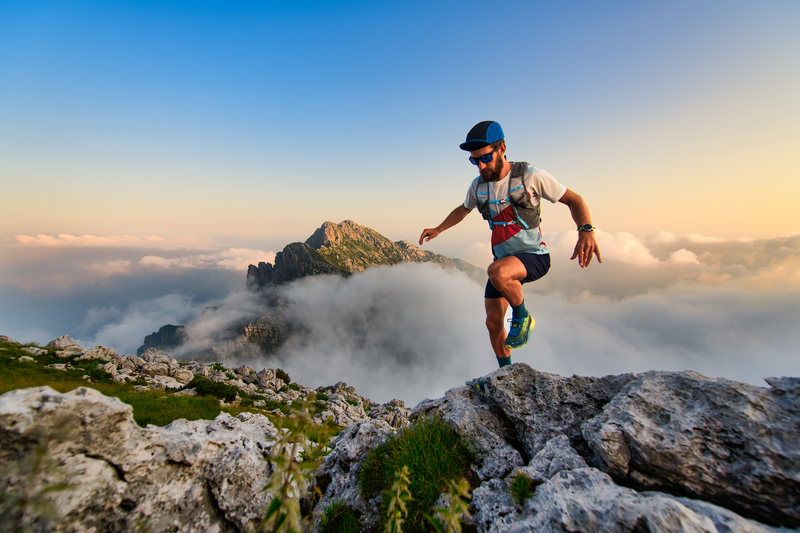
94% of researchers rate our articles as excellent or good
Learn more about the work of our research integrity team to safeguard the quality of each article we publish.
Find out more
PERSPECTIVE article
Front. Microbiol. , 22 February 2021
Sec. Evolutionary and Genomic Microbiology
Volume 12 - 2021 | https://doi.org/10.3389/fmicb.2021.635781
This article is part of the Research Topic Microbiome and Machine Learning View all 10 articles
The human microbiome has emerged as a central research topic in human biology and biomedicine. Current microbiome studies generate high-throughput omics data across different body sites, populations, and life stages. Many of the challenges in microbiome research are similar to other high-throughput studies, the quantitative analyses need to address the heterogeneity of data, specific statistical properties, and the remarkable variation in microbiome composition across individuals and body sites. This has led to a broad spectrum of statistical and machine learning challenges that range from study design, data processing, and standardization to analysis, modeling, cross-study comparison, prediction, data science ecosystems, and reproducible reporting. Nevertheless, although many statistics and machine learning approaches and tools have been developed, new techniques are needed to deal with emerging applications and the vast heterogeneity of microbiome data. We review and discuss emerging applications of statistical and machine learning techniques in human microbiome studies and introduce the COST Action CA18131 “ML4Microbiome” that brings together microbiome researchers and machine learning experts to address current challenges such as standardization of analysis pipelines for reproducibility of data analysis results, benchmarking, improvement, or development of existing and new tools and ontologies.
The microbiome has long been defined as a community of commensal, symbiotic, or pathogenic microorganisms that inhabit a particular body site or environment (Lederberg and McCray, 2001). The current apprehension of the microbiome encompasses the totality of microorganisms and their interactions, interplay with the host and the surrounding environment, and is further influenced by constant co-evolution (Berg et al., 2020). Understanding the composition, balance, and role of the microbiome in human health and disease has become a field of extensive research over the past decade (Wang and Kasper, 2014; Gagnière et al., 2016; Sampson et al., 2016; Barratt et al., 2017). The potential for applications in biomedicine and biotechnology has been especially evident from gut microbiome studies. Furthermore, microbiome research has become an important subject of popular science and led to the acceleration of development in different biotechnology industry sectors.
Some of the key topics in this field cover early life (Tamburini et al., 2016), mechanisms of colonization resistance against pathogens (Buffie and Pamer, 2013; Kim et al., 2017), and stability and individuality of adult microbiota (Mehta et al., 2018), and its associations with diseases, diet, medication, and lifestyle in various populations across the globe (Segata et al., 2011; Schmidt et al., 2018; Cullen et al., 2020). Moreover, the research focus is shifting toward considering the role of genetics and environment (Org et al., 2015; Roslund et al., 2020), as well as of diet (Singh et al., 2017), and to translate this knowledge into microbiota-based clinical solutions (Lynch et al., 2019).
Compared to many other fields of multi-omic studies, microbiomes are dynamic ecosystems with active host regulation. This adds interesting new dimensions and complexity to the analyses and interpretation of data. Thus, the field also requires additional ecological perspectives. The advances in high-throughput sequencing technologies have accelerated microbiome research (Malla et al., 2019), but the volume of data and their complexity sets challenges for analysis. Adaptive statistical and machine learning (ML) methodologies can help us to overcome many of these barriers, but these methodologies need to be adjusted to the specific properties of microbiome data.
Two commonly used strategies for microbiome profiling include the sequencing of a highly conserved region, such as the bacterial 16S ribosomal RNA (16S rRNA), and the untargeted sequencing of genetic material present in the sample, as in shotgun metagenomics (see Box 1 for more information) (Nayfach et al., 2019). The quality of microbiome data and profiling is influenced by experimental, biological, and environmental factors (Poussin et al., 2018). Further variation arises from differences in sequence filtering, clustering, taxonomic assignment and binning, as different bioinformatic tools and pipelines are in use. This lack of standardization introduces statistical biases, and subsequent challenges for reproducibility and cross-study comparisons (Lozupone et al., 2013; Falony et al., 2016; Zhernakova et al., 2016). Some of the first large microbiome profiling studies, as the Human Microbiome Project (Turnbaugh et al., 2007) and the MetaHIT project (Qin et al., 2010), were established as a population-scale framework to develop metagenomic protocols (for a more comprehensive list of large-scale microbiome studies, see Marcos-Zambrano et al., 2021). Despite various attempts to standardize methods, a gold standard of microbiome research is yet to be established (Quince et al., 2017; Knight et al., 2018).
BOX 1 | Common data types in microbiome research.
