- 1Aquatic Environmental Biotechnology and Nanotechnology Division, ICAR-Central Inland Fisheries Research Institute, Barrackpore, India
- 2Fisheries Resource Assessment and Informatics Division, ICAR-Central Inland Fisheries Research Institute, Barrackpore, India
- 3ICAR-Central Inland Fisheries Research Institute, Barrackpore, India
- 4Reservoir and Wetland Fisheries Division, ICAR-Central Inland Fisheries Research Institute, Barrackpore, India
The ultimate role of prokaryote (bacteria and archaea), the decomposer of the wetland ecosystem, depends on its community structure and its interaction with the environment. The present study has used three universal prokaryote primers to compare prokaryote community structure and diversity of three distinctly different wetlands. The study results revealed that α-diversity indices and phylogenetic differential abundance patterns did not differ significantly among primers, but they did differ significantly across wetlands. Microbial community composition revealed a distinct pattern for each primer in each wetland. Overall comparison of prokaryote communities in sediments of three wetlands revealed the highest prokaryote richness and diversity in Bhomra (freshwater wetland) followed by Malencho (brackish-water wetland) and East Kolkata wetland (EKW) (sewage-fed wetland). Indicator genus analysis identified 21, 4, and 29 unique indicator genera, having preferential abode for Bhomra, EKW, and Malencho, respectively. Prediction of potential roles of these microbes revealed a preference for sulfate-reducing microbes in Malencho and methanogens in Bhomra. The distinct phylogenetic differential abundance pattern, microbial abode preference, and their potential functional role predict ecosystem variables shaping microbial diversity. The variation in community composition of prokaryotes in response to ecosystem variables can serve as the most sensitive bioindicator of wetland ecosystem assessment and management.
Introduction
Wetlands are among the most productive ecosystems, hosting an array of ecosystem goods and services like sustaining biodiversity, ensuring livelihood, and nutritional security of millions of people (Sarkar and Borah, 2018; Sarkar et al., 2020). These wetlands play a vital role in regulating the Earth’s climate (Megonigal and Neubauer, 2019) and biogeochemical cycle (Cheung et al., 2018; Iliev et al., 2019), including organic matter decomposition, nutrient cycling, and greenhouse gas emissions (Sánchez, 2017; Zhang et al., 2019; Chandra and Kumar, 2020). The composition and functioning of microbiota—a decomposer of the ecosystem—depend highly on microbiota–environment interaction (Merkley et al., 2004; Cao et al., 2017). Their diversity and physiological dynamics are also concurrently affected by various natural and anthropogenic factors, viz., water table fluctuations, eutrophication, pollution, management intervention, and global warming (Jansson and Hofmockel, 2019; Zhou et al., 2020). The prokaryote (bacteria and archaea) sensitively responds to perturbations in the ecosystem; thereby, a change in its community diversity and structure can serve as the most sensitive and early bioindicator of ecosystem health assessment (Astudillo-García et al., 2019).
Extensive surveys based on next-generation high-throughput microbial 16S rRNA amplicon sequencing of various wetland sediments have revealed the potential effects of natural or anthropogenic processes on prokaryote structure at higher resolution (Adrados et al., 2014; Ansola et al., 2014; Horton et al., 2019; Li et al., 2019). More specifically, the bacterial communities are studied—on a large-scale and varied environments—more than archaeal communities, though archaea uniquely involved in methanogenic and other important pathways of the biogeochemical cycle (Offre et al., 2013; Fischer et al., 2016). Nowadays, the intense focus has shifted toward the underlying processes in sediment microbial assembly, identification of indicator taxa, and their functional properties from an initial focus typically on the description of phylum-level composition, diversity, and richness (Cao et al., 2017; Urakawa and Bernhard, 2017; De Mandal et al., 2020). Bacteria and archaea are evolutionarily and functionally diverse. And adequate wetland management requires genus-level assignment of 16S rRNA sequence data (Martens-Habbena et al., 2009; Hernández et al., 2015; Urakawa and Bernhard, 2017). Further, PCR conditions, sequencing methods, and specific short variable regions of 16S rRNA being sequenced might affect the richness and diversity of microbes of a unique ecosystem. Consequently, different sites or ecosystems must be evaluated under the same experimental conditions (Tremblay et al., 2015; Fouhy et al., 2016). Recently, Wei et al. (2019) compared China’s freshwater wetland and salt marsh to identify indicator bacterial taxa at the genus level. To the best of our knowledge, no comparative assessment of microbial diversity covering both archaea and bacteria in different wetland ecosystems is available; nor were their abode preferences identified under the same experimental conditions (Zhou et al., 2017; Wei et al., 2019; Zhao et al., 2020). Further, no evidence is available on the evaluation of widely used universal prokaryote primers for their use in distinct wetland ecosystems.
India has a wealth of wetland ecosystems covering an area of 1.33 million ha that support diverse and unique biodiversity. These wetlands are facing challenges emerging from both natural and anthropogenic disturbances for years (Raina et al., 2019; De Mandal et al., 2020; Sarkar et al., 2020). Research studies addressed the diversity and functional aspects of microbes in wetland ecosystems to a limited extent in the Indian context (Rathour et al., 2017; Raina et al., 2019; De Mandal et al., 2020). Therefore, the present study has worthily carried out a comparative analysis of microbial taxa (including bacteria and archaea) among three distinctly different wetland ecosystems (fresh, brackish, and sewage-fed)—under the same analytical condition. Three widely used universal prokaryote primers were evaluated for microbial identification of each wetland sediment. This research will provide the first evidence on the comparative evaluation of these primers, consequently bringing out more insights into the prokaryote profile involved in the biogeochemical cycle in distinct wetland ecosystems. The specific aim of the study is to evaluate three widely used universal prokaryote primers for (i) investigating microbial diversity, and community composition of three wetland sediments, and (ii) predicting microbial abode preference along with wetland-specific microbial indicators.
Materials and Methods
Description of Study Site
The study area belongs to West Bengal, India. Three distinct wetlands—a sewage-fed wetland (East Kolkata wetland; hereafter abbreviated as EKW), a freshwater wetland (Bhomra), and a brackish-water wetland (Malencho)—were selected from the study area (Figure 1). EKW, located in North 24 Pargana district, is one of the most unique sewage-fed natural wetland systems in the country, with an area of 12,500 ha. It has high conservation significance, getting designated as a Ramsar site (Ramsar Site No. 1208), and is recognized as the world’s largest sewage-fed aquaculture system. It provides a low-cost, efficient, and environmental friendly solid-waste and sewage treatment system for the city of Kolkata (Ghosh, 1998; Nag et al., 2019). EKW also supply water to nearby urban agricultural activities (Roy-Basu et al., 2020). The present study was conducted in one unit of EKW (22°59′14.59″N; 88°37′40.33″E) with a water surface area of 55 ha. Bhomra is a perennial and oxbow-shaped floodplain wetland—locally known as “Bhomra beel.” It is situated in the lower Gangetic plain (22°59′14.59″N; 88°37′40.33″E), Nadia district, with a water surface area of 45.7 ha (Figure 1). This wetland is classified as seasonally open and receives monsoon flood pulses from the river Ichhamati, a trans-boundary river between India and Bangladesh. The Malencho (Figure 1; 22°27′23.21″N; 88°18′43.91″E) is a brackish-water wetland—locally known as “Bheri” (man-made impoundments in coastal areas)—characterized by its alternate crop (rice and fish) cultivation practices. With a water surface area of 90 ha, the wetland is situated in the South 24 Pargana district. This ecosystem receives saline influx during high tide from the adjoining areas through sluices and is suitably used for polyculture of shrimp and fish species without incurring additional operational costs (Ghoshal et al., 2019).
Collection of Water and Sediment Samples
Sediment and water samples were collected from the selected wetlands during December–February 2017. Sediment samples were taken in triplicate from three sectors of the wetlands at the 15 cm of depth using Peterson grab to represent all types of microhabitats (Andrade et al., 2012). All the nine samples were pooled and thoroughly mixed before they were sieved through 2.0-mm mesh. The collected sediment samples were transferred immediately to a sterile plastic container and transported to the laboratory on ice within 6 h. Samples were subsequently divided into two parts. One part was air-dried under shade for analysis of physico-chemical properties, and the counterpart was stored at −20°C for molecular analysis of microbes. Water samples were collected from the subsurface layer using a standard water sampler (Das Sarkar et al., 2019).
Physico-Chemical Characterization of Water
Physico-chemical parameters of water—temperature, pH, specific conductivity, and salinity—were determined with a multi-parameter TestrTM 35 series (Eutech Instruments, Oakton, Singapore). Transparency of the water was measured using a 20-cm-diameter standard black and white Secchi disk (Strickland and Parsons, 1972). Further, the parameters—dissolved oxygen (DO; APHA, 2017), free carbon dioxide (free CO2), total alkalinity, total hardness, available nitrate-N (NO3–), and available phosphate-P (PO4–)—were determined following standard estimation processes (APHA, 2005). Chlorophyll a was estimated using the acetone extraction method and thereafter calculated from measured optical density (Jeffrey and Humphrey, 1975). The trophic state index (TSI) was estimated following Das Sarkar et al. (2020). Total organic carbon (TOC) and total inorganic carbon (TIC) in water samples (Urbansky, 2001) were determined in a TC Analyzer (OI Analytical, Model-Aurora 1030; Xylem Inc., Rye Brook, NY, United States).
Sediment Quality Parameter
Standard procedures were followed for the determination of soil pH (Thomas, 1996), specific conductivity (Rhoades, 1996), and total phosphorus (Olsen and Dean, 1965). Organic carbon (C) content of sediment was determined by dry combustion method at 900°C (Nelson and Sommers, 1996), and estimation of C was done using TC Analyzer (OI Analytical, Model-Aurora 1030; Xylem Inc., United States).
Isolation and Purification of DNA
DNA was isolated from sediment samples using Macherey-Nagel (Düren, Germany) NucleoSpin® Soil Kit according to the manufacturer’s instructions. Approximately 0.25 g of homogenized sediment was used for the isolation of genomic DNA. The quality of the extracted DNA was evaluated on 1% agarose gel, and the concentration of DNA was measured with a NanoDrop spectrophotometer (ND-1000; Isogen Life Science, De Meern, Netherlands).
Illumina-Based Sequencing of 16S rRNA Gene Amplicon
High-throughput paired-end sequencing of the prokaryote 16S rRNA gene was performed on the Illumina (San Diego, CA, United States) MiSeq platform. Briefly, the V3–V4 region of prokaryote 16S rRNA gene was amplified by the primer pair 341b4_F and 806_R (Lu et al., 2015), primer pair Pro341F and Pro805R (Takahashi et al., 2014), and modified primer pair N341b4_F and 806_R (present study; Supplementary Table 1). The modified primer pair in the present study was used to cover Verrucomicrobia and Opitutae groups, as it previously showed biases toward these groups (Takahashi et al., 2014; Lu et al., 2015). Further, three universal prokaryote primers covering both V3 and V4 regions were used to maximize taxonomic coverage and target specificity (Wilcox et al., 2013; Thijs et al., 2017; Schriefer et al., 2018).
Briefly, PCR was performed in 25 μl of mixture containing 5.0 μl of forward primer (1 μM), 5.0 μl of reverse primer (1 μM), 2.5 μl of DNA template (10 ng/μl), and 12.5 μl of 2 × KAPA HiFi HotStart ReadyMix (KAPA Biosystems, Woburn, MA, United States). The thermal program setting was as follows: 95°C for 3 min; 25 cycles of 95°C for 30 s, 55°C for 30 s, and 72°C for 30 s; and a final extension at 72°C for 5 min. PCR products were purified using an AMPure XP bead (Beckman Coulter, Brea, CA, United States) followed by second step PCR to attach dual indices and Illumina sequencing adapters using the Nextera XT Index Kit. Briefly, PCR was performed in 50 μl of mixture containing 5 μl of DNA, 5 μl of Nextera XT Index Primer 1 (N7xx), 5 μl of Nextera XT Index Primer 2 (S5xx), 25 μl of 2 × KAPA HiFi HotStart ReadyMix (KAPA Biosystems), and 10 μl of PCR-grade water. The thermal program was as follows: 95°C for 3 min; 8 cycles of 95°C for 30 s, 55°C for 30 s, and 72°C for 30 s; and a final extension at 72°C for 5 min followed by PCR cleanup using AMPure XP beads and Illumina MiSeq sequencing (2 × 300 bp).
Bioinformatics Processing
Figure 2 depicts the analytical framework of bioinformatics processing. First, the adapter and primers were removed from de-multiplexed raw pair-end reads–forward and reverse, using “cutadapt” (Martin, 2011). The core bioinformatics processing was then carried out, using DADA2 (Callahan et al., 2016) under R software (R Core Team, 2019). High-quality reads were retained through filtering and trimming, and low-quality reads to a consistent length. The filtering and trimming were done by setting truncation length of 284 and 200 bases for forward and reverse reads, respectively, and the maximum of one expected error. Dereplication further removed redundant sequences. A typical workflow further clusters sequence reads into operational taxonomic units (OTUs) with a fixed dissimilarity threshold. Instead, we used DADA2 method, which does not impose any arbitrary threshold, and thereby infer amplicon sequence variants (ASVs) more precisely (Callahan et al., 2016). This method relies on the model-based substitution of errors estimated from the sequence data itself. The “learnErrors” function generated the errors that were further plugged-in into the “DADA” function, inferring ASVs separately for forward and reverse sequences. The “mergePairs” function was used to merge forward and reverse ASVs to obtain the target length of 426 bp—with the typical overlap of 58 bp and a minimum overlap of 30 bps. The ASV count table was generated with the “makeSequenceTable” function, which was further refined by removing chimera using the “removeBimeraDenovo” function. Finally, the “assignTaxonomy” function, which uses naïve Bayesian classifier method (Wang et al., 2007), assigned taxonomy to ASVs—using the SILVA reference database (Quast et al., 2013) with 97% identity.
Based on the ASV count table, the data analysis set off with α-diversity measures—the rarefaction curve, estimates of richness (observed ASVs and expected ASVs), and diversity indices (Shanon and inverse Simpson)—using “vegan” (Oksanen et al., 2015) package under R software. An approximate pairwise test was performed to examine significant differences in the expected number of ASVs between primers within each wetland. Further, two-way ANOVA was applied to examine differences in diversity indices across wetlands and primers. The dominance pattern of ASVs was compared by “k-dominance” curves generated by plotting the cumulative proportion of abundances up to the k-th rank of the ASV.
Exploratory ordination technique—namely, non-metric multidimensional scaling (NMDS)—was used to compare the prokaryote community structure among samples. Two dissimilarity measures—weighted UniFrac (Lozupone et al., 2007) and abundance-based Bray–Curtis—were used for NMDS. The phylogenetic tree required to compute UniFrac was built with the “phangorn” package (Schliep et al., 2017), in R software; and it was then used with the “phyloseq” (McMurdie and Holmes, 2013) package of R. Further, analysis of similarity (ANOSIM) examined the statistical significance of dissimilarity among samples (primers and wetlands).
Analysis of Indicator Taxa
An indicator taxa analysis technique was used to diagnose the indicator taxa (phylum and genus levels), using two types of indices: “correlation” (Tichý and Chytrý, 2006) and “IndVal” (Dufrêne and Legendre, 1997). The correlation indices determined the taxon’s abode preference to a particular wetland, and the “IndVal” identified the diagnostic or bioindicator taxon for a particular wetland. The indicator taxa analysis was carried out by using the library “indispecies” (De Cáceres and Legendre, 2009) under the R software environment, which diagnosed two types of indicator taxa: single and second-order combination of taxa. The permutation test with 1,500 repetitions determined the statistical significance of both indices. The “IndVal” consists of two components: the positive predicting value of a taxon as an indicator (A) of a wetland in the present case and the positive value that determines how easy it is to detect an ASV (B) in a wetland. Dufrêne and Legendre (1997) argued that a taxon with high values of A and B is easy to detect as well as to predict, qualifying as a good bioindicator. But the taxon with either high A or high B refers to asymmetrical indicator taxon. The species level similarity search for indicator genus was conducted using National Center for Biotechnology Information (NCBI), Basic Local Alignment Search Tool (BLAST), against the 16S ribosomal database.
