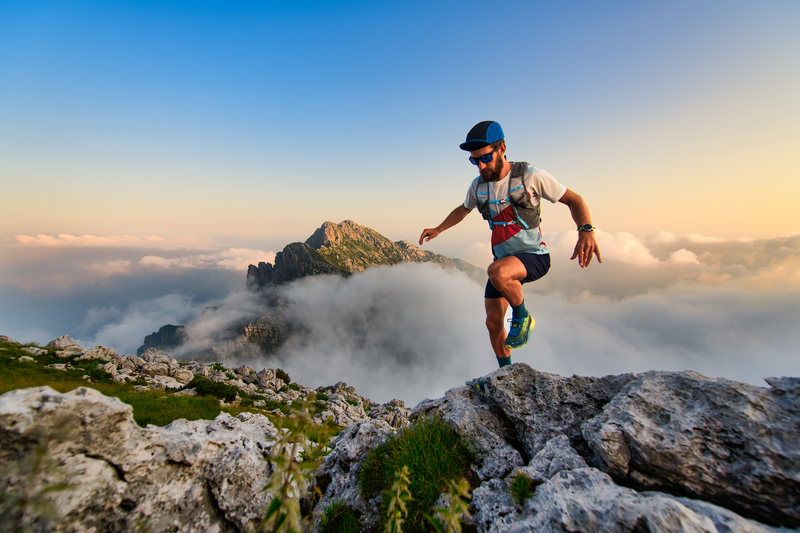
94% of researchers rate our articles as excellent or good
Learn more about the work of our research integrity team to safeguard the quality of each article we publish.
Find out more
ORIGINAL RESEARCH article
Front. Microbiol. , 20 April 2021
Sec. Antimicrobials, Resistance and Chemotherapy
Volume 12 - 2021 | https://doi.org/10.3389/fmicb.2021.651461
This article is part of the Research Topic Antimicrobial Resistance in Agricultural Environments View all 6 articles
Antibiotics are freqeuently used in the livestock sector in low- and middle-income countries for treatment, prophylaxis, and growth promotion. However, there is limited information into the zoonotic prevalence and dissemination patterns of antimicrobial resistance (AMR) within these environments. In this study we used pig farming in Thailand as a model to explore AMR; 156 pig farms were included, comprising of small-sized (<50 sows) and medium-sized (≥100 sows) farms, where bacterial isolates were selectively cultured from animal rectal and human fecal samples. Bacterial isolates were subjected to antimicrobial susceptibility testing (AST), and whole-genome sequencing. Our results indicate extensive zoonotic sharing of antibiotic resistance genes (ARGs) by horizontal gene transfer. Resistance to multiple antibiotics was observed with higher prevalence in medium-scale farms. Zoonotic transmission of colistin resistance in small-scale farms had a dissemination gradient from pigs to handlers to non-livestock contacts. We highly recommend reducing the antimicrobial use in animals’ feeds and medications, especially the last resort drug colistin.
Understanding zoonotic interactions is critical in controlling the plethora of infectious agents that affect our health as highlighted by COVID-19. One important zoonotic concern is the global challenge of antimicrobial resistance (AMR), which has been exacerbated by the overuse and misuse of antibiotic drugs both by the implementation in humans and domestic animals. It has been estimated that 60% of human pathogens are acquired from other animal species but quantification of transfer from humans to animals has been understudied and remain relatively unknown (Sheppard et al., 2018). Therefore, we used Thailand as a model country to explore zoonotic AMR patterns in the commensal bacteria Escherichia coli (E. coli), isolated from livestock pigs (P), contact handlers (C), and non-livestock contact individuals (NC) in the farm’s proximity. To understand which antibiotic drugs pose AMR risks and assess zoonotic AMR transfer events, we utilized antimicrobial susceptibility testing (AST) and whole genome sequencing (WGS) methods in a One Health multi-factorial model consisting of different meat production intensities and subjects within the farm environment.
Traditional antimicrobial usage (AMU) in the livestock sector aims to treat bacterial infections, provide prophylactic treatment to prevent infectious manifestations (McEwen and Fedorka−Cray, 2002; Lugsomya et al., 2018; McEwen and Collignon, 2018), and as growth promoters in animal feed to help generate higher yields (Hughes and Heritage, 2004; Van Boeckel et al., 2015). This paradigm is still followed in low- to middle-income countries (LMICs); whilst there has been a shift in many high-income countries where AMU is now banned for growth promotion and limited to treatment of sick animals with veterinary prescription (Call to phase out prophylactic use of antimicrobials in livestock; No Author, 2011) and reinforced by different interventions depending on the country (Carmo et al., 2018). Unfortunately, in LMICs regulatory framework is often missing or compliance to existing ones is weak (Sommanustweechai et al., 2018), in addition there is often limited surveillance or data on AMU (Carrique-Mas et al., 2020), and further complicated by many livestock producers understanding little about the agents they are using, and further exacerbated by a lack of knowledge in AMR (Ström et al., 2018).
Thailand is a middle-income South-East Asian country and popular travel destination with foreign tourists (Tängdén et al., 2010; Record 38.27m tourists in 2018; 41m expected in 2019, 2019) where antibiotics are freely available over-the-counter with a few exceptions (Sommanustweechai et al., 2018). In locations where there is high AMU, there is also a high AMR burden (O’neill, 2016). This is of critical concern with antibiotic drugs, such as colistin, that are used as a last-resort drugs in humans to treat extensively resistant bacterial infections, e.g., carbapenem resistant Enterobacteriaceae (Wangchinda et al., 2018; Prakobsrikul et al., 2019) where very limited treatment options are available. Where last-resort drugs, such as colistin, are frequently used in livestock and in clinical settings generalized AMR can occur making these crucial drugs void and some bacterial infections untreatable (McEwen and Collignon, 2018). A previous Thai study found a high AMR prevalence in E. coli from chicken and pig farms; isolates were resistant to several antibiotics: ampicillin (97.8 and 94.4% for chickens and pigs, respectively), ciprofloxacin (73.3 and 21.1%), gentamicin (42.2 and 35.6%), and colistin (22.2 and 24.4%)(Nguyen et al., 2016). Whilst a study from the neighboring country Cambodia, saw an Extended spectrum-beta lactamase (ESBL) E. coli prevalence of 20% in humans and 23% in livestock (Atterby et al., 2019).
We wanted to implement the principles of One Health by performing a muliti-displinary study in a LMIC model country where AMR knowledge is often scarce due to limited resources and the few studies published are often limited in scope for potential risk factors that may be pivotal in reducing morbidity and mortality. Therefore, we used as Thailand is a LMIC livestock model to provide much needed evidence-based recommendations on AMU in LMICs in order to save the clinical effect of important antibiotics.
