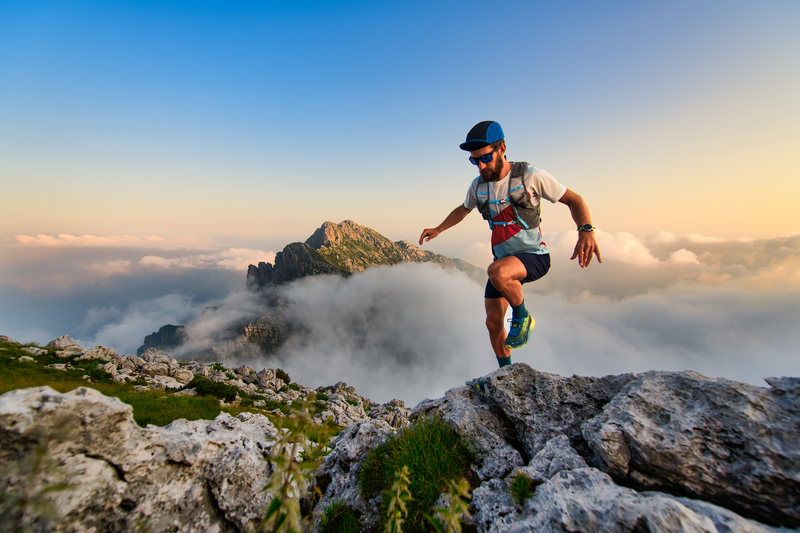
94% of researchers rate our articles as excellent or good
Learn more about the work of our research integrity team to safeguard the quality of each article we publish.
Find out more
ORIGINAL RESEARCH article
Front. Microbiol. , 15 April 2021
Sec. Microbe and Virus Interactions with Plants
Volume 12 - 2021 | https://doi.org/10.3389/fmicb.2021.660749
Intensive agriculture has major negative impacts on ecosystem diversity and functioning, including that of soils. The associated reduction of soil biodiversity and essential soil functions, such as nutrient cycling, can restrict plant growth and crop yield. By increasing plant diversity in agricultural systems, intercropping could be a promising way to foster soil microbial diversity and functioning. However, plant–microbe interactions and the extent to which they influence crop yield under field conditions are still poorly understood. In this study, we performed an extensive intercropping experiment using eight crop species and 40 different crop mixtures to investigate how crop diversity affects soil microbial diversity and activity, and whether these changes subsequently affect crop yield. Experiments were carried out in mesocosms under natural conditions in Switzerland and in Spain, two countries with drastically different soils and climate, and our crop communities included either one, two or four species. We sampled and sequenced soil microbial DNA to assess soil microbial diversity, and measured soil basal respiration as a proxy for soil activity. Results indicate that in Switzerland, increasing crop diversity led to shifts in soil microbial community composition, and in particular to an increase of several plant-growth promoting microbes, such as members of the bacterial phylum Actinobacteria. These shifts in community composition subsequently led to a 15 and 35% increase in crop yield in 2 and 4-species mixtures, respectively. This suggests that the positive effects of crop diversity on crop productivity can partially be explained by changes in soil microbial composition. However, the effects of crop diversity on soil microbes were relatively small compared to the effects of abiotic factors such as fertilization (three times larger) or soil moisture (three times larger). Furthermore, these processes were context-dependent: in Spain, where resources were limited, soil microbial communities did not respond to crop diversity, and their effect on crop yield was less strong. This research highlights the potential beneficial role of soil microbial communities in intercropping systems, while also reflecting on the relative importance of crop diversity compared to abiotic drivers of microbiomes and emphasizing the context-dependence of crop–microbe relationships.
The past century has seen the emergence and the development of modern, intensive agriculture that was accompanied by a strong increase in productivity per unit land area (Barrios, 2007). However, the negative effects associated with this intensification now call for a transformation toward more sustainable agricultural production (Tilman et al., 2011). Current intensive agriculture indeed has negative effects on biodiversity (Newbold et al., 2015) and ecosystem functioning (Barrios, 2007), including that of soils (Gardi et al., 2013). In particular, chemical inputs and the loss of crop diversity associated with intensive agriculture are known to negatively affect soil biodiversity (Cavicchioli et al., 2019), with consequences on soil ecosystem functioning, such as nutrient cycling, carbon storage, soil structure regulation, and pest and disease control (Wall and Bardgett, 2012; Wagg et al., 2014; Fanin et al., 2017). Losses of these essential soil functions can restrict plant growth and subsequently crop yield (Giller, 2001; Bardgett and Van Der Putten, 2014).
Numerous studies demonstrated a strong link between soil microbes and plants (Wardle et al., 2004; Fry et al., 2018). First, direct specific linkages between a plant species and a particular type of microbe, such as symbiotic interactions, might occur (Wubs and Bezemer, 2016). Moreover, plants have the ability to alter the soil chemical and physical conditions (López-Angulo et al., 2020). Some of these changes can be due to above-ground mechanisms, such as litter inputs or variations in microclimate associated with plant canopy cover (Maestre et al., 2009; Trinder et al., 2009; Delgado-Baquerizo et al., 2018). Others may be the result of below-ground processes, such as increases in soil loosening and aeration due to root growth, release of root exudates or plant signaling molecules, and changes in ion uptake (Doornbos et al., 2012; Eisenhauer et al., 2017). Therefore, a greater richness of plants, and subsequently, roots, would lead to a greater diversity of microhabitats, particularly in the rhizosphere (Philippot et al., 2013). The resulting microhabitat heterogeneity would then further enhance microbial diversity (Wardle, 2006; Eisenhauer et al., 2010), which could also increase soil functioning, following the diversity-ecosystem functioning relationship (Tilman et al., 2006). For instance, Steinauer et al. (2015) showed that increasing plant diversity led to a significant increase in soil microbial biomass and enzymatic activity. Similarly, results from a grassland biodiversity experiment demonstrated that higher plant diversity resulted in increased microbial activity and carbon storage (Lange et al., 2015). Most of these studies were performed in natural grasslands; however, the extent to which the same effects would arise in diversified cropping systems is still unclear. Indeed, intercropping, which is defined as growing at least two crops at the same time on the same field, could be a promising way to enhance soil microbial diversity and functioning by increasing plant diversity in arable systems. This increase in soil diversity and functioning could then feedback on crop yield through enhanced microbial activity and nutrient mobilization or decreased pathogen accumulation (Zhao et al., 2011; Bender and van der Heijden, 2015; Wang et al., 2017).
However, despite current technological advances to study soil microbes, soil microbial communities and their assembly mechanisms are still poorly understood (Van der Putten et al., 2013; López-Angulo et al., 2020). In particular, we are still unsure what soil microbes respond to—e.g., plant aboveground traits, plant root traits, plant community composition, plant functional diversity—and if these responses to the biotic environment are comparable to the effects of abiotic conditions, such as soil moisture, or nutrients. Consequently, the extent to which plant–microbe interactions in soils could influence crop yield of intercropping systems is still unclear. To shed light on this subject, we conducted an extensive common garden experiment including eight annual crop species belonging to four functional groups, and 40 different crop mixtures of two and four species, in which we determined soil microbial composition and measured soil respiration—as a proxy for soil functioning—in addition to crop yield. Moreover, we repeated the mesocosm experiment in two different countries—i.e., in Spain and Switzerland, which differ drastically in terms of climate and soil, and with and without fertilizer application in a fully factorial design. This experimental setup allowed us to examine the following research questions: (1) How does crop diversity affect soil microbial diversity and respiration in comparison to abiotic properties? (2) Are changes in soil microbial diversity and respiration related to crop yield? (3) Are crop–soil microbe relationships and their effects on crop yield environmentally context-dependent? We hypothesized that increasing crop diversity would lead to an increase in microbial (alpha) diversity as well as changes in bacterial and fungal community compositions—e.g., increases in symbionts, decomposers, or disease suppressive microbes—and that these changes would have a positive effect on soil basal respiration and crop yield. Finally, we hypothesized that changes in crop–microbe relationships and their impacts on crop yield would be context-dependent, with varying size effects in Switzerland and in Spain, which differ in terms of resource availability and environmental harshness.
The crop diversity experiment took place in outdoor experimental gardens in Zurich, Switzerland, and in Torrejón el Rubio, Cáceres, Spain. In Zurich, the garden was located at the Irchel campus of the University of Zurich (47.3961 N, 8.5510 E, 508 m a.s.l.). In Torrejón el Rubio, the garden was situated at the Aprisco de Las Corchuelas research station (39.8133 N, 6.0003 W, 350 m a.s.l.). Zurich is characterized by a temperate climate, while the Spanish site is located in a dry, Mediterranean climate. The main climatic differences during the respective growing seasons (i.e., between April and August in Switzerland and between February and June in Spain) were precipitation (587 mm in Zurich, 229 mm in Cáceres) and daily average hours of sunshine (5.7 h in Zurich, 8.8 h in Cáceres). Temperatures during the growing season did not vary substantially between the two sites: averages of daily mean, minimum and maximum temperatures were 15.8, 10.9, and 21.1°C in Zurich versus 15.5, 9.7, and 21.4°C in Cáceres. All climatic data come from the respective national meteorological archives and are average values over the years 2000–2018.1
The experimental gardens were irrigated during the growing season with the aim of maintaining the above-mentioned differences in precipitation between the two sites while assuring survival of the crops during drought periods. In Spain, the automated irrigation system was configured for a dry threshold of soil moisture of 17% of field capacity, with a target of 25%. In Switzerland, the dry threshold was set at 50% of field capacity, with a target of 90% of field capacity. Whenever dry thresholds were reached (measured through PlantCare soil moisture sensors (PlantCare Ltd., Switzerland), irrigation was started and water added until reaching the target value.
