyi and Roberts model, and logistic model (Tashiro and Yoshimura, 2019) are generally reported as the primary models. A secondary model deals with the response of parameters appearing in primary modeling approaches as a function of one or more environmental conditions like temperature, pH, etc. (Whiting and Buchanan, 1993). The commonly described secondary models include the Huang square root, Arrhenius type, and Ratkowsky square root (Whiting and Buchanan, 1993; Huang, 2014).
However, there is limited information in the literature about microbial communities and L. lactis growth behavior on fresh postharvest FVFB. Therefore, in this study, native microflora on the surface of FVFB were analyzed and discriminated using 16S rRNA gene amplification and high-throughput sequencing. The growth behavior of dominant microflora (L. lactis) was studied at different storage temperatures. Mathematical models were fitted to the data and compared to select an accurate model for describing the growth of the dominant microflora. This study could provide useful information to predict the spoilage of FVFB caused by L. lactis. The models developed in this study may be used by the food industry and regulatory agencies to estimate the growth of L. lactis in FVFB.
Materials and Methods
Sample Collection
The FVFB samples were purchased from five different local markets (FV1, FV2, FV3, FV4, and FV5) in Fuzhou, China. FV1 was from Laoguangcun market (farm market), FV2 from Yonghui superstore, FV3 from RT-Mart, FV4 from Walmart, and FV5 from Super Species.
Native Microflora Isolation and Identification
Twenty-five grams of FVFB were transferred into a sterile plastic bag (19 × 30 cm, Huankai Microbial Co., Ltd., Guangzhou, China) and homogenized in 225 ml 0.1% sterile peptone water (PW). Samples were serially diluted using 0.1% sterile PW. An aliquot of 100 μl was transferred onto Luria–Bertani (LB) agar plates (Huankai Microbial Co., Ltd., Guangzhou, China) and incubated at 37°C for 48 h. Different types of bacterial colonies on LB plates were isolated and transferred into 10 ml of LB broth (Huankai Microbial Co., Ltd., Guangzhou, China). The bacterial cultures were harvested after three consecutive transfers. The cultures were maintained in LB plates and stored at 4°C until use. Each isolated culture obtained was stained by Gram staining and examined using an Eclipse E100 microscope (Nikon, Japan).
Each isolated culture was mixed with 20 μl of double-distilled water and boiled for 2 mins. The bacterial suspension was centrifuged at 12,000 rpm for 1 min. DNA in the supernatants was extracted using a DNA extraction kit (Sangon Biotech, Shanghai, China) according to the manufacturer’s protocol. PCR was carried out on a Mastercycler gradient (Eppendorf, Germany) using 50 μl reaction volumes, containing 2 μl primers (5 μM), 25 μl 2 × Taq PCR Master Mix, 2 μl template DNA, and 21 μl ddH2O. The cycling conditions were as follows: initial denaturation at 94°C for 5 min, followed by 35 cycles of denaturation at 95°C for 45 s, annealing at 56°C for 45 s, extension at 72°C for 90 s, and final extension at 72°C for 10 min. The PCR products were qualified and visualized using 1% agarose gel electrophoresis (Sangon Biotech, Shanghai, China) and analyzed by 16S rRNA gene sequencing analysis. Sequences were compared with the National Center for Biotechnology Information (NCBI,1) database using the basic local alignment search tool (BLAST) algorithm.
High-Throughput Sequencing Analysis
The DNA of FVFB was extracted using PowerSoil DNA Isolation Kit (MoBio Laboratories, Carlsbad, CA, United States) according to the protocol provided by the manufacturer. The universal primer sets of 338F (5′-ACTCCTACGGGAGGCAGCAG-3′) and 806R (5′-GGACTACHVGGGTWTCTAAT-3′) were used to amplify the V3--V4 hypervariable region of 16S rRNA genes. High-throughput sequencing was conducted using the Illumina HiSeq 2500 platform at Allwegene Technology Co., Ltd., (Beijing, China). Qualified reads were separated using the sample-specific barcode sequences and trimmed with Illumina Analysis Pipeline Version 2.6. Sequences were analyzed using QIIME (Version 1.8,2) software package and in-house Perl scripts to determine alpha diversity and beta diversity. Sequences with the level of 97% similarity were assigned to the same operational taxonomic units (OTUs). The Ribosomal Database Project classifier was used to annotate taxonomic information for each representative sequence.
Dominant Bacteria and Preparation
Lactococcus lactis was obtained from FVFB. The culture of L. lactis was revived by inoculating into 10 ml of LB tube and incubating at 37°C for 20 h. After incubation, the bacterial culture was centrifuged at 4,500 rpm for 15 min (4°C), washed three times using 0.1% sterile PW, and then resuspended in 10 ml of 0.1% sterile PW. The suspension was serially diluted to obtain a final concentration of 105 to 106 CFU/ml as working culture.
Sample Preparation and Inoculation
Flammulina velutipes fruiting bodies were purchased from a local market and irradiated (Rice Research Institute, Fujian Academy of Agricultural Sciences, Fuzhou, Fujian, China) at a dose of 5 kg. Afterward, 25 g of FVFB were placed into a sterile plastic bag and inoculated with 0.1 ml of L. lactis working culture. The inoculated FVFB samples were incubated at constant temperatures of 4, 10, 16, 20, 25, 32, and 37°C, respectively. Depending on the incubation temperature, samples were retrieved from the incubators at different time intervals. For each sample, 225 ml 0.1% sterile PW was added and homogenized in a stomacher for 20 s (Model BagMixer 400 W, Interscience Co., France). Each sample was serially diluted with PW. Each sample was mixed with 225 ml of 0.1% sterile PW and homogenized in a stomacher (Model BagMixer 400 W, Interscience Co., France). The liquid portion of the homogenized samples was serially diluted with 0.1% sterile PW and plated onto de Man–Rogosa–Sharpe (MRS, Huankai Microbial Co., Ltd., Guangzhou, China) agar plates. All plates were incubated at 37°C for 48 h. The L. lactis colonies were enumerated and converted to the logarithm of base 10 or natural base, recorded as log CFU/g or ln CFU/g. Each experiment was repeated three times.