Amplicon data. Amplicon based approaches are the most widely used high-throughput method for microbiome studies. Amplicon studies comprise data from specific regions of various types of marker genes used for taxonomic profile determination of microbiome: 16S ribosomal RNA (16S rRNA) gene for prokaryotes; 18S ribosomal RNA (18S rRNA) gene for eukaryotes; internal transcribed spacers (ITS) for fungi. These data are characterized by variability in the selected regions, amplification primers and amplification protocols. Due to the sequence similarity, the data are often organized into operational taxonomic units (OTUs) (Schmitt et al., 2012). The two most popular approaches for obtaining groups of related OTUs are based on (i) aligning sequences to a reference database or (ii) clustering sequences based on sequence identity (de novo approach). Once OTU clusters are defined, taxonomic information is given for the representative sequences of each OTU to deduce the phylogeny. However, probabilistic techniques such as DADA2 (Callahan et al., 2016) have recently gained more attention, and are now increasingly used to replace the standard OTU clustering approaches by ASVs, which are un-clustered error-corrected reads. Although amplicon sequencing is cost-effective, the reliability of bacterial classification decreases below genus level, and this methodology does not directly quantify bacterial genes and functions.
Shotgun metagenomics data. A growing number of studies use shotgun metagenomics and offer untargeted sequence data from the analyzed samples. These data typically include contamination from host or environmental reads as well. The non-host DNA can be used for taxonomic analysis or functional profiling of all types of microorganisms present in the microbiome–it allows the analyses of bacteria, viruses, fungi and parasites at the same time. Sequences from metagenomic data can be classified using existing databases or assembled de novo. This type of analysis offers the possibility to analyze strain or even SNP level dynamics of the microbiome (Quince et al., 2017; Zeevi et al., 2019) as well as reconstruction of draft genomes, which enables the identification of novel organisms and provides a way to link functions with taxa. Depending on the aims of the study, shotgun metagenomics can provide a variable amount of data as shallow, deep, or even ultradeep sequencing (Hillmann et al., 2018).
Metatranscriptome data. Metatranscriptomics characterize the expressed transcripts of the analyzed community at a given time point/conditions transcripts of the analyzed community by RNA sequencing data. Depending on the sequencing depth, with this method it is possible to obtain information on gene expression levels both for the microbiome communities and for the host. This requires the highest sequencing depth, most stringent standards for sample storage and processing, and data analysis workflows and benchmarking for these data are only in the developmental stage. Despite these advantages, metatranscriptomes will need to be supported by additional shotgun metagenomics measurements for accurate interpretation.
Other-omics data such as metabolomics data, metaproteomics data. These data represent directly measured metabolites or expressed proteins, therefore providing additional functional information. Similarly, these data can contain information both from the microbiome and the host.
The special characteristics of metagenomic sequencing data are posing additional challenges for statistical analysis. For instance, the large inter-individual variability, heteroscedastic variation (i.e., variance increasing with mean abundance) and large biological and technical variations are often not properly approximated by classical Gaussian or log-normal models, requiring customized analytical approaches. Microbiome data sets tend to be sparse and skewed, and typically include many more microbial features compared to the number of samples or observations collected in most microbiome studies to date (Supplementary Table S1). Moreover, microbiome features often exhibit complex and hierarchical dependency structures in terms of taxonomies or co-variation in abundance and function. Moreover, unaligned and misaligned sequence reads, and challenges to distinguish technical and biological variation especially at the level of low-abundant organisms add additional challenges to the microbiome analyses. The demand to represent microbiome data with an arbitrary, but fixed sum of components without loss of information are known from the concept of compositional data (Aitchison, 1986; Gloor et al., 2017). Furthermore, complementary multi-omic and other data types (Box 1) may require different modeling approaches. The integration of different types of data often lacks rigorous model selection procedures, correction for multiple testing, handling of missing data features/labels, or data harmonization and integration (Namkung, 2020).
Finally, the reliability and integration of relevant metadata such as demographics, health, diet, age, medication, lifestyle, and other factors are critical for drawing informative insights from microbiome studies. However, these crucial pieces of information are most often missing or insufficiently machine-readable in publicly available data resources, thus forming bottlenecks on data reuse.
Microbiome research has set fresh challenges for statistical analysis. Instead of a thorough literature review of this rapidly expanding and heterogeneous field, we provide hereby a topical perspective on the application of ML techniques in microbiome research (for an extensive review, please see Marcos-Zambrano et al., 2021).
One of the most common applications of ML is dimensionality reduction, which facilitates the exploration and visualization of community similarity and distribution across the population of study samples. Non-linear approaches have become a common choice due to the inherent complexity of microbial communities, including methods such as PCoA, UMAP, and other techniques (Legendre and Legendre, 2012; Becht et al., 2019; Kobak and Berens, 2019), as well as autoencoders (Oh and Zhang, 2020) have been taken into use. Many automated analysis pipelines readily include these methods (Buza et al., 2019; Liao et al., 2019).
Clustering has found many applications in microbiome research, ranging from data preprocessing to downstream community analyses. A popular method is the denoiser DADA2 (Callahan et al., 2016), designed to identify unique 16S rRNA amplicon sequence variants (ASVs) (Davis et al., 2018). In metagenome sequencing studies, probabilistic methods have been used to assemble contigs into genome bins based on information of abundance and sequence information; CONCOCT (Alneberg et al., 2014) implements non-parametric clustering based on a variational Gaussian mixture model. The advantage of the non-parametric approach is the automated determination of the cluster number based on the model, rather than post hoc evaluation indices such as the Kalinski-Harabasz or Silhouette index. In the downstream analysis of microbiome data, a notable application of clustering algorithms has been the identification of microbiome community types, used to stratify individuals into specific subgroups based on microbiome composition (Holmes et al., 2012; Costea et al., 2018). Recently, more detailed assemblage models have been developed to identify latent factors and sub-communities that can complement ecosystem-wide stratification that focuses on overarching community types. Examples include phylofactor (Washburne et al., 2019), tipping elements (Lahti et al., 2014), non-negative matrix factorization, latent Dirichlet allocation, and other latent mixture models (Sankaran and Holmes, 2019).
Classification methods are commonly used in taxonomic assignment of metagenomic reads to annotate genome sequences (Treangen et al., 2013; Tamames et al., 2019) or in the production of metagenome-assembled genomes (Murovec et al., 2020). Another application is sample classification in diagnostic or prognostic studies (Pasolli et al., 2016; Aryal et al., 2020). Common ML algorithms such as random forest, support vector machines (SVM), elastic net, and LASSO have all been used for disease-prediction tasks (Pasolli et al., 2016), and automated feature selection schemes have been reported to perform will in comparison with standard tests in disease prediction (Ai et al., 2017). Instead of hard classification, some applications focus on detecting estimated percentage contribution, or soft classification, of each potential source environment related to the sample (Knights et al., 2011; Shenhav et al., 2019; McGhee et al., 2020).