Each experimental garden consisted of square plots of 0.25 m2 with a depth of 40 cm. Beneath 40 cm the plots were open, allowing unlimited root growth. The plots were embedded into larger beds: in Switzerland, there were 14 beds of 14 m × 1 m, each bed containing 28 plots. In Spain, beds were 20 m × 1 m and contained 40 plots. Inside a bed, plots were separated from each other by metal frames. Each plot was filled until 30 cm with standard, not enriched, agricultural soil coming from the local region. Therefore, soil structure and composition varied between sites and reflected the environmental histories of each site. In Spain, soil was composed of 78% sand, 20% silt, and 2% clay, and contained 0.05% nitrogen, 0.5% carbon, 253 mg total P/kg. In Switzerland, the soil consisted of 45% sand, 45% silt, and 10% clay, and contained 0.19% nitrogen, 3.39% carbon, and 332 mg total P/kg. Spanish and Swiss soils had a mean pH of 6.30 and 7.25, respectively (Stefan et al., 2021).
We fertilized half of the beds with nitrogen, phosphorus, and potassium at the concentration of 120 kg/ha N, 205 kg/ha P, and 120 kg/ha K. Fertilizers were applied three times, namely once just before sowing (50 kg/ha N, 85 kg/ha P, 50 kg/ha K), once when wheat was at the tillering stage (50 kg/ha N, 85 kg/ha P, 50 kg/ha K), and once when wheat was flowering (20 kg/ha N, 34 kg/ha P, 20 kg/ha K). The other half of the beds served as unfertilized controls. We randomly allocated individual beds to a fertilized or non-fertilized control treatment (Supplementary Figure 1).
At each site, experimental communities were constructed with eight annual crop species. We used crop species belonging to four different phylogenetic groups with varying functional characteristics: Triticum aestivum (wheat, C3 grass) and Avena sativa (oat, C3 grass), Lens culinaris (lentil, legume) and Lupinus angustifolius (lupin, legume), Linum usitatissimum [flax, herb (superrosids)] and Camelina sativa [false flax, herb (superrosids)], and Chenopodium quinoa [quinoa, herb (superasterids)] and Coriandrum sativum [coriander, herb (superasterids)]. The crop cultivars that we chose were commercially available in Switzerland (the list of cultivars and suppliers can be found in Supplementary Table 1).
Experimental communities consisted of control plots with no crops, monocultures, 2- and 4-species mixtures. We planted every possible combination of 2-species mixtures with two species from different phylogenetic groups and every possible 4-species mixture with a species from each of the four different phylogenetic groups present. We replicated the experiment two times with the exact same species composition in each country. Within each country, we replicated the complete setup with and without fertilizer; plots were randomized within each fertilizer treatment, with each plot receiving the same amount of fertilization in the fertilized treatment. Each monoculture and mixture community consisted of one, two or four species planted in four rows. Each row only consisted of a single species. Two species mixtures were organized following a speciesA| speciesB| speciesA| speciesB pattern, where the described pattern refers to the row in which they were planted. The order of the species was chosen randomly. For 4-species mixtures, i.e., A| B| C| D, the order of the rows was also randomized. Density of sowing differed among species groups and was based on current cultivation practices: 160 seeds/m2 for legumes, 240 seeds/m2 for superasterids, 400 seeds/m2 for cereals, and 592 seeds/m2 for superrosids. Seeds were sown by hand in early February 2018 in Spain and early April 2018 in Switzerland. The plots were lightly tilled before sowing (at a depth of 5 cm). To sow, we dug four lines in the plots at 1–2 cm depth for all the species expect for the legumes, which were sown at 5 cm depth and for Camelina, which was sown at 0.5 cm. We then spread out the seeds in these lines and gently covered the surface. Germination and seedling establishment was controlled after two weeks; if germination was lower than 50% of the original seeds, additional seeds were resown to ensure that the plots would reach a sufficient plant density to allow plant interactions.
Soil samples were collected in each plot during flowering of the crops (early May in Spain, early June in Switzerland). We took three samples per plot to a depth of 25 cm, one between each of the four plant rows of a plot, which we then pooled. These soil samples were transported cooled to the lab and sieved with a 2 mm mesh size.
With the sieved soil samples, we then measured water content, water holding capacity, and incubated 25 g equivalent dry soil at a normalized moisture level (60% WHC) for 12 h in airproof jars. The CO2 content in the headspace was measured with a LiCor LI820 once at the beginning of the incubation and once at the end. The difference between the two measures corresponded to the amount of CO2 that had been produced by soil microbial respiration (Curiel Yuste et al., 2007).
Total nucleic acids were extracted from 250 mg of sieved soil using the DNeasy PowerSoil Kits (Qiagen, Hilden, Germany) following the supplier’s protocol. Concentration of extracted DNA was measured photospectrometrically with the QIAxpert System (Qiagen). Amplicon sequencing library construction was performed following a two-step PCR approach. The first step was performed using specific primers targeting the bacterial and archeal 16S ribosomal RNA gene (region V3–V4) and the fungal internal transcribed spacer region ITS2 using primer pairs 341F and 806R (Frey et al., 2016) and ITS3ngs and ITS4ngsUni (Tedersoo and Lindahl, 2016), respectively, including the sequencing primer sites of the Illumina adapters P5 (CTTTCCCTACACGACGCTCTTCCGATCT) and P7 (GGAGTTCAGACGTGTGCTCTTCCGATCT) required for the second step index PCR (Illumina Inc., San Diego, CA, United States). PCR amplification was performed in a volume of 25 μl containing 20 ng of template DNA, 1x GoTaq® Flexi Buffer (Promega, Madison, WI, United States), 2.5 mM MgCl2 (Promega), 0.4 μM of each primer (Microsynth, Balgach, Switzerland), 0.2 mM dNTPs (Promega), 0.6 mg/ml BSA (VWR, Radnor, PA, United States) and 1.25 U GoTaq® G2 Hot Start Polymerase (Promega). The PCR conditions consisted of an initial denaturation at 95°C for 5 min, 28 (16S rRNA gene) or 35 (ITS2 rrn) cycles of denaturation at 95°C for 40 s, annealing at 58°C for 40 s and elongation at 72°C for 1 min, followed by a final elongation at 72°C for 10 min. Each sample was amplified in triplicates and subsequently pooled. Pooled DNA samples were sent to the Functional Genomics Center Zurich (FGCZ, Zurich, Switzerland) for the indexing PCR. Index PCR products were purified, quantified, and pooled in equimolar ratios prior to sequencing on the Illumina MiSeq platform using the v3 chemistry (Illumina Inc.).
At the same time as the collection of soil samples, volumetric soil water content was measured from the soil surface to a depth of 6 cm using a ML3 ThetaProbe Soil Moisture Sensor (Delta-T, Cambridge). The measurements were taken in the center of each of the three in-between rows per plot and averaged per plot.
Grain yield of each crop species was determined in each plot when grains reached maturity (duration of crop growth from sowing to harvest given in Table 1). As time of maturity slightly varied among the different crops, we harvested species by species.
Table 1. Sowing and harvesting dates, and crop growth duration in mean days after sowing from sowing until harvest for both countries and all eight species.
Due to wild birds foraging on wheat seeds in Spain, and consequently, loss of data on wheat yield, we discarded the plots that were affected by the birds foraging. A total of 341 out of 384 plots remained, 181 in Switzerland and 160 in Spain.
Sequences were processed using a customized pipeline largely based on VSEARCH. The main steps included paired-end read merging using the fastq_mergepairs algorithm implemented in VSEARCH (Edgar and Flyvbjerg, 2015); primer trimming using Cutadapt (Martin, 2011) allowing for one mismatch; removing PhiX control reads using Bowtie2 (Langmead and Salzberg, 2012); quality filtering by maximum expected error of one using the fastq_filter function (Edgar and Flyvbjerg, 2015) implemented in VSEARCH; dereplicating sequences using the derep_fulllength function in VSEARCH; delineation of sequences into amplicon sequence variants (ASVs) using the unoise3 algorithm (Edgar, 2016c) implemented as the cluster_unoise function in VSEARCH with an alpha of 2 and minsize of 4; removal of potentially chimeric sequences using the uchime2 algorithm (Edgar, 2016b) implemented as the uchime3_denovo function in VSEARCH; target verification using Metaxa2 (Bengtsson-Palme et al., 2015) and ITSx (Bengtsson-Palme et al., 2013) for the 16S rRNA gene and ITS2 sequences, respectively; mapping of the quality-filtered reads of each sample against the verified ASV sequences using the usearch_global function in VSEARCH; and taxonomic classification of each ASV sequence using the SINTAX classifier (Edgar, 2016a) implemented in VSEARCH with a bootstrap support of 0.8 against the SILVA database for the 16S rRNA sequences (Pruesse et al., 2007) and the UNITE database for the ITS2 sequences (Abarenkov et al., 2010). Non-fungal ITS2 sequences, as well as 16S rRNA sequences assigned to organelle structures (chloroplasts, mitochondria) were removed from the ASV table.
To remove effects of variability in sequencing read numbers, we performed 100-fold iterative subsampling of the ASV table using the function rrarefy from the vegan package in R (Oksanen et al., 2019; R Core Team, 2019), and subsequently computed the mean abundance for each ASV. Alpha diversity was assessed by calculating Shannon’s diversity (H) and Pielou’s evenness (J) indexes.