Primary Models
In this study, three primary models were used for describing L. lactis growth: Huang model (Eqs 1, 2, Huang, 2013), Baranyi and Roberts model (Eqs 3, 4, Baranyi and Roberts, 1995), and reparameterized Gompertz model (Eq. 5, Zwietering et al., 1990). The Huang model is expressed as
where t is the time point (h), λ is the lag phase duration (h), Y(t) represents the natural logarithm of microorganism count (ln CFU/g), Y0 is the initial microorganisms count (ln CFU/g), Ymax is the bacterial count (ln CFU/g) at the stationary phase, μmax is the maximum specific growth rate (h–1), and α is a constant (α = 4) that defines the transition from the lag phase to the exponential phase of a growth curve (Huang, 2013).
The Baranyi and Roberts model is expressed as
where h0 is the physiological state of the microorganism and equals to λ × μmax, and the other variables, i.e., Y(t), Y0, Ymax, and μmax, are defined as those used in the Huang model. Firstly, the h0 values are estimated and an h0 averaged value is established. Then, μmax, Y0, and Ymax are estimated with fixed h0 averaged value.
The reparameterized Gompertz model is expressed as
where λ, t, Y(t), Y0, Ymax, and μmax are identical to those used in the Huang model.
Secondary Models
Secondary models were fitted to the maximum specific growth rates (μmax) estimated from the primary models under different incubation temperatures. The Ratkowsky square-root (Eq. 6, Ratkowsky et al., 1982), Huang square-root (Eq. 7, Huang, 2014), and Arrhenius-type (Eq. 8, Huang et al., 2011) models were used to evaluate the effect of temperature on growth rate.
In Equations 6–8a is a regression coefficient for each model, T is the incubation temperature (°C) for microbial growth, T0 is the nominal minimum temperature (°C), Tmin is the estimated minimum growth temperature (°C), and a and n are regression coefficients. In Eq. 8, R is the universal gas constant [8.314 J/(mol K)], and ΔG′ is a type of activation energy related to bacterial growth (J/mol).
Modeling and Statistical Analysis
Growth curves of L. lactis on FVFB at different isothermal temperatures were analyzed using the United States Department of Agriculture (USDA) Integrated Pathogen Modeling Program (IPMP, Huang, 2014). Lag phase durations, specific growth rates, and maximum concentrations of L. lactis under different conditions were obtained from IPMP analysis.
Results and Discussion
Identification of the Native Microbiota in FVFB
The 16S rRNA gene amplification and high-throughput sequencing analysis were used to identify the native microflora in FVFB. In this research, a total of 21 types of bacterial colonies were isolated and obtained from FVFB in different markets (FV1, FV2, FV3, FV4, and FV5). Each isolated culture was analyzed as shown in Figure 1, and the lengths of DNA fragments extracted from isolated cultures were similar and approximately 1,800 bp. DNA sequences obtained from high-throughput analysis were compared with the NCBI database using BLAST algorithm. A total of 12 species were identified and confirmed (Table 1). FV1 had more species of the bacteria than FV2, FV3, FV4, and FV5, which suggests that FVFB from the farm market (FV1) had a higher risk of being contaminated by the microorganism.
Morphological Examination
The cultural morphology of each selected bacteria was done and tested with Gram staining and used as a basic separation of bacteria into their taxonomy (Table 2 and Figure 2). A total of five Gram-positive bacteria were obtained, including Staphylococcus warneri, Bacillus amyloliquefaciens, Bacillus paramycoides, L. lactis, and Staphylococcus saprophyticus. A total of seven Gram-negative bacteria were obtained as follows: Ewingella americana, Citrobacter freundii, Raoultella ornithinolytica, Kluyvera cryocrescens, Pseudomonas aeruginosa, Klebsiella aerogenes, and Chryseobacterium indologenes. The morphological examination results demonstrated that the number of Gram-negative bacteria was more than the Gram-positive bacteria isolated in FVFB. The most identified isolates showed the shape of rods except S. warneri, L. lactis, and S. saprophyticus.
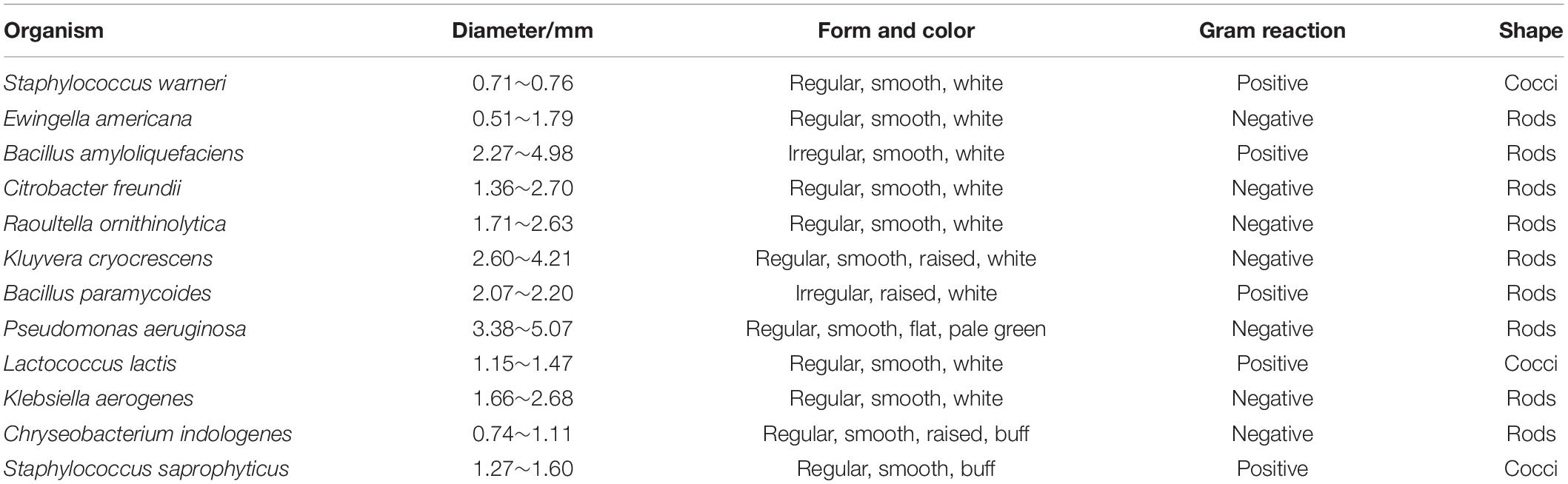
Table 2. Morphological characteristics of identified bacteria in Flammulina velutipes fruiting bodies.
