- 1Department of Biomedical Engineering, Faculty of Electrical Engineering and Communication, Brno University of Technology, Brno, Czechia
- 2Department of Biotechnology, University of Chemistry and Technology Prague, Prague, Czechia
Gene expression analysis through reverse transcription-quantitative real-time polymerase chain reaction (RT-qPCR) depends on correct data normalization by reference genes with stable expression. Although Clostridium beijerinckii NRRL B-598 is a promising Gram-positive bacterium for the industrial production of biobutanol, validated reference genes have not yet been reported. In this study, we selected 160 genes with stable expression based on an RNA sequencing (RNA-Seq) data analysis, and among them, seven genes (zmp, rpoB1, rsmB, greA, rpoB2, topB2, and rimO) were selected for experimental validation by RT-qPCR and gene ontology (GO) enrichment analysis. According to statistical analyses, zmp and greA were the most stable and suitable reference genes for RT-qPCR normalization. Furthermore, our methodology can be useful for selection of the reference genes in other strains of C. beijerinckii and it also suggests that the RNA-Seq data can be used for the initial selection of novel reference genes, however, their validation is required.
Introduction
Reverse transcriptase-quantitative real-time polymerase chain reaction (RT-qPCR) is the most commonly used technique to quantify gene expression due to its high sensitivity, specificity, and reproducibility (Ginzinger, 2002). Correct quantification of mRNA relies on data normalization that removes differences in the extraction yield, reverse-transcriptase activity and efficiency of PCR amplification (Vandesompele et al., 2002; Bustin et al., 2009). The most commonly used normalization method utilizes the so-called reference genes against which are gene expression data relatively quantified. Reference genes should maintain a constant mRNA expression regardless of the experimental conditions, different tissues, cells, or life cell phases (Derveaux et al., 2010). Thus, correct selection plays a crucial role in accurate data normalization. However, there is no universal reference gene and many studies have shown that the expression of commonly used reference genes is not always stable (Liang et al., 2014; Chapman and Waldenström, 2015; Jo et al., 2019), and reference genes should be selected individually for each organism and experimental condition (Liang et al., 2014). Furthermore, literature mining is not an appropriate approach for their selection and cannot be used in most cases. On the other hand, recent studies use RNA sequencing (RNA-Seq) technology for the evaluation of the whole transcriptomes to find novel candidates for reference genes (Carvalho et al., 2014; Hu et al., 2016; Pombo et al., 2017).
Thus far, suitable reference genes have been determined in different Clostridium species, such as Clostridium difficile (Metcalf et al., 2010), Clostridium botulinum (Kirk et al., 2014), and Clostridium ljungdahlii (Liu et al., 2013). In Clostridium beijerinckii NCIMB 8052, 177 putative housekeeping genes were previously identified based on transcriptomic data (Wang et al., 2011). However, thus far, no evaluation study of reference genes has been performed for this species. C. beijerinckii has been found to be a promising microorganism for industrial production of biobutanol, and efforts to increase butanol productivity by means of genetic and metabolic engineering of its strains have been recently reported (Agu et al., 2019; Xin et al., 2020). However, the strain engineering of C. beijerinckii is hindered by insufficient understanding of cellular physiology and regulatory mechanisms of gene expression. Despite the recent progress in CRISPR-associated methods of genetic engineering tailored to C. beijerinckii (Wang et al., 2016), the identification of valid endogenous reference genes for RT-qPCR is lacking.
In the present study, we selected seven putative reference genes (zmp, rpoB1, rsmB, greA, rpoB2, topB2, and rimO) for C. beijerinckii NRRL B-598 based on the RNA-Seq data that were obtained under different experimental conditions and at different time-points. The seven candidate reference genes were described and summarized by the gene ontology (GO) enrichment analysis and further tested for expression stability by RT-qPCR experiments and evaluated by four statistical algorithms: NormFinder (Andersen et al., 2004), RefFinder (Xie et al., 2012), Coefficient of variation (CV) analysis (Boda et al., 2009), and Pairwise ΔCt method (Silver et al., 2006). According to the stability rating, we identified a novel set of reference genes that can be used for the normalization of RT-qPCR data of C. beijerinckii NRRL B-598. As the strain C. beijerinckii NRRL B-598 shares high homology with other C. beijerinckii strains (Sedlar et al., 2017), the results will be useful for all strains of the species.
Materials and Methods
RNA-Seq Data Pre-processing
RNA sequencing data were obtained in our previous studies in which we first observed the transcription changes during standard ABE fermentation of C. beijerinckii (Sedlar et al., 2018; Patakova et al., 2019). The RNA-Seq data consisted of five replicates and six samples/time-points per replicate. Next, we evaluated the transcriptional response of C. beijerinckii to butanol shock (Sedlar et al., 2019) with two replicates and six samples/time-points each. Together, the available RNA-Seq transcriptomes consisted of 42 samples collected across 12 diverse time-points and conditions (see Table 1).
Pre-processing of the RNA-Seq data was performed in the same manner as in our previous studies. However, the analysis was recalculated to ensure the utilization of the same versions of particular tools for all samples. The data quality assessment was conducted by FastQC (v0.11.5) and summarized reports across samples were generated by MultiQC (v1.7) (Ewels et al., 2016). Trimmomatic software (v1.36) (Bolger et al., 2014) was used for quality and adapter trimming. The RNA-Seq reads corresponding to 16S and 23S rRNA genes sequences were filtered out by SortMeRNA (v2.1) (Kopylova et al., 2012) and SILVA database (Quast et al., 2013) (v132). Cleansed reads were mapped by STAR (v 2.7.3a) (Dobin et al., 2013) to a reference genome of C. beijerinckii available in the GenBank database under accession CP011966.3. Mapping results in SAM (Sequence Read Alignment/Map) file format were indexed and converted to BAM (Binary Read Alignment/Map) files by SAM-tools (v1.7) (Li et al., 2009).
Mapped reads were counted by the featureCounts function from R/Bioconductor Rsubread (v2.2.6) package (Liao et al., 2014, 2019), utilizing two counting strategies: one for uniquely mapped reads and the other for multi-mapping reads. Raw count tables were further processed in R (v4.0.2), using custom-made scripts, and then used for estimation of TPM (transcript per million) values for each gene and sample. From obtained TPM values, we determined the mean value and CV [standard deviation (SD) to the mean (μ)] of TPM values for each gene.
Furthermore, we performed differential expression analysis on raw count tables using R/Bioconductor DESeq2 (v1.28.1) package (Love et al., 2014) between all 12 time-points. A GO enrichment analysis was conducted by the R/Bioconductor topGO (v2.40.0) package (Alexa and Rahnenfurher, 2020) based on the C. beijerinckii GO annotation map created in our previous study (Sedlar et al., 2019).
