- 1Mars Global Food Safety Center, Beijing, China
- 2Center for Food Safety, University of Georgia, Griffin, GA, United States
- 3Department of Food Science, Cornell University, Ithaca, NY, United States
The use of whole genome sequencing (WGS) data generated by the long-read sequencing platform Oxford Nanopore Technologies (ONT) has been shown to provide reliable results for Salmonella serotype prediction in a previous study. To further meet the needs of industry for accurate, rapid, and cost-efficient Salmonella confirmation and serotype classification, we evaluated the serotype prediction accuracy of using WGS data from multiplex ONT sequencing with three, four, five, seven, or ten Salmonella isolates (each isolate represented one Salmonella serotype) pooled in one R9.4.1 flow cell. Each multiplexing strategy was repeated with five flow cells, and the loaded samples were sequenced simultaneously in a GridION sequencer for 48 h. In silico serotype prediction was performed using both SeqSero2 (for raw reads and genome assemblies) and SISTR (for genome assemblies) software suites. An average of 10.63 Gbp of clean sequencing data was obtained per flow cell. We found that the unevenness of data yield among each multiplexed isolate was a major barrier for shortening sequencing time. Using genome assemblies, both SeqSero2 and SISTR accurately predicted all the multiplexed isolates under each multiplexing strategy when depth of genome coverage ≥50× for each isolate. We identified that cross-sample barcode assignment was a major cause of prediction errors when raw sequencing data were used for prediction. This study also demonstrated that, (i) sequence data generated by ONT multiplex sequencing can be used to simultaneously predict serotype for three to ten Salmonella isolates, (ii) with three to ten Salmonella isolates multiplexed, genome coverage at ≥50× per isolate was obtained within an average of 6 h of ONT multiplex sequencing, and (iii) with five isolates multiplexed, the cost per isolate might be reduced to 23% of that incurred with single ONT sequencing. This study is a starting point for future validation of multiplex ONT WGS as a cost-efficient and rapid Salmonella confirmation and serotype classification tool for the food industry.
Introduction
Salmonella is a genus of rod-shaped, Gram-negative bacteria which has imposed great risk on food production and public health (GMA, 2009; Scallan et al., 2011; Oh and Park, 2017; EFSA and ECDC, 2019a,b). As one of the most common foodborne pathogens, non-typhoid Salmonella contributes the second most cases of infections in the U.S. (Tack et al., 2020). Contamination often results in significant financial loss throughout the food supply chain, which makes effective surveillance and control of this pathogen necessary. Although there are only two species of Salmonella, over 2,600 serotypes have been reported to date (Issenhuth-Jeanjean et al., 2014). Thus, accurate and rapid identification of Salmonella serotypes is important for efficient source tracking during incident investigation and Salmonella surveillance.
The widely used White-Kauffmann-Le minor scheme defines Salmonella serotypes mainly by 46 somatic (O) and 114 flagella (H1/H2) antigens, and an antigenic formula with specific combination of O and H antigens is used to differentiate serotypes (Grimont and Weill, 2007). Traditional serotyping methods that use this scheme involve the use of specific antisera (Herikstad et al., 2002), with some serotypes further defined by biochemical characteristics. Reliable serotyping with traditional methods is time consuming, and requires careful maintenance of a large number of different antisera (Wattiau et al., 2011; Shi et al., 2015). Several molecular methods have been developed to overcome the drawbacks of traditional serotyping methods (Foley et al., 2007; Wattiau et al., 2011; Diep et al., 2019); for example, PCR (Herrera-León et al., 2007) and microarray-based subtyping methods (McQuiston et al., 2011) have been widely used. Due to increasing utilization of next generation sequencing (NGS), in silico subtyping through whole genome sequencing (WGS) data is gradually becoming mainstream. Several in silico methods have been developed to predict Salmonella serotypes from WGS sequencing data (Zhang et al., 2015; Ashton et al., 2016; Yoshida et al., 2016; Zhang et al., 2019), among which SISTR (Yoshida et al., 2016), SeqSero (Zhang et al., 2015), and its recent upgrade SeqSero2 (Zhang et al., 2019) have been substantively validated and shown to deliver accurate predictions (Diep et al., 2019; Banerji et al., 2020; Cooper et al., 2020). Such methods are now being accepted and used by regulators, industry, and academia for source attribution, outbreak investigation, surveillance, and research purposes (Didelot et al., 2016; EFSA Panel on Biological Hazards et al., 2019).
Illumina sequencing platforms1, which use short read sequencing approaches have been used commonly to provide WGS data for surveillance of foodborne pathogens, including Salmonella (Allard et al., 2016; Ashton et al., 2016; CDC, 2006, 2018). While Illumina sequencing has advantages of low error rate and high throughput, its short reads have limited capability for the closed assembly of genomes. Oxford Nanopore Technologies (ONT) offers comparable sequencing platforms with extra-long reads, real time sequencing, and rapid processing time2, but has higher rates of sequencing errors than Illumina (Fox et al., 2014; Rang et al., 2018). Raw ONT reads are long enough to be treated as “contigs” by SeqSero2, and this serotype prediction tool offers two options for ONT data: (i) raw ONT reads using SeqSero2 raw reads workflow (ii) raw ONT reads using SeqSero2 assembly workflow (Zhang et al., 2019). In SeqSero2 v1.1.23, a single nanopore workflow is available for both raw ONT reads and their genome assemblies. This workflow algorithmically unifies the processing of both ONT raw reads and ONT assemblies by taking advantage of the long lengths of ONT reads, which are usually similar to those of contigs assembled from short reads (i.e., Illumina reads). With the serotype prediction tool SISTR, ONT raw reads have to first be assembled to correct base call errors, which have been reported to compromise SISTR prediction results (Zhang et al., 2019). Our previous evaluation of ONT sequencing based on sequencing a single Salmonella strain per flow cell and data analyzed by both the SeqSero2 raw reads workflow and SISTR revealed that a total of 2 h of ONT sequencing data were sufficient for successful Salmonella serotyping (Xu et al., 2020). This represents a considerable time saving compared to short-read-sequencing-based approaches. The ONT platforms have the capability for simultaneously sequencing multiple strains by applying DNA indexing (multiplex sequencing) (Karamitros and Magiorkinis, 2018). Combined with the continuously growing sequencing data yield of ONT sequencers, multiplex sequencing allows large quantities of complexed samples to be sequenced in one run (Piper et al., 2019; Kennedy et al., 2020). Several studies have successfully performed species identification by Multi Locus Sequence Typing (MLST) analysis from multiplexed sequencing data using the multiplex sequencing system provided by ONT (Imai et al., 2020; Liou et al., 2020). However, mis-assignment or cross-contamination of barcodes was also observed (Xu et al., 2018).
This study aimed to evaluate the efficiency, accuracy, and economic value of serotype prediction from ONT sequencing data generated from multiplexing up to ten Salmonella isolates. A combination of common, rare, and difficult-to-differentiate isolates were selected to (i) investigate whether correct antigenic formulae are assigned to isolates, (ii) determine whether mis-assignment or cross-contamination occurs during the process, and (iii) find the best combination of isolate number for multiplexing and the total sequencing time for the most efficient and accurate serotype prediction.
Materials and Methods
Bacterial Strains
Ten Salmonella isolates representing 10 different serotypes were assessed in the current study (Table 1). Seven isolates represented some of the most common serotypes, which included Salmonella serotype Typhimurium (including Typhimurium O5−), 4,[5],12:i:-, Paratyphi B (dt +), Enteritidis, Mbandaka, and Senftenberg. These were selected from i) the top 20 serotypes reported by the U.S. national Salmonella surveillance system (CDC, 2006, 2018) and ii) the top 20 serotypes causing human infections worldwide as reported to the WHO (Galanis et al., 2006). One isolate representing a rare serotype (serotype Havana) found in the food industry (information obtained by personal communication) was also included. Six isolates represented six serotypes that may be difficult to identify with molecular-level serotyping methods such as MLST and Pulsed-Field Gel Electrophoresis (PFGE). These six serotypes included (i) serotype Typhimurium, its O5– variant, and serotype 4,[5],12:i:-, which are difficult to differentiate by MLST and phylogenetic analysis due to their close relatedness (Ranieri et al., 2013); (ii) serotype Paratyphi B (dt +), which has been incorrectly predicted by other molecular subtyping methods such as PFGE (Bailey et al., 2002; Soyer et al., 2010; Zou et al., 2012), and (iii) one strain of S. enterica subspecies salamae, and one strain of S. bongori; these two strains represent subspecies and species distinct from the most common Salmonella subspecies (i.e., S. enterica subspecies enterica). Detailed isolate information, including all sequence data associated with a given isolate, can be found at www.foodmicrobetracker.com under the isolate ID (e.g., FSL S5-0393).
