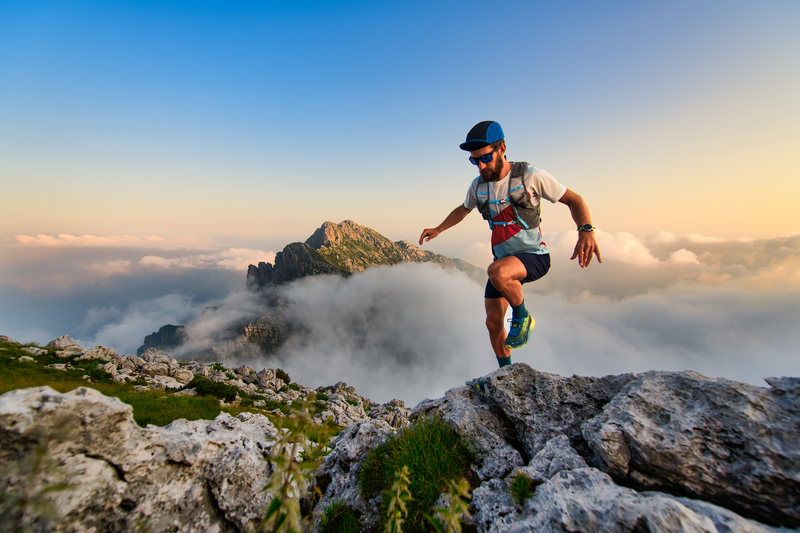
95% of researchers rate our articles as excellent or good
Learn more about the work of our research integrity team to safeguard the quality of each article we publish.
Find out more
ORIGINAL RESEARCH article
Front. Microbiol. , 31 March 2021
Sec. Evolutionary and Genomic Microbiology
Volume 12 - 2021 | https://doi.org/10.3389/fmicb.2021.657887
This article is part of the Research Topic Viruses, Genetic Exchange, and the Tree of Life, Volume II View all 6 articles
The members of the family Iridoviridae are large, double-stranded DNA viruses that infect various hosts, including both vertebrates and invertebrates. Although great progress has been made in genomic and phylogenetic analyses, the adequacy of the existing criteria for classification within the Iridoviridae family remains unknown. In this study, we redetermined 23 Iridoviridae core genes by re-annotation, core-pan analysis and local BLASTN search. The phylogenetic tree based on the 23 re-annotated core genes (Maximum Likelihood, ML-Tree) and amino acid sequences (composition vector, CV-Tree) were found to be consistent with previous reports. Furthermore, the information provided by synteny analysis and codon usage preference (relative synonymous codon usage, correspondence analysis, ENC-plot and Neutrality plot) also supports the phylogenetic relationship. Collectively, our results will be conducive to understanding the genera demarcation within the Iridoviridae family based on genomic synteny and component (codon usage preference) and contribute to the existing taxonomy methods for the Iridoviridae family.
Members within the family Iridoviridae (recently designated iridovirids to avoid confusion between members of this family and members of the genus Iridovirus with the same name) are nucleocytoplasmic, large double-stranded DNA (dsDNA) viruses, with diameter ranging from 120 to 300 nm and encoding between 100 and 200 putative proteins; morphologically, they are characterized by a DNA-protein core surrounded by an internal lipid membrane and an icosahedral protein capsid (Chinchar et al., 2017b). Owing to their wide distribution in nature, a large and diverse array of vertebrates and invertebrates can be infected by iridovirids, including insects, amphibians, reptiles and fish (King et al., 2011). The clinical manifestations of iridovirid infections vary greatly from mild symptoms to death, depending on the species. The high morbidity and mortality exhibited by certain species, such as the mandarin fish (Siniperca chuatsi), have severely impacted modern aquaculture (Liu et al., 2018). Human activities can also facilitate the spread of certain infectious diseases, as seen in the case of the amphibian population, where tiger salamanders and Chinese giant salamanders (Andrias davidianus) have been affected (Whittington et al., 2010; Geng et al., 2011).
With the identification of SHIV and CQIV (Xu et al., 2016; Qiu et al., 2017), as well as the phylogenetic analysis of the new isolates, a new genus (Decapodiridovirus) was established, leading to an increase in the number of genera under the family Iridoviridae from five to six. This includes Iridovirus, Chloriridovirus and Decapodiridovirus which infect invertebrates, Lymphocystivirus and Megalocytivirus which only affect bony fish, and Ranavirus which has a wide host spectrum (fish, amphibians and reptiles) (Chinchar et al., 2017a). Initially, the iridovirids were classified based on their particle size, host preference, GC content, the presence of a DNA methyltransferase, as well as the existence of major capsid protein (MCP) (King et al., 2011). With the development of complete genome sequencing, phylogenetic analysis based on pan-genomic data provided an insight into the criteria for virus taxonomy. As a result, a set of essential genes conserved among all viruses in the family Iridoviridae was defined (Eaton et al., 2010). However, while only limited sequences of iridovirids have been obtained, new iridovirids are still continuously being discovered, thus making it difficult to distinguish and classify the viruses precisely. Additionally, even though the core set of genes was conserved, some unique genes only exist within specific species (Eaton et al., 2007). Furthermore, it is still not clear whether the current criteria are sufficient to determine the genera of newly discovered viruses. Thus, deeper and more comprehensive insights into the existing classification approach are urgently needed for a more comprehensive and objective differentiation.