Selection of Candidate Reference Genes
The selection of candidate reference genes was conducted by a series of filtering steps according to the results of processed RNA-Seq data: TPM values, CV of TPM values and results from differential expression analysis. Based on the results from differential expression analysis between all 66 time-points pairs, we counted the number of times each gene was not significantly regulated, p-adjust value > 0.1 (Benjamini–Hochberg correction) and filtered out all genes that did not pass the threshold of 50 insignificant changes. In the next step, we eliminated genes with a mean TPM value lower or equal to 35 TPM. Finally, we removed genes with the CV of TPM greater or equal to 30%. After each data filtering step, we compared results from both counting methods (unique and multi-mapping options), and only the genes reported by both methods were preserved for further processing.
Samples for RT-qPCR
The gene expression of the seven candidate reference genes zmp, rpoB1, rsmB, greA, rpoB2, topB2, and rimO were assessed in the following cultivation samples: T1, T6, Tb0, Tb2, t0, t1, and t1_CH (see Supplementary Material 1). The origin of the samples T1, T6, Tb0, and Tb2 is described in our previous transcriptomic studies (Sedlar et al., 2018, 2019) (see Table 1). Briefly, samples T1 and T6 were obtained during a bioreactor batch cultivation of C. beijerinckii NRRL B-598 on the TYA medium at time points of 3.5 and 23 h, respectively (Sedlar et al., 2018). Samples Tb0 and Tb2 were obtained during bioreactor cultivation of C. beijerinckii NRRL B-598 on a TYA medium with added butanol at time points 0 and 1 h after butanol addition, respectively (Sedlar et al., 2019). The samples t0, t1, and t1_CH were obtained as follows: 480 ml of the TYA medium was inoculated with a spore suspension of C. beijerinckii NRRL B-598 and cultured overnight at 37°C in 90% N2/10% H2 atmosphere in an anaerobic chamber (Concept 400; Ruskinn Technology). An overnight grown culture (12 h old) was split into two parallels per 240 ml, and the antibiotic chloramphenicol (30 μg ml–1) was added to one of the parallels. This point was set as time point zero (t0). After 1 h of incubation, a sample was withdrawn from both untreated (t1) and chloramphenicol-treated (t1_CH) cultures.
RNA Isolation and Reverse Transcription
Samples of the culture broth for the RNA isolation were centrifuged (13,000 g, 2 min, 4°C). The pellets were then washed with ice-cold distilled water and resuspended and diluted to reach optical density (measured at 600 nm) of approximately 1.0. Next, 3 ml of the suspension with the OD600 ≈ 1.0 sample were centrifuged, and the cell pellet was immediately stored at −80°C for subsequent RNA isolation. Frozen samples were thawed on ice, and the total RNA was isolated using the High Pure RNA Isolation Kit (Roche Life Science, Basel, Switzerland), according to the manufacturer’s instructions. The quality and concentration of the RNA samples were checked on a nanodrop machine (Thermo Fisher Scientific, Waltham, MA, United States).
Reverse transcription of RNA samples was performed with the Transcriptor First Strand cDNA Synthesis Kit (Roche Life Science, Basel, Switzerland), according to the manufacturer’s instructions.
Quantitative Real-Time PCR
All RT-qPCR analyses were performed in QuantStudio 5 instrument (ThermoFisher Scientific, Waltham, MA, United States) using the 5 × HOT FIREPol EvaGreen qPCR Mix Plus (ROX) (Solis BioDyne, Tartu, Estonia). The reaction mix (20 μl) was prepared according to the manufacturer’s instructions, using each primer (see Table 2) in the final concentration of 200 nM. The cycling conditions were set according to the manufacturer’s instructions, using a primer annealing temperature of 61°C. Primer specificity was confirmed through melt curve analysis after the cycling stage (95°C for 15 min, 61°C for 1 min, 95°C for 1 s). All RT-qPCR analyses were performed in triplicates, and the absence of contamination was confirmed by running no-template and no-RT controls.
Polymerase chain reaction efficiency was determined for each sample using a 5x serial dilution of cDNA samples (5x, 25x, 125x, and 625x), ranging between 94 and 110% for all samples with the correlation coefficient R2 > 0.99. For each gene in each sample, the average Cq value from the dilution interval 5–625x was used for further data analysis.
RT-qPCR Data Analysis
Four different algorithms or statistical methods were used for the evaluation of the stability of gene expression. These included NormFinder (Andersen et al., 2004), RefFinder (Xie et al., 2012), CV analysis (Boda et al., 2009) and Pairwise ΔCt method (Silver et al., 2006).
Results
RNA-Seq Data Analysis
In order to identify putative candidates for reference genes, we evaluated 5,442 predicted genes of C. beijerinckii and applied several filtering steps to reveal genes with stable expression indifferent in time and under different conditions (see section “Materials and Methods”).
First, we removed 4,370 genes based on the results from the differential expression analysis. Next, we eliminated genes according to their mean TPM and the number of candidates dropped from 1,072 to 448 genes. Finally, we applied filtering step based on the CV of TPM and only 160 genes remained as candidates for reference genes (see Supplementary Material 2).
In the next step, we narrowed the list of the candidate genes by considering their biological function as well as the fact that the genes with the same function were reported to be putative reference genes and/or used as reference genes in RT-qPCR experiments in other publications (see Table 3).
Calculated CV values of seven selected genes based on TPM values ranging from 18.7 to 29.4%. The most stable genes were rsmB, zmp, and rimO (see Table 4).
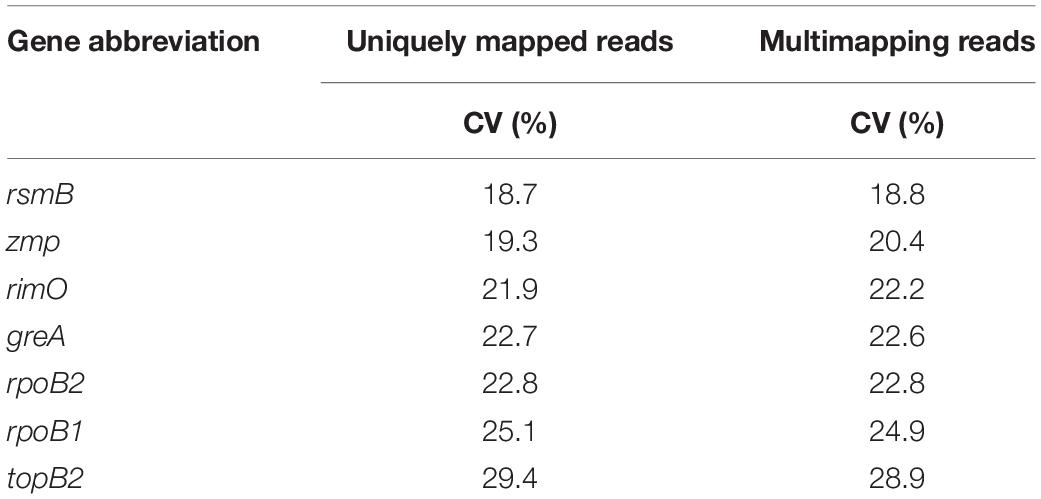
Table 4. Coefficient of variation of transcript per million (TPM) values from both count tables (uniquely mapped reads and multi-mapping reads).