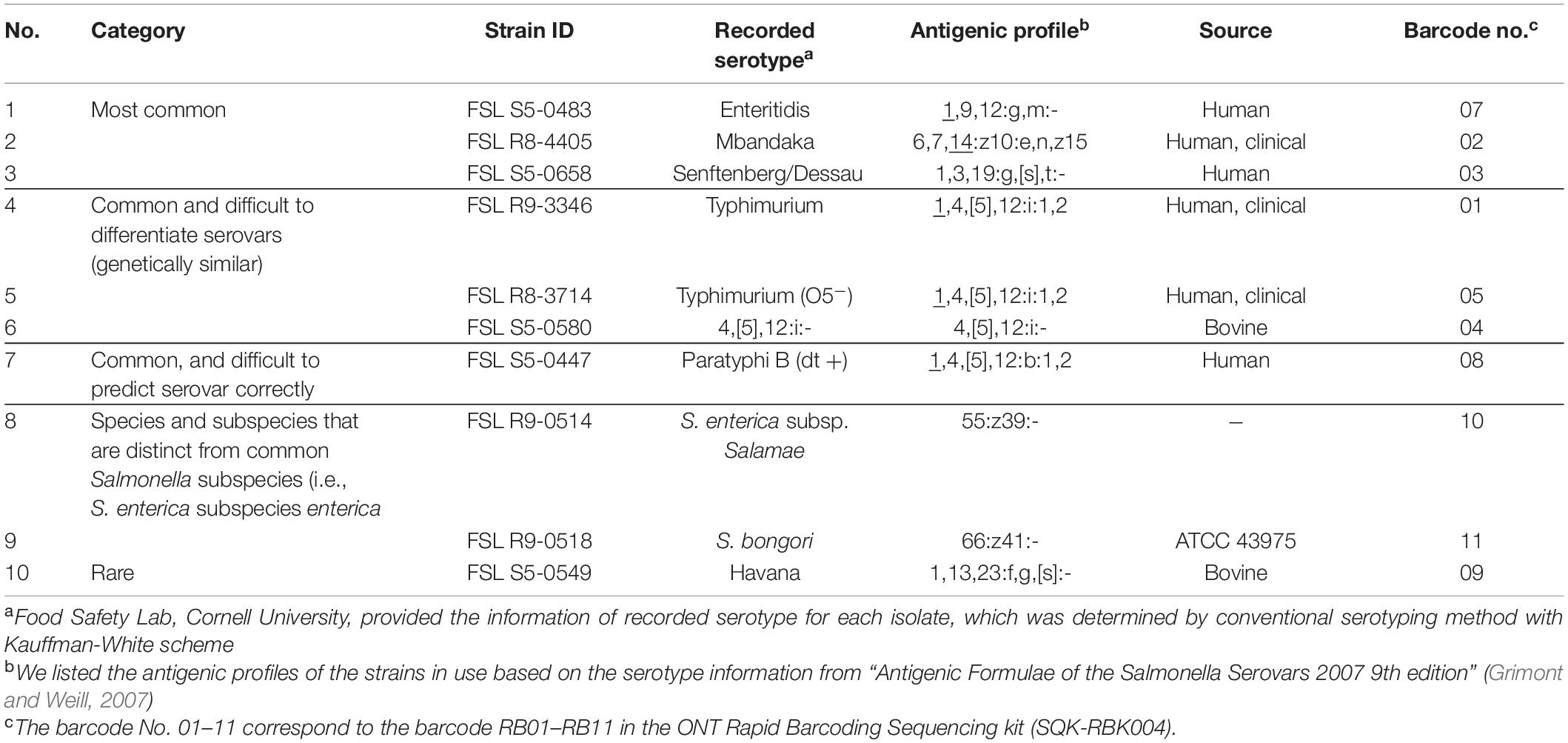
Table 1. Salmonella strains used for evaluating the performance of multiplex ONT sequencing for serotype prediction from whole genome sequencing data.
Genomic DNA Extraction
All Salmonella isolates were cultured on Trypticase Soy Agar at 37°C for 20∼22 h. Genomic DNA was extracted from single colonies using the QIAamp DNA mini kit (Qiagen, Hilden, Germany). The DNA quality and quantity were assessed with the NanoDrop 2000 (Thermo Fisher Scientific, Delaware, United States) for absorbance value (A) and the Qubit 3.0 fluorimeter (Life Technologies, Paisley, United Kingdom) for double strand DNA quantity, based on the guidance for qualification requirements for successful sequencing provided by ONT. The genomic DNA samples that met the following criteria were used for library construction: (i) A 260/280 between 1.8 and 1.9; (ii) A 260/230 between 2.0 and 2.2. The total input DNA was about 400 ng for each flow cell (FC).
Oxford Nanopore Library Preparation and Sequencing
The rapid Barcoding Sequencing kit (SQK-RBK004) was used according to the manufacturer’s instructions. Libraries were multiplexed and sequenced with qualified FLO-MIN106 flow cells (R9.4.1, active pore number ≥800) for 48 h on GridION (Oxford Nanopore Technologies, Oxford, United Kingdom) following the workflow described in Figure 1. Basecalling was performed in real time using Guppy with a basecalling model modified for 6 mA dam/5 mC dcm and CpG, which was integrated in the MinKNOW software v3.5.40 installed on GridION. Ten barcodes were assigned to ten isolates (Table 1). Five multiplexing strategies were tested, including multiplexing three (barcode No. 01 ∼ No. 03), four (barcode No. 01 ∼ No. 04), five (barcode No. 01 ∼ No. 05), seven (barcode No. 01 ∼ No. 05 and No. 07 ∼ No. 08), or ten (barcode No. 01 ∼ No. 05 and No. 07 ∼ No. 11) isolates on one flow cell. Repeats of each multiplexing strategy were tested on five flow cells, which were sequenced simultaneously on one GridION.
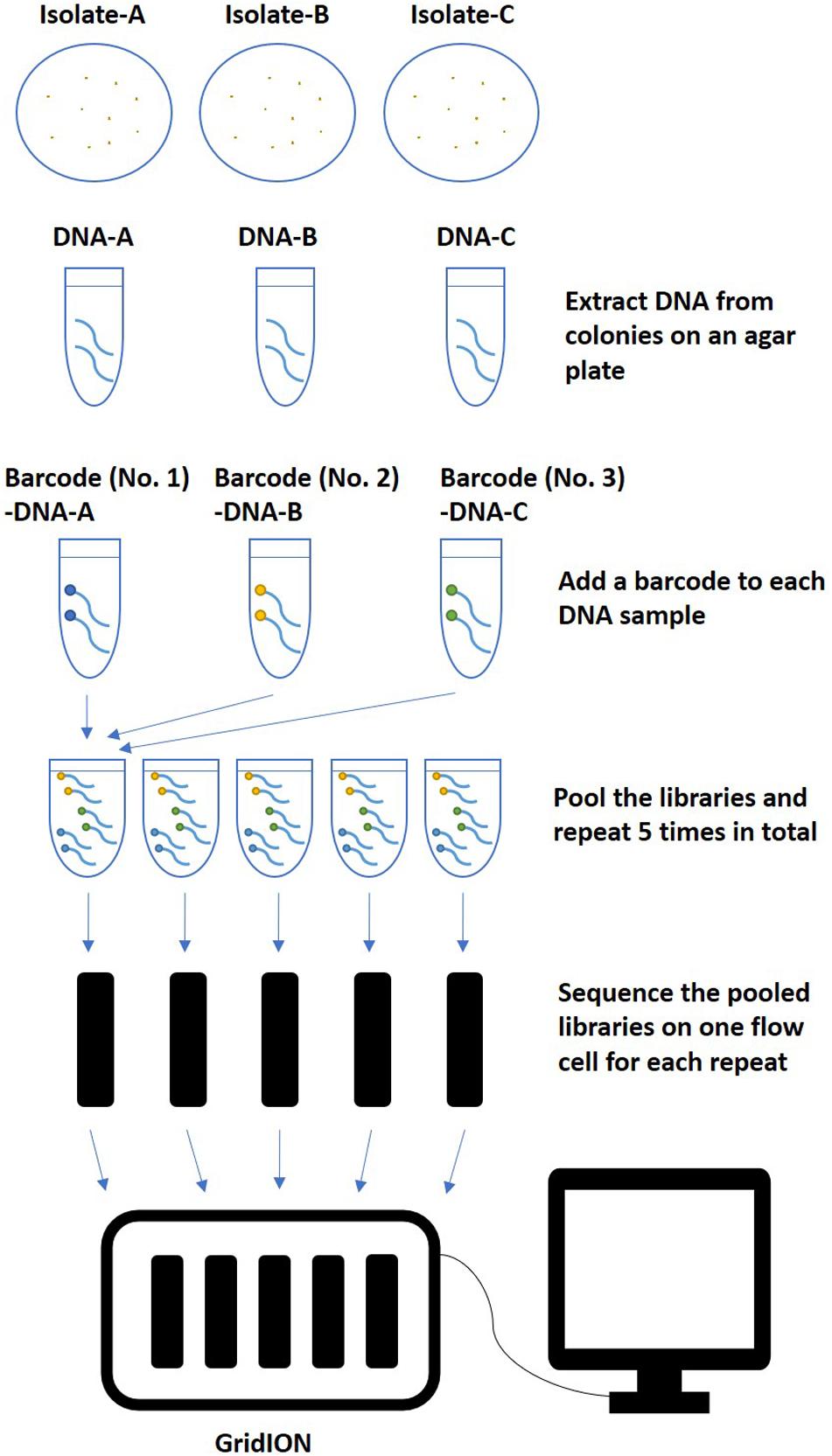
Figure 1. An example of the workflow of multiplex ONT sequencing library preparation and sequencing. After extracted from colonies on an agar plate, Salmonella genome DNA of three isolates were multiplexed and sequenced in one flow cell with five repeats. The same workflow was also used for multiplexing four, five, seven, or ten isolates in one flow cell.
