- 1Institute of Technology of Agricultural Products, Hellenic Agricultural Organization DIMITRA, Athens, Greece
- 2Laboratory of Food Microbiology and Biotechnology, Department of Food Science and Human Nutrition, School of Food and Nutritional Sciences, Agricultural University of Athens, Athens, Greece
Chicken liver is a highly perishable meat product with a relatively short shelf-life and that can get easily contaminated with pathogenic microorganisms. This study was conducted to evaluate the behavior of spoilage microbiota and of inoculated Salmonella enterica on chicken liver. The feasibility of Fourier-transform infrared spectroscopy (FTIR) to assess chicken liver microbiological quality through the development of a machine learning workflow was also explored. Chicken liver samples [non-inoculated and inoculated with a four-strain cocktail of ca. 103 colony-forming units (CFU)/g Salmonella] were stored aerobically under isothermal (0, 4, and 8°C) and dynamic temperature conditions. The samples were subjected to microbiological analysis with concomitant FTIR measurements. The developed FTIR spectral analysis workflow for the quantitative estimation of the different spoilage microbial groups consisted of robust data normalization, feature selection based on extra-trees algorithm and support vector machine (SVM) regression analysis. The performance of the developed models was evaluated in terms of the root mean square error (RMSE), the square of the correlation coefficient (R2), and the bias (Bf) and accuracy (Af) factors. Spoilage was mainly driven by Pseudomonas spp., followed closely by Brochothrix thermosphacta, while lactic acid bacteria (LAB), Enterobacteriaceae, and yeast/molds remained at lower levels. Salmonella managed to survive at 0°C and dynamic conditions and increased by ca. 1.4 and 1.9 log CFU/g at 4 and 8°C, respectively, at the end of storage. The proposed models exhibited Af and Bf between observed and predicted counts within the range of 1.071 to 1.145 and 0.995 to 1.029, respectively, while the R2 and RMSE values ranged from 0.708 to 0.828 and 0.664 to 0.949 log CFU/g, respectively, depending on the microorganism and chicken liver samples. Overall, the results highlighted the ability of Salmonella not only to survive but also to grow at refrigeration temperatures and demonstrated the significant potential of FTIR technology in tandem with the proposed spectral analysis workflow for the estimation of total viable count, Pseudomonas spp., B. thermosphacta, LAB, Enterobacteriaceae, and Salmonella on chicken liver.
Introduction
Meat is considered as the most nutritious and energy-rich food product that can provide the human body with all the essential amino acids and micronutrients needed for growth and development (Wood, 2017; Ahmad et al., 2018). Chicken meat has been evidenced to be of greater benefit for human health than red meat because of comparably higher contents of proteins as well as lower contents of fat and cholesterol (Probst, 2009; Pereira and Vicente, 2013; Wood, 2017). Among the edible components of chicken, giblets and especially livers are widely consumed in many countries throughout the world due to their low cost/price, high nutritional value, and short preparation time (Álvarez-Astorga et al., 2002; Shenoda et al., 2019). Chicken liver is an excellent source of important nutrients such as proteins, vitamins (e.g., A, B1, B3, B5, and B6), essential amino acids, and minerals (e.g., Fe, Cu, Mn, and Zn), which are sometimes at levels higher compared to muscle tissue (Jokanović et al., 2014; Seong et al., 2015). The rich nutritional composition along with the neutral pH and high water activity render the chicken liver highly perishable due to microbial growth (Nychas et al., 2008; Odeyemi et al., 2020).
Bacterial contaminants can be introduced at multiple stages along the food chain, including production, slaughter, processing, handling, storage, and preparation, leading thus to significant economic losses (Silva, 2013; Rouger et al., 2017b). The genera of Pseudomonas, Brochothrix, lactic acid bacteria, and Enterobacteriaceae are considered as potential spoilers of chicken meat during storage at low temperatures (Doulgeraki et al., 2012; Rouger et al., 2017b). Chicken microbiota may also harbor pathogenic species, with Salmonella being the most important zoonotic agent responsible for human gastroenteritis due to poultry meat consumption [EFSA (European Food Safety Authority) and ECDC (European Centre for Disease Prevention and Control), (2017); EFSA Panel on Biological Hazards et al., 2019]. Several reports of salmonellosis outbreaks associated with chicken liver consumption have been released worldwide, including the USA (Lanier et al., 2018) and EU [EFSA (European Food Safety Authority) and ECDC (European Centre for Disease Prevention and Control), (2017)]. At the EU level (2014–2016), Salmonella strains were responsible for more than 753 strong-evidence foodborne outbreaks, with 46 outbreaks attributed to broiler meat and products thereof (EFSA Panel on Biological Hazards et al., 2019). Moreover, Salmonella prevalence on chicken giblets has been reported to be over 53.4% in Greece (Zdragas et al., 2012), 59.4% in the mid-Atlantic region of the United States (Jung et al., 2019), and 4.8% in Argentina (Procura et al., 2019). Given the increased production of chicken meat in the last decade, ensuring the microbial safety and quality of chicken liver is of primary importance (Augère-Granier, 2019).
So far, the freshness, spoilage, or safety of meat and poultry products has been relying on sensory, microbiological, and chemical analyses on the finished product (European Commission, 2005). Sensory methods require highly trained personnel, which is costly and not convenient for routine analyses. On the other hand, chemical as well as microbiological analyses (conventional or molecular) are time-consuming, laborious, and destructive to the test products, with some of them requiring high-tech tools and providing retrospective results (Nychas et al., 2008). Thus, their potential to be used for on-, in-, or at-line monitoring in the food industry is limited (Nychas et al., 2016). Exploration of various analytical tools for rapid, non-invasive, and non-destructive quantitative assessment of safety and quality characteristics presents a scientific challenge given the importance of microbiological spoilage and safety on the deterioration of chicken liver freshness.
Nowadays, many different sensors, such as near-infrared spectroscopy, Fourier-transform infrared spectroscopy (FTIR), Raman spectroscopy, hyperspectral and multispectral imaging, have been employed to evaluate freshness, microbial quality, and adulteration of foods (Panagou et al., 2011; Alexandrakis et al., 2012; Argyri et al., 2014; He and Sun, 2015; Tsakanikas et al., 2015; Rateni et al., 2017; Ropodi et al., 2018; Keshavarzi et al., 2020). FTIR spectroscopy, a biochemical fingerprinting technique, in conjunction with chemometrics, machine learning, or computational intelligence methods, has shown significant potential in providing information related to food safety and quality of meat and poultry (Ellis et al., 2004; Ammor et al., 2009; Papadopoulou et al., 2011; Argyri et al., 2013; Ropodi et al., 2016; Pavli et al., 2018; Rahman et al., 2018). However, a repeated challenge that researchers often have to face is the choice of the machine learning approach in order to handle the complex, multivariate nature of the FTIR sensor output. This usually incorporates the analysis of various regression algorithms for reduction of FTIR data dimensionality to ultimately obtain accurate and reliable predictions (Torrione et al., 2014; Ropodi et al., 2016; Tsakanikas et al., 2016, 2020).
Limited research data on the microbiological quality of chicken liver are available nowadays, while studies on the potential of Salmonella to survive and/or proliferate on chicken liver during extended refrigerated storage are even rarer. Hasapidou and Savvaidis (2011) evaluated the effect of oregano essential oil and ethylenediaminetetraacetic acid chelator on the quality characteristics of chicken liver stored under refrigerated (4°C) modified atmosphere conditions. Papazoglou et al. (2012) investigated the effect of thyme oil on the quality of vacuum-packaged chicken liver stored under refrigeration (4°C). Recently, Jung et al. (2019) quantified the levels of Salmonella onto and into raw chicken liver following extended refrigerated (4°C) or frozen (−20°C) storage. Moreover, despite the extended implementation of FTIR spectroscopy to various plant and animal food commodities, to our knowledge, there is limited, if any, information available on chicken liver. On this respect, the present work was attempted (1) to record spoilage microbiota on chicken liver stored aerobically at isothermal and dynamic temperature conditions alone and in the presence of inoculated Salmonella (2) to monitor the behavior of Salmonella on chicken liver under the same storage conditions, and (3) to quantitatively assess spoilage on chicken liver based on FTIR spectral data and microbiological counts from non-inoculated and inoculated-with-Salmonella samples through the development of a spectral analysis and prediction model building workflow that will be specific to chicken liver.
Materials and Methods
Sample Preparation and Experimental Design
Fresh chicken giblets were obtained from a local industry on the day of production and transported (within 30 min) under refrigeration to the laboratory. The chicken livers (ca. 50 ± 2 g) were then aseptically removed from the giblets and packed aerobically in duplicate in styrofoam trays that were subsequently wrapped manually with air-permeable polyethylene plastic film (non-inoculated samples). In parallel, chicken livers (ca. 50 ± 2 g) were inoculated with Salmonella enterica subsp. enterica serovar Enteritidis (four-strain cocktail) and packed under the same conditions. The packed livers were stored under controlled isothermal conditions (0, 4, and 8°C) and dynamic temperature conditions (0, 4, and 8°C every 8 h) for up to 10 days in a high-precision (±0.1°C) cooled incubator (IC 150-R, Agrolab, Capri, Italy). The latter conditions were selected on the basis to simulate the chicken livers’ temperature fluctuations in chill chain. In total, two chronically independent experiments (different product batches) were performed for every treatment (non-inoculated and inoculated samples) and storage temperature, with duplicate samples analyzed microbiologically at each time interval (n = 4).
Inoculation of Chicken Liver With Salmonella
A four-strain cocktail of Salmonella enterica subsp. enterica serovar Enteritidis (FMCC B56 PT4, FMCC B-57 PT7, B64, and ATCC 13076) was used for the inoculation of chicken livers. The strains were kindly provided by Prof. Nychas G-J.E, Lab of Microbiology and Biotechnology, Agricultural University of Athens, Athens, Greece. The Salmonella strains were maintained at −80°C, were revived at 10 ml brain heart infusion (BHI, LAB M, LAB049) broth after overnight incubation at 37°C, and were subcultured in 10 ml fresh BHI broth (18 h, 37°C). Cells of the individual cultures were then harvested by centrifugation (5,000 × g, 10 min, 4°C) and washed twice in 10 ml Ringer’s solution (¼ strength, LAB M). The washed cells of each strain were resuspended in 10 ml Ringer’s solution and were combined in equal volumes to generate a four-strain cocktail. The chicken liver samples were separately inoculated with 50 μl of appropriate dilution of the pathogen cocktail and were then held for approximately 10 min at ambient temperature (21 ± 2°C) to allow the inocula to attach to the surface. The livers were then inverted with sterile tweezers, and the process was repeated on the opposite side to yield a final population of the pathogen of ca. 103 colony-forming units (CFU)/g.
Microbiological Analyses
The chicken liver samples (20 g) were separately weighted aseptically in a sterile stomacher bag containing ¼ strength Ringer’s solution (40 ml) and stomached for 60 s at room temperature (Stomacher 400 Circulator, Seward Limited, Norfolk, United Kingdom). Appropriate serial dilutions of the resulting homogenate were spread (0.1 ml) or poured (1 ml) on different selective and non-selective agar plates for the enumeration of the following bacterial groups: total viable count (TVC) on Tryptic soy agar (REF 4021502, Biolife) incubated at 30°C for 2–3 days, lactic acid bacteria (LAB) on de Man-Rogosa-Sharp medium (LAB233, LABM) overlaid with the same medium and incubated at 30°C for 3–5 days, Brochothrix thermosphacta on streptomycin thallous acetate actidione agar (REF 4020792 with the addition of antibiotic REF 4240052, Biolife) incubated at 25°C for 2 days, yeasts/molds on rose bengal chloramphenicol agar (BK151HA, Biokar) incubated at 25°C for 2–5 days, Enterobacteriaceae on violet red bile glucose agar (CM 0485, Oxoid) overlaid with the same medium and incubated at 37°C for 24 h, Pseudomonas spp. on Pseudomonas agar base (LAB108 supplemented with selective supplement cetrimide fucidin cephaloridine, Modified C.F.C X108, LABM) incubated at 25°C for 2 days, and Salmonella on xylose lysine deoxycholate (LAB032, LABM) incubated at 37°C for 16–18 h. The plates for each agar medium were examined visually for typical morphological characteristics of colonies. Additionally, the selectivity of growth media was checked by Gram staining and microscopic examination of smears prepared from randomly selected colonies obtained from all media.
Uninoculated chicken liver samples (three liver samples/batch) were also analyzed at the beginning of storage using enrichment method for the detection of Salmonella that could naturally appear on chicken liver (ISO 6579-1:2017).
pH Measurements
The pH value was monitored using a digital pH meter (HI 2211 pH-ORP Meter, HANNA Instruments, United States) after the end of microbiological analyses by immersing the glass electrode into the homogenate.