Finally, we performed GO enrichment analysis of the final set of genes using all genomic loci as the gene universe to describe their functionality. We found 22 biological process (BP) GO terms (see Table 5) and 12 molecular function (MF) GO terms (see Table 6) that were significantly enriched (p-value < 0.05, Fisher’s exact test). From BP terms, the most significant terms related to “nucleic acid metabolic process,” “macromolecule metabolic process” or “cellular nitrogen compound metabolic process.” The most common term for MF GO terms was “nucleic acid binding.”
Analysis of Expression Stability Based on RT-qPCR Data
The expression levels, represented by the Cq values, of the seven candidate reference genes (zmp, rpoB1, rsmB, greA, rpoB2, topB2, and rimO) were assessed by RT-qPCR in seven cultivation samples. The samples were acquired in experiments with different cultivation conditions (standard, i.e., untreated culture, butanol-treated culture, and chloramphenicol-treated culture) and at different time points. The mean Cq values ranged from 20.2 to 33.3 for different dilutions and different genes in different samples (see Supplementary Material 1). An average Cq value was calculated for each gene in each sample across all dilutions, which was used for further analysis of expression stability. The median Cq for the different candidate genes ranged from 24.3 to 28.6, indicating that the expression levels of the different genes were not dramatically different (see Figure 1).
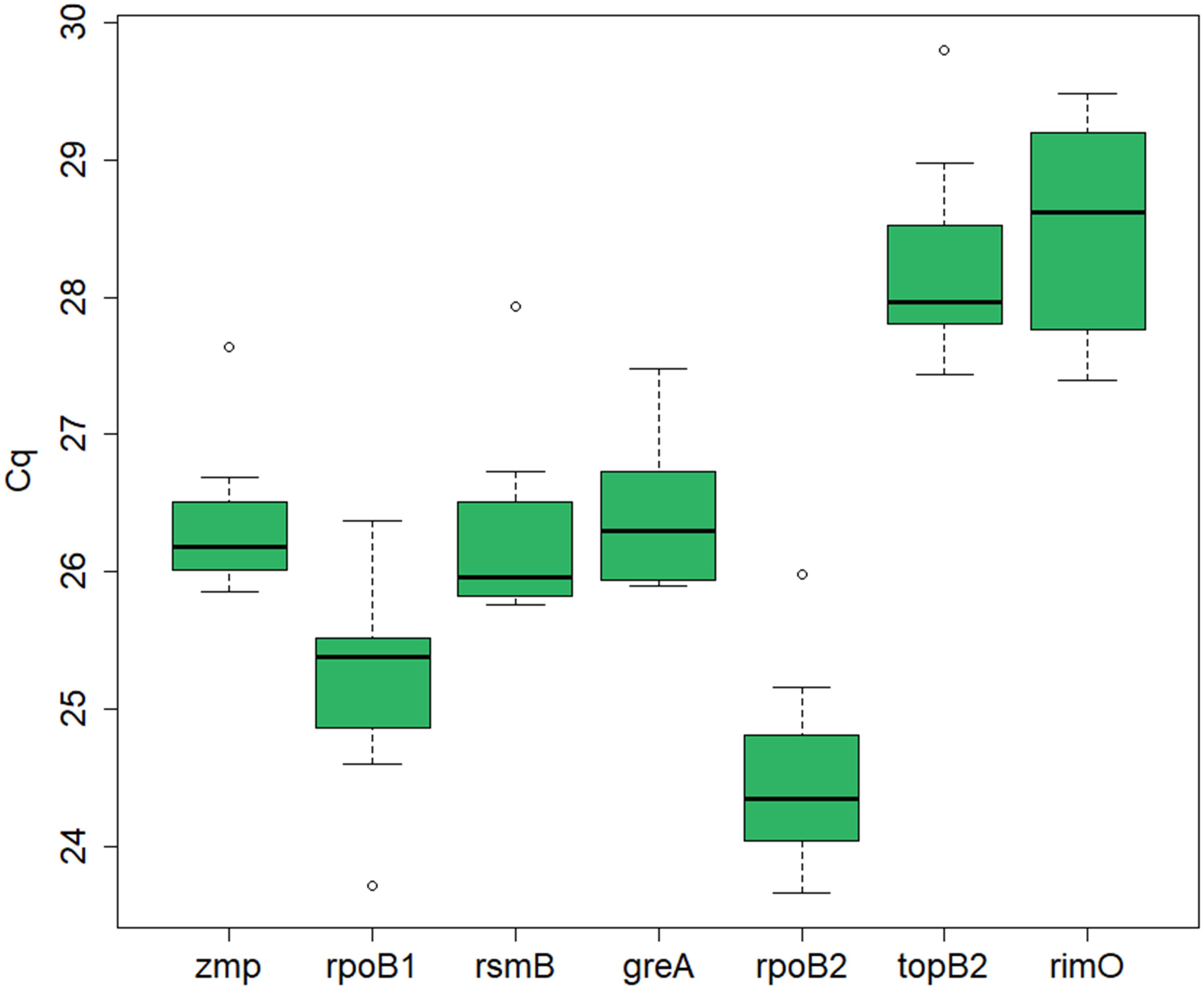
Figure 1. RT-qPCR Cq values for the candidate reference genes in C. beijerinckii NRRL B-598. Depicted is the median Cq value calculated for each candidate reference gene across all samples. The boxes indicate the 25th and 75th percentiles and the error bars indicate the maximum and minimum values gained across the samples for each gene.
According to NormFinder, CV and Pairwise ΔCt analyses, the genes with the most stable expression were zmp, greA, and rpoB2 (see Table 7 and Supplementary Material 1). Using NormFinder (Andersen et al., 2004), the samples were gathered into three groups with respect to the three different cultivation experiments from which they originated. According to RefFinder, including multiple analytical tools (Delta CT, BestKeeper, Normfinder, and Genorm), the comprehensive ranking of the genes with respect to expression stability was zmp, greA, rsmB, topB2, rpoB2, rpoB1, and rimO, with zmp and greA appearing as the best combination based on three out of the four tools (see Figure 2). Using the RefFinder tools, the samples were not gathered into groups. Taken together, zmp and greA appear to be the most suitable combination of genes that can be used for normalization of RT-qPCR experiments under tested conditions in C. beijerinckii NRRL B-598.
