- 1Life and Health Sciences Research Institute (ICVS), School of Medicine, University of Minho, Braga, Portugal
- 2ICVS/3B’s – PT Government Associate Laboratory, Braga, Portugal
- 3i3S – Instituto de Investigacão e Inovação em Saúde, University of Porto, Porto, Portugal
- 4Instituto de Biologia Molecular e Celular (IBMC), University of Porto, Porto, Portugal
- 5Department of Clinical Pathology, Centro Hospitalar São João, Porto, Portugal
- 6Biomedicine Institute of Valencia IBV-CSIC, Valencia, Spain
- 7CIBER in Epidemiology and Public Health, Valencia, Spain
- 8Institute of Public Health, University of Porto, Porto, Portugal
- 9Department of Biochemistry, Faculty of Medicine, University of Porto, Porto, Portugal
- 10Serviço de Pneumologia, Centro Hospitalar Universitário de São João EPE, Porto, Portugal
Widespread and frequent resistance to the second-line tuberculosis (TB) medicine streptomycin, suggests ongoing transmission of low fitness cost streptomycin resistance mutations. To investigate this hypothesis, we studied a cohort of 681 individuals from a TB epidemic in Portugal. Whole-genome sequencing (WGS) analyses were combined with phenotypic growth studies in culture media and in mouse bone marrow derived macrophages. Streptomycin resistance was the most frequent resistance in the cohort accounting for 82.7% (n = 67) of the resistant Mycobacterium tuberculosis isolates. WGS of 149 clinical isolates identified 13 transmission clusters, including three clusters containing only streptomycin resistant isolates. The biggest cluster was formed by eight streptomycin resistant isolates with a maximum of five pairwise single nucleotide polymorphisms of difference. Interestingly, despite their genetic similarity, these isolates displayed different resistance levels to streptomycin, as measured both in culture media and in infected mouse bone marrow derived macrophages. The genetic bases underlying this phenotype are a combination of mutations in gid and other genes. This study suggests that specific streptomycin resistance mutations were transmitted in the cohort, with the resistant isolates evolving at the cluster level to allow low-to-high streptomycin resistance levels without a significative fitness cost. This is relevant not only to better understand transmission of streptomycin resistance in a clinical setting dominated by Lineage 4 M. tuberculosis infections, but mainly because it opens new prospects for the investigation of selection and spread of drug resistance in general.
Introduction
Emergence of drug-resistant Mycobacterium tuberculosis strains, classically attributed to lack of patient adherence to the prolonged therapeutic regimens, hinders tuberculosis (TB) control (Murray et al., 2015; WHO, 2020). Resistant M. tuberculosis strains can transmit in the population, therefore amplifying the drug resistance (DR) problem (Becerra et al., 2019). Thus, understanding the mechanisms and molecular bases underlying DR acquisition and transmission is key to overcome a most challenging issue in TB treatment.
Resistance to streptomycin (STR), an antibiotic used in the past as a TB monotherapy, was the first M. tuberculosis DR described (Pyle, 1947; Crofton and Mitchison, 1948). Although STR is now a second-line TB medicine, STR resistant M. tuberculosis isolates remain frequent, with a special high incidence in Western-Europe (Manson et al., 2017). In Germany, monoresistance to STR was both the most prevalent form of DR and the most frequent DR among multidrug-resistant (MDR) stains (Glasauer et al., 2019). In Portugal, mutations in STR target genes were identified in isolates from the Lisbon family genetic cluster Q1, a cluster associated with MDR in the Lisbon area (Perdigão et al., 2013, 2014). Furthermore, a whole genome-based study suggested isoniazid and STR resistance mutations as precursors of MDR M. tuberculosis strains (Manson et al., 2017). The sustained transmission of STR resistant strains, as well as its possible implications for MDR acquisition, should not be neglected, calling for more studies in this area.
STR resistance is primarily associated with mutations in rpsL, rrs, and gid, genes, which, respectively, encode for the components of the 30S ribosome subunits S12, 16S rRNA and for a 7-methylguanosine methyltransferase specific for the 16S rRNA (Okamoto et al., 2007; Jagielski et al., 2014). The mutated gene might modulate the level of STR resistance. Polymorphisms in the rpsL gene were associated to high STR resistance levels, while those in the rrs locus or in the gid gene were linked to intermediate to high and low STR resistance levels, respectively (Wong et al., 2011; Smittipat et al., 2016; Cohen et al., 2020). The type of STR resistant mutation seems to vary according to M. tuberculosis phylogeography. For example, specific mutations in rpsL or rrs (rpsL:Lys43Arg, Lys88Arg; rrs: a514c or c517t) are very common among the STR-resistant isolates from South-East Asian countries with high incidence of lineage 2 (Nhu et al., 2012; Smittipat et al., 2016; Hlaing et al., 2017). In contrast, polymorphisms in gid were more associated with STR resistance in isolates from Euro-American lineage 4 (L4) (Hlaing et al., 2017; Oppong et al., 2019). The high frequency of STR resistance in Western-Europe may therefore result from the high prevalence of STR resistant mutations in gid and their efficient transmission in the absence of antibiotic pressure. Here, we retrospectively studied the STR resistance prevalence in a previously reported cohort of 681 individuals (Bastos et al., 2016; Sousa et al., 2020), from a TB epidemic in Portugal, and used genomics and culture models to compare STR resistant and susceptible clinical isolates in terms of inferred transmissibility, genetic diversity, and growth.
Materials and Methods
Bacterial Culture and Drug Susceptibility Tests
A cohort of 681 culture-confirmed TB cases diagnosed at Centro Hospitalar São João (CHUSJ), Porto-Portugal between 2007 and 2013 was used (Bastos et al., 2016; Sousa et al., 2020). Isolates were obtained from the sputum of the patients and then cultured in a biosafety level 3 facility on solid 7H11 agar supplemented with Oleic Albumin Dextrose Catalase Growth Supplement (OADC) and plymyxin B, amphotericin B, nalidixic acid, trimethoprim and azlocillin (PANTA antibiotic mixture, BD). The plates were incubated for 3 weeks at 37°C. After this period, colonies were suspended, optical density was adjusted to 0.01–0.03 (OD620nm) and BACTECTM MGITTM 960 tubes (Mycobacteria Growth Indicator Tubes, BD Biosciences) supplemented with OADC were inoculated and grown until mid-log phase. These bacterial cultures were subcultured for drug susceptibility testing (DST) to isoniazid (INH), rifampicin (RIF), STR, ethambutol (ETH), and pyrazinamide (PZA) using a protocol based on the proportion method (MOP) (Woods et al., 2011). Briefly, a 7.0 mL inoculum was prepared in a BD MGIT tube and used after positivity to inoculate a new BD MGIT tube with an antibiotic. In each tube, 800 μL of BD BACTEC MGIT SIRE Supplement (Middlebrook OADC) was aseptically added. In the drug-containing tube it was added 100 μL of antibiotic and then 500 μL of positive inoculum. In the growth control tube 100 μL of M. tuberculosis suspension was diluted in 10 mL of saline solution, then 500 mL of the diluted suspension was transferred into a BD MGIT suspension. The concentration used was the recommended critical concentration for MOP, 1.0 mg/L STR; 0.1 mg/L INH; 1.0 mg/L RIF; 5.0 mg/L ETH. For STR and INH a higher concentration was used to profile the degree of resistance, 4.0 and 0.4 mg/L, respectively. The isolates were considered resistant if 1% or more of the test population grew in the presence of the critical concentration. Monoresistance was defined as resistance exclusively to one of the four first-line anti-tuberculosis drugs tested. Polyresistance was defined as resistance to two or more anti-tuberculosis drugs, but not both isoniazid and rifampicin. MDR was resistance to at least isoniazid and rifampicin simultaneously.
Streptomycin Resistance Level
Mid-log phase cultures were used to test the phenotypic susceptibility to streptomycin. 500 μL of the bacterial cultures were inoculated in BACTECTM MGITTM 960 tubes (BD Biosciences) supplemented with 800 μL of OADC and 100 μL of STR. The cultures were incubated at 37 °C until the stationary phase of growth or a maximum of 42 days. The concentrations used to determine the resistance level were 1.0 and 10.0 mg/L of STR. For each M. tuberculosis clinical isolate, a drug-free control (M. tuberculosis only) was also included. Bacterial cultures were stopped at the planktonic growth phase or after 42 days of incubation. The BACTEC growth measurements were reported automatically. Resistance level was defined as high if the growth was observed at both STR concentrations with the same time to positivity, intermediate if the bacteria grew at both concentrations with high time to positivity at the highest STR concentration, and as low if the bacteria grew only at the lowest STR concentration.
BMDM Generation and Infection
Macrophages were derived in vitro from mouse bone marrow cell suspensions as previously described (Moreira-Teixeira et al., 2016; Bhatt et al., 2018). On day 7, cells were harvested, counted, plated in 96 well plates and infected with a MOI of 2. Four hours post-infection the cells were washed and incubated without STR or with 10.0 and 100.0 mg/L of STR. Six days post-infection macrophages were lysed with saponin and the number of viable bacteria determined by CFU enumeration.
Whole-Genome Sequencing and Analysis
DNA was extracted using the phenol-chloroform/bead beater method as described previously (Sousa et al., 2020). Paired-end sequencing of the DNA extracted from each isolate was performed in Illumina MiSeq, HiSeq 2000/2500 and NextSeq 500 instruments. Raw reads were subjected to quality trimming with Trimmomatic v.0.38 (Bolger et al., 2014) (minimum read length 20 and average base quality 20 in 4-base sliding windows) and quality control with FastQC v.0.11.7 (Andrews, 2010) and MultiQC v1.0 (Ewels et al., 2016). Filtered reads were mapped to the inferred MTBC ancestral strain (Comas et al., 2010) with bwa-mem (Li, 2013) and converted to BAM files with samtools v.1.3.1 (Li et al., 2009). Duplicated reads were removed with Picard MarkDuplicates v.2.18.14 (Broad Institute, 2018). Genomes with more than 5% of missing sites were excluded. High confidence single nucleotide polymorphisms and insertions/deletions were called with Pilon (Walker et al., 2014) v.1.22 (minimum depth of five valid read pairs, minimum mapping quality 20 and minimum base quality 20) and filtered with bcftools v.1.3.1 (Li, 2011) (keeping only biallelic sites with variant frequency ≥ 75% and with a minimum distance from indels of four nucleotides). Genomic variants were annotated with SnpEff (Cingolani et al., 2012) M. tuberculosis H37Rv reference genome annotation (NC_000962.3; GCF_000195955.2). To minimize false positives, previously identified repetitive genomic regions, mobile elements and genes containing ≥ 50 bp nucleotide chunks identical to other parts of the genome (Coscolla et al., 2015; Koch et al., 2017) were not considered to further analysis. Raw reads were also analyzed using TBProfiler v.2.8.12 with the database from 2nd of April of 2020.