FTIR Spectroscopy
FTIR analysis was performed on the surface of chicken liver samples at 25°C with a ZnSe 45° attenuated total reflectance flat plate crystal on a Perkin Elmer Frontier FTIR spectrometer equipped with DLaTGS detector with a KBr window. The spectrometer was equipped with software PerkinElmer Spectrum v10.4.2 to collect spectra over the wavelength range of 4,000 to 650 cm−1. Scans per measurement were four, with a resolution of 4 cm−1. Prior to the measurement of the tested samples, reference (background) spectra were acquired using the cleaned blank (no added liver sample) crystal. The tested samples were transferred to the crystal plate and then pressed with a gripper to ensure the best possible contact with the surface of the crystal. After each measurement, the crystal’s surface was cleaned, initially with detergent and distilled water and then with ethanol, and dried using lint-free tissue. For each treatment (non-inoculated and inoculated samples stored at isothermal and dynamic storage conditions) and time interval, three FTIR spectra were acquired for each of the two biological replicates of the experiment (n = 12). A total of 878 FTIR spectra were collected and used for further analyses (n = 442 for non-inoculated samples and n = 436 for inoculated-with-Salmonella samples). The FTIR spectra that were ultimately used in further analyses were in the approximate wavelength range of 1,800 to 900 cm−1 as this spectral region has been shown to provide useful metabolic fingerprints with regard to meat spoilage (Papadopoulou et al., 2011; Argyri et al., 2013; Fengou et al., 2019).
Mathematical Modeling of the Spectral Data
Three data sets from chicken liver samples stored at isothermal and dynamic temperature conditions, i.e., non-inoculated, inoculated with Salmonella, and their combination (i.e., non-inoculated and inoculated samples), were analyzed. For each of these data sets, the processing pipeline of acquired FTIR data consisted of a feature selection step (i.e., specific wavelengths/wavenumbers) on the bases of extra-trees regression ensemble (Geurts et al., 2006) followed by a support vector regression (SVR) of the different spoilage-related microbial groups (Smola and Scholkopf, 2004; Tsakanikas et al., 2016). Initially, prior to feature selection, the acquired FTIR spectra (S) were subjected to standard normal variate normalization under its robust version (Guo et al., 1999) according to:
where Si and Sisnv is the ith spectrum and the corresponding normalized spectrum, respectively, and mad is the median absolute deviation, a robust measure of the variability of a univariate sample of quantitative data s1, s2, …, sn (Hoaglin et al., 2000) computed as:
The specific normalization scheme was selected on the basis that gives more reasonable (i.e., without artifacts) and enhanced quality data, eliminating the inherent multiplicative noise while reducing the correlated information along spectra (Tsakanikas et al., 2018). Then, prior to regression and to avoid overfitting of the dataset, due to the small number of samples compared to the large number of input variables, a feature selection step was introduced by applying the extremely randomized trees (extra-trees) algorithm (Geurts et al., 2006). The extra-trees algorithm is a tree-based ensemble method for reducing the dimensionality of spectral data which is characterized by high accuracy and computational efficiency. In this context, the variable set was reduced by preserving the critical features that best represent the samples (in terms of predicting the inherent microbial load) and excluding all the expendable ones (Tsakanikas et al., 2018). Following the feature selection, SVR (Smola and Scholkopf, 2004) was applied to the reduced dataset for the estimation/prediction of the microbial populations from the corresponding spectroscopic data. SVM/R is a robust supervised tool for both classification and regression (Vapnik et al., 1997) and has been used in various food quality applications (Du et al., 2007; Argyri et al., 2013, 2014; Schmutzler et al., 2015; Estelles-Lopez et al., 2017; Ropodi et al., 2018; Yu et al., 2019; Fengou et al., 2020; Tsakanikas et al., 2020). Briefly, in SVMs, the original data x are mapped from the input space onto a high-dimensional feature space via a non-linear mapping function (kernel function) in order to construct an optimal hyperplane that minimizes the total square distance to all data points. In this study, the radial kernel (radial basis function) was used for fitting FTIR data. Grid search for the optimal cost (C) and gamma (γ) parameters, coupled with 10-fold cross-validation, was employed for model development and parameter optimization (Pedregosa et al., 2011).
In order to generate the predictive models, the pretreated dataset was randomly partitioned (using a random generator) over 50 iterations into a training (calibration) dataset, which contained 70% of the samples, and a test dataset composed of the remaining samples for external validation. The training dataset was used for calibration, i.e., model building, while the test (validation) dataset was used to externally evaluate the performance of such model. Samples stored at both isothermal and dynamic temperature conditions were equally represented in training and testing datasets in order to generate a realistic prediction model based on real-life conditions. Data pre-treatment, featuring selection, model development, and validation were implemented using Python 3.6 and scikit-learn library (Pedregosa et al., 2011).
Model Validation
The performance of the regression models was quantified by the calculation of the root mean square error (RMSE), the square of the correlation coefficient (R2), the bias (Bf) and accuracy (Af) factors (Ross, 1996), and the accuracy of prediction (Mohareb et al., 2016; Estelles-Lopez et al., 2017). The RMSE quantifies the average deviation between predicted and observed values (i.e., the smaller the value of the RMSE, the closer the predicted values are to the observed values). The Af provides a measure of how close predictions are to observations. An Af = 1 indicates a perfect agreement between observed and predicted values. The Bf gives a measure of systematic under- or over-prediction by the model. A Bf = 1 indicates a perfect agreement between predictions and observations, while a Bf < 1 indicates that a growth model is fail-safe (i.e., predicted values are smaller than observed values, giving a margin of safety). The accuracy of prediction provides a measure of the percentage of samples correctly predicted (i.e., difference between predicted and observed value is <1) out of the total number of the samples within the dataset.
Statistical Analysis
Differences in microbial populations between non-inoculated and inoculated-with-Salmonella samples (2 batches, n = 2 per batch) were tested with ANOVA. Significance was established at p < 0.05. Data analysis was carried out with SPSS (IBM SPSS Statistics for Windows, Version 26.0. Armonk, NY: IBM Corp.).
Results and Discussion
Population Dynamics on Non-inoculated Chicken Liver
The evolution of the spoilage microbiota (mean ± standard deviation, n = 4) on non-inoculated chicken liver stored aerobically at isothermal (0, 4, and 8°C) and dynamic temperature conditions (0, 4, and 8°C every 8 h) is presented in Figure 1. The initial (day 0) level of TVC on chicken liver was 5.6 ± 0.6 log CFU/g, which is in accordance with previous studies (Hasapidou and Savvaidis, 2011; Papazoglou et al., 2012; Jung et al., 2019). Various initial bacterial loads (2.0–6.3 log CFU/g) have been reported for other animal livers (Shelef, 1975; Gill and DeLacy, 1982; Hanna et al., 1982; Woolthuis et al., 1984; Rivas et al., 1992; Hernández-Herrero et al., 1999; Devatkal et al., 2004; Fernández-López et al., 2006; Silva et al., 2020). The elevated microbiological load observed in fresh liver could be attributed to cross-contamination during slaughter and fabrication (Silva, 2013; Rouger et al., 2017b).
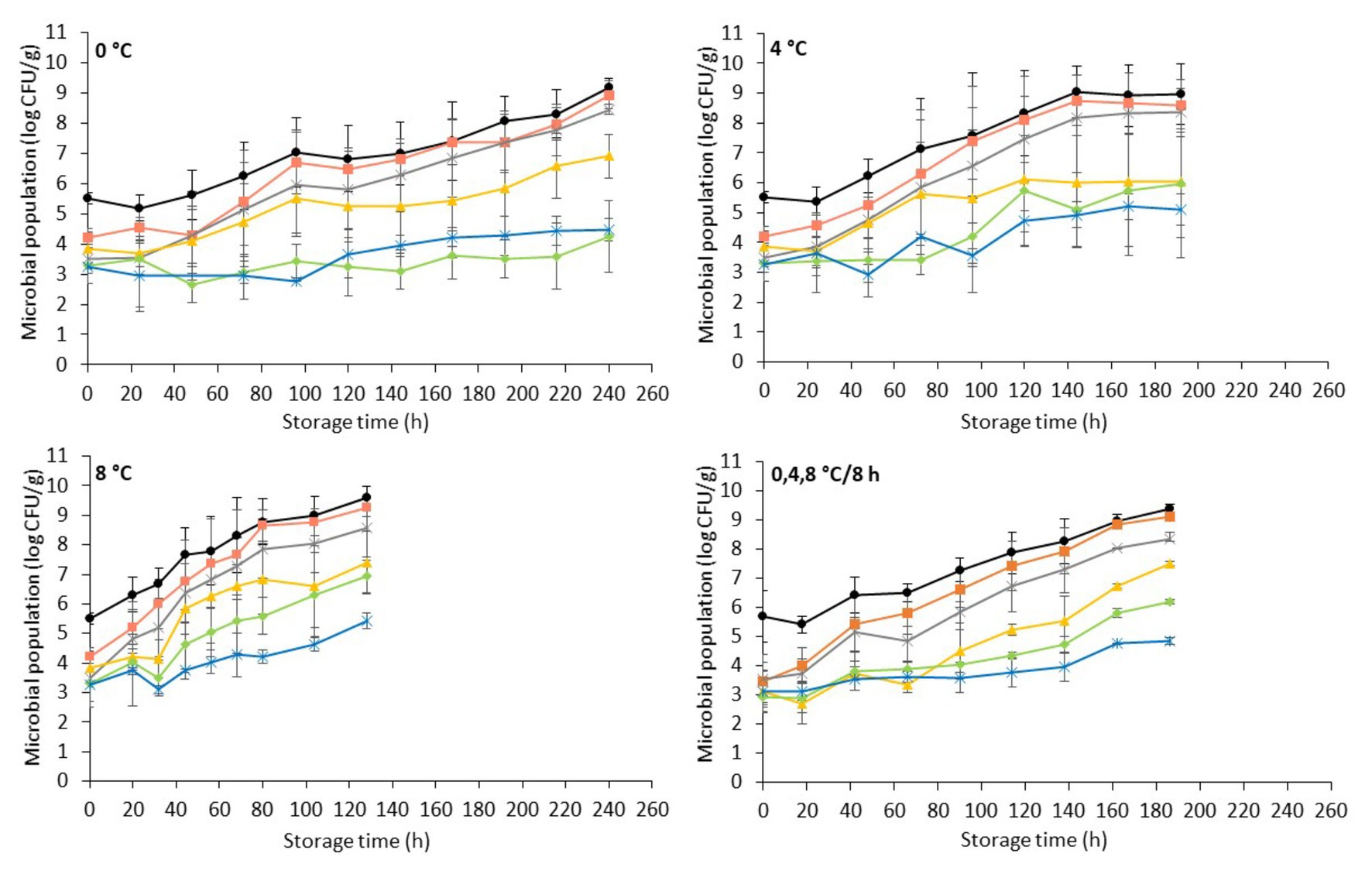
Figure 1. Evolution of indigenous spoilage microbiota (mean ± standard deviation, n = 4) on chicken liver during aerobic storage at different isothermal (0, 4, and 8°C) and dynamic temperature conditions (0, 4, and 8°C every 8 h). Total viable count (), Pseudomonas spp. (
), Brochothrix thermosphacta (
), lactic acid bacteria (
), Enterobacteriaceae (
), and yeast/molds (
).
The microbial association of chicken liver consisted mainly of Pseudomonas spp. (3.8 ± 0.7 log CFU/g), B. thermosphacta (3.5 ± 0.5 log CFU/g), LAB (3.5 ± 0.7 log CFU/g), Enterobacteriaceae (3.1 ± 0.5 log CFU/g), and yeasts/molds (3.2 ± 0.6 log CFU/g). The aerobic storage of chicken livers generally allowed the growth of microorganisms at high levels, with Pseudomonas spp. being the dominant spoilage microorganism, followed closely by B. thermosphacta, while LAB, Enterobacteriaceae, and yeasts/molds remained at lower levels at both isothermal and dynamic temperature conditions (Figure 1). The microbial profile described above is in accordance with other studies on aerobically stored chilled chicken liver and other poultry products (Balamatsia et al., 2007; Papazoglou et al., 2012; Rouger et al., 2017a; Lytou et al., 2018). The dominance of Pseudomonas spp. on aerobically stored red meat (Nychas et al., 2008; Ercolini et al., 2011; Pennacchia et al., 2011; Doulgeraki and Nychas, 2013) and poultry (Mellor et al., 2011; Sahar and Dufour, 2014; Vasconcelos et al., 2014; Lytou et al., 2018; Rahman et al., 2018; Saenz-García et al., 2020) is well documented. B. thermosphacta, a Gram-positive fermentative organism, has been recognized as the dominant spoilage species along with LAB on modified-atmosphere- and vacuum-stored meats (Kakouri and Nychas, 1994; Nychas et al., 2008; Doulgeraki et al., 2010; Ercolini et al., 2011; Pennacchia et al., 2011; Gribble and Brightwell, 2013). However, due to its ubiquitous nature and in agreement with the results of this study, it may also play an important role in shortening the shelf-life of aerobically stored chicken meat (Mikš-Krajnik et al., 2016; Lytou et al., 2018). The rest of the facultative anaerobic bacteria, LAB and Enterobacteriaceae, grew to similar levels in the final stages of storage at 4 and 8°C, while at 0°C LAB outgrew Enterobacteriaceae. Similar growth patterns have been observed by Vasconcelos et al. (2014) in poultry breast fillets stored at 3 and 7°C. In contrast, Papazoglou et al. (2012) reported lower levels for Enterobacteriaceae compared to LAB after storage of chicken livers at 4°C, while Rivas et al. (1992) reported lower levels for LAB on lamb liver stored at 0 and 3°C. The yeasts/mold levels appeared to be lower than the rest of the bacterial populations, in accordance with previous studies reporting yeasts and molds to be a minor part of the microbial association of poultry products, thus contributing less to poultry spoilage (Dillon and Board, 1991; Ismail et al., 2000; Balamatsia et al., 2007). As expected, the dynamics of these populations and their contribution to the final microbiota and, consequently, the spoilage process were temperature dependent, with the growth rate of the different microbial groups being progressively higher with increasing storage temperature (Nychas et al., 2008; Vasconcelos et al., 2014; Galarz et al., 2016).