Deep learning (DL) is increasingly applied in microbiome research Convolutional Neural Networks (CNNs) (Armour et al., 2019) have recently been augmented with phylogenetic tree information (Reiman et al., 2018), or combined neural networks with random forests (Rahman and Rangwala, 2020). Variable evaluation metrics including accuracy, precision, recall, F1-score and area under curve (AUC), have been used, highlighting the need for standardized benchmarks regarding well-defined modeling tasks; systematic evaluations have been carried out for instance for metagenome-based disease prediction and differentiation of body sites based on microbiome composition (Asgari et al., 2018; Reiman et al., 2018; Díez López et al., 2019; LaPierre et al., 2019). DL has been also applied to classify antibiotic resistance genes (ARGs) derived from metagenomic data (Arango-Argoty et al., 2018) and to overcome the lack of well-curated taxonomic trees for newly discovered species in metagenome assembled genomes (Murovec et al., 2020). DL has also been used to predict how gut microbiome responds to perturbations by antibiotics (Rahman et al., 2018). Whereas DL methods are notoriously data-hungry, recent applications have shown promising performance with moderate training sample sizes.
A vast number of microbiome studies quantify associations between the abundances of specific metagenomic and functional features, and key covariates such as health and disease, and other factors including diet, medication, geography, or stool consistency (Turnbaugh et al., 2007; Qin et al., 2010; Falony et al., 2016; Zhernakova et al., 2016). The analysis covers a vast spectrum of standard ML methods with additional adaptations to microbiome data. Popular approaches include adaptations of linear discriminant analysis (Segata et al., 2011), negative binomials (Love et al., 2014), and Dirichlet distributions (Fernandes et al., 2014), and non-parametric methods (Weiss et al., 2017; Lin and Peddada, 2020). Non-parametric regression models, such as Gaussian processes, have been also used to study associations between microbiome diversity and external conditions (Arbel et al., 2016). Common techniques for community comparisons include regularized discriminant analysis (RDA) (Legendre and Legendre, 2012), random forest (Sze and Schloss, 2018; Topçuoğlu et al., 2020), and gradient boosting (Qin et al., 2020; Topçuoğlu et al., 2020). Further strategies have been developed in order to consider hierarchical dependencies between taxonomic groups to control for multiple testing and to identify the appropriate taxonomic levels for associations (Sankaran and Holmes, 2014; Washburne et al., 2017).
Other emerging applications include spatio-temporal modeling of microbiome variation both at the individual and population levels as well as the biogeographical variation within and across body sites; agent-based models provide interesting opportunities in this area (Juhász et al., 2014; Lin et al., 2018). Probabilistic joint species distribution models have also been recently applied in the microbiome context (Björk et al., 2018). Bayesian ML techniques can help to deal with uncertainties related to the limited information in short and sparse time series or spatial sampling. The uncertainty, the limited sampling density, or the limited amount of labeled examples when training a model can also be addressed through semi-supervised methods. Prospective analyses predicting long-term incident of health and disease risk based on microbiome composition have remained scarce due to the lack of large-scale cohorts with long-term follow-ups, but the need for prospective analysis methods is now emerging (Liu et al., 2020; Salosensaari et al., 2020). Mendelian randomization and related techniques are finding applications to understand the causal role of gut microbiome in disease (Sanna et al., 2019; Hughes et al., 2020).
Statistics and ML provide tools to extract useful information from scarce, noisy, and limited data. In particular, within microbiome data, this has to be balanced with the complexity and limited understanding of the host-regulated ecological processes and the high levels of individual variation. ML has great potential to improve disease diagnosis and identify personalized biomarkers, due to its ability to detect informative patterns in the data with limited prior knowledge of the underlying system.
One of the main shortcomings is, however, the use of inappropriately small datasets, as apparent from the example studies (and their corresponding datasets) listed in Supplementary Table S1. Data accumulation will further enhance the use of more advanced ML technologies. Efficient data structures and making microbiome data Findable, Accessible, Interoperable, and Reusable (FAIR)1 can provide invaluable support for the open development of statistical and ML tools to help to advance the field (Shetty and Lahti, 2019). Consequently, data repositories maintained by large consortia could serve as a central resource for the research community (Meyer et al., 2008; Mitchell et al., 2020). However, to this aim, the submission of the metadata must follow controlled vocabulary and minimal standards (ten Hoopen et al., 2017).
Some of the main challenges in detecting associations between specific microbiome features and key covariates are related to choosing appropriate distributional assumptions including sparsity and compositionality, appropriate feature selection, controlling for technical biases such as read count variations, the potential confounding effects, and multiple testing. Successful solutions often present combinations of statistical techniques that have been specifically tailored to fit the particular characteristics of microbiome data. Besides, over-fitting, incomplete model selection or performance assessment can lead to poor generalizability of the results in previously unseen data sets and lack of reproducibility. It is essential to understand the principles underlying each method and follow the recommended guidelines in order to ensure compliance with the modeling assumptions (Rule et al., 2019) and avoid overfitting (Eetemadi et al., 2020). Another important driver for the field is the development of suitable data structures in statistical programming languages, such as the R/Bioconductor ecosystem as curatedMetagenomicData (Pasolli et al., 2016) and the phyloseq (McMurdie and Holmes, 2013) or TreeSummarizedExperiment classes (Huang et al., 2020), that permit standardization and efficient collaborative development of methods.
The microbiome field is moving from associations to causality, mechanisms, and prediction, and ML will aid in this transition. Data obtained from ML methods can help to propose new hypotheses to be tested in experimental models, as well as to accelerate the translation of the microbiome data into clinical practice. Its optimal use will presumably trigger the improvement of the searching of biomarker candidates for disease diagnostics, prognostics, and the use of statistical inference for causal insights (Pearl, 2009; Walhout et al., 2013), as with the increasing need to model temporal and dynamical variation. But these advances will appear through validation of the results obtained by sequencing (e.g., using an independent approach such as qPCR), followed by combinations with other omics, especially with metabolomics and metatranscriptomics.
Interpretability by non-experts is an essential consideration when ML models are put in practice by translational researchers. To overcome existing trade-offs between model interpretability and performance (Topçuoğlu et al., 2020) an active collaboration and joint education/training of researchers from statistical, biomedical and clinical fields is essential. Therefore, one main priority is the development of user-friendly tools for translational and clinical personnel, who may have limited experience with bioinformatics methods. In this line, popular software like mothur (Schloss et al., 2009, QIIME2 (Bolyen et al., 2019), and MicrobiomeAnalyst (Chong et al., 2020), the R/Bioconductor ecosystem (Qin et al., 2010), Anvi’o (Eren et al., 2015), and Biobakery (McIver et al., 2018) have incorporated ML methods into their applications in a readily usable format. Hence, the role of open source software ecosystems is critical for the overall development of the whole field. This can support and advance open collaboration networks and co-creation models that have been further complemented with open benchmark data sets (Olson et al., 2017) and reproducible notebooks (Rule et al., 2019). None of the above, however, can be achieved without multidisciplinary training of “next-generation” experts that could be integrated in clinical environments, ultimately facilitating clinical decision-making based on microbiome data as part of personalized medicine strategies (Gómez-López et al., 2019).
