- 1Department of Marine Microbiology and Biogeochemistry, NIOZ Royal Institute for Sea Research, Texel, Netherlands
- 2Department of Earth Sciences, Faculty of Geosciences, Utrecht University, Utrecht, Netherlands
Structurally diverse, specialized lipids are crucial components of microbial membranes and other organelles and play essential roles in ecological functioning. The detection of such lipids in the environment can reveal not only the occurrence of specific microbes but also the physicochemical conditions to which they are adapted to. Traditionally, liquid chromatography coupled with mass spectrometry allowed for the detection of lipids based on chromatographic separation and individual peak identification, resulting in a limited data acquisition and targeting of certain lipid groups. Here, we explored a comprehensive profiling of microbial lipids throughout the water column of a marine euxinic basin (Black Sea) using ultra high-pressure liquid chromatography coupled with high-resolution tandem mass spectrometry (UHPLC-HRMS/MS). An information theory framework combined with molecular networking based on the similarity of the mass spectra of lipids enabled us to capture lipidomic diversity and specificity in the environment, identify novel lipids, differentiate microbial sources within a lipid group, and discover potential biomarkers for biogeochemical processes. The workflow presented here allows microbial ecologists and biogeochemists to process quickly and efficiently vast amounts of lipidome data to understand microbial lipids characteristics in ecosystems.
Introduction
Microorganisms play a primary role in the biochemical cycles of ecosystems. Understanding what microorganisms are doing, rather than simply assessing which microorganisms are present, is essential for understanding their role within ecosystems (Vestal and White, 1989). The structural diversity of lipids and their varied physicochemical properties reflect their wide ranging functions, such as the building blocks of membranes, energy storage, signaling, and modulating protein activity (Brügger, 2014). Microbial lipids are structurally very diverse and have proven to be of great taxonomic value (Sohlenkamp and Geiger, 2016). Indeed, certain microbial lipid classes have been used as biomarkers of specific taxonomic groups: for instance, isorenieratene for the photosynthetic green sulfur bacteria Chlorobiaceae (Sinninghe Damsté et al., 2001; Brocks et al., 2005), ladderane lipids for anammox bacteria (Sinninghe Damsté et al., 2002; Kuypers et al., 2003; Rush et al., 2012), and heterocyst glycolipids for filamentous N2 fixing cyanobacteria (Wolk et al., 1994; Bauersachs et al., 2009; Kumar et al., 2010). In addition, many microorganisms regulate their membrane lipid composition in order to adapt to environmental stress, thus these lipids have the potential to be used as biomarkers for specific environmental conditions (e.g., Benning et al., 1995; Van Mooy et al., 2009; Geiger et al., 2010; Martin et al., 2011; Popendorf et al., 2011b; Elling et al., 2015).
With the development over recent decades of LC-MS methods for intact polar lipid (IPL) analysis in the environment (Sturt et al., 2004), their distributions have been reported in many marine settings (e.g., Rossel et al., 2008; Schubotz et al., 2009, 2018; Van Mooy and Fredricks, 2010; Popendorf et al., 2011a, b; Wakeham et al., 2012; Sollai et al., 2019), lakes (Ertefai et al., 2008; Bale et al., 2016, 2019) and soils (Liu et al., 2010; Rethemeyer et al., 2010; Peterse et al., 2011; Ding et al., 2020; Warren, 2020). Most of these studies either used a targeted MS/MS approach, which focused on the dominant lipids or performed MS/MS interpretation manually, based on knowledge of the fragmentation patterns of specific lipid classes. This system of data analysis is laborious, time consuming, and is restricted to a few selected spectra that can be annotated among thousands of collected spectra (Kang et al., 2020). The requirement of high-level MS expertise makes this field difficult for non-chemists to get involved in, which has been seen to preclude the scientific maturation of the field (Wakeham and Lee, 2019).
Recent advances in the field of lipidomics, using non-targeted approaches combing with computational methods, allows for comprehensive lipidome profiling without a priori expert MS fragmentation knowledge (Pluskal et al., 2010; Shevchenko and Simons, 2010; Han et al., 2012; Wörmer et al., 2014; Yang and Han, 2016; Collins et al., 2016; Law and Zhang, 2019; Steen et al., 2020). The combination of analytical and computational advances provides a holistic picture of the lipidome and makes it more accessible, for example for studies of microbial ecology. Information theory, a mathematical analysis of information that has been used in a broad scope of microbiome diversity (Eren et al., 2014) and transcriptome diversity (Martínez and Reyes-Valdés, 2008), has been recently applied to the resolution of UHPLC-MS/MS derived metabolomic data in plants (Li et al., 2016, 2020). Application of information theory to lipidomic data may also allow us to characterize lipidomic diversity and specificity in the environment.
Another approach, molecular networking, is an excellent tool for visualization and annotation of non-targeted mass spectra without the need for cross referencing against known spectra (Watrous et al., 2012; Wang et al., 2016; Nothias et al., 2020). In this analysis, molecules related to each other based on the similarity of their fragmentation patterns form a molecular network, resulting in automated identification of analogs and related compounds. In addition to this, molecular networking has the advantage of further clustering lipids after chromatography separation, in regard to both their headgroups and their core moieties, as its principle is based on their MS/MS fragments similarity but not on their polarity or hydrophobicity. The related lipids in a cluster often differ only marginally structurally, by simple transformations such as alkylation, unsaturation, and glycosylation (Nothias et al., 2020). In addition, improved data visualization using molecular networks allows for the discovery of unknown molecules and reveal not only their molecular diversity but also their potential biological relationships (Watrous et al., 2012; Winnikoff et al., 2014; Hartmann et al., 2017). Recently, a few studies have applied molecular networking to the natural environment, based on lipidomic or metabolomic data (Kharbush et al., 2016; Petras et al., 2017; Petras et al., 2021). The methodology applied in such studies has the potential to be applied to lipid biomarker research, and in particular to IPLs.
In our companion paper (Bale et al., 2021), we presented a method that uses two-way hierarchical clustering to visualize a large UHPLC-HRMS dataset, made up of MS1 spectra which had been extracted using MZmine software. This data analysis method provided an overview of the variability within a complex environmental lipidome from an euxinic marine basin (the Black Sea), without bias towards known or abundant components. However, the approach of Bale et al. (2021) does not include automated extraction of MS2 spectra, and hence component identification was carried out using traditional (manual) methods. Here, we use the same UHPLC-HRMS/MS dataset, but after extraction of both MS1 and MS2 spectra a combination of information theory and molecular networking was applied to group components by similarity in their structure, rather than by similarity in their depth profile (as per Bale et al., 2021). In doing so, we allow for rapid component identification, based on similarity to known lipids. This approach provides a complementary information to previous work (Wakeham et al., 2007; Schubotz et al., 2009; Sollai et al., 2019) and extracts more detailed lipidomic information, valuable for a better understanding of complex environmental lipidomes of microbial communities.
Materials and Methods
Sampling, Extraction, and UHPLC-HRMS/MS Analysis
A detailed description of sample collection, extraction and analysis is given in Bale et al. (2021). Briefly, suspended particulate matter (SPM) at various water depth in the water column [50–2,000 meter below sea level (mbsl)] was collected in 2013 during the PHOXY cruise (June–July 2013) in the Black Sea (Kraal et al., 2017; Sollai et al., 2019) from the PHOX2 sampling station located at 42°53.8’N, 30°40.7’E in the center of the western gyre of the Black Sea. SPM was collected on pre-ashed 142-mm-diameter 0.7-μm pore size glass fiber GF/F filters (Pall Corporation, Port Washington, NY), mounted on McLane WTS-LV in situ pumps (McLane Laboratories Inc., Falmouth). The filters were immediately stored at –80°C until extraction.
Freeze-dried filters were extracted using a modified Bligh-Dyer procedure. After extraction, the extracts were analyzed using Agilent 1290 Infinity I UHPLC coupled to a Q Exactive Orbitrap MS (Thermo Fisher Scientific, Waltham, MA). The output data files (∗.raw files) generated by the UHPLC-HRMS analyses were converted to ∗.mzXML files using MSconvert software. In addition to the method described in our companion paper (Bale et al., 2021), both MS1 and MS2 spectra were extracted using MZmine software (Pluskal et al., 2010) for subsequent data processing. Process steps included mass peak detection, chromatogram building and deconvolution, isotope grouping, feature alignment and gap filling (Wang et al., 2016). The absolute abundance of components was obtained after processing. Due to our extraction and analytical methods, and based on annotation by the GNPS library (see later), we expect most of the components from the molecular network we generated to be lipids, thus we used the term “lipidome” for parts of the discussion where the components are discussed.
Creation of Molecular Networks
The combined dataset of MS/MS spectra were analyzed through The Global Natural Product Social Molecular Networking (GNPS) platform (Wang et al., 2016) using the Feature Based Molecular Networking tool (Nothias et al., 2020) to build molecular networks of the detected components in the dataset. Details can be found online at https://ccms-ucsd.github.io/GNPSDocumentation. The MS/MS dataset was filtered to remove [M+H]+ if the [M+NH4]+ was more abundant and vice versa, by removing all MS/MS fragment ions within ± 17 Daltons (Da) of the precursor mass-to-charge ratio (m/z). MS/MS spectra were divided in 50 Da windows and only the top 6 fragment ions in each 50 Da window were used. The precursor ion mass tolerance was set to 0.02 Da and the MS/MS fragment ion tolerance of 0.02 Da. A molecular network was then created where edges were filtered to have a cosine score above 0.6 (an edge with a cosine score 1.0 means two nodes are identical). Each node was connected to a maximum of 6 analogs in the network. Meanwhile, consensus spectra were searched against the GNPS spectral library with maximum analog mass difference of m/z 100. Precursor mass deviation and matching score (cosine) can be found online https://gnps.ucsd.edu/ProteoSAFe/status.jsp?task=871685198b1949e2a46e0e471400cdce. Molecular networks were visualized using Cytoscape 3.7.2 (Shannon et al., 2003; Smoot et al., 2010). Peak area/intensity of each MS/MS spectra was added as a metadata for the lipidome visualization across the water column of the Black Sea sample set (50–2,000 mbsl).
Information Theory Framework
Lipids were characterized by their own unique MS/MS spectrum and relative frequency of occurrence across the water column. The Hj index, estimating lipid diversity in different samples (depths), was calculated using Shannon entropy of MS/MS (lipid species) frequency distribution derived from the abundance of MS/MS precursors by the following equation as described by Martínez and Reyes-Valdés (2008) and Li et al. (2016).
where Pij correspond to relative frequency of the ith MS/MS (i = 1, 2, …, m) in the jth sample (j = 1, 2, …, t), to illustrate how abundant a specific MS/MS spectrum is relative to all others.
The average frequency of the ith MS/MS among samples was calculated as
Individual ion components (lipid species) specificity, the Si index, was defined as the identity of a given MS/MS regarding frequencies among all the samples. The MS/MS specificity was calculated as
Individual lipid species specificity of specific environmental sample, was defined as Sij index.
The water depth lipid specificity δj index was measured for each jth sample, the average of the MS/MS specificities using the following formula
Results and Discussion
In the study of Bale et al. (2021) 14,648 UHPLC-HRMS1 components were extracted and quantified using Mzmine (Pluskal et al., 2010) in the Black Sea water dataset (SPM of 15 depths collected from 50 to 2,000 mbsl in 2013 and 10 depths from 2017). In this study we only used the dataset from 2013, from which 12,031 components with an associated MS/MS spectrum were extracted by MZmine. We applied two analytical and computational methodologies: (1) a molecular network based on the MS/MS spectra similarities (Wang et al., 2016; Nothias et al., 2020) and (2) information theory based on Shannon entropy of the lipidome distribution (Martínez and Reyes-Valdés, 2008; Li et al., 2020; Figure 1A). One of the benefits of this approach is that no code is needed to apply the workflow shown in Figure 1A. The molecular network (Figure 2) generated from GNPS (Nothias et al., 2020) contained 6625 components in familial groupings (55% of the total) and 5406 singletons (components without molecular relatives in the network). Familial groupings appear as subnetworks within the molecular network (cf. orange rings in Figure 2).