Phylogenetic Analysis
The high-confidence polymorphisms identified for each isolate were inputted into sequences from the inferred MTBC ancestral using bcftools v.1.3.1 (Li, 2011) consensus. The resulting whole-genome sequences were aligned using MAFFT v.7 (Katoh and Standley, 2013). Phylogenetic analysis was performed from the obtained multiple sequence alignment using IQ-TREE v.1.5.5 (Nguyen et al., 2015). The model TVM + R2 was used since it was the best-fitting model according to AIC, as determined by ModelFinder (Kalyaanamoorthy et al., 2017). Ancestral state reconstruction was done on the obtained rooted tree with log likelihood restricted maximum a posteriori (MAP) using the binary classification of each sequence as STR resistant or susceptible following the results from the performed phenotypic tests. The visual representations of the trees were done with FigTree v.1.4.3 (Rambaut, 2009) or PastML v.1.9.24 (Ishikawa et al., 2019). The transmission clusters were defined using a threshold of 12 or less pairwise SNPs between sequences as previously defined (Walker et al., 2013). The minimum spanning tree was performed with Phyloviz (Nascimento et al., 2017).
Statistical Analysis
Data from culture and drug susceptibility assays were analyzed using GraphPad Prism software, version 8.1.0 and checking for normality and log normality. Student’s t-test was used to determine differences between two different groups and One-way ANOVA for more than two groups. Tests were applied as referred in figure legends. To compare the proportions of genomic variants in STR resistant isolates and STR susceptible isolates, Fisher exact test was performed with the fisher.test command R 3.6.3 (R Core Team, 2014), followed by Bonferroni correction. Differences were considered significant for p ≤ 0.05 and represented as follows: ∗p < 0.05; ∗∗p < 0.01; ∗∗∗p < 0.001; and ****p < 0.0001.
Results
Characterization of the STR Resistance Prevalence in the Study Cohort
A phenotypic drug susceptibility test for INH, RIF, STR, ETH, and PZA was performed in the cohort as part of the routine diagnostic procedure. The analysis shows that 88.1% (n = 600) of the M. tuberculosis isolates in the cohort were susceptible to all tested antibiotics, while 9.0% (n = 61) of the isolates were resistant to only one antibiotic and 2.9% (n = 20) displayed resistance to more than one drug (Figure 1A). STR resistance accounted for 82.7% (n = 67) of the DR M. tuberculosis isolates, being present in 80.3% (n = 49) of the monoresistant isolates (Figures 1A,B). This was followed by monoresistance to INH, with 9.8% (n = 6) and by RIF, PZA, or ETH with 3.3% (n = 2) (Figures 1A,B). Furthermore, 90% (n = 18) of the polyresistant isolates also harbored STR resistance (Figure 1A). Finally, 15% (n = 3) of the polyresistant isolates were MDR strains, two of which harbored STR resistance (Figure 1B). These results are in line with a previous report in Western-European TB cohorts showing STR resistance as the dominant resistance with approximately 45.8% (n = 33/72), followed by INH resistance with 25% (n = 18/72) (Manson et al., 2017).
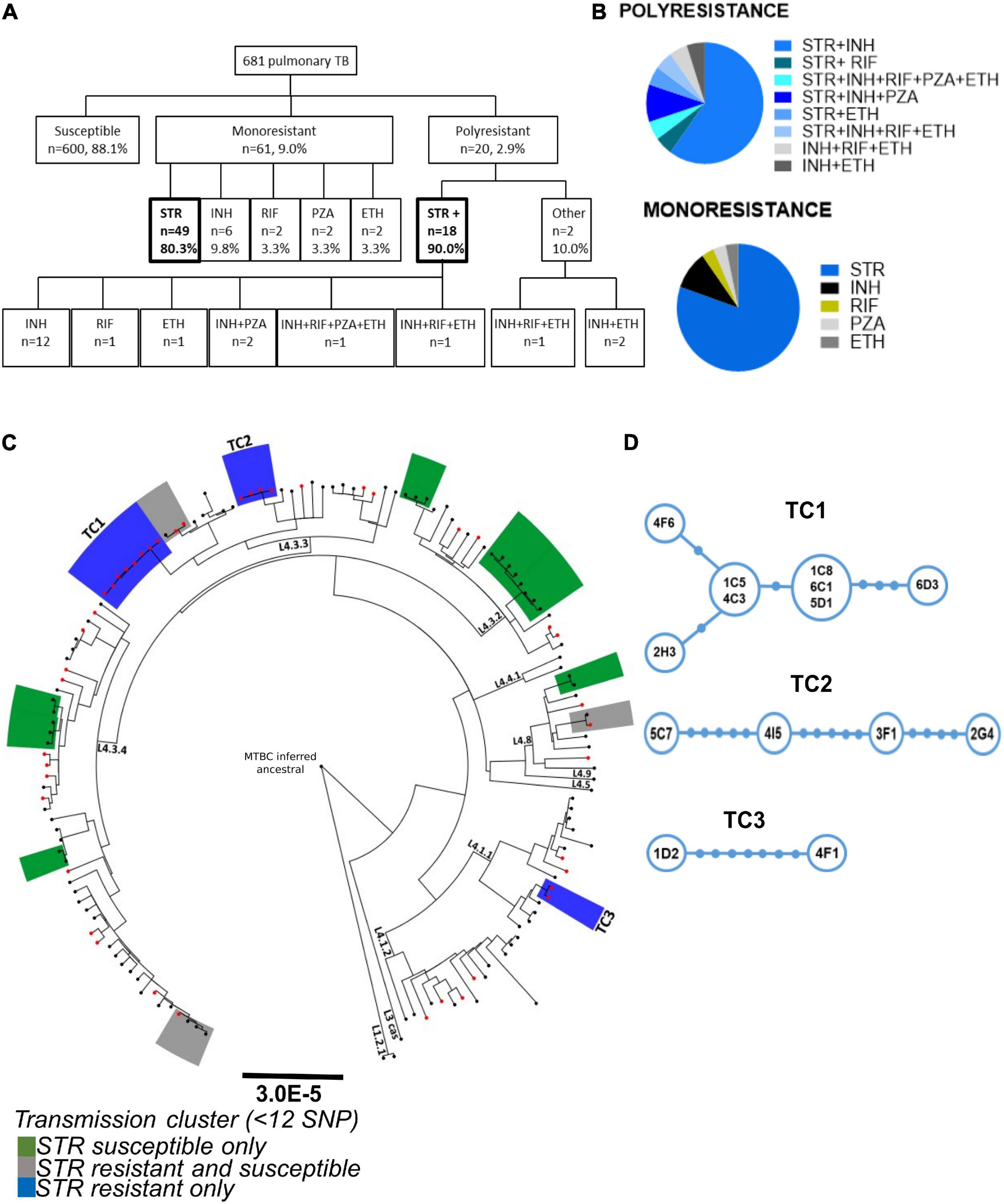
Figure 1. Characterization of the prevalence and transmission of STR resistance in the study cohort. (A) Data from the drug susceptibility test of 681 pulmonary TB patients from the São João Hospital cohort (CHSJ). Susceptible refers to strains which displayed no growth in the drug-susceptibility test; monoresistance was defined as resistance exclusively to one of the anti-tuberculosis drugs tested; polyresistance corresponds to resistance to two or more anti-tuberculosis drugs, but not both isoniazid and rifampicin simultaneously; multidrug-resistance (MDR), resistance to isoniazid and rifampicin simultaneously, and/or other anti-TB drugs tested. Boxes in bold highlight the number/percentage of the monoresistant STR resistant isolates and of the polyresistance including STR resistance. STR+, resistance to streptomycin and other(s) anti-TB drug; Other, polyresistance which does not include the STR resistance. STR, streptomycin; INH, isoniazid; RIF, rifampicin; PZA, pyrazinamide; ETH, ethambutol. (B) Pie charts with the percentage of monoresistance and polyresistance of the cohort. (C) Phylogeny and transmission clusters of M. tuberculosis isolates from the CHSJ. Boxes highlighted in blue, green, and gray represent the transmission clusters. Blue, STR resistant isolates only (TC1, TC2, and TC3); green: represent clusters with STR susceptible isolates only and gray, with STR resistant and susceptible isolates. The black dots and red dots indicate the STR susceptible isolates and the STR resistant isolates, respectively. (D) Minimum Spanning Tree representation of transmission clusters, TC1, TC2, and TC3. Blue circles represent a node of M. tuberculosis isolates. The size of the circle is proportional to the number of strains represented. Filled blue circles in the connections indicate the number of SNPs between the nodes.