We used linear mixed models followed by type I analysis of variance to analyze the effects of the experimental treatment on fungal and bacterial ASV richness, H, and J, respectively. The analyses were performed for Spain and Switzerland separately. Fixed factors included fertilizing condition, crop species number (i.e., two or four crop species) nested in monoculture vs. mixtures, presence of cereal, presence of legume, presence of superrosid herb, presence of superasterid herb, as well as the interactions between them (except interactions between crop species number and monoculture vs. mixture and presence of functional group, respectively). Presences of the different functional groups were all binary factors (yes, no). Species composition and bed were set as random factors. Homogeneity of variance and normality of residues were assessed visually and with Shapiro-Wilk tests (Royston, 1982).
To analyze the responses of the microbial community composition to the experimental treatments, we first performed principal coordinates analysis (PCoA) using Bray-Curtis dissimilarity on relative ASV abundance (Gower, 1966). To that end, sparse ASVs (i.e., ASVs that appeared in one or two plots only) were removed, and relative abundance was log-transformed. We first performed this analysis taking both countries together, and in a second time, per country separately. Then, we used permutational multivariate analyses of variance (PERMANOVA; Anderson, 2001) with Bray-Curtis dissimilarity on the previously described composition matrices (i.e., separated per country), using the function adonis from the vegan package with 999 permutations (Oksanen et al., 2019). Experimental factors tested included fertilization, crop diversity treatments (monocultures vs. mixtures, crop species number, presence of the different functional groups and/or species), and their interactions. Homogeneity of variance was tested using permutational analysis of multivariate dispersion (PERMDISP; Anderson, 2006) implemented as the betadisper function in vegan with 999 permutations. Finally, we conducted a canonical analysis of principal coordinates (CAP; Anderson and Willis, 2003) for each country using the function CAPdiscrim from the BiodiversityR package (Kindt and Coe, 2005).
We analyzed species-specific and phylum-specific responses to the experimental factors with indicator species analyses using the indicspecies package (Cáceres and Legendre, 2009), calculating the point-biserial correlation coefficient, and correcting for multiple testing using the Benjamini-Hochberg method with the p.adjust function from the stats package (Benjamini and Hochberg, 1995).
We calculated total crop yield per plot (measured as the sum of grain mass of each species per plot), and the net biodiversity effect (NE) following the method from Hector and Loreau as the deviation from total expected yield in the mixture (Loreau and Hector, 2001). We then used the same linear mixed models as described above to analyse the response of total crop yield and NE to the experimental treatment variables, in Spain and in Switzerland, respectively.
Considering the possibilities of direct and indirect effects of the different environmental and experimental variables (fertilizer, crop species richness) on soil moisture, soil microbes, soil respiration, and crop yield (measured as total grain mass per plot), we then applied structural equation modeling (SEM) to our data sets, separately for Switzerland and Spain. Because the effects of crop species number and crop community composition were correlated (for instance the 4-species mixtures automatically had one cereal, one legume, and two herbs) and thus difficult to disentangle in our study, we decided for simplicity of the SEM to only keep crop species number as a variable for crop diversity. The SEMs were built, run and evaluated with the lavaan package (Rosseel, 2012). We decided to fit the SEM to the Swiss and Spanish datasets separately because microbial composition in the two countries showed large differences. SEM allows to test complex a priori defined direct and indirect relationships in a unique framework and to assess the overall fit of the data to the model (Grace, 2006). For our a priori model, we considered environmental variables (fertilizer), crop species richness, soil moisture level in terms of volumetric water content, soil microbial alpha diversity measures (bacterial and fungal Shannon indexes), soil microbial beta diversity measures (coordinates from the three first axes of the principal coordinates analyses for fungi and bacteria, respectively), soil activity measured as CO2 flux, and total crop yield per plot. Our a priori model relating environmental and experimental factors, soil moisture, soil microbial diversity, soil activity and crop yield included the following hypotheses: (1) soil microbial diversity components (i.e., alpha diversity and community composition axes) are directly influenced by the environmental conditions (fertilizer), soil moisture, and the experimental treatment (crop species richness). (2) Soil activity can be affected directly or indirectly by crop species richness, soil moisture and fertilizer; the relationship can be indirect if effects on soil activity are mediated by effects on soil diversity measures. (3) Crop yield can also be directly or indirectly affected by crop species richness, soil moisture and fertilizer. The relationship can be indirect if effects on crop yield are mediated through changes in soil microbial diversity measures or soil activity. (4) All the soil microbial diversity measures can covary with each other.
To overcome scale differences among variables, we log-transformed crop yield before inclusion in the SEM. Path coefficients were estimated using maximum likelihood, and the model fits were tested with a chisquare goodness of fit test, a Bollen–Stine bootstrap test with 1000 bootstrap draws, a root mean square error of approximation (RMSEA) test, and the comparative fit index (CFI). A non-significant chisquare, Bollen–Stine and RMSEA test, as well as CFI values above 0.90, indicate a good fit of the model to the data (Kline, 2011).
Finally, we calculated the weighted average score of each ASV based on the previously mentioned PCoA decompositions, and subsequently investigated with linear models which bacterial and fungal genera were non-randomly distributed along the PCoA axes.
After sequencing, the fungal dataset contained 9,713,470 reads delineated into 7,856 ASVs. After removing all non-fungal ASVs, we obtained 6,161,288 reads assigned to 3,546 ASVs. The vast majority of the removed ASVs were assigned to plants despite having sieved the soils with 2 mm mesh size, which suggests low fungal biomass in our system. The bacterial dataset contained 6,394,402 reads delineated into 4,4136 ASVs. After removing all sequences associated with chloroplasts and mitochondria, we obtained 6,306,145 reads assigned to 43,857 ASVs.
All diversity metrics were consistently lower in Switzerland compared to Spain (Figure 1): mean fungal ASV richness was 608 in Spain, against 324 in Switzerland (-47%), whereas fungal H was lower by 22% and fungal J by 13%. Similarly, mean bacterial ASV richness was lower by 30% (i.e., 803 vs. 561 ASVs in Spain vs. Switzerland); bacterial H was 16% lower and bacterial J 11% lower in Switzerland compared to Spain.
Figure 1. Effects of fertilizer on fungal (A) and bacterial (B) Shannon’s diversity index (H), and of crop species number on fungal (C), and bacterial (D) ASV richness in Spain and Switzerland. Horizontal lines represent the median of the data, boxes represent the lower and upper quartiles (25 and 75%), with vertical lines extending from the hinge of the box to the smallest and largest values, no further than 1.5× the interquartile range. Data beyond the end of the whiskers are outlying and plotted individually. See Tables S2 and S3 for the results of the statistical analyses.
In Spain, bacterial H was marginally lower (2%) in fertilized compared to unfertilized plots (Supplementary Table 2 and Figure 1B). Furthermore, the presence of a superasterid herb marginally increased bacterial ASV richness (+2.5%) and decreased bacterial J (−1%).
In Switzerland, fertilization decreased bacterial ASV richness (−16% in fertilized compared to unfertilized plots) but increased bacterial evenness (+4%) (Supplementary Table 3). Fungal ASV richness marginally increased with crop species number (Figure 1C; +7% in 4-species mixtures compared to monocultures and +4% compared to 2-species mixtures), while bacterial ASV richness was higher in 2-species mixtures compared to 4-species mixtures (Figure 1D). Finally, the presence of a legume marginally decreased fungal H (−1%) and J (−1%) in comparison to plots without legumes, while cereal presence marginally decreased bacterial J (−2%).
Fungal and bacterial community composition differed strongly between countries (Supplementary Figure 2), with the country accounting for 66 and 52% of the variance in fungal and bacterial communities, respectively (Supplementary Table 4). All other factors explained less than 1% of the remaining variance. In Spain, fungal communities were characterized by higher relative abundance of Ascomycota, Mortierellomycota, and Mucoromycota, while the fungal communities in the Swiss soils had relatively more Basidiomycota, Chytridiomycota, and Rozellomycota (Supplementary Figures 3A, 4A). Notable changes in bacterial communities between the two countries include higher relative abundance of Planctomycetes, Actinobacteria, Acidobacteria, and Nitrospirae in Spain, while in Switzerland bacterial communities showed more Proteobacteria, Chloroflexi, Bacteroidetes, and Cyanobacteria (Supplementary Figures 3B, 4B).
In Spain, the community composition of both fungi and bacteria was significantly affected by fertilization, presence of cereal, as well as their interaction (Table 2 and Supplementary Figures 5, 6). The interaction between fertilizer and the presence of legume also had an effect on fungal communities in Spain. Fertilized plots had fungal communities with relatively more Chytridiomycota, while Mortierellmycota, Mucoromycota, and Kickxellomycota were positively linked to unfertilized plots (Supplementary Figure 7A). For bacterial communities, we observed a relative increase of Proteobacteria, Actinobacteria, Nitrospirae, Firmicutes, and Patescibacteria in fertilized plots, while Planctomycetes, Acidobacteria, and Chloroflexi were more abundant in unfertilized plots. Deinococcus-Thermus and Fibrobacteres increased in relative abundance in the presence of cereals, while Nitrospirae was associated with the absence of cereals (Supplementary Figure 9A).
Table 2. Results of the permutational analysis of variance, showing R2 and significance of the considered factors for the community composition of fungi and bacteria in Spain and Switzerland.
In Switzerland, fungal and bacterial communities were affected by fertilization, crop species number, presence of cereal and presence of legume (Table 2 and Supplementary Figures 5, 6). Fungal communities were also affected by the presence of superasterid herbs. The interaction of fertilization and presence of legume had an effect on bacterial communities. Mucoromycota were more abundant in fertilized plots, while Ascomycota were more present in unfertilized plots (Supplementary Figure 7B). For bacterial communities, we noticed an increase in Proteobacteria, Patescibacteria, Hydrogenedentes, Bacteroidetes, and Cyanobacteria in fertilized plots, while Planctomycetes, Actinobacteria, and Firmicutes were more abundant in unfertilized plots. Furthermore, there was an increase in the relative abundance of Fibrobacteres, Verrucomicrobia, and Armatimonadetes in the presence of cereals, while plots with no cereal had more Hydrogenedentes and Latescibacteria (Supplementary Figure 9B).