In order to accelerate this transition, the COST (European Cooperation in Science and Technology) Action “ML4Microbiome” (Machine Learning for Microbiome) started in 2019 with the aim to coordinate a synergistic network of the use of ML in Microbiome research at the European level. This COST Action CA18131 on Statistical and Machine Learning Techniques in Human Microbiome Studies is a step toward tackling the challenges by strengthening the network of European researchers in this emerging research area (Figure 1). A space of discussion to break down barriers of communication between fields, as well as their engagement, is being constructed through its four working groups (WG) and several networking and training events http://www.ml4microbiome.eu. It is also planned to launch a DREAM challenge2. DREAM challenges are crowdsourced benchmark efforts. By decoupling the method development (open to any scientist) to their evalution (by the organizers based on hold-back data, these challenges provide an unbiased and transparent assessment of methods (Saez-Rodriguez et al., 2016). Furthermore, the action ML4Microbiome identified multiple shortcomings in the current research that need to be taken into consideration. The field will benefit from increasing sample sizes, and the availability of spatial and longitudinal profiling that can be used to train more detailed and accurate models of microbiome variation. The development of interpretable and transparent ML methods will help to bridge the gap between methodological and applied fields. ML4Microbiome is open for new multi-disciplinary collaborations and collaborative ML methods development, and is welcoming researchers to participate in workshops, courses, source code/tool development aiming to promote the use of appropriate statistical and machine learning methods in metagenomics.
Figure 1. Approach of the Action ML4Microbiome to the implementation of machine learning methods in microbiome research, driving change of the field in personalized medicine.
The original contributions presented in the study are included in the article/Supplementary Material, further inquiries can be directed to the corresponding author/s.
IM-I, AZ, DG-C, and MC conceived the manuscript. IM-I, LL, MN, IE, and GR coordinated, supervised, and wrote the draft, the Supplementary Information, and the final manuscript. MA, OA, BB-G, ES, DD’E, MD, LF, AG, KH, TK, ML, LM-Z, CM, MM, PM, LP, GP, SP, VP, PP, AS, RSh, BS, RSu, JT, C-OT, BV, DV, EY, GZ, JS-R, AZ, DG-C, and MC revised draft manuscript, provided comments, included manual references, and wrote parts of the final manuscript. All the authors discussed and approved the final version of the manuscript.
This study was supported by the COST Action CA18131 “Statistical and machine learning techniques in human microbiome studies.” IM-I was supported by the “MS type I” program (CP16/00163) from the Instituto de Salud Carlos III and co-funded by Fondo Europeo de Desarrollo Regional-FEDER. MN was grateful for the additional support by the project “Information and Communication Technologies for a Single Digital Market in Science, Education and Security” of the Scientific Research Center, NIS-3317 and National roadmaps for research infrastructures (RIs) grant number NIS-3318. LL was supported by Academy of Finland (decision 295741). IE was supported by H2020-EU.4.b. project “Integration of knowledge and biobank resources in comprehensive translational approach for personalized prevention and treatment of metabolic disorders (INTEGROMED)” (grant agreement ID 857572). MD was supported by the Luxembourg National Research Fund (FNR) CORE grant (C18/BM/12585940).
The authors declare that the research was conducted in the absence of any commercial or financial relationships that could be construed as a potential conflict of interest.
We are grateful to all COST Action CA18131 “Statistical and machine learning techniques in human microbiome studies” members for their contributions to the discussion about the topics in this perspective, and especially to the WG4 and WG1.
The Supplementary Material for this article can be found online at: https://www.frontiersin.org/articles/10.3389/fmicb.2021.635781/full#supplementary-material
Supplementary Table 1 | Summary and main characteristics of human microbiome studies employing ML approaches.
Ai, L., Tian, H., Chen, Z., Chen, H., Xu, J., and Fang, J.-Y. (2017). Systematic evaluation of supervised classifiers for fecal microbiota-based prediction of colorectal cancer. Oncotarget 8, 9546–9556. doi: 10.18632/oncotarget.14488
Aitchison, J. (1986). THE statistical Analysis of Compositional Data. New York, NY: Chapman and Hall.