Evidence for Ongoing Transmission of STR Resistant Mycobacterium tuberculosis
The high number of STR resistant M. tuberculosis isolates found in this and other cohorts, in which STR is not used presently as first line TB treatment, argues in favor of transmission of these isolates in the population. To investigate this, we inspected the transmission profile of the STR resistant M. tuberculosis isolates on the cohort. The whole genomes of 149 M. tuberculosis clinical isolates (45 STR resistant strains and a set of 104 randomly selected STR susceptible isolates) were sequenced and a phylogenetic analysis was performed. By defining transmission clusters using a cutoff of 12 or fewer single nucleotide polymorphisms (SNPs) of distance (Walker et al., 2013), we identified a total of 13 transmission clusters (Figure 1C). Most of the transmission clusters (10 of 13) were composed of strains from L4 sublineage LAM (4.3), the most prevalent in this cohort in agreement with our previous study (Sousa et al., 2020). Three transmission clusters were entirely composed of STR resistant isolates, three included both STR resistant and susceptible isolates and seven contained susceptible isolates only (Figure 1C). The isolates in the STR resistant transmission clusters (TC1-3) showed pairwise distances varying from one to seven SNPs (Figure 1D). TC1 is the cluster with the highest number of strains (n = 8) and the one harboring the lowest maximum pairwise genetic distance (five SNPs; Figure 1D). These data strongly support that at least certain STR resistant M. tuberculosis strains are being transmitted in the studied cohort, thus contributing to the maintenance of this DR phenotype in the population.
Heterogeneous Growth Rates in the Presence of STR Among Mycobacterium tuberculosis Isolates From the Same Transmission Cluster
Given that the STR resistant isolates from TC1-3 were likely being transmitted in the population, we hypothesized that these isolates had unimpaired fitness. Since in vitro growth capacity is a commonly used surrogate for bacterial fitness (Castro et al., 2020; Degiacomi et al., 2020), we compared the in vitro growth profiles of the M. tuberculosis isolates in TC1-3 to those of a group of susceptible isolates from the same TB cohort (Figure 2A). M. tuberculosis isolates in TC1-3 presented lower times to positivity than the STR susceptible isolates, but similar growth rates and doubling times (Figure 2B). We next evaluated the in vitro growth profile of M. tuberculosis isolates in TC1, as this cluster included a higher number of STR resistant isolates, in the presence of two doses of STR: 1.0 or 10.0 mg/L. Despite the high genetic relatedness between the isolates in TC1, the addition of STR to the culture medium resulted in distinct growth profiles across the tested isolates. Isolate 2H3 showed the highest STR resistance level, displaying a growth profile that was independent of the dose of STR used (Figure 3A). Isolates 6C1 and 5D1 grew at both concentrations of STR but displayed a delayed growth with significantly higher times to positivity, reduced growth rates and increased doubling times in the presence of 10.0 mg/L of STR (Figures 3A,B). Finally, isolates 6D3, 4F6, 1C5, 4C3, and 1C8 did not grow at the highest STR dose, showing altered growth rates and doubling times in the presence of 1.0 mg/L of STR (Figures 3A,B).
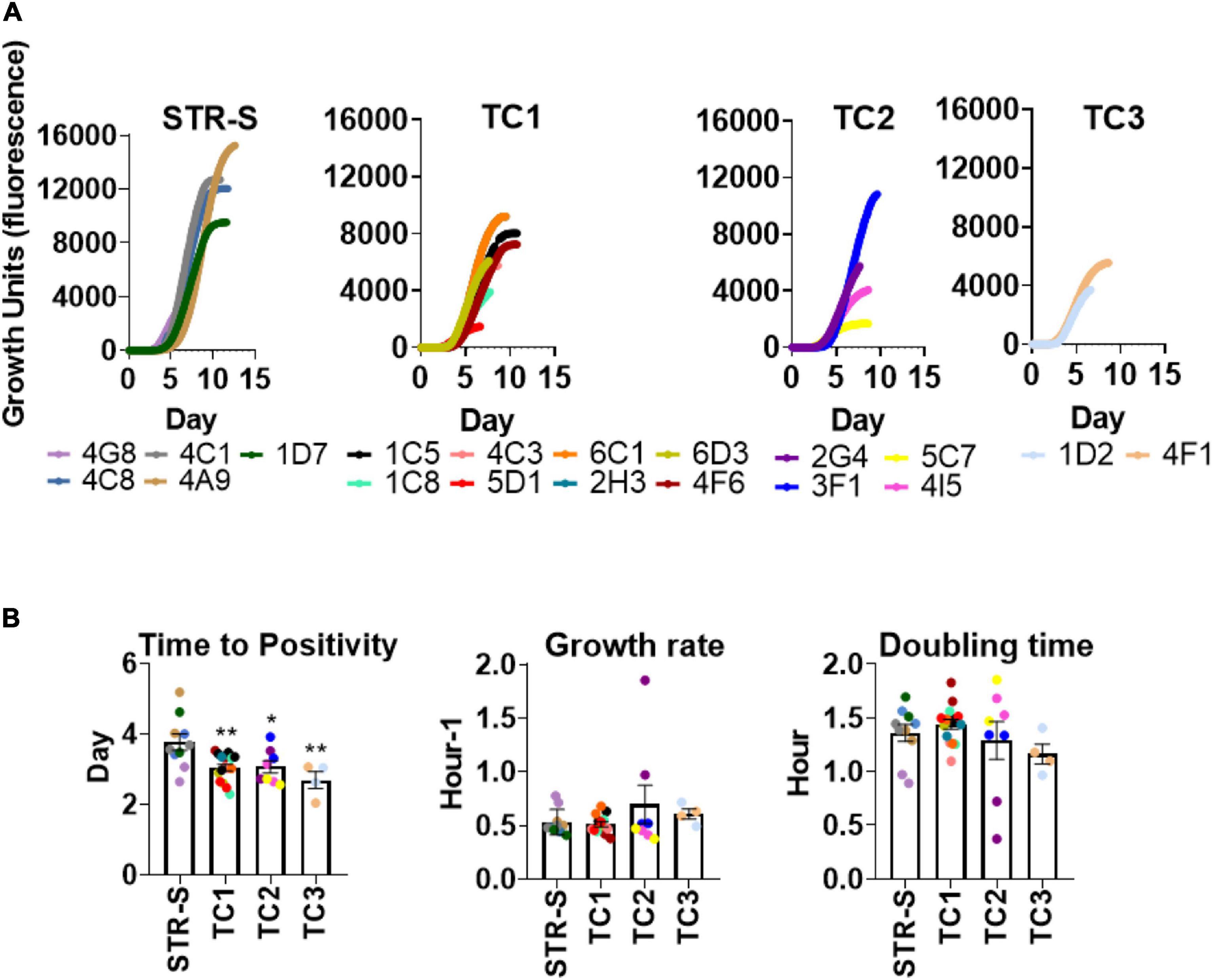
Figure 2. In vitro growth comparison of STR resistant and susceptible Mycobacterium tuberculosis clinical isolates. (A) Growth of M. tuberculosis isolates in MGITTM 960 tubes. Each curve illustrates the average growth of the isolate inoculated in the MGIT culture media, two replicates were included. STR-S, streptomycin susceptible isolates; TC1: T. cluster 1; TC2: T. cluster 2 and TC3: T. cluster 3. (B) Comparison of time to positivity, growth rate, and doubling time of different clinical isolates. Data represent the mean and SEM values (n = 2 for each strain). Statistical analysis was performed using one-way ANOVA (*p < 0.05 and**p < 0.01).
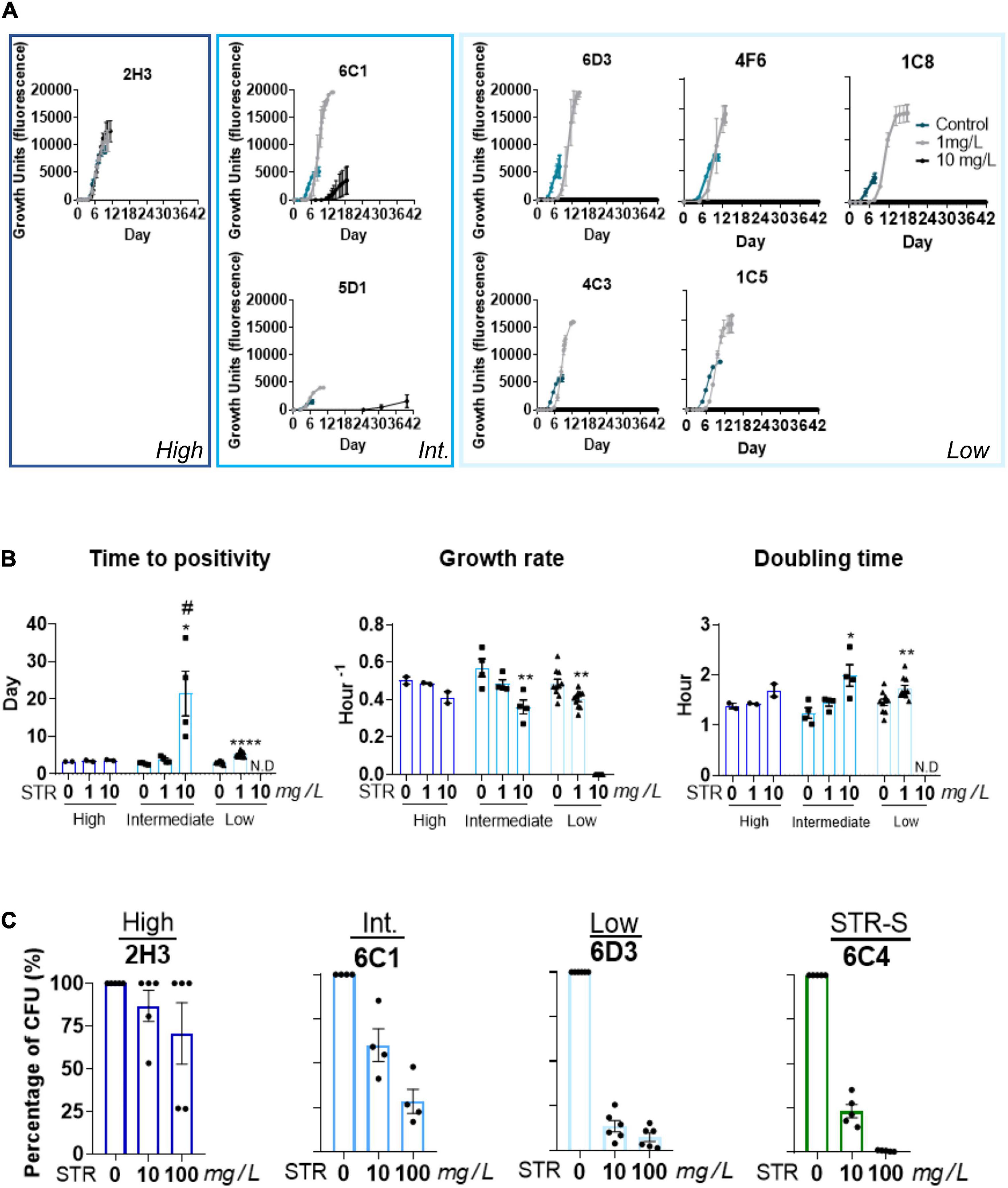
Figure 3. Heterogeneous growth rates in the presence of STR among strains from the same transmission cluster. (A) Comparison of the growth of M. tuberculosis isolates within TC1 (2H3, 6C1, 5D1, 6D3, 4F6, ICS, 4C3, and 1C5), in absence or presence of STR. The concentrations used were 1.0 mg/L. (gray curve) and 10.0 mg/L (black curve) of STR. The condition without STR was used as the control, represented with a blue curve. (B) Comparison of the growth parameters, time to positivity; growth rate, and doubling time of the strains with different STR resistance levels. Low STR resistance level (light blue) intermediate STR resistance level (blue), and high STR resistance level (dark blue). Time to positivity: the time needed to achieve the threshold of 75 GU in the BACTEC MGIT 960 system, growth rate of each isolate, doubling time: the time required for the bacteria density duplicate. The growth rates and doubling times were calculated in the exponential phase of growth. N.D means not detected. The error bar represents SEM values (n = 2 for each isolate). Statistical analysis was performed using Student’s t-test or one-way ANOVA. Significant statistical differences comparing 0 mg/L of STR to the other conditions are represented by *, and to 1 mg/L of STR by #(# or, *p < 0.05; **p < 0.01; ***p < 0.001; and ****p < 0.0001). (C) Percentage of survival of the intracellular bacteria after incubation with 10.0 or 100.0 mg/L of STR. The intracellular bacteria were quantified by CFU enumeration six days post-infection. Representation of the TC1. STR resistant strains with high STR resistance level (dark-blue), STR resistant isolates with intermediate resistance level (blue), STR resistant isolate with low resistance level (light-blue). STR-S refers to STR susceptible isolate (green).