It has to be noted that, during storage, chicken liver samples presented substantial inter-batch variability with regards to the levels of the different microbial groups (i.e., lower microbial counts in the first compared to the second batch) at all storage temperatures and most of the sampling points (data not shown). This variability could be attributed to factors such as the intrinsic characteristics of the liver tissue and the hygienic practices during slaughter and handling which seem to affect the structure of the indigenous microbial community and its evolution during storage (Huis In’t Veld, 1996; Nychas et al., 2008; Tougan et al., 2013; Luong et al., 2020; Odeyemi et al., 2020). As a result, microbiological spoilage on chicken liver (i.e., TVC > 7 log CFU/g; Nychas and Tassou, 1997; Mikš-Krajnik et al., 2016; Rouger et al., 2017b) was attained at 192 and 72 h of storage at 0°C for the first and second batch, respectively, at 120 and 72 h at 4°C and at 68 and 32 h at 8°C (data not shown). By taking this variability into account (i.e., averaging of TVC populations from both batches), chicken liver spoilage was evident at 144, 72, and 44 h of storage at 0, 4, and 8°C, respectively, and at 90 h of storage at dynamic temperatures (Figure 1). Similar results have been observed in the study by Hasapidou and Savvaidis (2011) who reported a shelf-life of 3 days for refrigerated (4°C) chicken liver.
Visible deterioration of chicken liver samples, i.e., surface browning and appearance of visible colonies, in most cases agreed with microbiological spoilage (TVC > 7 log CFU/g), while the onset of off odors was evident at later time intervals. Our observation is in agreement with the findings of Rivas et al. (1992) and Gill and DeLacy (1982) for lamb and sheep liver, respectively. The malodorous volatiles in high-protein meats are associated with microbial degradation of nitrogenous compounds, such as amino acids, and are usually the first indication of spoilage. Pseudomonads, which are in most cases responsible for the aerobic spoilage of meat, show a preference in utilizing simple carbohydrates (i.e., glucose) prior to free amino acids (Nychas et al., 2008). In the case of liver, which is characterized by high contents of glucose throughout storage, the preference of spoilage organisms for glucose allowed the formation of visible colonies on the surface of liver before the off odors’ accumulation (Gill and DeLacy, 1982; Rivas et al., 1992).
The pH of fresh chicken liver (day 0) was 6.50 ± 0.10, which is in agreement with previous studies (Hasapidou and Savvaidis, 2011; Papazoglou et al., 2012; Figure 2). Similar pH values (ranging from 6.15 to 6.84) have been reported for fresh beef, pork, lamb, buffalo, ostrich, and pork livers (Hanna et al., 1982; Hernández-Herrero et al., 1999; Devatkal et al., 2004; Fernández-López et al., 2006; Custódio et al., 2016). The pH of chicken liver fluctuated during storage but remained relatively constant throughout (minor reductions ranging from 0.15 to 0.28 units for the different storage temperatures). This result is in line with previous studies in aerobically stored animal livers (Gill and DeLacy, 1982; Hanna et al., 1982; Woolthuis et al., 1984; Papazoglou et al., 2012).
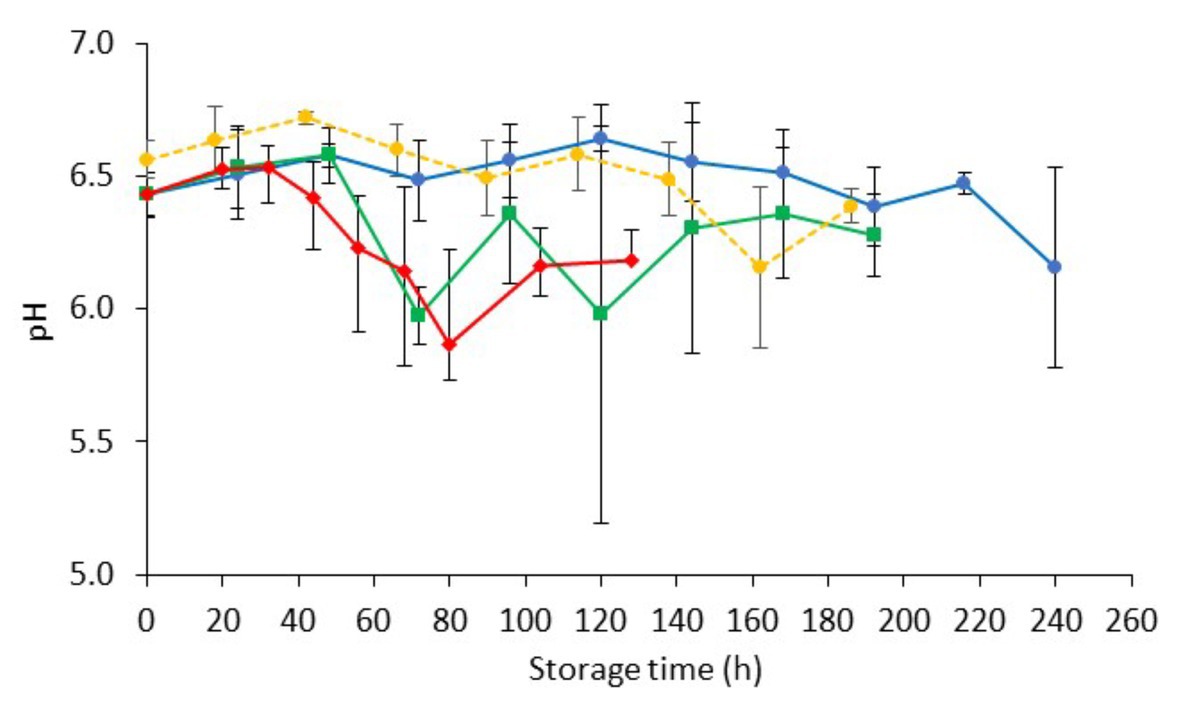
Figure 2. Changes on pH values (mean ± standard deviation, n = 4) of non-inoculated chicken liver during aerobic storage at different isothermal ( 0,
4, and
8°C) and dynamic temperature conditions (
0, 4, and 8°C every 8 h).
Population Dynamics on Chicken Liver Inoculated With Salmonella
The changes of the indigenous spoilage microbiota and Salmonella (mean ± standard deviation, n = 4) on chicken liver inoculated with the pathogen during aerobic storage at isothermal (0, 4, and 8°C) and dynamic temperature conditions (0, 4, and 8°C every 8 h) are presented in Figure 3. The dynamics of the spoilage bacterial populations were influenced by the storage temperature but not (p > 0.05) by the presence of the pathogen per se compared to the respective populations in non-inoculated liver (Figure 1 and Figure 3).
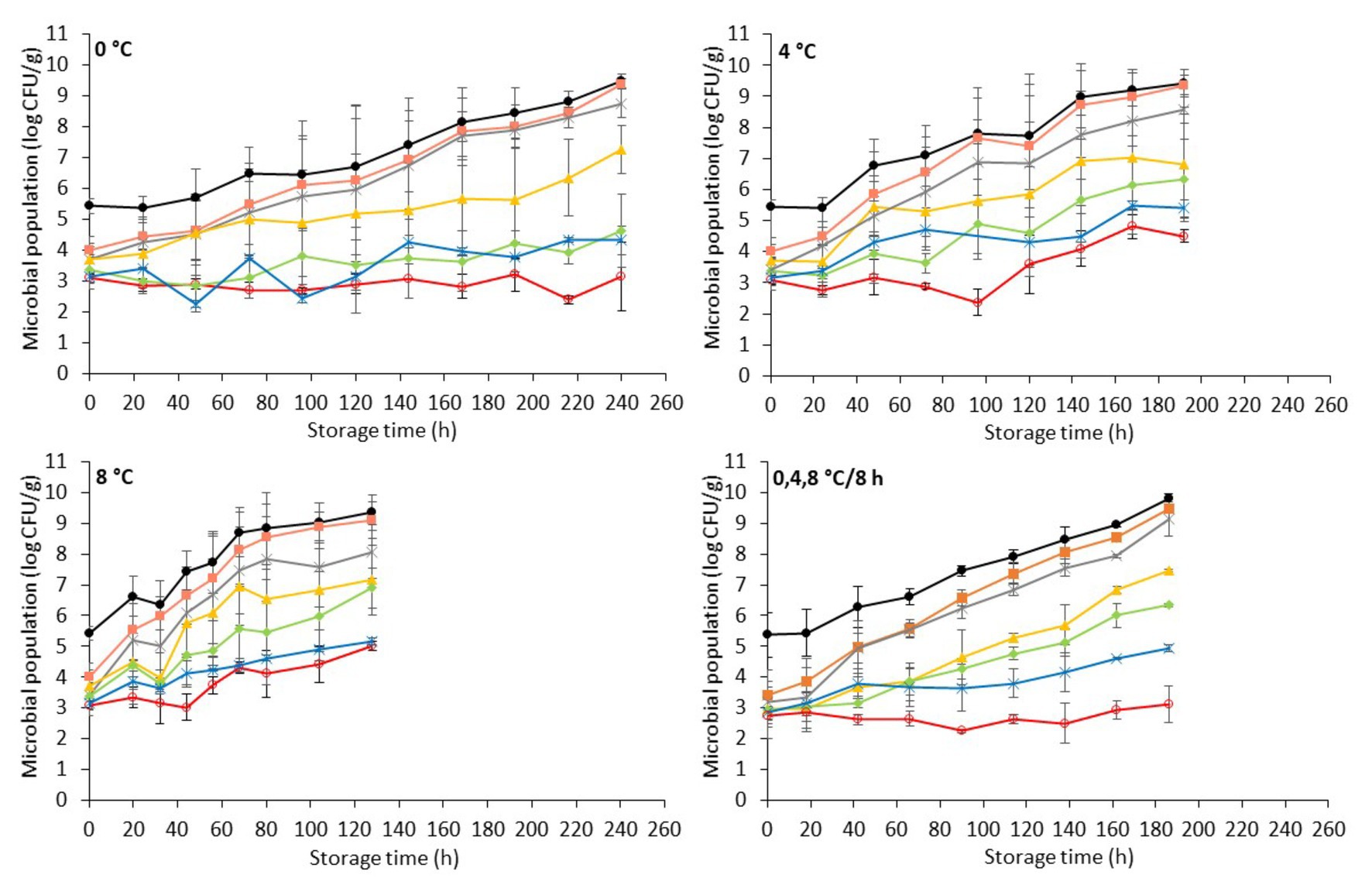
Figure 3. Evolution of Salmonella and indigenous spoilage microbiota (mean ± standard deviation, n = 4) on chicken liver during aerobic storage at different isothermal (0, 4, and 8°C) and dynamic temperature conditions (0, 4 and 8°C every 8 h). Total viable count (), Pseudomonas spp. (
), Brochothrix thermosphacta (
), lactic acid bacteria (
), Enterobacteriaceae (
), yeast/molds (
), and Salmonella (
).
Similarly, the population dynamics of Salmonella was significantly influenced by the storage temperature. Specifically, Salmonella remained at inoculation levels (2.9 ± 0.2 log CFU/g) during storage at 0°C (240 h), while at 4°C it presented a substantial increase after 96 h, reaching final populations of 4.5 ± 0.2 log CFU/g at 192 h. At 8°C, the pathogen increased after 44 h, reaching 5.0 ± 0.2 log CFU/g at the end of the storage period (128 h). However, Salmonella’s onset of growth at 4 and at 8°C coincided chronically with the microbiological spoilage (TVC > 7 log CFU/g) of chicken liver (Figure 3). At dynamic temperature conditions, the Salmonella levels remained practically unaffected during aerobic storage for 186 h (Figure 3). Salmonellae growth, most of the times, is prevented at chilled temperatures (Airoldi and Zottola, 1988; Rhoades et al., 2013; Lerasle et al., 2014). In the study by Oscar (2011), the researcher reported the survival of Salmonella Typhimurium at temperatures between 4 and 8°C on chicken skin. In addition, in the recent study by Jung et al. (2019), Salmonella inoculated either into or onto chicken liver was shown to decrease by ca. 1 log CFU/g after 2 days of storage at 4°C. However, in agreement with the results of the current study, salmonellae have been shown not only to survive but even grow on fresh meats, including minced chicken and chicken parts, stored at refrigerated temperatures (<10°C; Baker et al., 1986; D’Aoust, 1991; Smadi et al., 2012). Specifically, the growth of S. Typhimurium and S. Enteritidis has been displayed on minced meat and on chicken surfaces stored at 2°C within 1–6 days (Catsaras and Grebot, 1985; Baker et al., 1986). Kim et al. (2011) likewise reported the growth of S. Typhimurium at 5°C in kimbap, a Korean ready-to-eat food, while Kinsella et al. (2007) observed the survival and increased growth of a S. Typhimurium strain on beef after 72 h at 4°C. A possible explanation for the various growth patterns of Salmonella at low temperatures could be the different strains studied, the different types of raw material, and the variations on the levels and types of competing indigenous microbiota that could affect the survival or growth of Salmonella (Oscar, 2007; Silva et al., 2016).