Owing to the degeneracy of the genetic code, an amino acid may correspond to more than one codon; therefore, most of the codons are synonymous, while the frequency of occurrence of those are unbalanced among varied genes and most of organisms, which is referred as codon usage bias (Ikemura, 1981, 1985; Chen et al., 2014). Codon usage preference is a widespread phenomenon in nature and can be found in viruses, prokaryotes, eukaryotes and even in different genes within the same organism (Greenbaum et al., 2008; Rahman et al., 2018). An increasing number of studies have suggested that codon usage is influenced by multiple factors, such as mutation pressure, natural selection, GC content, tRNA abundance and protein secondary motifs, among others. Of these factors, the former two play a crucial role (Cristina et al., 2015; Wang et al., 2016; Ur Rahman et al., 2017; Rahman et al., 2018). The codon usage pattern has a strong connection with virus survival, adaptation, evolution and the control of host immune system (Butt et al., 2014; Rahman et al., 2018). Studying this codon usage bias may thus provide more information regarding virus molecular evolution, providing a further insight into virus taxonomy and phylogenetic analysis.
In the present study, phylogenetic analysis, synteny analysis and comprehensive codon usage analysis were performed based on a total of 53 iridovirids. We found that the genomic synteny relationship and codon preference within the family Iridoviridae may provide a new reference factor for the virus classification.
The complete genome sequences of 53 iridovirids spanning 39 years from 1979 to 2018 used in this study (Supplementary Table 1) were retrieved from the National Center for Biotechnology (NCBI) GenBank database1. Detailed information of these viruses is listed in Supplementary Table 1, including the sequence name, host information, country of origin, year of isolation and accession number.
The annotation of obtained virus genome was performed using Prokka [version 1.14.5; Seemann (2014); settings: –kingdom Viruses, remaining settings: default]. Core genes, which are highly conserved and shared by all iridovirids (Eaton et al., 2007; Jancovich et al., 2010), play a fundamental role in revealing the phylogenetic relationship among the species. With the emergence of high-throughput sequencing, there has been a paradigm shift in microbial genomics studies to a large-scale pan-genome analysis. Thus, the pan-genome detection of core genes was preferentially performed by PanX (Ding et al., 2018) for the published 53 completed iridovirids genomes (settings: –ngbk, –cg 0.9, –nsl, which mean sequence identity threshold and disable long branch splitting, respectively; remaining settings: default). Meanwhile, the remaining core genes were manually screened by local BLASTN search against the GenBank database.
Composition vector phylogenetic tree (CV-Tree) is considered a faithful and an objective method to deduce evolutionary relatedness and has previously been successfully applied to viruses, chloroplasts and fungi (Wu et al., 2017; Mao and Wang, 2019). Furthermore, it also has the advantages of being whole-genome-based and alignment free. To gain insight into the genetic variability and evolution of the viruses in different genera, the CVTree web server was employed according to the user’s manual. Briefly, FASTA formatted files containing all the genomic amino acid sequences were directly submitted to CVTree web server (version 32) and the K-value was set at 5. Subsequently, Evolview v3 (Subramanian et al., 2019) was recruited for the visualization and annotation of the phylogenetic tree.
Prior to the construction of the phylogenetic tree, different multiple sequence alignments based on the core genes of the iridovirids were generated by MUSCLE program (version 3.8.31) (Edgar, 2004). Subsequently, the aligned sequences were submitted to PhyloSuite (Zhang et al., 2020) for concatenation. Finally, Maximum Likelihood (ML) phylogenetic trees were constructed using MEGA 6.0.
Synteny analysis was implemented using MCScanX (Wang et al., 2012) for all iridovirid genera, including Iridovirus, Chloriridovirus, Decapodiridovirus, Lymphocystivirus, Megalocytivirus, and Ranavirus. Prior to analysis, the amino acid sequences of each virus was compared against itself and other members using BLASTP (version 2.8.1). The acquired results were then filtered according to identity >50%. Finally, the dot-plotter picture was drawn (refer to the software manual)3.