Results
Physico-Chemical Properties of Water and Sediment
The physico-chemical properties of water and sediment samples of the three wetlands varied considerably (Table 1). The wetland Malencho was found to be more saline in comparison with EKW and Bhomra. Among the two freshwater wetlands, EKW showed more conductivity due to the regular intake of sewage. The nutrients phosphate and nitrate were also the lowest in Bhomra compared with EKW, whereas the highest phosphate was observed in Malencho and nitrate in EKW. The TSI values revealed a higher trophic state in EKW and Malencho than Bhomra. Bhomra has the highest water TOC, followed by Malencho and EKW, whereas the lowest TIC was observed in Bhomra and highest in EKW. The average water pH varied from 7.94 to 9.14, with the highest and lowest values in Bhomra and EKW, respectively. With respect to sediment parameters, the average pH varied from 7.58 to 8.00, with the highest and lowest values observed in Bhomra and Malencho, respectively. Carbon content was noticeably the highest in Bhomra followed by nearly equal and low levels in Malencho and EKW. Specific conductivity was high in EKW and Malencho and low in Bhomra. The highest level of total phosphorus was detected in EKW and the lowest in Malencho.
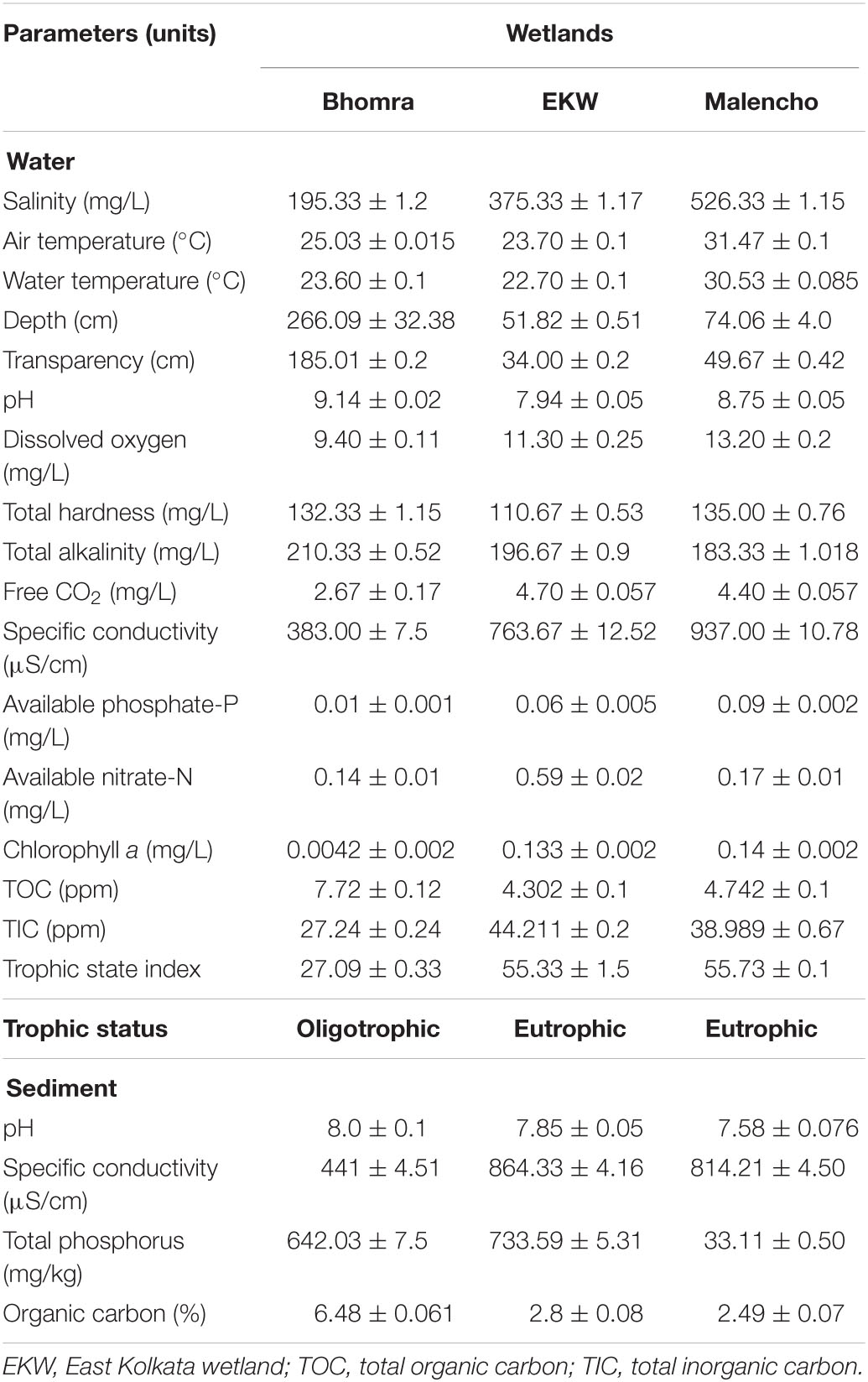
Table 1. Physico-chemical parameters (mean ± SD) of water and sediment samples in Bhomra (freshwater), EKW (sewage-fed), and Malencho (brackish-water) wetlands.
Read Output and Parametric Analyses of Illumina MiSeq Pyrosequencing Data
The Illumina MiSeq sequencing generated a total of 3,502,469 raw reads for the nine samples—the average number of reads per sample was 389,163, ranging between 262,163 and 461,999. Further bioinformatics processing retained a total of 1,277,354 reads, ranging between 93,436 and 209,785 (Supplementary Table 2).
Rarefaction curves were generated with a subsample size of 93,346, to identify the minimum number of observed ASVs in all samples. In general, the primer 341b4_F/806_R showed the steepest slope, followed by N341b4_F/806_R and Pro341F/Pro805R (Figure 3A); and among the wetlands, Bhomra showed the steepest slope, followed by Malencho and EKW (Figure 3B). In EKW, primer N341b4_F/806_R showed the steepest slope, followed by N341b4_F/806_R and Pro341F/Pro805R. The rarefaction curve attained saturation of around 70,000 sequence reads for all the samples signifying the sequencing depth adequacy. The estimated expected number of ASVs (with a random subsample size of 70,000) was 1,797 ± 4 to 4,719 ± 9 for nine samples (Supplementary Table 3).
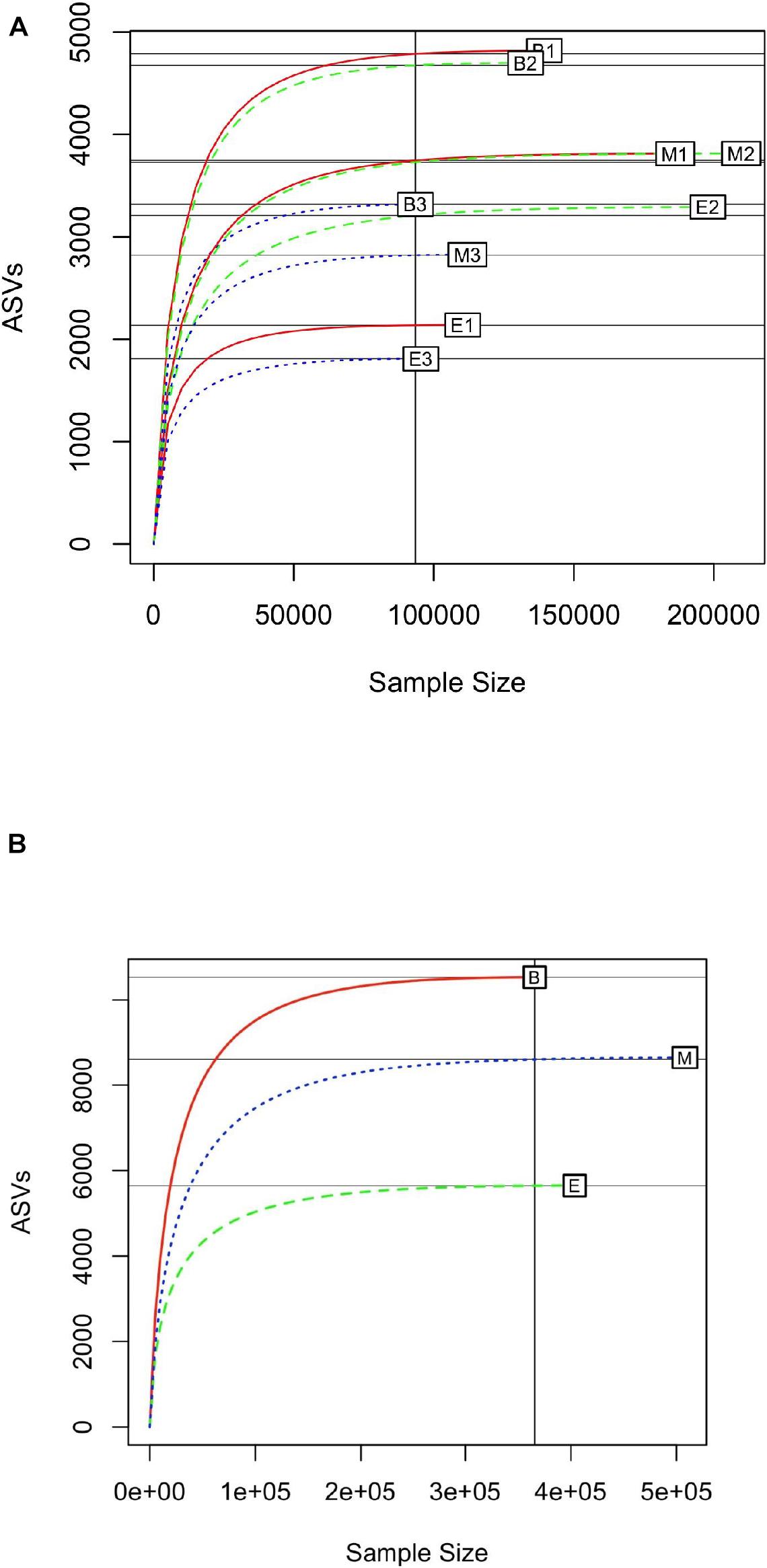
Figure 3. (A) Rarefaction curve of 16S prokaryote sequences detected by three universal prokaryote primers (341b4_F/806_R, N341b4_F/806_R, and Pro341F/Pro805R) in Bhomra (freshwater), EKW (sewage-fed), and Malencho (brackish-water) wetlands. The abbreviations and numerics used are as follows: B, Bhomra; E, EKW; M, Malencho; 1, 341b4_F/806_R; 2, N341b4_F/806_R; 3, Pro341F/Pro805R; EKW, East Kolkata wetland. (B) Rarefaction curve of 16S prokaryote sequences detected in three wetland sediments (Bhomra, EKW, and Malencho). The data are pooled over three primers in each wetland. The abbreviations used are as follows: B, Bhomra; E, EKW; M, Malencho.
α-Diversity indices of ASVs indicated that diversity indices did not differ significantly with primers; however, they were significantly different across wetlands (ANOVA, p-value < 0.05). Specifically, the Shannon index of Bhomra was significantly higher than that of EKW and Malencho, and similar results were obtained using inverse Simpson’s index (Figure 4). Concerning the ASV richness and the number of observed and expected ASVs, EKW was significantly lower than the other two wetlands (Figure 4). Diversity indices were statistically insignificant among primers (Figure 5).
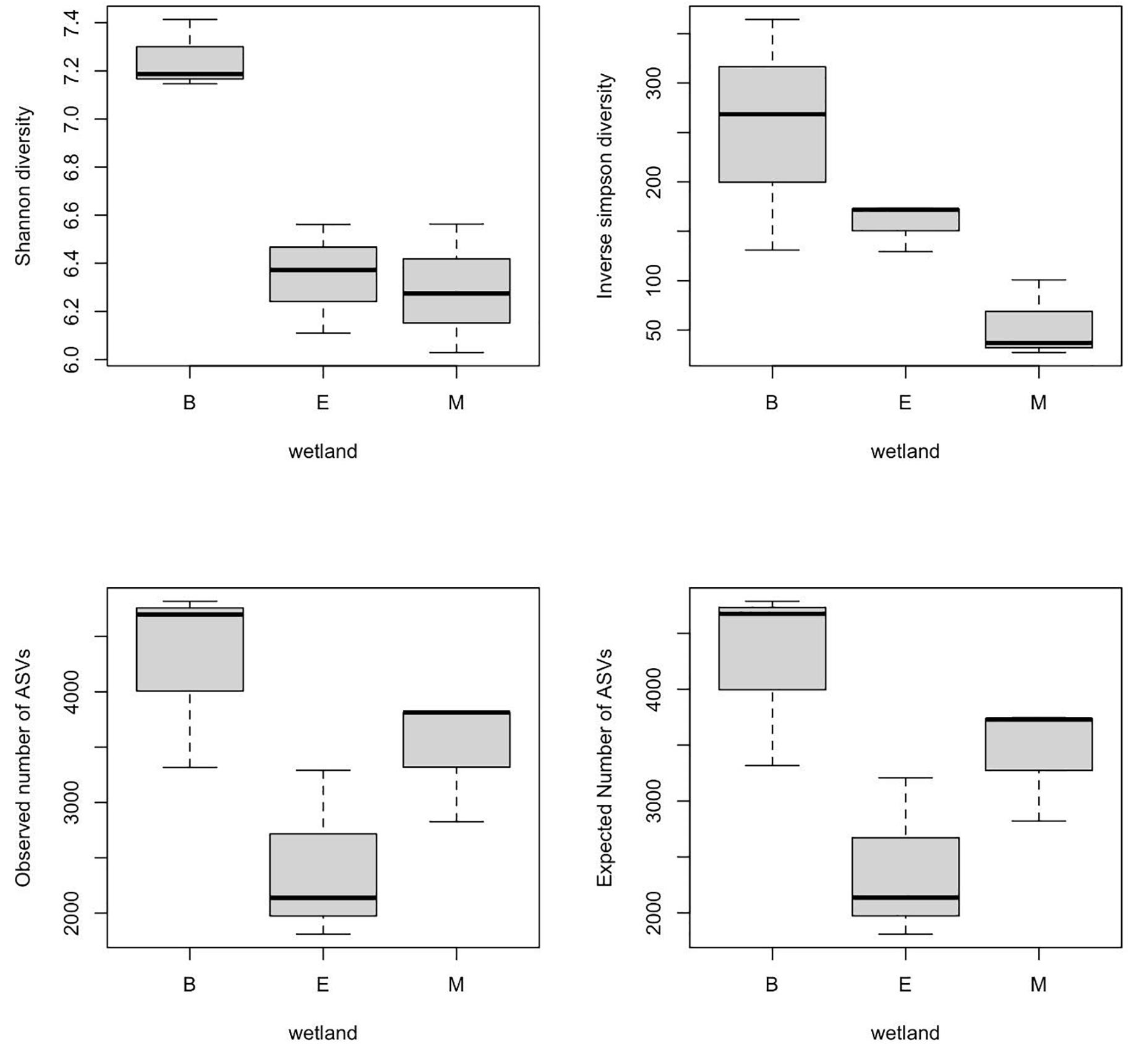
Figure 4. Wetland-wise comparison of different prokaryote ASV α-diversity indices (Shanon and inverse Simpson) and richness (observed ASVs and expected ASVs). The data are pooled over three primers for each wetland. The abbreviations used are as follows: B, Bhomra; E, EKW; M, Malencho; ASV, amplicon sequence variant; EKW, East Kolkata wetland.