The initial pH (day 0) of inoculated-with-Salmonella chicken liver was 6.48 ± 0.08 (Figure 4). The storage of samples resulted in minor decreases in pH (ranging from 0.09 to 0.27, depending on the temperature) that were comparable to those in non-inoculated samples.
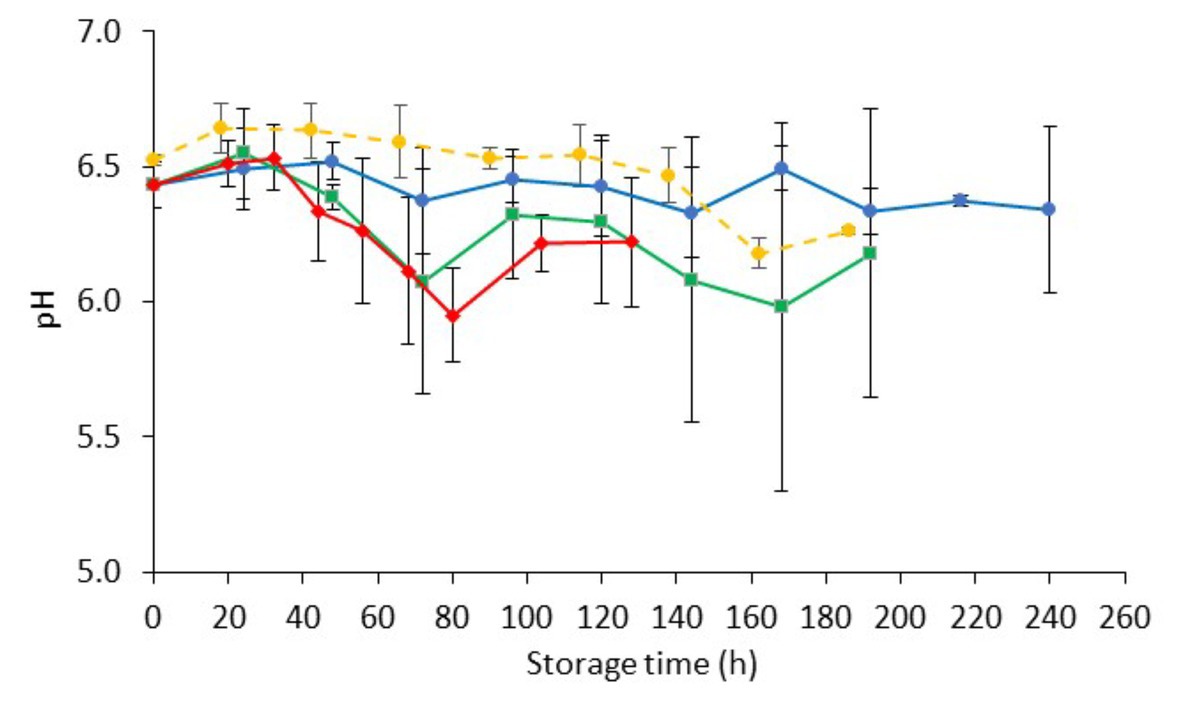
Figure 4. Changes on pH values (mean ± standard deviation, n = 4) of chicken liver inoculated with Salmonella during aerobic storage at different isothermal ( 0,
4, and
8°C) and dynamic temperature conditions (
0, 4, and 8°C every 8 h).
FTIR Spectral Interpretation
FTIR analyses were performed at each time point during storage of non-inoculated and inoculated-with-Salmonella chicken liver at different isothermal (0, 4, and 8°C) and dynamic (0, 4, and 8°C every 8 h) temperature conditions. Representative FTIR profiles, corresponding to non-inoculated and inoculated-with-Salmonella chicken liver samples at the beginning (fresh) and end (spoiled) of aerobic storage at different isothermal temperatures, are presented in Figure 5. Based on Figure 5, a major peak at 1,637 cm−1 was apparent in the chicken liver samples due to the presence of moisture (O–H stretch), with a contribution of amide I band of proteins (80% C=O stretch, 10% C–N stretch, and 10% C–N bend), whereas a second peak was observed at 1,549 cm−1 due to the absorbance of amide II band of proteins (40% C–N stretch, 60% N–H bend; Socrates, 2001; Ellis et al., 2002). Other minor peaks were observed at 1,473 cm−1 ascribed to lipids (CH3 asymmetric deformation, CH3 asymmetric bending, C–H deformation of CH2, CH2 scissoring vibration, C–H bending), with an underlying contribution of amines (asymmetric CH3 deformation vibration), at 1,453 cm−1 ascribed to fat (CH2 bending), at 1,402 cm−1 ascribed to amino acid side chains, lipids, and carbohydrates (C–H bend or C–O stretch in carboxylates) or nitro group (NO2 symmetric stretch), at 1,307 cm−1 attributed to amide III (30% C–N stretch, 30% N–H bend, 10% C=O–N bend, 20% others), at 1,243 cm−1 corresponding to lipids and nucleic acids (asymmetric PO2- stretch), with the contribution of amide III P=O stretch (30% C–N stretch, 30% N–H bend, 10% C=O–N bend, 20% others) and amines from free amino acids (C–N stretch), at 1,117 cm−1 ascribed to riboses (C–O stretch) and amines (NH2 rocking/twisting), at 1,081 cm−1 corresponding to nucleic acids and phospholipids (PO2 symmetric stretch)/C–O stretch, and finally at 1,044 cm−1 corresponding to lipids and polysaccharides (C–O, C–O–P stretch) (Socrates, 2001; Ellis et al., 2002, 2004; Böcker et al., 2007; Ammor et al., 2009).
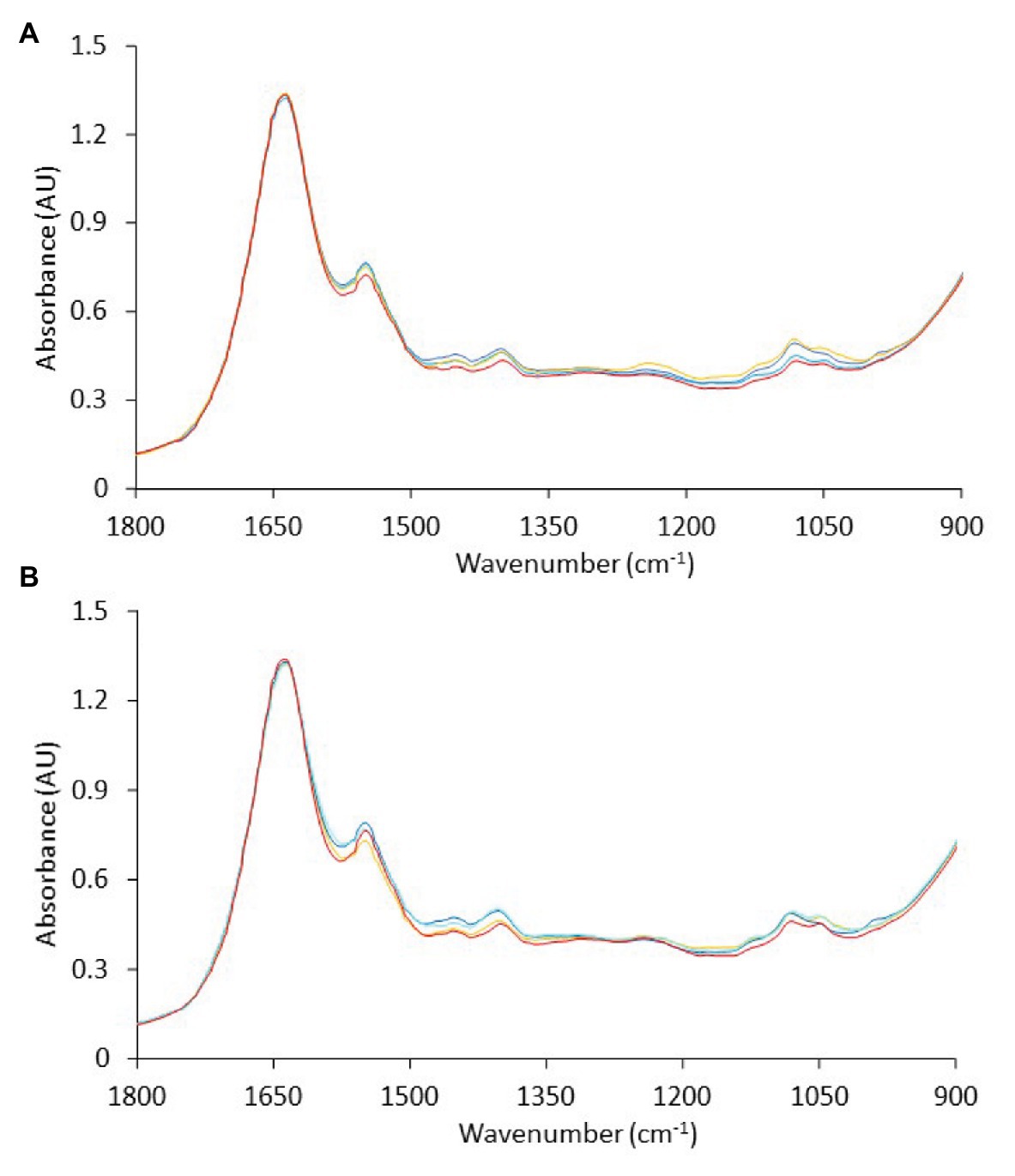
Figure 5. Representative Fourier-transform infrared spectra, in the wavenumber range of 1,800 to 900 cm−1, corresponding to (A) non-inoculated and (B) inoculated-with-Salmonella chicken liver samples at the beginning (, 0 h) and at the end of aerobic storage at 0°C (
, 240 h), 4°C (
, 192 h), and 8°C (
, 128 h).
The spectra presented generally similar patterns, with no profound feature peaks to reflect uniquely the difference in quality between fresh and spoiled chicken liver samples. However, features within this wavenumber area, commonly ascribed to amides and amines, are linked to microbiological and/or autolytic proteolysis of muscle meat proteins occurring during storage (Nychas and Tassou, 1997; Ellis et al., 2002; Alexandrakis et al., 2012; Vasconcelos et al., 2014; Fengou et al., 2019). At this point, it has to be noted that liver samples (fresh and spoiled) exhibited a substantial inter- and intra-batch variability in the approximate wavenumber range from 1,140 to 1,000 cm−1 (Figure 6), an area that is associated mostly with amines from free amino acids and strongly correlated to chicken spoilage (Ellis et al., 2002). Such variability could originate from differences in the intrinsic characteristics of the liver tissue as well as in the bacterial community structure and the associated biochemical changes on the surface of the chicken liver. Consequently, a machine learning approach was employed to analyze the spectra and quantify chicken liver spoilage along with FTIR data.
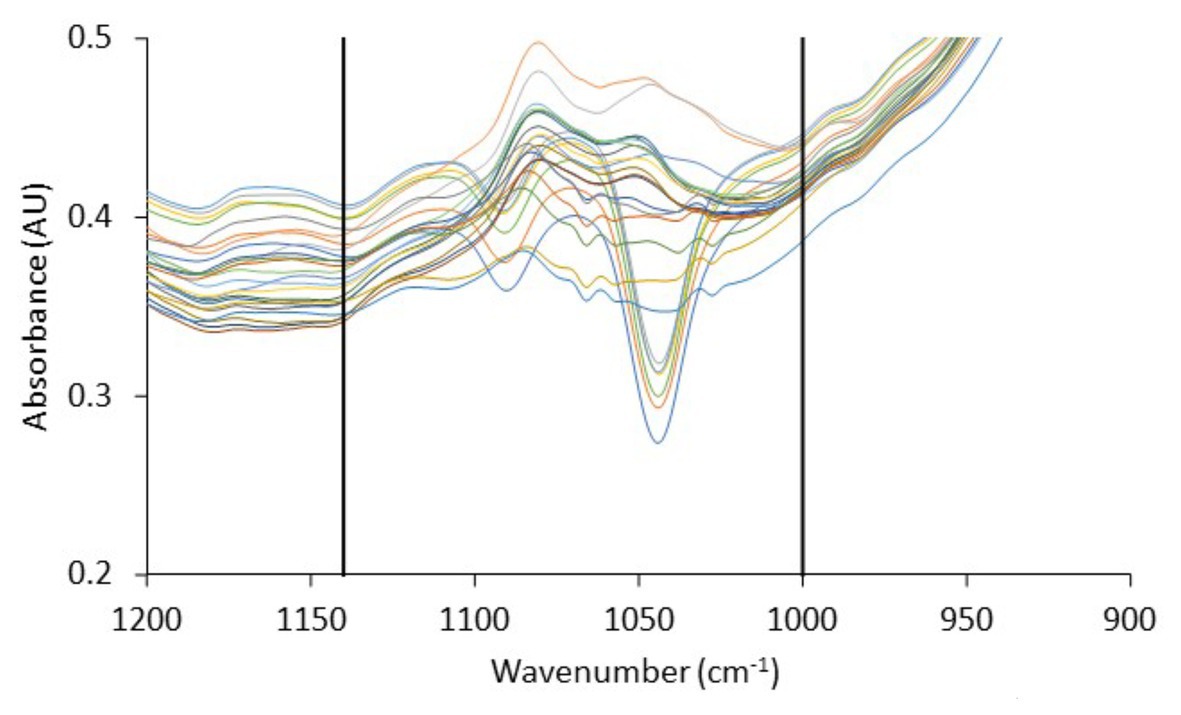
Figure 6. Fourier-transform infrared spectra, in the wavenumber range 1,200–900 cm−1, corresponding to fresh (0 h) chicken liver samples from the different batches used in the current study. Vertical lines delimit the wavenumber range (1,140–1,000 cm−1) in which significant inter- and intra-batch variability is observed.