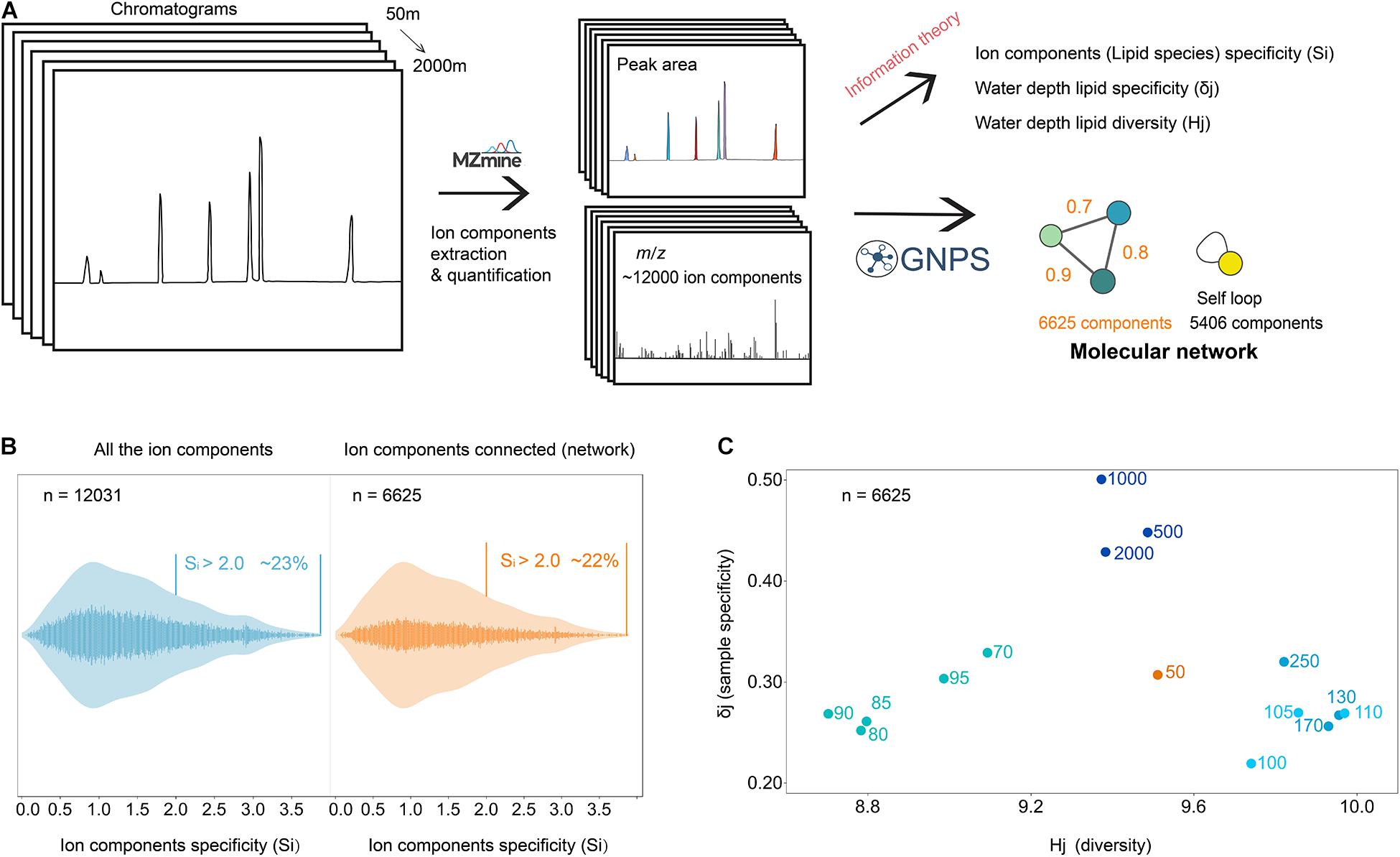
Figure 1. Testing the specificity and diversity of lipid species in the water column of the Black Sea using information theory and molecular network. (A) Schematic overview of the lipidomic data process and analysis workflow. (B) Specificity of the ion components among all the water column samples, left showed all the ion components while right showed the ion components connected through molecular network. Y axis represents the ion component counts, the different tones represent the trends of ion component specificity. The percentage shown here stands for the proportion of ion components that had high specificity (Si > 2), i.e., ca. 23% of all the ion components had high specificity Si > 2. (C) Scatter plot of Hj (diversity) vs. δj (specialization).
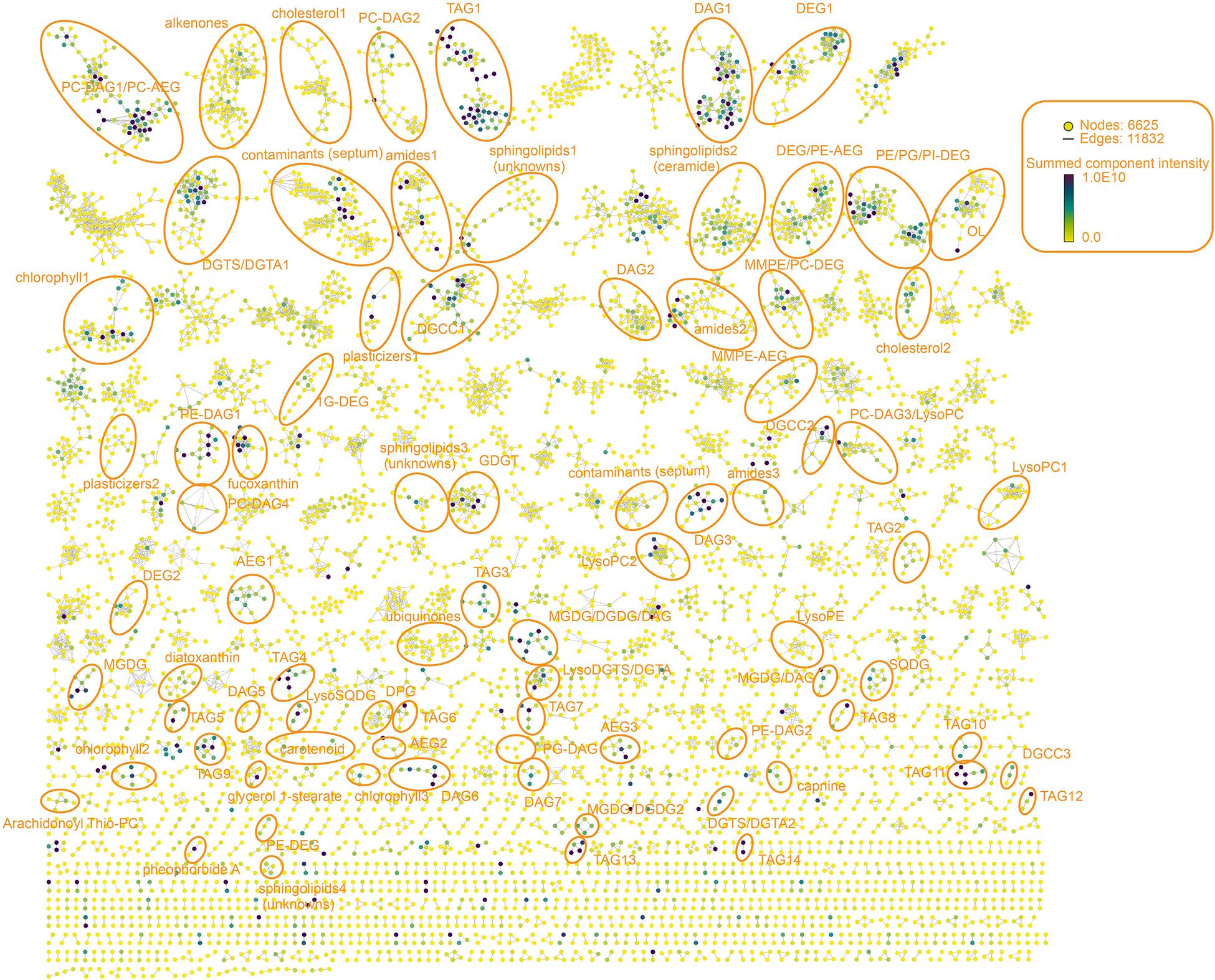
Figure 2. Molecular network of the water column in the Black Sea. Nodes represent MS/MS spectra of ion components (lipids) which are connected based on spectral similarity (cosine > 0.6). Nodes are labeled from light yellow to dark blue with the increasing of the summed intensity among all the water column samples (15 depths). The spatial orientation of the nodes in the MS/MS network (Figure 2) is randomly generated by Cytoscape (Shannon et al., 2003; Smoot et al., 2010) and does not relate to relationships between the subnetworks. Lipid classes (clusters) with the annotation are either tentatively identified in this study or have been reported by the previous studies (Schubotz et al., 2009, 2018; Van Mooy and Fredricks, 2010; Danielewicz et al., 2011; Wakeham et al., 2012; Bale et al., 2016; Kharbush et al., 2016; Becker et al., 2018b). DAG, diacylglycerol; DEG, dietherglycerol; AEG, acyletherglycerol; TAG, triacylglycerol; DGTS, diacylglycerylhydroxymethyltrimethyl-(N,N,N)-homoserine; DGCC, diacylglycerylcarboxyhydroxymethylcholine; DGTA, diacylglyceryl-hydroxymethyl)-tri-methyl-b-alanine; OL, ornithine lipid; DPG, diphosphatidylglycerol; PC, phosphatidylcholine; PE, phosphatidylethanolamine; PG, phosphatidylglycerol; PI, phosphatidylinositol; MMPE, phosphatidyl-(N)-methylethanolamine; MGDG, monoglycosyldiacylglycerol; GDGT, glycerol dialkyl glycerol tetraethers; SM, sphingomyelin; SQDG, sulfoquinovosyl diacylglycerol.
Information Theory-Diversity and Specificity of Lipidome
Based on information theory (Martínez and Reyes-Valdés, 2008; Li et al., 2020), we calculated a set of previously established indices: ion components (lipid species) specificity (Si index), Water depth lipid diversity (Hj index), and water depth lipid specificity (δj index). We examined the distribution of the Si index across all lipids (Figure 1B; n = 12,031) and across those in subnetworks (familial groupings), assigned as lipids (n = 6,625). For the Black Sea SPM, the Si specificity index highlights which lipids (n = 12,031) are specific for a certain depth of the water column, key for finding potential biomarkers associated with specific microbial communities or specific environmental conditions. A large number of lipids had small values of Si (Si < 2.0, 77% of the total), indicating they were either in low concentration or uniformly distributed throughout the water column. The distribution trend of specificity of the lipids connected through molecular network (Si < 2.0, 78%) was similar to that of the total components. With the help of the Si index (cf. Supplementary Table 2; Li et al., 2016), it is possible to focus on those lipids that are specific to certain water depths and thus for specific microbes and microbial niches.
The water depth lipid specificity (δj) is measured as the average degree of uniqueness of individual lipids and thus is an indicator for the average lipid specificity at a certain depth of the water column. A high value of the Hj diversity index at a specific depth, is either the result of a large proportion of the total lipids being detected at that depth, or because the lipids at that depth are evenly distributed in abundance. Cross plotting the specialization and diversity of the Black Sea lipidome result in groupings of depths (Figure 1C). The deep, euxinic waters (anoxic and sulfidic, 500–2,000 mbsl) had the most specialized lipidome profiles (i.e., unique occurrence of the lipid pattern), accompanied with a medium extent of diversity among all the water column depths. This supports observations that distinct microbial communities inhabit the deep euxinic waters of the Black Sea compared to the surface waters (Durisch-Kaiser et al., 2005; Wakeham et al., 2007; Sollai et al., 2019; Suominen et al., 2020a, b). The signature for low diversity and low specialization observed at depths 70–95 mbsl (including 80, 85, and 90 mbsl) was in line with the low numbers and low intensity of resolved chromatographic peaks seen for these depths (data not shown). The suboxic/euxinic zone interface (100–250 mbsl) exhibited the most diverse lipidome profile and relatively low specialization (i.e., the lipids generally were not found uniquely in this zone) among all the water column depth samples. This finding is in accordance with previous studies that showed that a wide range of microbially mediated processes including anammox, metal reduction, sulfide oxidation, anaerobic methane oxidation and anoxygenic photosynthesis co-occur within the suboxic and upper euxinic zone of the Black Sea (Wakeham et al., 2007).