This study was conducted in the Khon Kaen province of North-East Thailand during September-December 2018 in 166 pig farms. Farms were separated on production scale, all farms in this study were considered either small- or medium-scale farms. In this study small-scale farms were defined as having ≤50 sows (often family owned), whilst medium-scale farms were defined as having ≥100 and ≤500 sows and (company owned). In total there was 115 small-scale and 51 medium-scale farms. At each farm we tried to obtain a fecal samples from a contact human and a non-contact human and up-to 10 pigs (depending on the number of pigs on the farm) that were pooled together. Overall we collect 143 pig, 90 contact human and 54 non-contact human fecal sample and stored in accordance to the methods described by Lunha et al. (2020) at the Faculty of veterinary medicine, Khon Kaen University.
Pig rectal swabs from the same farm (n ≤ 10) were pooled in 25 mL of buffered peptone water for enrichment, information regarding numbers of pooled pig rectal swabs from specific farms is provided in Lunha et al. (2020). One gram of human faces was enriched in 5 mL of buffered peptone water. After incubation at 37°C overnight, 10 μL of suspension was plated onto MacConkey agar (Oxoid, Hampshire, England) containing cefotaxime 1 μg/mL (Sigma-Aldrich), CHROMagarTM mSuperCARBATM, and CHROMagarTM COL-APSE (CHROMagar, Paris, France). Different morphologic types of colonies were collected and identified by matrix-assisted laser desorption ionization time of flight (MALDI-TOF, Microflex, Bruker Daltonik GmbH). All isolates were re-streaked from frozen stocks onto blood-agar plates, incubated at 37°C 18 h and checked for potential contamination before AST. All AST were performed using the Sensititre EUVSEC AST microdilution plates using Sensititre Mueller Hinton Broth tubes and the Sensititre AIM pipetting robot (Thermo Fisher Scientific, Fair Lawn, NJ, United States). The AST was done in accordance to the Sensititre EU Surveillance Salmonella/E. coli EUVSEC Plate workflow (Thermo Scientific Sensititre Plate Guide for Antimicrobial Susceptibility Testing, 2018). Quality control was performed with E. coli ATCC 25922 isolate and produced the expected results. All plates were manually read with the sensititre manual viewbox and photographed for documentation. Isolates were catogorized in accordance to definitions of Enterobacteriaceae in Magiorakos et al. (2012) where multi-drug resistance (MDR) was defined in as when isolate is non-susceptible to at least 1 agent in ≥ 3 antimicrobial categories, extensively multi-drug resistance (XDR) when the isolate is non-susceptible to at least 1 agent in all but 2 or fewer antimicrobial drug catergories and pan mutli-drug resistance when the isolate is non-susceptible all antimicrobial drug catergories.
All isolates were re-streaked from frozen stocks onto blood-agar plates, incubated at 37°C 18 h and checked for potential contamination before DNA extraction. DNA was extracted using 10–15 μL of a fresh bacterial colonies and processed using the Qiagen DNeasy blood and tissue kit in accordance to the manufacturer’s instruction (Qiagen, Hilden, Germany). All processed samples had a minimum DNA concentration of 30 ng/μL verified using the dsDNA HS Assay kits on a Qubit Fluorometer (Thermo Fisher Scientific, Fair Lawn, NJ, United States) and OD260/280 in the range of 1.8–2.0 verified by the NanoDrop spectrophotometer (Thermo Fisher Scientific, Fair Lawn (NJ), United States). Samples were shipped on dry ice to the Novogene sequencing facility (Novogene, Hong Kong, China) and sequenced on the Novoseq Illumina platform (Illumina, San Diego, CA, United States) and produced ∼5 GB of 150 bp pair-end sequencing reads per isolate.
All AST data was converted into either a susceptible, intermediate or resistant classification for each isolate and all the individual tested antibiotic drugs in accordance to EUCAST clinical breakpoints (European Committee on Antimicrobial Susceptibility Testing, 2013). Then further processed in Python (3.8.3rc1 Documentation, 2020) using the matplotlib, pandas and seaborn packages. Genomic data processing was performed on the high computing capacity provided by SNIC through Uppsala Multidisciplinary Centre for Advance Computational Science (UPPMAX). All processing of the whole genome sequences was done with open software with an in-house bioinformatics pipeline. Our pipeline consists of four main modules: the first module performed a quality control (QC) assessment of the raw sequence files and trimming the sequence reads with FastQC (Wingett and Andrews, 2018), MultiQC (Ewels et al., 2016), and Trim Galore (Babraham Bioinformatics, 2020); the second module performed de novo assembly with Unicycler with minimum contigs of 400 bp produced (Wick et al., 2017) and assembly QC was performed by QUAST and MultiQC (Gurevich et al., 2013; Ewels et al., 2016); the third module compiled a molecular output excel report from running our genomic data through KmerFinder (Larsen et al., 2014), ARIBA (Hunt et al., 2017), ResFinder (Zankari et al., 2012), and PointFinder (Zankari et al., 2017); and the fourth module produced a core genome maximum likelihood phylogenetic tree by running Prokka (Seemann, 2014) to annotate all the isolate genomes, Roary (Page et al., 2015) to assess the pangenome and generate the core genome alignment, and IQ-Tree2 (Quang et al., 2020) to generate maximum likelihood phylogenetic tree (Figure 1). Downstream genomic data processing was done in Python (3.8.3rc1 Documentation, 2020) using the matplotlib, pandas and seaborn packages. Visualization of the maximum likelihood phylogenetic tree was done using iTOL webtool (Letunic and Bork, 2019). Additional SNP detection of matching ST isolates between groups on the same farm was done using snippy (Seemann, 2015), detection of virulence genes was done using the virulencefinder pipeline (Joensen et al., 2014) and pangenome query difference tables were produced between human and pig groups using Roary query pangenome function (Page et al., 2015).
Figure 1. Overview of experimental and computational set-up of the study. (A) Experimental workflow of the study. (B) Computational analysis work-flow of the study.
This study was performed in accordance to the Helsinki declaration for the human subjects and the EU Directive 2010/63/EU for animal experiments; the protocol involving human participants and animals was approved by the Khon Kaen University Ethics Committee (Project ID: HE612268 and 0514.1.75/66, respectively). Informed consent for each human subject was obtained after an explanation of the experimental procedures. Pig samples were collected at the farm with the farm owners permission of the pig herd.
Raw sequence data can be obtained from the European Nucleotide Archive (ENA) under the project accession number PRJEB38313. All sequence data from computation workflow is compiled in Supplementary File 1, virulencefinder unique results in Supplementary File 2 and genomic difference output is provided in Supplementary File 3. All scripts and Python code and metadata can be provided on request.