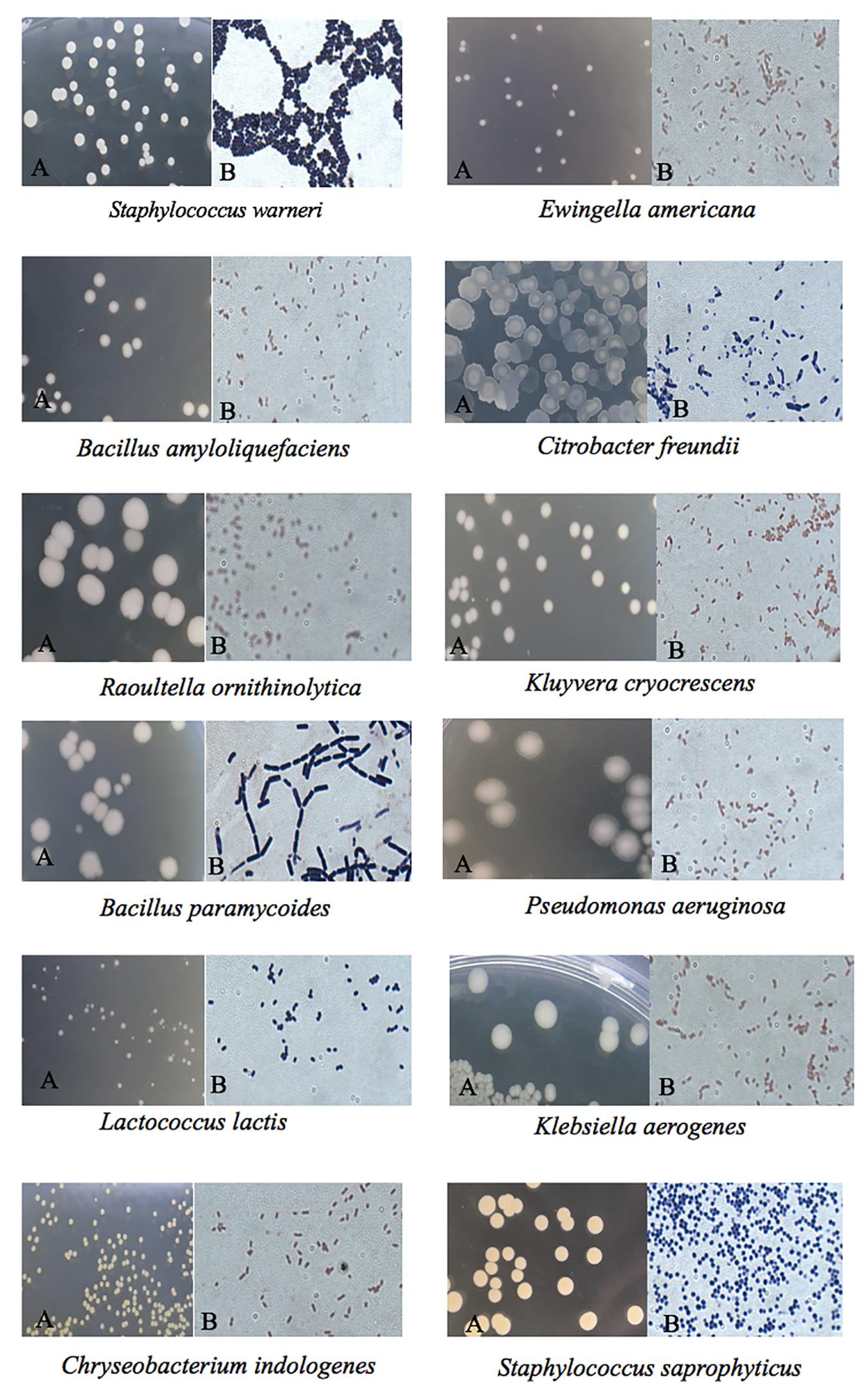
Figure 2. Colony morphology of the identified native microflora in Flammulina velutipes fruiting bodies under a microscope: (A) without staining and (B) Gram staining.
Native Microflora Composition and Diversity
The bacterial community, relative abundance, and diversity on the surfaces of FVFB were investigated by high-throughput sequencing analysis. The relative abundance at the phylum level of different bacteria in FVFB is shown in Figure 3A. Proteobacteria, Firmicutes, Bacteroidetes, Actinobacteria, Fusobacteria, and Tenericutes were identified on the surface of FVFB. Proteobacteria and Firmicutes were found to be the dominant microflora in FVFB.