Molecular Network: the Lipidome Throughout the Water Column
The MS/MS spectra search through the GNPS library resulted in 239 annotations (<2% annotation), which included ca. 20 contaminants, leaving the vast majority of components unknown. Such a low degree of annotation is consistent with other non-targeted environmental metabolomic studies (Petras et al., 2017, 2021). Lipidome annotation remains a bottleneck in lipidomic studies because public spectral databases are poorly populated. However, molecular networking enables the visualization of relationships between known and unknown lipids, based on the similarity of their MS/MS spectra. Despite the low percentage of library annotation, more than half the components clustered together in an individual network (Figure 2).
Most of the lipids that clustered together in the subnetworks were either analogs of each other with an identical head group or with a similar core, differing by simple transformations such as alkylation, unsaturation, and glycosylation (Nothias et al., 2020). Each node in a molecular network stands for an individual lipid associated with a specific MS/MS spectrum (see Supplementary Figure 1 as an example). Nodes in Figure 2 are labeled from light yellow to dark blue according to the lipid’s summed intensity (which is equated to abundance here) throughout the water column. Unknown lipids in a subnetwork were annotated if they were connected to one or two annotated lipids in the library (Supplementary Figure 2). Subnetworks of nodes without any library hits were annotated manually, either by comparison to data from previous studies (Rossel et al., 2008; Schubotz et al., 2009, 2018; Van Mooy and Fredricks, 2010; Danielewicz et al., 2011; Wakeham et al., 2012; Bale et al., 2016; Kharbush et al., 2016; Becker et al., 2018b) or putatively identified based on accurate mass and MS/MS fragmentation (Supplementary Table 1). Many of the subnetworks in our molecular network represent lipids that have been putatively identified earlier in studies of IPLs in the Black Sea water column and sediments (Wakeham et al., 2007; Schubotz et al., 2009; Becker et al., 2018b; Sollai et al., 2019). These included: diacylglycerol phosphatidylcholine (PC-DAG), diacylglycerol phosphatidylethanolamine (PE-DAG), diacylglycerol phosphatidylglycerol (PG-DAG), 1,2-diacylglyceryl-3-carboxyhydroxymethylcholine (DGCC), 1,2- diacylglyceryl-3-trimethylhomoserine (DGTS), diacylglyceryl-O-hydroxymethyl-(N,N,N-trimethyl)-β-alanine (DGTA), ornithine lipids (OL), monoglycosyldiacylglycerol (MGDG), dietherglycerol phosphatidylethanolamine (PE-DEG), glycosidic ceramides, isoprenoidal quinones, and glycerol dialkyl glycerol tetraethers (GDGT). Subnetworks tentatively identified within our molecular network (Figure 2) which were not reported in previous studies, included triacylglycerols (TAGs), some novel sphingolipids, certain DEG-based IPLs, and some specific chlorophylls. Our results demonstrate that a significant benefit of molecular networking is that a wider range of annotated lipids is produced with increased speed than arising by traditional analysis of LC-MS data. Molecular networking enables the grouping of different lipid classes both based on their polar head group and their core moiety. For example, there were four subnetworks of PC-DAGs (Figure 2). Some contained two short acyl carbon chain length (in sum C28 to C34) with 0–2 double bonds (Supplementary Table 1), most likely produced by algae or nitrogen–fixing or heterotrophic bacteria (Kato et al., 1996; Martínez-Morales et al., 2003; Sohlenkamp et al., 2003; Schubotz et al., 2009). The other PC-DAGs contained longer acyl carbon chain lengths (C34 to C40) with 0–8 double bonds, which are typically associated with algae (Kato et al., 1996).
In order to demonstrate how the molecular network can be used to understand lipidomic data in an environmental context, we discuss the depth distribution of three subnetworks: those of TAGs, carotenoids, and DEGs (Figures 3A–C). For this, the shape of each node was changed from a solid circle (as per Figure 2) to a heatmap (Figure 3), which represents the abundance gradient of the lipid from surface water to deep anoxic zone (50–2,000 mbsl).
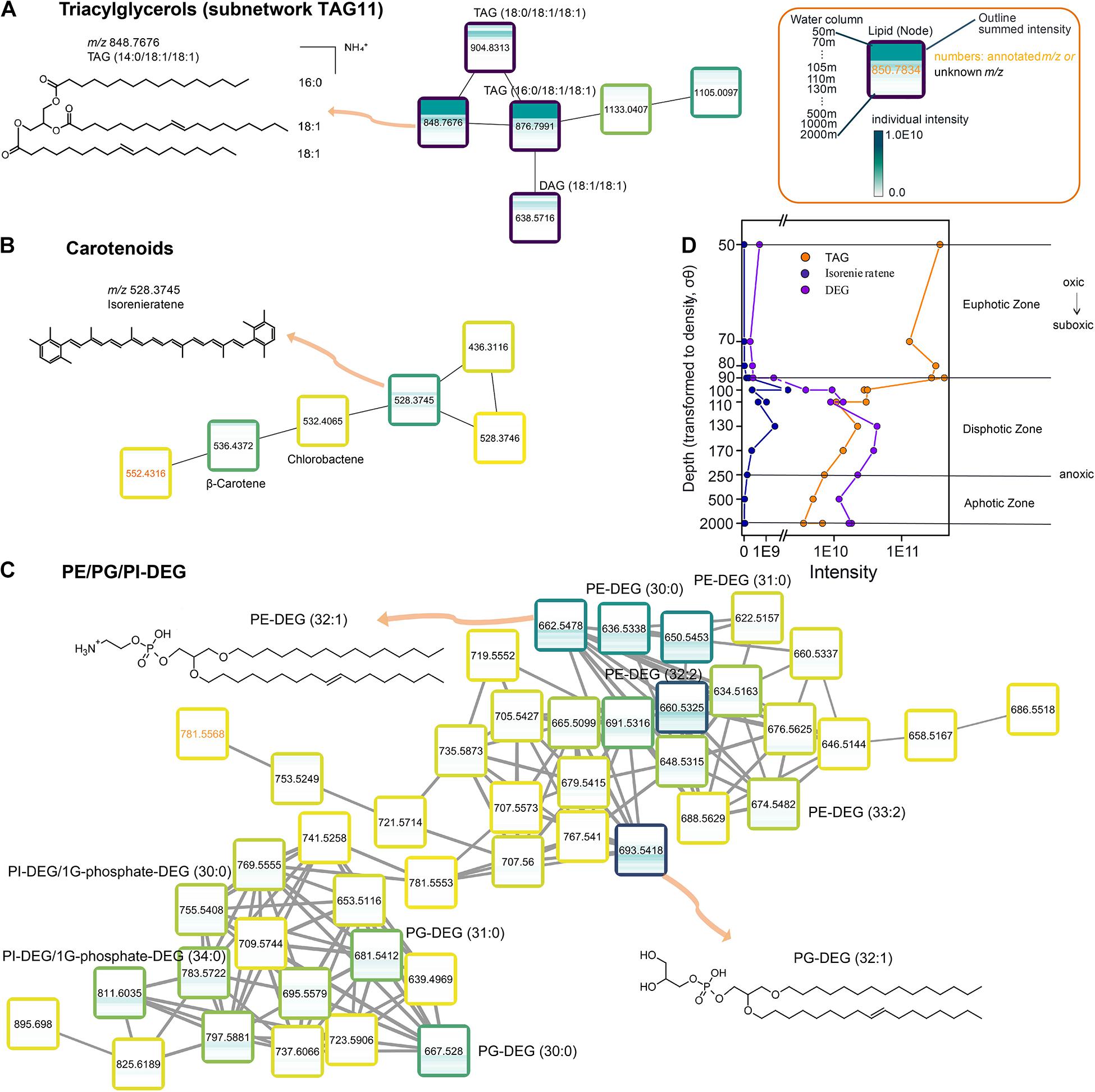
Figure 3. Molecular subnetwork of specific lipid classes determines the photic and aerobic zone in the Black Sea. (A) Subnetwork of triacylglycerol 11. (B) Part of subnetwork of carotenoid. (C) Subnetwork of PE/PG/PI-DEG. (D) Distribution and stratification of the summed intensity of TAG, isorenieratene and DEG in the water column of the Black Sea (50–2,000 mbsl). The names of these selected lipid classes are linked to the ones in the major network (Figure 2). Boxes shown here are the same as nodes shown in Figure 2, representing lipid species. The outlines of the boxes are labeled from light yellow to dark blue with the increasing of the summed intensity among all the water column samples (15 depths). Inside the box, a heatmap represents the distribution of this lipid species across the water column, from top (50 mbsl) to bottom (2,000 mbsl). The lipid components with closely related masses (e.g., m/z 707.5600 vs. 707.5573, C) have similar mass spectra but different retention time (20.9 min vs. 20.3 min), suggesting they are structural isomers.
TAGs are mostly known as energy storage lipids produced mainly by algal species under environmental stress, such as photo-oxidative stress or nitrogen deprivation (Thompson, 1996; Bigogno et al., 2002; Hu et al., 2008). They are generally synthesized in the light and then reutilized for the synthesis of other lipids in the dark (Thompson, 1996; Becker et al., 2018a), thus can be used as a marker for the euphotic and (sub)oxic zone (Figures 3A,D). The subnetwork TAG11 (Figure 3A) contains three TAGs (m/z 848.7676–904.8313; Supplementary Table 1), one DAG (m/z 638.5716, 18:1/18:1) and two unknown lipids. All these three TAGs contain a same DAG group (18:1/18:1) but differ in the third fatty acid chain length (C14, C16 or C18). They were present in the lower photic zone (50–90 mbsl, Figure 3A), below 90 mbsl their relative abundance decreased strikingly, by more than one order of magnitude.
The subnetwork B (Figure 3B) contains several carotenoids, including β-carotene (m/z 536.4372), chlorobactene (m/z 532.4065) and isorenieratene (m/z 528.3745). Isorenieratene is a carotenoid uniquely biosynthesized by the low-light-adapted photosynthetic green sulfur bacteria Chlorobiaceae (Repeta et al., 1989; Sinninghe Damsté et al., 1993; Sinninghe Damsté et al., 2001). Chlorobiaceae perform photosynthesis using sulfide, thus they require an euxinic, stratified water column with strictly anaerobic conditions and hence isorenieratene and its derivatives can be used as biomarkers for photic zone euxinia (Koopmans et al., 1996). Isorenieratene concentration was highest between 100 and 130 mbsl (Figures 3B,D), which is consistent with previous studies showing that green sulfur bacteria were present in the chemocline of the Black Sea (Overmann et al., 1992; Manske et al., 2005). Structurally closely related carotenoids such as chlorobactene and β-carotene are also found in this cluster, although with different depth distributions.
The subnetwork C (Figure 3C) contain diether glycerols (DEGs) with several types of head groups (PE/PG/PI). They differ from each other in chain length (C30 to C34) and degree of unsaturation (zero up to two). DEGs with different head groups (PE/PG/PI) have been commonly associated with sulfate-reducing bacteria (Sturt et al., 2004; Schubotz et al., 2009) and consequently are applied as indicators for anaerobic/sulfidic conditions. In our dataset, DEGs were firstly encountered in the upper anoxic zone with the appearance of sulfide (95 mbsl) and reached a maximum at 130 mbsl (Figures 3C,D).