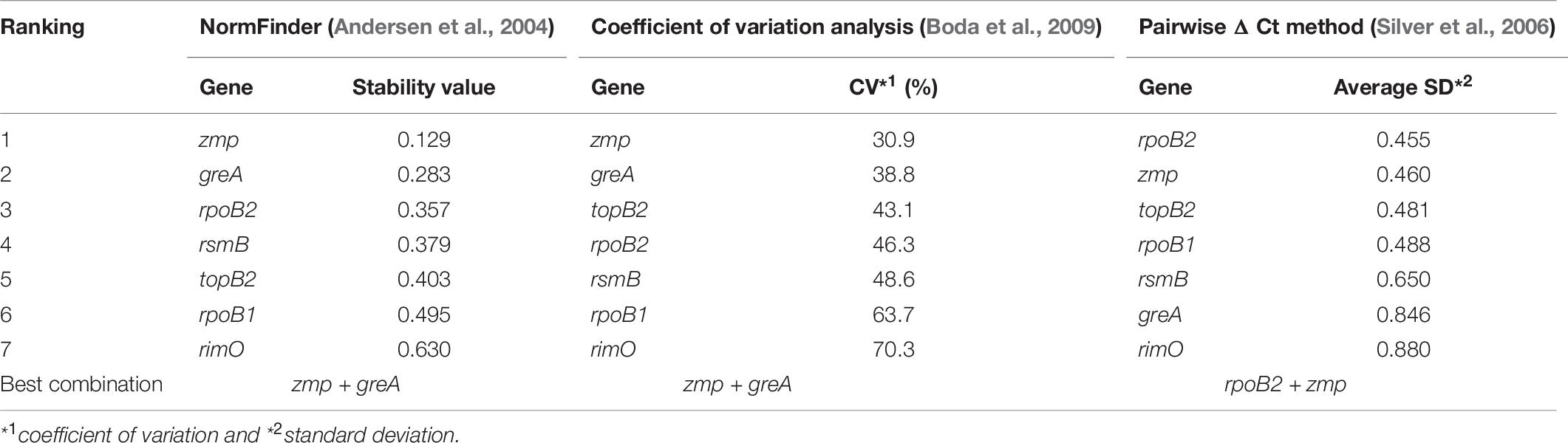
Table 7. Expression stability of candidate reference genes in C. beijerinckii NRRL B-598 assessed by different analytical tools.
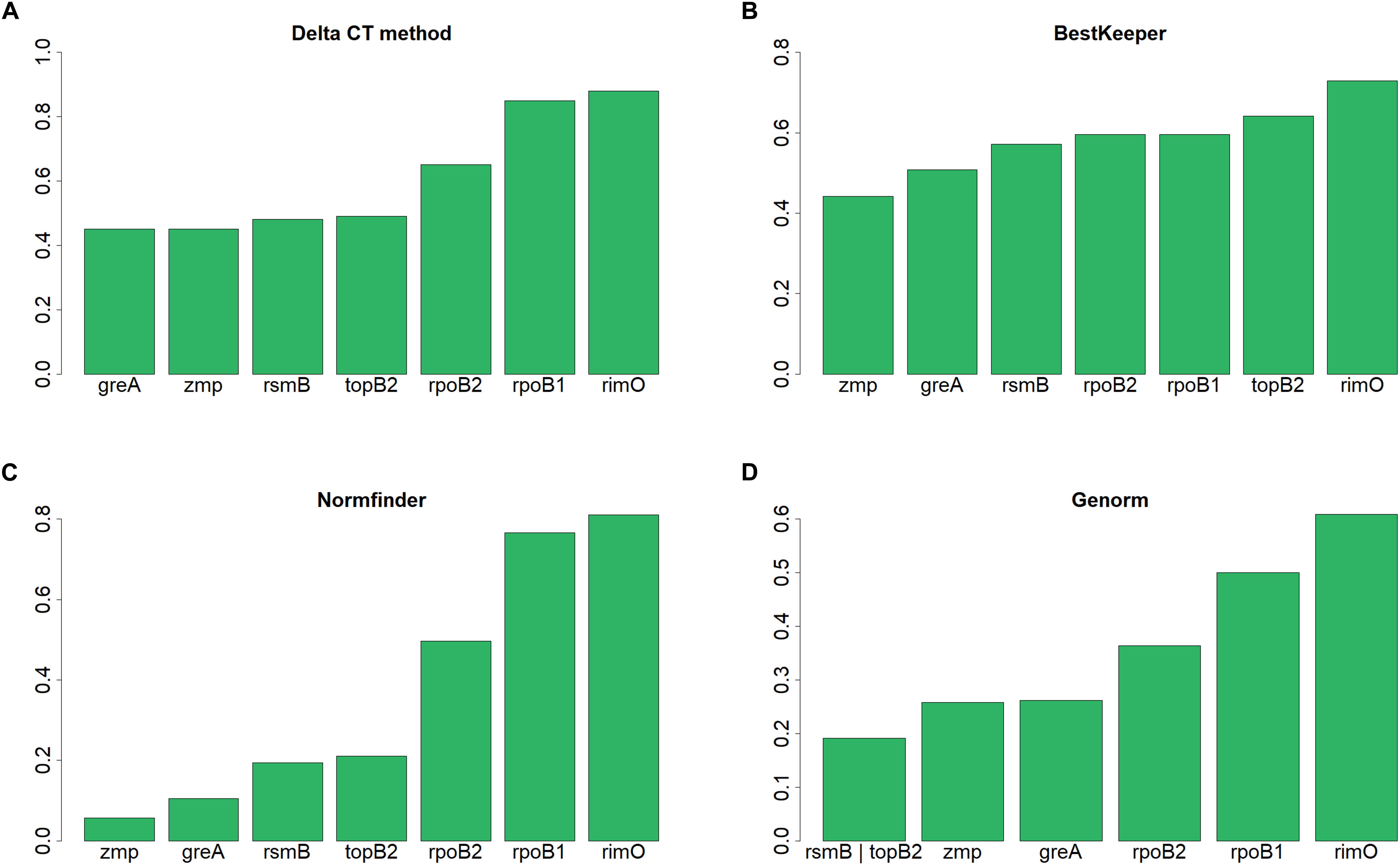
Figure 2. Expression stability of candidate reference genes in C. beijerinckii NRRL B-598 assessed by RefFinder tools. (A) Delta CT method, (B) BestKeeper, (C) Normfinder, and (D) Genorm.
Discussion
Normalization by reference genes is required for a precise analysis of gene expression by RT-qPCR. The choice of the reference genes, however, should not solely rely on the gold standard used for the particular cell type, such as 16S rRNA in the case of bacteria (Rocha et al., 2015), but should always be systematically and experimentally validated (Bustin et al., 2009). Moreover, according to the MIQE guidelines (Bustin et al., 2009), normalization against more than one reference gene is preferred.