Genomics Analysis and Data Distribution Analysis
Basecalled reads were demultiplexed using qcat v1.1.04, the argument “–min-score” was set to a default value of 60 for general analysis, and various values were manually defined for the purposes of error analysis. Demultiplexed reads were classified based on the barcode qcat identified, reads of each barcode were applied to a modified genomic analysis workflow derived from our previous study (Xu et al., 2020). Porechop v0.2.35 was used to trim adaptor sequences, and the quality of trimmed data was assessed using NanoPlot v1.18.1 (De Coster et al., 2018). A total of approximately 500 Mbp of high-quality long reads (minimum length > 1,000 bp) were selected through Filtlong v0.2.06. Filtered raw reads were subsequently applied to an initial serotype prediction by SeqSero2 v1.0.27 under a preset of arguments specifically designed for ONT raw data. Wtdbg2 v2.4 (Ruan and Li, 2020)8 was then used for initial genome assembly followed by one round of correction through Racon v1.3.3 (Vaser et al., 2017)9.
To assess the influence of barcode and isolate (as one barcode was always assigned to one isolate in this study, we combined these two factors into one as the barcode/isolate factor) on the distribution of data yield among multiplexed isolates in each flow cell, a one-way analysis of variance (ANOVA) was carried out to compare if the proportion of data yield of each barcoded isolate was significantly different from the other barcoded isolates multiplexed in the same flow cell. As the data yield per isolate was diverse within one flow cell, we calculated the coefficient of variation for the proportion of data yield per multiplexed isolate in each flow cell to compare the degree of diversity of data yield per multiplexed isolate between different multiplexing strategies. A one-way ANOVA was carried out to compare if this coefficient of variation for a given multiplexing strategy (e.g., three isolates) was significantly different from the other multiplexing strategies (e.g., four, five, seven, and ten isolates). Statistical significance for the ANOVA was assigned at α = 0.05.
Serotype Prediction Analysis
The nanopore workflow available in SeqSero2 v1.1.2 (see text footnote 3) was used for genome assemblies from ONT reads for serotype prediction analysis. To avoid any possible impact of barcode cross-assignment on serotype prediction, the nanopore workflow of SeqSero2 v1.1.2 was not used on ONT raw reads for the purpose of serotype prediction. Instead, it was used to detect cross-assigned reads. ONT raw reads were also first assembled to correct basecall errors that were reported to compromise SISTR prediction (Zhang et al., 2019) and then used for further serotype prediction using SISTR_cmd (The Salmonella in silico Typing Resource Command-line Tool) v1.1.0 (Yoshida et al., 2016) under a default setting of arguments for assembled data. A brief data analysis pipeline for serotype prediction is shown in Figure 2.
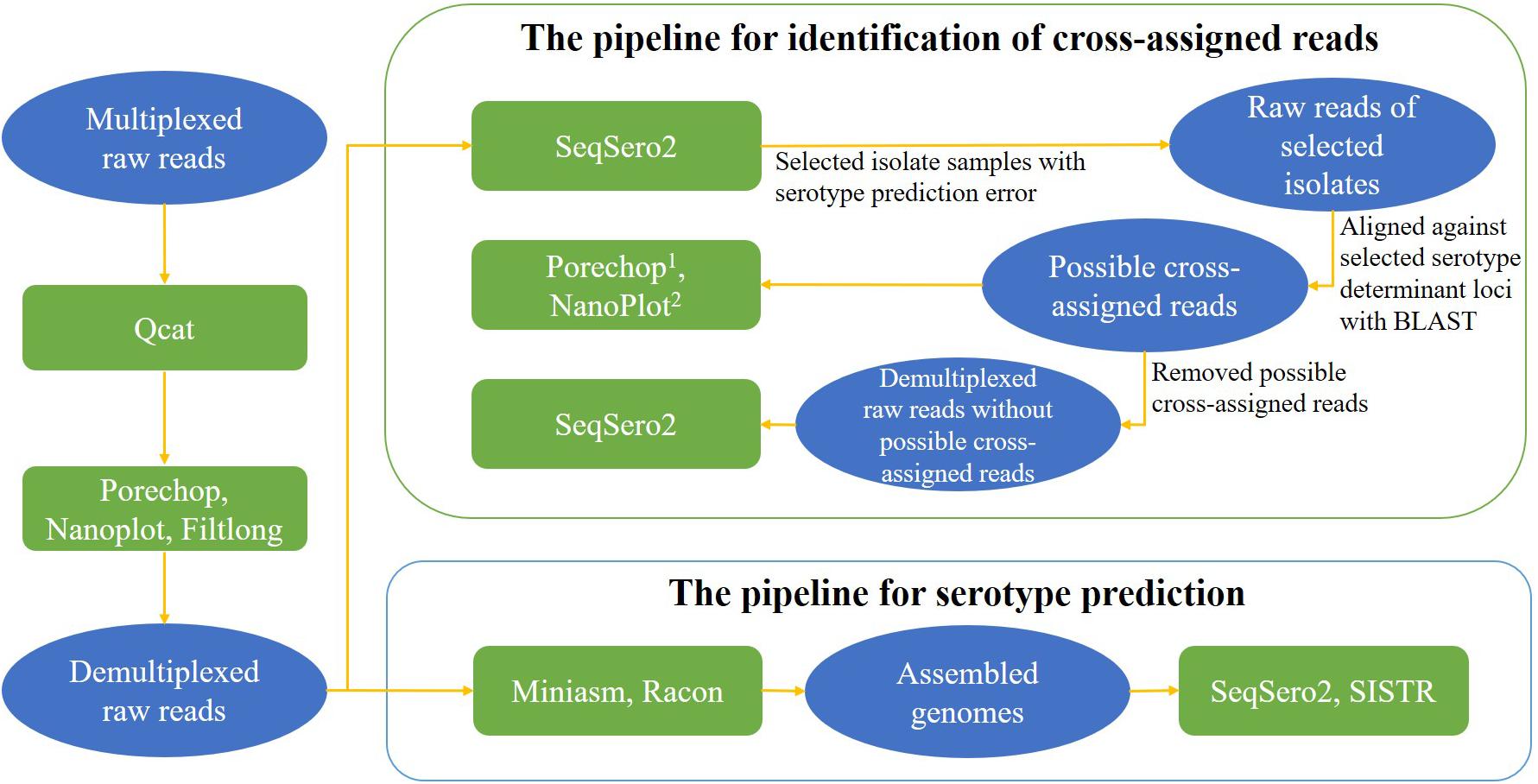
Figure 2. Data analysis pipelines for serotype prediction and identification of cross-assigned reads. Contents in ovals present the type of input data for each analysis, contents in rectangles present the tools for each analysis. 1Porechop was used to search for middle adaptors from the possible cross-assigned reads; 2NanoPlot was used to assess the sequencing quality of the possible cross-assigned reads.
Assessment of the Influence of Sequencing Time and Depth of Genome Coverage in Serotype Prediction
To assess the influence of sequencing depth and sequencing time on the accuracy of serotype prediction, serotype prediction analyses were carried out for each flow cell using different sizes of sequencing data collected as follows. The raw sequencing reads were arranged sequentially by their time of generation. For each flow cell, we first identified the isolate that accounted for the least amount of sequencing data, then we collected reads up to the time point when this isolate obtained the desired depth of genome coverage [average Salmonella genome size: 4.8 Mbp (McClelland et al., 2001; Parkhill et al., 2001)]. We defined this depth of genome coverage as Depthmin for this flow cell. For example, in FC01 (flow cell 01), sequencing data yield for isolates with BC01 (barcode 01), BC02, and BC03 after 48 h of ONT sequencing accounted for 29.36%, 34.95%, and 27.85% of the total sequencing data, respectively. If the desired Depthmin of FC01 for serotype prediction analysis was 15×, we collected sequencing data until the isolate with BC03 reached 15×depth of genome coverage (15 × 4.8 Mbp = 72 Mbp) since BC03 had the least share of sequencing data (27.85%) in this case.
Identification of Possible Cross-Assigned Reads and Influence of Cross-Assigned Reads on the Accuracy of Serotype Prediction
To further investigate if there were cross-assigned reads among multiplexed isolates on each flow cell, ONT raw reads was used as input of SeqSero2 to detect prediction errors caused by single ONT reads. Raw sequencing reads (at 99×depth of genome coverage) of the isolate that was predicted as a different serotype as recorded were aligned against selected serotype determinant loci using BLAST10. These antigen determinant loci were selected from the SeqSero2 antigen outputs that did not match the recorded antigen profile of this isolate. For example, the antigen profile prediction result using raw reads of isolate FSL R9-3346 from sample FC19-BC03 (recorded serotype: Senftenberg) showed its H2 antigen as “e,n,z15,” while serotype Senftenberg did not have an H2 antigen. We therefore included all four alleles of the H2 antigen “e,n,z15” from SeqSero2 database to align against the raw reads of FC19-BC03 for identifying possible cross-assigned reads. We defined a read as a possible cross-assigned read if it showed BLAST identity ≥90% and coverage = 100% to a selected antigen determinant allele.