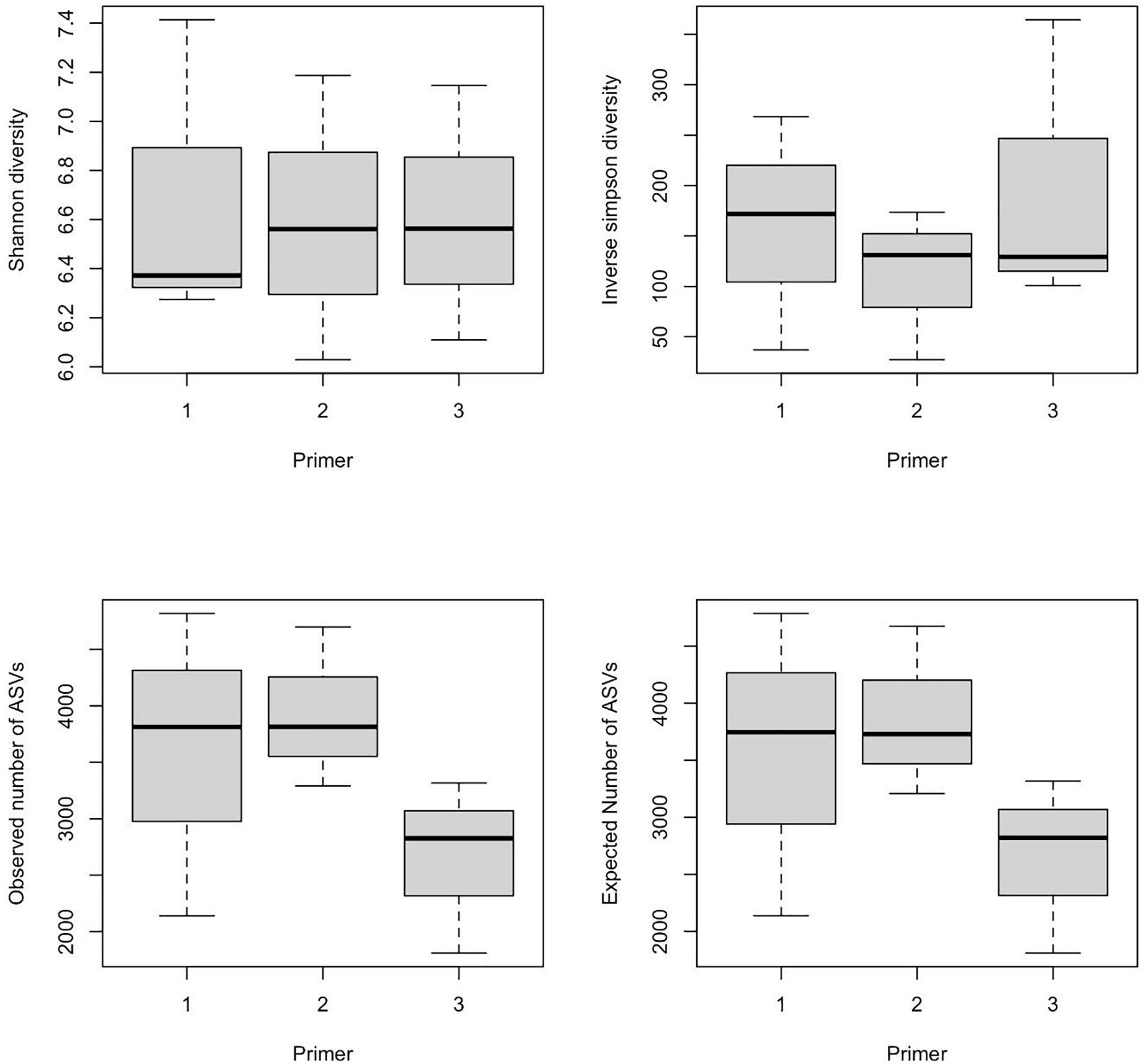
Figure 5. Primer-wise comparison of different prokaryote ASV α-diversity indices (Shanon and inverse Simpson) and richness (observed ASVs and expected ASVs). The data are pooled over three wetlands for each primer. The numerics and abbreviations used are as follows: 1, 341b4_F/806_R; 2, N341b4_F/806_R; 3, Pro341F/Pro805R; ASV, amplicon sequence variant.
The “k-dominance” curve (Figure 6) indicated fewer ASVs dominating the abundance distribution of primer Pro341F/Pro805R than those of the other two primers for all the wetlands. For example, ASVs ranked up to 1,000 accounted for 95%, 91%, and 87% of ASVs, respectively, for Pro341F/Pro805R, 341b4_F/806_R, and N341b4_F/806_R in EKW.
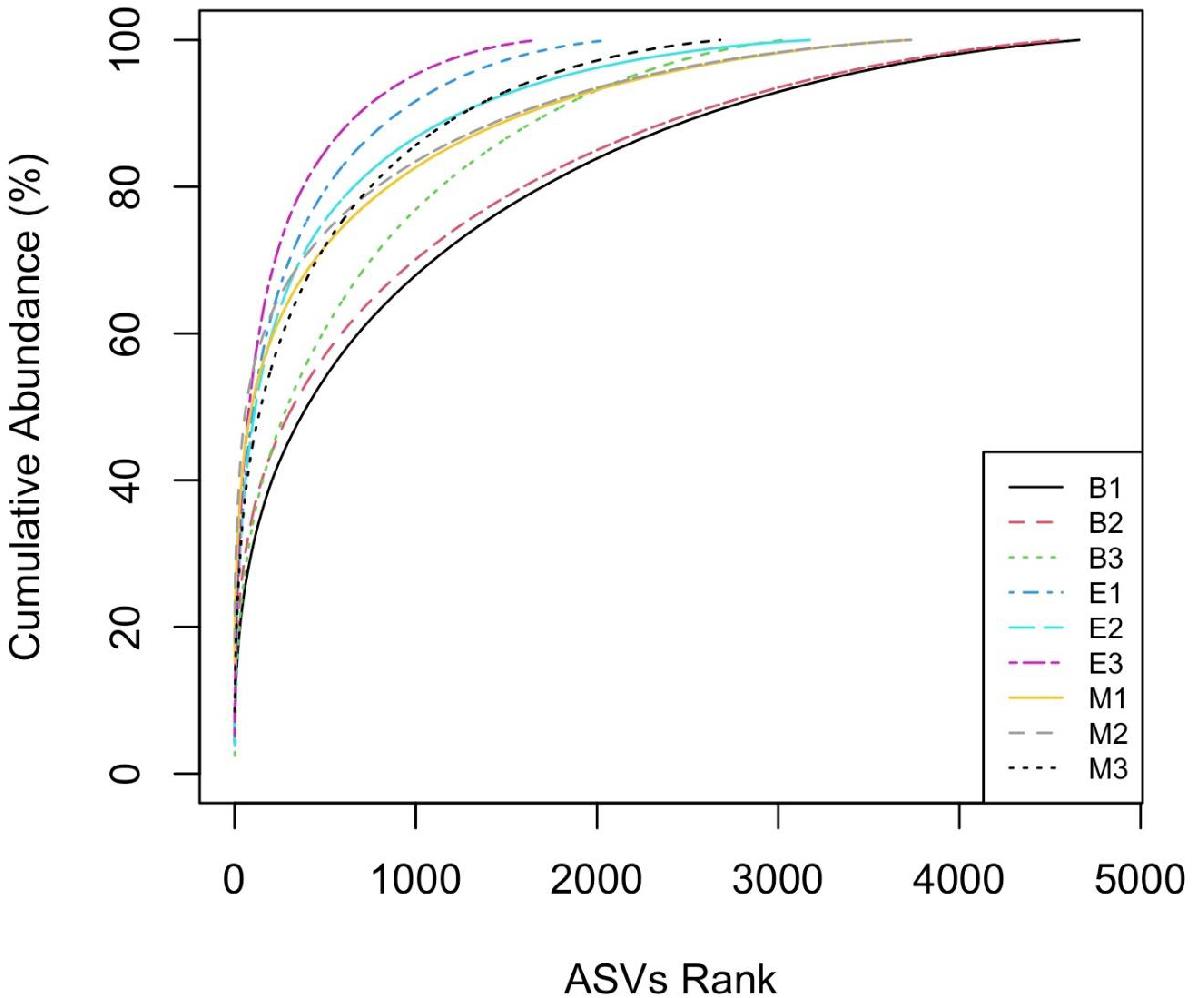
Figure 6. Comparative “k-dominance” curve to predict dominance pattern of ASVs for three universal prokaryote primers in each wetland. The abbreviations and numerics used are as follows: B, Bhomra; E, EKW; M, Malencho; 1, 341b4_F/806_R; 2, N341b4_F/806_R; 3, Pro341F/Pro805R; ASV, amplicon sequence variant; EKW, East Kolkata wetland.
Prokaryote Community Structure
Figure 7 suggests dissimilar community composition among wetlands but similar community composition among primers, according to phylogenetic relatedness (weighted UniFrac). Separate ANOSIM for wetlands and primers further ensured the statistical significance of those dissimilarities (Figure 8). Based on the analysis of the Bray–Curtis dissimilarity, a differential non-phylogenetic abundance pattern existed among the primers, which was statistically significant (Figure 9). Specifically, the community composition of the primer Pro341F/Pro805R evinced a quite dissimilar composition to the two primers (Figure 9). However, wetlands were not significantly different in community composition based on pool data over primers, albeit visual inspection suggests compositional dissimilarity between Malencho and the other two wetlands (Figure 9).
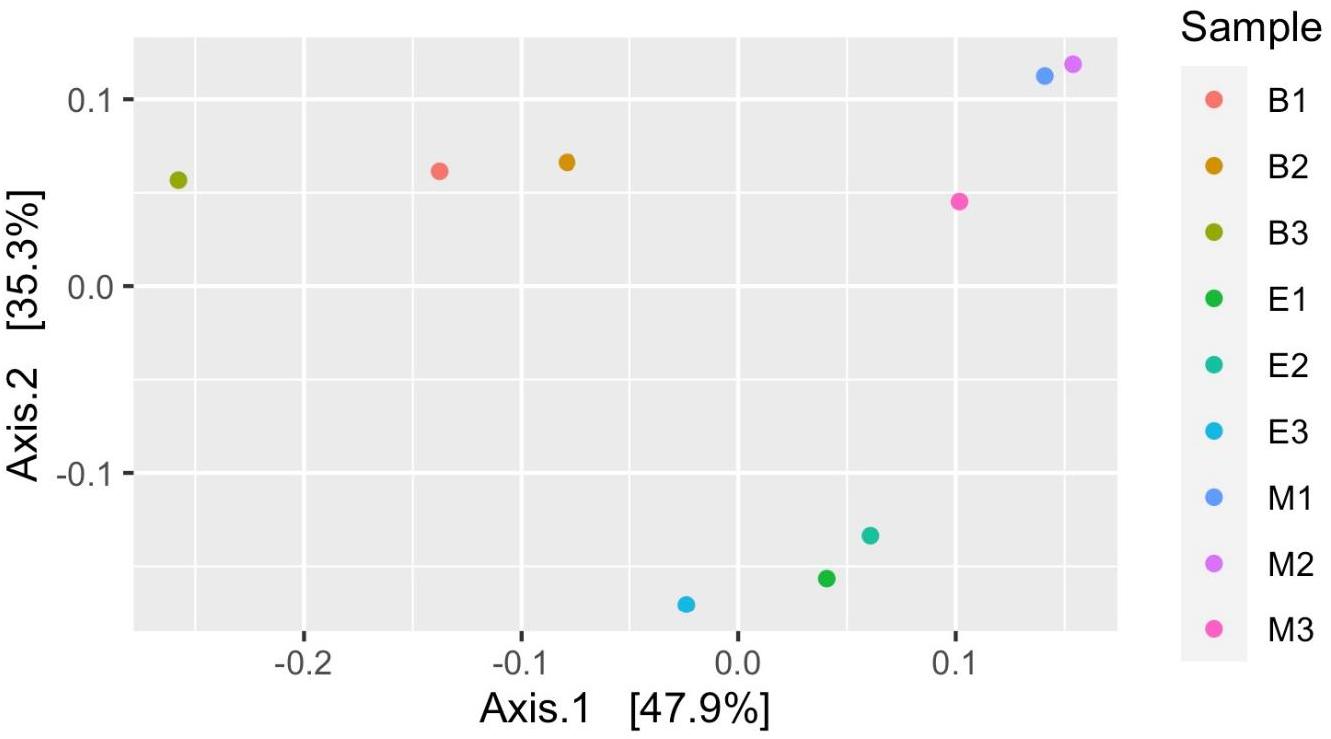
Figure 7. Prokaryote community structure (ASV level) among samples based on weighted UniFrac distance (phylogenetic relatedness). Each sample is represented as a dot and is colored distinctly based on wetland sediment and primer combination. The abbreviations and numerics used are as follows: B, Bhomra; E, EKW; M, Malencho; 1, 341b4_F/806_R; 2, N341b4_F/806_R; 3, Pro341F/Pro805R; ASV, amplicon sequence variant; EKW, East Kolkata wetland.
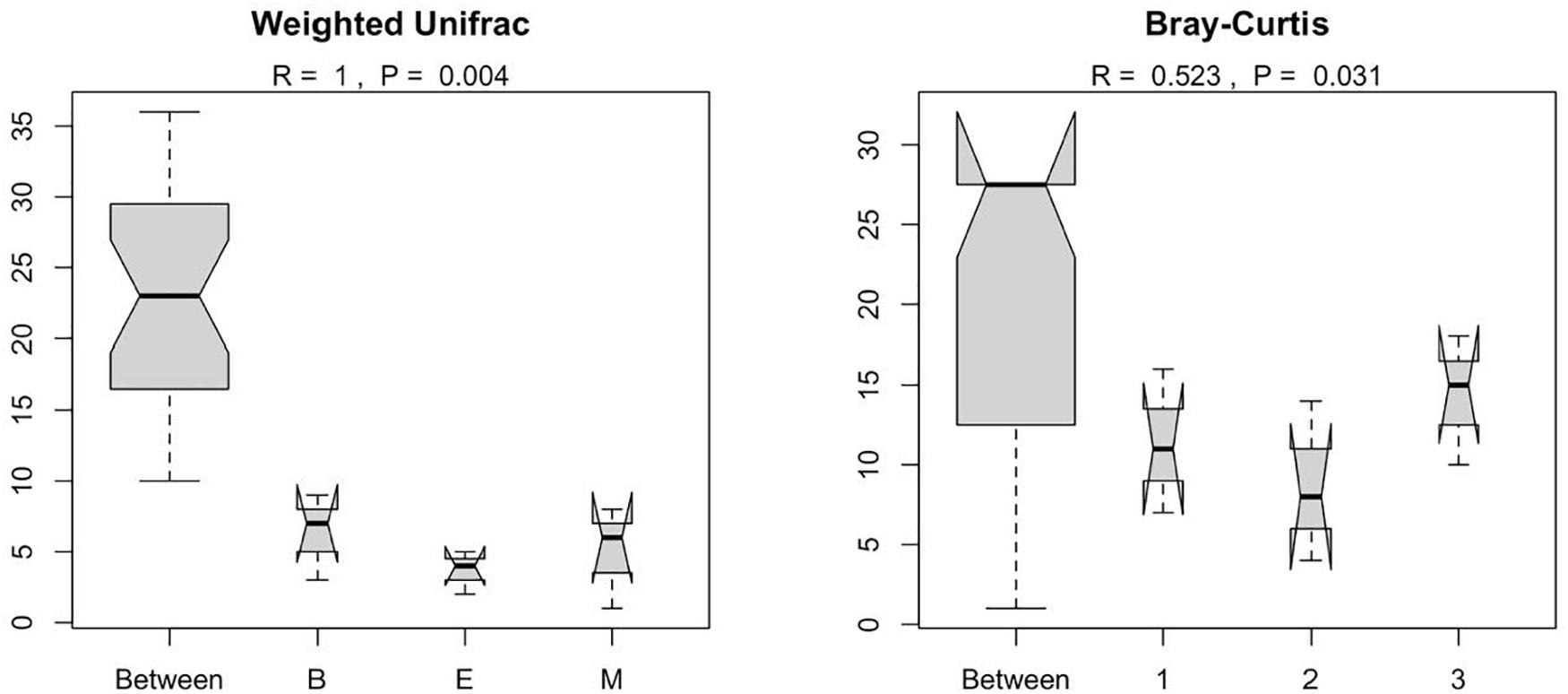
Figure 8. Results of ANOSIM showing the statistical significance of differential prokaryote community structure (based on weighted UniFrac and Bray–Curtis) between wetlands and primers; The abbreviations and numerics used are as follows: B, Bhomra; E, EKW; M, Malencho; 1, 341b4_F/806_R; 2, N341b4_F/806_R; 3, Pro341F/Pro805R; EKW, East Kolkata wetland.