The stratification highlighted using these three subnetworks is in agreement with the results achieved by hierarchical clustering of the same MZmine data by Bale et al. (2021). It shows that applying molecular networking to the water column lipidome has the potential to deliver biologically meaningful lipid-microbial community associations or environmental factors on lipid distribution.
Different Microbial Sources Within the Same Lipid Class
Molecular networking enables us to investigate the potential sources of individual groups of compounds in the same polar lipid classes. For example, there were fourteen separate subnetworks of TAGs (Figure 2), indicating that groups of TAGs differed significantly from each other in carbon chain length or degree of unsaturation. TAGs which contain a polyunsaturated fatty acid (FA; e.g., TAG-C16:0/C22:6/C22:6; clustered in TAG9) were predominant in the euphotic zone (50–90 m; Figure 4A). Algal species such as Euglenophyceae, Cryptophyceae, and Eustigmatophyceae possess the ability to synthesize these TAGs (Hu et al., 2008). Similar depth distributions were also detected in the subnetwork of TAG11 (Figure 3A). These TAGs contain a FA with carbon chain lengths of C16 and C18 and 0–2 double bonds (Figure 3A), they are also known as typical storage lipids in most algae (Volkman et al., 1989). Distinct from the subnetworks of TAGs mentioned above, subnetworks of TAGs with odd (C15 and C17; likely iso) and short (C12 and C14) acyl chain length, were only shown to be abundant at 90 mbsl (TAG3, Figure 4B), suggesting they have a different source. To further determine the source of different types of TAGs mentioned above, we compared betaine DGCC lipids to the long-chain polyunsaturated TAGs (which contain at least one acyl chain with > 20 carbon atoms) and short-odd-chain TAGs (which contain C12, C14, C15, or C17 acyl chains). DGCC lipids are supposed to be produced mainly by algae (Kato et al., 1994; Van Mooy et al., 2009). We found there was no correlation between the latter TAGs and DGCC lipids, while the DGCCs did correlate with TAGs containing even carbon numbered, long-chain polyunsaturated fatty acids (R2 = 0.85, P < 0.01; Figure 4C). This suggests non-algae sources for the TAGs with short and odd chain fatty acids, such as the bacteria actinomycetes which are capable of producing odd chain TAGs (Alvarez and Steinbüchel, 2002).
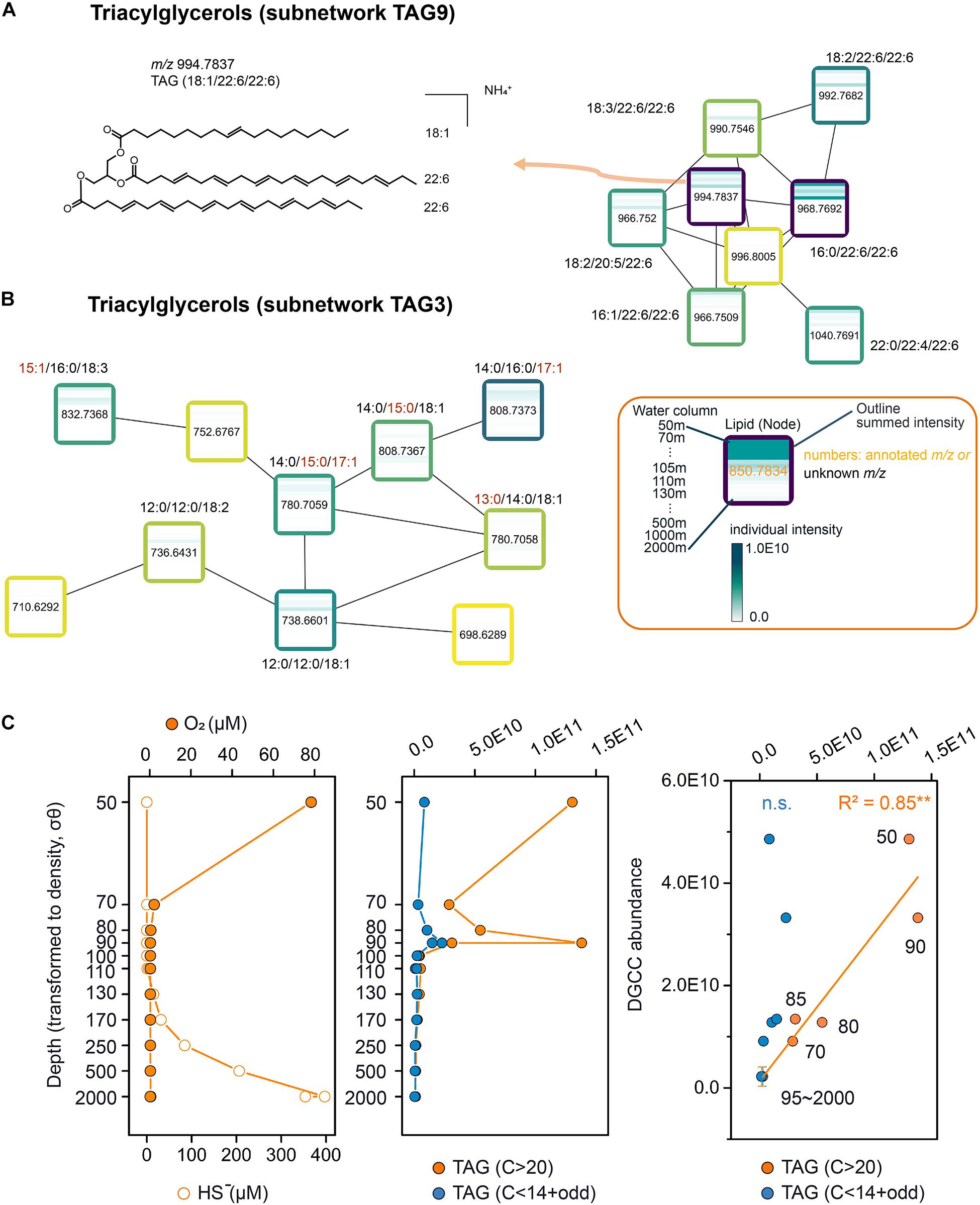
Figure 4. Molecular subnetwork of triacylglycerols and their source determination. (A) Subnetwork of TAG9. (B) Subnetwork of TAG3. (C) From left to right: Distribution of oxygen (O2, μM, orange solid cycles) and sulfide (HS–, μM, orange hollow cycles) in the water column of the Black Sea (50–2,000 mbsl); Distribution of summed TAGs with polyunsaturated fatty acids (at least one of the three acyl chain length with more than C20, orange cycles) and summed TAGs with odd (C15 and C17) and short (C12 and C14) carbon chain length (blue cycles); Scatter plots of DGCC vs. TAGs with polyunsaturated fatty acids (orange cycles) and TAGs with odd (C15 and C17) and short (C12 and C14) carbon chain length (blue cycles). Significance: **P < 0.01; n.s., not significant. The names of these selected lipid classes are linked to the ones in the major network (Figure 2). Boxes shown in subnetwork are the same as nodes shown in Figure 2, representing lipid species. The outlines of the boxes are labeled from light yellow to dark blue with the increasing of the summed intensity among all the water column samples (15 depths). Inside the box, a heatmap represents the distribution of this lipid species across the water column, from top (50 mbsl) to bottom (2,000 mbsl).
Another distinct depth pattern was found in the subnetwork of the betaine lipids DGTS and DGTA (Figure 5). DGTS and DGTA are structural isomers with the same characteristic fragment ions in the MS/MS spectra, and hence they cluster in the same subnetwork. Since DGTS cannot be distinguished from DGTA based on mass spectra, we hereafter indicate these betaine lipids as DGTS/DGTA for the following discussion. DGTS/DGTA lipids with C32:2 and C32:1 acyl chains were dominant in the oxic zone (50–90 mbsl; Figure 5A). The sum of their abundance, together with another cluster of DGTS/DGTA with longer (C34 to C40) acyl chains with 0–10 double bonds (DGTS/DGTA1 in Figure 2 and Supplementary Table 1), were significantly correlated with the abundance of headgroup-less DAGs (R2 = 0.97, P < 0.001; Figure 5B). DAGs were dominant in the oxic and suboxic zone and are likely catabolic products or biosynthetic intermediates of TAGs, phospholipids and glycolipids, derived mainly from algae (Kharbush et al., 2016; Becker et al., 2018a). The correlation strongly suggesting that these DGTS/DGTA lipids were also derived from algae (Dembitsky, 1996; Van Mooy and Fredricks, 2010). A different subnetwork of DGTS/DGTA with C31 to C35 acyl chains and 0–2 double bonds were absent from the surface waters but were relatively more abundant in deep suboxic and euxinic zone (110–2,000 mbsl) with a peak at 130 mbsl (Figure 5A). Significant correlation was found between the intensity of these DGTS/DGTA lipids with that of DEGs with and without headgroups (R2 = 0.70, P < 0.01; Figure 5B). In contrast to DAGs, DEGs in the Black Sea water column has been shown to correlate with sulfate reducing bacteria (Neretin et al., 2007) and in general are mostly associated with anaerobic bacteria (Grossi et al., 2015). This suggests these types of betaine lipids were derived from anaerobic bacteria such as sulfate reducing bacteria (López-Lara et al., 2003) or other bacteria residing in the euxinic zone. Although distinct patterns of DGTS/DGTA lipids throughout the water column were also observed in previous studies (Schubotz et al., 2009; Van Mooy and Fredricks, 2010), here the application of molecular networking enables the direct observation of individual DGTS/DGTA distributions across the water column.