Relative synonymous codon usage (RSCU) was first proposed by Sharp and Li (1986) and helps to remove the influence caused by amino acid composition on codon usage. Thus, it has been widely used for evaluating the codon usage preference between genes. To investigate the codon usage bias pattern of indicated viruses, the codon nucleotide sequences of core genes were subjected to software CodonW. Meanwhile, the RSCU values of all codons in them were calculated. A RSCU value higher or lower than 1.0 would suggest a positive or negative bias toward that codon, respectively. In contrast, a RSCU value that is nearly equal to 1.0 indicates that the codons were chosen equally and randomly.
Correspondence analysis (COA), a type of multivariate statistical analysis, not only displays the sets of rows and columns in specific dataset with geometrical representation (Wong et al., 2010), it also shows the major variable trends and helps to detect the relationships between variables and samples. In this study, COA based on RSCU values was performed by means of the CodonW software. Briefly, each coding region of the virus was represented as a 59-dimensional vector and every dimension corresponds to the RSCU value for each codon (excluding AUG, UGG and stop codons). The visualized graphics were drawn by R ggplot2 package.
Effective number of codons (ENC) was proposed by Wright (1990) and has been widely used to determine the codon bias. The ENC value ranges from 20 (only one specific codon is recruited for each amino acid) to 61 (the recruitment percentage for all synonymous codons is equal). ENC-plot (ENC vs CG3s) is an efficient and visual method to determine whether the codon usage bias is caused by mutation only (the corresponding points would lie on or be close to the standard curve) or by multiple factors such as natural selection (points would be distributed away from the expected curve). The expected ENC values were calculated following the method documented by Singh et al. (2016).
Neutral evolution analysis (Neutrality plot analysis) was used to determine and compare between the extent of the influence exerted by natural selection and mutation pressure on the codon usage patterns of coding segments within the genera. Briefly, the GC12 values of synonymous codons were plotted against the GC3 values (Butt et al., 2016). The values of GC12 and GC3 of iridovirids were calculated by the CodonW program and subjected to neutrality plot analysis.
The Parity rule 2 (PR2) plot analysis was used to identify the effects of natural selection and mutation on codon usage bias, which is characterized by the value of AT-bias [A3/(A3 + T3)] as the ordinate and GC-bias [G3/(G3 + C3)] as the abscissa (Sueoka, 1995). A3, T3, G3 and C3 correspond to the A, T, G, and C content at the third position of four-codon amino acids, respectively. In this plot, the center, where both coordinates are 0.5 (means A = T and G = C), indicates that mutation and selection have an equal effect on codon usage (Sueoka, 1995, 1999).
To clarify the genetic composition of 53 members from the family Iridovirdae and normalize the differences caused by various sequencing and annotation platforms, Prokka program was employed. After re-annotation, core-pan analysis and BLASTN search were conducted for the detection of core genes within the Iridovirdae family. As a result, a total of 26 Iridoviridae core genes were detected in most of the 53 iridovirids [the Iridoviridae core genes were identified by Eaton et al. (2007)], although only 23 of them were shared by all 53 iridovirids. The genes coding for ribonucleotide reductase small subunit and proliferating cell nuclear antigen were absent within CQIV and SHIV (CQIV_MF197913/SHIV_MF599468) and serine-threonine protein kinase was absent within AMIV (AMIV_KF938901).
To understand the probable genetic relationships within the Iridoviridae family, a genome-wide phylogenetic analysis was performed by using CVTree web server based on genomic amino acid sequences of the Iridoviridae family members (Figure 1A). The result (CV-Tree) showed that the 53 viruses could be classified into the 6 genera: Iridovirus, Chloriridovirus, Decapodiridovirus, Megalocytivirus, Lymphocystivirus, and Ranavirus. The genera Ranavirus could be further divided into four subgroups: SGIV-like, EHNV-like, CMTV-like and FV3-like.
Figure 1. Phylogenetic analysis of the Iridoviridae family. (A) Phylogenetic tree based on genomic amino acid sequences constructed using composition vector method (CV-Tree). (B) Phylogenetic tree based on the 23 Iridoviridae core genes constructed using maximum likelihood method (ML-Tree).
Owing to the different alignment methods or insufficient data, the branching order of the Iridoviridae family is often inconsistent between genomic papers (Jancovich et al., 2003; Huang et al., 2009). Thus, to clarify the evolutionary relationships within the family Iridoviridae, a phylogenetic analysis (ML-Tree) based on the core-genes was conducted (Figure 1B). The resulting ML-phylogenetic tree exhibited similar distribution pattern of the given viruses, as well as a similar subgroup classification within Ranaviruses.
To clarify the linear relationships of the Iridoviridae family members, MCScanX was employed to obtain a full landscape of the 53 virus genomes. After screening (identity threshold set as 50%), the dot-plotter results showed that members within the same genus shared certain regions of collinearity as compared to those in different genera and there was an obvious demarcation between the different genera (Figure 2). The specific collinearity in each genus was summarized in Table 1.