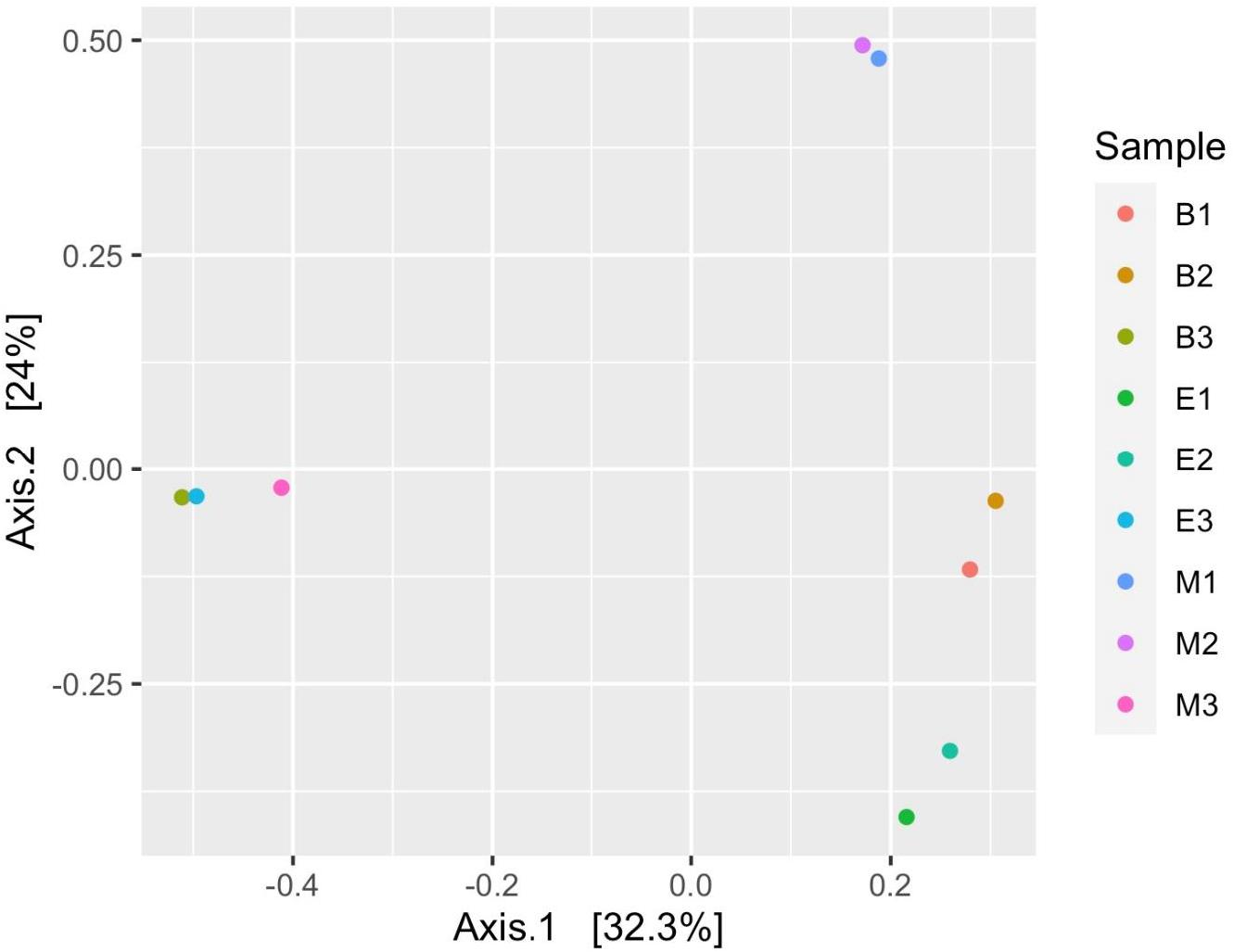
Figure 9. Prokaryote (ASV level) compositional dissimilarity among samples, based on the Bray–Curtis dissimilarity (non-phylogenetic relatedness). Each sample is represented as a dot and is colored distinctly based on wetland sediment and primer combination. The abbreviations and numerics used are as follows: B, Bhomra; E, EKW; M, Malencho; 1, 341b4_F/806_R; 2, N341b4_F/806_R; 3, Pro341F/Pro805R; ASV, amplicon sequence variant; EKW, East Kolkata wetland.
Phylum-Level Taxonomic Distribution
All the nine samples consisted of 47 phyla. The 12 most abundant phyla included Proteobacteria, Firmicutes, Acidobacteria, Euryarchaeota, Actinobacteria, Chloroflexi, Bacteroidetes, Aminicenantes, Planctomycetes, Crenarchaeota, Cyanobacteria, and Verrucomicrobia (Figure 10). Of the top 12 abundant phyla, Proteobacteria, Acidobacteria, Chloroflexi, Planctomycetes, Cyanobacteria, and Verrucomicrobia were more abundant in EKW. The relative abundance of Euryarchaeota, Aminicenantes, and Crenarchaeota was more in Bhomra, while the relative abundance of Firmicutes, Bacteroidetes, and Actinobacteria was more in Malencho.
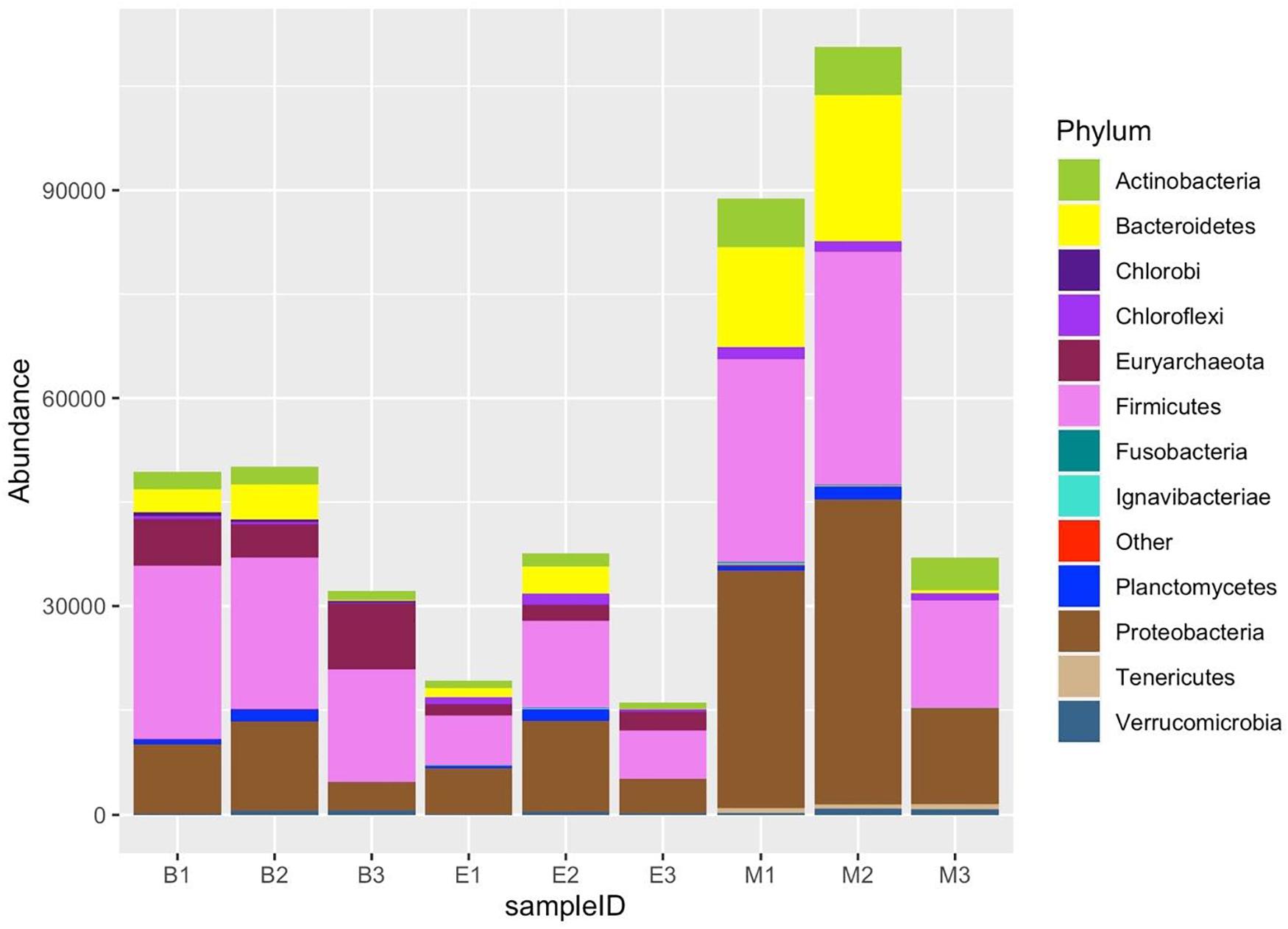
Figure 10. Twelve most abundant phyla, detected by three universal prokaryote primers in each wetland. The remaining taxa with lower abundance are classified into “Other.” The abbreviations and numerics used are as follows: B, Bhomra; E, EKW; M, Malencho; 1, 341b4_F/806_R; 2, N341b4_F/806_R; 3, Pro341F/Pro805R; EKW, East Kolkata wetland.
Proteobacteria was the most dominant phylum in all wetlands. Firmicutes was the second most abundant phylum in Bhomra and Malencho, whereas Acidobacteria was the second most abundant phylum in EKW. Primer-specific differences were observed in many higher-abundance group phyla (Supplementary Table 4). Additionally, primer-specific difference was noticed in the case of many low abundant phyla (Supplementary Table 4)—an abundance of < 1% in all the samples.
Sharing pattern of primers showed primers—341b4_F/806_R, N341b4_F/806_R, and Pro341F/Pro805R—accounted for more than 90% of the ASVs. Wetland-specific phyla sharing was as follows: 32 in Bhomra and 30 in EKW and Malencho (Supplementary Figure 1).
Genus and Amplicon Sequence Variant Level Analysis
Of 254 genera recognized altogether, Figure 11 shows the distribution of the top 14 most abundant genera: Clostridium sensu stricto, Psychrobacter, Methanothrix, Bacillus, Romboutsia, Planomicrobium, Prevotella, Sporacetigenium, Trichococcus, Gaiella, Planococcus, Bifidobacterium, Ilumatobacter, and Litorilinea. The genera excluding the top 14 jointly dominated the abundance in Bhomra and EKW. Clostridium sensu stricto and Psychrobacter were the two most dominant in Bhomra; Psychrobacter and Trichococcus were the two most dominant in EKW; Methanothrix and Trichococcus were less abundant in Malencho wetland. The genus Sporacetigenium could not be identified in EKW.
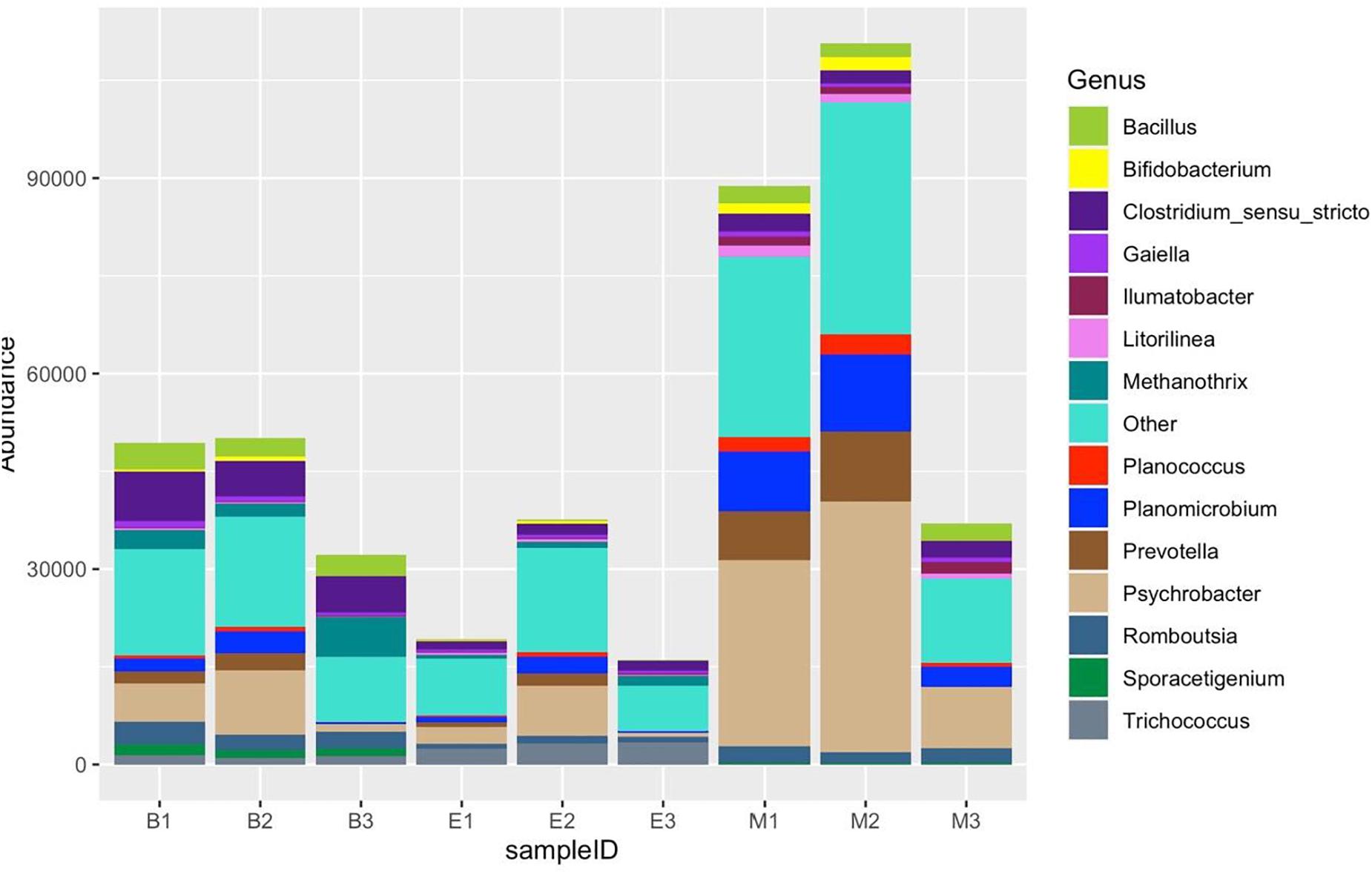
Figure 11. Fourteen most abundant genera, detected by three universal prokaryote primers in each wetland. The remaining taxa with lower abundance are classified into “Other.” The abbreviations and numerics used are as follows: B, Bhomra; E, EKW; M, Malencho; 1, 341b4_F/806_R; 2, N341b4_F/806_R; 3, Pro341F/Pro805R; EKW, East Kolkata wetland.
The numbers of the shared genera of primers within wetlands were 150, 83, and 178 for Bhomra, EKW, and Malencho, respectively (Supplementary Figure 2). The same for wetland among primers were 104, 131, and 58 for primers 341b4_F/806_R, N341b4_F/806_R, and Pro341F/Pro805R, respectively. As for ASV sharing, a few ASVs—i.e., 123 (1.24%), 159 (1.47%), and 51 (0.68%)—were shared by primers 341b4_F/806_R, N341b4_F/806_R, and Pro341F/Pro805R, respectively, when pooled over wetlands. Two primers—341b4_F/806_R and N341b4_F/806_R—shared 2,299 (21%), 1,591 (28%), and 1,800 (20.8%) ASVs within Bhomra, EKW, and Malencho, respectively. ASV detection capability of the primer— Pro341F/Pro805R—was completely distinct (Figure 12) from that of the other two primers in wetlands. The total ASVs—when pooled over wetlands—identified were more for newly developed primer (N341b4_F/806_R) than for the other two.
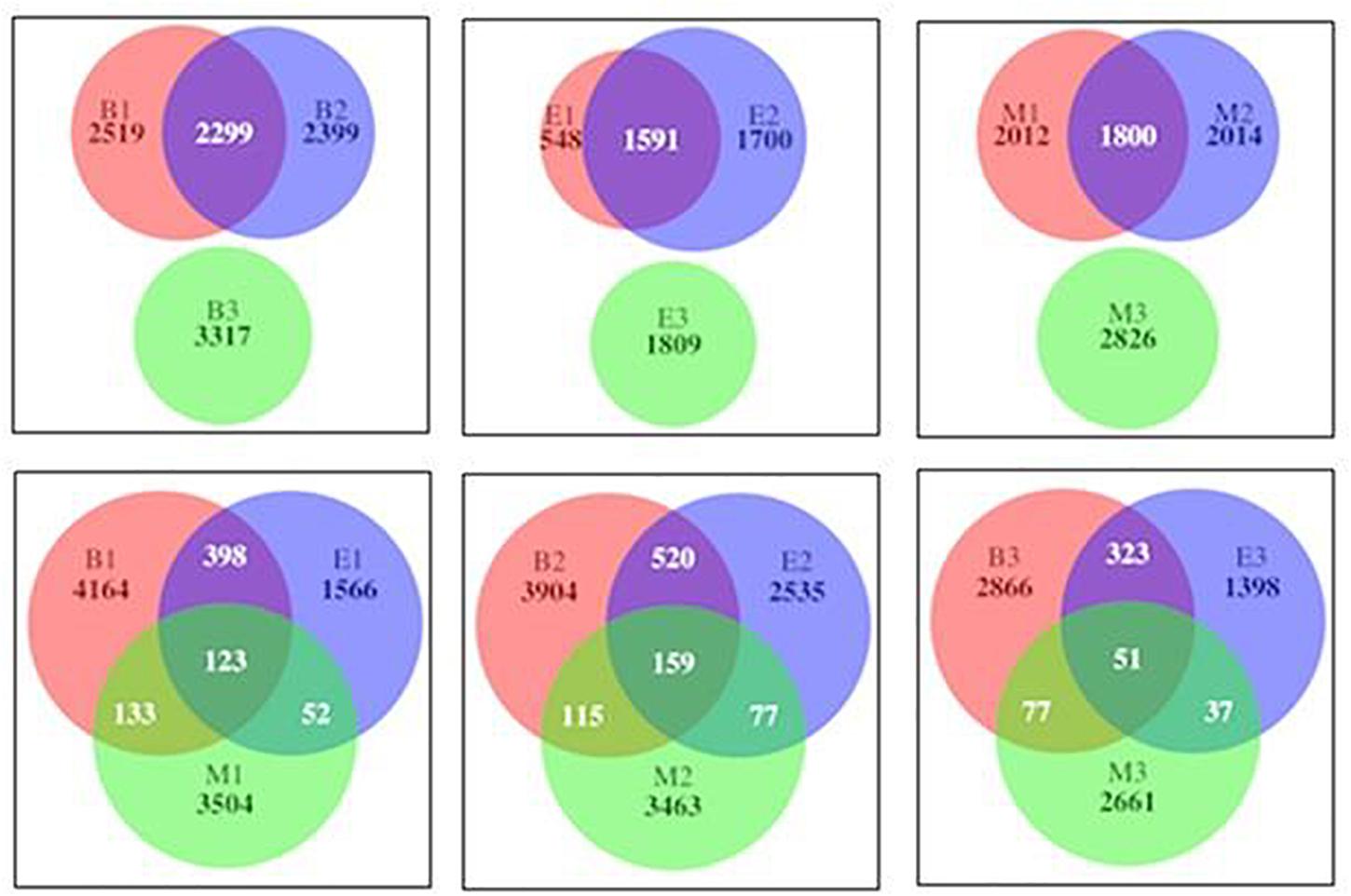
Figure 12. Venn diagram showing number of shared and specific prokaryote ASVs among primers within wetlands (above) and among wetlands of primers (below). The abbreviations and numerics used are as follows: B, Bhomra; E, EKW; M, Malencho; 1, 341b4_F/806_R; 2, N341b4_F/806_R; 3, Pro341F/Pro805R; ASV, amplicon sequence variant; EKW, East Kolkata wetland.