Alneberg, J., Bjarnason, B. S., de Bruijn, I., Schirmer, M., Quick, J., Ijaz, U. Z., et al. (2014). Binning metagenomic contigs by coverage and composition. Nat. Methods 11, 1144–1146. doi: 10.1038/nmeth.3103
Arango-Argoty, G., Garner, E., Pruden, A., Heath, L. S., Vikesland, P., and Zhang, L. (2018). DeepARG: a deep learning approach for predicting antibiotic resistance genes from metagenomic data. Microbiome 6:23. doi: 10.1186/s40168-018-0401-z
Arbel, J., Mengersen, K., and Rousseau, J. (2016). Bayesian nonparametric dependent model for partially replicated data: the influence of fuel spills on species diversity. Ann. Appl. Stat. 10, 1496–1516. doi: 10.1214/16-AOAS944
Armour, C. R., Nayfach, S., Pollard, K. S., and Sharpton, T. J. (2019). A metagenomic meta-analysis reveals functional signatures of health and disease in the human gut microbiome. mSystems 4:e00332-18. doi: 10.1128/mSystems.00332-18
Aryal, S., Alimadadi, A., Manandhar, I., Joe, B., and Cheng, X. (2020). Machine learning strategy for gut microbiome-based diagnostic screening of cardiovascular disease. Hypertens. Dallas Tex 1979, 1555–1562. doi: 10.1161/HYPERTENSIONAHA.120.15885
Asgari, E., Garakani, K., McHardy, A. C., and Mofrad, M. R. K. (2018). MicroPheno: predicting environments and host phenotypes from 16S rRNA gene sequencing using a k-mer based representation of shallow sub-samples. Bioinform. Oxf. Engl. 34, i32–i42. doi: 10.1093/bioinformatics/bty296
Barratt, M. J., Lebrilla, C., Shapiro, H.-Y., and Gordon, J. I. (2017). The gut microbiota, food science, and human nutrition: a timely marriage. Cell Host Microbe 22, 134–141. doi: 10.1016/j.chom.2017.07.006
Becht, E., McInnes, L., Healy, J., Dutertre, C.-A., Kwok, I. W. H., Ng, L. G., et al. (2019). Dimensionality reduction for visualizing single-cell data using UMAP. Nat. Biotechnol. 37, 38–44. doi: 10.1038/nbt.4314
Berg, G., Rybakova, D., Fischer, D., Cernava, T., Vergès, M.-C. C., Charles, T., et al. (2020). Microbiome definition re-visited: old concepts and new challenges. Microbiome 8:103. doi: 10.1186/s40168-020-00875-0
Björk, J. R., Hui, F. K. C., O’Hara, R. B., and Montoya, J. M. (2018). Uncovering the drivers of host-associated microbiota with joint species distribution modelling. Mol. Ecol. 27, 2714–2724. doi: 10.1111/mec.14718
Bolyen, E., Rideout, J. R., Dillon, M. R., Bokulich, N. A., Abnet, C. C., Al-Ghalith, G. A., et al. (2019). Reproducible, interactive, scalable and extensible microbiome data science using QIIME 2. Nat. Biotechnol. 37, 852–857. doi: 10.1038/s41587-019-0209-9
Buffie, C. G., and Pamer, E. G. (2013). Microbiota-mediated colonization resistance against intestinal pathogens. Nat. Rev. Immunol. 13, 790–801. doi: 10.1038/nri3535
Buza, T. M., Tonui, T., Stomeo, F., Tiambo, C., Katani, R., Schilling, M., et al. (2019). iMAP: an integrated bioinformatics and visualization pipeline for microbiome data analysis. BMC Bioinformatics 20:374. doi: 10.1186/s12859-019-2965-4
Callahan, B. J., McMurdie, P. J., Rosen, M. J., Han, A. W., Johnson, A. J. A., and Holmes, S. P. (2016). DADA2: high-resolution sample inference from Illumina amplicon data. Nat. Methods 13, 581–583. doi: 10.1038/nmeth.3869
Chong, J., Liu, P., Zhou, G., and Xia, J. (2020). Using MicrobiomeAnalyst for comprehensive statistical, functional, and meta-analysis of microbiome data. Nat. Protoc. 15, 799–821. doi: 10.1038/s41596-019-0264-1
Costea, P. I., Hildebrand, F., Arumugam, M., Bäckhed, F., Blaser, M. J., Bushman, F. D., et al. (2018). Enterotypes in the landscape of gut microbial community composition. Nat. Microbiol. 3, 8–16. doi: 10.1038/s41564-017-0072-8
Cullen, C. M., Aneja, K. K., Beyhan, S., Cho, C. E., Woloszynek, S., Convertino, M., et al. (2020). Emerging priorities for microbiome research. Front. Microbiol. 11:136. doi: 10.3389/fmicb.2020.00136
Davis, N. M., Proctor, D. M., Holmes, S. P., Relman, D. A., and Callahan, B. J. (2018). Simple statistical identification and removal of contaminant sequences in marker-gene and metagenomics data. Microbiome 6:226. doi: 10.1186/s40168-018-0605-2
Díez López, C., Vidaki, A., Ralf, A., Montiel González, D., Radjabzadeh, D., Kraaij, R., et al. (2019). Novel taxonomy-independent deep learning microbiome approach allows for accurate classification of different forensically relevant human epithelial materials. Forensic Sci. Int. Genet. 41, 72–82. doi: 10.1016/j.fsigen.2019.03.015
Eetemadi, A., Rai, N., Pereira, B. M. P., Kim, M., Schmitz, H., and Tagkopoulos, I. (2020). The computational diet: a review of computational methods across diet, microbiome, and health. Front. Microbiol. 11:393. doi: 10.3389/fmicb.2020.00393
Eren, A. M., Esen, ÖC., Quince, C., Vineis, J. H., Morrison, H. G., Sogin, M. L., et al. (2015). Anvi’o: an advanced analysis and visualization platform for ‘omics data. PeerJ 3:e1319. doi: 10.7717/peerj.1319
Falony, G., Joossens, M., Vieira-Silva, S., Wang, J., Darzi, Y., Faust, K., et al. (2016). Population-level analysis of gut microbiome variation. Science 352, 560–564. doi: 10.1126/science.aad3503
Fernandes, A. D., Reid, J. N., Macklaim, J. M., McMurrough, T. A., Edgell, D. R., and Gloor, G. B. (2014). Unifying the analysis of high-throughput sequencing datasets: characterizing RNA-seq, 16S rRNA gene sequencing and selective growth experiments by compositional data analysis. Microbiome 2:15. doi: 10.1186/2049-2618-2-15
Gagnière, J., Raisch, J., Veziant, J., Barnich, N., Bonnet, R., Buc, E., et al. (2016). Gut microbiota imbalance and colorectal cancer. World J. Gastroenterol. 22, 501–518. doi: 10.3748/wjg.v22.i2.501
Gloor, G. B., Macklaim, J. M., Pawlowsky-Glahn, V., and Egozcue, J. J. (2017). Microbiome datasets are compositional: and this is not optional. Front. Microbiol. 8:2224. doi: 10.3389/fmicb.2017.02224