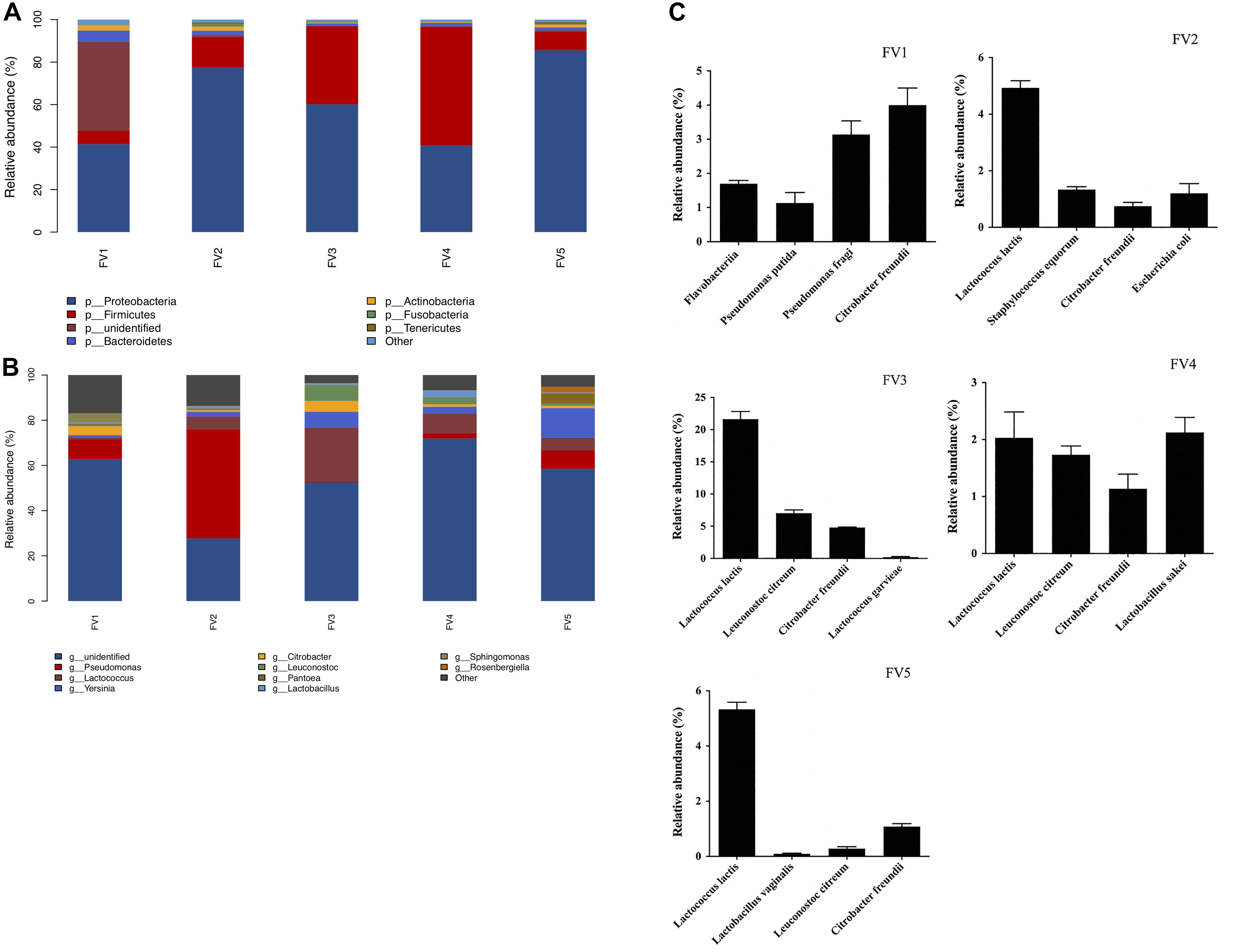
Figure 3. Relative abundance of native microflora in Flammulina velutipes fruiting bodies. (A) Phylum level, (B) genus level, and (C) species level.
At the genus level, 212 bacteria genera were obtained (the top 10 genera are shown in Figure 3B). Results demonstrated that there were complex microbial communities in FVFB. Pseudomonas was determined as the dominant microflora in FV1 and FV2. Based on the observations of Li et al. (2020), the dominant population of microflora was Pseudomonas spp., along with small proportions of Sphingobacterium, Pedobacter, Corynebacterium, and Pasteurella in Agaricus bisporus. Therefore, Pseudomonas spp. had high relative abundances in bacterial community structure in mushrooms. Lactococcus was determined as the dominant microflora in FV3 and FV4. Yersinia was determined as the dominant microflora in FV5. As shown in Figure 3C, the dominant microflora was Citrobacter freundii (4%) in FV1 at the species level. However, the dominant microflora was L. lactis in FV2 (4.9%), FV3 (21.6%), FV4 (5.3%), and FV5 (2%). These results were the same as those shown in Table 1, which indicated that L. lactis was the dominant microflora in FVFB. Lee et al. (2016) demonstrated that lactic acid bacteria were isolated from traditional Korean fermented vegetable. L. lactis was one of the most important microorganisms in the dairy industry and generally recognized as safe status. Tormo et al. (2015) reported that L. lactis was the dominant species in raw goat milk. However, some studies reported that L. lactis was the causal agent of postharvest decay of mushroom (Zhao et al., 2013, 2018). Furthermore, L. lactis had a homofermentative metabolism, and it does not directly cause soft rot, but it can produce lactic acid from carbohydrates and create favorable conditions for soft rot symptoms of various vegetables (Baheyeldin and Gahan, 2010; Song et al., 2017). During storage, the increasing amount of L. lactis in FVFB could be harmful to its quality by causing softening, browning, sunken lesions, and deterioration in FVFB. Therefore, the growth of L. lactis isolated from FVFB was further investigated in this study.
Mathematical Modeling
Lactococcus lactis and other bacteria could grow well in FVFB. At chilled temperature, competition from other psychotropics may not allow L. lactis to grow well and reach the maximum population. Due to the limitations of MRS plates, a few other bacteria could also grow in the MRS plate, and L. lactis could not be accurately determined by MRS plating (Dave and Shah, 1996). Therefore, to accurately assess the growth of L. lactis in FVFB, FVFB was irradiated to eliminate the influence of background microorganisms.