To clarify the patterns of synonymous codon usage of members in Iridoviridae family, relative synonymous codon usage (RSCU) analysis of 59 codons was performed to describe the codon usage bias among different genera. All values and large variances obtained were highlighted in the Supplementary Table 1 and were further corroborated by the summary of the hierarchical clustering of RSCU values (Figure 3). Among all the codons, UUU (Phe) was the most common one that was shared by all 53 iridovirids. Viruses in the genus Decapodiridovirus were found to prefer U- and A- ending codons and the RSCU values of CQIV and SHIV were identical. Similarly, viruses in Iridovirus, also exhibited a preference for U- and A- ending codons. Although, the topology of the cluster analysis of these viruses was generally consistent with the results of the phylogenetic analysis, there were still some interesting observations. For example, AMIV and IIV3 that were clustered into the same group in the ML-phylogenetic tree exhibited two different codon usage bias patterns, of which a total of 11 amino acids (Leu, Val, Ser, Pro, Thr, Tyr, Gln, Lys, Glu, Arg, and Gly) were involved. In addition, the codon usage bias of GIV and SGIV was slightly different as compared to other members in the Ranavirus genus. For example, GIV and SGIV preferred using AGA (RSCU values: 2.22 and 2.24) to encode for arginine, while the other members preferred AGG. Similar differences were also observed in the codon choices for Leu, lle, Ser, Thr, Ala, Gln, Lys, Glu, and Gly. Different preferences in codon usage within the same genus may suggest that the virus codon preference is not fully consistent with phylogenetic analysis.
Figure 3. Heat map of RSCU values of 53 iridovirids. Each column represents a codon and each row represents a different virus species.
As a multivariate statistical method, correspondence analysis (COA) can minimize the effect caused by amino acid composition and reduce the dimensions of datasets to obtain awareness of multiple variables. To detect the trends in codon usage variation, COA was implemented based on RSCU values. The genera Iridovirus, Decapodiridovirus and Lymphocystivirus displayed similar distribution pattern, with all the members in each genus forming a main cluster (Figures 4A,C,D). However, in the Chloriridovirus genus, AMIV and IIV3 were widely spread in the second, third and fourth quadrants, unlike other members which were grouped together (Figure 4B). Interestingly, a similar phenomenon was observed for Megalocytivirus and Ranavirus. Specifically, SDDV in Megalocytivirus formed an isolated cluster away from other Megalocytivirus members (Figure 4E). Similarly, the distribution of the synonymous codon usage patterns in Ranavirus showed a division into two clusters, one composed of GIV and SGIV and the other formed by the remaining viruses, including CMTV-like, EHNV-like, and FV3-like (Figure 4F). These results were consistent with the landscape presented in the ML-phylogenetic tree (Figure 1B), indicating that codon usage bias may reflect evolutionary relationships to some extent.
Figure 4. Correspondence analysis of the synonymous codon usage in the Iridoviridae family. The analysis was based on the RSCU value of the 59 synonymous codons. Green circles suggest a high dispersion degree among the points inside the circle, while red circles indicate that points inside the circle have a high degree of dispersion as compared to those outside the circle. (A) Iridovirus, (B) Chloriridovirus, (C) Decapodiridovirus, (D) Lymphocystivirus, (E) Megalocytivirus, and (F) Ranavirus.
To investigate the factors affecting codon usage patterns among the coding sequences in the Iridoviridae family, an ENC-GC3 plot was generated. This was used to evaluate the codon usage pattern is dominated by mutational bias or selection pressure (Wright, 1990; Wu et al., 2015). In the ENC-GC3 plot, the points that lie on or close to the curve represent that the genetic evolution is only affected by mutational pressure. Conversely, the points below the curve indicate that the codon usage pattern is still subjected to natural selection. The results showed that the majority of the points fell beneath the expected curve, except a few points that were located on or close to the curve, indicating that overall, codon usage bias was also shaped by the presence of natural selection rather than by mutational pressure alone (Figure 5). Interestingly, the distribution pattern of Chloriridovirus, Megalocytivirus and Ranavirus were consistent with the COA results and their points were spread out while being clearly divided into two clusters (AMIV and IIV3 vs others; SDDV vs others; GIV and SGIV vs others). Thus, it is reasonable to infer that codon usage pattern is associated with evolutionary relationships.
Figure 5. ENC–GC3 plot of 53 Iridoviridae viruses. The solid line indicates the expected curve of ENC vs GC3 only under mutational pressure. Points on or close to the expected curve mean that the bias is caused by mutation pressure, while points below the curve indicate the presence of other influential factors such as natural selection. Green circles suggest a high dispersion degree among points inside the circle, while red circles indicate that points inside the circle have a high degree of dispersion as compared to those outside the circle. (A) Iridovirus, (B) Chloriridovirus, (C) Decapodiridovirus, (D) Lymphocystivirus, (E) Megalocytivirus, and (F) Ranavirus.