Total crop yield had an average of 416 g/m2 in Spain and 784 g/m2 in Switzerland. In Spain, total crop yield was only increased by the presence of a legume (+72%) (Figure 2A and Supplementary Table 5). In Switzerland, total crop yield significant increased with crop species number (Figure 2B and Supplementary Table 6): two-species and four-species mixtures showed an increase in average yield of 43 and 102% compared to monocultures, while 4-species mixture showed an increase in average yield of 41% compared to 2-species mixtures. The presence of a cereal and a superasterid herb increased total yield in Switzerland (+102%, +96%), while the presence of a legume decreased total yield (-20%) (Figure 2A).
Figure 2. Effects of legume presence and crop species number on total crop yield (A,B), and effects of crop species number, presence of cereal and legume on Net biodiversity Effects (C–E) in Spain and Switzerland. Horizontal lines represent the median of the data, boxes represent the lower and upper quartiles (25 and 75%), with vertical lines extending from the hinge of the box to the smallest and largest values, no further than 1.5 × the interquartile range. Data beyond the end of the whiskers are outlying and plotted individually. See Tables S5, S6, S7 and S8 for the results of the statistical analyses. *p < 0.05, **p < 0.01, and ***p < 0.001.
Net biodiversity effect was positive in both countries, which means that the mixtures consistently overyielded. However, NE was much higher in Switzerland compared to Spain (+680%) (Figure 2C). In Spain, NE was significantly reduced by the presence of a cereal (−108%) and increased in the presence of a legume (+219%) (Figures 2D,E and Supplementary Table 7). In Switzerland, NE was significantly higher in 4-species mixtures compared to 2-species mixtures (+150%) (Figure 2C and Supplementary Table 8).
Our data showed overall good fit to our a priori SEM in Spain and Switzerland: chisquare = 3.828 (Spain) and 4.939 (Switzerland); P(chisquare) = 0.281 (Spain) and 0.173 (Switzerland); P(Bollen–Stine Bootstrap) = 0.459 (Spain) and 0.464 (Switzerland); RMSEA = 0.046 (Spain) and 0.064 (Switzerland); P(RMSEA) = 0.415 (Spain) and 0.321 (Switzerland); CFI = 0.999 (Spain) and 0.997 (Switzerland).
In Spain, soil diversity measures were mostly influenced by fertilizer and soil moisture (Figure 3A). Soil microbial activity was directly influenced by soil moisture, fungal PCoA 2 and bacterial PCoA 2. Crop yield was only linked to bacterial PCoA axis 2. Bacterial PCoA axis 2 was associated with a response of Bacilli, Actinobacteria and Acidobacteria, among others (Supplementary Figure 11), which therefore correlated with an increase in crop yield in our study. Fertilization had an indirect positive effect on crop yield via changes in bacterial PCoA axis 2.
Figure 3. Structural Equation Modeling showing the relationships between crop diversity, fertilizer, soil moisture, soil activity, and bacterial and fungal diversity measures in Spain (A) and Switzerland (B). H: Shannon’s diversity. Only significant (solid line) and marginally significant (dashed line) relationships are shown. Width of arrows are proportional to the strength of the standardized path coefficients indicated by the numbers above the arrows. The numbers in brackets indicate associated p-values. Colors of the arrows show positive (green) and negative (gray) effects. Residual correlations are not shown.
In Switzerland, fertilizer and soil moisture also significantly influenced almost all the soil diversity measures (Figure 3B). Moreover, crop diversity had a direct effect on soil moisture, bacterial and fungal H, fungal PCoA axis 2, and bacterial PCoA axis 2. Soil microbial activity was negatively affected by bacterial H, and positively by the first and second axes of bacterial PCoA. Crop yield was directly affected by some soil diversity measures (fungal PCoA axes 1 and 2, bacterial PCoA axes 1 and 2), and also by crop diversity, fertilizer and soil moisture.
Crop diversity indirectly increased soil activity in Switzerland through changes in bacterial H and the second axis of bacterial PCoA.
Crop diversity indirectly affected crop yield in Switzerland through five different pathways. Firstly, there were two indirect positive effects through the second axis of fungal PCoA (Figure 4); fungi genera associated with negative coordinates along the PCoA axis 2 included for instance Kondoa, Serendipita, Mucor, or Leucospiridium, among others (Supplementary Figure 10). The presence of these fungal genera is therefore linked to an increase in crop yield. Secondly, there were two positive effects of crop diversity on crop yield mediated by the second axis of bacterial PCoA (Figure 5). Bacterial PCoA axis 2 is related to a response of Nitriliruptoria, Chloroflexia, Acidimicrobia, and Actinobacteria, among others (Supplementary Figure 12B); these bacteria thus form another potential group of yield promoting soil microbes in our study (Figures 5, 6). Finally, crop diversity indirectly increased crop yield through the first axis of bacterial PCoA. Bacterial genera associated with negative coordinates along the PCoA axis 1 include Nitriliruptoria, Actinobacteria, Acidimicrobia, Chloroflexia, and Bacilli, among others (Supplementary Figure 12A), i.e., a third group of yield promoting soil microbes in our study (Figure 6). Fertilization had an indirect positive effect on crop yield, via changes in fungal PCoA axes 2 and 3, but also an indirect negative effect on crop yield, mediated by changes in bacterial PCoA axis 1.
Figure 4. Effects of crop species number on the coordinates of the second axis of fungal PCoA decomposition (A) and correlation between these coordinates and total crop yield (B) in Switzerland. In panel (B), the line represents the linear regression (coefficient: −288, p-value = 0.00037). *** indicates the significance level of the effects of crop species number on fungal PCoA 2 (p-value < 0.001).
Figure 5. Effects of crop species number on the coordinates of the second axis of bacterial PCoA decomposition (A) and correlation between these coordinates and total crop yield (B) in Switzerland. In panel (B), the line represents the linear regression (coefficient: 779, p-value < 0.001). **indicates the significance level of the effects of crop species number on bacterial PCoA 2 (p-value < 0.01).
Figure 6. Effects of crop species number on the proportion of Actinobacteria (A) and correlation between the proportion of Actinobacteria and total crop yield (B) in Switzerland. In panel (B), the line represents the linear regression (coefficient: 26.8, p-value < 0.001). **indicates the significance level of the effects of crop species number on bacterial PCoA 2 (p-value < 0.01).
Our study shows that fertilization, as well as—in some cases—crop diversity and composition, can affect soil microbial community composition, and that these compositional changes can go along with changes in crop yield. In particular, the data suggests that in Switzerland, the positive effects of increasing crop diversity on crop yield are partially mediated by changes in microbial composition, notably through a response of plant-growth promoting bacteria. However, as expected, changes in soil microbes do not fully explain yield variations and thus, other processes must play an important role in increasing crop yield in mixtures. Furthermore, the effects of crop diversity on microbial communities remained small compared to the effects of fertilization. In Spain, where soil resources were limited and both crop and soil communities experienced more stressful environmental conditions than in Switzerland, we did not observe any significant responses of soil microbes to crop diversity. We suggest that this context-dependency of crop diversity effects on soil microbes and ecosystem functioning might be explained by differences in abiotic factors; however, further research in various environmental conditions is needed to better understand the reasons behind this context-dependency.
Our first hypothesis was that an increase in crop diversity would lead to an increase in microbial alpha diversity as well as changes in soil microbial community composition. We did observe an increase in fungal ASV richness in response to crop diversity, but this effect was only marginally present in Switzerland. This is consistent with results from studies in natural environments, where plant species richness positively correlated with fungal richness (Yang et al., 2017). There was no effect of crop diversity on any of the bacterial alpha diversity measures; however, in both countries, we observed changes in bacterial and fungal beta diversity in response to crop diversity and crop composition (Table 2). In Switzerland notably, we observed a shift in soil fungal and bacterial composition between 2-species mixtures and 4-species mixtures. This is in agreement with previous research demonstrating that plant diversity did not correlate with microbial alpha diversity, but it did with beta diversity (Grüter et al., 2006; Prober et al., 2015). Moreover, crop composition also affected microbial communities: for instance, the presence of a cereal induced changes in fungal and bacterial soil communities in both countries, with a notable increase in abundance of Fibrobacteres, Armatimonadetes, and Verrucomicrobia. The two latter groups have commonly been found in the rhizosphere of wheat or oat, even though little is known about their functions (Rascovan et al., 2016; Dai et al., 2020; Lupwayi et al., 2020). The response of Fibrobacteres—which are mostly involved in the degradation of plant-based cellulose (Gupta, 2004)—to the presence of a cereal has been scarcely mentioned in previous research (Mahoney et al., 2017). These changes in microbial composition may be due to above-ground processes linked to crop diversity and composition, such as variations in microclimate associated with plant canopy cover (Delgado-Baquerizo et al., 2018), or below-ground processes, such as increased diversity of root exudates or plant signaling molecules (Eisenhauer et al., 2017); however, more research is needed to investigate the mechanisms behind these specific responses.
Secondly, we hypothesized that crop diversity would enhance soil activity; this was indeed the case in Switzerland, where crop diversity increased soil respiration via changes in bacterial richness and composition (Figure 3B). This is consistent with grassland studies demonstrating a positive link between plant diversity and soil microbial activity (Lange et al., 2015; Steinauer et al., 2015), possibly due to increases in root inputs, changes in root exudates (Bais et al., 2006), or a reduction in evapotranspiration from the topsoil due to denser vegetation in diverse plant communities (Lange et al., 2014).