Gómez-López, G., Dopazo, J., Cigudosa, J. C., Valencia, A., and Al-Shahrour, F. (2019). Precision medicine needs pioneering clinical bioinformaticians. Brief. Bioinform. 20, 752–766. doi: 10.1093/bib/bbx144
Hillmann, B., Al-Ghalith, G. A., Shields-Cutler, R. R., Zhu, Q., Gohl, D. M., Beckman, K. B., et al. (2018). Evaluating the information content of shallow shotgun metagenomics. mSystems 3, e69–e18. doi: 10.1128/mSystems.00069-18
Holmes, I., Harris, K., and Quince, C. (2012). Dirichlet multinomial mixtures: generative models for microbial metagenomics. PLoS One 7:e30126. doi: 10.1371/journal.pone.0030126
Huang, R., Soneson, C., Ernst, F. G. M., Rue-Albrecht, K. C., Yu, G., Hicks, S. C., et al. (2020). TreeSummarizedExperiment: a S4 class for data with hierarchical structure. F1000Research 9:1246. doi: 10.12688/f1000research.26669.1
Hughes, D. A., Bacigalupe, R., Wang, J., Rühlemann, M. C., Tito, R. Y., Falony, G., et al. (2020). Genome-wide associations of human gut microbiome variation and implications for causal inference analyses. Nat. Microbiol. 5, 1079–1087. doi: 10.1038/s41564-020-0743-8
Juhász, J., Kertész-Farkas, A., Szabó, D., and Pongor, S. (2014). Emergence of collective territorial defense in bacterial communities: horizontal gene transfer can stabilize microbiomes. PLoS One 9:e0095511. doi: 10.1371/journal.pone.0095511
Kim, S., Covington, A., and Pamer, E. G. (2017). The intestinal microbiota: antibiotics, colonization resistance, and enteric pathogens. Immunol. Rev. 279, 90–105. doi: 10.1111/imr.12563
Knight, R., Vrbanac, A., Taylor, B. C., Aksenov, A., Callewaert, C., Debelius, J., et al. (2018). Best practices for analysing microbiomes. Nat. Rev. Microbiol. 16, 410–422. doi: 10.1038/s41579-018-0029-9
Knights, D., Kuczynski, J., Charlson, E. S., Zaneveld, J., Mozer, M. C., Collman, R. G., et al. (2011). Bayesian community-wide culture-independent microbial source tracking. Nat. Methods 8:761. doi: 10.1038/nmeth.1650
Kobak, D., and Berens, P. (2019). The art of using t-SNE for single-cell transcriptomics. Nat. Commun. 10:5416. doi: 10.1038/s41467-019-13056-x
Lahti, L., Salojärvi, J., Salonen, A., Scheffer, M., and de Vos, W. M. (2014). Tipping elements in the human intestinal ecosystem. Nat. Commun. 5:4344. doi: 10.1038/ncomms5344
LaPierre, N., Ju, C. J.-T., Zhou, G., and Wang, W. (2019). MetaPheno: a critical evaluation of deep learning and machine learning in metagenome-based disease prediction. Methods San Diego Calif. 166, 74–82. doi: 10.1016/j.ymeth.2019.03.003
Lederberg, J., and McCray, A. T. (2001). ‘Ome sweet ‘omics– a genealogical treasury of words. Scientist 15:8. doi: 10.1089/clinomi.03.09.05
Liao, T., Wei, Y., Luo, M., Zhao, G.-P., and Zhou, H. (2019). tmap: an integrative framework based on topological data analysis for population-scale microbiome stratification and association studies. Genome Biol. 20:293. doi: 10.1186/s13059-019-1871-4
Lin, C., Culver, J., Weston, B., Underhill, E., Gorky, J., and Dhurjati, P. (2018). GutLogo: agent-based modeling framework to investigate spatial and temporal dynamics in the gut microbiome. PLoS One 13:e0207072. doi: 10.1371/journal.pone.0207072
Lin, H., and Peddada, S. D. (2020). Analysis of compositions of microbiomes with bias correction. Nat. Commun. 11:3514. doi: 10.1038/s41467-020-17041-7
Liu, Y., Meric, G., Havulinna, A. S., Teo, S. M., Ruuskanen, M., Sanders, J., et al. (2020). Early prediction of liver disease using conventional risk factors and gut microbiome-augmented gradient boosting. medRxiv [Preprint]. doi: 10.1101/2020.06.24.20138933
Love, M. I., Huber, W., and Anders, S. (2014). Moderated estimation of fold change and dispersion for RNA-seq data with DESeq2. Genome Biol. 15:550. doi: 10.1186/s13059-014-0550-8
Lozupone, C. A., Stombaugh, J., Gonzalez, A., Ackermann, G., Wendel, D., Vázquez-Baeza, Y., et al. (2013). Meta-analyses of studies of the human microbiota. Genome Res. 23, 1704–1714. doi: 10.1101/gr.151803.112
Lynch, S. V., Ng, S. C., Shanahan, F., and Tilg, H. (2019). Translating the gut microbiome: ready for the clinic? Nat. Rev. Gastroenterol. Hepatol. 16, 656–661. doi: 10.1038/s41575-019-0204-0
Malla, M. A., Dubey, A., Kumar, A., Yadav, S., Hashem, A., and Abd_Allah, E. F. (2019). Exploring the human microbiome: the potential future role of next-generation sequencing in disease diagnosis and treatment. Front. Immunol. 9:2968. doi: 10.3389/fimmu.2018.02868
Marcos-Zambrano, L. J., Karaduzovic-Hadziabdic, K., Przymus, P., Trajkovik, V., Aasmets, O., Berland, M., et al. (2021). Applications of machine learning in human microbiome studies: a review on feature selection, biomarker identification, disease prediction and treatment. Front. Microbiol. doi: 10.3389/fmicb.2021.634511
McGhee, J. J., Rawson, N., Bailey, B. A., Fernandez-Guerra, A., Sisk-Hackworth, L., and Kelley, S. T. (2020). Meta-SourceTracker: application of Bayesian source tracking to shotgun metagenomics. PeerJ 8:e8783. doi: 10.7717/peerj.8783
McIver, L. J., Abu-Ali, G., Franzosa, E. A., Schwager, R., Morgan, X. C., Waldron, L., et al. (2018). bioBakery: a meta’omic analysis environment. Bioinformatics 34, 1235–1237. doi: 10.1093/bioinformatics/btx754
McMurdie, P. J., and Holmes, S. (2013). phyloseq: an R package for reproducible interactive analysis and graphics of microbiome census data. PLoS One 8:e61217. doi: 10.1371/journal.pone.0061217
Mehta, R. S., Abu-Ali, G. S., Drew, D. A., Lloyd-Price, J., Subramanian, A., Lochhead, P., et al. (2018). Stability of the human faecal microbiome in a cohort of adult men. Nat. Microbiol. 3, 347–355. doi: 10.1038/s41564-017-0096-0
Meyer, F., Paarmann, D., D’Souza, M., Olson, R., Glass, E., Kubal, M., et al. (2008). The metagenomics RAST server – a public resource for the automatic phylogenetic and functional analysis of metagenomes. BMC Bioinformatics 9:386. doi: 10.1186/1471-2105-9-386