We next questioned whether these differences in STR resistance were only seen in in vitro axenic medium, or if they prevailed during macrophage infection. For that, mouse BMDM were infected with isolates 2H3, 6C1, or 6D3 showing, respectively, high, intermediate or low STR resistance levels, and the intracellular bacterial growth quantified by CFU enumeration 6 days post-infection. A STR susceptible isolate (6C4) was included as a control. Similar findings were observed upon infection of BMDM and MGIT in vitro growth. Whereas the isolate 2H3 grew intracellularly at all tested concentrations (Figure 3C), isolate 6C1 grew at both concentrations of STR, but showed growth reduction as compared with the condition without STR (Figure 3C). This reduction was more pronounced at 100.0 mg/L of antibiotic. In turn, isolate 6D3 showed significant growth impairment in the presence of both concentrations of the antibiotic (Figure 3C). As expected, the STR susceptible isolate grew normally only in the absence of antibiotic and presented approximately 65–85% growth reduction on bacterial load in the presence of 10.0 mg/L of STR and 99% growth reduction at 100.0 mg/L of STR (Figure 3C). The internal bacterial load of each isolate without STR is represented in the Supplementary Figure 1.
Overall, these findings suggest that the M. tuberculosis isolates within TC1 have similar in vitro growth capacity, but different STR susceptibility levels which are observed both in axenic medium and during intracellular macrophage infection.
Mycobacterium tuberculosis Isolates Found in Transmission Clusters Harbor Novel STR Resistance Mutations
To investigate the genetic bases of STR resistance we started by analyzing the whole genomes of the 149 M. tuberculosis clinical isolates using TBProfiler (Phelan et al., 2019). The results corroborated only part of the results of the phenotypic drug susceptibility tests (Supplementary Table 1). Six STR resistant M. tuberculosis clinical isolates, not found in transmission clusters, harbored described STR resistance mutations (rpsL p.Lys-88→Gln, rpsL p.Lys-43→Arg, or rrs r.514 a→t) and were detected by both methods. However, the clinical isolates belonging to TC1-3 were incorrectly classified by TBProfiler as susceptible (Supplementary Table 1), suggesting unknown genetic bases for STR resistance. Thus, we performed whole-genome analysis to compare all SNPs and indels between the 45 STR resistant and the 104 STR susceptible isolates. We found a total of 11,516 high confidence polymorphisms (SNPs or indels, Supplementary Figure 2). These included 1,693 polymorphisms that were exclusively found in STR resistant isolates but a statistical association to STR resistance was not found (Supplementary Table 2). This was likely explained by the dispersion in different underlying genetic basis for the multiple introductions of STR resistance in the study cohort. Indeed, the ancestral state reconstruction of STR resistance in the study cohort suggests 30 independent events of STR resistance acquisition that were likely associated with different mutations including several novel gid mutations found only on STR resistant isolates (Figure 4). Also, some genetic variants previously associated with low level STR resistance, including gid (p.Val-124→Gly) (Bouziane et al., 2019), were in fact found in our cohort in both STR susceptible and resistant M. tuberculosis isolates and are unlikely to account to STR resistance. To further characterize possible candidate polymorphisms of drug resistance we selected all polymorphisms that were in previously described M. tuberculosis DR genes (Supplementary Figure 2). This allowed narrowing to 75 polymorphisms that were exclusively present in resistant isolates (Supplementary Table 3). Among these SNPs and indels, the ones found in inferred transmission clusters entirely composed of STR resistant isolates (TC1-3) were heterogeneous (Figure 5A). Notably, TC1 shared three non-synonymous SNPs (nsSNP) on Cytochrome p450 (p.Ile-359→Thr, p.His-353→Arg, and p.Ile-344→Val in cyp125, cyp126, and cyp130, respectively), one nsSNP in gid (p.Ile-11→Thr) and one nsSNP on a probable conserved transporter from the Major Facilitator Superfamily (p.Arg-798→Cys, Rv3728). The isolates from TC2 shared three nsSNPs (gid p.Cys-191→Trp, dnaE1 p.Ser-4Pro, and panB p.Gly-211→Ser). Finally, in TC3 all isolates shared two nsSNPs (gid p.Val-112Gly and devS p.Leu-381Phe). The mutations found in gid (p.Ile-11→Thr, p.Cys-191→Trp and p.Val-112→Gly) were most likely underlying STR resistance in TC1-3 although a contribution of other mutations shared in the cluster and not present in susceptible clinical isolates could not be excluded.
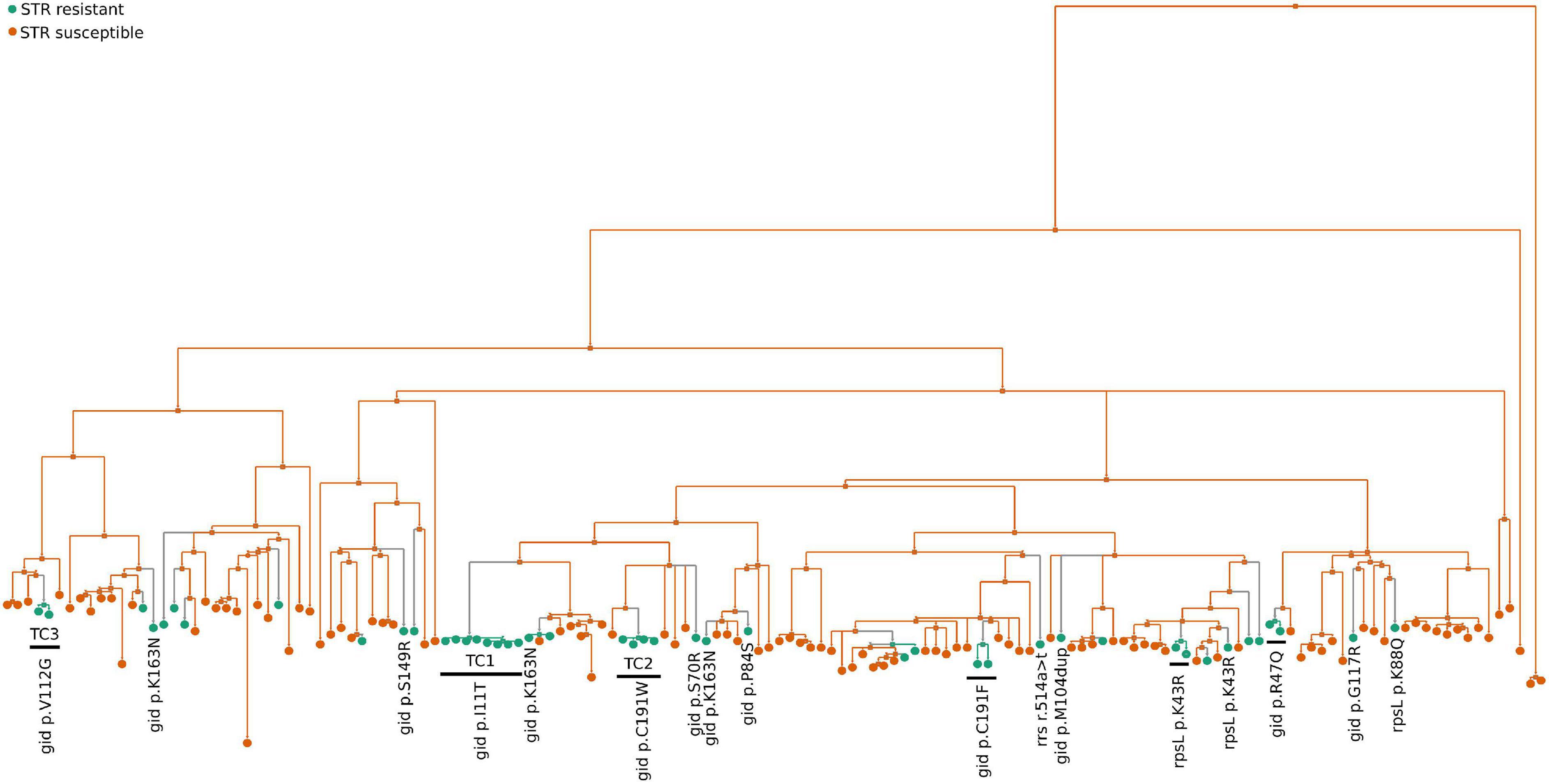
Figure 4. Ancestral state reconstruction (ASR) of STR resistant M. tuberculosis isolates. ASR was done using log likelihood restricted maximum a posteriori (MAP) analysis of the phylogenetic tree with the binary classification of each sequence as STR resistant (green circles) or susceptible (orange circles) following the results from the phenotypic tests. Previously known rrs and rpsL STR resistance mutations (rrs r.514a>t, rpsL p.Lys43Arg and p.Lys88Gln) and gid mutations (gid p.Arg47Gln, p.Ser70Arg, p.Pro84Ser, p.Met104dup, p.Va1112Gly, p.Ser149Arg, p.Gly117Arg and p.Lys163Asn) that exclusively present in STR resistant isolates are indicated.