Abode Preference and Ecosystem-Specific Indicator Microbial Taxa
The indicator analysis based on correlation index identified 11 phyla as an indicator for wetland abode preference (Table 2). Acetothermia has a high positive index value (= 0.944), indicating a tendency to live in EKW, whereas it avoids staying in Bhomra and Malencho, as it has moderate negative values. Actinobacteria and Tenericutes preferred Malencho to Bhomra and EKW as their abode. The other phyla, viz., Aminicenantes, Chlorobi, Crenarchaeota, Euryarchaeota, Spirochaetes, and Thaumarchaeota, preferred Bhomra as their abode.
The threshold cutoff value of 0.90 for both A and B revealed diagnostic phyla for three wetlands, as follows: Chlorobi and Thaumarchaeota for Bhomra; Acetothermia for EKW; and Tenericutes for Malencho (p-values < 0.05). Further, of 989 second-order phyla, 173 were statistically significant indicators (Supplementary Table 5).
For the genus, the indicator analysis indicated statistical significance (p-value < 0.05) in 142 indicator genera (Supplementary Table 6). Among those, 38, 10, and 43 genera were more associated with Bhomra, EKW, and Malencho, respectively, at a threshold correlation index > 0.90. Setting the value of both A and B as 1.00 means that the genus is detectable as well as predictable with certainty; the analysis showed 21, 4, and 29 genera as the diagnostic genera for Bhomra, EKW, and Malencho, respectively (Supplementary Table 6). Most of the diagnostic genera had sequence similarities (NCBI BLAST) greater than 97% (Table 3) with their closest relative (at species level).
Discussion
Richness and Diversity of Microbial Taxa
Wetlands are recognized as a hot spot for studying microbial diversity and their role in ecology. However, research investigating microbial communities in different types of wetlands has seldom been reported (Gutknecht et al., 2006; Iliev et al., 2019; Wang et al., 2020). The microbial richness, evenness, and diversity are very important early indicators to predict the possible variation in community composition and the systems functionalities (Santos et al., 2019). In the present study, we compared the richness and diversity indices of microbial ASVs obtained from next-generation sequencing (NGS) following the same experimental conditions as suggested by Urakawa et al. (2017), to avoid biases associated with target amplification and bioinformatics analysis (Huse et al., 2010; Wang et al., 2012; Thijs et al., 2017).
The saturation of rarefaction curve in the present study around 70,000 sequence reads indicates that the probability of encountering a new ASV beyond 70,000 reads is almost negligible. Bhomra showed the steepest slope of the rarefaction curve among the wetlands, indicating higher chances of ASV detection rate followed by Malencho and EKW. Similarly, primer combination 341b4_F/806_R showed the steepest slope in Bhomra and Malencho. Therefore, the ASV detection rate is higher by primer 341b4_F/806_R than other primers in these two wetlands. The EKW revealed a noticeably different pattern of ASVs, wherein the detection rate of primer N341b4_F/806_R is the highest. This indicates the better performance of primer N341b4_F/806_R for a sewage-fed wetland. Further, the estimated expected number of ASVs for all samples suggested that the primer 341b4_F/806_R has the highest ASV detection capability followed by N341b4_F/806_R and Pro341F/Pro805R within Bhomra and Malencho wetlands. On the contrary, primer N341b4_F/806_R has the highest ASV detection capability followed by 341b4_F/806_R and Pro341F/Pro805R within EKW.
The “k-dominance” curve indicates more representation of certain taxa by primer Pro341F/Pro805R and less coverage of all other taxa in three wetlands, which is in agreement with Thijs et al. (2017). A similar pattern was also evident from the lowest observed and expected numbers of ASVs identified by the primer for all the wetlands. Contrary to the richness, Shannon’s diversity index is much less sensitive to sequencing and PCR errors (Kunin et al., 2010; Wu et al., 2010; Zinger et al., 2011). The Shannon indices of the three types of sediments determined in the present study (6.3 to 6.9) were comparable with those recently reported using pyrosequencing methods, even though different methodologies were employed (Thijs et al., 2017; Iliev et al., 2019; Wang et al., 2020).
Prokaryote community composition (weighted UniFrac distance matrix) was similar between primers but differed between wetlands. Thijs et al. (2017) found the bulk soil and rhizosphere soil clustered with high concordance based on weighted UniFrac distance matrix per primer pairs. However, Iliev et al. (2019) showed significant differences in the taxa composition between a natural wetland and rice paddies based on MANOVA and ANOSIM. Our result based on weighted UniFrac is also supported by ANOSIM analysis.
The present study results revealed the highest prokaryote richness and diversity in the freshwater wetland (Bhomra), followed by the brackish-water bheri (Malencho) and the sewage-fed wetland (EKW). For richness, and the number of observed and expected ASVs, the three wetlands behaved distinctly. Higher richness values were observed in two natural wetlands, Bhomra and Malencho, and very low richness value was observed in sewage-fed wetland. Further, the Shannon diversity indices differentiated Bhomra in one group and Malencho and EKW in another group. The Simpson index further differentiates the diversity between EKW and Malencho. The highest microbial diversity in Bhomra wetland may be attributed to nutrient-rich environment and more availability of organic matter in sediment due to decay of aquatic macrophytes present in the system (Decamp and Warren, 2001; Urakawa and Bernhard, 2017). The higher soil organic carbon in Bhomra due to the decay of macrophytes provides buffering effects on the environment (Marschner et al., 2003), which supports higher microbial diversity. The lower prokaryote diversity in Malencho compared with Bhomra is also consistent with recent meta-analysis results—higher levels of diversity of both bacterial and archaeal communities in inland freshwater than those of saline waters (Auguet et al., 2010; Barberán and Casamayor, 2010; Wang et al., 2012). The low prokaryote diversity in EKW might be due to sewage, which decreased the bacterial and archaeal diversity (Gu et al., 2018). It has also been reported that compared with natural wetlands, constructed wetlands used to treat city effluents have lower microbial diversity with higher abundance and functions (Hartman et al., 2008; Urakawa et al., 2017). Furthermore, the higher microbial diversity signals pristine, healthy, and balanced microbial community—the low microbial diversity is not desirable, implicating distressed communities in terms of ecosystem functioning (Bell et al., 2005; Delgado-Baquerizo et al., 2016). The Bhomra (with the highest diversity) is a less perturbed wetland than the sewage-fed EKW (with the lowest diversity).
Preferential Wetland Abode Suitability of Phyla and Genera
The dominant prokaryote communities detected in wetland sediments include Proteobacteria, Firmicutes, Acidobacteria, Euryarchaeota, Actinobacteria, Chloroflexi, Bacteroidetes, Aminicenantes, Planctomycetes, Crenarchaeota, Cyanobacteria, and Verrucomicrobia. Many other studies also reported Proteobacteria, Bacteroidetes, Firmicutes, Acidobacteria, and Actinobacteria as dominant phyla in sediment (Hartman et al., 2008; Ligi et al., 2014; Lv et al., 2014; Sánchez, 2017), whereas Chloroflexi, Planctomycetes, Cyanobacteria, and Verrucomicrobia contribute a large fraction of microbial communities occasionally (Dorador et al., 2013; Peralta et al., 2013). We observed Firmicutes as the second dominant taxon (24.47–26.72%) in Bhomra and Malencho, whereas Acidobacteria (11.55%) in EKW. However, Lv et al. (2014) identified Bacteroidetes as the second dominant taxa (Lv et al., 2014). The microbes of these groups play a distinct role in the carbon cycle; they are capable of degrading polysaccharides—including cellulose and hemicellulose (Chapman et al., 2017; Speirs et al., 2019)—and have been identified in various habitats worldwide.
Taxonomic classification of bacteria and archaea at a higher rank, for example, phylum level, exhibits ecological coherence; and their community composition may be a valuable indicator in predicting ecosystem function (Philippot et al., 2010; Gu et al., 2018). Our results evidently reflected it: the wetland revealed a distinct pattern of the microbial community composition at the phylum level. Similar to other studies (Liu et al., 2014; Fang et al., 2015; Sun et al., 2019), the Proteobacteria dominates the community, which might be due to its diverse role in the transformation of major compounds like organic matter, nitrogen, and sulfur (Wang et al., 2016; Zheng et al., 2018). Also, the higher abundance of Proteobacteria in EKW might be due to the availability of more biodegradable organic pollutants (Cheng et al., 2014; Liu et al., 2014; Zhang et al., 2014). Apart from Proteobacteria, we found Acidobacteria, Chloroflexi, Planctomycetes, and Cyanobacteria in the EKW, similar to the findings of Torres et al. (2019) in the polluted mangrove ecosystem. It indicates that pollutants might regulate these microbial communities. Many members of these phyla have also been found to play a vital role in some processes like sewage remediation by ammonia oxidation (Wiegand et al., 2020), metal-ion-contaminated soil recovery by detoxification (Jiang et al., 2013; Torres et al., 2019), organic matter (Yamada et al., 2005; Kawaichi et al., 2013; Torres et al., 2019) and xenobiotic compound (Huang et al., 2016; Cao et al., 2017; Torres et al., 2019) decomposition and eutrophication. We also discovered a higher abundance of Verrucomicrobia in EKW, which could be attributed to high nutrient availability (He et al., 2017).
Higher abundance of saprophytic microbes—e.g., Bacteroidetes, Firmicutes, and Actinobacteria—in the sediment of the brackish-water wetland than freshwater is comparable with sediment microbial diversity of Pearl river wetland (Wang et al., 2012). These bacterial groups contain the majority of the genes encoding enzymes involved in the degradation of the soluble organic compound for the marine carbon cycle (Chapman et al., 2017), particularly those involved in the degradation of high-molecular-weight compounds such as cellulose, agar, chitin, chitosan, and amino polysaccharides (Dorador et al., 2009; Chater, 2016; Lacombe-Harvey et al., 2018). We also detected the second-order indicator phyla combinations; however, their ecological significance has yet to be determined.
In the Bhomra wetland, the archaea (Euryarchaeota and Crenarchaeota) and bacteria Aminicenantes formed the dominant microbiota. Inconsistent results available on the major archaeal groups present in wetland ecosystems (Li et al., 2017). A metadata analysis of prokaryote diversity revealed Euryarchaeota and Crenarchaeota as two major phyla in wetlands with a more relative abundance of Euryarchaeota (Lv et al., 2014)—similar to the present finding. The higher abundance of archaea in Bhomra might be due to the presence of diverse aquatic vegetation (Li et al., 2017), higher soluble organic matter (Steinmuller and Chambers, 2019), and less pollutants (Wei et al., 2019). Aminicenantes represent poorly characterized bacterial lineage in various ecosystems. They are most abundant in the freshwater environment and least abundant in heavy metal-polluted and hydrocarbon impacted environments (Farag et al., 2014; Bai et al., 2017), which might be the reason for their higher abundance in the Bhomra wetland.
Microbial communities in sediment differ depending on the types of wetlands and the anthropogenic loads from its catchment, indicating their potential use in monitoring wetland ecosystems (Urakawa and Bernhard, 2017). The present study has identified 11 phyla as putative indicators of different wetland: Acetothermia prefer EKW; four phyla (i.e., Actinobacteria, Deinococcus–Thermus, Tenericutes, and Woesearchaeota) prefer Malencho wetland; and six phyla (i.e., Aminicenantes, Chlorobi, Crenarchaeota, Euryarchaeota, Spirochaetes, and Thaumarchaeota) prefer Bhomra as their abode. Actinobacteria, Aminicenantes, Crenarchaeota, and Euryarchaeota are also dominant communities in their respective wetlands. Other phyla represent minor microbial communities—rare taxa may serve as important microbial indicators (Sogin et al., 2006; Rodriguez-Sanchez et al., 2016; Urakawa et al., 2017) for the ecosystem.
More knowledge of microbial populations through genus level ecotype identification is needed to use them as better indicators in wetland management (Martens-Habbena et al., 2009; Hernández et al., 2015; Urakawa et al., 2017). In the present study, we identified 54 diagnostic genera having differential abode preference for Bhomra, EKW, and Malencho wetlands. Close relatives for many of the ASVs of the diagnostic genera were distinct and significantly different in each wetland ecosystem, yet some ASVs remained unidentified. This indicates that these ASVs are likely to be a potential novel species (Zhao et al., 2018). Furthermore, identification of the abode preference of microorganisms based on the 16S rRNA gene (physiological capacities of bacteria) can provide significant insights into the potential roles that they play in ecological functioning of the wetlands (Zhao et al., 2018).
Various aerobic and anaerobic microorganisms derive their energy from the terminal process of organic matter mineralization by respiration using different electron acceptors available in the wetland sediments. However, the availability of different electron acceptors may depend on the metabolism of decomposers and fermenters that produce simpler organic compounds (Bongoua-Devisme et al., 2013). In the present study, we found primary decomposers and fermenters, such as a putative carbohydrate-fermenting bacterium Hydrogenispora in Bhomra and Dorea in Malencho and the putative cellulose-decomposing microorganism of genus Sorangium in Bhomra and genus Cellulosilyticum in Malencho. The secondary fermenters observed are a putative lactate-oxidizing bacterium Serpens in Bhomra; Lactococcus and an anaerobic propionate-degrading syntroph Smithella in EKW; and a hydrogen-oxidizing bacterium Hydrogenophaga in Malencho. These secondary metabolites are used by sulfate reducer and methanogens (Plugge et al., 2011). Further, the availability of these electron acceptors and their sequential depletion with an increase of depth in the sediment vertically provide thermodynamic constraints on respiration (Froelich et al., 1979). Electron acceptors, which provide higher energy yields, such as oxygen, nitrate, Mn(IV), and Fe(III), prevail near the sediment surface, followed by sulfate reducers and methanogens (Jørgensen et al., 2019b).
When oxygen is depleted at a depth of usually 3–10 cm, nitrate, Mn oxides, and Fe oxides are the next most important oxidants for organic matter. The present study has also encountered a putative Mn-oxidizing bacterium Caldimonas manganoxidans, an Fe(II)-oxidizing microorganism Sideroxydans, Fe-reducing microorganisms Aciditerrimonas and Anaerosolibacter, and nitrate-reducing bacteria Shivajiella indica and Vulcanibacillus modesticaldus in brackish-water bheri. In addition to oxidizing organic matter, these microbes are also oxidants for a significant fraction of the sulfide (Jørgensen, 1982; Canfield et al., 1992; Jørgensen and Nelson, 2004; Pellerin et al., 2015; Jørgensen et al., 2019a) produced by sulfate reducer.