Mitchell, A. L., Almeida, A., Beracochea, M., Boland, M., Burgin, J., Cochrane, G., et al. (2020). MGnify: the microbiome analysis resource in 2020. Nucleic Acids Res. 48, D570–D578. doi: 10.1093/nar/gkz1035
Murovec, B., Deutsch, L., and Stres, B. (2020). Computational framework for high-quality production and large-scale evolutionary analysis of metagenome assembled genomes. Mol. Biol. Evol. 37, 593–598. doi: 10.1093/molbev/msz237
Namkung, J. (2020). Machine learning methods for microbiome studies. J. Microbiol. 58, 206–216. doi: 10.1007/s12275-020-0066-8
Nayfach, S., Shi, Z. J., Seshadri, R., Pollard, K. S., and Kyrpides, N. C. (2019). New insights from uncultivated genomes of the global human gut microbiome. Nature 568, 505–510. doi: 10.1038/s41586-019-1058-x
Oh, M., and Zhang, L. (2020). DeepMicro: deep representation learning for disease prediction based on microbiome data. Sci. Rep. 10:6026. doi: 10.1038/s41598-020-63159-5
Olson, R. S., La Cava, W., Orzechowski, P., Urbanowicz, R. J., and Moore, J. H. (2017). PMLB: a large benchmark suite for machine learning evaluation and comparison. BioData Min. 10:36. doi: 10.1186/s13040-017-0154-4
Org, E., Parks, B. W., Joo, J. W. J., Emert, B., Schwartzman, W., Kang, E. Y., et al. (2015). Genetic and environmental control of host-gut microbiota interactions. Genome Res. 25, 1558–1569. doi: 10.1101/gr.194118.115
Pasolli, E., Truong, D. T., Malik, F., Waldron, L., and Segata, N. (2016). Machine Learning Meta-analysis of Large Metagenomic Datasets: Tools and Biological Insights. PLoS Comput. Biol. 12:e1004977. doi: 10.1371/journal.pcbi.1004977
Pearl, J. (2009). Causal inference in statistics: an overview. Stat. Surv. 3, 96–146. doi: 10.1214/09-SS057
Poussin, C., Sierro, N., Boué, S., Battey, J., Scotti, E., Belcastro, V., et al. (2018). Interrogating the microbiome: experimental and computational considerations in support of study reproducibility. Drug Discov. Today 23, 1644–1657. doi: 10.1016/j.drudis.2018.06.005
Qin, J., Li, R., Raes, J., Arumugam, M., Burgdorf, K. S., Manichanh, C., et al. (2010). A human gut microbial gene catalog established by metagenomic sequencing. Nature 464, 59–65. doi: 10.1038/nature08821
Qin, Y., Meric, G., Long, T., Watrous, J., Burgess, S., Havulinna, A., et al. (2020). Genome-wide association and Mendelian randomization analysis prioritizes bioactive metabolites with putative causal effects on common diseases. medRxiv [Preprint]. doi: 10.1101/2020.08.01.20166413
Quince, C., Walker, A. W., Simpson, J. T., Loman, N. J., and Segata, N. (2017). Shotgun metagenomics, from sampling to analysis. Nat. Biotechnol. 35, 833–844. doi: 10.1038/nbt.3935
Rahman, M. A., and Rangwala, H. (2020). IDMIL: an alignment-free interpretable deep multiple instance learning (MIL) for predicting disease from whole-metagenomic data. Bioinformatics 36, i39–i47. doi: 10.1093/bioinformatics/btaa477
Rahman, S. F., Olm, M. R., Morowitz, M. J., and Banfield, J. F. (2018). Machine learning leveraging genomes from metagenomes identifies influential antibiotic resistance genes in the infant gut microbiome. mSystems 3:e00123-17. doi: 10.1128/mSystems.00123-17
Reiman, D., Metwally, A. A., and Dai, Y. (2018). PopPhy-CNN: a phylogenetic tree embedded architecture for convolution neural networks for metagenomic data. bioRxiv [Preprint]. doi: 10.1101/257931
Roslund, M. I., Puhakka, R., Gr nroos, N., Nurminen, N., Oikarinen, N., and Gazal, A. M. (2020). Biodiversity intervention enhances immune regulation and health-associated commensal microbiota among daycare children. Sci. Adv. 6:eaba2578. doi: 10.1126/sciadv.aba2578
Rule, A., Birmingham, A., Zuniga, C., Altintas, I., Huang, S.-C., Knight, R., et al. (2019). Ten simple rules for writing and sharing computational analyses in Jupyter Notebooks. PLoS Comput. Biol. 15:e1007007. doi: 10.1371/journal.pcbi.1007007
Saez-Rodriguez, J., Costello, J. C., Friend, S. H., Kellen, M. R., Mangravite, L., Meyer, P., et al. (2016). Crowdsourcing biomedical research: leveraging communities as innovation engines. Nat. Rev. Genet. 17, 470–486. doi: 10.1038/nrg.2016.69
Salosensaari, A., Laitinen, V., Havulinna, A. S., Meric, G., Cheng, S., Perola, M., et al. (2020). Taxonomic signatures of long-term mortality risk in human gut microbiota. medRxiv [Preprint]. doi: 10.1101/2019.12.30.19015842
Sampson, T. R., Debelius, J. W., Thron, T., Janssen, S., Shastri, G. G., Ilhan, Z. E., et al. (2016). Gut microbiota regulate motor deficits and neuroinflammation in a model of Parkinson’s disease. Cell 167, 1469.e12–1480.e12. doi: 10.1016/j.cell.2016.11.018
Sankaran, K., and Holmes, S. (2014). structSSI: simultaneous and selective inference for grouped or hierarchically structured data. J. Stat. Softw. 59, 1–21. doi: 10.18637/jss.v059.i13
Sankaran, K., and Holmes, S. P. (2019). Latent variable modeling for the microbiome. Biostat. Oxf. Engl. 20, 599–614. doi: 10.1093/biostatistics/kxy018
Sanna, S., van Zuydam, N. R., Mahajan, A., Kurilshikov, A., Vich Vila, A., Võsa, U., et al. (2019). Causal relationships among the gut microbiome, short-chain fatty acids and metabolic diseases. Nat. Genet. 51, 600–605. doi: 10.1038/s41588-019-0350-x
Schmidt, T. S. B., Raes, J., and Bork, P. (2018). The human gut microbiome: from association to modulation. Cell 172, 1198–1215. doi: 10.1016/j.cell.2018.02.044
Schmitt, S., Tsai, P., Bell, J., Fromont, J., Ilan, M., Lindquist, N., et al. (2012). Assessing the complex sponge microbiota: core, variable and species-specific bacterial communities in marine sponges. ISME J. 6, 564–576. doi: 10.1038/ismej.2011.116