In the case of C. beijerinckii NCIMB 8,052, a list of putative reference genes based on the analysis of transcriptomic data was previously published (Wang et al., 2011), and one of the candidate genes (peptidase T, Cbei_2428) was subsequently used as a reference for RT-qPCR experiments (Wang et al., 2012). However, completely different set of putative reference genes was generated for closely-related C. beijerinckii NRRL B-598 during our analysis of RNA-Seq data of the strain. Only Cbei_1214 was matched by X276_20450 in C. beijerinckii NRRL B-598, and X276_14515, a homolog of Cbei_2428 used in Wang et al. (2012) study, has not found to be a suitable reference gene in our case (see Supplementary Material 2 and section “Discussion” below). We investigated the gene expression stability in C. beijerinckii NRRL B-598 by evaluating both transcriptomic and RT-qPCR data. Based on these, we suggest a set of appropriate reference genes regarding the given set of tested conditions (see section “Materials and Methods”).
Identification of candidate reference genes in C. beijerinckii was performed by bioinformatics analysis of our previously published RNA-Seq data (Sedlar et al., 2018, 2019; Patakova et al., 2019). All data were processed again with up-to-date software and packages and we have introduced a new approach to the selection of reference genes based on RNA-Seq data. Pre-processing of our RNA-Seq data remained the same until the counting of mapped reads. Here, we decided to create two different count tables with two counting options. The first counting method is strict counting of unique reads mapped to the genomic loci and the second method also considers multi-mapping reads. Contribution of all multi-mapping reads were split equally among all genomic objects they mapped to. Therefore, the number of reads in the sample remained the same. Multi-mapping reads present a problem for downstream analyses as they reduce sensitivity (Canzar et al., 2016). Although several strategies and specialized tools were proposed to count multi-mapping reads (Roberts et al., 2013; Zytnicki, 2017), for our purposes, specificity was the principal issue. By selecting only genes where differential expression was not detected using uniquely neither multi mapped reads, we reduced the possibility of a type I error and, therefore, improved specificity. We are aware that this approach led to a higher possibility of type II error and, thus, to lower sensitivity. However, this would be problem only for following differential analysis aiming at finding differentially expressed genes. In our case, the aim was to completely reduce ambiguities. Next, we decided to calculate TPM values from obtained count tables, as TPM corrects differences in library sizes and gene lengths and enables comparisons among samples (Wagner et al., 2012).
A selection of housekeeping genes was performed by evaluation of the counts of insignificant changes in expression obtained from differential expression analysis, mean TPM values, and the CV of TPM values. First, filtering based on the results of the differential expression analysis, which we used to find genes with stable expression and minimal regulation, removed genes that were regulated in more than 25% of possible time-points pairs. In the next step, we eliminated genes with low mean TPM values as we were looking for genes with moderate stable expression. Low TPM values can result from technical noise in the data even after filtration. In the last step, we focused on the filtration of genes with a gradual increase or decrease in expression, which would not be discovered by differential expression analysis. For filtering, we used the CV of TPM values that can reflect those slow changes in expression and is often used in other studies geared toward the selection of reference genes (Carvalho et al., 2014; Hu et al., 2016; Pombo et al., 2017). Only 16 out of 160 genes identified by the RNA-Seq data analysis corresponded to the previously identified 177 housekeeping genes in C. beijerinckii NCIMB 8,052 (Wang et al., 2011) (see Supplementary Material 2). While this might suggest that the reference genes identified in this study are not utilizable for other C. beijerinckii strains, we believe this is rather a matter of the simplified methodology used by Wang et al. (2011). In their study, the identification of HKGs was only a supplementary result and calculating CV from RPKM values appeared to be inappropriate (Wagner et al., 2012). Moreover, identification of HKG based solely on the RNA-Seq data is less specific, see section “Discussion” below.
Final selection of the seven candidate reference genes (see Table 3) for RT-qPCR experiments was summarized by a GO enrichment analysis. GO enrichment analysis of biological processes revealed 22 enriched terms at significance level α = 0.05 of the Fisher’s exact test (see Table 5) and most of the enriched terms related to “nucleic acid metabolic process” (GO:0090304) and “RNA metabolic process” (GO:0016700) terms, which correspond with terms identified in other bacterial strains (Rocha et al., 2015). MF GO enrichment analysis revealed ten enriched terms and the most common term “nucleic acid binding” (GO:0003676) corresponds with identified biological processes (see Table 6).
Based on the RT-qPCR experiments, zmp (zinc metallopeptidase), greA (transcription elongation factor GreA), and rsmB (16S rRNA (cytosine(967)-C(5))-methyltransferase RsmB) were the most stably expressed genes (see Table 7 and Figure 2). In pathogenic Clostridium species, the zinc metalloproteases act like neurotoxins (Breidenbach and Brunger, 2004) or are involved in the degradation of extracellular substrates (Cafardi et al., 2013). The transcription factor GreA is evolutionarily conserved and widely distributed in prokaryotes (Marr and Roberts, 2000). The 16S rRNA-methyltransferases ensure methylation of 16S rRNA and in Gram-negative bacteria are involved in resistance to aminoglycosides (Lioy et al., 2014). In Gram-positive bacteria, 16S rRNA-methyltransferases was required for resistance to tetracycline antibiotics in the case of Streptococcus pneumoniae (Lupien et al., 2015), and to oxidative stress in the case of Staphylococcus aureus (Kyuma et al., 2015). The zmp gene figured amongst the best candidate genes in Vigna angularis (Chi et al., 2016), the transcription elongation factor gene in Vernicia fordii Hemsl. (Han et al., 2012), and the 16S rRNA methyltransferase gene in Rhodococcus opacus (Delorenzo and Moon, 2018). Generally, the three most commonly tested and validated bacterial reference genes are 16S ribosomal RNA, DNA gyrase A, and recombinase A (Rocha et al., 2015). In our case, the genes of 16S rRNA and recombinase were not within the list of the 160 candidate genes after the bioinformatics analysis of the transcriptomic data (see Supplementary Material 2). This was expected for the 16S rRNA gene as wet-lab ribodepletion was performed before sequencing and the remaining contamination was removed in silico with SortMeRNA. The DNA topoisomerase (gyrase) subunit was within the list, though did not rank amongst the genes with the most stable expression, according to the RT-qPCR results. In C. beijerinckii NCIMB 8,052, gene encoding peptidase T was chosen as a reference gene (Wang et al., 2012). In C. difficile, the genes of 16S rRNA, adenylate kinase, and 30S ribosomal protein S10 displayed the most stable expression within eight tested candidate genes (Metcalf et al., 2010). Nevertheless, the ranking differed for three different C. difficile strains tested (Metcalf et al., 2010), confirming the need for a careful selection of reference genes for each species (Bustin et al., 2009). The 16S rRNA gene was also chosen as a reference in C. botulinum (Kirk et al., 2014). The most suitable genes for normalization of gene expression in C. ljungdahlii were genes of gyrase subunit A, transcriptional termination factor, and formate-tetrahydrofolate ligase (Liu et al., 2013). Within the set of the tested candidate genes, the bacterial standards of 16S rRNA and recombinase A genes (Rocha et al., 2015) displayed the least stable expression in C. ljungdahlii (Liu et al., 2013).