Porechop v0.2.3 (see text footnote 5) was used to further assess whether there was a barcode in the middle of these reads (we choose a -middle_threshold of 60). Sequencing quality of these putative cross-assigned reads was assessed using NanoPlot v1.18.1 (De Coster et al., 2018). To assess the influence of the possible cross-assigned reads on the accuracy of serotype prediction, serotype prediction analyses with SeqSero2 were performed again after removal of these reads for the aforementioned two isolates. A brief data analysis pipeline for identification of cross-assigned reads is shown in Figure 2.
Assessment of the Influence of Sequencing Quality and Barcode Quality on the Accuracy of Serotype Prediction
To further assess the influence of (i) sequencing quality and (ii) barcode quality on the accuracy of serotype prediction for the two isolates FSL R9-3346 and FSL S5-0658 from the two samples noted in 2.4.2, serotype prediction analyses with SeqSero2 were performed with the raw reads selected with the following settings as input: (i) sequencing quality score ≥12, 13, or 14 (selected by Filtlong v0.2.0), qcat barcode score ≥60 (default setting), and Depthmin = 99×, as well as (ii) sequencing quality ≥7 (default setting), qcat barcode score ≥70, 80, or 90, and Depthmin = 99×.
Results and Discussion
Overview of Nanopore Sequencing Data and Assembly of Salmonella Genomes
The quality of raw sequencing data from the multiplex ONT sequencing experiments was analyzed by NanoPlot (version 1.18.1) (Table 2). An average of 10,629 Mbp of clean sequencing data per flow cell were obtained in 48 h from 25 experiments (across different numbers of multiplexed isolates). Data outputs of flow cells ranged from 5,650 to 17,998 Mbp, with the exception of FC17 which generated only 3,201 Mbp data; the mean read length and read length N50 varied much less (Table 2). Sequencing quality was shown to be highly consistent among 25 experiments, with mean quality scores for a given flow cell ranging from 10.8 to 12.3. The average quality score was 11.7.
Overview of Demultiplexed Sequencing Data
An average of 8.02% (N = 25) reads per flow cell failed to be assigned to any barcode after demultiplexing analysis of the sequencing data generated with 48 h of ONT sequencing; these reads were defined as Non-assigned reads. An average of 0.03% reads per flow cell were assigned to barcodes that were not included in the flow cell; these reads were defined as mis-assigned reads (Figure 3 and Supplementary Table 1). Demultiplexed raw sequencing data of each multiplexed isolate in each flow cell from 48 h ONT sequencing (No. = 145) were submitted to NCBI–SRA (Accession number: PRJNA694442).
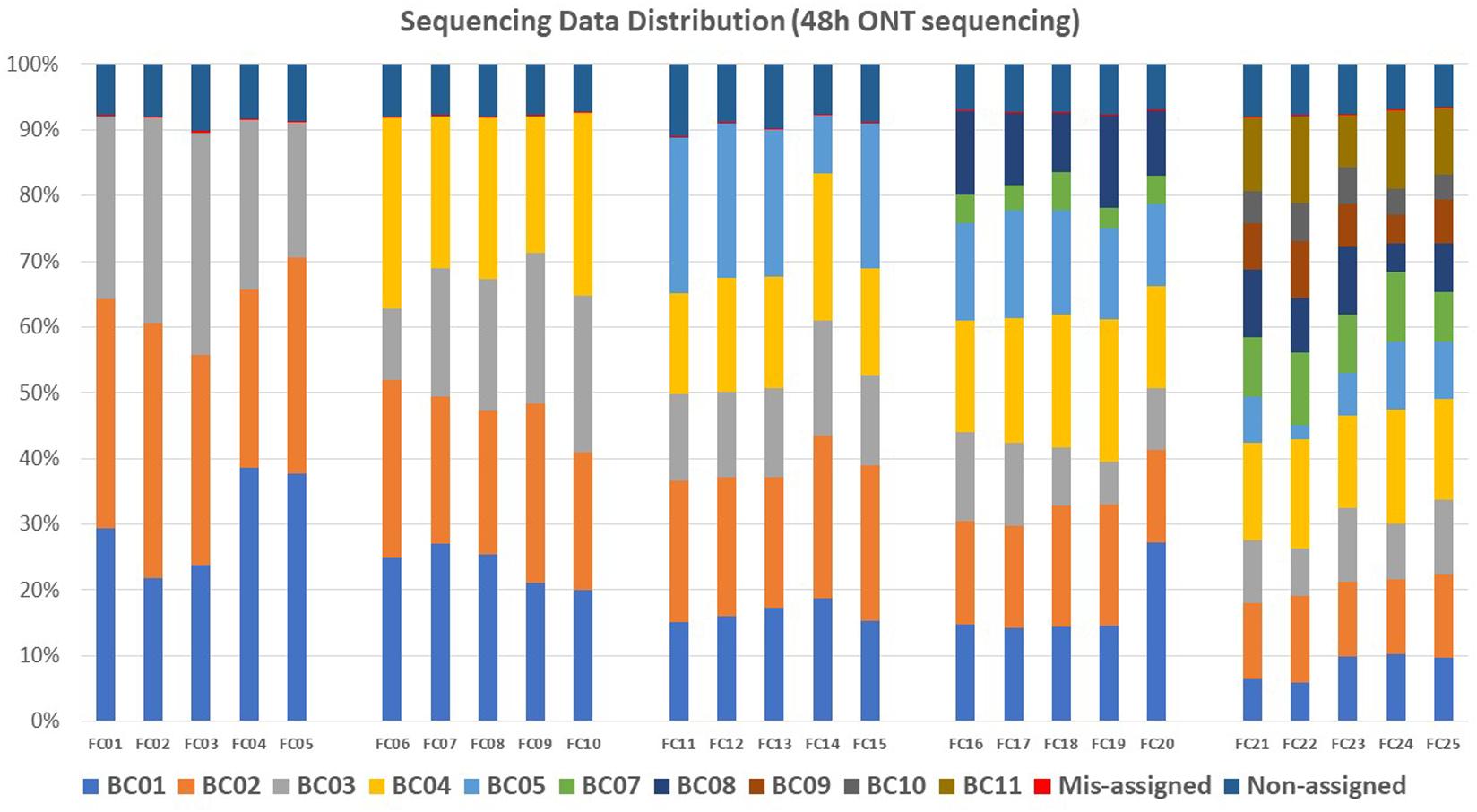
Figure 3. Sequencing data distribution within each flow cell (FC) for each barcode (BC). Each color represents one barcode. Within each flow cell, sequencing reads that were not assigned to any barcode were defined as non-assigned reads, reads assigned to a barcode that was not included in the flow cell were defined as mis-assigned reads.
The unevenness of data yield among multiplexed isolates was a major barrier for shortening the total sequencing time for each flow cell. There was no significant difference among the data yields for different barcoded samples after 48 h of ONT sequencing when multiplexing three or four isolates (Figures 4A,B and Supplementary Table 1). However, ANOVA indicated a significant difference (P < 0.05) in data yields among isolates when multiplexing five, seven, or ten isolates (Figures 4C–E and Supplementary Table 1). For example, post hoc comparisons using the Tukey HSD test indicated that BC03 showed a significantly lower data yield (P < 0.05) than some other isolates when multiplexing five or seven isolates (Figures 4C,D), and BC07 showed a significantly lower data yield (P < 0.05) than some other isolates when multiplexing seven or ten isolates (Figures 4D,E).