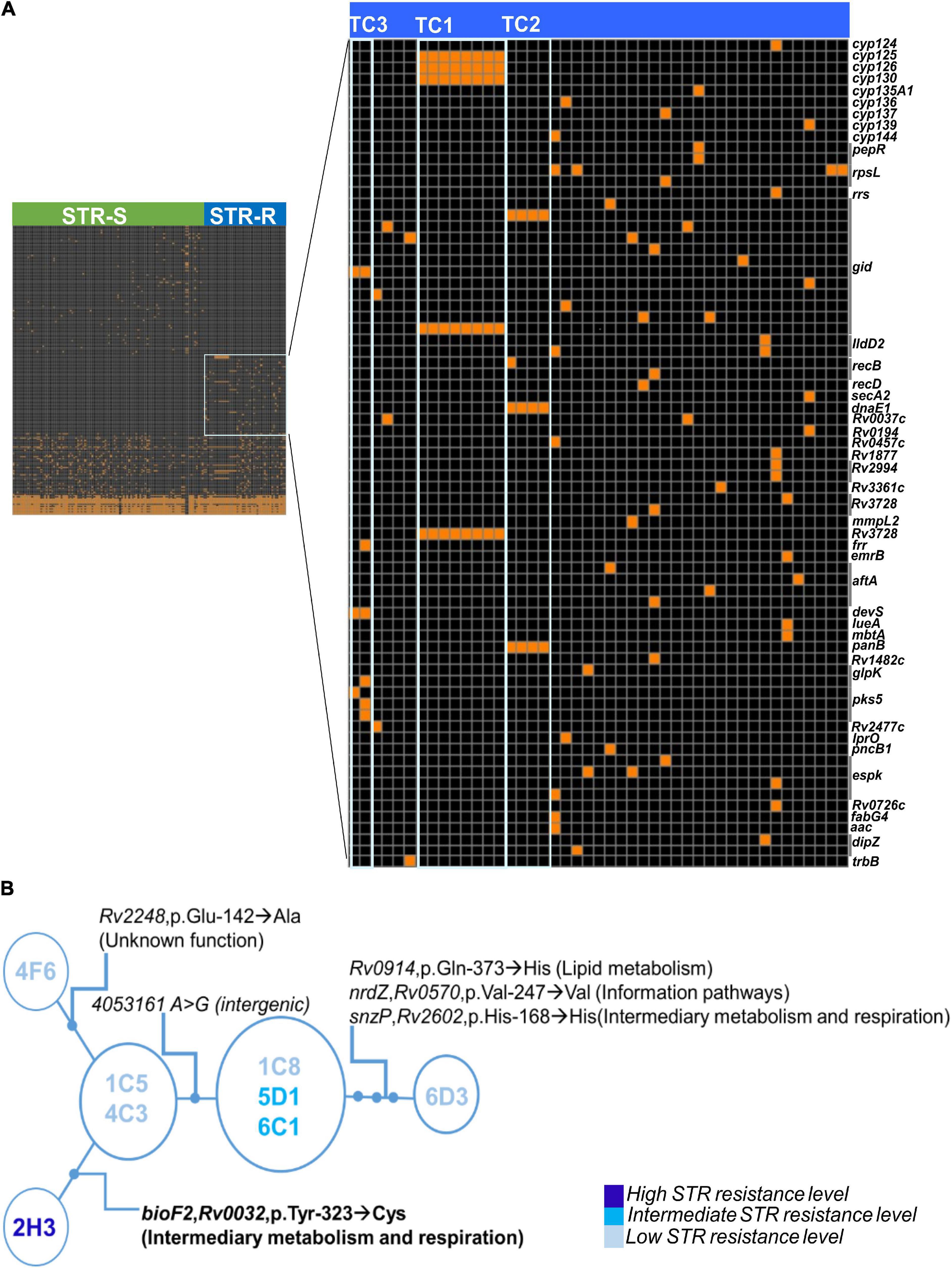
Figure 5. Whole-genome sequencing analysis unveils novel candidate STR resistance mutations. (A) Genome-wide analysis of 149 M. tuberculosis clinical isolates, 104 STR susceptible and 45 STR resistant strains. The header symbolizes the M. tuberculosis isolates, green represent the STR susceptible isolates (STR-S) and blue STR resistant isolates (STR-R). The right axis includes 150 polymorphisms. The black area of the heat map corresponds to the absence of polymorphisms and the orange squares are shown when the polymorphism (SNP or Indel) is found in the different isolates. The area highlighted with a square in light-blue shows the polymorphisms identified exclusively in STR resistant isolates. In the heat map with the STR resistant exclusive polymorphisms, gray line group the polymorphisms presented in the same gene, size of the line depends on the number of SNPs or Indels. Polymorphisms of TC1, TC2, and TC3 are highlighted in the heat map with the light blue boxes. (B) Minimum Spanning Tree Representation of cluster TC1 clinical isolates indicating the polymorphisms separating the nodes and the level of STR resistance of each isolate. SNP = single nucleotide polymorphism and Indels = insertions or deletions.
We next investigated genetic variants that might impact on the different STR resistance levels within TC1 (Figure 5B). The TC1 isolate with the highest level of STR resistance (2H3) had one nsSNP (p.Tyr-323→Cys) in the gene bioF2 that was not present in the other TC1 isolates. The gene bioF2 (Rv0032) is likely involved in biotin biosynthesis. bioF2 p.Tyr-323→Cys was not found in any other isolates of the cohort, but a nsSNP in an adjacent position of bioF2 (p.Ile-322→Val) was present in another STR resistant isolate of the cohort (4E9). These substitutions were not included in known bioF2 functional sites. We then investigated the presence of these bioF2 SNPs in an unrelated database of genomes from 1,450 laboratory confirmed STR resistant clinical isolates and 1,450 susceptible strains from PATRIC (Wattam et al., 2014). The SNPs p.Tyr-323→Cys and p.Ile-322→Val in bioF2 were not found in any of the queried sequences. The remaining polymorphisms defining the isolates of TC1 were identified in genes with different categorical functions, such as lipid metabolism, cell wall and cell process, or unknown hypothetical proteins (Figure 5B). Except for the bioF2 nsSNP none of the other SNPs matched the phenotypic differences in STR resistance found among the TC1 strains (Figure 5B). Indeed, isolates 1C8, 5D1 and 6C1 showed mild differences in growth in the presence of STR and did not harbor any high confidence polymorphisms of difference [minimum depth ≥ 5, minimum quality 20, variant frequency ≥ 75% and not located on repetitive genomic regions or mobile elements (Coscolla et al., 2015; Koch et al., 2017)]. Thus, we next analyzed polymorphisms with lower variant frequency (<75% of the reads) to investigate less stable mutations. The analysis highlighted a possible mutation in 1C8 in the homopolymeric tract of seven cytosines of the glpK gene, a result that was supported by PCR amplification and resequencing of the samples by the Sanger method (Supplementary Figure 3). In all the studied isolates this mutation was found in two isolates with low STR resistance from TC1 (1C8, 6D3) and two STR susceptible genome sequences (4H7, 5D4). Further studies are needed to investigate if mutations in glpK could be related to the observed phenotypes. Collectively, our results highlight novel candidate genetic variants for STR resistance.
Discussion
Recent data reinforces that transmission of DR strains evolving from mono- to multi-antimicrobial resistance phenotypes fuels the threat of antimicrobial resistance (Al-Mutairi et al., 2019; Becerra et al., 2019; Khan et al., 2019; Knight et al., 2019). Elusively, STR resistance remains the most common type of resistance found in M. tuberculosis clinical isolates collected from several parts of the world (Manson et al., 2017), even though the use of STR in TB treatment was demoted.
Development of STR resistance in M. tuberculosis is often associated with mutations in rpsL, rrs, and gid genes (Okamoto et al., 2007; Jagielski et al., 2014; Khosravi et al., 2017; Islam et al., 2020; Shrestha et al., 2020). The type of DR mutation might associate with the M. tuberculosis lineage and influence the resistance level and fitness (Spies et al., 2011; Ballif et al., 2012; Miotto et al., 2017; Macedo et al., 2018; Sun et al., 2018). The relative fitness of drug resistant vs. susceptible strains has been highlighted as a key factor driving the transmission of DR M. tuberculosis (Ballif et al., 2012; Morcillo et al., 2014; Knight et al., 2015). In agreement, we found no growth defect in the STR resistant clinical isolates belonging to the inferred transmission clusters suggesting a normal fitness and likelihood of transmission. Interestingly, the clinical isolates transmitted in this cohort harbored several novel candidate STR resistance mutations in gid shared by the isolates in each transmission cluster and exclusive to STR resistant clinical isolates (p.Ile-11→Thr, p.Cys-191→Trp, and p.Val-112→Gly). The substitution p.Ile-11→Asn in gid was previously associated to STR resistance (Walker et al., 2015; Coll et al., 2018). As Asn and Thr are uncharged polar side chain amino acids the mutation gid p.Ile-11→Thr is likely a novel STR resistance determinant. Furthermore, we found genetic variants in Rv3728 and Cytochrome p450, both previously associated with antimicrobial resistance phenotypes (Raman and Chandra, 2008; Gupta et al., 2010; Kanji et al., 2017, 2019). It will be interesting to in the future further address all these novel candidates at the functional level.