The need for the validation of genes selected using only RNA-Seq data is supported by the comparison of the coefficients of variation calculated from Cq (see Table 7) and the TPM values (see Table 4). Although genes zmp and greA maintain a high rating in both statistics, suggesting that rankings can in some cases correlate, results for rsmB and rimO genes tell otherwise. The rsmB gene was the most stable according to the RNA-Seq data, yet RT-qPCR experiments show that the other three respectively, four genes were more suitable as candidate genes (see Table 7). In the case of the rimO gene, which was the third most stable gene according to the TPM values, it dropped to the last place after experimental validation. Moreover, the average CV of Cq values was more than two times higher than the average CV of TPM values (2.14 × for uniquely mapped and 2.13 × for multi mapped reads) (see Tables 4, 7), indicating that the RNA-Seq provides only less specific results selecting a wider range of genes on a given CV threshold value. These results suggest that the selection of reference genes cannot be performed by RNA-Seq data analysis alone, yet it can be used for the compilation of candidate genes list. However, validation by RT-qPCR experiments is always needed.
Conclusion
We identified and validated a novel set of reference genes of C. beijerinckii NRRL B-598. We selected 160 candidate reference genes based on analysis of all currently available RNA-Seq data for the strain covering several different experimental conditions. Selection of seven genes (zmp, rpoB1, rsmB, greA, rpoB2, topB2, and rimO) was summarized by GO enrichment analysis and further validated by RT-qPCR assays and statistical testing by four statistical algorithms (NormFinder, RefFinder, CV analysis, and Pairwise ΔCt method). The analysis ranked seven genes by their expression stability, presenting zinc metallopeptidase (zmp) and transcription elongation factor GreA (greA) as an appropriate set of reference genes regarding tested set of conditions. Our results can be helpful for selection of reference genes in other C. beijerinckii strains, and our methodology suggests that RNA-Seq data can be used for identification of novel reference genes, but their validation by RT-qPCR experiments is always needed.
Data Availability Statement
Publicly available datasets were analyzed in this study. This data can be found here: all computational analyses using sequencing data in this work are done against the reference sequence deposited at DDBJ/ENA/GenBank under the accession number CP011966.3. RNA-Seq data used in this study are available from NCBI SRA database under the accession numbers SRR6375604, SRR10556738–SRR10556761 and SRR10569082–SRR10569093.
Author Contributions
KJ: conceptualization, methodology, formal analysis, data curation, visualization, and writing-original draft preparation. HS: conceptualization, methodology, validation, formal analysis, visualization, and writing-original draft preparation. JK: writing-original draft preparation. MV: validation and investigation. BB: conceptualization and investigation. PP and IP: supervision, resources, and funding. KS: conceptualization, methodology, formal analysis, and writing-original draft preparation. All authors contributed to the article and approved the submitted version.
Funding
This work has been supported by grant project GACR 17-00551S.
Conflict of Interest
The authors declare that the research was conducted in the absence of any commercial or financial relationships that could be construed as a potential conflict of interest.
Supplementary Material
The Supplementary Material for this article can be found online at: https://www.frontiersin.org/articles/10.3389/fmicb.2021.640054/full#supplementary-material
References
Agu, C. V., Ujor, V., and Ezeji, T. C. (2019). Metabolic engineering of Clostridium beijerinckii to improve glycerol metabolism and furfural tolerance. Biotechnol. Biofuels 12:50. doi: 10.1186/s13068-019-1388-9
Andersen, C. L., Jensen, J. L., and Ørntoft, T. F. (2004). Normalization of real-time quantitative reverse transcription-PCR data: a model-based variance estimation approach to identify genes suited for normalization, applied to bladder and colon cancer data sets. Cancer Res. 64, 5245–5250. doi: 10.1158/0008-5472.CAN-04-0496
Boda, E., Pini, A., Hoxha, E., Parolisi, R., and Tempia, F. (2009). Selection of reference genes for quantitative real-time RT-PCR studies in mouse brain. J. Mol. Neurosci. 37, 238–253. doi: 10.1007/s12031-008-9128-9
Bolger, A. M., Lohse, M., and Usadel, B. (2014). Trimmomatic: a flexible trimmer for Illumina sequence data. Bioinformatics 30, 2114–2120. doi: 10.1093/bioinformatics/btu170
Breidenbach, M. A., and Brunger, A. T. (2004). Substrate recognition strategy for butulinum neurotoxin serotype A. Nature 432, 925–929. doi: 10.1038/nature03123
Bustin, S. A., Benes, V., Garson, J. A., Hellemans, J., Huggett, J., Kubista, M., et al. (2009). The MIQE guidelines: minimum information for publication of quantitative real-time PCR experiments. Clin. Chem. 55, 611–622. doi: 10.1373/clinchem.2008.112797
Cafardi, V., Biagini, M., Martinelli, M., Leuzzi, R., Rubino, J. T., Cantini, F., et al. (2013). Identification of a novel zinc metalloprotease through a global analysis of Clostridium difficile extracellular proteins. PLoS One 8:e81306. doi: 10.1371/journal.pone.0081306
Canzar, S., Elbassioni, K., Jones, M., and Mestre, J. (2016). Resolving conflicting predictions from multimapping reads. J. Comput. Biol. 23, 203–217. doi: 10.1089/cmb.2015.0164
Carvalho, D. M., de Sá, P. H., Castro, T. L. P., Carvalho, R. D., Pinto, A., Gil, D. J. P., et al. (2014). Reference genes for RT-qPCR studies in Corynebacterium pseudotuberculosis identified through analysis of RNA-seq data. Antonie van Leeuwenhoek 106, 605–614. doi: 10.1007/s10482-014-0231-3