Results from our SEM in Switzerland suggest that the changes in soil microbial communities induced by crop diversity further affected crop yield (Figure 3B). We had previously shown that there was a positive effect of crop species richness on crop yield in Switzerland, demonstrating a positive diversity–productivity relationship (Figure 2B; Chen et al., 2020; Engbersen et al., 2020). Here, we provide evidence that these positive effects of intercropping on crop yield can partially be explained by changes in microbial communities. Indeed, increasing crop diversity was linked to an increase in yield through five indirect pathways mediated by changes in fungal or bacterial composition (Figure 3B). We noticed that those changes in microbial composition associated with increases in crop yield were characterized by a response of potentially beneficial bacteria such as plant growth-promoting rhizobacteria (PGPRs). For instance, this was the case of Actinobacteria (Figure 6 and Supplementary Figure 11, 12), which are known to be symbionts of plants as well as saprophytes (Bhattacharyya and Jha, 2012). They also play a role as biocontrol agents against a range of pathogenic fungi and promote plant growth (Merzaeva and Shirokikh, 2006; Franco-Correa et al., 2010). Some examples include Glycomyces, Agromyces, or Nocardioides, which are genera of PGPRs (Qin et al., 2011; Hamedi and Mohammadipanah, 2014), and Streptomyces, known to associate with wheat and possessing anti-fungal properties (Conn and Franco, 2004). Other potential PGPRs that were found to positively correlate with crop yield in our study include Pseudomonas, Burkholderia, Bacillus, and Massilia (Supplementary Figure 11, 12). Pseudomonas has been shown to promote plant growth as well as inhibit pathogenic fungi (Gray and Smith, 2005; Melo et al., 2016). Burkholderia and Bacillus can be involved in phosphate solubilization (Dimkpa et al., 2009; Zhao et al., 2014) and symbiotic associations with wheat (Shaharoona et al., 2007; Moreno-Lora et al., 2019). Finally, Massilia has been shown to correlate with an increase in plant biomass for alfalfa and soybean (Xiao et al., 2017). Some bacteria involved in nitrogen cycling also positively correlated with the PCoA coordinates leading to an increase in crop yield in Switzerland, such as Nitrosospira—ammonia-oxidizing genus (Fierer et al., 2007), and Nitrolancea—nitrite-oxidizing genus (Daims et al., 2016).
When looking at fungi, our results showed that in Switzerland crop yield was positively associated with fungal genera including Claroideoglomus, Myrmecridium, Serendipita, Mucor, as well as several yeasts (e.g., Torula, Kondoa, Leucosporidium) (Supplementary Figure 10). Claroideoglomus belongs to the Glomerales order, which are biotrophic mutualists and can establish arbuscular mycorrhizal networks with wheat (Dai et al., 2014). Myrmecridium and Mucor are saprophytes, involved in decomposition of organic matter and nutrient cycling (Schlatter et al., 2018), while Serendipita are plant growth promoting fungi which have been shown to have beneficial effects on many plants, including wheat (Singhal et al., 2017). Finally, yeasts have also been suggested as potential bio-agents and plant growth promoters (Mukherjee et al., 2020); furthermore, they are a nutrient source for some bacteria and contribute to essential soil ecological processes such as mineralization of organic matter (Botha, 2011). Among the fungal genera associated with positive PCoA coordinates, i.e., lower crop yield, we found a few plant pathogens, such as Protomyces, causing stem gall disease in coriander (Khan and Parveen, 2018), and Pyrenochaeta, a parasite for plant roots (Aragona et al., 2014). Our study thus suggests that in Switzerland, increasing crop diversity leads to changes in microbial communities that enhance the presence of beneficial microbes and reduce pathogen loads, which may promote plant growth and contribute to the observed increase in crop yield.
While changes in soil microbial composition explained part of the positive effects of crop diversity on crop yield, there were also direct effects of intercropping on yield (i.e., not mediated by microbes) in Switzerland. This demonstrates that other mechanisms must play a role in increasing crop productivity in diverse mixtures. These processes could include a complementary use of resources, such as nutrient or light partitioning (Jesch et al., 2018; Engbersen et al., 2020), trait differentiation (Cadotte, 2017), or changes in crop–weed interactions (Stefan et al., 2020).
Moreover, our study highlights the context-dependency and relative importance of crop diversity effects on soil microbes. Indeed, our results suggest that abiotic factors such as fertilization or soil moisture were more important drivers of soil microbial communities than crop diversity. This is consistent with previous research indicating that soil microbial community composition mainly depends on soil moisture, temperature, and organic matter contents, and that these factors are often more important than crop diversification (Ren et al., 2018; Guo et al., 2020). In our case, in Switzerland, the effects of soil moisture and fertilization on microbial composition were indeed up to three times stronger than the effect of crop diversity (Figure 2B). Interestingly, fertilization in Switzerland seemed to have conflicting indirect effects on yield: on the one hand, it promoted fungal communities that were beneficial for crop yield, such as Mucoromycota (Supplementary Figure 7B), but on the other hand, it also favored bacteria that were not positively associated with crop yield, such as Proteobacteria. Bacterial phyla responding to mineral fertilization included Proteobacteria, Hydrogenedentes, Bacteroidetes, and Cyanobacteria (Supplementary Figure 8B). This is consistent with previous studies demonstrating that the relative abundance of Proteobacteria and Bacteroidetes generally increase under high-N conditions (Fierer et al., 2012). Bacteroidetes and many proteobacterial groups have been identified as copiotrophic taxa, which tend to thrive in resource-rich environments (Fierer et al., 2007; Ramirez et al., 2010). On the contrary, Actinobacteria, which include many PGPRs, was significantly more abundant in unfertilized plots (Supplementary Figure 8B). Therefore, we might hypothesize that high-N conditions would favor the growth of Proteobacteria at the expense of Actinobacteria (Lupwayi et al., 2018), thereby limiting the abundance of plant beneficial bacteria.
In Spain, soil microbes responded to soil moisture and fertilization but did not respond to crop diversity. We propose several reasons for this lack of crop–microbe relationship in Spain, while acknowledging the speculative character of the following suggestions due to the limitations of having one unique site per country. First, soil texture in Spain was dominated by sand (78%) with only a small proportion of clay (2%), unlike Switzerland where sand and silt were in equal proportions (45%) with a higher clay content (10%). Higher clay and silt content usually protect and favor microbial biomass (Bach et al., 2010) through larger aggregates (Wick et al., 2009), greater water holding capacity (Voroney, 2007), and increased nutrient retention (Knops and Tilman, 2000). This is supported by the fact that microbial DNA concentration was indeed much lower in Spain (Supplementary Figure 13), which indicates that the soil microbial communities were poorer in Spain in terms of biomass, and consequently perhaps more resistant to change (Landesman and Dighton, 2010). Secondly, the climate in Spain is more arid than in Switzerland, which led to lower soil moisture and increased water stress for plant and soil communities. Also, soil nutrient content was lower in Spain. Spanish soil communities thus experienced greater abiotic stress than in Switzerland, which may be why they primarily responded to soil moisture or fertilization—factors that can directly alleviate their stress—rather than crop diversity. Furthermore, there was almost no link between microbial communities and crop yield in Spain; in particular, fungal communities did not have any effect on crop yield, while only one axis of the bacterial composition positively affected yield in response to fertilization (Figure 3A). This decoupling between yield and soil microbes suggests that crop productivity in Spain might be limited by other, more impactful factors that could overshadow any potential effects of microbes, such as nutrient or water availability. This is consistent with a recent study by Adomako et al. (2020), in which they found that the positive effects of soil microbes on plant biomass were stronger under high nutrient and water availability compared to low resource conditions. Indeed, in conditions of nutrient stress, microbial communities may compete for the limited available nutrients (Ho and Chambers, 2019). If the amount of nutrients is insufficient, it is possible that microbes cannot exchange nutrients for carbon with the plants anymore, which can lead to negative plant–soil feedbacks (Petermann et al., 2008; Zandt et al., 2019). In the other way around, nutrient or water limitation, by restricting plant growth—as it was the case in Spain (Figure 2A)—might also reduce the amount of carbon provided by the plants to fuel the microbial communities (Harrison and Bardgett, 2010). This reduction in plant–soil feedbacks raises important questions on the universality of the role of soil microbes for plant growth, and calls for more experiments in various soil and climatic conditions to confirm and improve our understanding of crop–soil microbes relationships in agricultural systems.
Our study shows that in some cases, crop diversity has the potential to influence soil microbial community composition and that these changes partially explain the positive effects of intercropping on yield. However, these effects are relative and less important than changes in abiotic factors, such as the addition of mineral fertilizer. Furthermore, these processes are context-dependent: in Spain, soil microbial communities do not respond to crop diversity, and their effect on crop yield is weaker. We hypothesize that these contrasting responses could be explained by differences in abiotic conditions and resources. However, further investigation is required to test this context-dependency hypothesis. Considered as a whole, this research suggests that soil microbial communities can play a beneficial role in intercropped systems. Yet this positive result is not universal; on the contrary, it reflects on the relative importance of microbial communities compared to abiotic factors for increasing crop productivity, and highlights the context-dependency of crop–microbe relationships.
The datasets presented in this study can be found in online repositories. The names of the repository/repositories and accession number(s) can be found below: https://doi.org/10.5281/zenodo.4479277.
LS and CS conceived the study with input from MH, JS, and NE. LS, NE, and CS collected the data. LS assembled and analyzed the data with the help of CS and MH. LS, MH, and CS wrote the manuscript. All authors discussed the data analyses and results.