The Huang, Baranyi, and reparameterized Gompertz models were fitted to the growth data of L. lactis in FVFB at different isothermal storage temperatures (4, 10, 16, 20, 25, 32, and 37°C). As shown in Table 3 and Figure 4, the growth curves of L. lactis exhibited lag, exponential, and stationary phases at different temperatures. It can be seen that lag time (λ) and specific growth rate (μmax) of L. lactis were affected by temperature. As temperature increases, the lag time of L. lactis decreased, while the growth rates increased. The maximum population of L. lactis on FVFB could reach 19.11 ± 0.10 ln CFU/g. For the Huang, Baranyi, and reparameterized Gompertz models, the values of mean square error (MSE) ranged from 0.076 to 0.251, 0.219 to 0.321, and 0.023 to 0.161, respectively, and the root mean square error (RMSE) ranged from 0.276 to 0.501, 0.468 to 0.566, and 0.152 to 0.401, respectively. Compared with the Huang and Baranyi and Robert’s models, the reparameterized Gompertz model had the lowest MSE and RMSE values, indicating that the model was more suitable for describing the growth of L. lactis than the Huang and Baranyi and Robert’s models. Wang et al. (2017) demonstrated that the Gompertz model was suitable to estimate the shelf-life of the mushroom A. bisporus. Therefore, the Gompertz model was suitable for describing the microbial growth on mushroom.
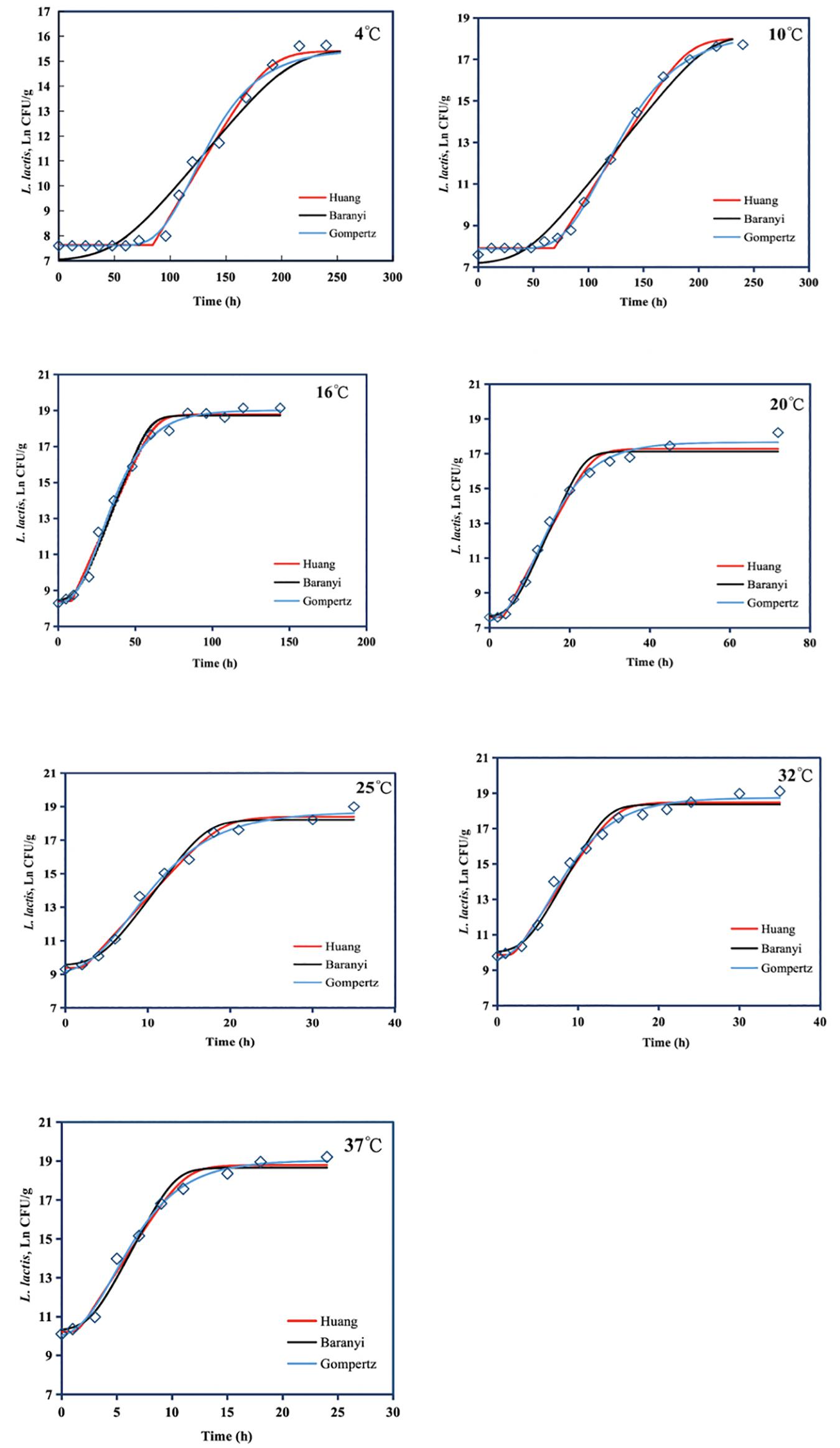
Figure 4. Growth of Lactococcus lactis in Flammulina velutipes fruiting bodies at temperatures between 4 and 37°C. Solid red line: Huang model, solid black line: Baranyi and Roberts model, solid blue line: reparameterized Gompertz model, diamond: observed growth data.