In order to investigate the contribution of mutation pressure and natural selection, a neutrality plot analysis was produced based on GC12 against GC3. The neutrality plot revealed that the correlation between GC12 and GC3 values among all the genera of the Iridoviridae family was relatively low (r2 = 0.14) (Figure 6). The slope of regression line was calculated to be 0.18, indicating that mutation pressure and natural selection accounted for 18 and 82%, respectively. Thus, both mutation bias and natural selection had influence over the codon usage pattern and the latter was dominant. In addition, viruses in the genera Choriridovirus, Megalocytivirus and Ranavirus clearly gathered into two clusters, which was consistent with the results of the COA and ENC-GC3 plot.
Figure 6. Neutrality plot analysis based on GC12 against GC3 of 53 Iridoviridae viruses. The blue line is the linear regression of GC12 against GC3 (r2 = 0.14). Green circles suggest a high dispersion degree among points inside the circle, while red circles indicate that points inside the circle have a high degree of dispersion as compared to those outside the circle. (A) Iridovirus, (B) Chloriridovirus, (C) Decapodiridovirus, (D) Lymphocystivirus, (E) Megalocytivirus, and (F) Ranavirus.
To further analyze the influence of highly biased gene restriction on codon usage pattern, PR2 bias plots were conducted by comparing the relationships between the AT and GC contents in four-codon sequences (Ala, Arg, Gly, Leu, Pro, Ser, Thr, and Val). The center of the plot (both coordinates are 0.5) is the place where A = T and C = G, suggesting that the impact of mutation pressure and natural selection are equal. In the present study, A3/(A3 + T3) and G3/(G3 + C3) were plotted as the ordinate and abscissa, respectively (Figure 7). Across all six genera, the points were clustered into one group and also spread out in all four quadrants (A≠T, G≠C), denoting the presence of different driving forces other than mutation pressure, such as natural selection, and that the influence of each factor was not equal.
Figure 7. PR2 plot analysis of the viruses within the indicated genera. The positions of the points were based on the AT and GC bias in the third codon position. (A) Iridovirus, (B) Chloriridovirus, (C) Decapodiridovirus, (D) Lymphocystivirus, (E) Megalocytivirus, and (F) Ranavirus.
Initially, owing to the characteristics of highly conserved variable domains, the major capsid protein (MCP) was thought to be reliable for the evolutionary analysis of iridovirids (Tidona et al., 1998). However, with the deepening of our understanding of iridovirid genomics, there has also been increasing evidence that phylogenetic analysis based solely on MCP may not be sufficiently comprehensive. Developments in the evolutionary analysis of iridovirids were made in 2007 following the re-analysis of 12 different genomes that found 26 core-set genes across all iridoviruses (Eaton et al., 2007). These core genes are typically conserved and associated with the steps involved in virus infection such as virulence, replication and gene expression (Eaton et al., 2007; Jancovich et al., 2015). Owing to the common access to the genome sequences of iridovirids, information from newer studies about molecular function could be updated over time, and may provide additional insights into phylogenetic analysis. Some core genes might have been exchanged during evolution, bringing the number of core genes down to 24. For example, genes coding for deoxynucleotide reductase and small subunit of ribonucleotide reductase were absent in European Chub Iridovirus and Shrimp hemocyte iridescent virus, respectively (Qiu et al., 2018). Interestingly, in the present study, only 23 core genes were shared by all the iridovirids studied. Furthermore, the phylogenetic analysis based on these core genes showed a similar map of genus demarcation as compared with previous reports (Chinchar et al., 2017b; İnce et al., 2018). Thus, it is reasonable to infer that the definition of core genes might need to be re-considered.
Currently, the co-linear arrangement analysis among viruses is mainly dependent on Java-Dotter (JDotter), a widely used method to visualize linear relationships and genomic structural changes. However, it is best utilized with a limited number of virus samples and would be time-consuming for a sample size of more than 10. Consequently, in order to obtain an all-inclusive understanding of collinear relationship among 53 iridovirids, BLASTP and MCScanX were employed. According to the description of ICTV (file code: 2018.007D), the members between different genera should share less than 50% amino acid sequence identity. MCScanX can visualize this genus demarcation criteria by the preliminary screening (identity > 50%). Briefly, the corresponding box would be blank (both viruses share less than 50% identity) for those viruses failed screening (Figure 2). Collectively, MCScanX shows more advantages as compared to Java-Dotter, such as by being faster, capable of analyzing more samples (over 50) and allowing for visualization of the genus demarcation criteria. To the best of our knowledge, this is the first study that applied MCScanX in the analysis of virus genomes.