This study was funded by the Swiss National Science Foundation (PP00P3_170645).
The authors declare that the research was conducted in the absence of any commercial or financial relationships that could be construed as a potential conflict of interest.
We are grateful to Elisa Pizarro Carbonell, Carlos Barriga Cabanillas, Anja Schmutz, Roman Hüppi, and Simon Baumgartner for their help with the field experiment, Manon Longepierre for her mentoring and help with the DNA lab work and analyses, Matti Barthel for his help with the soil respiration method, and Barryette Oberholzer for her help with the DNA lab work. We thank Maria Domenica Moccia at the Functional Genomics Center Zurich (FGCZ) for optimizing the sequencing protocol and providing the sequencing service on the Illumina MiSeq platform. We also thank the Aprisco de Las Corchuelas Field Station and the University of Zurich for the use of their fields.
The Supplementary Material for this article can be found online at: https://www.frontiersin.org/articles/10.3389/fmicb.2021.660749/full#supplementary-material
Abarenkov, K., Nilsson, R. H., Larsson, K. H., Alexander, I. J., Eberhardt, U., Erland, S., et al. (2010). The UNITE database for molecular identification of fungi - recent updates and future perspectives. N. Phytol. 186, 281–285. doi: 10.1111/j.1469-8137.2009.03160.x
Adomako, M. O., Xue, W., Tang, M., Du, D. L., and Yu, F. H. (2020). Synergistic Effects of Soil Microbes on Solidago canadensis Depend on Water and Nutrient Availability. Microb. Ecol. 80, 837–845. doi: 10.1007/s00248-020-01537-2
Anderson, M. J. (2001). A new method for non-parametric multivariate analysis of variance. Aust. Ecol. 26, 32–46. doi: 10.1111/j.1442-9993.2001.01070.pp.x
Anderson, M. J. (2006). Distance-based tests for homogeneity of multivariate dispersions. Biometrics 62, 245–253. doi: 10.1111/j.1541-0420.2005.00440.x
Anderson, M. J., and Willis, T. J. (2003). Canonical Analysis of Principal Coordinates: A Useful Method of Constrained Ordination for Ecology. Ecology 84, 511–525. doi: 10.1890/0012-9658(2003)084[0511:caopca]2.0.co;2
Aragona, M., Minio, A., Ferrarini, A., Valente, M. T., Bagnaresi, P., Orrù, L., et al. (2014). De novo genome assembly of the soil-borne fungus and tomato pathogen Pyrenochaeta lycopersici. BMC Genomics 15:313. doi: 10.1186/1471-2164-15-313
Bach, E. M., Baer, S. G., Meyer, C. K., and Six, J. (2010). Soil texture affects soil microbial and structural recovery during grassland restoration. Soil Biol. Biochem. 42, 2182–2191. doi: 10.1016/j.soilbio.2010.08.014
Bais, H. P., Weir, T. L., Perry, L. G., Gilroy, S., and Vivanco, J. M. (2006). The role of root exudates in rhizosphere interactions with plants and other organisms. Ann. Rev. Plant Biol. 57, 233–266. doi: 10.1146/annurev.arplant.57.032905.105159
Bardgett, R. D., and Van Der Putten, W. H. (2014). Belowground biodiversity and ecosystem functioning. Nature 515, 505–511. doi: 10.1038/nature13855
Barrios, E. (2007). Soil biota, ecosystem services and land productivity. Ecol. Econom. 64, 269–285. doi: 10.1016/j.ecolecon.2007.03.004
Bender, S. F., and van der Heijden, M. G. A. (2015). Soil biota enhance agricultural sustainability by improving crop yield, nutrient uptake and reducing nitrogen leaching losses. J. Appl. Ecol. 52, 228–239. doi: 10.1111/1365-2664.12351
Bengtsson-Palme, J., Hartmann, M., Eriksson, K. M., Pal, C., Thorell, K., Larsson, D. G. J., et al. (2015). metaxa2: Improved identification and taxonomic classification of small and large subunit rRNA in metagenomic data. Mole. Ecol. Resour. 15, 1403–1414. doi: 10.1111/1755-0998.12399
Bengtsson-Palme, J., Ryberg, M., Hartmann, M., Branco, S., Wang, Z., Godhe, A., et al. (2013). Improved software detection and extraction of ITS1 and ITS2 from ribosomal ITS sequences of fungi and other eukaryotes for analysis of environmental sequencing data. Methods Ecol. Evol. 2013:4.
Benjamini, Y., and Hochberg, Y. (1995). Controlling the false discovery rate: a practical and powerful approach to multiple testing. J. R. Statist. Soc. Ser. B (Methodol.) 57, 289–300.
Bhattacharyya, P. N., and Jha, D. K. (2012). Plant growth-promoting rhizobacteria (PGPR): Emergence in agriculture. World J. Microb. Biotechnol. 28, 1327–1350. doi: 10.1007/s11274-011-0979-9
Botha, A. (2011). The importance and ecology of yeasts in soil. Soil Biol. Biochem. 43, 1–8. doi: 10.1016/j.soilbio.2010.10.001
Cáceres, M., and Legendre, P. (2009). Associations between species and groups of sites: indices and statistical inference. Ecology 90, 3566–3574. doi: 10.1890/08-1823.1
Cadotte, M. W. (2017). Functional traits explain ecosystem function through opposing mechanisms (H Hillebrand, Ed.). Ecol. Lett. 20, 989–996. doi: 10.1111/ele.12796
Cavicchioli, R., Ripple, W. J., Timmis, K. N., Azam, F., Bakken, L. R., Baylis, M., et al. (2019). Scientists’ warning to humanity: microorganisms and climate change. Nat. Rev. Microbiol. 17, 569–586.
Chen, J., Engbersen, N., Stefan, L., Schmid, B., Sun, H., and Schob, C. (2020). Diversity increases yield but reduces reproductive effort in crop mixtures. bioRxiv 2020:149187.
Conn, V. M., and Franco, C. M. M. (2004). Analysis of the Endophytic Actinobacterial Population in the Roots of Wheat (Triticum aestivum L.) by Terminal Restriction Fragment Length Polymorphism and Sequencing of 16S rRNA Clones. Appl. Env. Microb. 70, 1787–1794. doi: 10.1128/aem.70.3.1787-1794.2004
Curiel Yuste, J., Baldocchi, D. D., Gershenson, A., Goldstein, A., Misson, L., and Wong, S. (2007). Microbial soil respiration and its dependency on carbon inputs, soil temperature and moisture. Glob. Change Biol. 13, 2018–2035. doi: 10.1111/j.1365-2486.2007.01415.x
Dai, M., Hamel, C., Bainard, L. D., Arnaud, M. S., Grant, C. A., Lupwayi, N. Z., et al. (2014). Negative and positive contributions of arbuscular mycorrhizal fungal taxa to wheat production and nutrient uptake efficiency in organic and conventional systems in the Canadian prairie. Soil Biol. Biochem. 74, 156–166. doi: 10.1016/j.soilbio.2014.03.016
Dai, Y., Yang, F., Zhang, L., Xu, Z., Fan, X., Tian, Y., et al. (2020). Wheat-associated microbiota and their correlation with stripe rust reaction. J. Appl. Microb. 128, 544–555. doi: 10.1111/jam.14486
Daims, H., Lücker, S., and Wagner, M. (2016). A New Perspective on Microbes Formerly Known as Nitrite-Oxidizing Bacteria. Trends Microb. 24, 699–712. doi: 10.1016/j.tim.2016.05.004
Delgado-Baquerizo, M., Fry, E. L., Eldridge, D. J., de Vries, F. T., Manning, P., Hamonts, K., et al. (2018). Plant attributes explain the distribution of soil microbial communities in two contrasting regions of the globe. N. Phytol. 219, 574–587. doi: 10.1111/nph.15161
Dimkpa, C., Weinand, T., and Asch, F. (2009). Plant-rhizobacteria interactions alleviate abiotic stress conditions. Plant Cell Env. 32, 1682–1694. doi: 10.1111/j.1365-3040.2009.02028.x
Doornbos, R. F., Van Loon, L. C., and Bakker, P. A. H. M. (2012). Impact of root exudates and plant defense signaling on bacterial communities in the rhizosphere. Rev. Agron. Sustain. Develop. 32, 227–243. doi: 10.1007/s13593-011-0028-y
Edgar, R. C. (2016a). SINTAX: a simple non-Bayesian taxonomy classifier for 16S and ITS sequences. biorxiv 2016:074161. doi: 10.1101/074161
Edgar, R. C. (2016b). UCHIME2: improved chimera prediction for amplicon sequencing. biorxiv 2016:074252.
Edgar, R. C. (2016c). UNOISE2: improved error-correction for Illumina 16S and ITS amplicon sequencing. Biorxiv 2016:081257.
Edgar, R. C., and Flyvbjerg, H. (2015). Error filtering, pair assembly and error correction for next-generation sequencing reads. Bioinformatics 31, 3476–3482. doi: 10.1093/bioinformatics/btv401
Eisenhauer, N., Beßler, H., Engels, C., Gleixner, G., Habekost, M., Milcu, A., et al. (2010). Plant diversity effects on soil microorganisms support the singular hypothesis. Ecology 91, 485–496. doi: 10.1890/08-2338.1
Eisenhauer, N., Lanoue, A., Strecker, T., Scheu, S., Steinauer, K., Thakur, M. P., et al. (2017). Root biomass and exudates link plant diversity with soil bacterial and fungal biomass. Sci. Rep. 7, 1–8.