To explore zoonotic AMR patterns in E. coli isolates we examined 156 farms in Khon Kaen province of North-East Thailand, utilizing our livestock and human participant One Health study model. A total of 143 pooled pig samples, 90 C and 54 NC human fecal samples and were collected and processed. We generated an isolate collection of 492 E. coli isolates derived from culturing fecal material on agar plates selective for ESBL-producing, carbapenemase-producing, or colistin-resistant gram-negative bacteria. From the 107 small-scale farms (SSF) 174 isolates were from livestock pigs (P), 99 isolates from livestock producers (C), and 57 isolates were from and non-livestock contact individuals (NC). From the 49 medium-scale farms (MSF), 94 isolates were from Ps, 44 isolates from Cs, and 24 isolates were from NCs. Several studies in farms in South-East Asia have looked at E. coli isolates from different livestock and farm workers (Nguyen et al., 2016; Lugsomya et al., 2018; Prakobsrikul et al., 2019); to build upon these studies we wanted to additionally assess farm production size in relation to P, C, and NC and look for differences between the six groups. To record significant differences between the isolate groups we used AST and WGS with in silico methods (Figure 1) to explore the genomic diversity (Read and Massey, 2014; Abdelgader et al., 2018; Boehmer et al., 2018).
Utilizing the E. coli isolates to assess AMR, we could establish AMR patterns to demonstrate single antibiotic drug efficacy. Several antibiotic drugs had an average group MIC concentration that exceeded the clinical breakpoint: ampicillin, chloramphenicol, ciprofloxacin, cefotaxime, gentamicin, and trimethoprim (Figure 2). This was not surprising for ampicillin or cefotaxime due to the selective culturing method, enriching, e.g., for ESBL E. coli. Whilst for other drugs we observed clustering around the clinical breakpoints, e.g., colistin, ceftazidime, and tigecycline. Yet again these results were not surprising for colistin or ceftazidime due to the selection for ESBL and colistin-resistant E. coli. Our results demonstrate that ceftazidime and tigecycline both had a higher phenotypic AMR bias in the human isolates especially those from MSFs. This was not the case for colistin where there was a resistance bias toward SSFs, with the highest results occurring in the SSF-P isolates with an average MIC concentration of 4.2 mg/L, second highest in SSF-C isolates with an average MIC concentration of 3.3 mg/L, and third was the SSF-NC isolates with an average MIC concentration of 2.8 mg/L. These results suggest SSF pigs as a colistin AMR reservoir and zoonotic transfer to the human population. Colistin resistance in Thailand has been frequently reported (Nguyen et al., 2016; McEwen and Collignon, 2018; Ström et al., 2018; Wangchinda et al., 2018; Collignon and McEwen, 2019) but here we report an accumulation in SSFs and signs of zoonotic transmission to humans. However, for chloramphenicol and cefotaxime we saw a preference for human-associated host AMR with a bias toward MSFs rather than SSFs. The average MIC value for chloramphenicol had the highest average MIC value in MSFs in NCs 53.7 mg/L, followed by 44.4 mg/L in Cs and lowest in Ps 31.7 mg/L. Cefotaxime had the highest average MIC value in MSF in C 3.9 mg/L, followed 3.5 mg/L in NCs and lowest in Ps 3.1 mg/L. The only drug that had wide-scale average MIC concentration that indicated susceptibility in all groups was meropenem. In our isolate collection only 6 isolates were cultivated from our carbapenem-selective plates, out of these isolates only 2 were confirmed by AST testing to be meropenem resistant (Supplementary Figure 1). Both isolates were obtained from humans on independent farms; one from a NC and the other from a C. To ensure our findings were statistically relevant we performed one-way ANOVA statistical tests with a Bartlett’s test for each antibiotic based on the group MIC values to see significant differences both in the mean and the standard deviation. Four antibiotic drugs had a significant difference between groups: chloramphenicol, colistin, cefotaxime, and meropenem. In addition we also converted our group isolates into the susceptible (S), intermediate (I) or resistant (R) categories using the EUCAST breakpoints (Supplementary Figure 2). In this analysis we observed the same trends for the four antibiotic drugs that had significant differences in the MIC-value comparison. For instance we still observed with colisin the zoonotic spill-over effect in the SSFs where overall resistant isolates in the groups were 55, 38, and 29% in P, C and NC, respectively, compared to in MSFs 5.3, 13.6, and 20.8% in the same respective order.
Figure 2. Average Minimal inhibitory concentration (MIC) for drug investigation group to each drug reagent with their respective standard deviation and clinical breakpoints for each drug are displayed across the y-axis.
We also wanted to examine the collateral damage of AMR of multiple agents in different antibiotic classes in our isolate data. As others have stated that this is a prolific AMR problem in South-East Asia, from our data we can also confirm this problem both in pigs and humans (Lai et al., 2014; Chereau et al., 2017). Our results are summarized in Table 1 showing the distribution of drug resistance from 0 up to 8 different classes of antibiotics. Following the definitions provided by Magiorakos et al. (2012) we found the most extensive multi-drug resistant (XDR) isolates in the NC cohort. We also found more XDR in the MSFs compared to the SSFs in all groups, with the highest occurrence difference being in P and C. Further, we observed significantly more multi-drug resistant (MDR) in the MSF-C group compared to the SSF-C group.
Following our phenotypic data, we continued with genotypic characterization. Unlike other preceding studies we used WGS of our 492 isolates to see the full extent of the antibiotic resistance genes (ARGs) and known chromosomal mutations present. Within our data we saw higher diversity of ARGs in the SSFs compared to the MSFs (Figure 3), consistent with a less regulated and uniform AMU in family owned SSFs as compared to company owned MSFs. The majority of these ARGs were in the aminoglycoside, beta-lactam, colistin, fosfomycin, rifampicin, quinolone and trimethoprim antibiotic drug classes (Figure 4A). A total of 118 different ARGs were discovered in the SSFs compared to 92 ARGs in the MSFs, with 31 unique to SSFs and 6 unique to MSFs (Supplementary Table 1). The most striking difference was observed in the SSF-P group where resistance genes to beta-lactam and colistin antibiotic drug classes accounted for 39 and 15% of total ARGs, respectively. The abundance of colistin ARGs in the SSF also correlated with our previously discussed phenotypic results where the highest abundance of colistin resistance genes was observed in Ps followed by Cs and lastly by NCs. We suspect this is due to the fact that it is common for colistin to be used in pig feed and treatments given to SSF-Ps, and consistent with reports of high colistin resistance prevalence in Thai E. coli isolates from livestock (Nguyen et al., 2016). We did not detect the same correlation with the beta-lactam ARGs where pigs in SSFs had the highest abundance whilst the other groups appeared to have similar values.
Figure 3. Venn diagrams displaying number of antibiotic resistance genes (ARGs) shared between different investigation groups. (A) Comparison of total antibiotic resistance genes in small-scale farms. (B) Comparison of total antibiotic resistance genes in medium-scale farms.