Chapman, J. R., and Waldenström, J. (2015). With reference to reference genes: a systematic review of endogenous controls in gene expression studies. PLoS One 10:e0141853. doi: 10.1371/journal.pone.0141853
Chi, C., Shen, Y., Yin, L., Ke, X., Han, D., and Zuo, Y. (2016). Selection and validation of reference genes for gene expression analysis in Vigna angularis using quantitative real-time RT-PCR. PLoS One 11:e0168479. doi: 10.1371/journal.pone.0168479
Delorenzo, D. M., and Moon, T. S. (2018). Selection of stable reference genes for RT-qPCR in Rhodococcus opacus PD630. Sci. Rep. 8:6019. doi: 10.1038/s41598-018-24486-w
Derveaux, S., Vandesompele, J., and Hellemans, J. (2010). How to do successful gene expression analysis using real-time PCR. Methods 50, 227–230. doi: 10.1016/j.ymeth.2009.11.001
Dobin, A., Davis, C. A., Schlesinger, F., Drenkow, J., Zaleski, C., Jha, S., et al. (2013). STAR: ultrafast universal RNA-seq aligner. Bioinformatics 29, 15–21. doi: 10.1093/bioinformatics/bts635
Ewels, P., Magnusson, M., Lundin, S., and Käller, M. (2016). MultiQC: summarize analysis results for multiple tools and samples in a single report. Bioinformatics 32, 3047–3048. doi: 10.1093/bioinformatics/btw354
Ginzinger, D. G. (2002). Gene quantification using real-time quantitative PCR. Exp. Hematol. 30, 503–512. doi: 10.1016/S0301-472X(02)00806-8
Gomes, A. ÉI., Stuchi, L. P., Siqueira, N. M. G., Henrique, J. B., Vicentini, R., Ribeiro, M. L., et al. (2018). Selection and validation of reference genes for gene expression studies in Klebsiella pneumoniae using reverse transcription quantitative real-time PCR. Sci. Rep. 8:9001. doi: 10.1038/s41598-018-27420-2
Han, X., Lu, M., Chen, Y., Zhan, Z., Cui, Q., and Wang, Y. (2012). Selection of reliable reference genes for gene expression studies using real-time PCR in tung tree during seed development. PLoS One 7:e43084. doi: 10.1371/journal.pone.0043084
Hu, Y., Xie, S., and Yao, J. (2016). Identification of novel reference genes suitable for qRT-PCR normalization with respect to the zebrafish developmental stage. PLoS One 11:e0149277. doi: 10.1371/journal.pone.0149277
Jo, J., Choi, S., Oh, J., Lee, S.-G., Choi, S. Y., Kim, K. K., et al. (2019). Conventionally used reference genes are not outstanding for normalization of gene expression in human cancer research. BMC Bioinformatics 20(Suppl. 10):245. doi: 10.1186/s12859-019-2809-2
Kirk, D. G., Palonen, E., Korkeala, H., and Lindström, M. (2014). Evaluation of normalization reference genes for RT-qPCR analysis of spo0A and four sporulation sigma factor genes in Clostridium botulinum group I strain ATCC 3502. Anaerobe 26, 14–19. doi: 10.1016/j.anaerobe.2013.12.003
Kopylova, E., Noé, L., and Touzet, H. (2012). SortMeRNA: fast and accurate filtering of ribosomal RNAs in metatranscriptomic data. Bioinformatics 28, 3211–3217. doi: 10.1093/bioinformatics/bts611
Kyuma, T., Kimura, S., Hanada, Y., Suzuki, T., Sekimizu, K., and Kaito, C. (2015). Ribosomal RNA methyltransferases contribute to Staphylococcus aureus virulence. FEBS J. 282, 2570–2584. doi: 10.1111/febs.13302
Li, H., Handsaker, B., Wysoker, A., Fennell, T., Ruan, J., Homer, N., et al. (2009). The sequence alignment/map format and SAMtools. Bioinformatics 25, 2078–2079. doi: 10.1093/bioinformatics/btp352
Liang, P., Guo, Y., Zhou, X., and Gao, X. (2014). Expression profiling in Bemisia tabaci under insecticide treatment: indicating the necessity for custom reference gene selection. PLoS One 9:e87514. doi: 10.1371/journal.pone.0087514
Liao, Y., Smyth, G. K., and Shi, W. (2014). FeatureCounts: an efficient general purpose program for assigning sequence reads to genomic features. Bioinformatics 30, 923–930. doi: 10.1093/bioinformatics/btt656
Liao, Y., Smyth, G. K., and Shi, W. (2019). The R package Rsubread is easier, faster, cheaper and better for alignment and quantification of RNA sequencing reads. Nucleic Acids Res. 47:e47. doi: 10.1093/nar/gkz114
Lioy, V. S., Goussard, S., Guerineau, V., Yoon, E. J., Courvalin, P., Galimand, M., et al. (2014). Aminoglycoside resistance 16S rRNA methyltransferases block endogenous methylation, affect translation efficiency and fitness of the host. RNA 20, 382–391. doi: 10.1261/rna.042572.113
Liu, D., Hao, K., Wang, W., Peng, C., Dai, Y., Jin, R., et al. (2017). Rv2629 overexpression delays Mycobacterium smegmatis and Mycobacteria tuberculosis entry into log-phase and increases pathogenicity of Mycobacterium smegmatis in mice. Front. Microbiol. 8:2231. doi: 10.3389/fmicb.2017.02231
Liu, J., Tan, Y., Yang, X., Chen, X., and Li, F. (2013). Evaluation of Clostridium ljungdahlii DSM 13528 reference genes in gene expression studies by qRT-PCR. J. Biosci. Bioeng. 116, 460–464. doi: 10.1016/j.jbiosc.2013.04.011
Love, M. I., Huber, W., and Anders, S. (2014). Moderated estimation of fold change and dispersion for RNA-seq data with DESeq2. Genome Biol. 15:550. doi: 10.1186/s13059-014-0550-8
Lupien, A., Gingras, H., Leprohon, P., and Ouellette, M. (2015). Induced tigecycline resistance in Streptococcus pneumoniae mutants reveals mutations in ribosomal proteins and rRNA. J. Antimicrob. Chemother. 70, 2973–2980. doi: 10.1093/jac/dkv211
Marr, M. T., and Roberts, J. W. (2000). Function of transcription cleavage factors GreA and GreB at a regulatory pause site. Mol. Cell 6, 1275–1285. doi: 10.1016/S1097-2765(00)00126-X
Metcalf, D., Sharif, S., and Weese, J. S. (2010). Evaluation of candidate reference genes in Clostridium difficile for gene expression normalization. Anaerobe 16, 439–443. doi: 10.1016/j.anaerobe.2010.06.007
Patakova, P., Branska, B., Sedlar, K., Vasylkivska, M., Jureckova, K., Kolek, J., et al. (2019). Acidogenesis, solventogenesis, metabolic stress response and life cycle changes in Clostridium beijerinckii NRRL B-598 at the transcriptomic level. Sci. Rep. 9:1371. doi: 10.1038/s41598-018-37679-0