Estimation of Chicken Liver Spoilage Using FTIR Data
FTIR spectroscopy in tandem with machine learning methodologies has been widely used in the literature for a rapid microbial quality assessment of foods (He and Sun, 2015). A major challenge in the field of machine learning that engineers have to overcome is the high dimensionality of spectral data which may negatively affect the performance of the models (Cai et al., 2018). In this study, to overcome this problem, we introduced an ensemble of feature selection based on extra-trees algorithm to select a subset of relevant and non-redundant features (Geurts et al., 2006). With this approach, features that had no and/or low correlation with the output variables, i.e., microbial populations, were reduced from 900 (wavelength 1,800–900 cm−1) to less than 110 (data not shown). With dimensionality reduction of FTIR data, the robustness and performance of the developed models would increase through the elimination of bias other than microbiological factors (e.g., bias attributed to inherent chicken liver samples and/or batch variability) and prevention of data overfitting (Tsakanikas et al., 2018).
Following dimensionality reduction, SVM radial regression models (Smola and Scholkopf, 2004) were developed for the correlation of spectral data with the population of selected microbial groups, namely, total viable counts, Pseudomonas spp., B. thermosphacta, LAB, Enterobacteriaceae, and Salmonella, on the surface of chicken liver samples. It should be noted that, in an effort to develop robust and realistic models, different sources of variability were incorporated in the analysis beyond the biological aspect (i.e., different batches). These included the biochemical fingerprint of Salmonella (Grewal et al., 2015) potentially present on chicken liver (i.e., datasets from inoculated-with-Salmonella liver alone or in combination with data from non-inoculated samples, in this study) as well as the various storage temperatures encountered in the cold chain [i.e., well-controlled refrigeration (0 and 4°C) to slightly abusive (8°C) and dynamic temperature conditions, in this study] and the resulting swifts in the structure of bacterial communities and microbial metabolites produced (Nychas et al., 2008; Doulgeraki et al., 2012; Tsakanikas et al., 2018, 2020).
The relation between the measured (via microbiological analysis) and the estimated (by the model) microbial populations on chicken liver is illustrated in Figures 7–9. The predictive reliability and accuracy of the developed models for the different bacterial groups in the three datasets, i.e., from non-inoculated, inoculated with Salmonella, and their combination, were evaluated by calculating different statistical metrics (Tables 1–3). In the case of non-inoculated liver samples, the plot of the observed and predicted counts presented positive association and good distribution around the line of equity without any particular trend, with more than 80% of predictions (ranging from 80.49 to 82.52%, depending on the estimated microbial group) within the ±1 log unit area of the actually observed ones (Figure 7, Table 1). The bias factor Bf was generally very close to unity (ranging from 0.995 to 1.022, depending on the microbial group), indicating no structural deviation of the models, i.e., systematic overprediction (Bf > 1) or underprediction (Bf < 1; Table 1; Koutsoumanis et al., 2006; Oscar, 2009). Actually, Bf values within the range of 0.9–1.05 are considered as adequate in model development (Ross, 1999; Ross et al., 2000). In addition, the value of accuracy factor Af indicated that the average deviation between predictions and observations was 8.4% (either below or above the line of equity) for Pseudomonas spp. and Enterobacteriaceae, 8.5% for B. thermosphacta, 8.6% for LAB, and 8.7% for TVC (Table 1). It has been suggested that Af values with an increase 0.15 (15%) are considered as satisfactory in models employing only one variable, which is the case of this study (Ross et al., 2000). The calculated R2 and RMSE metrics were found to be similar for all the microbial groups predicted, ranging from 0.768 to 0.791 and 0.698 to 0.733 log CFU/g, respectively, indicating a good model performance.
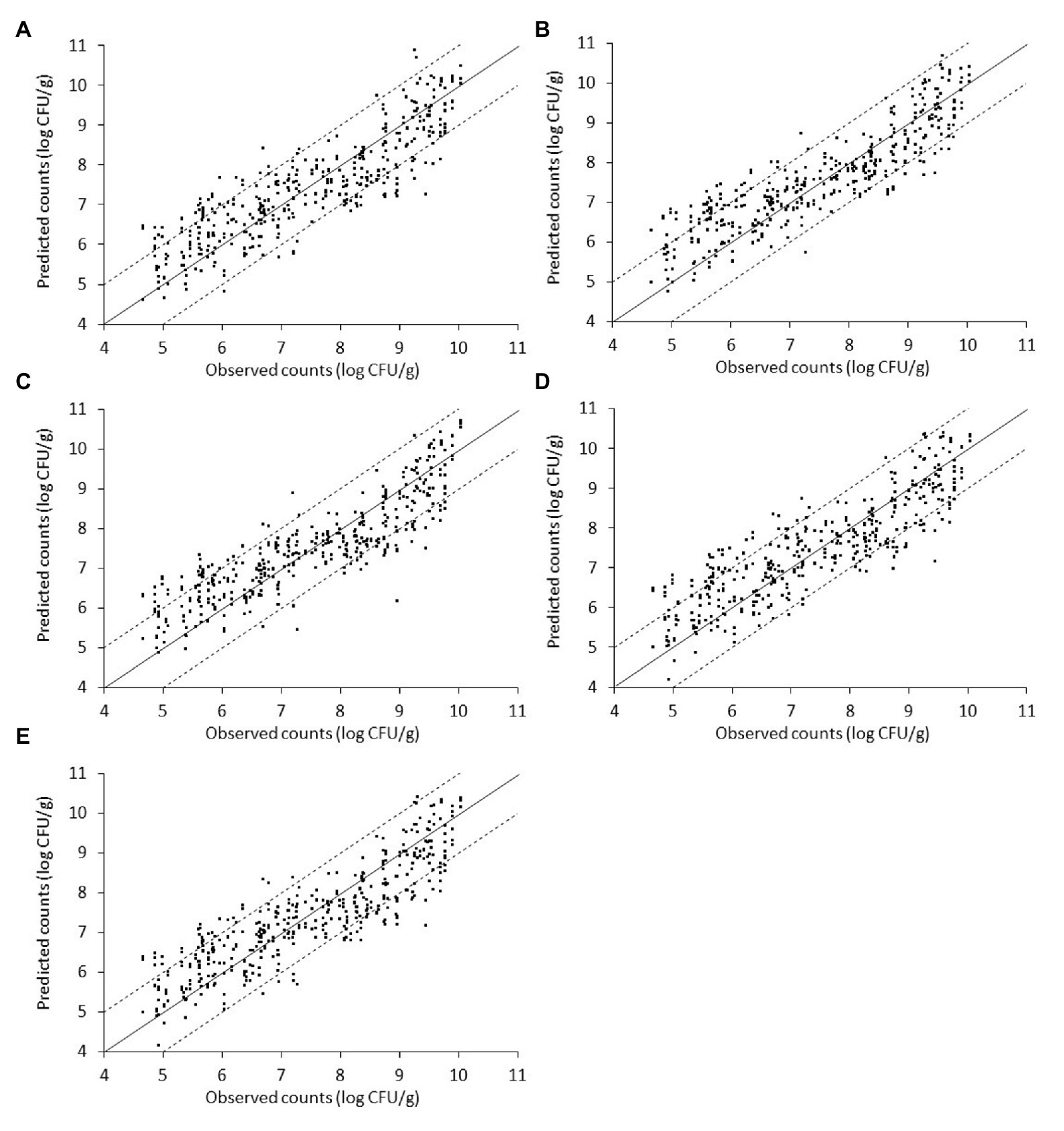
Figure 7. Scatterplot of the microbial populations measured via microbiological analysis and estimated by the support vector machine regression model (external validation) based on Fourier-transform infrared spectra from non-inoculated liver. (A) TVC (B) Pseudomonas spp. (C) Brochothrix thermosphacta (D) lactic acid bacteria, and (E) Enterobacteriaceae (solid line: the ideal y = x line; dashed lines: ±1 log unit area). Data points from 50 iterations are presented.
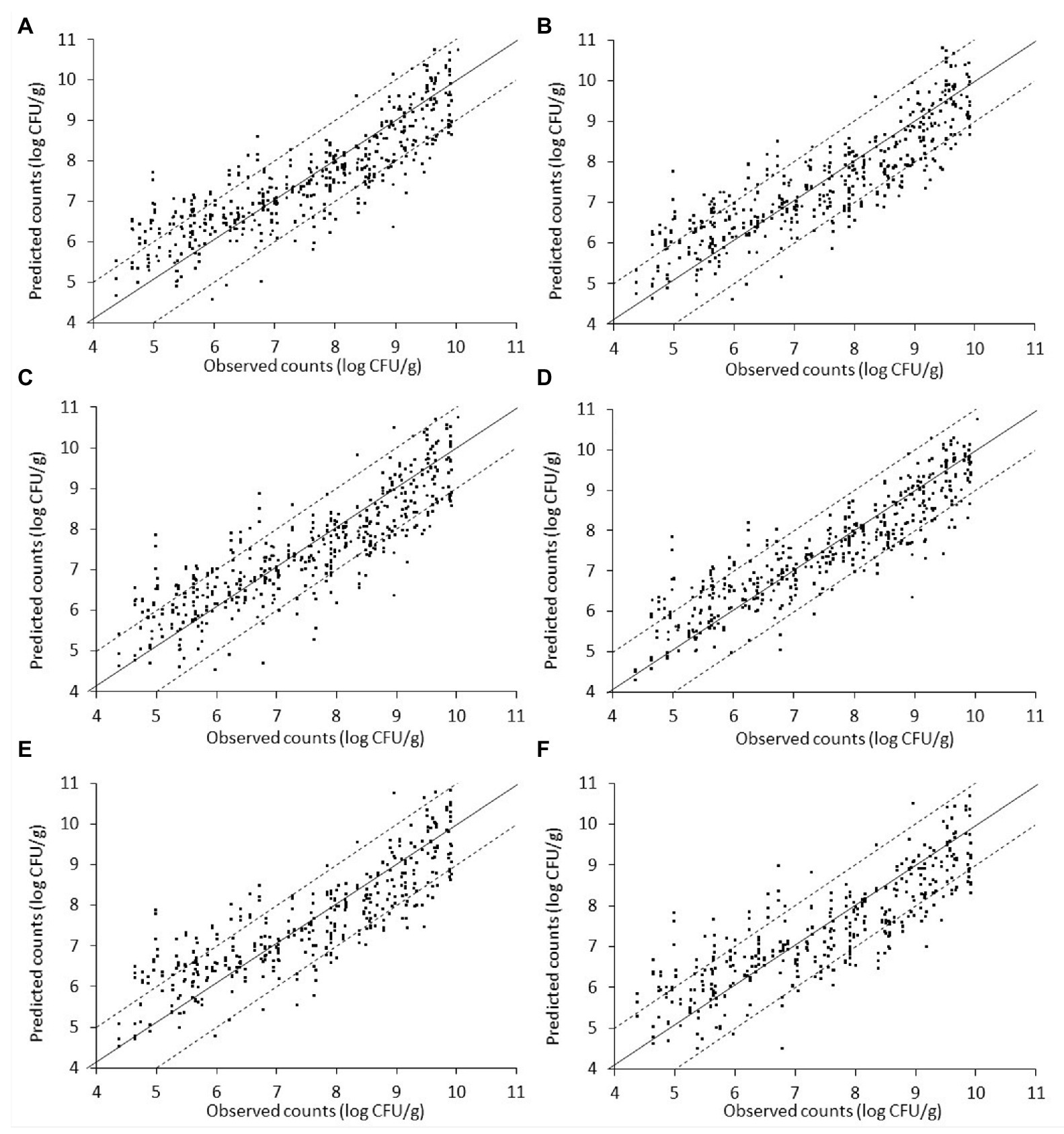
Figure 8. Scatterplot of the microbial populations measured via microbiological analysis and estimated by the support vector machine regression model (external validation) based on Fourier-transform infrared spectra from liver inoculated with Salmonella. (A) TVC (B) Pseudomonas spp. (C) Brochothrix thermosphacta (D) lactic acid bacteria (E) Enterobacteriaceae, and (F) Salmonella (solid line: the ideal y = x line; dashed lines: ±1log unit area). Data points from 50 iterations are presented.