According to the description of ICTV (file code: 2018.007D), the species demarcation mainly depends on following features: genomic component (genomic size and G+C content), phylogenetic relatedness and a co-linear arrangement of genes. However, the information provided by these features is relatively limited. In current study, we found that the characteristics of codon usage preference can provide more evidence, which were consistent with the results of phylogenetic and synteny analysis. As a result, the 53 iridovirids from 6 genera can be divided into the following categories: first, Decapodiridovirus, where members shared similar content and low degree of dispersion (COIV and SHIV); second, Iridovirus and Lymphocystivirus, where members shared different content and high degree of dispersion (IIV31 and IIV6; LDVSa, LCDVC, and LCDV1); third, Chloriridovirus, Megalocytivirus and Ranavirus, where members shared different content and high degree of dispersion as compared to their counterparts within the same genus (AMIV and IIV3; SDDV; GIV and SGIV). Interestingly, codon usage preference was consistent with the results of phylogenetic and synteny analysis (Table 2), which provides more information about genomic component than genomic size or G+C content. Thus, it is likely that the discovery of this phenomenon will be able contribute to the existing research on virus taxonomy.
Table 2. Comparison of codon usage preference, synteny analysis and phylogenetic analysis between different genera in the family Iridoviridae.
The datasets presented in this study can be found in online repositories. The names of the repository/repositories and accession number(s) can be found in the article/Supplementary Material.
ZY, ZD, JW, and WZ: conceptualization. ZY, ZD, JW, WZ, and YG: methodology. ZY, YG, and MZ: software. CG, LF, MH, QX, and WX: formal analysis. LH, QY, JH, and XY: investigation. ZY and ZD: writing–original draft preparation. JW and WZ: writing–review and editing. ZY: funding acquisition. All authors contributed to the article and approved the submitted version.
This work was supported by “Luzhou Municipal People’s Government–Southwest Medical University” science and technology strategic cooperation project (2020LZXNYDJ45).
The authors declare that the research was conducted in the absence of any commercial or financial relationships that could be construed as a potential conflict of interest.
The Supplementary Material for this article can be found online at: https://www.frontiersin.org/articles/10.3389/fmicb.2021.657887/full#supplementary-material
Butt, A. M., Nasrullah, I., Qamar, R., and Tong, Y. (2016). Evolution of codon usage in Zika virus genomes is host and vector specific. Emerg. Microbes Infect. 5:e107. doi: 10.1038/emi.2016.106
Butt, A. M., Nasrullah, I., and Tong, Y. (2014). Genome-wide analysis of codon usage and influencing factors in chikungunya viruses. PLoS One 9:e90905. doi: 10.1371/journal.pone.0090905
Chen, H., Sun, S., Norenburg, J. L., and Sundberg, P. (2014). Mutation and selection cause codon usage and bias in mitochondrial genomes of ribbon worms (Nemertea). PLoS One 9:e85631. doi: 10.1371/journal.pone.0085631
Chinchar, V. G., Hick, P., Ince, I. A., Jancovich, J. K., Marschang, R., Qin, Q., et al. (2017a). ICTV virus taxonomy profile: Iridoviridae. J. Gen. Virol. 98, 890–891.
Chinchar, V. G., Waltzek, T. B., and Subramaniam, K. (2017b). Ranaviruses and other members of the family Iridoviridae: their place in the virosphere. Virology 511, 259–271. doi: 10.1016/j.virol.2017.06.007
Cristina, J., Moreno, P., Moratorio, G., and Musto, H. (2015). Genome-wide analysis of codon usage bias in Ebolavirus. Virus Res. 196, 87–93. doi: 10.1016/j.virusres.2014.11.005
Ding, W., Baumdicker, F., and Neher, R. A. (2018). panX: pan-genome analysis and exploration. Nucleic Acids Res. 46:e5. doi: 10.1093/nar/gkx977
Eaton, H. E., Metcalf, J., Penny, E., Tcherepanov, V., Upton, C., and Brunetti, C. R. (2007). Comparative genomic analysis of the family Iridoviridae: re-annotating and defining the core set of iridovirus genes. Virol. J. 4:11.