Within the largest transmission cluster of STR resistant isolates (TC1), we found differences in growth in the presence of STR suggesting a continuing process of adaptation. Interestingly, isolates 6C1, 5D1 and 1C8 were genetically indistinguishable in the sense that they were not separated by any high confidence genetic variant. Yet, whether isolates 6C1 and 5D1 showed delayed growth in the presence of a high (10.0 mg/L) STR concentration, isolate 1C8 did not grow at this concentration of the drug. These findings suggest that the phenotype observed in 6C1 and 5D1, and not in 1C8, might be drug tolerance associated with recent or transient genetic variants within the isolates (Wallis et al., 1999; Torrey et al., 2016; Vargas and Farhat, 2020). Drug tolerance mechanisms are elusive, challenging to study and prone to technical- and culture-related artifacts (Lee et al., 2019). Our analysis of low frequency variants in NGS data and Sanger re-sequencing support that 1C8 differs from 6C1 and 5D1 by having one additional cytosine in the homopolymeric cytosine tract of the glpK gene. This polymorphism was found in four isolates from the studied cohort including two with low STR resistance. Nonetheless, it was also found in two STR susceptible isolates. Of note, the possible role of glpK in M. tuberculosis drug resistance has been a topic of recent debate (Bellerose et al., 2019; Safi et al., 2019, 2020; Vargas and Farhat, 2020). It is thus tempting to speculate that changes homopolymeric cytosine tract of glpK may be related to the observed phenotype of low-to-intermediate STR resistance, a hypothesis that will need to be further addressed in future studies (Bellerose et al., 2019; Safi et al., 2019, 2020; Vargas and Farhat, 2020).
The highest level of STR resistance was found in the isolate 2H3 that diverged from the other TC1 isolates by harboring one mutation in the gene bioF2 (p.Tyr-323→Cys). Although a SNP in an adjacent position of bioF2 (p.Ile-322→Val) was found in another STR resistant isolate (4E9) from the studied cohort, both genetic variants seem to be rare in unrelated cohorts. The gene bioF2 is likely involved in M. tuberculosis biotin biosynthesis (Salaemae et al., 2011). Mycobacteria rely primarily on de novo synthesis of biotin, an important micronutrient serving as an essential cofactor for several enzymes (Park et al., 2011; Salaemae et al., 2011). Consequently, affecting biotin biosynthesis might influence bacilli growth. Thus, bioF2 p.Tyr-323→Cys, and possibly also p.Ile-322→Val, might influence bioF2 function and modulate growth in the presence of high concentrations of STR. It will be relevant to test this hypothesis in future experiments using genetically engineered mutants targeting these bioF2 residues. Importantly, the phenotype of high STR resistance of the isolate 2H3 found in in vitro cultures was maintained when using the infection model of mouse BMDM in which the bacilli grow within the controlled intracellular microenvironment of the macrophage phagosome.
STR resistance has not been perceived as a major clinical threat since this antibiotic was replaced in first line TB treatment by more efficient combinations of other antimicrobials. However, the hypothesis that STR might predispose to MDR was previously suggested (Buu et al., 2012; Manson et al., 2017; Holt et al., 2018; Bouziane et al., 2019). The mechanisms underlying this process remain elusive, but likely involve secondary mutations that compensate the fitness cost of resistance to more than one drug. M. tuberculosis isolates like the ones herein studied probably have the same likelihood to be transmitted as susceptible strains but could represent an additional threat regarding the development of high level STR resistance or even resistance to other drugs. If this hypothesis proves correct, it is of great relevance to survey and increase the efforts to control the transmission of STR resistant strains. In this context, our findings raise concerns on the potential inaccuracy of molecular diagnostic methods to predict STR resistance and the necessity to combine phenotypic and genotypic analysis to generate more comprehensive DR mutation databases. Also, our results propose the modulation of biotin biosynthesis as a novel putative compensatory mechanism for STR resistance.
Data Availability Statement
The datasets presented in this study can be found in online repositories. The names of the repository/repositories and accession number(s) can be found below: NCBI sequence read archive, SRR13591335-13591460 and European Nucleotide Archive, ERR1193734-1465744.
Author Contributions
DR, CM, and BC performed experiments. AR, TC, JG, HB, and IC provided essential resources for the study and discussed the manuscript. DR, CM, MS, and NSO analyzed the data. DR prepared the figures, tables, and drafted the manuscript. MS and NSO supervised the study, planned the experiments, and wrote the final version of the manuscript. All authors read and approved the final manuscript.
Funding
This work has been funded by of the projects NORTE-01-0145-FEDER-000039, NORTE-01-0145-FEDER-000013, and NORTE-01-0145-FEDER-00002, supported by Norte Portugal Regional Operational Program (NORTE 2020), under the PORTUGAL 2020 Partnership Agreement, through the European Regional Development Fund (ERDF). It was also funded through the Foundation for Science and Technology (FCT) – project FCT IF/00474/2014, UIDB/50026/2020 and UIDP/50026/2020. DR and CM were funded by FCT Ph.D. scholarships (grant numbers SFRH/BD/135422/2017 and SFRH/BD/132797/2017, respectively). DR was partially funded by the PGCD – Graduate Program Science for Development. MS is supported by FCT through the Estimulo ao Emprego Científico.
Conflict of Interest
The authors declare that the research was conducted in the absence of any commercial or financial relationships that could be construed as a potential conflict of interest.
Supplementary Material
The Supplementary Material for this article can be found online at: https://www.frontiersin.org/articles/10.3389/fmicb.2021.659545/full#supplementary-material
References
Al-Mutairi, N. M., Ahmad, S., and Mokaddas, E. M. (2019). Molecular characterization of multidrug-resistant Mycobacterium tuberculosis (MDR-TB) isolates identifies local transmission of infection in Kuwait, a country with a low incidence of TB and MDR-TB. Eur. J. Med. Res. 24:38. doi: 10.1186/s40001-019-0397-2
Andrews, S. (2010). FASTQC. A quality control tool for high throughput sequence data. Cambrige, Uk. Babraham Institute.
Ballif, M., Harino, P., Ley, S., Coscolla, M., Niemann, S., Carter, R., et al. (2012). Drug resistance-conferring mutations in Mycobacterium tuberculosis from Madang, Papua New Guinea. BMC Microbiol. 12:191. doi: 10.1186/1471-2180-12-191
Bastos, H. N., Osório, N. S., Castro, A. G., Ramos, A., Carvalho, T., Meira, L., et al. (2016). A prediction rule to stratify mortality risk of patients with pulmonary tuberculosis. PLoS One 11:e162797. doi: 10.1371/journal.pone.0162797
Becerra, M. C., Huang, C. C., Lecca, L., Bayona, J., Contreras, C., Calderon, R., et al. (2019). Transmissibility and potential for disease progression of drug resistant Mycobacterium tuberculosis: Prospective cohort study. BMJ 367:l5894. doi: 10.1136/bmj.l5894
Bellerose, M. M., Baek, S. H., Huang, C. C., Moss, C. E., Koh, E. I., Proulx, M. K., et al. (2019). Common variants in the glycerol kinase gene reduce tuberculosis drug efficacy. MBio. 10:e00663–19. doi: 10.1128/mBio.00663-19
Bhatt, K., Machado, H., Osório, N. S., Sousa, J., Cardoso, F., Magalhães, C., et al. (2018). A Nonribosomal Peptide Synthase Gene Driving Virulence in Mycobacterium tuberculosis. mSphere 3, 1–14. doi: 10.1128/mSphere.00352-18
Bolger, A. M., Lohse, M., and Usadel, B. (2014). Trimmomatic: A flexible trimmer for Illumina sequence data. Bioinformatics 30, 2114–2120. doi: 10.1093/bioinformatics/btu170
Bouziane, F., Allem, R., Sebaihia, M., Kumanski, S., Mougari, F., Sougakoff, W., et al. (2019). First genetic characterisation of multidrug-resistant Mycobacterium tuberculosis isolates from Algeria. J. Glob. Antimicrob. Resist. 19, 301–307. doi: 10.1016/j.jgar.2019.05.010
Buu, T. N., van Soolingen, D., Huyen, M. N. T., Lan, N. T. N., Quy, H. T., Tiemersma, E. W., et al. (2012). Increased transmission of Mycobacterium tuberculosis Beijing Genotype Strains associated with resistance to Streptomycin: a population-based study. PLoS One 7:e42323. doi: 10.1371/journal.pone.0042323
Castro, R. A. D., Ross, A., Kamwela, L., Reinhard, M., Loiseau, C., Feldmann, J., et al. (2020). The genetic background modulates the evolution of fluoroquinolone-resistance in Mycobacterium tuberculosis. Mol. Biol. Evol. 37, 195–207. doi: 10.1093/molbev/msz214
Cingolani, P., Platts, A., Wang, L. L., Coon, M., Nguyen, T., Wang, L., et al. (2012). A program for annotating and predicting the effects of single nucleotide polymorphisms, SnpEff: SNPs in the genome of Drosophila melanogaster strain w1118; iso-2; iso-3. Fly (Austin). 6, 80–92. doi: 10.4161/fly.19695
Cohen, K. A., Stott, K. E., Munsamy, V., Manson, A. L., Earl, A. M., and Pym, A. S. (2020). Evidence for expanding the role of streptomycin in the management of Drug-Resistant Mycobacterium tuberculosis. Antimicrob. Agents Chemother. 64:e00860–20. doi: 10.1128/AAC.00860-20
Coll, F., Phelan, J., Hill-Cawthorne, G. A., Nair, M. B., Mallard, K., Ali, S., et al. (2018). Genome-wide analysis of multi- and extensively drug-resistant Mycobacterium tuberculosis. Nat. Genet. 50, 307–316. doi: 10.1038/s41588-017-0029-0
Comas, I., Chakravartti, J., Small, P. M., Galagan, J., Niemann, S., Kremer, K., et al. (2010). Human T cell epitopes of Mycobacterium tuberculosis are evolutionarily hyperconserved. Nat. Genet. 42, 498–503.