Figure 4. Overview of total ARGs and chromosomal mutations. (A) Display of % of total ARGs according to antibiotic drug class for each investigation group of this study. (B) Display of % of antibiotic resistant associated chromosomal for each investigation group of this study.
Looking in our WGS dataset we were also interested in detecting chromosomal mutations that confer known antibiotic resistance (Figure 4B). From our literature-supported results, most of the chromosomal mutations conferred resistance to the quinolone antibiotic drug class with the exception of one associated with kasugamycin resistance and the other to ampicillin and clavulanic acid resistance and cephalosporin drugs. We found three point mutations that were present in all sample groups ≥10%, these were: gyrA S83L (Everett et al., 1996), parC S80I (Kumagai et al., 1996), and gyrA D87N (Sáenz et al., 2003). All of these mutations are well-known to confer quinolone resistance and are frequently seen both in human and farm animal samples. All the detected chromosomal mutations in our dataset had a human rather than animal bias and were generally biased toward MSFs compared to SSFs.
From our genomic analysis we wanted to observe the prevalence of ESBL and colistin resistance genes within our isolate groups (Table 2). We saw a high prevalence of ESBL genes in all groups with the lowest value being 96.6% in MSF-C group, this was much higher than expected due to previous reports in South-East Asia (Nguyen et al., 2016; Atterby et al., 2019). Despite the higher prevalence in our study compared to other reports, the observation of ESBL genes matches our phenotypic results as the average MIC for all groups as was above the clinical breakpoint for cefotaxime, a third-generation cephalosporin (Figure 2). We also saw the same trend for colistin resistance as seen in phenotypic and ARG diversity results. There was a diminishing resistance trend in SSF from P, C to NC, whilst the trend was in the opposite direction on MSF. It should be noted that exact resistance prevalence per farm cannot be directly compared between human and pig samples, as human samples were obtained from single individuals, and pig samples from pools of up to 10 swabs from different pigs. However, the difference in trends is not dependent on exact prevalence, and thus also these results suggest the highest colistin AMU on SSFs, that could be a hot-spot for colistin resistance development and zoonotic spread, unless colistin in pigs is prohibited.
To assess the clonality of the isolates from the different study groups we did in silico multi-locus sequence typing analysis to identify the sequence types (ST). Then we looked for the most frequent ST in each group (Table 3) and plotted overlapping STs in the different groups (Figure 5). There was a large variation of STs among our isolates and the data suggests some ST types having a high propensity toward a given host (i.e., a large ST overlap between contact and non-contact humans in small-scale farms in Figure 5, and a large number of STs only present in pigs) (Sheppard et al., 2018). However, there were also signs of clonal transfer or shared STs between pigs and humans: (i) ST48 was the most common ST in small-scale farm pigs and contact humans but in no other groups; (ii) the ST overlaps between contact humans and pigs were larger than the overlaps between pigs and non-contact humans in both farm types. To further investigate the clonality of the different isolates within our collection we generated a maximum-likelihood phylogenetic tree (Supplementary Figure 2). From our maximum-likelihood phylogenetic tree we did not observe clonality from farm isolates, we only saw some clustering based on MLST type and sample group. ST48 has been widely reported for harboring ESBLs mainly in LMICs or from imports (Seenama et al., 2019; Tian et al., 2020) but also from human contamination in drinking water in a high-income country (Madec et al., 2016). However, we concluded that several sequence types were circulating within the farm environment with different host preferences that all may play a key role in AMR.
Figure 5. Venn diagram of Multi Locus Sequence Types shared between different investigation groups. (A) Comparison of STs in small-scale farms. (B) Comparison of total STs genes in medium-scale farms.
To further investigate clonality we examined total variant differences between matching ST isolates in different groups found on the same farm (Table 4). We saw 6 instances where ≤25 variants were detected between group isolates, the majority of these were between C and NC isolates where 3 were from SSFs and 2 from MSFs and one case between a C and P on an MSF. We assumed this bias was due to gene differences required between pigs and humans, such as virulence genes. To confirm this we did virulence gene assessment using virulencefinder (Joensen et al., 2014) but only found four different genes between the human isolates and P isolates, these were cma, air and eilA in the human sourced isolates and stb in Ps (Supplementary File 2). We also use the query pangenome function of the Roary pipeline (Sitto and Battistuzzi, 2020), however we did not see any conclusive differences (Supplementary File 3). We tended to observe higher clonality amongst isolates from the same host and similarity of ARG carriage depending on the farm type, this was most clearly observed between small-scale farms between pigs and contact humans with colistin resistance. From further analysis we were able to detect the origin of replication of multiple plasmids (Supplementary Figure 3), thus supporting that transient zoonotic transmission could allow for acquisition of ARGs by horizontal gene transfer to the host’s endogenous E. coli population, as we could observe ST differentiation, but no significant difference in ARG carriage depending on the antibiotic drug. In our data set we only saw one shared strain with 11 SNP or INDEL genomic variants difference between a contact human and a pig on farm 120, a medium-scale farm. We could not see extensive zoonotic sharing of clones between human and pigs. The exception in farm 120 is likely the result of a transient zoonotic transmission event, where the transient bacterial strain could disseminate any of its ARGs to the host’s endogenous Gram-negative bacterial community thus giving rise to new antibiotic resistant strains. The frequency of these events and the amount of antibiotics used both in humans and pigs as a selective pressure for ARGs maintenance will affect the impact.
From the few previous published studies it has become apparent that there is high AMU burden in South-East Asian LMICs. Additionally in Thailand like several other LMICs antimicrobial pharmaceuticals are generally available over-the-counter, as well as in medicated feed and due to their cost old antibiotics are often recycled for alternative uses (Sommanustweechai et al., 2018). For instance the drug fosfomycin (in addition to beta-lactamase inhibitors and carbapenems) requires a special permit for purchase in Thailand, therefore it would be assumed not to be used in farm animals (Sommanustweechai et al., 2018). Potential explanations for resistance to fosfomycin in livestock still being detected include; use of recycled fosfomycin prescriptions, zoonotic transfer from humans to livestock, or unauthorized sale of fosfomycin as an animal treatment or feed additive. For the other special-permit drugs that belong in the class of carbapenems we saw low AMR prevalence with only two isolates detected in two human individuals (Supplementary Figure 1). We assume this is a result of these drugs being expensive, hard to acquire outside the hospital environment, and potentially that they require intravenous administration. Hopefully inaccessibility in treating animals remains in place to help maintain the susceptibility of these drugs. However, we may see more carbapenem resistance in the pig populations may be seen in the future either from zoonotic transfer events or if an informal market for these drugs occur due to a lack of other antibacterial treatment options.