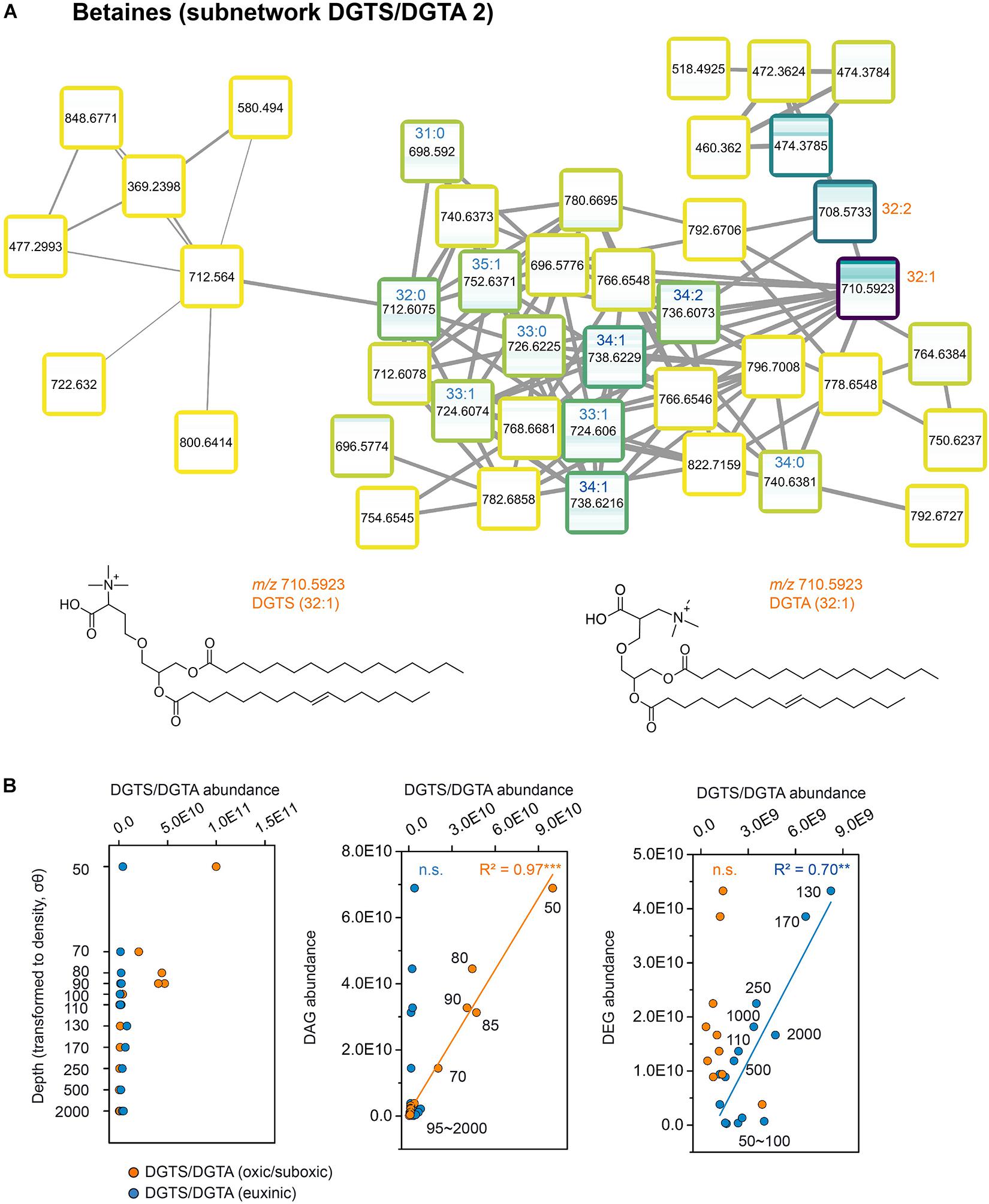
Figure 5. Molecular subnetwork of betaine lipids (DGTS/DGTA) and their source determination. (A) Subnetwork of betaine lipids DGTS/DGTA. (B) From left to right: Distribution of DGTS/DGTA (oxic to suboxic, abundant at the surface water 50–90 mbsl, including DGTS/DGTA with C32:2, C32:1, C34 to C40 carbon chain length and 0–10 double bonds, orange cycles) and DGTS/DGTA(euxinic, abundant at the deep water 110–2,000 mbsl, including DGTS/DGTA with C31 to C35 carbon chain length and 0–2 double bonds, blue cycles); Scatter plots of DAG vs. DGTS/DGTA (oxic/suboxic, orange cycles) and DGTS/DGTA (euxinic, blue cycles); Scatter plots of DEG with several head groups (PE/PG/PI) vs. DGTS/DGTA (oxic/suboxic, orange cycles) and DGTS/DGTA (euxinic, blue cycles). Significance: ***P < 0.001; **P < 0.01; n.s., not significant. The name of selected DGTS/DGTA is linked to the one in the major network (Figure 2). Boxes shown in subnetwork are the same as nodes shown in Figure 2, representing lipid species. The outlines of the boxes are labeled from light yellow to dark blue with the increasing of the summed intensity among all the water column samples (15 depths). Inside the box, a heatmap represents the distribution of this lipid species across the water column, from top (50 mbsl) to bottom (2,000 mbsl).
Targeting Unknown Lipids
Given the diversity and complexity of lipids from environmental samples, less abundant but perhaps ecologically informative lipids cannot be easily detected if they coelute with more dominant components of the lipidome. With the help of a molecular network, embedded with the heatmaps of the lipids’ variation across the ecosystem, one can rapidly pick out significant unknown lipids at certain depths or specific environmental conditions. A great number of such lipids can then be putatively identified by comparing their MS/MS fragmentation pattern to the associated ones if they are in a subnetwork.
Many of the subnetworks in our dataset (Figure 2) contained unknown components. The MS/MS pattern recognition, intrinsic to the molecular network data processing, provide an indication as to their structures, based on similarity to known lipids within the same subnetwork. To illustrate this, we focus first on a subnetwork (Sphingolipids1) that contains four cluster of unassigned lipids (Figure 6). From a first cursory inspection of the MS/MS mass spectra associated with these components (Supplementary Figures 3A–D), it became clear that all these unassigned components shared structural features, explaining why these clusters are connected, but differed from each other in complexity of the whole molecule (see Supplementary Text and Supplementary Figure 3 for details). The first unknown cluster (Figure 6, cluster 1) contains 13 lipid species. The MS/MS spectrum from one of the members of this cluster (m/z 704.7254, Figure 6 and Supplementary Figure 2A) was very similar to that of a ceramide standard (d18:1/24:0, m/z 650.6454, Supplementary Figure 4), but with one less loss of H2O in the unknown lipid. We therefore tentatively identify it as 1-deoxyceramide (d20:0/27:1). Other lipid species in the cluster 1 are also 1-deoxyceramides, differing from each other in the chain lengths of 1-deoxysphinganine base (C19 to C21, Figure 6) and/or fatty acid groups (C20 to C32).
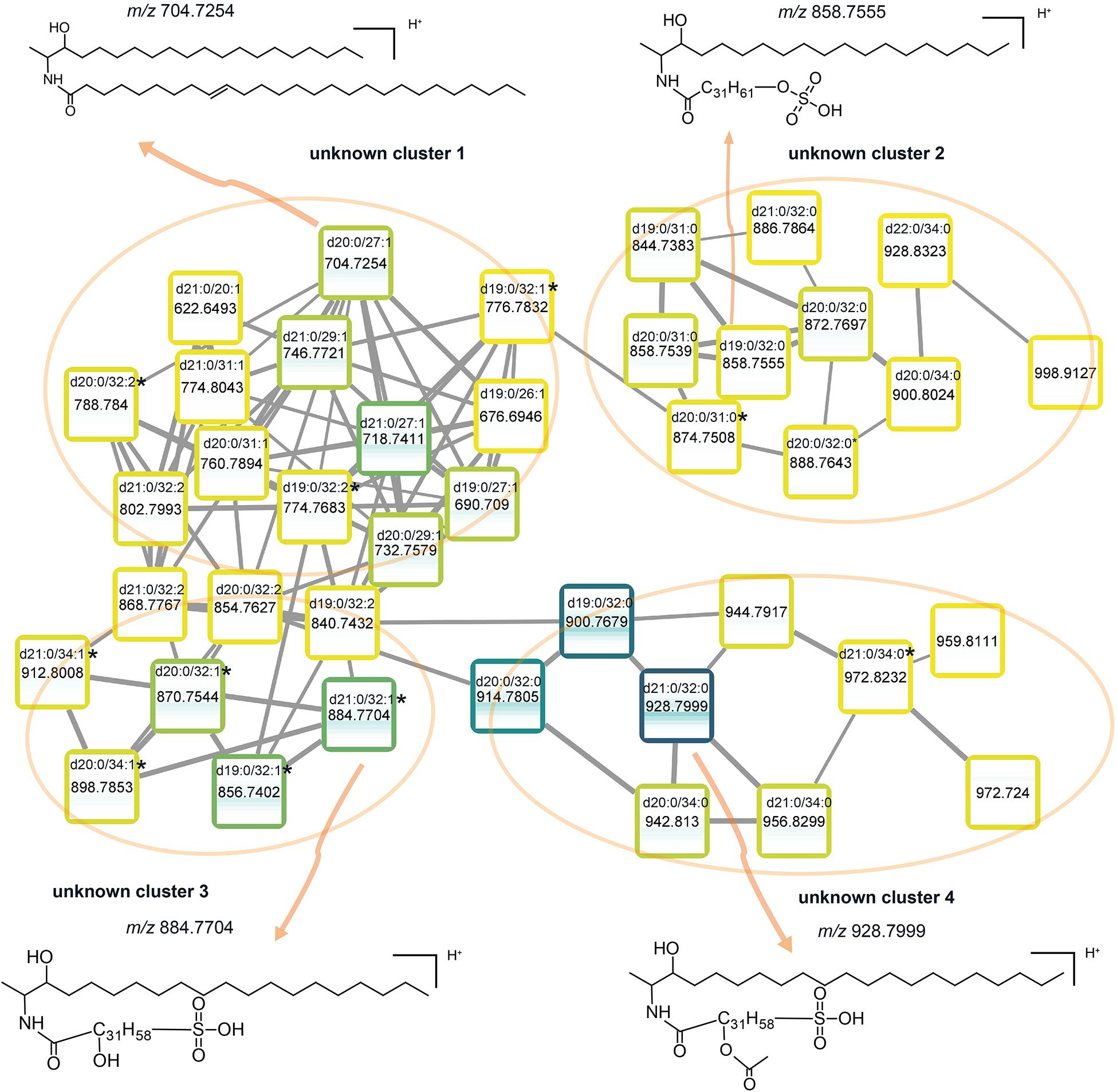
Figure 6. Molecular subnetwork of sphingolipids1. Boxes shown in subnetwork are the same as nodes shown in Figure 2, representing lipid species. The outlines of the boxes are labeled from light yellow to dark blue with the increasing of the summed intensity among all the water column samples (15 depths). Inside the box, a heatmap represents the distribution of this lipid species across the water column, from top (50 mbsl) to bottom (2,000 mbsl). ∗Represents there is a –OH group in the fatty acid chain of the lipid. Mass spectra of novel lipids are shown in Supplementary Figures 3A–D.
1-deoxysphinganine, the sphingoid base of the 1-deoxyceramides was first found in a marine organism, Spisula polynyma (Cuadros et al., 2000). Since then, 1-deoxyceramides were typically reported as “total 1-deoxysphinganines” because they were quantified after acid hydrolysis to release the sphingoid bases (Merrill, 2011). With the technical progress in HPLC-HRMS over the last few years, it is now possible to analyze 1-deoxycermide as individual molecular species (Duan and Merrill, 2015). These atypical headless sphingolipids cannot be degraded over the canonical catabolic pathways and are incapable to be converted to complex sphingolipids (Lone et al., 2019). Due to the lack of the C1-OH, they cannot be further metabolized into more complex sphingolipids and the understanding of their biological functions is still limited (Carreira et al., 2019).
The other three clusters of unknown components that connected to the cluster 1 are 1-deoxyceramides with polar moieties (Figure 6). Unlike the common sphingolipids which contain polar moieties as “headgroups” at the C1-OH position (Walker et al., 2017; Heaver et al., 2018), the polar moieties of these unusual 1-deoxyceramides appear to be located on their fatty acid chain (Supplementary Text and Supplementary Figures 3B–D). Based on the MS/MS spectrum, the lipid species in the second unknown cluster contain a sulfate moiety on their fatty acid chain, thus they are tentatively identified as sulfate-1-deoxyceramides (Supplementary Figure 3B). The third unknown cluster consists of 1-deoxyceramides containing a hydroxy-fatty acid modified with a sulfur trioxide moiety (Supplementary Figure 3C). We therefore proposed them as sulfono-1-deoxyceramides. The fourth and last unknown cluster has 1-deoxyceramides with an extra acetic acid and a sulfur trioxide moiety on their fatty acid chain (Supplementary Figure 3D), hence, we assigned these components as acetylsulfono-1-deoxyceramides.
All these newly putatively identified sphingolipids were at their maximum abundance at the interface between the suboxic and euxinic zones (95–250 mbsl; Figure 6). Bacterial sphingolipids are phylogenetically restricted to be produced by mainly members of the Bacteroidetes and selected Proteobacteria (Heaver et al., 2018). Certain Bacteroidetes are known to produce capnines, sulfono-analogs of sphinganines (Godchaux III, and Leadbetter, 1980). Recently three members of the Bacteroidetes, Ancylomarina euxinus sp. nov. Labilibaculum euxinus sp. nov., and Lutibacter sp., all isolated from the euxinic zone of the Black Sea, were found to have capnines among their most abundant lipids (Bale et al., 2020; Yadav et al., 2020). Capnines were also found in the molecular network (Figure 2). We hypothesize that the novel 1-deoxysphingolipids putatively identified in this study, may also be produced by anaerobic heterotrophs related to Bacteroidetes.