Eaton, H. E., Ring, B. A., and Brunetti, C. R. (2010). The genomic diversity and phylogenetic relationship in the family Iridoviridae. Viruses 2, 1458–1475. doi: 10.3390/v2071458
Edgar, R. C. (2004). MUSCLE: a multiple sequence alignment method with reduced time and space complexity. BMC Bioinformatics 5:113. doi: 10.1186/1471-2105-5-113
Geng, Y., Wang, K. Y., Zhou, Z. Y., Li, C. W., Wang, J., He, M., et al. (2011). First report of a ranavirus associated with morbidity and mortality in farmed Chinese giant salamanders (Andrias davidianus). J. Comp. Pathol. 145, 95–102. doi: 10.1016/j.jcpa.2010.11.012
Greenbaum, B. D., Levine, A. J., Bhanot, G., and Rabadan, R. (2008). Patterns of evolution and host gene mimicry in influenza and other RNA viruses. PLoS Pathog. 4:e1000079. doi: 10.1371/journal.ppat.1000079
Huang, Y., Huang, X., Liu, H., Gong, J., Ouyang, Z., Cui, H., et al. (2009). Complete sequence determination of a novel reptile iridovirus isolated from soft-shelled turtle and evolutionary analysis of Iridoviridae. BMC Genomics 10:224. doi: 10.1186/1471-2164-10-224
Ikemura, T. (1981). Correlation between the abundance of Escherichia coli transfer RNAs and the occurrence of the respective codons in its protein genes. J. Mol. Biol. 146, 1–21. doi: 10.1016/0022-2836(81)90363-6
Ikemura, T. (1985). Codon usage and tRNA content in unicellular and multicellular organisms. Mol. Biol. Evol. 2, 13–34.
İnce, İA., Özcan, O., Ilter-Akulke, A. Z., Scully, E. D., and Özgen, A. (2018). Invertebrate Iridoviruses: a glance over the last decade. Viruses 10:161. doi: 10.3390/v10040161
Jancovich, J. K., Bremont, M., Touchman, J. W., and Jacobs, B. L. (2010). Evidence for multiple recent host species shifts among the Ranaviruses (family Iridoviridae). J. Virolo. 84, 2636–2647.
Jancovich, J. K., Mao, J., Chinchar, V. G., Wyatt, C., Case, S. T., Kumar, S., et al. (2003). Genomic sequence of a ranavirus (family Iridoviridae) associated with salamander mortalities in North America. Virology 316, 90–103. doi: 10.1016/j.virol.2003.08.001
Jancovich, J. K., Qin, Q., Zhang, Q. Y., and Chinchar, V. G. (2015). “Ranavirus replication: molecular, cellular, and immunological events,” in Ranaviruses, eds M. J. Gray and V. G. Chinchar (Cham: Springer), 71–104.
King, A. M., Lefkowitz, E. J., Adams, M. J., and Carstens, E. B. (2011). Virus Taxonomy. Classification and Nomenclature of Viruses. 9th Report of the International Committee on Taxonomy of Viruses. London: Elsevier.
Liu, X., Chen, N., Gao, X., Zhang, Y., Li, X., Zhang, Y., et al. (2018). The infection of red seabream iridovirus in mandarin fish (Siniperca chuatsi) and the host immune related gene expression profiles. Fish Shellfish Immunol. 74, 474–484. doi: 10.1016/j.fsi.2018.01.020
Mao, H., and Wang, H. (2019). Resolution of deep divergence of club fungi (phylum Basidiomycota). Synth. Syst. Biotechnol. 4, 225–231. doi: 10.1016/j.synbio.2019.12.001
Qiu, L., Chen, M. M., Wan, X. Y., Li, C., Zhang, Q. L., Wang, R. Y., et al. (2017). Characterization of a new member of Iridoviridae, Shrimp hemocyte iridescent virus (SHIV), found in white leg shrimp (Litopenaeus vannamei). Sci. Rep. 7:11834. doi: 10.1038/s41598-017-10738-8
Qiu, L., Chen, M. M., Wang, R. Y., Wan, X. Y., Li, C., Zhang, Q. L., et al. (2018). Complete genome sequence of shrimp hemocyte iridescent virus (SHIV) isolated from white leg shrimp, Litopenaeus vannamei. Arch. Virol. 163, 781–785. doi: 10.1007/s00705-017-3642-4
Rahman, S. U., Yao, X., Li, X., Chen, D., and Tao, S. (2018). Analysis of codon usage bias of Crimean-Congo hemorrhagic fever virus and its adaptation to hosts. Infect. Genet. Evol. 58, 1–16. doi: 10.1016/j.meegid.2017.11.027
Seemann, T. (2014). Prokka: rapid prokaryotic genome annotation. Bioinformatics 30, 2068–2069. doi: 10.1093/bioinformatics/btu153
Sharp, P. M., and Li, W. H. (1986). An evolutionary perspective on synonymous codon usage in unicellular organisms. J. Mol. Evol. 24, 28–38. doi: 10.1007/BF02099948
Singh, N. K., Tyagi, A., Kaur, R., Verma, R., and Gupta, P. K. (2016). Characterization of codon usage pattern and influencing factors in Japanese encephalitis virus. Virus Res. 221, 58–65. doi: 10.1016/j.virusres.2016.05.008
Subramanian, B., Gao, S., Lercher, M. J., Hu, S., and Chen, W. H. (2019). Evolview v3: a webserver for visualization, annotation, and management of phylogenetic trees. Nucleic Acids Res. 47, W270–W275. doi: 10.1093/nar/gkz357
Sueoka, N. (1995). Intrastrand parity rules of DNA base composition and usage biases of synonymous codons. J. Mol. Evol. 40, 318–325.