Utilizing our One Health multi-factorial livestock/human model in Thailand and AST of 492 E. coli isolates, we observe prolific AMR to several antibiotic agents especially in MSF where a majority of isolates were MDR or XDR (Table 1). These results corroborate earlier findings from the same study population but using disc diffusion AST analysis of random E. coli isolates (not selectively cultured, i.e., not using antibiotic-containing media) (Lunha et al., 2020). In the present study, all isolates were resistant to ampicillin and all groups had an average MIC above the clinical breakpoint for cefotaxime (Figure 2). We also observed an average MIC above the clinical breakpoint for the antibiotics chloramphenicol, gentamicin and trimethroprim/sulfamethoxazole. Additionally we could see indications of zoonotic spill-over of colistin resistance within SSFs with average MIC P > C > NC, i.e., a decreasing colistin MIC gradient.
From WGS analysis we saw high overall farm prevalence of ESBLs detection ranging from 96.6 to 100% across all the investigatory groups, whilst overall farm prevalence of colistin ARGs was 48.7% with the highest group being in the SSF-P with a detection rate of 62.1% (Table 2). The ESBL prevalance was much higher than expected when compared to previous reports in South-East Asia (Nguyen et al., 2016; Atterby et al., 2019). The most common ESBL gene detected was blaCTX-M-14 accession number AF25622; 43 other ESBL genes were also identified. Whilst the most common colistin resistance gene was mcr-1.1 accession number KP347127, whereas 12 other colistin resistance genes were also detected in our data set. The colistin ARG detection frequencies had the same zoonotic spill-over indication in SSFs as the AST data (P > C > NC), therefore providing a plausible molecular mechanism for the phenotypic observation seen. From the WGS data we in silco analyzed MLST of each isolate; in farms where the same MLST was detected in different sample groups, additional variant analysis was performed. We observed only one case of suspected clonality between human and pig isolates (same ST with less than 25 variants) (Table 2), indicating limited zoonotic sharing of bacterial strains. The most frequent ST shared between P and C in SSFs was ST48, a common E. coli ST found in livestock and infamous for ESBL carriage (Seenama et al., 2019; Tian et al., 2020), but no clonal similarity was found when performing the additional variant analysis. Therefore we conclude that horizontal gene transfer is likely the major mechanism of zoonotic ARG dissemination in our study, and potentially in LMIC farm environments in general.
Strengths of this study include providing a large isolate collection that has AST data coupled with extensive molecular data on sequence types, ARGs and clonal similarities between isolates. Furthermore, a One Health, multi-disciplinary approach was used, and concurrent samples from humans and animals were obtained. From our approach we could select isolates that exhibited the ESBL, colistin and carbapenem resistant phenotypes and detect any other AMR genotypic traits that can hitch-hike with these phenotypes by our WGS analysis. We observed both farm-scale difference where more MDR was detected in MSFs and XDR in the MSF NC cohort, and we also observed the zoonotic spill-over effect in the SSF from P to C to NC. Whilst limitations of the study include skewed data due to the antibiotic selection used to obtain isolates from the raw fecal samples and that pig samples were pooled whereas human samples were not. Additionally our study was limited to E. coli isolates therefore follow-up studies could be warranted looking at the resistome in all these groups by metagenomic sequencing enabling a broader picture.
This study presents a snap shot of resistant isolates that contribute to ESBL and colistin-resistant phenotypes as well as ARGs that can hitch-hike with these AMR traits. Our AMR surveillance data highlights the AMR/AMU problem, whilst publications based on questionnaire and interviews revealed that farmers, human and animal health professionals and the general public have little awareness of the problem of AMR or knowledge to counter-act the AMR effects (Sommanustweechai et al., 2018; Ström et al., 2018). Especially as it is well known that the down-stream effects of AMR have several stake-holders (Cars et al., 2011; Cantas et al., 2013; Dyar et al., 2018). It is important to state that attitudes are changing and the effects of AMR concerns, for instance Thailand has a National Action Plan on Antimicrobial Resistance endorsed by the Thai Cabinet in 2016 to reduce antibiotic consumption by 30% in veterinary medicine by 2021 (Sommanustweechai et al., 2018). Our and other’s data provide additional evidence to policymakers in the SE Asia region to highlight the importance of meeting AMU reduction goals and continue with AMR surveillance of farms to develop future AMU guidelines as well as gain a more general understanding of zoonotic transmission of AMR. We demonstrate a strong colistin AMR reservoir among pigs on small-scale farms which is likely maintained by colistin use. We can also see clear signs of zoonotic transmission/spill-over from small-scale farm pigs to contact humans to non-livestock contacts both genotypically (colistin ARG prevalence and abundance) and phenotypically (colistin MIC values). Therefore, we argue that AMU of colistin in animals should be prohibited due to previous colistin AMR reporting and further evidence we have generated (Laxminarayan et al., 2013; Carmo et al., 2018; Magnusson et al., 2019).
From our work we demonstrate the importance of surveillance to detect AMR in LMICs to highlight potential AMR risks as data from these countries are often scarce (Van Boeckel et al., 2019). Also, AMR global transfer events do occur—blaNDM–1 and mcr resistance genes are prime examples, where their extenence on mobile genetic elements with other resistance can confer multiple-drug resistance that can easily be shared by a multitude of Gram-negative bacteria. Currently, we present the data of ARGs and known chromosomal mutations that are present in our isolate collection from the present-day versions of the databases available. However, on the discovery of novel ARGs or chromosomal mutations we encourage fellow researchers to use our data to see if these novel ARGs or mutations are also present in our study conducted in 2018. Furthermore, we would like to highlight the importance of combining interventions to reduce AMU with surveillance to reduce the resistance to antibiotic drugs such as colistin, ciprofloxacin and gentamicin and maintain the potency of drugs such as meropenem. This could be done by utilizing phenotypic AST microbroth plate testing and genotypic WGS analysis like our study or by traditional techniques such as AST disc diffusion testing and PCR used in other studies in Thailand (Lugsomya et al., 2018; Ström et al., 2018; Wangchinda et al., 2018; Ström Hallenberg et al., 2019) or even using high-throughput technologies such as AST by microfluidics or metagenomics. Our results indicate that farm-host group differences are a result of mobile genetic elements containing ARGs that are transferred from transient E. coli strains to endogenous E. coli strains by horizontal gene transfer.
Using our One Health multi-factorial model to compare E. coli isolates from livestock, contact humans and non-contact humans on small-scale and medium-scale Thai pig farms we demonstrated: (i) prolific multi-drug resistance that was more prevelent on medium-scale farms and (ii) likely zoonotic transmission of colistin resistance in small-scale farms as there was a dissemination gradient from pigs to handlers to non-livestock contacts. We highly recommend reducing the antimicrobial use in animals’ feeds and medications, especially the last resort drug colistin.