Another example of a subnetwork is one that only contained unknown lipids (Figure 7) and which was associated within the euxinic zone (130–2,000 mbsl). All 14 lipids in the subnetwork exhibited sphingolipid-like MS/MS fragmentation, but unlike the earlier-mentioned sphingolipid subnetwork (Figure 6), these MS/MS spectra (Figure 7 and Supplementary Text) revealed that these lipids contained relatively short dehydrosphinganine bases (C15 to C18) connected to a longer chain hydroxy fatty acid (C19). The polar head group was tentatively identified as a lysine (Moore et al., 2016). Therefore, they were tentatively assigned as lysine-dihydroceramides (Figure 7 and Supplementary Text). To the best of our knowledge, this is the first report to indicate the presence of lysinesphingolipids in environmental samples. Their presence in the euxinic zone suggests they are derived from anaerobic bacteria. Among all the annotated lipids, the newly putatively identified sphingolipids (Figures 6, 7) have the highest Sij index (Supplementary Table 2) in the deep zone (130–1,000 mbsl). The higher Sij index is, the more the occurrence of a certain lipids is restricted to at certain depth. Thus, these newly putatively identified sphingolipids are one of the most specialized lipids in this distinct euxinic region.
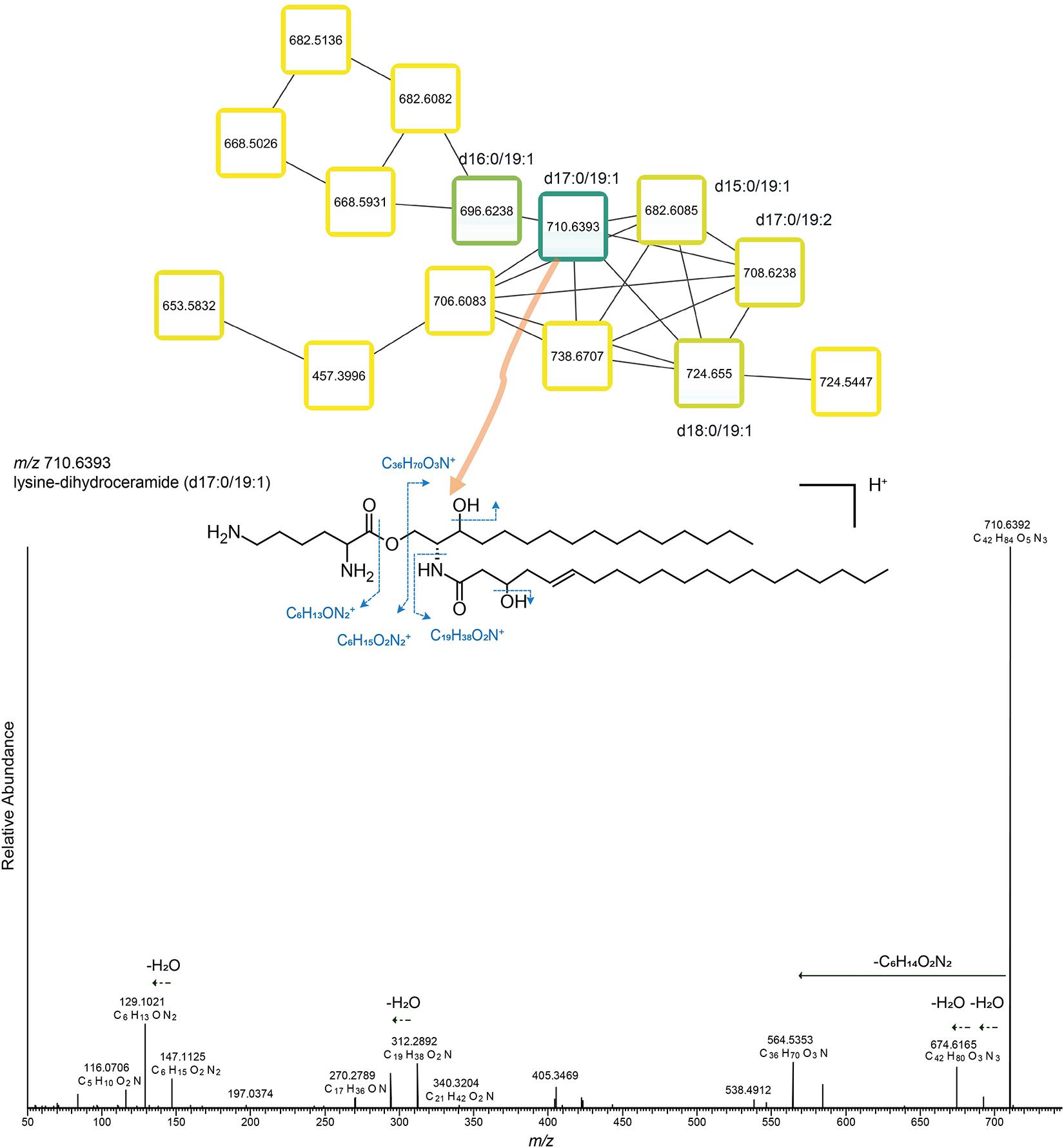
Figure 7. Molecular subnetwork of sphingolipids3. Boxes shown in subnetwork are the same as nodes shown in Figure 2, representing lipid species. The outlines of the boxes are labeled from light yellow to dark blue with the increasing of the summed intensity among all the water column samples (15 depths). Inside the box, a heatmap represents the distribution of this lipid species across the water column, from top (50 mbsl) to bottom (2,000 mbsl).
Conclusion
In this study, we carried out comprehensive lipidomic profiling of microbial communities throughout the water column of the Black Sea using UHPLC-HRMS/MS spectra. A major strength of our data processing method is that we combined information theory and molecular networking to capture a holistic view of the lipidome as well as specific signatures in the environment. Indeed, the molecular network provided a comprehensible visualization of the lipidome throughout the water column, while information theory allowed us to capture the signatures of diversity and specialization within the lipidome. Application of molecular networking has proven to be useful in discovering novel lipids, helping to determine their origin, and associating biomarkers with potential microbial niches. Another advantage on this method is that the diversity of unknown lipids is revealed before they are identified. In conclusion, this study reinforces a powerful set of computational approaches to accelerate our understanding of lipidomic information in environmental microbial ecology.
Data Availability Statement
The raw data supporting the conclusions of this article will be made available by the authors, without undue reservation.
Author Contributions
SD, NB, EH, LV, SS, and JSSD designed the study. SD performed the data analysis. SD, NB, and EH did the lipid identification and wrote the draft manuscript. MGIA provided methodological support. SS and JSSD supervised the study. All authors read, discussed, and approved the final manuscript.
Funding
This project received funding from the European Research Council (ERC) under the European Union’s Horizon 2020 Research and Innovation Program (grant agreement no. 694569, MICROLIPIDS).
Conflict of Interest
The authors declare that the research was conducted in the absence of any commercial or financial relationships that could be construed as a potential conflict of interest.
Acknowledgments
We thank C. P. Slomp for the acquisition of funding of the PHOXY cruise 64PE371 and G. J. Reichart for leading it. We acknowledge the captains and crew of the R/V Pelagia. We thank P. Dorrestein’s lab, A. Haas, and C. Simon for the advice of GNPS network. We thank D. Li for discussions on the information theory, D. Maat and P. Kraal for discussions on the Black Sea water column, and Jan de Leeuw for the suggestion of certain lipid nomenclature. We also thank two reviewers, FS and JC, for their helpful comments to improve the manuscript.
Supplementary Material
The Supplementary Material for this article can be found online at: https://www.frontiersin.org/articles/10.3389/fmicb.2021.659315/full#supplementary-material
References
Alvarez, H., and Steinbüchel, A. (2002). Triacylglycerols in prokaryotic microorganisms. Appl. Microbiol. Biotechnol. 60, 367–376. doi: 10.1007/s00253-002-1135-0
Bale, N. J., Ding, S., Hopmans, E. C., Villanueva, L., Boschman, C., Arts, M. G. I., et al. (2021). Lipidomics of environmental microbial communities. I: visualization of specific niches using untargeted analysis of high-resolution mass spectrometry data. Front. Microbiol. 2021:659302. doi: 10.3389/fmicb.2021.659302
Bale, N. J., Hopmans, E. C., Schoon, P. L., de Kluijver, A., Downing, J. A., Middelburg, J. J., et al. (2016). Impact of trophic state on the distribution of intact polar lipids in surface waters of lakes. Limnol. Oceanogr. 61, 1065–1077. doi: 10.1002/lno.10274
Bale, N. J., Koenen, M., Yadav, S., Hopmans, E. C., Villanueva, L., Sinninghe Damsté, J. S., et al. (2020). Diagnostic amide products of amino lipids detected in the microaerophilic bacteria lutibacter during routine fatty acid analysis using gas chromatography. Org. Geochem. 144:104027. doi: 10.1016/j.orggeochem.2020.104027
Bale, N. J., Sorokin, D. Y., Hopmans, E. C., Koenen, M., Rijpstra, W. I. C., Villanueva, L., et al. (2019). New insights into the polar lipid composition of extremely halo(alkali)philic Euryarchaea from hypersaline lakes. Front. Microbiol. 10:377. doi: 10.3389/fmicb.2019.00377
Bauersachs, T., Compaoré, J., Hopmans, E. C., Stal, L. J., Schouten, S., and Sinninghe Damsté, J. S. (2009). Distribution of heterocyst glycolipids in cyanobacteria. Phytochemistry 70, 2034–2039. doi: 10.1016/j.phytochem.2009.08.014
Becker, K. W., Collins, J. R., Durham, B. P., Groussman, R. D., White, A. E., Fredricks, H. F., et al. (2018a). Daily changes in phytoplankton lipidomes reveal mechanisms of energy storage in the open ocean. Nat. Commun. 9:5179.
Becker, K. W., Elling, F. J., Schröder, J. M., Lipp, J. S., Goldhammer, T., Zabel, M., et al. (2018b). Isoprenoid quinones resolve the stratification of redox processes in a biogeochemical continuum from the photic zone to deep anoxic sediments of the Black sea. Appl. Environ. Microbiol. 84, e02736–17.
Benning, C., Huang, Z. H., and Gage, D. A. (1995). Accumulation of a novel glycolipid and a betaine lipid in cells of Rhodobacter sphaeroides grown under phosphate limitation. Arch. Biochem. Biophys. 317, 103–111. doi: 10.1006/abbi.1995.1141
Bigogno, C., Khozin-Goldberg, I., Boussiba, S., Vonshak, A., and Cohen, Z. (2002). Lipid and fatty acid composition of the green oleaginous alga Parietochloris incisa, the richest plant source of arachidonic acid. Phytochemistry 60, 497–503. doi: 10.1016/s0031-9422(02)00100-0
Brocks, J. J., Love, G. D., Summons, R. E., Knoll, A. H., Logan, G. A., and Bowden, S. A. (2005). Biomarker evidence for green and purple sulphur bacteria in a stratified Palaeoproterozoic sea. Nature 437, 866–870. doi: 10.1038/nature04068
Brügger, B. (2014). Lipidomics: analysis of the lipid composition of cells and subcellular organelles by electrospray ionization mass spectrometry. Annu. Rev. Biochem. 83, 79–98. doi: 10.1146/annurev-biochem-060713-035324
Carreira, A. C., Santos, T. C., Lone, M. A., Zupančič, E., Lloyd-Evans, E., de Almeida, R. F. M., et al. (2019). Mammalian sphingoid bases: biophysical, physiological and pathological properties. Prog. Lipid Res. 75:100988. doi: 10.1016/j.plipres.2019.100988
Collins, J. R., Edwards, B. R., Fredricks, H. F., and Van Mooy, B. A. S. (2016). LOBSTAHS: an adduct-based lipidomics strategy for discovery and identification of oxidative stress biomarkers. Anal. Chem. 88, 7154–7162. doi: 10.1021/acs.analchem.6b01260
Cuadros, R., Montejo de Garcini, E., Wandosell, F., Faircloth, G., Fernández-Sousa, J. M., and Avila, J. (2000). The marine compound spisulosine, an inhibitor of cell proliferation, promotes the disassembly of actin stress fibers. Cancer Lett. 152, 23–29. doi: 10.1016/s0304-3835(99)00428-0
Danielewicz, M. A., Anderson, L. A., and Franz, A. K. (2011). Triacylglycerol profiling of marine microalgae by mass spectrometry. J. Lipid Res. 52, 2101–2108. doi: 10.1194/jlr.d018408
Dembitsky, V. M. (1996). Betaine ether-linked glycerolipids: chemistry and biology. Prog. Lipid Res. 35, 1–51. doi: 10.1016/0163-7827(95)00009-7
Ding, S., Lange, M., Lipp, J., Schwab, V. F., Chowdhury, S., Pollierer, M. M., et al. (2020). Characteristics and origin of intact polar lipids in soil organic matter. Soil. Biol. Biochem. 151:108045. doi: 10.1016/j.soilbio.2020.108045
Duan, J., and Merrill, A. H. Jr. (2015). 1-Deoxysphingolipids encountered exogenously and made de novo: dangerous mysteries inside an enigma. J. Biol. Chem. 290, 15380–15389. doi: 10.1074/jbc.r115.658823
Durisch-Kaiser, E., Klauser, L., Wehrli, B., and Schubert, C. (2005). Evidence of intense archaeal and bacterial methanotrophic activity in the Black sea water column. Appl. Environ. Microbiol. 71:8099. doi: 10.1128/aem.71.12.8099-8106.2005
Elling, F. J., Könneke, M., Mußmann, M., Greve, A., and Hinrichs, K.-U. (2015). Influence of temperature, pH, and salinity on membrane lipid composition and TEX86 of marine planktonic thaumarchaeal isolates. Geochim. Cosmochim. Acta 171, 238–255. doi: 10.1016/j.gca.2015.09.004
Eren, A. M., Borisy, G. G., Huse, S. M., and Mark Welch, J. L. (2014). Oligotyping analysis of the human oral microbiome. Proc. Natl. Acad. Sci. U.S.A. 111:E2875.