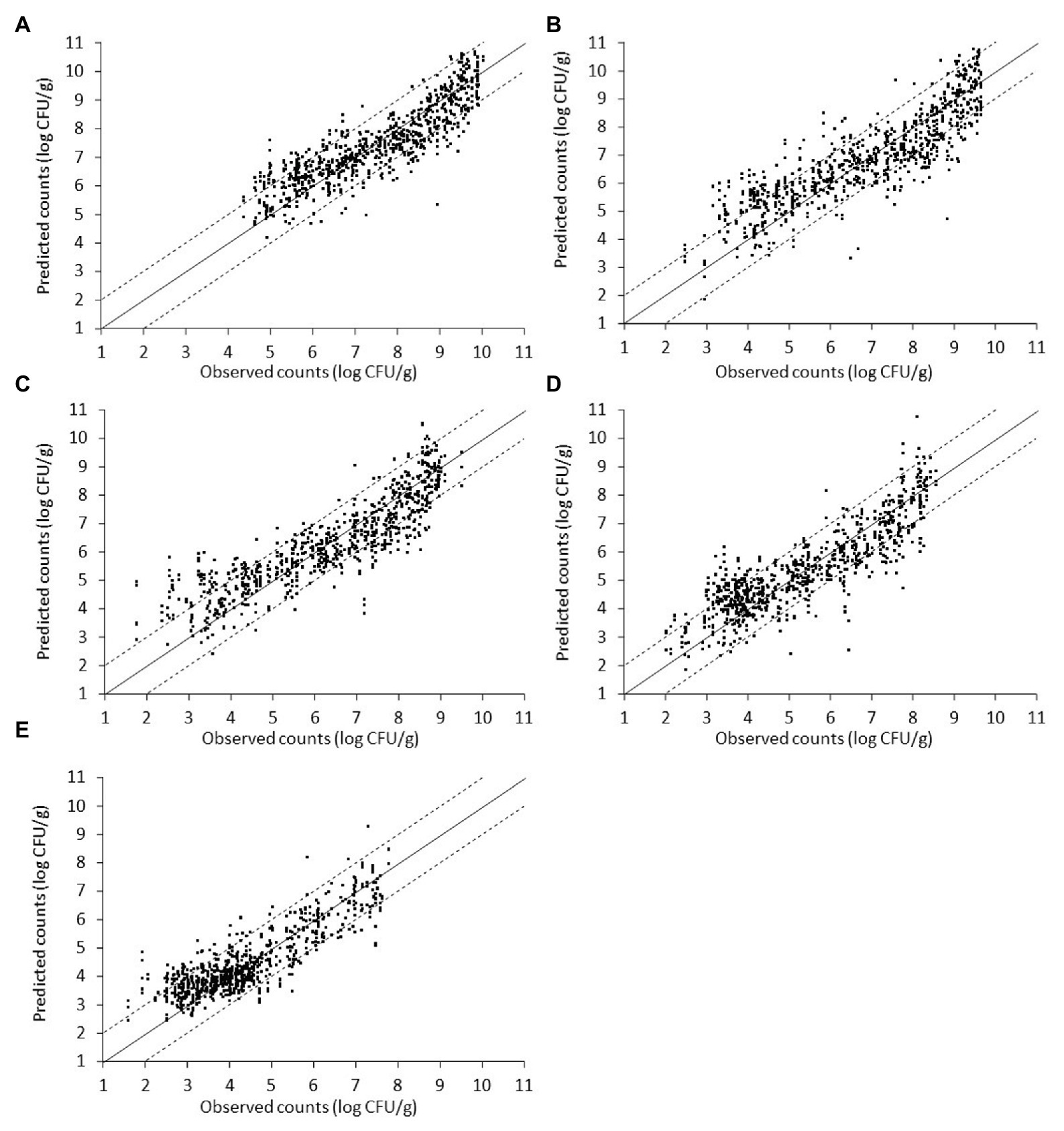
Figure 9. Scatterplot of the microbial populations measured via microbiological analysis and estimated by the support vector machine regression model (external validation) based on combined Fourier-transform infrared spectra (i.e., from non-inoculated and inoculated with Salmonella liver). (A) TVC, (B) Pseudomonas spp., (C) Brochothrix thermosphacta, (D) lactic acid bacteria, and (E) Enterobacteriaceae (solid line: the ideal y = x line; dashed lines: ±1 log unit area). Data points from 50 iterations are presented.
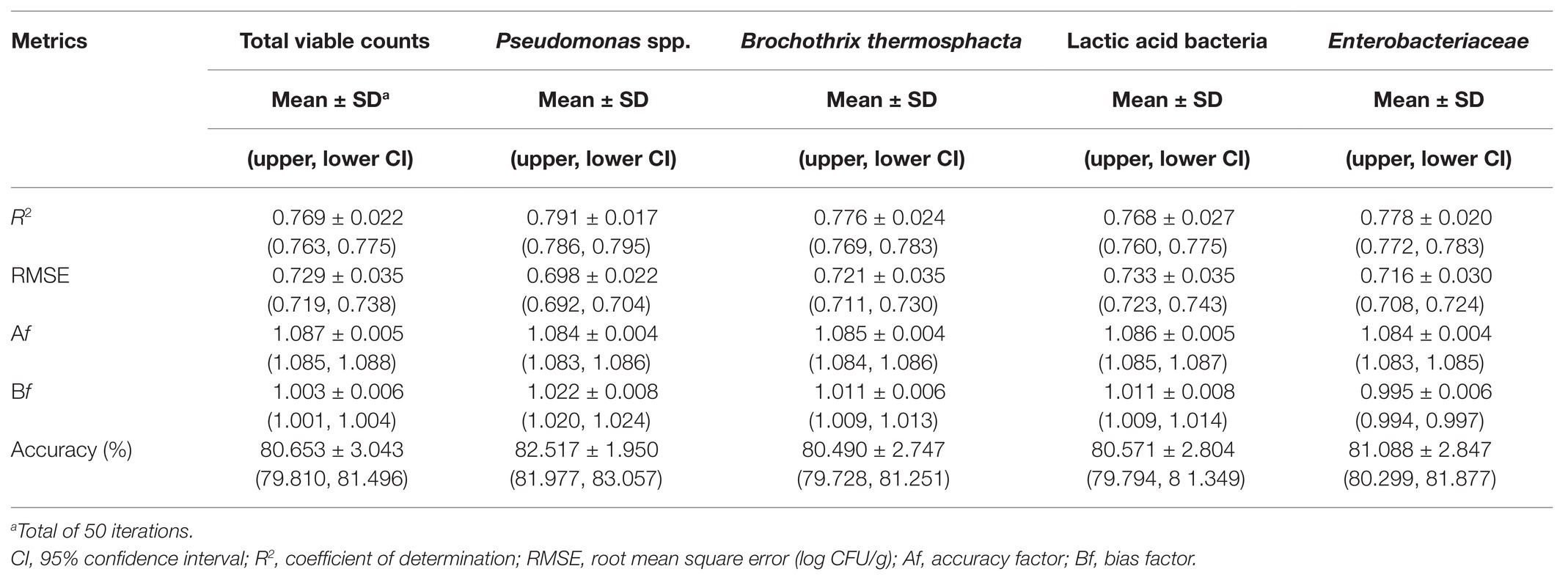
Table 1. Performance metrics of the support vector machine models for the prediction of the different microbial populations of chicken liver (non-inoculated) stored under aerobic conditions at isothermal and dynamically changing conditions.
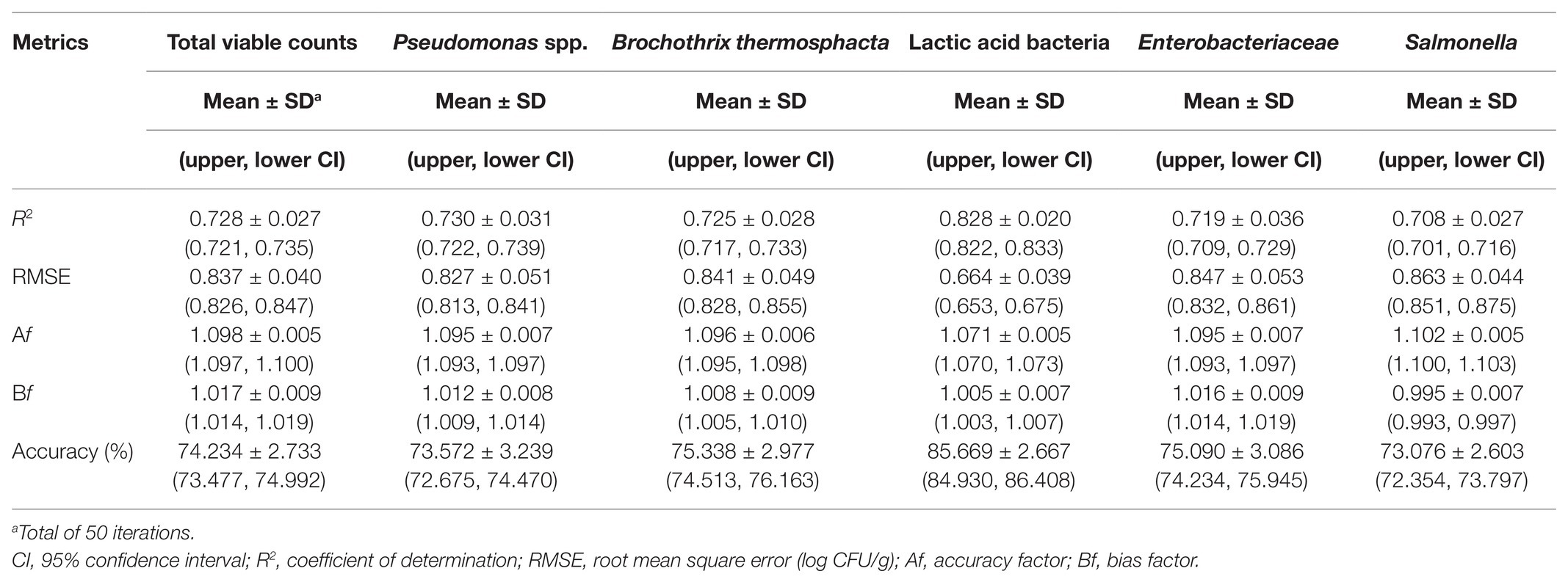
Table 2. Performance metrics of the support vector machine models for the prediction of the different microbial populations of chicken liver inoculated with Salmonella stored under aerobic conditions at isothermal and dynamically changing conditions.
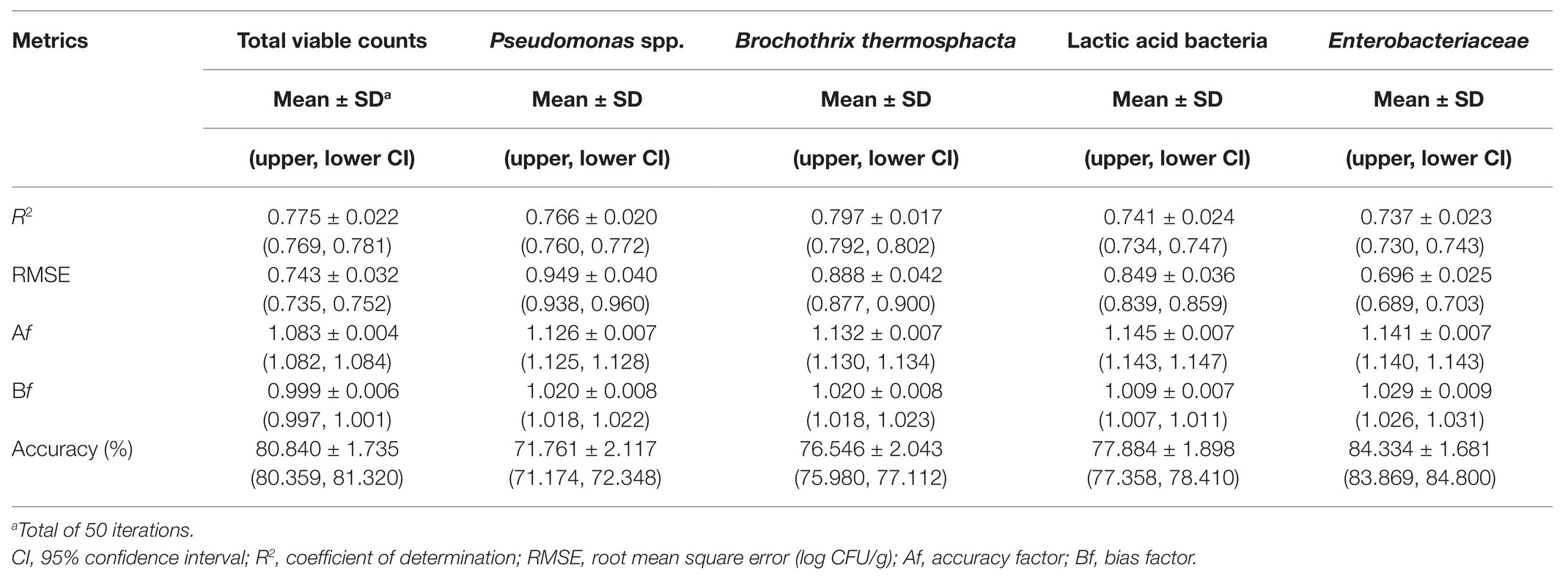
Table 3. Performance metrics of the support vector machine models for the prediction of the different microbial populations of chicken liver based on a combination of spectral data from non-inoculated and inoculated-with-Salmonella chicken liver samples.