In the marine sediments, dissimilatory sulfate reduction to sulfide by anaerobic microorganisms (Jørgensen and Kasten, 2006) may account for half of the organic carbon mineralization (Jørgensen, 1982; Jørgensen et al., 2019b). In the present study, the presence of diverse sulfate reducers (Desulfocapsa, Desulfopila, Desulfosarcina, Desulfosporosinus, Desulfobulbus, and Desulfuromonas) in the brackish-water bheri, Malencho, might indicate their association with sulfate reduction. Although documented primarily in salt or brackish marshes, recently, their occurrence is evident even in freshwater wetlands and peatlands, where a relatively small population of sulfate-reducing bacteria (SRB) represent an important fate for C (Pester et al., 2012; Hausmann et al., 2016; Kearns et al., 2016). In the present analysis, we noticed abode preference for green sulfur bacteria (Chlorobaculum limnaeum and Chlorobium phaeobacteroides) and purple photosynthetic bacteria (Blastochloris sulfoviridis) in Bhomra. These obligate photolithoautotrophs perform anaerobic photosynthesis with oxidation of inorganic sulfur compounds such as sulfide, polysulfide, or thiosulfate (Gregersen et al., 2011; Madigan et al., 2015). Besides, our study also has detected abode preference of sulfate reducers and an acetate-degrading bacterium (Desulfobacca) and SRB oxidizing aniline and 4-chlorophenol (Desulfomonile and Desulfatiglans) in EKW. In addition, sulfur-oxidizing bacteria such as Thioprofundum, Sulfurovum, and Thiobacillus are also encountered in Malencho. At present, little information is available about sulfur-oxidizing bacteria. However, it drives the oxidization of sulfur compounds produced by the activity of SRB and other sulfur compounds to sulfuric acid (Okabe et al., 2007; Tian et al., 2017).
Methanogens play an important role in the decay of organic matter in an anaerobic ecosystem and act as a terminal process of organic matter mineralization in sulfate-depleted zone (Jørgensen et al., 2019a). The present study has not found the abode preference of methanogenic archaea in Malencho. This might be due to rapid re-oxidation of the produced sulfide, more availability of sulfate, and less availability of organic C content in coastal sediment (Canfield et al., 1993; Egger et al., 2018; Jørgensen et al., 2019b). In freshwater also, methanogenesis is restricted to sulfate-depleted zone. The present study has recognized abode preference of different methanogens of the genera Methanosarcina, Methanoregula, Methanobacterium, Methanocella, and Methanomassiliicoccus and methanotrophs Methylocaldum, Methanothrix, and Methylobacter in Bhomra wetland, which indicates that methanogen bacteria preferred freshwater wetlands. It might be due to the depletion of sulfate with depth, presence of high organic carbon, and low sulfate content in freshwater sediment (Pester et al., 2012). The methanotrophs Methylocaldum, Methanothrix, and Methylobacter have also been observed in Bhomra. We also recorded abode preference of methanogen Methanomethylovorans and methanotrophs Methylosarcina and Methylocystis in EKW. This indicates that salinity could expand the abundance and diversity of SRB and decrease the diversity of methanogenic archaea and subsequently the methane emission from soil (Kroeger et al., 2017; Urakawa and Bernhard, 2017). These microbial populations could be good microbial indicators to assess the impacts of salinity.
Further, in respect to the nitrogen cycle, we found abode preference of many microbes such as Cellvibrio diazotrophicus, a nitrogen-fixing bacterium; Azoarcus toluclasticus, a denitrifying bacterium associated with nitrogen transformation that degrade aromatic compounds; and nitrate-reducing microbes S. indica and V. modesticaldus in Malencho. In Bhomra, we found abode preference for nitrous oxide-producing microbes Luteimonas marina and Pseudoxanthomonas mexicana and a nitrogen-fixing bacterium Bradyrhizobium elkanii, which are associated with root nodule. Besides these bacteria, we also found rhizosphere-associated bacteria: Non-omuraea in Bhomra, Sideroxydans and Tetrasphaera in Malencho, and an endophytic bacterium Phycicoccus endophyticus in Malencho.
Additionally, many of the spore-forming microorganisms, such as Actinocorallia aurantiaca, Rummeliibacillus pycnus, Saccharomonospora xinjiangensis, Sporomusa, Micromonospora, Sporacetigenium, Paenibacillus, and Anaerobacter, showed abode preference for Bhomra; a few microorganisms, e.g., Proteocatella sphenisci, showed abode preference for EKW; and Desulfosporosinus showed abode preference for Malencho. This result signifies spore formation as an alternate strategy for the survival of these microorganisms in the wetland environment. We also found microorganisms that are thermotolerant or halophilic or found from hydrothermal areas such as Rubinisphaera, Sporosalibacterium, Thioprofundum, Vulcanibacillus, Roseiflexus, and Thermoleophilum; radiation-resistant genus Truepera; and air-loving microorganism Litorilinea aerophila in Malencho. This abode preference in Malencho might be due to the less depth of ecosystem as well as periodical drying of the ecosystem.
Distinct microorganism in each wetland ecosystem has been found with their closest relative in the ecosystem having a similar environmental condition. We found microorganisms such as Defluviicoccus, Lactococcus chungangensis, Rhodoligotrophos, and Trichococcus in EKW, whose closest relatives were inhabitants of sewage/sludge; and Anaerovorax, a putrescine-fermenting, strictly anaerobic bacterium associated with the a foul odor in Malencho. Similarly, we found haloalkaliphilic/halophilic microorganism (Nitriliruptor and Haloplasma), a bacterium liking alkaline environments (Alkaliphilus), and microorganisms that inhabit sea sediment/seawater/saline water/brackish-water/estuary/mangrove (Ilumatobacter, Anderseniella baltica, Aquiflexum balticum, Filomicrobium, Haliea salexigens, Mariniradius saccharolyticus, Marinobacter koreensis, Halobacillus, Microbulbifer aggregans, Oceanirhabdus sediminicola, and Haliangium) in Malencho. In Bhomra, we found freshwater inhabitants (Aquihabitans). In Bhomra, we also found abode preference of microorganisms inhabiting forest soil (Parasegetibacter), which might be due to the presence of organic matter derived from diverse aquatic vegetation. The abode preference of these microorganism signifies salinity along with sewage, playing a vital role in shaping microbial communities. Previous studies have also found salinity and sewage (Hu et al., 2014; Chambers et al., 2016; Wei et al., 2019) as important factors for distinct microbial community structure.
Microorganisms Eubacterium sulci, Stomatobaculum, Coprococcus, Roseburia, Paraprevotella, and Dorea in Malencho; Cetobacterium in EKW; and Aerococcus in Bhomra associated with human urine, pig urine, vagina, feces, and intestine were observed. Microorganisms such as Succinivibrio and Blautia from Malencho, Proteocatella from EKW, and Erythrobacter vulgaris and Symbiobacterium from Malencho, associated with other animal source and marine invertebrates, were also found. The putative genus of the infectious microorganism of animal, Brevinema, and disease-causing microorganism Clostridium_III and Klebsiella pneumoniae were observed in EKW, Bhomra, and Malencho, respectively. Further, Thermomonas, a blood-dissolving microorganism, in EKW and Turicibacter in Malencho wetland were observed. As the wetlands are closely located in human habitation, the release of pathogens associated with different secretions and excretions of human, animal, and fish is inevitable. Further, it indicates wetlands are conducive enough to act as habitats for these microorganisms (Wu et al., 2016); however, concern on their public health aspects can only be ascertained by their seasonal abundance pattern in the wetlands and their transfer to human and animals through fish and other edible components and drinking water.
Conclusion
This study presents the first detailed and simultaneous characterization of the bacterial and archaeal communities and the identification of their abode in the freshwater wetland (Bhomra), brackish-water bheri (Malencho), and sewage-fed wetland (EKW) sediment, using three universal primers. It revealed that α-diversity indices and phylogenetic differential abundance pattern (weighted UniFrac) did not differ significantly among primers; however, those were significantly different across wetlands. Further, ASV similarity via Venn diagram analysis showed that primer pairs 341b4_F/806_R and N341b4_F/806_R shared 20.8–28% ASVs within the wetland, whereas primer pair Pro341F/Pro805R was completely distinct. More numbers of ASVs have been identified by the newly developed primer combination (N341b4_F/806_R), opening further useful scope for the study of diverse ecosystems. Overall, a distinct diversity pattern differentiates freshwater wetland, brackish-water bheri, and sewage-fed wetland ecosystems. Comparison of prokaryote communities in three different wetland sediments revealed the highest prokaryote richness and diversity in freshwater wetland, followed by brackish-water bheri and sewage-fed wetland. The identification of closest relative (at species level) of the diagnostic genus revealed the abode preference of prokaryotes such as sulfate reducers in Malencho, methanogens in Bhomra, and sewage-loving microbes in EKW. The phylogenetic differential abundance pattern along with distinct abode preference of these microorganisms signifies salinity along with sewage and plays a vital role in shaping microbial communities. Such information is essential to better understand the microbial ecology of wetlands.
Data Availability Statement
Raw read data are available at the National Center for Biotechnology Information Sequence Read Archive (Bio project Number PRJNA492841). Prokaryote 16S rRNA sequences are available on GenBank (accession number SAMN10107905 - SAMN10107913).
Author Contributions
KK did the conceptualization, methodology, investigation, sample collection, data curation, laboratory analysis, bioinformatics, writing—original draft, and writing—review and editing. MN did the bioinformatics and statistical analysis, writing—original draft, and writing—review and editing. MA wrote—review and edited. SD did the sample collection, manuscript review, and editing. BG did the preparation of location map and analysis of water and sediment quality parameters. US did the supervision and overall guidance. SN did the analysis of water and sediment quality parameters. CJ did the statistical computation. BD did the guidance for manuscript preparation. All authors contributed to the article and approved the submitted version.
Conflict of Interest
The authors declare that the research was conducted in the absence of any commercial or financial relationships that could be construed as a potential conflict of interest.
Acknowledgments
The authors are thankful to the Indian Council of Agricultural Research, New Delhi, for financial help under the project National Innovations in Climate Resilient Agriculture (NICRA). The assistance rendered by research scholars of the project and Bablu Naskar and Yusuf Ali (Technical staffs of ICAR-CIFRI) during collection of samples is also duly acknowledged. The authors also deeply acknowledge reviewers for their constructive advice that has substantially improved the manuscript’s quality.
Supplementary Material
The Supplementary Material for this article can be found online at: https://www.frontiersin.org/articles/10.3389/fmicb.2021.643945/full#supplementary-material
Additional information on primers detail, raw and processed reads data statistics, primer specific taxa abundance, phylum and genus level analysis has been given in Supplementary information section.
Abbreviations
ANOSIM, analysis of Similarities; ANOVA, analysis of Variance; ASVs, amplicon sequence variants; bp, base pair; DNA, deoxyribonucleic acid; EKW, East Kolkata Wetland; MANOVA, multivariate analysis of variance; PCR, polymerase chain reaction; SRB, sulfate-reducing bacteria; TIC, total inorganic carbon; TOC, total organic carbon.
References
Adrados, B., Sánchez, O., Arias, C. A., Becares, E., Garrido, L., Mas, J., et al. (2014). Microbial communities from different types of natural wastewater treatment systems: vertical and horizontal flow constructed wetlands and biofilters. Water Res. 55, 304–312. doi: 10.1016/j.watres.2014.02.011
Andrade, L. L., Leite, D. C., Ferreira, E. M., Ferreira, L. Q., Paula, G. R., Maguire, M. J., et al. (2012). Microbial diversity and anaerobic hydrocarbon degradation potential in an oil-contaminated mangrove sediment. BMC Microbiol. 12:186. doi: 10.1186/1471-2180-12-186
Ansola, G., Arroyo, P., and de Miera, L. E. S. (2014). Characterisation of the soil bacterial community structure and composition of natural and constructed wetlands. Sci. Total Environ. 473-474, 63–71. doi: 10.1016/j.scitotenv.2013.11.125
APHA (2017). Standard Methods for the Examination of Water and Wastewater, 23rd Edn, eds R. B. Baird, A. D. Eaton, E. W. Rice, and L. S. Clesceri (Washington D.C: American Public Health Association).
APHA (2005). Standard Methods for the Examination of Water and Wastewater, 22nd Edn. (Washington DC: American Public Health Association).
Astudillo-García, C., Hermans, S. M., Stevenson, B., Buckley, H. L., and Lear, G. (2019). Microbial assemblages and bioindicators as proxies for ecosystem health status: potential and limitations. Appl. Microbiol. Biotechnol. 103, 6407–6421. doi: 10.1007/s00253-019-09963-0
Auguet, J. C., Barberan, A., and Casamayor, E. O. (2010). Global ecological patterns in uncultured Archaea. ISME J. 4, 182–190. doi: 10.1038/ismej.2009.109
Bai, Y., Wang, J., Zhan, Z., Guan, L., Jin, L., and Zheng, G. (2017). Investigation of bacterial communities in peat land of the Gahai Lake natural conservation area. AIP Conf. Proc. 1890:040024.
Barberán, A., and Casamayor, E. O. (2010). Global phylogenetic community structure and β-diversity patterns in surface bacterioplankton metacommunities. Aquat. Microb. Ecol. 59, 1–10. doi: 10.3354/ame01389
Bell, T., Newman, J. A., Silverman, B. W., Turner, S. L., and Lilley, A. K. (2005). The contribution of species richness and composition to bacterial services. Nature 436, 1157–1160. doi: 10.1038/nature03891
Bongoua-Devisme, A. J., Cebron, A., Kassin, K. E., Yoro, G. R., Mustin, C., and Berthelin, J. (2013). Microbial communities involved in Fe reduction and mobility during soil organic matter (SOM) mineralization in two contrasted paddy soils. Geomicrobiol. J. 30, 347–361. doi: 10.1080/01490451.2012.688928
Callahan, B. J., McMurdie, P. J., Rosen, M. J., Han, A. W., Johnson, A. J. A., and Holmes, S. P. (2016). DADA2: high-resolution sample inference from Illumina amplicon data. Nat. Methods 13, 581–583. doi: 10.1038/nmeth.3869
Canfield, D. E., Jørgensen, B. B., Fossing, H., Glud, R., Gundersen, J., Ramsing, N. B., et al. (1993). Pathways of organic carbon oxidation in three continental margin sediments. Mar. Geol. 113, 27–40. doi: 10.1016/0025-3227(93)90147-n
Canfield, D. E., Raiswell, R., and Bottrell, S. H. (1992). The reactivity of sedimentary iron minerals toward sulfide. Am. J. Sci. 292, 659–683. doi: 10.2475/ajs.292.9.659
Cao, Q., Wang, H., Chen, X., Wang, R., and Liu, J. (2017). Composition and distribution of microbial communities in natural river wetlands and corresponding constructed wetlands. Ecol. Eng. 98, 40–48. doi: 10.1016/j.ecoleng.2016.10.063
Chambers, L. G., Guevara, R., Boyer, J. N., Troxler, T. G., and Davis, S. E. (2016). Effects of salinity and inundation on microbial community structure and function in a mangrove peat soil. Wetlands 36, 361–371. doi: 10.1007/s13157-016-0745-8
Chandra, P., and Kumar, M. (2020). “Contribution of microbes in the renovation of wetlands,” in Restoration of Wetland Ecosystem: A Trajectory Towards a Sustainable Environment, eds A. K. Upadhyay et al. (Singapore: Springer), 101–124. doi: 10.1007/978-981-13-7665-8_8
Chapman, E. J., Cadillo-Quiroz, H., Childers, D. L., Turetsky, M. R., and Waldrop, M. P. (2017). Soil microbial community composition is correlated to soil carbon processing along a boreal wetland formation gradient. Eur. J. Soil Biol. 82, 17–26. doi: 10.1016/j.ejsobi.2017.08.001
Chater, K. F. (2016). Recent advances in understanding Streptomyces. F1000Res. 5:2795. doi: 10.12688/f1000research.9534.1
Cheng, W., Zhang, J., Wang, Z., Wang, M., and Xie, S. (2014). Bacterial communities in sediments of a drinking water reservoir. Ann. Microbiol. 64, 875–878. doi: 10.1007/s13213-013-0712-z
Cheung, M. K., Wong, C. K., Chu, K. H., and Kwan, H. S. (2018). Community structure, dynamics and interactions of bacteria, Archaea and fungi in subtropical coastal wetland sediments. Sci. Rep. 8:14397.
Das Sarkar, S., Naskar, M., Gogoi, P., Raman, R. K., Manna, R. K., Samanta, S., et al. (2019). Impact assessment of barge trafficking on phytoplankton abundance and Chl a concentration, in River Ganga, India. PLoS One 14:e0221451. doi: 10.1371/journal.pone.0221451
Das Sarkar, S., Sarkar, U. K., Lianthuamluaia, L., Ghosh, B. D., Roy, K., Mishal, P., et al. (2020). Pattern of the state of eutrophication in the floodplain wetlands of eastern India in context of climate change: a comparative evaluation of 27 wetlands. Environ. Monit. Assess. 192:183.