The values of μmax and λ obtained from the reparameterized Gompertz model were used for secondary models’ development (Figure 5). Table 4 lists the estimated parameters of the Huang, Ratkowsky, and Arrhenius models. The minimum growth temperatures predicted by the Huang square-root model was −1.7°C. The minimum growth temperature estimated by the Ratkowsky square-root model was −7.2°C, which was close to the value (−12°C) reported by Zhou et al. (2014). However, the Arrhenius-type model did not predict minimum growth temperature. Additionally, the values of MSE and RMSE (0.004 and 0.065) for the Ratkowsky square-root model were much lower than those for the Huang square-root model and Arrhenius-type model. Therefore, the Ratkowsky-type model was more accurate to predict the effect of temperature on the specific growth rate of L. lactis on FVFB.
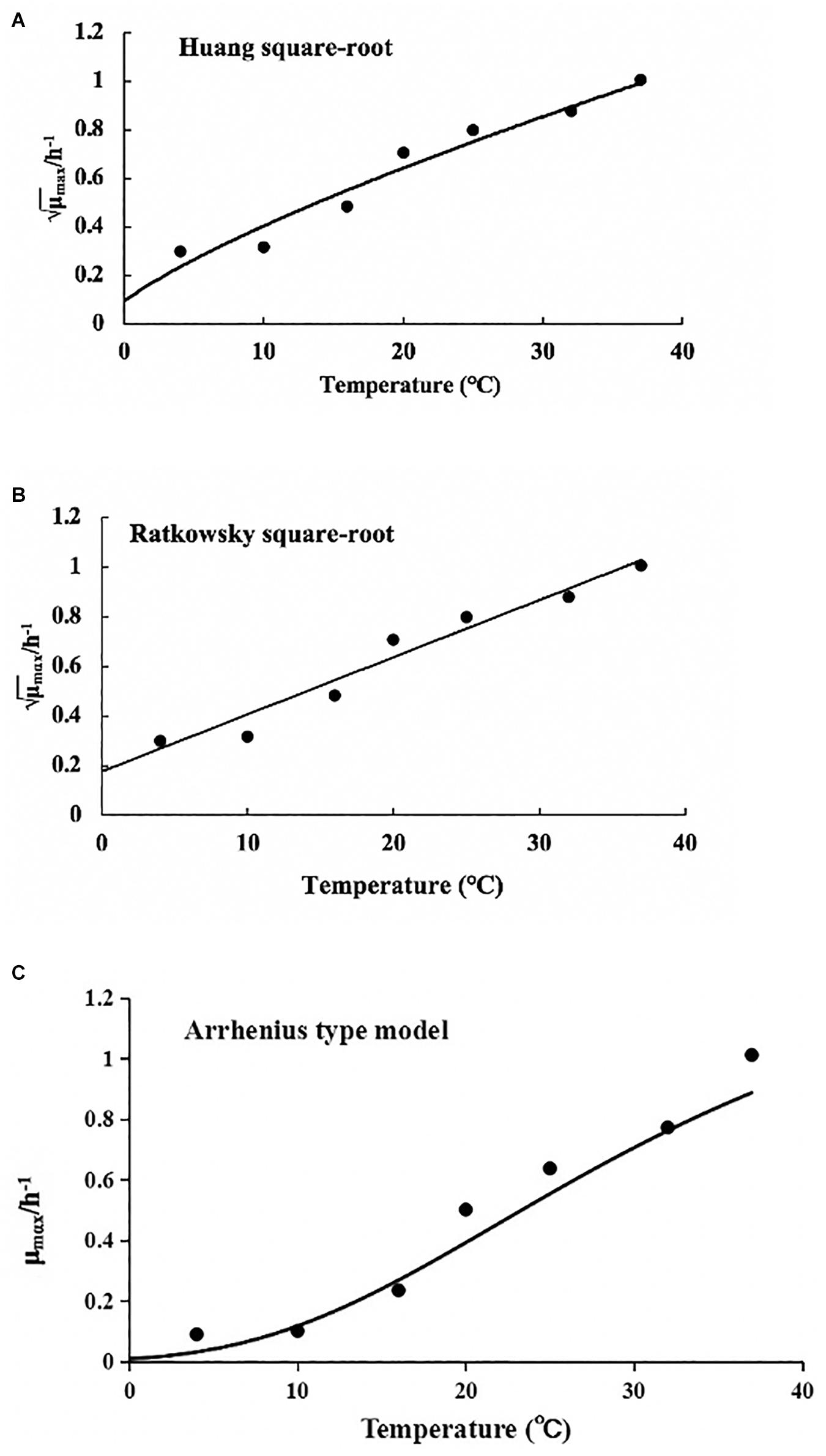
Figure 5. Effect of temperature on the specific growth rate of Lactococcus lactis in Flammulina velutipes fruiting bodies. (A) Huang square-root model, (B) Ratkowsky square-root model, and (C) Arrhenius-type model.
Conclusion
In this study, morphological examination and high-throughput sequencing were used to investigate the bacterial community in FVFB. Proteobacteria, Firmicutes, Bacteroidetes, Actinobacteria, Fusobacteria, and Tenericutes were the dominant phyla among all the FVFB samples collected from five different markets. L. lactis was the dominant microflora in FVFB. FVFB were most likely stored at a refrigerated temperature. However, it might be exposed to temperature abuse during storage. Due to their high moisture and delicate epidermal structure, FVFB were an ideal medium for bacteria growth, including L. lactis. The initial count of L. lactis in FVFB was estimated at around 9.73 ± 0.41 ln CFU/g. The maximum population of L. lactis was 19.11 ± 0.10 ln CFU/g in the stationary phase. This study proved that the Huang, Baranyi, and reparameterized Gompertz models could describe the growth of L. lactis in FVFB. The reparameterized Gompertz model was recommended as the primary model for L. lactis growth in FVFB, with the lowest MSE and RMSE values, ranging from 0.023 to 0.161, and 0.152 to 0.401, respectively. This study also explored and compared three different secondary models, namely the Ratkowsky square-root model, Huang square-root model, and Arrhenius-type model, and estimated the minimum, optimum, and maximum growth temperatures and the optimum growth rate of L. lactis in FVFB. Also, the Ratkowsky square-root model might provide a more accurate estimation of the specific growth rate of L. lactis in FVFB.