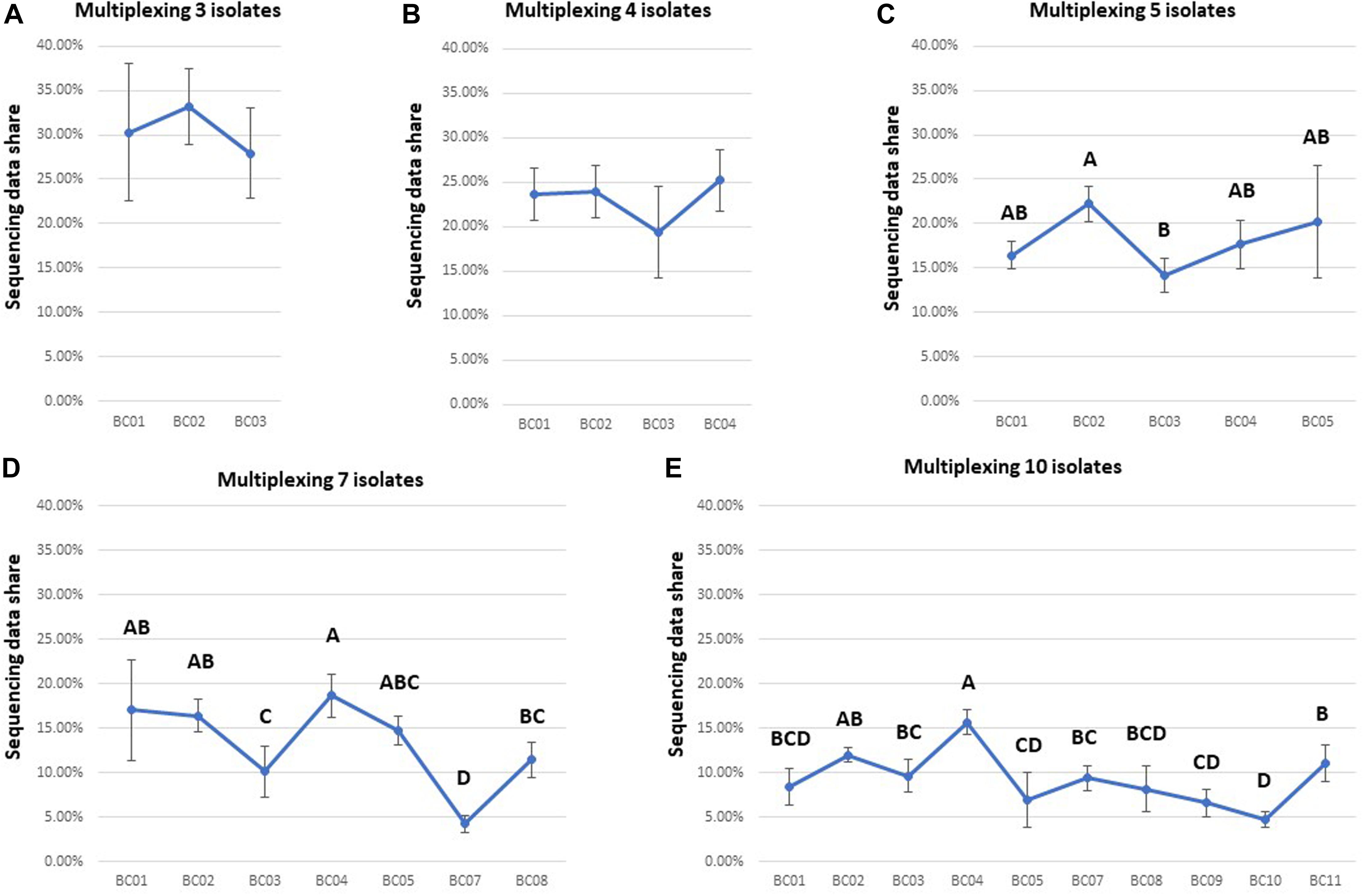
Figure 4. Comparison of the data yield of each isolate multiplexed in one flow cell for each multiplexing strategy (sequencing time: 48 h). Based on the statistical analysis results of Tukey HSD test, barcodes with different letters were significantly different (P-value < 0.05) from each other in data yield. Ideally, data share of each isolate was expected to be 31, 23, 18, 13, and 9% in (A–E).
We also found that that the level of unevenness of data yield of each multiplexed isolate is different among multiplexing strategies (Figure 5). Comparison of the coefficient of variation of the proportion of data yield per multiplexed isolate among multiplexing strategies showed that, the level of isolate-data-yield variation of multiplexing seven or ten isolates is significantly greater (P < 0.05) as compared to multiplexing three, four, or five isolates. For example, on FC01 (three isolates multiplexed), the isolate (BC02) with the highest data yield accounted for 34.95% of total data (at 1,004×depth of genome coverage), which was 1.25 times the data yield of the isolate (BC03) with the smallest data sharing proportion (BC03 with 27.85% data yield at 800 × depth of genome coverage). On the other hand, for FC22 (ten isolates multiplexed), the isolate with the highest data yield (BC04) accounted for 16.72% of the total data (at 337 × depth of genome coverage); thus was 7.92 times the data yield of the isolate with the smallest data sharing proportion (BC05), which accounted for only 2.11% of the total data (at 42 × depth of genome coverage), after 48 h ONT sequencing.
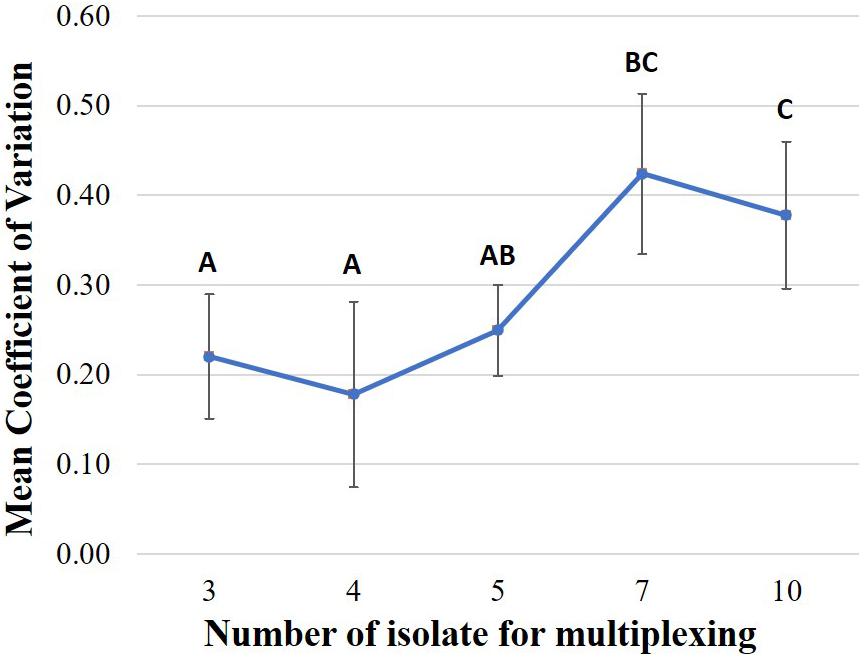
Figure 5. Comparison of the coefficient of variation of the proportion of data yield per multiplexed isolate in one flow cell under each multiplexing strategy including multiplexing three, four, five, seven, or ten isolates (Sequencing time: 48 h). Based on the statistical analysis results of Tukey HSD test, multiplexing strategies with different letters were significantly different (P-value < 0.05) from each other in coefficient of variation.
Influence of Sequencing Time and Depth on Accuracy of Salmonella Serotype Prediction Using ONT Assembled Genomes
For flow cells multiplexing three, four, or five isolates, five levels of Depthmin (15×, 30×, 50×, 75×, 99×) were used to perform serotype prediction analysis. Using genomes assembled from the raw reads with (i) sequencing quality ≥7 and (ii) qcat barcode score ≥60, both SeqSero2 and SISTR generated the same results at the serotype level as the recorded serotype of each isolate for all tested Depthmin levels when multiplexing up to five isolates. The ONT sequencing time for each flow cell at different Depthmin levels are shown below (Table 3).
For flow cells multiplexing seven or ten isolates, none of the flow cells achieved Depthmin = 99 × within 48 h of ONT sequencing. Hence Depthmin levels 15×, 30×, 50×, 75×, and/or the maximum Depthmin of each flow cell were used to perform serotype prediction analysis. One flow cell multiplexing seven isolates (FC17) generated only 3.20 Gbp trimmed data after 48 h of sequencing, which was 70% lower than the average total sequencing data; this flow cell was not included in the serotype prediction analyses. When multiplexing seven isolates, earliest accurate serotype predictions for tested isolates were obtained using sequencing data at Depthmin = 15×. When multiplexing ten isolates, SISTR identified isolate FSL S5-0483 (recorded serotype and antigen profile: Enteritidis, 1,9,12:g,m:-) as Gallinarum | Pullorum (predicted antigen profile: 1,9,12:-:-) in one (FC24) of the five replicates/flow cells, while SeqSero2 reported the correct serotype (with a depth of genome coverage of 41 × for this isolate in FC24). Earliest accurate serotype predictions for tested isolates were obtained using sequencing data at Depthmin = 15 × with SeqSero2 alone, and at Depthmin = 30 × with both SeqSero2 and SISTR.
While molecular serotyping does not typically identify serotype variants caused by ancillary O antigens, Typhimurium var. O5- (FSL R8-3714) was consistently (across flow cells) correctly identified by SeqSero2 [as SeqSero2 targets a mutation that causes the O5- phenotype (Hauser et al., 2011)]; SISTR, on the other hand, identified FSL R8-3714 as Typhimurium, without specifying the variant O5-.
In summary, for all multiplexing strategies tested in the current study, (i) with SeqSero2 alone, the earliest accurate serotype predictions were obtained using genomes assembled from sequence data at Depthmin 15 × as input, generated from 1.02 to 5.47 h of ONT sequencing for multiplexing three to ten isolates; (ii) with SISTR alone, the earliest accurate serotype predictions were obtained using genomes assembled from sequence data Depthmin at 30 × as input, generated from 1.85 to 11.87 h for multiplexing three to ten isolates (Table 3); (iii) both SISTR and SeqSero2 correctly predicted Salmonella serotypes from genomes assembled using multiplexed ONT raw data with 50 × genome coverage (please note that this is an actual coverage of a given isolate, not a Depthmin indicating the lowest genome coverage that each one of the multiplexed isolates can reach on one flow cell).
Higher quality of the assembled genome was shown to be associated with higher sequencing depth for both the WGS and ONT platforms in our previous study (Xu et al., 2020), while here we demonstrated a genome coverage of 50× is generally sufficient for assembling genomes to support accurate serotyping. An average sequencing duration of approximately 6 h for multiplexing five isolates was sufficient to reach this genome coverage. The majority of previous evaluations of WGS-based Salmonella serotype prediction used Illumina data (Zhang et al., 2015, 2019; Yachison et al., 2017; Uelze et al., 2020), since Illumina platforms can generally yield high quality sequencing data. Currently, a minimum of 30 × genome coverage (≥10 kb reads) of ONT sequencing data is recommended for bacteria assembly11.