De Cáceres, M., and Legendre, P. (2009). Associations between species and groups of sites: indices and statistical inference. Ecology 90, 3566–3574. doi: 10.1890/08-1823.1
De Mandal, S., Laskar, F., Panda, A. K., and Mishra, R. (2020). “Microbial diversity and functional potential in wetland ecosystems,” in Recent Advancements in Microbial Diversity, eds S. De Mandal and P. Bhatt (Cambridge, MA: Academic Press), 289–314. doi: 10.1016/b978-0-12-821265-3.00012-8
Decamp, O., and Warren, A. (2001). Abundance, biomass and viability of bacteria in wastewaters: impact of treatment in horizontal subsurface flow constructed wetlands. Water Res. 35, 3496–3501. doi: 10.1016/s0043-1354(01)00064-1
Delgado-Baquerizo, M., Maestre, F. T., Reich, P. B., Jeffries, T. C., Gaitan, J. J., Encinar, D., et al. (2016). Microbial diversity drives multifunctionality in terrestrial ecosystems. Nat. Commun. 7:10541.
Dorador, C., Meneses, D., Urtuvia, V., Demergasso, C., Vila, I., Witzel, K. P., et al. (2009). Diversity of Bacteroidetes in high-altitude saline evaporitic basins in northern Chile. J. Geophys. Res. Biogeosci. 114:G00D05.
Dorador, C., Vila, I., Witzel, K. P., and Imhoff, J. F. (2013). Bacterial and archaeal diversity in high altitude wetlands of the Chilean Altiplano. Fundam. Appl. Limnol. 182, 135–159. doi: 10.1127/1863-9135/2013/0393
Dufrêne, M., and Legendre, P. (1997). Species assemblages and indicator species: the need for a flexible asymetrical approach. Ecol. Monogr. 67, 345–366. doi: 10.2307/2963459
Egger, M., Riedinger, N., Mogollón, J. M., and Jørgensen, B. B. (2018). Global diffusive fluxes of methane in marine sediments. Nat. Geosci. 11, 421–425. doi: 10.1038/s41561-018-0122-8
Fang, L., Chen, L., Liu, Y., Tao, W., Zhang, Z., Liu, H., et al. (2015). Planktonic and sedimentary bacterial diversity of Lake Sayram in summer. Microbiol. Open 4, 814–825. doi: 10.1002/mbo3.281
Farag, I. F., Davis, J. P., Youssef, N. H., and Elshahed, M. S. (2014). Global patterns of abundance, diversity and community structure of the Aminicenantes (candidate phylum OP8). PLoS One 9:e92139. doi: 10.1371/journal.pone.0092139
Fischer, M. A., Güllert, S., Neulinger, S. C., Streit, W. R., and Schmitz, R. A. (2016). Evaluation of 16S rRNA gene primer pairs for monitoring microbial community structures showed high reproducibility within and low comparability between datasets generated with multiple archaeal and bacterial primer pairs. Front. Microbiol. 7:1297. doi: 10.3389/fmicb.2016.01297
Fouhy, F., Clooney, A. G., Stanton, C., Claesson, M. J., and Cotter, P. D. (2016). 16S rRNA gene sequencing of mock microbial populations-impact of DNA extraction method, primer choice and sequencing platform. BMC Microbiol. 16:123. doi: 10.1186/s12866-016-0738-z
Froelich, P., Klinkhammer, G. P., Bender, M. L., Luedtke, N. A., Heath, G. R., Cullen, D., et al. (1979). Early oxidation of organic matter in pelagic sediments of the eastern equatorial Atlantic: suboxic diagenesis. Geochim. Cosmochim. Acta 43, 1075–1090. doi: 10.1016/0016-7037(79)90095-4
Ghosh, D. (1998). “Wastewater-fed aquaculture in the Wetlands of Calcutta—an overview,” in Proceedings of the International Seminar on Wastewater Reclamation and Reuse for Aquaculture, Kolkata.
Ghoshal, T. K., De, D., Biswas, G., Kumar, P., and Vijayan, K. K. (2019). “Brackishwater aquaculture: opportunities and challenges for meeting livelihood demand in Indian sundarbans,” in The Sundarbans: A Disaster-Prone Eco-Region, ed. H. S. Sen (Cham: Springer), 321–349. doi: 10.1007/978-3-030-00680-8_11
Gregersen, L. H., Bryant, D. A., and Frigaard, N. U. (2011). Mechanisms and evolution of oxidative sulfur metabolism in green sulfur bacteria. Front. Microbiol 2:116. doi: 10.3389/fmicb.2011.00116
Gu, Y., Bai, Y., Xiang, Q., Yu, X., Zhao, K., Zhang, X., et al. (2018). Degradation shaped bacterial and archaeal communities with predictable taxa and their association patterns in Zoige wetland at Tibet plateau. Sci. Rep. 8:3884.
Gutknecht, J. L., Goodman, R. M., and Balser, T. C. (2006). Linking soil process and microbial ecology in freshwater wetland ecosystems. Plant Soil 289, 17–34. doi: 10.1007/s11104-006-9105-4
Hartman, W. H., Richardson, C. J., Vilgalys, R., and Bruland, G. L. (2008). Environmental and anthropogenic controls over bacterial communities in wetland soils. Proc. Natl. Acad. Sci. U.S.A. 105, 17842–17847. doi: 10.1073/pnas.0808254105
Hausmann, B., Knorr, K. H., Schreck, K., Tringe, S. G., del Rio, T. G., Loy, A., et al. (2016). Consortia of low-abundance bacteria drive sulfate reduction-dependent degradation of fermentation products in peat soil microcosms. ISME J. 10, 2365–2375. doi: 10.1038/ismej.2016.42
He, S., Stevens, S. L., Chan, L. K., Bertilsson, S., del Rio, T. G., Tringe, S. G., et al. (2017). Ecophysiology of freshwater Verrucomicrobia inferred from metagenome-assembled genomes. mSphere 2:e00277-17.
Hernández, M., Dumont, M. G., Yuan, Q., and Conrad, R. (2015). Different bacterial populations associated with the roots and rhizosphere of rice incorporate plant-derived carbon. Appl. Environ. Microbiol. 81, 2244–2253. doi: 10.1128/aem.03209-14
Horton, D. J., Theis, K. R., Uzarski, D. G., and Learman, D. R. (2019). Microbial community structure and microbial networks correspond to nutrient gradients within coastal wetlands of the Laurentian Great Lakes. FEMS Microbiol. Ecol. 95:fiz033.
Hu, Y., Wang, L., Tang, Y., Li, Y., Chen, J., Xi, X., et al. (2014). Variability in soil microbial community and activity between coastal and riparian wetlands in the Yangtze River estuary–Potential impacts on carbon sequestration. Soil Biol. Biochem. 70, 221–228. doi: 10.1016/j.soilbio.2013.12.025
Huang, S. S., Benskin, J. P., Chandramouli, B., Butler, H., Helbing, C. C., and Cosgrove, J. R. (2016). Xenobiotics produce distinct metabolomic responses in zebrafish larvae (Danio rerio). Environ. Sci. Technol. 50, 6526–6535. doi: 10.1021/acs.est.6b01128
Huse, S. M., Welch, D. M., Morrison, H. G., and Sogin, M. L. (2010). Ironing out the wrinkles in the rare biosphere through improved OTU clustering. Environ. Microbiol. 12, 1889–1898. doi: 10.1111/j.1462-2920.2010.02193.x
Iliev, I., Marhova, M., Kostadinova, S., Gochev, V., Tsankova, M., Ivanova, A., et al. (2019). Metagenomic analysis of the microbial community structure in protected wetlands in the Maritza River Basin. Biotechnol. Biotechnol. Equip. 33, 1721–1732. doi: 10.1080/13102818.2019.1697364
Jansson, J. K., and Hofmockel, K. S. (2019). Soil microbiomes and climate change. Nat. Rev. Microbiol. 18, 35–46. doi: 10.1038/s41579-019-0265-7
Jeffrey, S. T., and Humphrey, G. F. (1975). New spectrophotometric equations for determining chlorophylls a, b, c1 and c2 in higher plants, algae and natural phytoplankton. Biochem. Physiol. Pflanzen 167, 191–194. doi: 10.1016/s0015-3796(17)30778-3
Jiang, Y., Xu, H., Hu, X., Warren, A., and Song, W. (2013). Functional groups of marine ciliated protozoa and their relationships to water quality. Environ. Sci. Pollut. Res. 20, 5272–5280. doi: 10.1007/s11356-013-1525-0
Jørgensen, B. B. (1982). Mineralization of organic matter in the sea bed—the role of sulphate reduction. Nature 296, 643–645. doi: 10.1038/296643a0
Jørgensen, B. B., Beulig, F., Egger, M., Petro, C., Scholze, C., and Røy, H. (2019a). Organoclastic sulfate reduction in the sulfate-methane transition of marine sediments. Geochim. Cosmochim. Acta 254, 231–245. doi: 10.1016/j.gca.2019.03.016
Jørgensen, B. B., Findlay, A. J., and Pellerin, A. (2019b). The biogeochemical sulfur cycle of marine sediments. Front. Microbiol. 10:849. doi: 10.3389/fmicb.2019.00849
Jørgensen, B. B., and Kasten, S. (2006). “Marine geochemistry,” in Sulfur Cycling and Methane Oxidation, eds H. D. Schulz and M. Zabel (Berlin: Springer), 271–309. doi: 10.1002/9781118349083.ch13
Jørgensen, B. B., and Nelson, D. C. (2004). “Sulfide oxidation in marine sediments: geochemistry meets microbiology,” in Sulfur Biogeochemistry – Past and Present, eds J. P. Amend, K. J. Edwards and T. W. Lyons (Boulder, CO: Geological Society of America), 36–81.
Kawaichi, S., Ito, N., Kamikawa, R., Sugawara, T., Yoshida, T., and Sako, Y. (2013). Ardenticatena maritima gen. nov., sp. nov., a ferric iron-and nitrate-reducing bacterium of the phylum ‘Chloroflexi’ isolated from an iron-rich coastal hydrothermal field, and description of Ardenticatenia classis nov. Int. J. Syst. Evol. Microbiol. 63, 2992–3002. doi: 10.1099/ijs.0.046532-0
Kearns, P. J., Weston, N. B., Bowen, J. L., Živković, T., and Vile, M. A. (2016). Tidal freshwater marshes harbor phylogenetically unique clades of sulfate reducers that are resistant to climate-change-induced salinity intrusion. Estuaries Coast. 39, 981–991. doi: 10.1007/s12237-016-0067-3
Kroeger, K. D., Crooks, S., Moseman-Valtierra, S., and Tang, J. (2017). Restoring tides to reduce methane emissions in impounded wetlands: a new and potent Blue Carbon climate change intervention. Sci. Rep. 7:11914.
Kunin, V., Engelbrektson, A., Ochman, H., and Hugenholtz, P. (2010). Wrinkles in the rare biosphere: pyrosequencing errors can lead to artificial inflation of diversity estimates. Environ. Microbiol. 12, 118–123. doi: 10.1111/j.1462-2920.2009.02051.x
Lacombe-Harvey, M. È., Brzezinski, R., and Beaulieu, C. (2018). Chitinolytic functions in actinobacteria: ecology, enzymes, and evolution. Appl. Microbiol. Biotechnol. 102, 7219–7230. doi: 10.1007/s00253-018-9149-4
Li, B., Chen, H., Li, N., Wu, Z., Wen, Z., Xie, S., et al. (2017). Spatio-temporal shifts in the archaeal community of a constructed wetland treating river water. Sci. Total Environ. 605, 269–275. doi: 10.1016/j.scitotenv.2017.06.221
Li, H., Chi, Z., Li, J., Wu, H., and Yan, B. (2019). Bacterial community structure and function in soils from tidal freshwater wetlands in a Chinese delta: potential impacts of salinity and nutrient. Sci. Total Environ. 696:134029. doi: 10.1016/j.scitotenv.2019.134029
Ligi, T., Oopkaup, K., Truu, M., Preem, J. K., Nõlvak, H., Mitsch, W. J., et al. (2014). Characterization of bacterial communities in soil and sediment of a created riverine wetland complex using high-throughput 16S rRNA amplicon sequencing. Ecol. Eng. 72, 56–66. doi: 10.1016/j.ecoleng.2013.09.007
Liu, Y., Zhang, J., Zhao, L., Zhang, X., and Xie, S. (2014). Spatial distribution of bacterial communities in high-altitude freshwater wetland sediment. Limnology 15, 249–256. doi: 10.1007/s10201-014-0429-0
Lozupone, C. A., Hamady, M., Kelley, S. T., and Knight, R. (2007). Quantitative and qualitative β diversity measures lead to different insights into factors that structure microbial communities. Appl. Environ. Microbiol. 73, 1576–1585. doi: 10.1128/aem.01996-06
Lu, Y. Z., Ding, Z. W., Ding, J., Fu, L., and Zeng, R. J. (2015). Design and evaluation of universal 16S rRNA gene primers for high-throughput sequencing to simultaneously detect DAMO microbes and anammox bacteria. Water Res. 87, 385–394. doi: 10.1016/j.watres.2015.09.042
Lv, Y., Wang, C., Jia, Y., Wang, W., Ma, X., Du, J., et al. (2014). Effects of sulfuric, nitric, and mixed acid rain on litter decomposition, soil microbial biomass, and enzyme activities in subtropical forests of China. Appl. Soil Ecol. 79, 1–9. doi: 10.1016/j.apsoil.2013.12.002
Madigan, M. T., Martinko, J. M., Bender, K. S., Buckley, D. H., and Stahl, D. A. (2015). Brock Biology of Microorganisms, 14th Edn. Boston, MA: Pearson.
Marschner, P., Kandeler, E., and Marschner, B. (2003). Structure and function of the soil microbial community in a long-term fertilizer experiment. Soil Biol. Biochem. 35, 453–461. doi: 10.1016/s0038-0717(02)00297-3
Martens-Habbena, W., Berube, P. M., Urakawa, H., José, R., and Stahl, D. A. (2009). Ammonia oxidation kinetics determine niche separation of nitrifying Archaea and Bacteria. Nature 461, 976–979. doi: 10.1038/nature08465
Martin, M. (2011). Cutadapt removes adapter sequences from high-throughput sequencing reads. EMBnet J. 17, 10–12. doi: 10.14806/ej.17.1.200
McMurdie, P. J., and Holmes, S. (2013). Phyloseq: an R package for reproducible interactive analysis and graphics of microbiome census data. PLoS One 8:e61217. doi: 10.1371/journal.pone.0061217
Megonigal, J. P., and Neubauer, S. C. (2019). “Biogeochemistry of tidal freshwater wetlands,” in Coastal Wetlands, eds G. M. E. Perillo, E. Wolanski, D. R. Cahoon, M. Brinson (Amsterdam: Elsevier), 641–683. doi: 10.1016/b978-0-444-63893-9.00019-8
Merkley, M., Rader, R., McArthur, J., and Eggett, D. (2004). Bacteria as bioindicators in wetlands: bioassessment in the bonneville basin of Utah, USA. Wetlands 24, 600–607. doi: 10.1672/0277-5212(2004)024[0600:babiwb]2.0.co;2
Nag, S. K., Nandy, S. K., Roy, K., Sarkar, U. K., and Das, B. K. (2019). Carbon balance of a sewage-fed aquaculture wetland. Wetl. Ecol. Manage. 27, 311–322. doi: 10.1007/s11273-019-09661-8
Nelson, D. W., and Sommers, L. E. (1996). “Total carbon, organic carbon and organic matter: laboratory methods,” in Methods of Soil Analysis. Part 3. Chemical Methods, eds D. L. Sparks, A. L. Page, P. A. Helmke, and R. H. Loeppert (Madison, WI: American Society of Agronomy), 961–1010. doi: 10.2136/sssabookser5.3.c34
Offre, P., Spang, A., and Schleper, C. (2013). Archaea in biogeochemical cycles. Annu. Rev. Microbiol. 67, 437–457. doi: 10.1146/annurev-micro-092412-155614
Okabe, S., Odagiri, M., Ito, T., and Satoh, H. (2007). Succession of sulfur-oxidizing bacteria in the microbial community on corroding concrete in sewer systems. Appl. Environ. Microbiol. 73, 971–980. doi: 10.1128/aem.02054-06
Oksanen, J., Blanchet, F. G., Kindt, R., Legendre, P., Minchin, P. R., O’Hara, R. B., et al. (2015). Vegan: Community Ecology Package. Ordination Methods, Diversity Analysis and Other Functions for Community and Vegetation Ecologists. Available online at: http://cran.r-project.org/web/packages/vegan/index.html (accessed January, 2020).