Engbersen, N., Stefan, L., Brooker, R. W., and Schöb, C. (2020). Using plant traits to understand the contribution of biodiversity effects to community productivity in an agricultural system. bioRxiv 2020:157008.
Fanin, N., Gundale, M. J., Farrell, M., Ciobanu, M., Baldock, J. A., Nilsson, M.-C., et al. (2017). Consistent effects of biodiversity loss on multifunctionality across contrasting ecosystems. Nat. Ecol. Evol. 2017:1.
Fierer, N., Bradford, M. A., and Jackson, R. B. (2007). Toward an ecological classification of soil bacteria. Ecology 88, 1354–1364. doi: 10.1890/05-1839
Fierer, N., Lauber, C. L., Ramirez, K. S., Zaneveld, J., Bradford, M. A., and Knight, R. (2012). Comparative metagenomic, phylogenetic and physiological analyses of soil microbial communities across nitrogen gradients. ISME J. 6, 1007–1017. doi: 10.1038/ismej.2011.159
Franco-Correa, M., Quintana, A., Duque, C., Suarez, C., Rodríguez, M. X., and Barea, J. M. (2010). Evaluation of actinomycete strains for key traits related with plant growth promotion and mycorrhiza helping activities. Appl. Soil Ecol. 45, 209–217. doi: 10.1016/j.apsoil.2010.04.007
Frey, B., Rime, T., Phillips, M., Stierli, B., Hajdas, I., Widmer, F., et al. (2016). Microbial diversity in European alpine permafrost and active layers. FEMS Microb. Ecol. 2016:92.
Fry, E. L., Savage, J., Hall, A. L., Oakley, S., Pritchard, W. J., Ostle, N. J., et al. (2018). Soil multifunctionality and drought resistance are determined by plant structural traits in restoring grassland. Ecology 99, 2260–2271. doi: 10.1002/ecy.2437
Gardi, C., Jeffery, S., and Saltelli, A. (2013). An estimate of potential threats levels to soil biodiversity in EU. Glob. Change Biol. 2013:19.
Gower, J. C. (1966). Some distance properties of latent root and vector methods used in multivariate analysis. Biometrika 53, 325–338. doi: 10.2307/2333639
Grace, J. (2006). Structural equation modeling and natural systems. Cambridge: Cambridge University Press
Gray, E. J., and Smith, D. L. (2005). Intracellular and extracellular PGPR: Commonalities and distinctions in the plant-bacterium signaling processes. Soil Biol. Biochem. 37, 395–412. doi: 10.1016/j.soilbio.2004.08.030
Grüter, D., Schmid, B., and Brandl, H. (2006). Influence of plant diversity and elevated atmospheric carbon dioxide levels on belowground bacterial diversity. BMC Microb. 6:1–8. doi: 10.1201/b10812-2
Guo, Z., Wan, S., Hua, K., Yin, Y., Chu, H. Y., Wang, D., et al. (2020). Fertilization regime has a greater effect on soil microbial community structure than crop rotation and growth stage in an agroecosystem. Appl. Soil Ecol. 2020:149.
Gupta, R. S. (2004). The phylogeny and signature sequences characteristics of Fibrobacteres, Chlorobi, and Bacteroidetes. Crit. Rev. Microb. 30, 123–143. doi: 10.1080/10408410490435133
Hamedi, J., and Mohammadipanah, F. (2014). Biotechnological application and taxonomical distribution of plant growth promoting actinobacteria. J. Industr. Microb. Biotechnol. 42, 157–171. doi: 10.1007/s10295-014-1537-x
Harrison, K. A., and Bardgett, R. D. (2010). Influence of plant species and soil conditions on plant-soil feedback in mixed grassland communities. J. Ecol. 98, 384–395. doi: 10.1111/j.1365-2745.2009.01614.x
Ho, J., and Chambers, L. G. (2019). Altered soil microbial community composition and function in two shrub-encroached marshes with different physicochemical gradients. Soil Biol. Biochem. 130, 122–131. doi: 10.1016/j.soilbio.2018.12.004
Zandt, D., van den Brink, A., de Kroon, H., and Visser, E. J. W. (2019). Plant-soil feedback is shut down when nutrients come to town. Plant Soil 439, 541–551. doi: 10.1007/s11104-019-04050-9
Jesch, A., Barry, K. E., Ravenek, J. M., Bachmann, D., Strecker, T., Weigelt, A., et al. (2018). Below-ground resource partitioning alone cannot explain the biodiversity–ecosystem function relationship: A field test using multiple tracers. J. Ecol. 106, 2002–2018. doi: 10.1111/1365-2745.12947
Khan, M. R., and Parveen, G. (2018). Supplementing biocontrol agents with botanicals improved growth and yield of coriander (Coriandrum sativum L.) infected with Protomyces macrosporus Unger. Curr. Plant Biol. 15, 44–50. doi: 10.1016/j.cpb.2018.10.005
Kindt, R., and Coe, R. (2005). Tree Diversity Analysis. A manual and software for common statistical methods and biodiversity studies. Nairobi: World Agroforestry Centre (ICRAF)
Kline, R. B. (2011). Principles and Practice of Structural Equation Modeling. New York, NY: Guilford Press
Knops, J. M. H., and Tilman, D. (2000). Dynamics of soil nitrogen and carbon accumulation for 61 years after agricultural abandonment. Ecology 81, 88–98. doi: 10.1890/0012-9658(2000)081[0088:dosnac]2.0.co;2
Landesman, W. J., and Dighton, J. (2010). Response of soil microbial communities and the production of plant-available nitrogen to a two-year rainfall manipulation in the New Jersey Pinelands. Soil Biol. Biochem. 42, 1751–1758.
Lange, M., Eisenhauer, N., Sierra, C. A., Bessler, H., Engels, C., Griffiths, R. I., et al. (2015). Plant diversity increases soil microbial activity and soil carbon storage. Nat. Commun. 2015:6.
Lange, M., Habekost, M., Eisenhauer, N., Roscher, C., Bessler, H., Engels, C., et al. (2014). Biotic and abiotic properties mediating plant diversity effects on soil microbial communities in an experimental grassland. PLoS One 2014:9.
Langmead, B., and Salzberg, S. L. (2012). Fast gapped-read alignment with Bowtie 2. Nat. Methods 9, 357–359. doi: 10.1038/nmeth.1923
López-Angulo, J., Cruz, M., Chacón-Labella, J., Illuminati, A., Matesanz, S., Pescador, D. S., et al. (2020). The role of root community attributes in predicting soil fungal and bacterial community patterns. N. Phytologist 228, 1070–1082. doi: 10.1111/nph.16754
Loreau, M., and Hector, A. (2001). Partitioning selection and complementarity in biodiversity experiments. Nature 412, 72–76. doi: 10.1038/35083573
Lupwayi, N. Z., Fernandez, M. R., Kanashiro, D. A., and Petri, R. M. (2020). Profiles of wheat rhizobacterial communities in response to repeated glyphosate applications, crop rotation and tillage. Can. J. Soil Sci. 2020:0008.
Lupwayi, N. Z., May, W. E., Kanashiro, D. A., and Petri, R. M. (2018). Soil bacterial community responses to black medic cover crop and fertilizer N under no-till. Appl. Soil Ecol. 124, 95–103. doi: 10.1016/j.apsoil.2017.11.003
Maestre, F. T., Bowker, M. A., Puche, M. D., Belén Hinojosa, M., Martínez, I., García-Palacios, P., et al. (2009). Shrub encroachment can reverse desertification in semi-arid Mediterranean grasslands. Ecol. Lett. 12, 930–941. doi: 10.1111/j.1461-0248.2009.01352.x
Mahoney, A. K., Yin, C., and Hulbert, S. H. (2017). Community structure, species variation, and potential functions of rhizosphere-associated bacteria of different winter wheat (Triticum aestivum) cultivars. Front. Plant Sci. 8:132.
Martin, M. (2011). Cutadapt removes adapter sequences from high-throughput sequencing reads. EMBnet.J. 17:10. doi: 10.14806/ej.17.1.200
Melo, J., Carolino, M., Carvalho, L., Correia, P., Tenreiro, R., Chaves, S., et al. (2016). Crop management as a driving force of plant growth promoting rhizobacteria physiology. SpringerPlus 5, 1–16. doi: 10.1094/cm-2004-0301-05-rv
Merzaeva, O. V., and Shirokikh, I. G. (2006). Colonization of plant rhizosphere by actinomycetes of different genera. Microbiology 75, 226–230. doi: 10.1134/s0026261706020184
Moreno-Lora, A., Recena, R., and Delgado, A. (2019). Bacillus subtilis QST713 and cellulose amendment enhance phosphorus uptake while improving zinc biofortification in wheat. Appl. Soil Ecol. 142, 81–89. doi: 10.1016/j.apsoil.2019.04.013
Mukherjee, A., Prakash Verma, J., Kumar Gaurav, A., Kumar Chouhan, G., Singh Patel, J., and El-Latif Hesham, A. (2020). Yeast a potential bio-agent: future for plant growth and postharvest disease management for sustainable agriculture. Appl. Microb. Biotechnol. 2020, 1497–1510. doi: 10.1007/s00253-019-10321-3
Newbold, T., Hudson, L. N., Hill, S. L. L., Contu, S., Lysenko, I., Senior, R. A., et al. (2015). Global effects of land use on local terrestrial biodiversity. Nature 520, 45–50.
Oksanen, J., Blanchet, F. G., Friendly, M., Kindt, R., Legendre, P., Mcglinn, D., et al. (2019). R package version 2.2-5. vegan: Community Ecology Package.