Ertefai, T. F., Fisher, M. C., Fredricks, H. F., Lipp, J. S., Pearson, A., Birgel, D., et al. (2008). Vertical distribution of microbial lipids and functional genes in chemically distinct layers of a highly polluted meromictic lake. Org. Geochem. 39, 1572–1588. doi: 10.1016/j.orggeochem.2008.07.009
Geiger, O., González-Silva, N., López-Lara, I. M., and Sohlenkamp, C. (2010). Amino acid-containing membrane lipids in bacteria. Prog. Lipid Res. 49, 46–60. doi: 10.1016/j.plipres.2009.08.002
Godchaux, W. III, and Leadbetter, E. R. (1980). Capnocytophaga spp. contain sulfonolipids that are novel in procaryotes. J. Bacteriol. 144, 592–602. doi: 10.1128/jb.144.2.592-602.1980
Grossi, V., Mollex, D., Vinçon-Laugier, A., Hakil, F., Pacton, M., and Cravo-Laureau, C. (2015). Mono- and dialkyl glycerol ether lipids in anaerobic bacteria: biosynthetic insights from the mesophilic sulfate reducer Desulfatibacillum alkenivorans PF2803T. Appl. Environ. Microbiol. 81:3157. doi: 10.1128/aem.03794-14
Han, X., Yang, K., and Gross, R. W. (2012). Multi-dimensional mass spectrometry-based shotgun lipidomics and novel strategies for lipidomic analyses. Mass Spectrom. Rev. 31, 134–178. doi: 10.1002/mas.20342
Hartmann, A. C., Petras, D., Quinn, R. A., Protsyuk, I., Archer, F. I., Ransome, E., et al. (2017). Meta-mass shift chemical profiling of metabolomes from coral reefs. Proc. Natl. Acad. Sci. U.S.A. 114:11685. doi: 10.1073/pnas.1710248114
Heaver, S. L., Johnson, E. L., and Ley, R. E. (2018). Sphingolipids in host–microbial interactions. Curr. Opin. Microbiol. 43, 92–99. doi: 10.1016/j.mib.2017.12.011
Hu, Q., Sommerfeld, M., Jarvis, E., Ghirardi, M., Posewitz, M., Seibert, M., et al. (2008). Microalgal triacylglycerols as feedstocks for biofuel production: perspectives and advances. Plant J. 54, 621–639.
Kang, K. B., Woo, S., Ernst, M., van der Hooft, J. J. J., Nothias, L.-F., da Silva, R. R., et al. (2020). Assessing specialized metabolite diversity of alnus species by a digitized LC–MS/MS data analysis workflow. Phytochemistry 173:112292. doi: 10.1016/j.phytochem.2020.112292
Kato, M., Adachi, K., Hajiro-Nakanishi, K., Ishigaki, E., Sano, H., and Miyachi, S. (1994). A betaine lipid from Pavlova lutheri. Phytochemistry 37, 279–280. doi: 10.1016/0031-9422(94)85041-0
Kato, M., Sakai, M., Adachi, K., Ikemoto, H., and Sano, H. (1996). Distribution of betaine lipids in marine algae. Phytochemistry 42, 1341–1345. doi: 10.1016/0031-9422(96)00115-x
Kharbush, J. J., Allen, A. E., Moustafa, A., Dorrestein, P. C., and Aluwihare, L. I. (2016). Intact polar diacylglycerol biomarker lipids isolated from suspended particulate organic matter accumulating in an ultraoligotrophic water column. Org. Geochem. 100, 29–41. doi: 10.1016/j.orggeochem.2016.07.008
Koopmans, M. P., Köster, J., Van Kaam-Peters, H. M. E., Kenig, F., Schouten, S., Hartgers, W. A., et al. (1996). Diagenetic and catagenetic products of isorenieratene: molecular indicators for photic zone anoxia. Geochim. Cosmochim. Acta 60, 4467–4496. doi: 10.1016/s0016-7037(96)00238-4
Kraal, P., Dijkstra, N., Behrends, T., and Slomp, C. P. (2017). Phosphorus burial in sediments of the sulfidic deep Black Sea: key roles for adsorption by calcium carbonate and apatite authigenesis. Geochim. Cosmochim. Acta 204, 140–158.
Kumar, K., Mella-Herrera, R. A., and Golden, J. W. (2010). Cyanobacterial heterocysts. Cold Spring Harb. Perspect. Biol. 2:a000315.
Kuypers, M. M. M., Sliekers, A. O., Lavik, G., Schmid, M., Jørgensen, B. B., Kuenen, J. G., et al. (2003). Anaerobic ammonium oxidation by anammox bacteria in the Black sea. Nature 422, 608–611. doi: 10.1038/nature01472
Law, K. P., and Zhang, C. L. (2019). Current progress and future trends in mass spectrometry-based archaeal lipidomics. Org. Geochem. 134, 45–61. doi: 10.1016/j.orggeochem.2019.04.001
Li, D., Halitschke, R., Baldwin, I. T., and Gaquerel, E. (2020). Information theory tests critical predictions of plant defense theory for specialized metabolism. Sci. Adv. 6:eaaz0381. doi: 10.1126/sciadv.aaz0381
Li, D., Heiling, S., Baldwin, I. T., and Gaquerel, E. (2016). Illuminating a plant’s tissue-specific metabolic diversity using computational metabolomics and information theory. Proc. Natl. Acad. Sci. U.S.A. 113:E7610.
Liu, X.-L., Leider, A., Gillespie, A., Gröger, J., Versteegh, G. J. M., and Hinrichs, K.-U. (2010). Identification of polar lipid precursors of the ubiquitous branched GDGT orphan lipids in a peat bog in Northern Germany. Org. Geochem. 41, 653–660. doi: 10.1016/j.orggeochem.2010.04.004
Lone, M. A., Santos, T., Alecu, I., Silva, L. C., and Hornemann, T. (2019). 1-Deoxysphingolipids. Biochim. Biophys. Acta 1864, 512–521.
López-Lara, I. M., Sohlenkamp, C., and Geiger, O. (2003). Membrane lipids in plant-associated bacteria: their biosyntheses and possible functions. Mol. Plant Microbe Interact. 16, 567–579. doi: 10.1094/mpmi.2003.16.7.567
Manske, A. K., Glaeser, J., Kuypers, M. M. M., and Overmann, J. (2005). Physiology and phylogeny of green sulfur bacteria forming a monospecific phototrophic assemblage at a depth of 100 meters in the Black sea. Appl. Environ. Microbiol. 71:8049. doi: 10.1128/aem.71.12.8049-8060.2005
Martin, P., Van Mooy, B. A. S., Heithoff, A., and Dyhrman, S. T. (2011). Phosphorus supply drives rapid turnover of membrane phospholipids in the diatom Thalassiosira pseudonana. ISME J. 5, 1057–1060. doi: 10.1038/ismej.2010.192
Martínez, O., and Reyes-Valdés, M. H. (2008). Defining diversity, specialization, and gene specificity in transcriptomes through information theory. Proc. Natl. Acad. Sci. U.S.A. 105:9709. doi: 10.1073/pnas.0803479105
Martínez-Morales, F., Schobert, M., López-Lara, I. M., and Geiger, O. (2003). Pathways for phosphatidylcholine biosynthesis in bacteria. Microbiology 149, 3461–3471. doi: 10.1099/mic.0.26522-0
Merrill, A. H. Jr. (2011). Sphingolipid and glycosphingolipid metabolic pathways in the era of sphingolipidomics. Chem.Rev. 111, 6387–6422. doi: 10.1021/cr2002917
Moore, E. K., Hopmans, E. C., Rijpstra, W. I. C., Villanueva, L., and Sinninghe Damsté, J. S. (2016). Elucidation and identification of amino acid containing membrane lipids using liquid chromatography/high-resolution mass spectrometry. Rapid Commun. Mass Spectrom. 30, 739–750. doi: 10.1002/rcm.7503
Neretin, L. N., Abed, R. M. M., Schippers, A., Schubert, C. J., Kohls, K., and Kuypers, M. M. M. (2007). Inorganic carbon fixation by sulfate-reducing bacteria in the Black sea water column. Environ. Microbiol. 9, 3019–3024. doi: 10.1111/j.1462-2920.2007.01413.x
Nothias, L.-F., Petras, D., Schmid, R., Dührkop, K., Rainer, J., Sarvepalli, A., et al. (2020). Feature-based molecular networking in the GNPS analysis environment. Nat. Methods 17, 905–908.