The effect of temperature on the growth kinetics of L. lactis in FVFB was investigated in this study. The primary models and secondary models could be used to predict the growth of L. lactis in FVFB during refrigerated storage and temperature abuse. The application of these models can also predict the shelf-life of spoilage microorganism shelf-life of FVFB and be used by regulatory agencies and food processors for conducting risk assessments of L. lactis in FVFB. The mathematical models could be used to describe the microbial growth under isothermal conditions in this study. However, temperature abuse during commercial production and distribution, isothermal studies were not suitable to investigate growth kinetics under fluctuating temperature conditions. Therefore, further studies are needed to develop dynamic models under fluctuating temperature conditions during storage.
Data Availability Statement
The datasets presented in this study can be found in online repositories. The names of the repository/repositories and accession number(s) can be found below: https://www.ncbi.nlm.nih.gov/sra/PRJNA705553.
Author Contributions
All authors listed have made substantial, direct and intellectual contribution to the work, and approved it for publication.
Funding
This work was supported by the Key Cultivating Program of Ningde Normal University (Grant Number 2020ZDK03), the Scientific Research Foundation of Ningde Normal University (Grant Number 2020Y010), and the Scientific Research Fund for Young Teachers in Fujian Province (Grant Number JAT200689).
Conflict of Interest
XP was employed by company Fujian Anjoy Foods Co., Ltd.
The remaining authors declare that the research was conducted in the absence of any commercial or financial relationships that could be construed as a potential conflict of interest.
Footnotes
References
Aguirre, M., and Collins, M. D. (1993). Lactic acid bacteria and human clinical infection. J. Appl. Bacteriol. 75, 95–107. doi: 10.1111/j.1365-2672.1993.tb02753.x
Akkermans, S., Logist, F., and Impe, J. F. V. (2018). Parameter estimations in predictive microbiology: statistically sound modelling of the microbial growth rate. Food Res. Int. 106, 1105–1113. doi: 10.1016/j.foodres.2017.11.083
Baheyeldin, M., and Gahan, C. G. M. (2010). Lactococcus lactis: from the dairy industry to antigen and therapeutic protein delivery. Discov. Med. 9, 455–461. doi: 10.3109/14653241003587637
Baranyi, J., and Roberts, T. A. (1995). Mathematics of predictive food microbiology. Int. J. Food Microbiol. 26, 199–218. doi: 10.1016/0168-1605(94)00121-L
Buchanan, R. L., Whiting, R. C., and Damert, W. C. (1997). When is simple good enough: a comparison of the Gompertz, Baranyi, and three-phase linear models for fitting bacterial growth curves. Food Microbiol. 14, 313–326. doi: 10.1006/fmic.1997.0125
Dave, R. I., and Shah, N. P. (1996). Evaluation of media for selective enumeration of Streptococcus thermophilus, Lactobacillus delbrueckii ssp. bulgaricus, Lactobacillus acidophilus, and Bifidobacteria. J. Dairy Sci. 79, 1529–1536. doi: 10.3168/jds.S0022-0302(96)76513-X
Fang, D. L., Yang, W. J., Kimatu, B. M., and Hu, Q. (2015). Effect of nanocomposite-based packaging on storage stability of mushrooms (Flammulina velutipes). Innov. Food Sci. Emerg. Technol. 33, 489–497. doi: 10.1016/j.ifset.2015.11.016
Food and Drug Administration (2012). History of the GRAS List and SCOGS Reviews. Silver Spring, MD: Food and Drug Administration.
Huang, L. H. (2013). Optimization of a new mathematical model for bacterial growth. Food Control. 32, 283–288. doi: 10.1016/j.foodcont.2012.11.019
Huang, L. H. (2014). IPMP 2013-A comprehensive data analysis tool for predictive microbiology. Int. J. Food Microbiol. 171, 100–107. doi: 10.1016/j.ijfoodmicro.2013.11.019
Huang, L., Hwang, A., and Phillips, J. (2011). Effect of temperature on microbial growth rate-Mathematical analysis: the arrhenius and eyring-polanyi connections. J. Food Sci. 76, 553–560. doi: 10.1111/j.1750-3841.2011.02377.x
Laurent, G. (2016). Predictive microbiology models and operational readiness. Proc. Food Sci. 7, 133–136. doi: 10.1016/j.profoo.2016.05.003
Lee, K. W., Shim, J. M., Park, S. K., Heo, H. J., Ham, K. S., and Kim, J. H. (2016). Isolation of lactic acid bacteria with probiotic potentials from kimchi, traditional Korean fermented vegetable. LWT Food Sci. Technol. 71, 130–137. doi: 10.1016/j.lwt.2016.03.029
Li, J., Wei, Q., Huang, L. X., Fang, T., Chen, B. Z., and Jiang, Y. J. (2020). Mathematical modeling Pseudomonas spp. growth and microflora composition variation in Agaricus bisporus fruiting bodies during chilled storage. Postharvest Biol. Technol. 163:111144. doi: 10.1016/j.postharvbio.2020.111144
Mannion, P. T., and Rothburn, M. M. (1990). Diagnosis of bacterial endocarditis caused by Streptococcus lactis and assisted by immunoblotting of serum antibodies. J. Infect. 21, 317–318. doi: 10.1016/0163-4453(90)94149-T
Niu, Y. X., Yun, J. M., Bi, Y., Wang, T., Zhang, Y., Liu, H., et al. (2020). Predicting the shelf life of postharvest Flammulina velutipes at various temperatures based on mushroom quality and specific spoilage organisms. Postharvest Biol. Technol. 176:111235. doi: 10.1016/j.postharvbio.2020.111235
Odamaki, T., Xiao, J. Z., Yonezawa, S., Yaeshima, T., and Iwatsuki, K. (2011). Improved viability of bifidobacteria in fermented milk by cocultivation with Lactococcus lactis subspecies lactis. J. Dairy Sci. 94, 1112–1121. doi: 10.3168/jds.2010-3286
Ratkowsky, D. A., Olley, J., McMeekin, T. A., and Ball, A. (1982). Relationship between temperature and growth rate of bacterial cultures. J. Bacteriol. 149, 1–5.