Petermann, J. S., Fergus, A. J. F., Turnbull, L. A., and Schmid, B. (2008). Janzen-Connell effects are widespread and strong enough to maintain diversity in grasslands. Ecology 89, 2399–2406. doi: 10.1890/07-2056.1
Philippot, L., Raaijmakers, J. M., Lemanceau, P., and Van Der Putten, W. H. (2013). Going back to the roots: The microbial ecology of the rhizosphere. Nat. Rev. Microb. 11, 789–799. doi: 10.1038/nrmicro3109
Prober, S. M., Leff, J. W., Bates, S. T., Borer, E. T., Firn, J., Harpole, W. S., et al. (2015). Plant diversity predicts beta but not alpha diversity of soil microbes across grasslands worldwide. Ecol. Lett. 18, 85–95. doi: 10.1111/ele.12381
Pruesse, E., Quast, C., Knittel, K., Fuchs, B. M., Ludwig, W., Peplies, J., et al. (2007). SILVA: a comprehensive online resource for quality checked and aligned ribosomal RNA sequence data compatible with ARB. Nucleic Acids Res. 35, 7188–7196. doi: 10.1093/nar/gkm864
Van der Putten, W. H., Bardgett, R. D., Bever, J. D., Bezemer, T. M., Casper, B. B., Fukami, T., et al. (2013). Plant-soil feedbacks: The past, the present and future challenges. J. Ecol. 101, 265–276.
Qin, S., Xing, K., Jiang, J. H., Xu, L. H., and Li, W. J. (2011). Biodiversity, bioactive natural products and biotechnological potential of plant-associated endophytic actinobacteria. Appl. Microb. Biotechnol. 89, 457–473. doi: 10.1007/s00253-010-2923-6
R Core Team. (2019). A language and environment for statistical computing. Vienna: R Foundation for Statistical Computing.
Ramirez, K. S., Lauber, C. L., Knight, R., Bradford, M. A., and Fierer, N. (2010). Consistent effects of nitrogen fertilization on soil bacterial communities in contrasting systems. Ecology 91, 3463–3470. doi: 10.1890/10-0426.1
Rascovan, N., Carbonetto, B., Perrig, D., Díaz, M., Canciani, W., Abalo, M., et al. (2016). Integrated analysis of root microbiomes of soybean and wheat from agricultural fields. Sci. Rep. 6, 1–12.
Ren, C., Zhang, W., Zhong, Z. K., Han, X., Yang, G., Feng, Y., et al. (2018). Differential responses of soil microbial biomass, diversity, and compositions to altitudinal gradients depend on plant and soil characteristics. Sci. Total Env. 61, 750–758. doi: 10.1016/j.scitotenv.2017.08.110
Rosseel, Y. (2012). lavaan: an R package for structural equation modeling and more. Version 0.5-12. J. Statist. Softw. 48, 1–36.
Royston, J. P. (1982). An Extension of Shapiro and Wilk’s W Test for Normality to Large Samples. Appl. Stat. 31:115. doi: 10.2307/2347973
Schlatter, D. C., Yin, C., Burke, I., Hulbert, S., and Paulitz, T. (2018). Location, Root Proximity, and Glyphosate-Use History Modulate the Effects of Glyphosate on Fungal Community Networks of Wheat. Microb. Ecol. 76, 240–257. doi: 10.1007/s00248-017-1113-9
Shaharoona, B., Jamro, G. M., Zahir, Z. A., Arshad, M., and Memon, K. S. (2007). Effectiveness of various Pseudomonas spp. and Burkholderia caryophylli containing ACC-deaminase for improving growth and yield of wheat (Triticum aestivum L.). J. Microb. Biotechnol. 17, 1300–1307.
Singhal, U., Prasad, R., and Varma, A. (2017). Piriformospora indica (Serendipita indica): The novel symbiont. In: Mycorrhiza - Function, Diversity, State of the Art: Fourth Edition. New York, NY: Springer International Publishing, 349–364.
Stefan, L., Engbersen, N., and Schöb, C. (2020). Weeds are not always evil: crop-weed relationships are context-dependent and cannot fully explain the positive effects of intercropping on yield. bioRxiv 2020:021402.
Stefan, L., Engbersen, N., and Schöb, C. (2021). Crop–weed relationships are context-dependent and cannot fully explain the positive effects of intercropping on yield. Ecol. Appl. 2021:e2311.
Steinauer, K., Tilman, D., Wragg, P. D., Cesarz, S., Cowles, J. M., Pritsch, K., et al. (2015). Plant diversity effects on soil microbial functions and enzymes are stronger than warming in a grassland experiment. Ecology 96, 99–112. doi: 10.1890/14-0088.1
Tedersoo, L., and Lindahl, B. (2016). Fungal identification biases in microbiome projects. Env. Microb. Rep. 8, 774–779. doi: 10.1111/1758-2229.12438
Tilman, D., Balzer, C., Hill, J., and Befort, B. L. (2011). Global food demand and the sustainable intensification of agriculture. Proc. Natl. Acad. Sci. 108, 20260–20264.
Tilman, D., Reich, P. B., and Knops, J. M. (2006). Biodiversity and ecosystem stability in a decade-long grassland experiment. Nature 441, 629–632. doi: 10.1038/nature04742
Trinder, C. J., Johnson, D., and Artz, R. R. E. (2009). Litter type, but not plant cover, regulates initial litter decomposition and fungal community structure in a recolonising cutover peatland. Soil Biol. Biochem. 41, 651–655. doi: 10.1016/j.soilbio.2008.12.006
Voroney, R. P. (2007). Chapter 2 THE SOIL HABITAT. Soil Microb. Ecol. Biochemistr. 2007, 25–49. doi: 10.1016/b978-0-08-047514-1.50006-8
Wagg, C., Bender, S. F., Widmer, F., and van der Heijden, M. G. A. (2014). Soil biodiversity and soil community composition determine ecosystem multifunctionality. Proc. Natl. Acad. Sci. 111, 5266–5270. doi: 10.1073/pnas.1320054111
Wang, G. Z., Li, H. G., Christie, P., Zhang, F. S., Zhang, J. L., and Bever, J. D. (2017). Plant-soil feedback contributes to intercropping overyielding by reducing the negative effect of take-all on wheat and compensating the growth of faba bean. Plant Soil 415, 1–12. doi: 10.1007/s11104-016-3139-z
Wardle, D. A. (2006). The influence of biotic interactions on soil biodiversity. Ecol. Lett. 9, 870–886. doi: 10.1111/j.1461-0248.2006.00931.x
Wardle, D. A., Bardgett, R. D., Klironomos, J. N., Setälä, H., Van Der Putten, W. H., and Wall, D. H. (2004). Ecological linkages between aboveground and belowground biota. Science 304, 1629–1633. doi: 10.1126/science.1094875
Wick, A. F., Huzurbazar, S. V., and Stahl, P. D. (2009). Use of Bayesian Methods to Model Soil Aggregation in Undisturbed Semiarid Grasslands. Soil Sci. Soc. Am. J. 73, 1707–1714. doi: 10.2136/sssaj2008.0185
Wubs, E. R. J., and Bezemer, T. M. (2016). Effects of spatial plant–soil feedback heterogeneity on plant performance in monocultures (A Austin. Ed.). J. Ecol. 104, 364–376. doi: 10.1111/1365-2745.12521
Xiao, X., Fan, M., Wang, E., Chen, W., and Wei, G. (2017). Interactions of plant growth-promoting rhizobacteria and soil factors in two leguminous plants. Appl. Microb. Biotechnol. 101, 8485–8497. doi: 10.1007/s00253-017-8550-8
Yang, T., Adams, J. M., Shi, Y., He, J., Jing, X., Chen, L., et al. (2017). Soil fungal diversity in natural grasslands of the Tibetan Plateau: associations with plant diversity and productivity. N. Phytol. 215, 756–765. doi: 10.1111/nph.14606
Zhao, K., Penttinen, P., Zhang, X., Ao, X., Liu, M., Yu, X., et al. (2014). Maize rhizosphere in Sichuan, China, hosts plant growth promoting Burkholderia cepacia with phosphate solubilizing and antifungal abilities. Microb. Res. 169, 76–82. doi: 10.1016/j.micres.2013.07.003
Keywords: intercropping, soil microbial communities, biodiversity–productivity relationship, sustainable agriculture, annual crop yield, crop diversification
Citation: Stefan L, Hartmann M, Engbersen N, Six J and Schöb C (2021) Positive Effects of Crop Diversity on Productivity Driven by Changes in Soil Microbial Composition. Front. Microbiol. 12:660749. doi: 10.3389/fmicb.2021.660749
Received: 29 January 2021; Accepted: 19 March 2021;
Published: 15 April 2021.
Edited by:
Anna Maria Pirttilä, University of Oulu, FinlandReviewed by:
Biao Zhu, Peking University, ChinaCopyright © 2021 Stefan, Hartmann, Engbersen, Six and Schöb. This is an open-access article distributed under the terms of the Creative Commons Attribution License (CC BY). The use, distribution or reproduction in other forums is permitted, provided the original author(s) and the copyright owner(s) are credited and that the original publication in this journal is cited, in accordance with accepted academic practice. No use, distribution or reproduction is permitted which does not comply with these terms.
*Correspondence: Laura Stefan, bGF1cmEuc3RlZmFuQHVzeXMuZXRoei5jaA==
Disclaimer: All claims expressed in this article are solely those of the authors and do not necessarily represent those of their affiliated organizations, or those of the publisher, the editors and the reviewers. Any product that may be evaluated in this article or claim that may be made by its manufacturer is not guaranteed or endorsed by the publisher.
Research integrity at Frontiers
Learn more about the work of our research integrity team to safeguard the quality of each article we publish.