Patakova, P., Kolek, J., Jureckova, K., Branska, B., Sedlar, K., Vasylkivska, M., et al. (2020). Deeper below the surface—transcriptional changes in selected genes of Clostridium beijerinckii in response to butanol shock. Microbiologyopen 10:e1146. doi: 10.1002/mbo3.1146
Peng, S., Stephan, R., Hummerjohann, J., and Tasara, T. (2014). Evaluation of three reference genes of Escherichia coli for mRNA expression level normalization in view of salt and organic acid stress exposure in food. FEMS Microbiol. Lett. 355, 78–82. doi: 10.1111/1574-6968.12447
Pombo, M. A., Zheng, Y., Fei, Z., Martin, G. B., and Rosli, H. G. (2017). Use of RNA-seq data to identify and validate RT-qPCR reference genes for studying the tomato-Pseudomonas pathosystem. Sci. Rep. 7:44905. doi: 10.1038/srep44905
Quast, C., Pruesse, E., Yilmaz, P., Gerken, J., Schweer, T., Yarza, P., et al. (2013). The SILVA ribosomal RNA gene database project: improved data processing and web-based tools. Nucleic Acids Res. 41, 590–596. doi: 10.1093/nar/gks1219
Roberts, A., Schaeffer, L., and Pachter, L. (2013). Updating RNA-Seq analyses after re-annotation. Bioinformatics 29, 1631–1637. doi: 10.1093/bioinformatics/btt197
Rocha, D. J. P., Santos, C. S., and Pacheco, L. G. C. (2015). Bacterial reference genes for gene expression studies by RT-qPCR: survey and analysis. Antonie van Leeuwenhoek 108, 685–693. doi: 10.1007/s10482-015-0524-1
Sedlar, K., Kolek, J., Gruber, M., Jureckova, K., Branska, B., Csaba, G., et al. (2019). A transcriptional response of Clostridium beijerinckii NRRL B-598 to a butanol shock. Biotechnol. Biofuels 12, 1–16. doi: 10.1186/s13068-019-1584-7
Sedlar, K., Kolek, J., Provaznik, I., and Patakova, P. (2017). Reclassification of non-type strain Clostridium pasteurianum NRRL B-598 as Clostridium beijerinckii NRRL B-598. J. Biotechnol. 244, 1–3. doi: 10.1016/j.jbiotec.2017.01.003
Sedlar, K., Koscova, P., Vasylkivska, M., Branska, B., Kolek, J., Kupkova, K., et al. (2018). Transcription profiling of butanol producer Clostridium beijerinckii NRRL B-598 using RNA-Seq. BMC Genomics 19:415. doi: 10.1186/s12864-018-4805-8
Silver, N., Best, S., Jiang, J., and Thein, S. L. (2006). Selection of housekeeping genes for gene expression studies in human reticulocytes using real-time PCR. BMC Mol. Biol. 7:33. doi: 10.1186/1471-2199-7-33
Song, Y., Wang, Y., Guo, D., and Jing, L. (2019). Selection of reference genes for quantitative real-time PCR normalization in the plant pathogen Puccinia helianthi Schw. BMC Plant Biol. 19:20. doi: 10.1186/s12870-019-1629-x
Vandesompele, J., De Preter, K., Pattyn, F., Poppe, B., Van Roy, N., De Paepe, A., et al. (2002). Accurate normalization of real-time quantitative RT-PCR data by geometric averaging of multiple internal control genes. Genome Biol. 3:research0034. doi: 10.1186/gb-2002-3-7-research0034
Vasylkivska, M., Jureckova, K., Branska, B., Sedlar, K., Kolek, J., Provaznik, I., et al. (2019). Transcriptional analysis of amino acid, metal ion, vitamin and carbohydrate uptake in butanol-producing Clostridium beijerinckii NRRL B-598. PLoS One 14:e0224560. doi: 10.1371/journal.pone.0224560
Wagner, G. P., Kin, K., and Lynch, V. J. (2012). Measurement of mRNA abundance using RNA-seq data: RPKM measure is inconsistent among samples. Theory Biosci. 131, 281–285. doi: 10.1007/s12064-012-0162-3
Wang, C., Ye, F., Kumar, V., Gao, Y. G., and Zhang, L. H. (2014). BswR controls bacterial motility and biofilm formation in Pseudomonas aeruginosa through modulation of the small RNA rsmZ. Nucleic Acids Res. 42, 4563–4576. doi: 10.1093/nar/gku106
Wang, Y., Li, X., Mao, Y., and Blaschek, H. P. (2011). Single-nucleotide resolution analysis of the transcriptome structure of Clostridium beijerinckii NCIMB 8052 using RNA-Seq. BMC Genomics 12:479. doi: 10.1186/1471-2164-12-479
Wang, Y., Li, X., Mao, Y., and Blaschek, H. P. (2012). Genome-wide dynamic transcriptional profiling in Clostridium beijerinckii NCIMB 8052 using single-nucleotide resolution RNA-Seq. BMC Genomics 13:102. doi: 10.1186/1471-2164-13-102
Wang, Y., Zhang, Z. T., Seo, S. O., Lynn, P., Lu, T., Jin, Y. S., et al. (2016). Bacterial genome editing with CRISPR-Cas9: deletion, integration, single nucleotide modification, and desirable “clean” mutant selection in Clostridium beijerinckii as an example. ACS Synth. Biol. 5, 721–732. doi: 10.1021/acssynbio.6b00060
Xie, F., Xiao, P., Chen, D., Xu, L., and Zhang, B. (2012). miRDeepFinder: a miRNA analysis tool for deep sequencing of plant small RNAs. Plant Mol. Biol. doi: 10.1007/s11103-012-9885-2 ∗∗Q.
Xin, X., Cheng, C., Du, G., Chen, L., and Xue, C. (2020). Metabolic engineering of histidine kinases in Clostridium beijerinckii for enhanced butanol production. Front. Bioeng. Biotechnol. 8:214. doi: 10.3389/fbioe.2020.00214
Keywords: HKG, housekeeping genes, non-model organisms, biofuel, Clostridium
Citation: Jureckova K, Raschmanova H, Kolek J, Vasylkivska M, Branska B, Patakova P, Provaznik I and Sedlar K (2021) Identification and Validation of Reference Genes in Clostridium beijerinckii NRRL B-598 for RT-qPCR Using RNA-Seq Data. Front. Microbiol. 12:640054. doi: 10.3389/fmicb.2021.640054
Received: 10 December 2020; Accepted: 17 February 2021;
Published: 18 March 2021.
Edited by:
Krishnaveni Mishra, University of Hyderabad, IndiaReviewed by:
Yi Wang, Auburn University, Auburn, United StatesPeter-Dürre, University of Ulm, Ulm, Germany
Copyright © 2021 Jureckova, Raschmanova, Kolek, Vasylkivska, Branska, Patakova, Provaznik and Sedlar. This is an open-access article distributed under the terms of the Creative Commons Attribution License (CC BY). The use, distribution or reproduction in other forums is permitted, provided the original author(s) and the copyright owner(s) are credited and that the original publication in this journal is cited, in accordance with accepted academic practice. No use, distribution or reproduction is permitted which does not comply with these terms.
*Correspondence: Karel Sedlar, c2VkbGFyQHZ1dC5jeg==