Raw sequence data can be obtained from the European Nucleotide Archive (ENA) under the project accession number PRJEB38313. All sequence data from computation workflow is compiled in Supplementary File 1, virulence finder unique results in Supplementary File 2 and genomic difference output is provided in Supplementary File 3. All scripts and Python code and metadata can be provided on request.
This study was performed in accordance to the Helsinki declaration for the human subjects and the EU Directive 2010/63/EU for animal experiments; the protocol involving human participants and animals was approved by the Khon Kaen University Ethics Committee (Project ID: HE612268 and 0514.1.75/66, respectively). Informed consent for each human subject was obtained after an explanation of the experimental procedures. The patients/participants provided their written informed consent to participate in this study. Pig samples were collected at the farm with the farm owners permission of the pig herd. Written informed consent was obtained from the owners for the participation of their animals in this study.
RH, TL, UM, MS, and JDJ designed the study. KL, JJ, SA, and MS collected the original samples. RH, TL, and KL did the Laboratory work. RH analyzed the data and prepared figures. RH and JDJ interpreted the data. RH wrote the manuscript with critical revisions by JDJ. All authors revised and approved the final manuscript.
The study was financially supported by the fifth call of the Swedish Research Council Vetenskapsrådet/Joint Programming Initiative for AMR (JPIAMR) to the ReduceAMU project (2017-026) and Swedish Research Council Vetenskapsrådet (2016-02606).
The authors declare that the research was conducted in the absence of any commercial or financial relationships that could be construed as a potential conflict of interest.
We would like to thank for the additional help and contribution to this work by DVM Ellen Gullbransen for her contribution to the field work and Hanna Karin Ilag and Fiona Valerie Franklin-Alming for their contribution to the laboratory work.
The Supplementary Material for this article can be found online at: https://www.frontiersin.org/articles/10.3389/fmicb.2021.651461/full#supplementary-material
8.3rc1 Documentation (2020). 3.8.3rc1 Documentation. Delaware: Python. Available online at: https://docs.python.org/3/ (accessed May 12, 2020).
Abdelgader, S. A., Shi, D., Chen, M., Zhang, L., Hejair, H. M. A., Muhammad, U., et al. (2018). Antibiotics Resistance Genes Screening and Comparative Genomics Analysis of Commensal Escherichia coli Isolated from Poultry Farms between China and Sudan. Biomed. Res. Int. 2018:5327450. doi: 10.1155/2018/5327450
Atterby, C., Osbjer, K., Tepper, V., Rajala, E., Hernandez, J., Seng, S., et al. (2019). Carriage of carbapenemase− and extended−spectrum cephalosporinase−producing Escherichia coli and Klebsiella pneumoniae in humans and livestock in rural Cambodia; gender and age differences and detection of bla OXA–48 in humans. Zoonoses Public Health 66, 603–617. doi: 10.1111/zph.12612
Babraham Bioinformatics (2020). Trim Galore. Available online at: http://www.bioinformatics.babraham.ac.uk/projects/trim_galore/ (accessed May 12, 2020)
Boehmer, T., Vogler, A. J., Thomas, A., Sauer, S., Hergenroether, M., Straubinger, R. K., et al. (2018). Phenotypic characterization and whole genome analysis of extended-spectrum beta-lactamase-producing bacteria isolated from dogs in Germany. PLoS One 13:e0206252. doi: 10.1371/journal.pone.0206252
Cantas, L., Shah, S. Q. A., Cavaco, L. M., Manaia, C. M., Walsh, F., Popowska, M., et al. (2013). A brief multi-disciplinary review on antimicrobial resistance in medicine and its linkage to the global environmental microbiota. Front. Microbiol. 4:96. doi: 10.3389/fmicb.2013.00096
Carmo, L. P., Nielsen, L. R., Alban, L., da Costa, P. M., Schüpbach-Regula, G., and Magouras, I. (2018). Veterinary Expert Opinion on Potential Drivers and Opportunities for Changing Antimicrobial Usage Practices in Livestock in Denmark, Portugal, and Switzerland. Front. Vet. Sci. 5:29. doi: 10.3389/fvets.2018.00029
Carrique-Mas, J. J., Choisy, M., Van Cuong, N., Thwaites, G., and Baker, S. (2020). An estimation of total antimicrobial usage in humans and animals in Vietnam. Antimicrob. Resist. Infect. Control 9:16. doi: 10.1186/s13756-019-0671-7
Cars, O., Hedin, A., and Heddini, A. (2011). The global need for effective antibiotics—Moving towards concerted action. Drug Resist. Updat. 14, 68–69. doi: 10.1016/J.DRUP.2011.02.006
Chereau, F., Opatowski, L., Tourdjman, M., and Vong, S. (2017). Risk assessment for antibiotic resistance in South East Asia. BMJ 358:j3393. doi: 10.1136/bmj.j3393
Collignon, P. J., and McEwen, S. A. (2019). One health-its importance in helping to better control antimicrobial resistance. Trop. Med. Infect. Dis. 4:22. doi: 10.3390/tropicalmed4010022
Dyar, O. J., Yin, J., Ding, L., Wikander, K., Zhang, T., Sun, C., et al. (2018). Antibiotic use in people and pigs: a One Health survey of rural residents’ knowledge, attitudes and practices in Shandong province. China J. Antimicrob. Chemother. 73, 2893–2899. doi: 10.1093/jac/dky240
European Committee on Antimicrobial Susceptibility Testing (2013). Breakpoint tables for interpretation of MICs and zone diameters. Sweden: European Committee on Antimicrobial Susceptibility Testing.
Everett, M. J., Jin, Y. F., Ricci, V., and Piddock, L. J. V. (1996). Contributions of individual mechanisms to fluoroquinolone resistance in 36 Escherichia coli strains isolated from humans and animals. Antimicrob. Agents Chemother. 40, 2380–2386. doi: 10.1128/aac.40.10.2380
Ewels, P., Magnusson, M., Lundin, S., and Käller, M. (2016). MultiQC: Summarize analysis results for multiple tools and samples in a single report. Bioinformatics 32, 3047–3048. doi: 10.1093/bioinformatics/btw354
Gurevich, A., Saveliev, V., Vyahhi, N., and Tesler, G. (2013). QUAST: quality assessment tool for genome assemblies. Bioinformatics 29, 1072–1075. doi: 10.1093/bioinformatics/btt086
Hughes, P., and Heritage, J. (2004). Assessing quality and safety of animal feeds Antibiotic growth-promoters in food animals. Rome: FAO.