The relation between observed and predicted values appeared slightly inferior in the case of inoculated-with-Salmonella samples compared to the non-inoculated samples (Figure 8). However, based on related plots in Figure 8, predictions vs. observations again presented a relatively good distribution around the line of equity, with more than 73% of the data included within the ±1 log unit area. Briefly, accuracy (%) varied from 73.08 to 85.67% in descending order for microbial populations of LAB, B. thermosphacta, Enterobacteriaceae, TVC, Pseudomonas spp., and Salmonella (Figure 8, Table 2). With regards to Bf, analogous results were obtained, with values ranging from 0.995 to 1.017 for the different bacterial groups, signifying an optimum correlation between observed and predicted values (Ross et al., 2000; Argyri et al., 2010). On the other hand, Af was close to 1, indicating that predictions were close to observations, and ranged from 7.1% for LAB to 9.5% for Pseudomonas spp. and Enterobacteriaceae, 9.6% for B. thermosphacta, and 10.2% for Salmonella. The calculated R2 and RMSE values for the different microbial groups predicted ranged from 0.708 to 0.828 and 0.664 to 0.863 log CFU/g, respectively, with a slightly better performance observed in the case of LAB. Models derived from inoculated samples had a comparable performance with models derived from non-inoculated ones.
Finally, spectral and microbiological data from the combination of non-inoculated and inoculated-with-Salmonella samples were employed into the analysis. In this case, the input dataset consisted of a random mixture of non-inoculated and inoculated-with-Salmonella samples in order to evaluate the potential of the developed pipeline in achieving a good prediction accuracy regardless of the presence or absence of the pathogen. Plots of observed vs. predicted microbial counts presented a reasonably good distribution around the equity line, close to that observed for non-inoculated data (Figure 9). As can be visualized from Figure 9 and Table 3, approximately 84.33, 80.84, 77.88, 76.55, and 71.76% of the predicted microbial counts for Enterobacteriaceae, TVC, LAB, B. thermosphacta, and Pseudomonas spp., respectively, were within the ±1 log unit area. The Bf was close to 1 (ranging from 0.999 to 1.029) for all bacterial groups, while Af was 1.083 for TVC, 1.126 for Pseudomonas spp., 1.132 for B. thermosphacta, 1.145 for Enterobacteriaceae, and 1.141 for LAB. The calculated R2 and RMSE values ranged from 0.737 to 0.797 and 0.696 to 0.949 log CFU/g, respectively, suggesting a satisfactory relationship between spectra and the specific spoilage organisms studied.
Available research data on the potential of FTIR spectroscopy to determine the microbiological spoilage on the surface of chicken products are relatively limited. Ellis et al. (2002) employed a partial least squares (PLS) regression model to accurately estimate (RMSE of 0.27 log CFU/g) TVC populations on chicken breasts during storage at room temperature. PLS regression was also carried out by Vasconcelos et al. (2014) to determine specific microorganisms on chicken breast such as Pseudomonas spp., LAB, Enterobacteriaceae, and B. thermosphacta from related FTIR spectra, obtaining R2 of 0.789, 0.832, 0.857, and 0.810, respectively. Slightly less accurate results were observed by Rahman et al. (2018), who developed a PLS model to predict total plate count (TPC) and Enterobacteriaceae on chicken breast surfaces during aerobic refrigerated storage, generating a good performance with R2 being 0.66 for TPC and 0.52 for Enterobacteriaceae, respectively. However, to our knowledge, this is the first study reporting the considerable potential of FTIR spectroscopy in rapid and non-destructive quantitative assessment of microbiological spoilage on chicken liver.
Conclusion
Chicken liver constitutes a highly perishable food commodity due to the relatively high contents of readily available nutrients and water activity that support microbial growth. In this context, the growth of indigenous spoilage microbiota and the behavior of inoculated Salmonella on chicken liver stored aerobically under refrigeration (isothermal and dynamically changing temperatures) were initially investigated. Spoilage was mainly attributed to the presence of Pseudomonas spp. as well as of B. thermosphacta, followed by LAB and Enterobacteriaceae, while the contribution of yeasts/molds was limited. Microbiological spoilage was affected by the storage temperature as well as the inherent and microbiological variability observed on chicken liver (fresh and/or spoiled). Chicken liver supported the survival of inoculated Salmonella at 0°C and, most importantly, its growth at 4 and 8°C, indicating the need for the application of sanitation and safe food handling procedures.
Furthermore, the ability of FTIR spectroscopy to estimate the populations of TVC, Pseudomonas spp., B. thermosphacta, Enterobacteriaceae, LAB, and Salmonella on chicken liver was explored. The proposed pipeline incorporated the inherent (batch) variability of chicken liver samples, the variability of storage temperature (isothermal and dynamically changing temperatures), and the biochemical fingerprint of Salmonella in the event of cross-contamination. The results of the current study outline the efficiency of FTIR spectroscopy in tandem with the described data analysis and model building workflow to satisfactorily describe spoilage on chicken liver.
Data Availability Statement
The raw data supporting the conclusions of this article will be made available by the authors, without undue reservation.
Author Contributions
AA and CT contributed to the conceptualization of this study. The methodology was an equal contribution of DD and AG. DD, AG, and GF conducted the validation. DD, AG, AA, AD, and GF performed the formal analysis. CT and G-JN took charge of the resources. DD, AA, and PT were in charge of data curation. DD and AG were responsible for the original draft preparation. DD, AA, AD, NC, and CT reviewed and edited the manuscript. AA, G-JN, and CT supervised the study. CT was in charge of project administration. All authors contributed to the article and approved the submitted version.
Funding
This research has been financed by the project “A Model Smart Quality Assurance and Safety System for Fresh Poultry Products” (QAPP: T1EDK-04344) that is co-financed by the EU and Greek national funds through the Operational Program Competitiveness, Entrepreneurship and Innovation, RESEARCH–CREATE–INNOVATE.
Conflict of Interest
The authors declare that the research was conducted in the absence of any commercial or financial relationships that could be construed as a potential conflict of interest.
References
Ahmad, R. S., Imran, A., and Hussain, M. B. (2018). “Nutritional composition of meat” in Meat science and nutrition. ed. M. S. Arshad (London, UK: IntechOpen), 61–77.
Airoldi, A. A., and Zottola, E. A. (1988). Growth and survival of Salmonella typhimurium at low temperature in nutrient deficient media. J. Food Sci. 53, 1511–1513. doi: 10.1111/j.1365-2621.1988.tb09311.x
Alexandrakis, D., Downey, G., and Scannell, A. G. M. (2012). Rapid non-destructive detection of spoilage of intact chicken breast muscle using near-infrared and Fourier transform mid-infrared spectroscopy and multivariate statistics. Food Bioprocess Technol. 5, 338–347. doi: 10.1007/s11947-009-0298-4
Álvarez-Astorga, M., Capita, R., Alonso-Calleja, C., Moreno, B., and García-Fernández, M. d. C. (2002). Microbiological quality of retail chicken by-products in Spain. Meat Sci. 62, 45–50. doi: 10.1016/S0309-1740(01)00225-X
Ammor, M. S., Argyri, A., and Nychas, G. J. E. (2009). Rapid monitoring of the spoilage of minced beef stored under conventionally and active packaging conditions using Fourier transform infrared spectroscopy in tandem with chemometrics. Meat Sci. 81, 507–514. doi: 10.1016/j.meatsci.2008.10.015
Argyri, A. A., Jarvis, R. M., Wedge, D., Xu, Y., Panagou, E. Z., Goodacre, R., et al. (2013). A comparison of Raman and FT-IR spectroscopy for the prediction of meat spoilage. Food Control 29, 461–470. doi: 10.1016/j.foodcont.2012.05.040
Argyri, A. A., Panagou, E. Z., and Nychas, G. J. (2014). “Monitoring microbial spoilage of foods by vibrational spectroscopy (FT-IR and raman)” in Novel food preservation and microbial assessment techniques. ed. I. S. Boziaris (Boca Raton, FL, USA: CRC Press), 386–434.
Argyri, A. A., Panagou, E. Z., Tarantilis, P. A., Polysiou, M., and Nychas, G. J. E. (2010). Rapid qualitative and quantitative detection of beef fillets spoilage based on Fourier transform infrared spectroscopy data and artificial neural networks. Sensors Actuators B Chem. 145, 146–154. doi: 10.1016/j.snb.2009.11.052
Augère-Granier, M. -L. (2019). The EU poultry meat and egg sector - Main features, challenges and prospects. EPRS-European Parliam. Res. Serv. doi: 10.2861/33350
Baker, R. C., Qureshi, R. A., and Hotchkiss, J. H. (1986). Effect of an elevated level of carbon dioxide containing atmosphere on the growth of spoilage and pathogenic bacteria at 2, 7, and 13°C. Poult. Sci. 65, 729–737. doi: 10.3382/ps.0650729
Balamatsia, C. C., Patsias, A., Kontominas, M. G., and Savvaidis, I. N. (2007). Possible role of volatile amines as quality-indicating metabolites in modified atmosphere-packaged chicken fillets: correlation with microbiological and sensory attributes. Food Chem. 104, 1622–1628. doi: 10.1016/j.foodchem.2007.03.013
Böcker, U., Ofstad, R., Wu, Z., Bertram, H. C., Sockalingum, G. D., Manfait, M., et al. (2007). Revealing covariance structures in Fourier transform infrared and Raman microspectroscopy spectra: a study on pork muscle fiber tissue subjected to different processing parameters. Appl. Spectrosc. 61, 1032–1039. doi: 10.1366/000370207782217707
Cai, J., Luo, J., Wang, S., and Yang, S. (2018). Feature selection in machine learning: a new perspective. Neurocomputing 300, 70–79. doi: 10.1016/j.neucom.2017.11.077
Catsaras, M., and Grebot, D. (1985). Multiplication des Salmonella dans la viande hachée. Bull. Acad. Vet. Fr. 58, 413–421. doi: 10.4267/2042/65093
Custódio, F. B., Theodoro, K. H., and Gloria, M. B. A. (2016). Bioactive amines in fresh beef liver and influence of refrigerated storage and pan-roasting. Food Control 60, 151–157. doi: 10.1016/j.foodcont.2015.07.037
D’Aoust, J. Y. (1991). Psychrotrophy and foodborne Salmonella. Int. J. Food Microbiol. 13, 207–215. doi: 10.1016/0168-1605(91)90004-9
Devatkal, S., Mendiratta, S. K., Kondaiah, N., Sharma, M. C., and Anjaneyulu, A. S. R. (2004). Physicochemical, functional and microbiological quality of buffalo liver. Meat Sci. 68, 79–86. doi: 10.1016/j.meatsci.2004.02.006
Dillon, V. M., and Board, R. G. (1991). Yeasts associated with red meats. J. Appl. Bacteriol. 71, 93–108. doi: 10.1111/j.1365-2672.1991.tb02962.x
Doulgeraki, A. I., Ercolini, D., Villani, F., and Nychas, G. J. E. (2012). Spoilage microbiota associated to the storage of raw meat in different conditions. Int. J. Food Microbiol. 157, 130–141. doi: 10.1016/j.ijfoodmicro.2012.05.020
Doulgeraki, A. I., and Nychas, G. J. E. (2013). Monitoring the succession of the biota grown on a selective medium for pseudomonads during storage of minced beef with molecular-based methods. Food Microbiol. 34, 62–69. doi: 10.1016/j.fm.2012.11.017
Doulgeraki, A. I., Paramithiotis, S., Kagkli, D. M., and Nychas, G. J. E. (2010). Lactic acid bacteria population dynamics during minced beef storage under aerobic or modified atmosphere packaging conditions. Food Microbiol. 27, 1028–1034. doi: 10.1016/j.fm.2010.07.004
Du, Z., Jeong, M. K., and Kong, S. G. (2007). Band selection of hyperspectral images for automatic detection of poultry skin tumors. IEEE Trans. Autom. Sci. Eng. 4, 332–339. doi: 10.1109/TASE.2006.888048
EFSA (European Food Safety Authority) and ECDC (European Centre for Disease Prevention and Control) (2017). The European Union summary report on trends and sources of zoonoses, zoonotic agents and food-borne outbreaks in 2016. EFSA J. 15, 1–228. doi: 10.2903/j.efsa.2017.5077
EFSA Panel on Biological Hazards (EFSA BIOHAZ Panel) Koutsoumanis, K., Allende, A., Alvarez-Ordóñez, A., Bolton, D., Bover-Cid, S., et al. (2019). Salmonella control in poultry flocks and its public health impact. EFSA J. 17:5596. doi: 10.2903/j.efsa.2019.5596
Ellis, D. I., Broadhurst, D., and Goodacre, R. (2004). Rapid and quantitative detection of the microbial spoilage of beef by Fourier transform infrared spectroscopy and machine learning. Anal. Chim. Acta 514, 193–201. doi: 10.1016/j.aca.2004.03.060
Ellis, D. I., Broadhurst, D., Kell, D. B., Rowland, J. J., and Goodacre, R. (2002). Rapid and quantitative detection of the microbial spoilage of meat by fourier transform infrared spectroscopy and machine learning. Appl. Environ. Microbiol. 68, 2822–2828. doi: 10.1128/AEM.68.6.2822-2828.2002
Ercolini, D., Ferrocino, I., Nasi, A., Ndagijimana, M., Vernocchi, P., La Storia, A., et al. (2011). Monitoring of microbial metabolites and bacterial diversity in beef stored under different packaging conditions. Appl. Environ. Microbiol. 77, 7372–7381. doi: 10.1128/AEM.05521-11
Estelles-Lopez, L., Ropodi, A., Pavlidis, D., Fotopoulou, J., Gkousari, C., Peyrodie, A., et al. (2017). An automated ranking platform for machine learning regression models for meat spoilage prediction using multi-spectral imaging and metabolic profiling. Food Res. Int. 99, 206–215. doi: 10.1016/j.foodres.2017.05.013
European Commission (2005). Commission regulation (EC) no 2073/2005 of November 2005 on microbiological criteria for foodstuffs. Off. J. Eur. Union L 338, 1–26.