Overmann, J., Cypionka, H., and Pfennig, N. (1992). An extremely low-light adapted phototrophic sulfur bacterium from the Black sea. Limnol. Oceanogr. 37, 150–155. doi: 10.4319/lo.1992.37.1.0150
Peterse, F., Hopmans, E. C., Schouten, S., Mets, A., Rijpstra, W. I. C., and Sinninghe Damsté, J. S. (2011). Identification and distribution of intact polar branched tetraether lipids in peat and soil. Org. Geochem. 42, 1007–1015. doi: 10.1016/j.orggeochem.2011.07.006
Petras, D., Koester, I., Da Silva, R., Stephens, B. M., Haas, A. F., Nelson, C. E., et al. (2017). High-resolution liquid chromatography tandem mass spectrometry enables large scale molecular characterization of dissolved organic matter. Front. Mar. Sci. 4:405. doi: 10.3389/fmars.2017.00405
Petras, D., Minich, J. J., Cancelada, L. B., Torres, R. R., Kunselman, E., Wang, M., et al. (2021). Non-targeted tandem mass spectrometry enables the visualization of organic matter chemotype shifts in coastal seawater. Chemosphere 271:129450. doi: 10.1016/j.chemosphere.2020.129450
Pluskal, T., Castillo, S., Villar-Briones, A., and Orešič, M. (2010). MZmine 2: modular framework for processing, visualizing, and analyzing mass spectrometry-based molecular profile data. BMC Bioinformatics 11:395. doi: 10.1186/1471-2105-11-395
Popendorf, K. J., Lomas, M. W., and Van Mooy, B. A. S. (2011a). Microbial sources of intact polar diacylglycerolipids in the western North Atlantic ocean. Org. Geochem. 42, 803–811. doi: 10.1016/j.orggeochem.2011.05.003
Popendorf, K. J., Tanaka, T., Pujo-Pay, M., Lagaria, A., Courties, C., Conan, P., et al. (2011b). Gradients in intact polar diacylglycerolipids across the Mediterranean sea are related to phosphate availability. Biogeosciences 8, 3733–3745. doi: 10.5194/bg-8-3733-2011
Repeta, D. J., Simpson, D. J., Jorgensen, B. B., and Jannasch, H. W. (1989). Evidence for anoxygenic photosynthesis from the distribution of bacterio-chlorophylls in the Black sea. Nature 342, 69–72. doi: 10.1038/342069a0
Rethemeyer, J., Schubotz, F., Talbot, H. M., Cooke, M. P., Hinrichs, K.-U., and Mollenhauer, G. (2010). Distribution of polar membrane lipids in permafrost soils and sediments of a small high Arctic catchment. Org. Geochem. 41, 1130–1145. doi: 10.1016/j.orggeochem.2010.06.004
Rossel, P. E., Lipp, J. S., Fredricks, H. F., Arnds, J., Boetius, A., Elvert, M., et al. (2008). Intact polar lipids of anaerobic methanotrophic archaea and associated bacteria. Org. Geochem. 39, 992–999. doi: 10.1016/j.orggeochem.2008.02.021
Rush, D., Wakeham, S. G., Hopmans, E. C., Schouten, S., and Sinninghe Damsté, J. S. (2012). Biomarker evidence for anammox in the oxygen minimum zone of the Eastern Tropical North Pacific. Org. Geochem. 53, 80–87. doi: 10.1016/j.orggeochem.2012.02.005
Schubotz, F., Wakeham, S. G., Lipp, J. S., Fredricks, H. F., and Hinrichs, K.-U. (2009). Detection of microbial biomass by intact polar membrane lipid analysis in the water column and surface sediments of the Black sea. Environ. Microbiol. 11, 2720–2734. doi: 10.1111/j.1462-2920.2009.01999.x
Schubotz, F., Xie, S., Lipp, J. S., Hinrichs, K. U., and Wakeham, S. G. (2018). Intact polar lipids in the water column of the eastern tropical North Pacific: abundance and structural variety of non-phosphorus lipids. Biogeosciences 15, 6481–6501. doi: 10.5194/bg-15-6481-2018
Shannon, P., Markiel, A., Ozier, O., Baliga, N. S., Wang, J. T., Ramage, D., et al. (2003). Cytoscape: a software environment for integrated models of biomolecular interaction networks. Genome Res. 13, 2498–2504. doi: 10.1101/gr.1239303
Shevchenko, A., and Simons, K. (2010). Lipidomics: coming to grips with lipid diversity. Nat. Rev. Mol. Cell Biol. 11, 593–598. doi: 10.1038/nrm2934
Sinninghe Damsté, J. S., Schouten, S., and van Duin, A. C. T. (2001). Isorenieratene derivatives in sediments: possible controls on their distribution. Geochim. Cosmochim. Acta 65, 1557–1571. doi: 10.1016/s0016-7037(01)00549-x
Sinninghe Damsté, J. S., Strous, M., Rijpstra, W. I. C., Hopmans, E. C., Geenevasen, J. A. J., van Duin, A. C. T., et al. (2002). Linearly concatenated cyclobutane lipids form a dense bacterial membrane. Nature 419, 708–712. doi: 10.1038/nature01128
Sinninghe Damsté, J. S., Wakeham, S. G., Kohnen, M. E. L., Hayes, J. M., and de Leeuw, J. W. (1993). A 6,000–year sedimentary molecular record of chemocline excursions in the Black sea. Nature 362, 827–829. doi: 10.1038/362827a0
Smoot, M. E., Ono, K., Ruscheinski, J., Wang, P.-L., and Ideker, T. (2010). Cytoscape 2.8: new features for data integration and network visualization. Bioinformatics 27, 431–432. doi: 10.1093/bioinformatics/btq675
Sohlenkamp, C., and Geiger, O. (2016). Bacterial membrane lipids: diversity in structures and pathways. FEMS Microbiol. Rev. 40, 133–159. doi: 10.1093/femsre/fuv008
Sohlenkamp, C., López-Lara, I. M., and Geiger, O. (2003). Biosynthesis of phosphatidylcholine in bacteria. Prog. Lipid Res. 42, 115–162. doi: 10.1016/s0163-7827(02)00050-4
Sollai, M., Villanueva, L., Hopmans, E. C., Reichart, G.-J., and Sinninghe Damsté, J. S. (2019). A combined lipidomic and 16S rRNA gene amplicon sequencing approach reveals archaeal sources of intact polar lipids in the stratified Black sea water column. Geobiology 17, 91–109. doi: 10.1111/gbi.12316
Steen, A. D., Kusch, S., Abdulla, H. A., Cakić, N., Coffinet, S., Dittmar, T., et al. (2020). Analytical and computational advances, opportunities, and challenges in marine organic biogeochemistry in an era of “Omics”. Front. Mar. Sci. 7:718. doi: 10.3389/fmars.2020.00718
Sturt, H. F., Summons, R. E., Smith, K., Elvert, M., and Hinrichs, K.-U. (2004). Intact polar membrane lipids in prokaryotes and sediments deciphered by high-performance liquid chromatography/electrospray ionization multistage mass spectrometry—new biomarkers for biogeochemistry and microbial ecology. Rapid Commun. Mass Spectrom. 18, 617–628. doi: 10.1002/rcm.1378
Suominen, S., Dombrowski, N., Sinninghe Damsté, J. S., and Villanueva, L. (2020a). A diverse uncultivated microbial community is responsible for organic matter degradation in the Black sea sulphidic zone. Environ. Microbiol. doi: 10.1111/1462-2920.14902
Suominen, S., Doorenspleet, K., Sinninghe Damsté, J. S., and Villanueva, L. (2020b). Microbial community development on model particles in the deep sulfidic waters of the Black sea. Environ. Microbiol. doi: 10.1111/1462-2920.15024
Thompson, G. A. (1996). Lipids and membrane function in green algae. Biochim. Biophys. Acta 1302, 17–45. doi: 10.1016/0005-2760(96)00045-8
Van Mooy, B. A. S., and Fredricks, H. F. (2010). Bacterial and eukaryotic intact polar lipids in the eastern subtropical South Pacific: water-column distribution, planktonic sources, and fatty acid composition. Geochim. Cosmochim. Acta 74, 6499–6516. doi: 10.1016/j.gca.2010.08.026
Van Mooy, B. A. S., Fredricks, H. F., Pedler, B. E., Dyhrman, S. T., Karl, D. M., Koblížek, M., et al. (2009). Phytoplankton in the ocean use non-phosphorus lipids in response to phosphorus scarcity. Nature 458, 69–72. doi: 10.1038/nature07659
Vestal, J. R., and White, D. C. (1989). Lipid analysis in microbial ecology. Bioscience 39, 535–541. doi: 10.2307/1310976
Volkman, J. K., Jeffrey, S. W., Nichols, P. D., Rogers, G. I., and Garland, C. D. (1989). Fatty acid and lipid composition of 10 species of microalgae used in mariculture. J. Exp. Mar. Biol. Ecol. 128, 219–240. doi: 10.1016/0022-0981(89)90029-4
Wakeham, S. G., Amann, R., Freeman, K. H., Hopmans, E. C., Jørgensen, B. B., Putnam, I. F., et al. (2007). Microbial ecology of the stratified water column of the Black sea as revealed by acomprehensive biomarker study. Org. Geochem. 38, 2070–2097. doi: 10.1016/j.orggeochem.2007.08.003
Wakeham, S. G., and Lee, C. (2019). Limits of our knowledge, part 2: selected frontiers in marine organic biogeochemistry. Mar. Chem. 212, 16–46. doi: 10.1016/j.marchem.2019.02.005
Wakeham, S. G., Turich, C., Schubotz, F., Podlaska, A., Li, X. N., Varela, R., et al. (2012). Biomarkers, chemistry and microbiology show chemoautotrophy in a multilayer chemocline in the Cariaco Basin. Deep Sea Res. 1 Oceanogr. Res. Pap. 63, 133–156. doi: 10.1016/j.dsr.2012.01.005
Walker, A., Pfitzner, B., Harir, M., Schaubeck, M., Calasan, J., Heinzmann, S. S., et al. (2017). Sulfonolipids as novel metabolite markers of Alistipes and Odoribacter affected by high-fat diets. Sci. Rep. 7:11047.
Wang, M., Carver, J. J., Phelan, V. V., Sanchez, L. M., Garg, N., Peng, Y., et al. (2016). Sharing and community curation of mass spectrometry data with global natural products social molecular networking. Nat. Biotechnol. 34, 828–837.
Warren, C. R. (2020). Soil microbial populations substitute phospholipids with betaine lipids in response to low P availability. Soil Biol. Biochem. 140:107655. doi: 10.1016/j.soilbio.2019.107655
Watrous, J., Roach, P., Alexandrov, T., Heath, B. S., Yang, J. Y., Kersten, R. D., et al. (2012). Mass spectral molecular networking of living microbial colonies. Proc. Natl. Acad. Sci. U.S.A. 109:E1743.
Winnikoff, J. R., Glukhov, E., Watrous, J., Dorrestein, P. C., and Gerwick, W. H. (2014). Quantitative molecular networking to profile marine cyanobacterial metabolomes. J. Antibiot. 67, 105–112. doi: 10.1038/ja.2013.120
Wolk, C. P., Ernst, A., and Elhai, J. (1994). “Heterocyst metabolism and development,” in The Molecular Biology of Cyanobacteria, ed. D. A. Bryant (Dordrecht: Springer), 769–823. doi: 10.1007/0-306-48205-3_27
Wörmer, L., Elvert, M., Fuchser, J., Lipp, J. S., Buttigieg, P. L., Zabel, M., et al. (2014). Ultra-high-resolution paleoenvironmental records via direct laser-based analysis of lipid biomarkers in sediment core samples. Proc. Natl. Acad. Sci. U.S.A. 111, 15669–15674. doi: 10.1073/pnas.1405237111
Yadav, S., Villanueva, L., Bale, N., Koenen, M., Hopmans, E. C., and Damsté, J. S. S. (2020). Physiological, chemotaxonomic and genomic characterization of two novel piezotolerant bacteria of the family Marinifilaceae isolated from sulfidic waters of the Black sea. Syst. Appl. Microbiol. 43:126122. doi: 10.1016/j.syapm.2020.126122
Keywords: microbial membrane lipids, lipidome, Black Sea, molecular network, information theory, mass spectometry, biomarker, intact polar lipids (IPLs)
Citation: Ding S, Bale NJ, Hopmans EC, Villanueva L, Arts MGI, Schouten S and Sinninghe Damsté JS (2021) Lipidomics of Environmental Microbial Communities. II: Characterization Using Molecular Networking and Information Theory. Front. Microbiol. 12:659315. doi: 10.3389/fmicb.2021.659315
Received: 27 January 2021; Accepted: 18 June 2021;
Published: 12 July 2021.
Edited by:
Marcelino T. Suzuki, Sorbonne Universités, FranceReviewed by:
Florence Schubotz, University of Bremen, GermanyJames Robert Collins, Portland State University, United States
Copyright © 2021 Ding, Bale, Hopmans, Villanueva, Arts, Schouten and Sinninghe Damsté. This is an open-access article distributed under the terms of the Creative Commons Attribution License (CC BY). The use, distribution or reproduction in other forums is permitted, provided the original author(s) and the copyright owner(s) are credited and that the original publication in this journal is cited, in accordance with accepted academic practice. No use, distribution or reproduction is permitted which does not comply with these terms.
*Correspondence: Su Ding, c3UuZGluZ0BuaW96Lm5s