Coscolla, M., Copin, R., Sutherland, J., Gehre, F., De Jong, B., Owolabi, O., et al. (2015). M. tuberculosis T cell epitope analysis reveals paucity of antigenic variation and identifies rare variable TB antigens. Cell Host Microbe 18, 538–548. doi: 10.1016/j.chom.2015.10.008
Crofton, J., and Mitchison, D. A. (1948). Streptomycin resistance in pulmonary tuberculosis. Br. Med. J. 2, 1009–1015. doi: 10.1136/bmj.2.4588.1009
Degiacomi, G., Sammartino, J. C., Sinigiani, V., Marra, P., Urbani, A., and Pasca, M. R. (2020). In vitro study of bedaquiline resistance in Mycobacterium tuberculosis Multi-drug resistant clinical isolates. Front. Microbiol. 11:559469. doi: 10.3389/fmicb.2020.559469
Ewels, P., Magnusson, M., Lundin, S., and Käller, M. (2016). MultiQC: Summarize analysis results for multiple tools and samples in a single report. Bioinformatics 32, 3047–3048. doi: 10.1093/bioinformatics/btw354
Glasauer, S., Altmann, D., Hauer, B., Brodhun, B., Haas, W., and Perumal, N. (2019). First-line tuberculosis drug resistance patterns and associated risk factors in Germany, 2008-2017. PLoS One 14:e217597. doi: 10.1371/journal.pone.0217597
Gupta, A. K., Katoch, V. M., Chauhan, D. S., Sharma, R., Singh, M., Venkatesan, K., et al. (2010). Microarray analysis of efflux pump genes in multidrug-resistant Mycobacterium tuberculosis during stress induced by common anti-tuberculous drugs. Microb. Drug Resist. 16, 21–28. doi: 10.1089/mdr.2009.0054
Hlaing, Y. M., Tongtawe, P., Tapchaisri, P., Thanongsaksrikul, J., Thawornwan, U., Archanachan, B., et al. (2017). Mutations in streptomycin resistance genes and their relationship to streptomycin resistance and lineage of Mycobacterium tuberculosis Thai isolates. Tuberc. Respir. Dis. (Seoul). 80:159. doi: 10.4046/trd.2017.80.2.159
Holt, K. E., McAdam, P., Thai, P. V. K., Thuong, N. T. T., Ha, D. T. M., Lan, N. N., et al. (2018). Frequent transmission of the Mycobacterium tuberculosis Beijing lineage and positive selection for the EsxW Beijing variant in Vietnam. Nat. Genet. 50, 849–856. doi: 10.1038/s41588-018-0117-9
Ishikawa, S. A., Zhukova, A., Iwasaki, W., Gascuel, O., and Pupko, T. (2019). A fast likelihood method to reconstruct and visualize ancestral scenarios. Mol. Biol. Evol. 36, 2069–2085. doi: 10.1093/molbev/msz131
Islam, M. M., Tan, Y., Hameed, H. M. A., Chhotaray, C., Liu, Z., Liu, Y., et al. (2020). Phenotypic and genotypic characterization of streptomycin-resistant multidrug-resistant Mycobacterium tuberculosis clinical isolates in Southern China. Microb. Drug Resist. 26, 766–775. doi: 10.1089/mdr.2019.0245
Jagielski, T., Ignatowska, H., Bakuła, Z., Dziewit, Ł, Napiórkowska, A., Augustynowicz-Kopeć, E., et al. (2014). Screening for streptomycin resistance-conferring mutations in Mycobacterium tuberculosis clinical isolates from Poland. PLoS One 9:e100078. doi: 10.1371/journal.pone.0100078
Kalyaanamoorthy, S., Minh, B. Q., Wong, T. K. F., Von Haeseler, A., and Jermiin, L. S. (2017). ModelFinder: Fast model selection for accurate phylogenetic estimates. Nat. Methods 14, 587–589. doi: 10.1038/nmeth.4285
Kanji, A., Hasan, R., Ali, A., Zaver, A., Zhang, Y., Imtiaz, K., et al. (2017). Single nucleotide polymorphisms in efflux pumps genes in extensively drug resistant Mycobacterium tuberculosis isolates from Pakistan. Tuberculosis 107, 20–30. doi: 10.1016/j.tube.2017.07.012
Kanji, A., Hasan, R., and Hasan, Z. (2019). Efflux pump as alternate mechanism for drug resistance in Mycobacterium tuberculosis. Indian J. Tuberc. 66, 20–25. doi: 10.1016/j.ijtb.2018.07.008
Katoh, K., and Standley, D. M. (2013). MAFFT multiple sequence alignment software version 7: Improvements in performance and usability. Mol. Biol. Evol. 30, 772–780. doi: 10.1093/molbev/mst010
Khan, P. Y., Yates, T. A., Osman, M., Warren, R. M., van der Heijden, Y., Padayatchi, N., et al. (2019). Transmission of drug-resistant tuberculosis in HIV-endemic settings. Lancet Infect. Dis. 19, e77–e88. doi: 10.1016/S1473-3099(18)30537-1
Khosravi, A. D., Etemad, N., Hashemzadeh, M., Khandan Dezfuli, S., and Goodarzi, H. (2017). Frequency of rrs and rpsL mutations in streptomycin-resistant Mycobacterium tuberculosis isolates from Iranian patients. J. Glob. Antimicrob. Resist. 9, 51–56. doi: 10.1016/j.jgar.2017.01.005
Knight, G. M., Colijn, C., Shrestha, S., Fofana, M., Cobelens, F., White, R. G., et al. (2015). The distribution of fitness costs of Resistance-Conferring Mutations Is a Key determinant for the future burden of drug-resistant tuberculosis: a model-based analysis. Clin. Infect. Dis. 61, S147–S154. doi: 10.1093/cid/civ579
Knight, G. M., McQuaid, C. F., Dodd, P. J., and Houben, R. M. G. J. (2019). Global burden of latent multidrug-resistant tuberculosis: trends and estimates based on mathematical modelling. Lancet Infect. Dis. 19, 903–912. doi: 10.1016/S1473-3099(19)30307-X
Koch, A. S., Brites, D., Stucki, D., Evans, J. C., Seldon, R., Heekes, A., et al. (2017). The influence of HIV on the evolution of Mycobacterium tuberculosis. Mol. Biol. Evol. 34, 1654–1668. doi: 10.1093/molbev/msx107
Lee, J. J., Lee, S. K., Song, N., Nathan, T. O., Swarts, B. M., Eum, S. Y., et al. (2019). Transient drug-tolerance and permanent drug-resistance rely on the trehalose-catalytic shift in Mycobacterium tuberculosis. Nat. Commun. 10:2928. doi: 10.1038/s41467-019-10975-7
Li, H. (2011). A statistical framework for SNP calling, mutation discovery, association mapping and population genetical parameter estimation from sequencing data. Bioinformatics 27, 2987–2993. doi: 10.1093/bioinformatics/btr509
Li, H. (2013). Aligning sequence reads, clone sequences and assembly contigs with BWA-MEM. arXiv 1–3.