Schloss, P. D., Westcott, S. L., Ryabin, T., Hall, J. R., Hartmann, M., Hollister, E. B., et al. (2009). Introducing mothur: open-source, platform-independent, community-supported software for describing and comparing microbial communities. Appl. Environ. Microbiol. 75, 7537–7541. doi: 10.1128/AEM.01541-09
Segata, N., Izard, J., Waldron, L., Gevers, D., Miropolsky, L., Garrett, W. S., et al. (2011). Metagenomic biomarker discovery and explanation. Genome Biol. 12:R60. doi: 10.1186/gb-2011-12-6-r60
Shenhav, L., Thompson, M., Joseph, T. A., Briscoe, L., Furman, O., Bogumil, D., et al. (2019). FEAST: fast expectation-maximization for microbial source tracking. Nat. Methods 16, 627–632. doi: 10.1038/s41592-019-0431-x
Singh, R. K., Chang, H.-W., Yan, D., Lee, K. M., Ucmak, D., Wong, K., et al. (2017). Influence of diet on the gut microbiome and implications for human health. J. Transl. Med. 15:73. doi: 10.1186/s12967-017-1175-y
Sze, M. A., and Schloss, P. D. (2018). Leveraging existing 16S rRNA gene surveys to identify reproducible biomarkers in individuals with colorectal tumors. mBio 9:e00630-18. doi: 10.1128/mBio.00630-18
Tamames, J., Cobo-Simón, M., and Puente-Sánchez, F. (2019). Assessing the performance of different approaches for functional and taxonomic annotation of metagenomes. BMC Genomics 20:960. doi: 10.1186/s12864-019-6289-6
Tamburini, S., Shen, N., Wu, H. C., and Clemente, J. C. (2016). The microbiome in early life: implications for health outcomes. Nat. Med. 22, 713–722. doi: 10.1038/nm.4142
ten Hoopen, P., Finn, R. D., Bongo, L. A., Corre, E., Fosso, B., Meyer, F., et al. (2017). The metagenomic data life-cycle: standards and best practices. GigaScience 6:gix047. doi: 10.1093/gigascience/gix047
Topçuoğlu, B. D., Lesniak, N. A., Ruffin, M. T., Wiens, J., and Schloss, P. D. (2020). A framework for effective application of machine learning to microbiome-based classification problems. mBio 11:e00434-20. doi: 10.1128/mBio.00434-20
Treangen, T. J., Koren, S., Sommer, D. D., Liu, B., Astrovskaya, I., Ondov, B., et al. (2013). MetAMOS: a modular and open source metagenomic assembly and analysis pipeline. Genome Biol. 14:R2. doi: 10.1186/gb-2013-14-1-r2
Turnbaugh, P. J., Ley, R. E., Hamady, M., Fraser-Liggett, C. M., Knight, R., and Gordon, J. I. (2007). The human microbiome project. Nature 449, 804–810. doi: 10.1038/nature06244
Wang, Y., and Kasper, L. H. (2014). The role of microbiome in central nervous system disorders. Brain. Behav. Immun. 38, 1–12. doi: 10.1016/j.bbi.2013.12.015
Washburne, A. D., Silverman, J. D., Leff, J. W., Bennett, D. J., Darcy, J. L., Mukherjee, S., et al. (2017). Phylogenetic factorization of compositional data yields lineage-level associations in microbiome datasets. PeerJ 5:e2969. doi: 10.7717/peerj.2969
Washburne, A. D., Silverman, J. D., Morton, J. T., Becker, D. J., Crowley, D., Mukherjee, S., et al. (2019). Phylofactorization: a graph partitioning algorithm to identify phylogenetic scales of ecological data. Ecol. Monogr. 89:e01353. doi: 10.1002/ecm.1353
Weiss, S., Xu, Z. Z., Peddada, S., Amir, A., Bittinger, K., Gonzalez, A., et al. (2017). Normalization and microbial differential abundance strategies depend upon data characteristics. Microbiome 5:27. doi: 10.1186/s40168-017-0237-y
Zeevi, D., Korem, T., Godneva, A., Bar, N., Kurilshikov, A., Lotan-Pompan, M., et al. (2019). Structural variation in the gut microbiome associates with host health. Nature 568, 43–48. doi: 10.1038/s41586-019-1065-y
Keywords: machine learning, microbiome, ML4Microbiome, personalized medicine, biomarker identification
Citation: Moreno-Indias I, Lahti L, Nedyalkova M, Elbere I, Roshchupkin G, Adilovic M, Aydemir O, Bakir-Gungor B, Santa Pau EC-d, D’Elia D, Desai MS, Falquet L, Gundogdu A, Hron K, Klammsteiner T, Lopes MB, Marcos-Zambrano LJ, Marques C, Mason M, May P, Pašić L, Pio G, Pongor S, Promponas VJ, Przymus P, Saez-Rodriguez J, Sampri A, Shigdel R, Stres B, Suharoschi R, Truu J, Truică C-O, Vilne B, Vlachakis D, Yilmaz E, Zeller G, Zomer AL, Gómez-Cabrero D and Claesson MJ (2021) Statistical and Machine Learning Techniques in Human Microbiome Studies: Contemporary Challenges and Solutions. Front. Microbiol. 12:635781. doi: 10.3389/fmicb.2021.635781
Received: 30 November 2020; Accepted: 28 January 2021;
Published: 22 February 2021.
Edited by:
Nikos Kyrpides, Lawrence Berkeley National Laboratory, United StatesReviewed by:
Stephen Nayfach, Lawrence Berkeley National Laboratory, United StatesCopyright © 2021 Moreno-Indias, Lahti, Nedyalkova, Elbere, Roshchupkin, Adilovic, Aydemir, Bakir-Gungor, Santa Pau, D’Elia, Desai, Falquet, Gundogdu, Hron, Klammsteiner, Lopes, Marcos-Zambrano, Marques, Mason, May, Pašić, Pio, Pongor, Promponas, Przymus, Saez-Rodriguez, Sampri, Shigdel, Stres, Suharoschi, Truu, Truică, Vilne, Vlachakis, Yilmaz, Zeller, Zomer, Gómez-Cabrero and Claesson. This is an open-access article distributed under the terms of the Creative Commons Attribution License (CC BY). The use, distribution or reproduction in other forums is permitted, provided the original author(s) and the copyright owner(s) are credited and that the original publication in this journal is cited, in accordance with accepted academic practice. No use, distribution or reproduction is permitted which does not comply with these terms.
*Correspondence: Isabel Moreno-Indias, aXNhYmVsLm1vcmVub0BpYmltYS5ldQ==
Disclaimer: All claims expressed in this article are solely those of the authors and do not necessarily represent those of their affiliated organizations, or those of the publisher, the editors and the reviewers. Any product that may be evaluated in this article or claim that may be made by its manufacturer is not guaranteed or endorsed by the publisher.
Research integrity at Frontiers
Learn more about the work of our research integrity team to safeguard the quality of each article we publish.