Multiplexing several isolates inevitably caused variations in size of data allocation for each isolate, unlike sequencing a single isolate on a single flow cell. It is thus important to manage multiplex sequencing to allow each isolate to reach at least the genome coverage of 50 × for reliable serotype prediction, hence sequencing duration for multiplex runs will necessarily be longer than the sequencing duration required for a single isolate. Our results from pooling 3–10 isolates demonstrated that the unevenness of data yield among multiplexed isolates increased significantly and sequencing times required for accurate serotyping exceeded 19 h when multiplexing more than five isolates. Consequently, multiplexing more than five isolates may potentially undermine the benefit of the relatively short time required for ONT sequencing. Based on these observations, we conclude that multiplexing five isolates represents the optimum for obtaining the sequencing depth required for reliable serotype prediction within a reasonable time frame.
Identification of Possible Cross-Assigned Reads and Their Influence on the Accuracy of Serotype Prediction
One and two possible cross-assigned reads were identified for samples FC10-BC01 (isolate FSL R9-3346; serotype: Typhimurium) and FC19-BC03 (isolate FSL S5-0658; serotype: Senftenberg), respectively (Supplementary Table 2). Please note that the analysis method we used could only identify possible cross-assigned reads leading to serotype prediction errors. Therefore, it was highly possible that the cross-assigned reads we identified were just a small fraction of all types of existing cross-assigned reads. The mean read length and sequencing quality of these reads were 10.96 Kbp and 12.93 Kbp, respectively. We did not detect any middle adaptors among these reads. These possible cross-assigned reads each had a barcode score ≥95.8 at one end of the read (Supplementary Table 2), and < 90 at the other end, as determined by Porechop (data not shown), consistent with the fact that the ONT library preparation kit added barcode adaptors to only one end of each read. Serotype predictions with raw reads by SeqSero2 after removal of these possible cross-assigned reads, were consistent with recorded serotypes of these samples, suggesting that these reads were the cause of serotype prediction errors for these two isolates. In addition, we did not identify any possible cross-assigned reads with our screening criteria from the corresponding isolates on the other flow cells multiplexing the same number of isolates but showing correct serotype predictions. As the barcode score and quality score of these detected cross-assigned reads were quite high and as no chimeric reads were detected (no evidence of middle adaptor), we speculated that one of the major causes of barcode cross-assignment was contamination from free adapters after pooling the libraries. These reads possibly captured wrong barcodes after multiplexing during library preparation. We did not perform cleaning-up to remove short sequences (< 100 bp) after pooling, hence these free barcodes might have had opportunities to link to the DNA sequences from multiple isolates. Tyler et al. (2018) also found erroneously barcoded reads that standard filtering practices could not remove as they were of high quality, and suggested running only a single sample at a time on a flow cell to avoid contaminating reads. It has been reported that clean-up by a bead-based or gel purification step could remove free adapters12, therefore a clean-up step after pooling the indexed libraries might be added in future studies. This may help alleviate index misassignment.
This study showed that cross-assigned reads might cause serotyping errors only when raw reads were directly used for serotype prediction. However, such errors may be avoided by assembling genomes prior to prediction. Barcode misassignment (including cross-assignment also known as index hopping) between multiplexed libraries is a recognized cause of misidentification (Kircher et al., 2012) for NGS. The Illumina sequencing technology has been reported to typically generate 0.1–2% barcode misassignment (see text footnote 12). Similarly, ONT sequencing has been reported to generate 0.02–0.3% barcode switching or misassignment to an unused barcode when using the 1D ligation sequencing kit (SQK-LSK108) and the native barcoding expansion kit (EXP-NBD103) (Tyler et al., 2018; Wick et al., 2018; Xu et al., 2018). In the current study, using the Rapid Barcoding Sequencing kit (SQK-RBK004), the index misassignment level was around 0.04% of the total sequencing data size (data not shown). Barcode misassignment levels for multiplex sequencing have shown to be highly dependent on the library preparation kit used, as well as quality and handling of the library (see text footnote 12). As the mean read length of the 25 ONT flow cells tested in the current study was above 8 Kbp (Table 2) and the length of an antigen determent loci in Salmonella is usually between 0.1 and 5 Kbp (data not shown), a small number of ONT cross-assigned reads can possibly alter the serotype prediction result. High molecular weight DNA extraction methods are available for purification of DNA in the 50 Kbp to 1 Mbp + size range. Reads with greater mean length may lead to higher quality of genome assembly, yet their influence on the possible cross-assigned reads and accuracy of serotype prediction are unknown. With DNA extraction method generating regular read length of genome DNA (mean read length around 8 Kbp), we recommend using assembled genomes for serotype prediction through SeqSero2 with multiplex ONT sequencing data, if more rapid serotype prediction enabled by raw reads input [∼5 s/million bases using SeqSero2 nanopore workflow (Zhang et al., 2019)] is not the primary concern.
Influence of Sequencing Quality and Barcode Quality on the Accuracy of Serotype Prediction
Accurate serotype prediction of the two isolates noted above (FSL R9-3346, FC10-BC01, serotype Typhimurium, and isolate FSL S5-0658, FC19-BC03, serotype Senftenberg) was obtained when sequencing quality score was raised to ≥13 and qcat barcode score was set at ≥60 (default setting) using ONT raw reads as input of SeqSero2. However, this approach removed about 60% of the raw sequence data. Setting the quality score to ≥13 may have removed most of the error-causing reads from the data set, as the average sequencing quality score of the possible cross-assigned reads was 12.93. When the sequencing quality score was set to ≥14, more than 90% of the raw sequence data were lost. Consequently, the depth of genome coverage of some of the other isolates multiplexed in the same flow cell dropped to below 10×, which led to serotype prediction errors for these isolates due to low genome coverage.
When sequencing quality score was set at 7 (default setting) for filtering raw reads, and qcat barcode score was raised to ≥90 at the same time, accurate serotype prediction was obtained for these two samples by using raw reads with SeqSero2. However, these settings still resulted in loss of more than 90% of the raw sequence data, and reduction of the depth of genome coverage of some of the other isolates in the same flow cell to below 10×, again leading to serotype prediction errors for these isolates.
In summary, raising the quality score of raw reads to ≥13 improved serotype prediction accuracy for the isolates tested in the current study, while removing more than half of the total sequencing data. On the other hand, raising the barcode score of raw sequencing reads did improve prediction accuracy, but resulted in exclusion of 90% of the raw sequencing data, thus introducing errors due to lack of sequencing depth for some of the isolates multiplexed in the same flow cell. This approach could be used to avoid errors caused by cross-assigned reads when sequencing data depth permits, or where sequencing time length is not the primary concern. Xu et al. (2018) found that chimeric reads and low-barcode-score reads were the main causes of cross sample contamination in their data set. In contrast, we found the error-causing reads were of high barcode quality and without evidence of having internal adaptors. Removing all the reads with middle adaptors from the raw sequencing data did not alter the prediction results generated by using ONT raw reads (data not shown). This discrepancy in the cause of cross contamination may be attributed to the difference in library preparation and barcoding kits used in the current study and Xu’s study (Xu et al., 2018).
Recommendation for Cost-Effective Multiplexing Strategy and Limitations of the Current Study
Multiplexing has the advantage of higher time and cost efficiencies compared to sequencing single isolates, particularly in a practical scenario where large numbers of samples are routinely analyzed. The sequencing kit (RBK004) used in this study has the capability of multiplexing up to 12 different isolates in one flow cell, which will yield considerable costs savings relative to sequencing a single isolate on a flow cell. Multiplexing three isolates could reduce consumable cost per isolate by 64% compared to the cost of sequencing a single isolate, and the cost reduction can be increased to 87% when ten isolates are multiplexed. However, the total sequencing time needs to be increased above the 1∼2 h required for single isolate sequencing, s so that sufficient data are obtained for each multiplexed isolate to allow for correct serotype prediction. Also, the unevenness of data yield between each multiplexed isolate increases as the number of isolates being multiplexed increases. The total number of multiplexed isolates, therefore, should be carefully considered. In this study 50 × depth of genome coverage (∼ 5 Gbp of raw data) could be obtained within an average of 6.0 h of ONT sequencing when multiplexing five isolates, while multiplexing seven isolates took an average of 19.5 h to reach equivalent depth (Table 4). Hence multiplexing seven to ten isolates resulted in only a small cost benefit compared with multiplexing five isolates (Table 4). Moreover, our previous study showed that both the data yield and quality started to decline after 12 h of sequencing on the flow cells of GridION, and the flow cells usually showed remarkably low numbers of active pores after 48 h of sequencing (Xu et al., 2020). These data suggest that multiplexing five isolates is likely to be more efficient overall, than multiplexing greater numbers of isolates. Total turnaround time is of critical importance in the practical application of serotype prediction in the food industry; multiplexing five isolates will allow serotyping results to be obtained within one day. Increasing the number of isolates being multiplexed resulted in increased sequencing time, and more total time for DNA extraction, library construction and data analysis (Table 4).