Olsen, S. R., and Dean, L. A. (1965). “Phosphorous,” in Methods of Soil Analysis, Part 2, Chemical and Microbiological Properties, ed. C. A. Black (Madison, WI: American Society of Agronomy), 1036–1037.
Pellerin, A., Bui, T. H., Rough, M., Mucci, A., Canfield, D. E., and Wing, B. A. (2015). Mass-dependent sulfur isotope fractionation during reoxidative sulfur cycling: a case study from Mangrove Lake, Bermuda. Geochim. Cosmochim. Acta 149, 152–164. doi: 10.1016/j.gca.2014.11.007
Peralta, R. M., Ahn, C., and Gillevet, P. M. (2013). Characterization of soil bacterial community structure and physicochemical properties in created and natural wetlands. Sci. Total Environ. 443, 725–732. doi: 10.1016/j.scitotenv.2012.11.052
Pester, M., Knorr, K. H., Friedrich, M. W., Wagner, M., and Loy, A. (2012). Sulfate-reducing microorganisms in wetlands–fameless actors in carbon cycling and climate change. Front. Microbiol. 3:72. doi: 10.3389/fmicb.2012.00072
Philippot, L., Andersson, S. G., Battin, T. J., Prosser, J. I., Schimel, J. P., Whitman, W. B., et al. (2010). The ecological coherence of high bacterial taxonomic ranks. Nat. Rev. Microbiol. 8, 523–529. doi: 10.1038/nrmicro2367
Plugge, C. M., Zhang, W., Scholten, J., and Stams, A. J. (2011). Metabolic flexibility of sulfate-reducing bacteria. Front. Microbiol. 2:81. doi: 10.3389/fmicb.2011.00081
Quast, C., Pruesse, E., Yilmaz, P., Gerken, J., Schweer, T., Yarza, P., et al. (2013). The SILVA ribosomal RNA gene database project: improved data processing and web-based tools. Nucleic Acids Res. 41, D590–D596. doi: 10.1093/nar/gks1219
R Core Team (2019). R: A Language and Environment for Statistical Computing. Vienna: R Foundation for Statistical Computing.
Raina, V., Panda, A. N., Mishra, S. R., Nayak, T., and Suar, M. (2019). “Microbial biodiversity study of a brackish water ecosystem in eastern India: the Chilika Lake,” in Microbial Diversity in the Genomic Era, eds S. Das and H. Dash (Cambridge, MA: Academic Press), 47–63. doi: 10.1016/b978-0-12-814849-5.00004-6
Rathour, R., Gupta, J., Kumar, M., Hiloidhari, M., Mehrotra, A. K., and Thakur, I. S. (2017). Metagenomic sequencing of microbial communities from brackish water of Pangong Lake of the northwest Indian Himalayas. Genome Announc. 5:e01029-17.
Rhoades, J. D. (1996). “Salinity: electrical conductivity and total dissolved solids,” in Methods of Soil Analysis. Part 3. Chemical methods, eds D. L. Sparks, A. L. Page, P. A. Helmke, and R. H. Loeppert (Madison, WI: American Society of Agronomy), 417–435. doi: 10.2136/sssabookser5.3.c14
Rodriguez-Sanchez, A., Purswani, J., Lotti, T., Maza-Marquez, P., van Loosdrecht, M. C. M., Vahala, R., et al. (2016). Distribution and microbial community structure analysis of a single-stage partial nitritation/anammox granular sludge bioreactor operating at low temperature. Environ. Technol. 37, 2281–2291. doi: 10.1080/09593330.2016.1147613
Roy-Basu, A., Bharat, G. K., Chakraborty, P., and Sarkar, S. K. (2020). Adaptive co-management model for the East Kolkata wetlands: a sustainable solution to manage the rapid ecological transformation of a peri-urban landscape. Sci. Total Environ. 698:134203. doi: 10.1016/j.scitotenv.2019.134203
Sánchez, O. (2017). Constructed wetlands revisited: microbial diversity in the–omics era. Microb. Ecol. 73, 722–733. doi: 10.1007/s00248-016-0881-y
Santos, M. S., Nogueira, M. A., and Hungria, M. (2019). Microbial inoculants: reviewing the past, discussing the present and previewing an outstanding future for the use of beneficial bacteria in agriculture. AMB Express 9:205.
Sarkar, U. K., and Borah, B. C. (2018). Flood plain wetland fisheries of India: with special reference to impact of climate change. Wetl. Ecol. Manage. 26, 1–15. doi: 10.1007/s11273-017-9559-6
Sarkar, U. K., Mishal, P., Borah, S., Karnatak, G., Chandra, G., Kumari, S., et al. (2020). Status, potential, prospects, and issues of floodplain wetland fisheries in India: synthesis and review for sustainable management. Rev. Fish. Sci. Aquac. 29, 1–32. doi: 10.1080/23308249.2020.1779650
Schliep, K., Potts, A. J., Morrison, D. A., and Grimm, G. W. (2017). Intertwining phylogenetic trees and networks. Methods Ecol. Evol. 8, 1212—-1220. doi: 10.1111/2041-210X.12760
Schriefer, A. E., Cliften, P. F., Hibberd, M. C., Sawyer, C., Brown-Kennerly, V., Burcea, L., et al. (2018). A multi-amplicon 16S rRNA sequencing and analysis method for improved taxonomic profiling of bacterial communities. J. Microbiol. Methods 154, 6–13. doi: 10.1016/j.mimet.2018.09.019
Sogin, M. L., Morrison, H. G., Huber, J. A., Welch, D. M., Huse, S. M., Neal, P. R., et al. (2006). Microbial diversity in the deep sea and the underexplored “rare biosphere”. Proc. Natl. Acad. Sci. U.S.A. 103, 12115–12120. doi: 10.1073/pnas.0605127103
Speirs, L., Rice, D. T., Petrovski, S., and Seviour, R. J. (2019). The phylogeny, biodiversity, and ecology of the chloroflexi in activated sludge. Front. Microbiol. 10:2015. doi: 10.3389/fmicb.2019.02015
Steinmuller, H. E., and Chambers, L. G. (2019). Characterization of coastal wetland soil organic matter: implications for wetland submergence. Sci. Total Environ. 677, 648–659. doi: 10.1016/j.scitotenv.2019.04.405
Strickland, J. D. H., and Parsons, T. R. (1972). A Practical Handbook of Seawater Analysis. Bulletin 167. (Ottawa: Fisheries Research Board of Canada), 326.
Sun, Y., Li, X., Liu, J., Yao, Q., Jin, J., Liu, X., et al. (2019). Comparative analysis of bacterial community compositions between sediment and water in different types of wetlands of northeast China. J. Soils Sediments 19, 3083–3097. doi: 10.1007/s11368-019-02301-x
Takahashi, S., Tomita, J., Nishioka, K., Hisada, T., and Nishijima, M. (2014). Development of a prokaryotic universal primer for simultaneous analysis of Bacteria and Archaea using next-generation sequencing. PLoS One 9:e105592. doi: 10.1371/journal.pone.0105592
Thijs, S., Op De Beeck, M., Beckers, B., Truyens, S., Stevens, V., Van Hamme, J. D., et al. (2017). Comparative evaluation of four bacteria-specific primer pairs for 16S rRNA gene surveys. Front. Microbiol. 8:494. doi: 10.3389/fmicb.2017.00494
Thomas, G. W. (1996). “Soil pH and soil acidity,” in Methods Of soil Analysis, Part 3. Chemical Methods, eds D. L. Sparks, A. L. Page, P. A. Helmke, and R. H. Loeppert (Madison, WI: American Society of Agronomy), 475–490. doi: 10.2136/sssabookser5.3.c16
Tian, H., Gao, P., Chen, Z., Li, Y., Li, Y., Wang, Y., et al. (2017). Compositions and abundances of sulfate-reducing and sulfur-oxidizing microorganisms in water-flooded petroleum reservoirs with different temperatures in China. Front. Microbiol. 8:143. doi: 10.3389/fmicb.2017.00143
Tichý, L., and Chytrý, M. (2006). Statistical determination of diagnostic species for site groups of unequal size. J. Veg. Sci. 17, 809–818. doi: 10.1111/j.1654-1103.2006.tb02504.x
Torres, G. G., Figueroa-Galvis, I., Muñoz-García, A., Polanía, J., and Vanegas, J. (2019). Potential bacterial bioindicators of urban pollution in mangroves. Environ. Pollut. 255:113293. doi: 10.1016/j.envpol.2019.113293
Tremblay, J., Singh, K., Fern, A., Kirton, E. S., He, S., Woyke, T., et al. (2015). Primer and platform effects on 16S rRNA tag sequencing. Front. Microbiol. 6:771. doi: 10.3389/fmicb.2015.00771
Urakawa, H., and Bernhard, A. E. (2017). Wetland management using microbial indicators. Ecol. Eng. 108, 456–476. doi: 10.1016/j.ecoleng.2017.07.022
Urakawa, H., Dettmar, D. L., and Thomas, S. (2017). The uniqueness and biogeochemical cycling of plant root microbial communities in a floating treatment wetland. Ecol. Eng. 108, 573–580. doi: 10.1016/j.ecoleng.2017.06.066
Urbansky, E. T. (2001). Total organic carbon analyzers as tools for measuring carbonaceous matter in natural waters. J. Environ. Monit. 3, 102–112. doi: 10.1039/B006564L
Wang, J., Bai, J., Zhao, Q., Lu, Q., and Xia, Z. (2016). Five-year changes in soil organic carbon and total nitrogen in coastal wetlands affected by flow-sediment regulation in a Chinese delta. Sci. Rep. 6:21137.
Wang, Q., Garrity, G. M., Tiedje, J. M., and Cole, J. R. (2007). Naive Bayesian classifier for rapid assignment of rRNA sequences into the new bacterial taxonomy. Appl. Environ. Microbiol. 73, 5261–5267. doi: 10.1128/aem.00062-07
Wang, X., Zhang, Z., Yu, Z., Shen, G., Cheng, H., and Tao, S. (2020). Composition and diversity of soil microbial communities in the alpine wetland and alpine forest ecosystems on the Tibetan Plateau. Sci. Total Environ. 747:141358. doi: 10.1016/j.scitotenv.2020.141358
Wang, Y., Sheng, H. F., He, Y., Wu, J. Y., Jiang, Y. X., Tam, N. F. Y., et al. (2012). Comparison of the levels of bacterial diversity in freshwater, intertidal wetland, and marine sediments by using millions of illumina tags. Appl. Environ. Microbiol. 78, 8264–8271. doi: 10.1128/aem.01821-12
Wei, J., Gao, J., Wang, N., Liu, Y., Wang, Y., Bai, Z., et al. (2019). Differences in soil microbial response to anthropogenic disturbances in Sanjiang and Momoge Wetlands, China. FEMS Microbiol. Ecol. 95:fiz110.
Wiegand, S., Jogler, M., Boedeker, C., Pinto, D., Vollmers, J., Rivas-Marín, E., et al. (2020). Cultivation and functional characterization of 79 Planctomycetes uncovers their unique biology. Nat. Microbiol. 5, 126–140. doi: 10.1038/s41564-019-0588-1
Wilcox, T. M., McKelvey, K. S., Young, M. K., Jane, S. F., Lowe, W. H., Whiteley, A. R., et al. (2013). Robust detection of rare species using environmental DNA: the importance of primer specificity. PLoS One 8:e59520. doi: 10.1371/journal.pone.0059520
Wu, J. Y., Jiang, X. T., Jiang, Y. X., Lu, S. Y., Zou, F., and Zhou, H. W. (2010). Effects of polymerase, template dilution and cycle number on PCR based 16 S rRNA diversity analysis using the deep sequencing method. BMC Microbiol. 10:255. doi: 10.1186/1471-2180-10-255
Wu, S., Carvalho, P. N., Müller, J. A., Manoj, V. R., and Dong, R. (2016). Sanitation in constructed wetlands: a review on the removal of human pathogens and fecal indicators. Sci. Total Environ. 541, 8–22. doi: 10.1016/j.scitotenv.2015.09.047
Yamada, T., Sekiguchi, Y., Imachi, H., Kamagata, Y., Ohashi, A., and Harada, H. (2005). Diversity, localization, and physiological properties of filamentous microbes belonging to Chloroflexi subphylum I in mesophilic and thermophilic methanogenic sludge granules. Appl. Environ. Microbiol. 71, 7493–7503. doi: 10.1128/aem.71.11.7493-7503.2005
Zhang, X., Xin, X., Yang, W., Zhu, A., and Ding, S. (2019). Short-term decomposition, turnover and retention of residue-derived carbon are influenced by the fertility level in a sandy loam soil. Geoderma 349, 68–78. doi: 10.1016/j.geoderma.2019.04.043
Zhang, X., Xu, S., Li, C., Zhao, L., Feng, H., Yue, G., et al. (2014). The soil carbon/nitrogen ratio and moisture affect microbial community structures in alkaline permafrost-affected soils with different vegetation types on the Tibetan plateau. Res. Microbiol. 165, 128–139. doi: 10.1016/j.resmic.2014.01.002
Zhao, Q., Zhao, H., Gao, Y., Zheng, L., Wang, J., and Bai, J. (2020). Alterations of bacterial and archaeal communities by freshwater input in coastal wetlands of the Yellow River Delta, China. Appl. Soil Ecol. 153:103581. doi: 10.1016/j.apsoil.2020.103581
Zhao, S., Zhuang, L., Wang, C., Li, Y., Wang, S., and Zhu, G. (2018). High-throughput analysis of anammox bacteria in wetland and dryland soils along the altitudinal gradient in Qinghai–Tibet Plateau. Microbiologyopen 7:e00556. doi: 10.1002/mbo3.556
Zheng, H., Wang, X., Luo, X., Wang, Z., and Xing, B. (2018). Biochar-induced negative carbon mineralization priming effects in a coastal wetland soil: roles of soil aggregation and microbial modulation. Sci. Total Environ. 610, 951–960. doi: 10.1016/j.scitotenv.2017.08.166
Zhou, Z., Meng, H., Liu, Y., Gu, J. D., and Li, M. (2017). Stratified bacterial and archaeal community in mangrove and intertidal wetland mudflats revealed by high throughput 16S rRNA gene sequencing. Front. Microbiol. 8:2148. doi: 10.3389/fmicb.2017.02148
Zhou, Z., Wang, C., and Luo, Y. (2020). Meta-analysis of the impacts of global change factors on soil microbial diversity and functionality. Nat. Commun. 11:3072.
Keywords: indicator taxa, floodplain wetland, brackishwater wetland, high-throughput sequencing, sediment microbes, sewage-fed ecosystem
Citation: Kumari K, Naskar M, Aftabuddin M, Das Sarkar S, Ghosh BD, Sarkar UK, Nag SK, Jana C and Das BK (2021) Evaluation of Three Prokaryote Primers for Identification of Prokaryote Community Structure and Their Abode Preference in Three Distinct Wetland Ecosystems. Front. Microbiol. 12:643945. doi: 10.3389/fmicb.2021.643945
Received: 19 December 2020; Accepted: 10 June 2021;
Published: 14 July 2021.
Edited by:
Anne Bernhard, Connecticut College, United StatesReviewed by:
Craig Lee Moyer, Western Washington University, United StatesPrem Prashant Chaudhary, National Institutes of Health (NIH), United States
Iva Buriankova, Masaryk University, Czechia
Copyright © 2021 Kumari, Naskar, Aftabuddin, Das Sarkar, Ghosh, Sarkar, Nag, Jana and Das. This is an open-access article distributed under the terms of the Creative Commons Attribution License (CC BY). The use, distribution or reproduction in other forums is permitted, provided the original author(s) and the copyright owner(s) are credited and that the original publication in this journal is cited, in accordance with accepted academic practice. No use, distribution or reproduction is permitted which does not comply with these terms.
*Correspondence: Kavita Kumari, kavitacof@gmail.com
†These authors have contributed equally to this work and share first authorship
‡ORCID: Kavita Kumari, orcid.org/0000-0002-2898-7850; Malay Naskar, orcid.org/0000-0002-1865-8113; Md. Aftabuddin, orcid.org/0000-0003-3135-0698; Soma Das Sarkar, orcid.org/0000-0002-6115-9838; Subir Kumar Nag, orcid.org/0000-0003-3929-503X; Uttam Kumar Sarkar, orcid.org/0000-0001-8166-4375; Basanta Kumar Das, orcid.org/0000-0002-6629-8992