Li, H., Handsaker, B., Wysoker, A., Fennell, T., Ruan, J., Homer, N., et al. (2009). The Sequence Alignment/Map format and SAMtools. Bioinformatics 25, 2078–2079. doi: 10.1093/bioinformatics/btp352
Macedo, R., Nunes, A., Portugal, I., Duarte, S., Vieira, L., and Gomes, J. P. (2018). Dissecting whole-genome sequencing-based online tools for predicting resistance in Mycobacterium tuberculosis: can we use them for clinical decision guidance? Tuberculosis 110, 44–51. doi: 10.1016/j.tube.2018.03.009
Manson, A. L., Cohen, K. A., Abeel, T., Desjardins, C. A., Armstrong, D. T., Barry, C. E., et al. (2017). Genomic analysis of globally diverse Mycobacterium tuberculosis strains provides insights into the emergence and spread of multidrug resistance. Nat. Genet. 49, 395–402. doi: 10.1038/ng.3767
Miotto, P., Tessema, B., Tagliani, E., Chindelevitch, L., Starks, A. M., Emerson, C., et al. (2017). A standardised method for interpreting the association between mutations and phenotypic drug resistance in Mycobacterium tuberculosis. Eur. Respir. J. 50:1701354. doi: 10.1183/13993003.01354-2017
Morcillo, N. S., Imperiale, B. R., Di Giulio, Á, Zumárraga, M. J., Takiff, H., and Cataldi, ÁA. (2014). Fitness of drug resistant Mycobacterium tuberculosis and the impact on the transmission among household contacts. Tuberculosis 94, 672–677. doi: 10.1016/j.tube.2014.08.003
Moreira-Teixeira, L., Sousa, J., McNab, F. W., Torrado, E., Cardoso, F., Machado, H., et al. (2016). Type I IFN inhibits alternative macrophage activation during Mycobacterium tuberculosis Infection and Leads to Enhanced Protection in the Absence of IFN-γ Signaling. J. Immunol. 197, 4714–4726. doi: 10.4049/jimmunol.1600584
Murray, J. F., Schraufnagel, D. E., and Hopewell, P. C. (2015). Treatment of tuberculosis: a historical perspective. Ann. Am. Thorac. Soc. 12, 1749–1759. doi: 10.1513/AnnalsATS.201509-632PS
Nascimento, M., Sousa, A., Ramirez, M., Francisco, A. P., Carriço, J. A., and Vaz, C. (2017). PHYLOViZ 2.0: providing scalable data integration and visualization for multiple phylogenetic inference methods. Bioinformatics 33, 128–129. doi: 10.1093/bioinformatics/btw582
Nguyen, L. T., Schmidt, H. A., Von Haeseler, A., and Minh, B. Q. (2015). IQ-TREE: A fast and effective stochastic algorithm for estimating maximum-likelihood phylogenies. Mol. Biol. Evol. 32, 268–274. doi: 10.1093/molbev/msu300
Nhu, N. T. Q., Lan, N. T. N., Phuong, N. T. N., Van, V., Chau, N., Farrar, J., et al. (2012). Association of streptomycin resistance mutations with level of drug resistance and Mycobacterium tuberculosis genotypes. Int. J. Tuberc. Lung Dis. 16, 527–531. doi: 10.5588/ijtld.11.0202
Okamoto, S., Tamaru, A., Nakajima, C., Nishimura, K., Tanaka, Y., Tokuyama, S., et al. (2007). Loss of a conserved 7-methylguanosine modification in 16S rRNA confers low-level streptomycin resistance in bacteria. Mol. Microbiol. 63, 1096–1106. doi: 10.1111/j.1365-2958.2006.05585.x
Oppong, Y. E. A., Phelan, J., Perdigão, J., Machado, D., Miranda, A., Portugal, I., et al. (2019). Genome-wide analysis of Mycobacterium tuberculosis polymorphisms reveals lineage-specific associations with drug resistance. BMC Genom. 20:252. doi: 10.1186/s12864-019-5615-3
Park, S., Klotzsche, M., Wilson, D. J., Boshoff, H. I., Eoh, H., Manjunatha, U., et al. (2011). Evaluating the sensitivity of Mycobacterium tuberculosis to biotin deprivation using regulated gene expression. PLoS Pathog. 7:e1002264. doi: 10.1371/journal.ppat.1002264
Perdigão, J., Macedo, R., Machado, D., Silva, C., Jordão, L., Couto, I., et al. (2014). GidB mutation as a phylogenetic marker for Q1 cluster Mycobacterium tuberculosis isolates and intermediate-level streptomycin resistance determinant in Lisbon, Portugal. Clin. Microbiol. Infect. 20, 278–284. doi: 10.1111/1469-0691.12392
Perdigão, J., Macedo, R., Silva, C., Machado, D., Couto, I., Viveiros, M., et al. (2013). From multidrug-resistant to extensively drug-resistant tuberculosis in Lisbon, Portugal: the stepwise mode of resistance acquisition. J. Antimicrob. Chemother. 68, 27–33. doi: 10.1093/jac/dks371
Phelan, J. E., O’Sullivan, D. M., Machado, D., Ramos, J., Oppong, Y. E. A., Campino, S., et al. (2019). Integrating informatics tools and portable sequencing technology for rapid detection of resistance to anti-tuberculous drugs. Genome Med. 11:41. doi: 10.1186/s13073-019-0650-x
Pyle, M. M. (1947). Relative numbers of resistant Tubercle bacilli in sputa of patients before and during treatment with streptomycin. Proc. Staff Meet. Mayo Clin. 22, 465–473.
R Core Team. (2014). R: A Language and Environment for Statistical Computing. Vienna, Austria: R Foundation for Statistical Computing.
Raman, K., and Chandra, N. (2008). Mycobacterium tuberculosis interactome analysis unravels potential pathways to drug resistance. BMC Microbiol. 8:234. doi: 10.1186/1471-2180-8-234
Rambaut, A. (2009). FigTree v1.4.3: Tree Figure Drawing Tool. Available online at: http://tree.bio.ed.ac.uk/software/figtree/ (accessed May 30, 2019).
Safi, H., Gopal, P., Lingaraju, S., Ma, S., Levine, C., Dartois, V., et al. (2019). Phase variation in Mycobacterium tuberculosis glpK produces transiently heritable drug tolerance. Proc. Natl. Acad. Sci. U. S. A. 116, 19665–19674. doi: 10.1073/pnas.1907631116
Safi, H., Sherman, D. R., Dick, T., and Alland, D. (2020). Mycobacterium tuberculosis glpK mutants in human tuberculosis. Proc. Natl. Acad. Sci. U. S. A. 117, 3913–3914. doi: 10.1073/pnas.1922199117
Salaemae, W., Azhar, A., Booker, G. W., and Polyak, S. W. (2011). Biotin biosynthesis in Mycobacterium tuberculosis: physiology, biochemistry and molecular intervention. Protein Cell. 2, 691–695. doi: 10.1007/s13238-011-1100-8
Shrestha, D., Maharjan, B., Thida Oo, N. A., Isoda, N., Nakajima, C., and Suzuki, Y. (2020). Molecular analysis of streptomycin-resistance associating genes in Mycobacterium tuberculosis isolates from Nepal. Tuberculosis 125:101985. doi: 10.1016/j.tube.2020.101985
Smittipat, N., Juthayothin, T., Billamas, P., Jaitrong, S., Rukseree, K., Dokladda, K., et al. (2016). Mutations in rrs, rpsL and gidB in streptomycin-resistant Mycobacterium tuberculosis isolates from Thailand. J. Glob. Antimicrob. Resist. 4, 5–10. doi: 10.1016/j.jgar.2015.11.009
Sousa, J., Cá, B., Maceiras, A. R., Simões-Costa, L., Fonseca, K. L., Fernandes, A. I., et al. (2020). Mycobacterium tuberculosis associated with severe tuberculosis evades cytosolic surveillance systems and modulates IL-1β production. Nat. Commun. 11:1949. doi: 10.1038/s41467-020-15832-6
Spies, F. S., Ribeiro, A. W., Ramos, D. F., Ribeiro, M. O., Martin, A., Carlos Palomino, J., et al. (2011). Streptomycin resistance and lineage-specific polymorphisms in Mycobacterium tuberculosis gidB Gene. J. Clin. Microbiol. 49, 2625–2630. doi: 10.1128/JCM.00168-11
Sun, H., Zeng, J., Li, S., Liang, P., Zheng, C., Liu, Y., et al. (2018). Interaction between rpsL and gyrA mutations affects the fitness and dual resistance of Mycobacterium tuberculosis clinical isolates against streptomycin and fluoroquinolones. Infect. Drug Resist. 11, 431–440. doi: 10.2147/IDR.S152335
Torrey, H. L., Keren, I., Via, L. E., Lee, J. S., and Lewis, K. (2016). High persister mutants in Mycobacterium tuberculosis. PLoS One 11:e0155127. doi: 10.1371/journal.pone.0155127
Vargas, R., and Farhat, M. R. (2020). Antibiotic treatment and selection for glpK mutations in patients with active tuberculosis disease. Proc. Natl. Acad. Sci. U. S. A. 117, 3910–3912. doi: 10.1073/pnas.1920788117
Walker, B. J., Abeel, T., Shea, T., Priest, M., Abouelliel, A., Sakthikumar, S., et al. (2014). Pilon: An integrated tool for comprehensive microbial variant detection and genome assembly improvement. PLoS One 9:e0112963. doi: 10.1371/journal.pone.0112963
Walker, T. M., Ip, C. L. C., Harrell, R. H., Evans, J. T., Kapatai, G., Dedicoat, M. J., et al. (2013). Whole-genome sequencing to delineate Mycobacterium tuberculosis outbreaks: a retrospective observational study. Lancet Infect. Dis. 13, 137–146. doi: 10.1016/S1473-3099(12)70277-3
Walker, T. M., Kohl, T. A., Omar, S. V., Hedge, J., Del Ojo Elias, C., Bradley, P., et al. (2015). Whole-genome sequencing for prediction of Mycobacterium tuberculosis drug susceptibility and resistance: a retrospective cohort study. Lancet Infect. Dis. 15, 1193–1202. doi: 10.1016/S1473-3099(15)00062-6
Wallis, R. S., Patil, S., Cheon, S. H., Edmonds, K., Phillips, M., Perkins, M. D., et al. (1999). Drug tolerance in Mycobacterium tuberculosis. Antimicrob. Agents Chemother. 43, 2600–2606. doi: 10.1128/aac.43.11.2600
Wattam, A. R., Abraham, D., Dalay, O., Disz, T. L., Driscoll, T., Gabbard, J. L., et al. (2014). PATRIC, the bacterial bioinformatics database and analysis resource. Nucleic Acids Res. 42, 581–591. doi: 10.1093/nar/gkt1099
WHO. (2020). Global tuberculosis report 2020. Available Online at: https://www.who.int/publications/i/item/9789240013131 [Accessed December 9, 2020]
Wong, S. Y., Lee, J. S., Kwak, H. K., Via, L. E., Boshoff, H. I. M., and Barry, C. E. (2011). Mutations in gidB confer low-level streptomycin resistance in Mycobacterium tuberculosis. Antimicrob. Agents Chemother. 55, 2515–2522.
Keywords: tuberculosis, streptomycin, resistance, transmission, evolution
Citation: Rocha DMGC, Magalhães C, Cá B, Ramos A, Carvalho T, Comas I, Guimarães JT, Bastos HN, Saraiva M and Osório NS (2021) Heterogeneous Streptomycin Resistance Level Among Mycobacterium tuberculosis Strains From the Same Transmission Cluster. Front. Microbiol. 12:659545. doi: 10.3389/fmicb.2021.659545
Received: 27 January 2021; Accepted: 06 May 2021;
Published: 11 June 2021.
Edited by:
Sandeep Sharma, Lovely Professional University, IndiaReviewed by:
Francesc Coll, University of London, United KingdomAlvaro Mourenza Flórez, Universidad de León, Spain
Copyright © 2021 Rocha, Magalhães, Cá, Ramos, Carvalho, Comas, Guimarães, Bastos, Saraiva and Osório. This is an open-access article distributed under the terms of the Creative Commons Attribution License (CC BY). The use, distribution or reproduction in other forums is permitted, provided the original author(s) and the copyright owner(s) are credited and that the original publication in this journal is cited, in accordance with accepted academic practice. No use, distribution or reproduction is permitted which does not comply with these terms.
*Correspondence: Nuno S. Osório, nosorio@med.uminho.pt
†These authors have contributed equally to this work