It is not known if certain strains of Salmonella would alter the accuracy of serotyping under the recommended settings, as only Ten serotypes were involved in the current study. Further validation with more Salmonella serotypes is necessary to operationalize the serotyping of Salmonella through multiplex ONT WGS, for example in the food industry or in public health.
Conclusion
This study demonstrated that accurate serotype prediction results could be obtained when multiplexing five or less Salmonella isolates with an average of 6 h of multiplex ONT sequencing, where each multiplexed isolate received at least 50 × depth of genome coverage of sequencing data after demultiplexing. Multiplexing up to five isolates in one flow cell is recommended to achieve high coverage of the genome and high accuracy of prediction within one day. Multiplexing five isolates results in a cost reduction to 23% of the cost of ONT sequencing of a single isolate per flow cell. Our study helps to identify the optimal combinations of isolate multiplexing number and sequencing time to achieve the most accurate, rapid, and cost-efficient Salmonella serotype prediction with multiplex ONT sequencing. This study also sets a starting point for the future validation of multiplex ONT WGS as a cost-efficient, rapid Salmonella confirmation, and serotype classification tool for the food industry.
Data Availability Statement
The datasets presented in this study can be found in online repositories. The names of the repository/repositories and accession number(s) can be found below: https://www.ncbi.nlm.nih.gov/, PRJNA694442.
Author Contributions
ST and CG: conception and design of the work. XW, ST, and HL: data collection, data analysis, and drafting the article. CG, FX, GZ, SL, XD, MW, AS, and RB: critical revision of the article. All authors contributed to the article and approved the submitted version.
Funding
We self-funded by the Mars Global Food Safety Center, a non-competitive and non-profit research institute. The funders declare that the research was conducted in the absence of any commercial or financial relationships that could be construed as a potential conflict of interest.
Conflict of Interest
The authors declare that the research was conducted in the absence of any commercial or financial relationships that could be construed as a potential conflict of interest.
Acknowledgments
We thank Peter Markwell and Dr. Bala Ganesan for comments that greatly improved the manuscript. We also would like to thank Oxford Nanopore Technologies for supporting the establishment of ONT capability at the Mars Global Food Safety Center.
Supplementary Material
The Supplementary Material for this article can be found online at: https://www.frontiersin.org/articles/10.3389/fmicb.2021.637771/full#supplementary-material
Footnotes
- ^ https://www.illumina.com/systems/sequencing-platforms.html
- ^ https://nanoporetech.com/products
- ^ https://github.com/denglab/SeqSero2
- ^ https://github.com/nanoporetech/qcat
- ^ https://github.com/rrwick/Porechop
- ^ https://github.com/rrwick/Filtlong
- ^ https://github.com/denglab/SeqSero2/releases
- ^ https://github.com/ruanjue/wtdbg2/releases/tag/v2.4
- ^ https://github.com/isovic/racon/releases
- ^ https://blast.ncbi.nlm.nih.gov/Blast.cgi
- ^ https://nanoporetech.com/sites/default/files/s3/literature/microbial-genome-assembly-workflow.pdf
- ^ https://www.illumina.com/science/education/minimizing-index-hopping.html
References
Allard, M. W., Strain, E., Melka, D., Bunning, K., Musser, S. M., Brown, E. W., et al. (2016). Practical value of food pathogen traceability through building a whole-genome sequencing network and database. J. Clin. Microbiol. 54, 1975–1983. doi: 10.1128/JCM.00081-16
Ashton, P. M., Nair, S., Peters, T. M., Bale, J. A., Powell, D. G., Painset, A., et al. (2016). Identification of Salmonella for public health surveillance using whole genome sequencing. PeerJ 4:e1752. doi: 10.7717/peerj.1752
Bailey, J. S., Fedorka-Cray, P. J., Stern, N. J., Craven, S. E., Cox, N. A., and Cosby, D. E. (2002). Serotyping and ribotyping of Salmonella using restriction enzyme PvuII. J. Food Prot. 65, 1005–1007. doi: 10.4315/0362-028x-65.6.1005
Banerji, S., Simon, S., Tille, A., Fruth, A., and Flieger, A. (2020). Genome-based Salmonella serotyping as the new gold standard. Sci. Rep. 10:4333. doi: 10.1038/s41598-020-61254-1
CDC (2006). Salmonella Surveillance: Annual Summary, 2006. Atlanta, GA: U.S. Department of Health and Human Services, Centers for Disease Control and Prevention.
CDC (2018). National Enteric Disease Surveillance — Salmonella Annual Report, 2016. Atlanta, GA: US Centers for Disease Control and Prevention (CDC).
Cooper, A. L., Low, A. J., Koziol, A. G., Thomas, M. C., Leclair, D., Tamber, S., et al. (2020). Systematic evaluation of whole genome sequence-based predictions of Salmonella serotype and antimicrobial resistance. Front. Microbiol. 11:549. doi: 10.3389/fmicb.2020.00549
De Coster, W., D’Hert, S., Schultz, D. T., Cruts, M., and Van Broeckhoven, C. (2018). NanoPack: visualizing and processing long-read sequencing data. Bioinformatics 34, 2666–2669. doi: 10.1093/bioinformatics/bty149
Didelot, X., Walker, A. S., Peto, T. E., Crook, D. W., and Wilson, D. J. (2016). Within-host evolution of bacterial pathogens. Nat. Rev. Microbiol. 14, 150–162. doi: 10.1038/nrmicro.2015.13
Diep, B., Barretto, C., Portmann, A. C., Fournier, C., Karczmarek, A., Voets, G., et al. (2019). Salmonella serotyping; comparison of the traditional method to a microarray-based method and an in silico platform using whole genome sequencing data. Front. Microbiol. 10:2554. doi: 10.3389/fmicb.2019.02554
EFSA, and ECDC (2019a). The European Union One Health 2018 zoonoses report. EFSA. J. 17:e05926. doi: 10.2903/j.efsa.2019.5926
EFSA, and ECDC (2019b). The European Union summary report on antimicrobial resistance in zoonotic and indicator bacteria from humans, animals and food in 2017. EFSA. J. 17:e05598. doi: 10.2903/j.efsa.2019.5598
EFSA Panel on Biological Hazards, Koutsoumanis, K., Allende, A., Alvarez-Ordóñez, A., Bolton, D., Bover-Cid, S., et al. (2019). Whole genome sequencing and metagenomics for outbreak investigation, source attribution and risk assessment of food-borne microorganisms. EFSA. J. 17:e05898. doi: 10.2903/j.efsa.2019.5898
Foley, S. L., Zhao, S., and Walker, R. D. (2007). Comparison of molecular typing methods for the differentiation of Salmonella foodborne pathogens. Foodborne Pathog. Dis. 4, 253–276. doi: 10.1089/fpd.2007.0085
Fox, E. J., Reid-Bayliss, K. S., Emond, M. J., and Loeb, L. A. (2014). Accuracy of next generation sequencing platforms. Next Gener. Seq. Appl. 1:1000106. doi: 10.4172/jngsa.1000106
Galanis, E., Lo Fo Wong, D. M., Patrick, M. E., Binsztein, N., Cieslik, A., Chalermchikit, T., et al. (2006). Web-based surveillance and global Salmonella distribution, 2000-2002. Emerg. Infect. Dis. 12, 381–388. doi: 10.3201/eid1205.050854
GMA (2009). Control of Salmonella in Low-Moisture Foods. Rosslyn, VA: The Association of Food, Beverage and Consumer Products Companies (GMA).
Grimont, P., and Weill, F. (2007). Antigenic Formulae of the Salmonella Serovars, 9th Edn. Paris: WHO Collaborating Centre for Reference and Research on Salmonella.