Sueoka, N. (1999). Translation-coupled violation of Parity Rule 2 in human genes is not the cause of heterogeneity of the DNA G+C content of third codon position. Gene 238, 53–58. doi: 10.1016/S0378-1119(99)00320-0
Tidona, C. A., Schnitzler, P., Kehm, R., and Darai, G. (1998). Is the major capsid protein of Iridoviruses a suitable target for the study of viral evolution? Virus Genes 16, 59–66. doi: 10.1023/A:1007949710031
Ur Rahman, S., Mao, Y., and Tao, S. (2017). Codon usage bias and evolutionary analyses of Zika virus genomes. Genes Genomics 39, 855–866. doi: 10.1007/s13258-017-0549-0
Wang, H., Liu, S., Zhang, B., and Wei, W. (2016). Analysis of synonymous codon usage bias of Zika virus and its adaption to the hosts. PLoS One 11:e0166260. doi: 10.1371/journal.pone.0166260
Wang, Y., Tang, H., Debarry, J. D., Tan, X., Li, J., Wang, X., et al. (2012). MCScanX: a toolkit for detection and evolutionary analysis of gene synteny and collinearity. Nucleic Acids Res. 40:e49. doi: 10.1093/nar/gkr1293
Whittington, R. J., Becker, J. A., and Dennis, M. M. (2010). Iridovirus infections in finfish – critical review with emphasis on ranaviruses. J. Fish Dis. 33, 95–122. doi: 10.1111/j.1365-2761.2009.01110.x
Wong, E. H., Smith, D. K., Rabadan, R., Peiris, M., and Poon, L. L. (2010). Codon usage bias and the evolution of influenza A viruses. Codon usage biases of influenza virus. BMC Evol. Biol. 10:253. doi: 10.1186/1471-2148-10-253
Wright, F. (1990). The ‘effective number of codons’ used in a gene. Gene 87, 23–29. doi: 10.1016/0378-1119(90)90491-9
Wu, Q., Yu, Z. G., and Yang, J. (2017). DLTree: efficient and accurate phylogeny reconstruction using the dynamical language method. Bioinformatics 33, 2214–2215.
Wu, Y., Zhao, D., and Tao, J. (2015). Analysis of codon usage patterns in herbaceous peony (Paeonia lactiflora Pall) Based on Transcriptome Data. Genes (Basel) 6, 1125–1139. doi: 10.3390/genes6041125
Xu, L., Wang, T., Li, F., and Yang, F. (2016). Isolation and preliminary characterization of a new pathogenic iridovirus from redclaw crayfish Cherax quadricarinatus. Dis. Aquat. Organ. 120, 17–26. doi: 10.3354/dao03007
Keywords: Iridoviridae, synteny analysis, taxonomy, codon usage, phylogenetic analysis
Citation: Deng Z, Wang J, Zhang W, Geng Y, Zhao M, Gu C, Fu L, He M, Xiao Q, Xiao W, He L, Yang Q, Han J, Yan X and Yu Z (2021) The Insights of Genomic Synteny and Codon Usage Preference on Genera Demarcation of Iridoviridae Family. Front. Microbiol. 12:657887. doi: 10.3389/fmicb.2021.657887
Received: 24 January 2021; Accepted: 09 March 2021;
Published: 31 March 2021.
Edited by:
Gustavo Caetano-Anollés, University of Illinois at Urbana–Champaign, United StatesReviewed by:
Dennis Ken Bideshi, California Baptist University, United StatesCopyright © 2021 Deng, Wang, Zhang, Geng, Zhao, Gu, Fu, He, Xiao, Xiao, He, Yang, Han, Yan and Yu. This is an open-access article distributed under the terms of the Creative Commons Attribution License (CC BY). The use, distribution or reproduction in other forums is permitted, provided the original author(s) and the copyright owner(s) are credited and that the original publication in this journal is cited, in accordance with accepted academic practice. No use, distribution or reproduction is permitted which does not comply with these terms.
*Correspondence: Zehui Yu, eXV6ZWh1aV9zd211QG91dGxvb2suY29t
†These authors have contributed equally to this work
Disclaimer: All claims expressed in this article are solely those of the authors and do not necessarily represent those of their affiliated organizations, or those of the publisher, the editors and the reviewers. Any product that may be evaluated in this article or claim that may be made by its manufacturer is not guaranteed or endorsed by the publisher.
Research integrity at Frontiers
Learn more about the work of our research integrity team to safeguard the quality of each article we publish.