Hunt, M., Mather, A. E., Sánchez-Busó, L., Page, A. J., Parkhill, J., Keane, J. A., et al. (2017). ARIBA: Rapid antimicrobial resistance genotyping directly from sequencing reads. Microb. Genomics 3:e000131. doi: 10.1099/mgen.0.000131
Joensen, K. G., Scheutz, F., Lund, O., Hasman, H., Kaas, R. S., Nielsen, E. M., et al. (2014). Real-time whole-genome sequencing for routine typing, surveillance, and outbreak detection of verotoxigenic Escherichia coli. J. Clin. Microbiol. 52, 1501–1510. doi: 10.1128/JCM.03617-13
Kumagai, Y., Kato, J. I., Hoshino, K., Akasaka, T., Sato, K., and Ikeda, H. (1996). Quinolone-resistant mutants of Escherichia coli DNA topoisomerase IV parC gene. Antimicrob. Agents Chemother. 40, 710–714. doi: 10.1128/aac.40.3.710
Lai, C. C., Lee, K., Xiao, Y., Ahmad, N., Veeraraghavan, B., Thamlikitkul, V., et al. (2014). High burden of antimicrobial drug resistance in Asia. J. Glob. Antimicrob. Resist. 2, 141–147. doi: 10.1016/j.jgar.2014.02.007
Larsen, M. V., Cosentino, S., Lukjancenko, O., Saputra, D., Rasmussen, S., Hasman, H., et al. (2014). Benchmarking of methods for genomic taxonomy. J. Clin. Microbiol. 52, 1529–1539. doi: 10.1128/JCM.02981-13
Laxminarayan, R., Duse, A., Wattal, C., Zaidi, A. K., Wertheim, H. F., Sumpradit, N., et al. (2013). The Lancet Infectious Diseases Commission Antibiotic resistance-the need for global solutions Part 1: Global epidemiology of antibiotic resistance and use. Lancet Infect. Dis. 13, 1057–1098. doi: 10.1016/S1473-3099(13)70318-9
Letunic, I., and Bork, P. (2019). Interactive Tree Of Life (iTOL) v4: recent updates and new developments. Nucleic Acids Res. 47, W256–W259. doi: 10.1093/nar/gkz239
Lugsomya, K., Chatsuwan, T., Niyomtham, W., Tummaruk, P., Hampson, D. J., and Prapasarakul, N. (2018). Routine Prophylactic Antimicrobial Use Is Associated with Increased Phenotypic and Genotypic Resistance in Commensal Escherichia coli Isolates Recovered from Healthy Fattening Pigs on Farms in Thailand. Microb. Drug Resist. 24, 213–223. doi: 10.1089/mdr.2017.0042
Lunha, K., Leangapichart, T., Jiwakanon, J., Angkititrakul, S., Sunde, M., Järhult, J. D., et al. (2020). Antimicrobial Resistance in Fecal Escherichia coli from Humans and Pigs at Farms at Different Levels of Intensification. Antibiotics 2020:662. doi: 10.3390/antibiotics9100662
Madec, J. Y., Haenni, M., Ponsin, C., Kieffer, N., Rion, E., and Gassilloud, B. (2016). Sequence type 48 Escherichia coli carrying the blaCTX-M-1 IncI1/ST3 plasmid in drinking water in France. Antimicrob. Agents Chemother. 60, 6430–6432. doi: 10.1128/AAC.01135-16
Magiorakos, A.-P., Srinivasan, A., Carey, R. B., Carmeli, Y., Falagas, M. E., Giske, C. G., et al. (2012). Multidrug-resistant, extensively drug-resistant and pandrug-resistant bacteria: an international expert proposal for interim standard definitions for acquired resistance. Clin. Microbiol. Infect. 18, 268–281. doi: 10.1111/j.1469-0691.2011.03570.x
Magnusson, U., Sternberg, S., Eklund, G., and Rozstalnyy, A. (2019). Prudent and efficient use of antimicrobials in pigs and poultry. Rome: FAO.
McEwen, S. A., and Collignon, P. J. (2018). Antimicrobial Resistance: a One Health Perspective. Microbiol. Spectr. 6:2017. doi: 10.1128/microbiolspec.arba-0009-2017
McEwen, S. A., and Fedorka−Cray, P. J. (2002). Antimicrobial Use and Resistance in Animals. Clin. Infect. Dis. 34, S93–S106. doi: 10.1086/340246
Nguyen, N. T., Nguyen, H. M., Nguyen, C. V., Nguyen, T. V., Nguyen, M. T., Thai, H. Q., et al. (2016). Use of colistin and other critical antimicrobials on pig and chicken farms in southern Vietnam and its association with resistance in commensal Escherichia coli bacteria. Appl. Environ. Microbiol. 82, 3727–3735. doi: 10.1128/AEM.00337-16
No Author (2011). Call to phase out prophylactic use of antimicrobials in livestock. Vet. Rec. 169, 479–480. doi: 10.1136/vr.d7090
O’neill, J. (2016). “TACKLING DRUG-RESISTANT INFECTIONS GLOBALLY,” in FINAL REPORT AND RECOMMENDATIONS THE REVIEW ON ANTIMICROBIAL RESISTANCE, (Geneva: WHO).
Page, A. J., Cummins, C. A., Hunt, M., Wong, V. K., Reuter, S., Holden, M. T. G., et al. (2015). Roary: rapid large-scale prokaryote pan genome analysis. Bioinformatics 31, 3691–3693. doi: 10.1093/bioinformatics/btv421
Prakobsrikul, N., Malathum, K., Santanirand, P., Chumnumwat, S., Piebpien, P., and Montakantikul, P. (2019). Correlation between antimicrobial consumption and the prevalence of carbapenem-resistant Escherichia coli and carbapenem-resistant Klebsiella pneumoniae at a university hospital in Thailand. J. Clin. Pharm. Ther. 44, 292–299. doi: 10.1111/jcpt.12791
Quang, B., Schmidt, H. A., Chernomor, O., Schrempf, D., Woodhams, M. D., Von Haeseler, A., et al. (2020). IQ-TREE 2: New Models and Efficient Methods for Phylogenetic Inference in the Genomic Era. Mol. Biol. Evol. 37, 1530–1534. doi: 10.1093/molbev/msaa015
Read, T. D., and Massey, R. C. (2014). Characterizing the genetic basis of bacterial phenotypes using genome-wide association studies: A new direction for bacteriology. Genome Med. 6:109. doi: 10.1186/s13073-014-0109-z
Record 38.27m tourists in 2018; 41m expected in 2019 (2019). Record 38.27m tourists in 2018; 41m expected in 2019. Available online at: https://www.bangkokpost.com/business/1619182/record-38-27m-tourists-in-2018-41m-expected-in-2019 (accessed November 6, 2020)
Sáenz, Y., Zarazaga, M., Briñas, L., Ruiz-Larrea, F., and Torres, C. (2003). Mutations in gyrA and parC genes in nalidixic acid-resistant Escherichia coli strains from food products, humans and animals. J. Antimicrob. Chemother. 51, 1001–1005. doi: 10.1093/jac/dkg168
Seemann, T. (2014). Prokka: rapid prokaryotic genome annotation. Bioinformatics 30, 2068–2069. doi: 10.1093/bioinformatics/btu153
Seemann, T. (2015). snippy: fast bacterial variant calling from NGS reads. snippy: fast bacterial variant calling from NGS re. San Francisco: github.