Hauser, E., Tietze, E., Helmuth, R., and Malorny, B. (2011). Different mutations in the oafA gene lead to loss of O5-antigen expression in Salmonella enterica serovar typhimurium. J. Appl. Microbiol. 110, 248–253. doi: 10.1111/j.1365-2672.2010.04877.x
Herikstad, H., Motarjemi, Y., and Tauxe, R. V. (2002). Salmonella surveillance: a global survey of public health serotyping. Epidemiol. Infect. 129, 1–8. doi: 10.1017/s0950268802006842
Herrera-León, S., Ramiro, R., Arroyo, M., Díez, R., Usera, M. A., and Echeita, M. A. (2007). Blind comparison of traditional serotyping with three multiplex PCRs for the identification of Salmonella serotypes. Res. Microbiol. 158, 122–127. doi: 10.1016/j.resmic.2006.09.009
Imai, K., Nemoto, R., Kodana, M., Tarumoto, N., Sakai, J., Kawamura, T., et al. (2020). Rapid and accurate species identification of Mitis group Streptococci using the MinION nanopore sequencer. Front. Cell. Infect. Microbiol. 10:11. doi: 10.3389/fcimb.2020.00011
Issenhuth-Jeanjean, S., Roggentin, P., Mikoleit, M., Guibourdenche, M., de Pinna, E., Nair, S., et al. (2014). Supplement 2008-2010 (no. 48) to the White-Kauffmann-Le Minor scheme. Res. Microbiol. 165, 526–530. doi: 10.1016/j.resmic.2014.07.004
Karamitros, T., and Magiorkinis, G. (2018). Multiplexed targeted sequencing for Oxford Nanopore MinION: a detailed library preparation procedure. Methods Mol. Biol. 1712, 43–51. doi: 10.1007/978-1-4939-7514-3_4
Kennedy, S. R., Prost, S., Overcast, I., Rominger, A. J., Gillespie, R. G., and Krehenwinkel, H. (2020). High-throughput sequencing for community analysis: the promise of DNA barcoding to uncover diversity, relatedness, abundances and interactions in spider communities. Dev. Genes Evol. 230, 185–201. doi: 10.1007/s00427-020-00652-x
Kircher, M., Sawyer, S., and Meyer, M. (2012). Double indexing overcomes inaccuracies in multiplex sequencing on the Illumina platform. Nucleic Acids Res. 40:e3. doi: 10.1093/nar/gkr771
Liou, C. H., Wu, H. C., Liao, Y. C., Yang Lauderdale, T. L., Huang, I. W., and Chen, F. J. (2020). nanoMLST: accurate multilocus sequence typing using Oxford Nanopore Technologies MinION with a dual-barcode approach to multiplex large numbers of samples. Microb. Genom. 6:e000336. doi: 10.1099/mgen.0.000336
McClelland, M., Sanderson, K. E., Spieth, J., Clifton, S. W., Latreille, P., Courtney, L., et al. (2001). Complete genome sequence of Salmonella enterica serovar Typhimurium LT2. Nature 413, 852–856. doi: 10.1038/35101614
McQuiston, J. R., Waters, R. J., Dinsmore, B. A., Mikoleit, M. L., and Fields, P. I. (2011). Molecular determination of H antigens of Salmonella by use of a microsphere-based liquid array. J. Clin. Microbiol. 49, 565–573. doi: 10.1128/JCM.01323-10
Oh, J. H., and Park, M. K. (2017). Recent trends in Salmonella outbreaks and emerging technology for biocontrol of Salmonella using phages in foods: a review. J. Microbiol. Biotechnol. 27, 2075–2088. doi: 10.4014/jmb.1710.10049
Parkhill, J., Dougan, G., James, K. D., Thomson, N. R., Pickard, D., Wain, J., et al. (2001). Complete genome sequence of a multiple drug resistant Salmonella enterica serovar Typhi CT18. Nature 413, 848–852. doi: 10.1038/35101607
Piper, A. M., Batovska, J., Cogan, N. O. I., Weiss, J., Cunningham, J. P., Rodoni, B. C., et al. (2019). Prospects and challenges of implementing DNA metabarcoding for high-throughput insect surveillance. Gigascience 8:giz092. doi: 10.1093/gigascience/giz092
Rang, F. J., Kloosterman, W. P., and de Ridder, J. (2018). From squiggle to basepair: computational approaches for improving nanopore sequencing read accuracy. Genome Biol. 19:90. doi: 10.1186/s13059-018-1462-9
Ranieri, M. L., Shi, C., Moreno Switt, A. I., den Bakker, H. C., and Wiedmann, M. (2013). Comparison of typing methods with a new procedure based on sequence characterization for Salmonella serovar prediction. J. Clin. Microbiol. 51, 1786–1797. doi: 10.1128/JCM.03201-12
Ruan, J., and Li, H. (2020). Fast and accurate long-read assembly with wtdbg2. Nat. Methods 17, 155–158. doi: 10.1038/s41592-019-0669-3
Scallan, E., Hoekstra, R. M., Angulo, F. J., Tauxe, R. V., Widdowson, M. A., Roy, S. L., et al. (2011). Foodborne illness acquired in the United States–major pathogens. Emerg. Infect. Dis. 17, 7–15. doi: 10.3201/eid1701.p11101
Shi, C., Singh, P., Ranieri, M. L., Wiedmann, M., and Moreno Switt, A. I. (2015). Molecular methods for serovar determination of Salmonella. Crit. Rev. Microbiol. 41, 309–325. doi: 10.3109/1040841X.2013.837862
Soyer, Y., Alcaine, S. D., Schoonmaker-Bopp, D. J., Root, T. P., Warnick, L. D., McDonough, P. L., et al. (2010). Pulsed-field gel electrophoresis diversity of human and bovine clinical Salmonella isolates. Foodborne Pathog. Dis. 7, 707–717. doi: 10.1089/fpd.2009.0424
Tack, D. M., Ray, L., and Griffin, P. M. (2020). Preliminary incidence and trends of infections with pathogens transmitted commonly through food — foodborne diseases active surveillance network, 10 U.S. sites, 2016–2019. MMWR Morb. Mortal. Wkly. Rep. 69, 509–514. doi: 10.15585/mmwr.mm6917a1
Tyler, A. D., Mataseje, L., Urfano, C. J., Schmidt, L., Antonation, K. S., Mulvey, M. R., et al. (2018). Evaluation of Oxford Nanopore’s MinION sequencing device for microbial whole genome sequencing applications. Sci. Rep. 8:10931. doi: 10.1038/s41598-018-29334-5
Uelze, L., Borowiak, M., Deneke, C., Szabó, I., Fischer, J., Tausch, S. H., et al. (2020). Performance and accuracy of four open-source tools for in silico serotyping of Salmonella spp. based on whole-genome short-read sequencing data. Appl. Environ. Microbiol. 86:e02265-19. doi: 10.1128/AEM.02265-19
Vaser, R., Soviæ, I., Nagarajan, N., and Šikiæ, M. (2017). Fast and accurate de novo genome assembly from long uncorrected reads. Genome Res. 27, 737–746. doi: 10.1101/gr.214270.116
Wattiau, P., Boland, C., and Bertrand, S. (2011). Methodologies for Salmonella enterica subsp. enterica subtyping: gold standards and alternatives. Appl. Environ. Microbiol. 77, 7877–7885. doi: 10.1128/AEM.05527-11
Wick, R. R., Judd, L. M., and Holt, K. E. (2018). Deepbinner: demultiplexing barcoded Oxford Nanopore reads with deep convolutional neural networks. PLoS Comput. Biol. 14:e1006583. doi: 10.1371/journal.pcbi.1006583
Xu, F., Ge, C., Luo, H., Li, S., Wiedmann, M., Deng, X., et al. (2020). Evaluation of real-time nanopore sequencing for Salmonella serotype prediction. Food Microbiol. 89:103452. doi: 10.1016/j.fm.2020.103452
Xu, Y., Lewandowski, K., Lumley, S., Pullan, S., Vipond, R., Carroll, M., et al. (2018). Detection of viral pathogens with multiplex Nanopore MinION sequencing: be careful with cross-talk. Front. Microbiol. 9:2225. doi: 10.3389/fmicb.2018.02225
Yachison, C. A., Yoshida, C., Robertson, J., Nash, J. H. E., Kruczkiewicz, P., Taboada, E. N., et al. (2017). The validation and implications of using whole genome sequencing as a replacement for traditional serotyping for a national Salmonella reference laboratory. Front. Microbiol. 8:1044. doi: 10.3389/fmicb.2017.01044
Yoshida, C., Gurnik, S., Ahmad, A., Blimkie, T., Murphy, S. A., Kropinski, A. M., et al. (2016). Evaluation of molecular methods for identification of Salmonella serovars. J. Clin. Microbiol. 54, 1992–1998. doi: 10.1128/JCM.00262-16
Zhang, S., den Bakker, H. C., Li, S., Chen, J., Dinsmore, B. A., Lane, C., et al. (2019). SeqSero2: rapid and improved Salmonella serotype determination using whole-genome sequencing data. Appl. Environ. Microbiol. 85, e01746–19.
Zhang, S., Yin, Y., Jones, M. B., Zhang, Z., Deatherage Kaiser, B. L., Dinsmore, B. A., et al. (2015). Salmonella serotype determination utilizing high-throughput genome sequencing data. J. Clin. Microbiol. 53, 1685–1692. doi: 10.1128/JCM.00323-15
Keywords: whole genome sequencing, Oxford Nanopore Technologies, multiplex, Salmonella, subtyping, foodborne pathogens, serotyping, food industry
Citation: Wu X, Luo H, Xu F, Ge C, Li S, Deng X, Wiedmann M, Baker RC, Stevenson A, Zhang G and Tang S (2021) Evaluation of Salmonella Serotype Prediction With Multiplex Nanopore Sequencing. Front. Microbiol. 12:637771. doi: 10.3389/fmicb.2021.637771
Received: 04 December 2020; Accepted: 08 February 2021;
Published: 10 March 2021.
Edited by:
Nigel Cook, Jorvik Food & Environmental Virology Ltd., United KingdomReviewed by:
James Pettengill, United States Food and Drug Administration, United StatesChristopher John Grim, United States Food and Drug Administration, United States
Copyright © 2021 Wu, Luo, Xu, Ge, Li, Deng, Wiedmann, Baker, Stevenson, Zhang and Tang. This is an open-access article distributed under the terms of the Creative Commons Attribution License (CC BY). The use, distribution or reproduction in other forums is permitted, provided the original author(s) and the copyright owner(s) are credited and that the original publication in this journal is cited, in accordance with accepted academic practice. No use, distribution or reproduction is permitted which does not comply with these terms.
*Correspondence: Silin Tang, Silin.tang@effem.com