Rodrigues, M. X., Lima, S. F., Higgins, C. H., Canniatti-Brazaca, S. G., and Bicalho, R. C. (2016). The Lactococcus genus as a potential emerging mastitis pathogen group: a report on an outbreak investigation. J. Dairy Sci. 99, 9864–9874. doi: 10.3168/jds.2016-11143
Shi, C., Wu, Y. Y., Fang, D. L., Pei, F., Mariga, A. M., Yang, W. J., et al. (2018). Effect of nanocomposite packaging on postharvest senescence of Flammulina velutipes. Food Chem. 246, 414–421. doi: 10.1016/j.foodchem.2017.10.103
Song, A. A. L., In, L. L. A., Lim, S. H. E., and Rahim, R. A. (2017). A review on Lactococcus lactis: from food to factory. Microb. Cell Fact. 16, 1–15. doi: 10.1186/s12934-017-0669-x
Tashiro, T., and Yoshimura, F. (2019). A neo-logistic model for the growth of bacteria. Physica A 525, 199–215. doi: 10.1016/j.physa.2019.03.049
Tormo, H., Lekhal, D. A. H., and Roques, C. (2015). Phenotypic and genotypic characterization of lactic acid bacteria isolated from raw goat milk and effect of farming practices on the dominant species of lactic acid bacteria. Int. J. Food Microbiol. 210, 9–15. doi: 10.1016/j.ijfoodmicro.2015.02.002
Wang, J. M., Chen, J. R., Hu, Y. F., Hu, H. Y., Liu, G. H., and Yan, R. X. (2017). Application of a predictive growth model of Pseudomonas spp. for estimating shelf life of fresh Agaricus bisporus. J. Food Prot. 80, 1676–1681. doi: 10.4315/0362-028X.JFP-17-055
Wang, Y. X., and Zhang, H. (2021). Advances in the extraction, purification, structural-property relationships and bioactive molecular mechanism of Flammulina velutipes polysaccharides: a review. Int. J. Biol. Macromol. 167, 528–538. doi: 10.1016/j.ijbiomac.2020.11.208
Wessels, S., Axelsson, L., Hansen, E. B., Vuyst, L. D., Laulund, S., Hteenm, L. L., et al. (2004). The lactic acid bacteria, the food chain, and their regulation. Trends Food Sci. Technol. 15, 498–505. doi: 10.1016/j.tifs.2004.03.003
Whiting, R. C., and Buchanan, R. L. (1993). A classification of models for predictive microbiology. Food Technol. 19, 175–177.
Zhao, R. Q., Hu, Q. H., Ma, G. X., Su, A. X., Xie, M. H., Li, X. F., et al. (2019). Effects of Flammulina velutipes polysaccharide on immune response and intestinal microbiota in mice. J. Funct Foods 56, 255–264.
Zhao, Y., Li, P., Hu, H., Wang, Y., Sun, Y., and Huang, K. (2013). Postharvest decay of the cultivated mushroom Pleurotus eryngii caused by Lactococcus lactis subsp. lactis. J. Plant Pathol. 95, 247–253. doi: 10.1614/IPSM-D-12-00059.1
Zhao, Y. C., Zhu, R. J., Wang, Y., Song, Z. W., Shan, C. J., Qiu, J. P., et al. (2018). Complete genome sequence of Lactococcus lactis subsp. lactis SLPE1-3, a novel lactic acid bacterium causing postharvest decay of the mushroom Pleurotus eryngii. J. Plant Pathol. 100, 467–476. doi: 10.1007/s42161-018-0101-3
Zhou, K., Wei, J., Jiang, C. L., Han, X. F., and Liu, S. L. (2014). Growth dynamics of Lactococcus lactis SQ117 under different temperatures and pHs. Food Sci. 35, 192–195. doi: 10.7506/spkx1002-6630-201407038
Keywords: Flammulina velutipes, microflora, mathematical model, Lactococcus lactis, growth
Citation: Wei Q, Pan X, Li J, Jia Z, Fang T and Jiang Y (2021) Isolation and Molecular Identification of the Native Microflora on Flammulina velutipes Fruiting Bodies and Modeling the Growth of Dominant Microbiota (Lactococcus lactis). Front. Microbiol. 12:664874. doi: 10.3389/fmicb.2021.664874
Received: 06 February 2021; Accepted: 09 April 2021;
Published: 21 May 2021.
Edited by:
Anindya Chanda, Mycologics LLC, United StatesReviewed by:
Amit Kumar Singh, Albany Medical College, United StatesTheofania Tsironi, Agricultural University of Athens, Greece
Daniel Angelo Longhi, Federal University of Paraná, Brazil
Jun-Fang Lin, South China Agricultural University, China
Copyright © 2021 Wei, Pan, Li, Jia, Fang and Jiang. This is an open-access article distributed under the terms of the Creative Commons Attribution License (CC BY). The use, distribution or reproduction in other forums is permitted, provided the original author(s) and the copyright owner(s) are credited and that the original publication in this journal is cited, in accordance with accepted academic practice. No use, distribution or reproduction is permitted which does not comply with these terms.
*Correspondence: Zhen Jia, zhen-jia@uml.edu; Ting Fang, fangting930@163.com; Yuji Jiang, jyj1209@163.com