Seenama, C., Thamlikitkul, V., and Ratthawongjirakul, P. (2019). Multilocus sequence typing and blaESBL characterization of extended-spectrum beta-lactamase-producing escherichia coli isolated from healthy humans and swine in Northern Thailand. Infect. Drug Resist. 12, 2201–2214. doi: 10.2147/IDR.S209545
Sheppard, S. K., Guttman, D. S., and Fitzgerald, J. R. (2018). Population genomics of bacterial host adaptation. Nat. Rev. Genet. 19, 549–565. doi: 10.1038/s41576-018-0032-z
Sitto, F., and Battistuzzi, F. U. (2020). Estimating Pangenomes with Roary. Mol. Biol. Evol. 37, 933–939. doi: 10.1093/molbev/msz284
Sommanustweechai, A., Chanvatik, S., Sermsinsiri, V., Sivilaikul, S., Patcharanarumol, W., Yeung, S., et al. (2018). Antibiotic distribution channels in Thailand: results of key-informant interviews, reviews of drug regulations and database searches. Bull. World Heal. Organ. 96, 101–109. doi: 10.2471/BLT.17.199679
Ström Hallenberg, G., Börjesson, S., Sokerya, S., Sothyra, T., and Magnusson, U. (2019). Detection of mcr-mediated colistin resistance in Escherichia coli isolates from pigs in small-scale farms in Cambodia. Antimicrob. Agents Chemother. 63:18. doi: 10.1128/AAC.02241-18
Ström, G., Boqvist, S., Albihn, A., Fernström, L. L., Andersson Djurfeldt, A., Sokerya, S., et al. (2018). Antimicrobials in small-scale urban pig farming in a lower middle-income country - arbitrary use and high resistance levels. Antimicrob. Resist. Infect. Control 7:35. doi: 10.1186/s13756-018-0328-y
Tängdén, T., Cars, O., and Melhus, Å, and Löwdin, E. (2010). Foreign travel is a major risk factor for colonization with Escherichia coli producing CTX-M-type extended-spectrum β-lactamases: A prospective study with Swedish volunteers. Antimicrob. Agents Chemother. 54, 3564–3568. doi: 10.1128/AAC.00220-10
Thermo Scientific Sensititre Plate Guide for Antimicrobial Susceptibility Testing (2018). Available online at: https://assets.thermofisher.com/TFS-Assets/MBD/brochures/Sensititre-Plate-Guide-Booklet-EN.pdf (accessed March 31, 2021).
Tian, D., Wang, B., Zhang, H., Pan, F., Wang, C., Shi, Y., et al. (2020). Dissemination of the bla NDM-5 Gene via IncX3-Type Plasmid among Enterobacteriaceae in Children. mSphere 5:19. doi: 10.1128/msphere.00699-19
Van Boeckel, T. P., Brower, C., Gilbert, M., Grenfell, B. T., Levin, S. A., Robinson, T. P., et al. (2015). Global trends in antimicrobial use in food animals. Proc. Natl. Acad. Sci. U S A. 112, 5649–5654. doi: 10.1073/pnas.1503141112
Van Boeckel, T. P., Pires, J., Silvester, R., Zhao, C., Song, J., Criscuolo, N. G., et al. (2019). Global trends in antimicrobial resistance in animals in low- And middle-income countries. Science 365:aaw1944. doi: 10.1126/science.aaw1944
Wangchinda, W., Pati, N., Maknakhon, N., Seenama, C., Tiengrim, S., and Thamlikitkul, V. (2018). Collateral damage of using colistin in hospitalized patients on emergence of colistin-resistant Escherichia coli and Klebsiella pneumoniae colonization and infection. Antimicrob. Resist. Infect. Control 7:84. doi: 10.1186/s13756-018-0375-4
Wick, R. R., Judd, L. M., Gorrie, C. L., and Holt, K. E. (2017). Unicycler: Resolving bacterial genome assemblies from short and long sequencing reads. PLoS Comput. Biol. 13:e1005595. doi: 10.1371/journal.pcbi.1005595
Wingett, S. W., and Andrews, S. (2018). FastQ Screen: A tool for multi-genome mapping and quality control. F1000Research 7:1338. doi: 10.12688/f1000research.15931.2
Zankari, E., Allesøe, R., Joensen, K. G., Cavaco, L. M., Lund, O., and Aarestrup, F. M. (2017). PointFinder: a novel web tool for WGS-based detection of antimicrobial resistance associated with chromosomal point mutations in bacterial pathogens. J. Antimicrob. Chemother. 72, 2764–2768. doi: 10.1093/jac/dkx217
Keywords: one-health approach, antibiotic resistance, zoonotic transmission, E. coli, livestock, pigs, meat-production
Citation: Hickman RA, Leangapichart T, Lunha K, Jiwakanon J, Angkititrakul S, Magnusson U, Sunde M and Järhult JD (2021) Exploring the Antibiotic Resistance Burden in Livestock, Livestock Handlers and Their Non-Livestock Handling Contacts: A One Health Perspective. Front. Microbiol. 12:651461. doi: 10.3389/fmicb.2021.651461
Received: 09 January 2021; Accepted: 16 March 2021;
Published: 20 April 2021.
Edited by:
Zulqarnain Baloch, Kunming University of Science and Technology, ChinaReviewed by:
Mohsin Khurshid, Government College University Faisalabad, PakistanCopyright © 2021 Hickman, Leangapichart, Lunha, Jiwakanon, Angkititrakul, Magnusson, Sunde and Järhult. This is an open-access article distributed under the terms of the Creative Commons Attribution License (CC BY). The use, distribution or reproduction in other forums is permitted, provided the original author(s) and the copyright owner(s) are credited and that the original publication in this journal is cited, in accordance with accepted academic practice. No use, distribution or reproduction is permitted which does not comply with these terms.
*Correspondence: Josef D. Järhult, am9zZWYuamFyaHVsdEBtZWRzY2kudXUuc2U=
Disclaimer: All claims expressed in this article are solely those of the authors and do not necessarily represent those of their affiliated organizations, or those of the publisher, the editors and the reviewers. Any product that may be evaluated in this article or claim that may be made by its manufacturer is not guaranteed or endorsed by the publisher.
Research integrity at Frontiers
Learn more about the work of our research integrity team to safeguard the quality of each article we publish.