Fengou, L. C., Mporas, I., Spyrelli, E., Lianou, A., and Nychas, G. J. (2020). Estimation of the microbiological quality of meat using rapid and non-invasive spectroscopic sensors. IEEE Access 8, 106614–106628. doi: 10.1109/ACCESS.2020.3000690
Fengou, L. C., Spyrelli, E., Lianou, A., Tsakanikas, P., Panagou, E. Z., and Nychas, G. J. E. (2019). Estimation of minced pork microbiological spoilage through Fourier transform infrared and visible spectroscopy and multispectral vision technology. Foods 8:238. doi: 10.3390/foods8070238
Fernández-López, J., Yelo, A., Sayas-Barberá, E., Sendra, E., Navarro, C., and Pérez-Alvarez, J. A. (2006). Shelf life of ostrich (Struthio camelus) liver stored under different packaging conditions. J. Food Prot. 69, 1920–1927. doi: 10.4315/0362-028X-69.8.1920
Galarz, L. A., Fonseca, G. G., and Prentice, C. (2016). Predicting bacterial growth in raw, salted, and cooked chicken breast fillets during storage. Food Sci. Technol. Int. 22, 461–474. doi: 10.1177/1082013215618519
Geurts, P., Ernst, D., and Wehenkel, L. (2006). Extremely randomized trees. Mach. Learn. 63, 3–42. doi: 10.1007/s10994-006-6226-1
Gill, C. O., and DeLacy, K. M. (1982). Microbial spoilage of whole sheep livers. Appl. Environ. Microbiol. 43, 1262–1266. doi: 10.1128/AEM.43.6.1262-1266.1982
Grewal, M. K., Jaiswal, P., and Jha, S. N. (2015). Detection of poultry meat specific bacteria using FTIR spectroscopy and chemometrics. J. Food Sci. Technol. 52, 3859–3869. doi: 10.1007/s13197-014-1457-9
Gribble, A., and Brightwell, G. (2013). Spoilage characteristics of Brochothrix thermosphacta and campestris in chilled vacuum packaged lamb, and their detection and identification by real time PCR. Meat Sci. 94, 361–368. doi: 10.1016/j.meatsci.2013.03.016
Guo, Q., Wu, W., and Massart, D. L. (1999). The robust normal variate transform for pattern recognition with near-infrared data. Anal. Chim. Acta 382, 87–103. doi: 10.1016/S0003-2670(98)00737-5
Hanna, M. O., Smith, G. C., Savell, J. W., McKeith, F. K., and Vanderzant, C. (1982). Effects of packaging methods on the microbial flora of livers and kidneys from beef or pork. J. Food Prot. 45, 74–81. doi: 10.4315/0362-028X-45.1.74
Hasapidou, A., and Savvaidis, I. N. (2011). The effects of modified atmosphere packaging, EDTA and oregano oil on the quality of chicken liver meat. Food Res. Int. 44, 2751–2756. doi: 10.1016/j.foodres.2011.06.011
He, H. J., and Sun, D. W. (2015). Microbial evaluation of raw and processed food products by visible/infrared, Raman and fluorescence spectroscopy. Trends Food Sci. Technol. 46, 199–210. doi: 10.1016/j.jpgs.2015.10.004
Hernández-Herrero, M. M., Roig-Sagués, A. X., López-Sabater, E. I., Rodríguez-Jerez, J. J., and Mora-Ventura, M. T. (1999). Influence of storage temperature on the quality of beef liver; pH as a reliable indicator of beef liver spoilage. J. Sci. Food Agric. 79, 2035–2039. doi: 10.1002/(SICI)1097-0010(199911)79:14<2035::AID-JSFA481>3.0.CO;2-I
Hoaglin, D. C., Mosteller, F., and Tukey, J. W. (2000). Understanding robust and exploratory data analysis. New York: Wiley.
Huis In’t Veld, J. H. J. (1996). Microbial and biochemical spoilage of foods: an overview. Int. J. Food Microbiol. 33, 1–18. doi: 10.1016/0168-1605(96)01139-7
Ismail, S. A. S., Deak, T., Abd El-Rahman, H. A., Yassien, M. A. M., and Beuchat, L. R. (2000). Presence and changes in populations of yeasts on raw and processed poultry products stored at refrigeration temperature. Int. J. Food Microbiol. 62, 113–121. doi: 10.1016/S0168-1605(00)00414-1
Jokanović, M. R., Tomović, V. M., Jović, M. T., Škaljać, S. B., Šojić, B. V., Ikonić, P. M., et al. (2014). Proximate and mineral composition of chicken giblets from Vojvodina (Northern Serbia). Int. J. Nutr. Food Eng. 8, 986–989. doi: 10.5281/zenodo.1094787
Jung, Y., Porto-Fett, A. C. S., Shoyer, B. A., Henry, E., Shane, L. E., Osoria, M., et al. (2019). Prevalence, levels, and viability of Salmonella in and on raw chicken livers. J. Food Prot. 82, 834–843. doi: 10.4315/0362-028X.JFP-18-430
Kakouri, A., and Nychas, G. J. E. (1994). Storage of poultry meat under modified atmospheres or vacuum packs: possible role of microbial metabolites as indicator of spoilage. J. Appl. Bacteriol. 76, 163–172. doi: 10.1111/j.1365-2672.1994.tb01612.x
Keshavarzi, Z., Barzegari Banadkoki, S., Faizi, M., Zolghadri, Y., and Shirazi, F. H. (2020). Comparison of transmission FTIR and ATR spectra for discrimination between beef and chicken meat and quantification of chicken in beef meat mixture using ATR-FTIR combined with chemometrics. J. Food Sci. Technol. 57, 1430–1438. doi: 10.1007/s13197-019-04178-7
Kim, B. Y., Choi, S. Y., Seo, K. Y., and Do Ha, S.. (2011). Temperature dependent growth characteristics and a predictive mathematical model of Salmonella enterica Typhimurium in Kimbab. J. Appl. Biol. Chem. 54, 454–459. doi: 10.3839/jksabc.2011.070
Kinsella, K. J., Rowe, T. A., Blair, I. S., McDowell, D. A., and Sheridan, J. J. (2007). The influence of attachment to beef surfaces on the survival of cells of Salmonella enterica serovar Typhimurium DT104, at different aw values and at low storage temperatures. Food Microbiol. 24, 786–793. doi: 10.1016/j.fm.2006.12.004
Koutsoumanis, K., Stamatiou, A., Skandamis, P., and Nychas, G. -J. E. (2006). Development of a microbial model for the combined effect of temperature and pH on spoilage of ground meat, and validation of the model under dynamic temperature conditions. Appl. Environ. Microbiol. 72, 124–134. doi: 10.1128/AEM.72.1.124-134.2006
Lanier, W. A., Hale, K. R., Geissler, A. L., and Dewey-Mattia, D. (2018). Chicken liver-associated outbreaks of campylobacteriosis and salmonellosis, United States, 2000-2016: identifying opportunities for prevention. Foodborne Pathog. Dis. 15, 726–733. doi: 10.1089/fpd.2018.2489
Lerasle, M., Guillou, S., Simonin, H., Anthoine, V., Chéret, R., Federighi, M., et al. (2014). Assessment of Salmonella and Listeria monocytogenes level in ready-to-cook poultry meat: effect of various high pressure treatments and potassium lactate concentrations. Int. J. Food Microbiol. 186, 74–83. doi: 10.1016/j.ijfoodmicro.2014.06.019
Luong, N. -D. M., Coroller, L., Zagorec, M., Membré, J. -M., and Guillou, S. (2020). Spoilage of chilled fresh meat products during storage: a quantitative analysis of literature data. Microorganisms 8:1198. doi: 10.3390/microorganisms8081198
Lytou, A. E., Nychas, G. -J. E., and Panagou, E. Z. (2018). Effect of pomegranate based marinades on the microbiological, chemical and sensory quality of chicken meat: a metabolomics approach. Int. J. Food Microbiol. 267, 42–53. doi: 10.1016/j.ijfoodmicro.2017.12.023
Mellor, G. E., Bentley, J. A., and Dykes, G. A. (2011). Evidence for a role of biosurfactants produced by Pseudomonas fluorescens in the spoilage of fresh aerobically stored chicken meat. Food Microbiol. 28, 1101–1104. doi: 10.1016/j.fm.2011.02.003
Mikš-Krajnik, M., Yoon, Y. J., Ukuku, D. O., and Yuk, H. G. (2016). Identification and quantification of volatile chemical spoilage indexes associated with bacterial growth dynamics in aerobically stored chicken. J. Food Sci. 81, M2006–M2014. doi: 10.1111/1750-3841.13371
Mohareb, F., Papadopoulou, O., Panagou, E., Nychas, G. -J., and Bessant, C. (2016). Ensemble-based support vector machine classifiers as an efficient tool for quality assessment of beef fillets from electronic nose data. Anal. Methods 8, 3711–3721. doi: 10.1039/C6AY00147E
Nychas, G. -J. E., Panagou, E. Z., and Mohareb, F. (2016). Novel approaches for food safety management and communication. Curr. Opin. Food Sci. 12, 13–20. doi: 10.1016/j.cofs.2016.06.005
Nychas, G. -J. E., Skandamis, P. N., Tassou, C. C., and Koutsoumanis, K. P. (2008). Meat spoilage during distribution. Meat Sci. 78, 77–89. doi: 10.1016/j.meatsci.2007.06.020
Nychas, G. -J. E., and Tassou, C. C. (1997). Spoilage processes and proteolysis in chicken as detected by HPLC. J. Sci. Food Agric. 74, 199–208. doi: 10.1002/(SICI)1097-0010(199706)74:2<199::AID-JSFA790>3.0.CO;2-4
Odeyemi, O. A., Alegbeleye, O. O., Strateva, M., and Stratev, D. (2020). Understanding spoilage microbial community and spoilage mechanisms in foods of animal origin. Compr. Rev. Food Sci. Food Saf. 19, 311–331. doi: 10.1111/1541-4337.12526
Oscar, T. P. (2007). Predictive models for growth of Salmonella Typhimurium DT104 from low and high initial density on ground chicken with a natural microflora. Food Microbiol. 24, 640–651. doi: 10.1016/j.fm.2006.11.003
Oscar, T. P. (2009). Predictive model for survival and growth of Salmonella Typhimurium DT104 on chicken skin during temperature abuse. J. Food Prot. 72, 304–314. doi: 10.4315/0362-028X-72.2.304
Oscar, T. P. (2011). Development and validation of a predictive microbiology model for survival and growth of Salmonella on chicken stored at 4 to 12°C. J. Food Prot. 74, 279–284. doi: 10.4315/0362-028X.JFP-10-314
Panagou, E. Z., Mohareb, F. R., Argyri, A. A., Bessant, C. M., and Nychas, G. -J. E. (2011). A comparison of artificial neural networks and partial least squares modelling for the rapid detection of the microbial spoilage of beef fillets based on Fourier transform infrared spectral fingerprints. Food Microbiol. 28, 782–790. doi: 10.1016/j.fm.2010.05.014
Papadopoulou, O., Panagou, E. Z., Tassou, C. C., and Nychas, G. -J. E. (2011). Contribution of Fourier transform infrared (FTIR) spectroscopy data on the quantitative determination of minced pork meat spoilage. Food Res. Int. 44, 3264–3271. doi: 10.1016/j.foodres.2011.09.012
Papazoglou, S., Tsiraki, M., and Savvaidis, I. N. (2012). Effect of thyme oil on the preservation of vacuum-packaged chicken liver. J. Food Sci. 77, 473–480. doi: 10.1111/j.1750-3841.2012.02823.x
Pavli, F., Argyri, A. A., Nychas, G. -J. E., Tassou, C., and Chorianopoulos, N. (2018). Use of Fourier transform infrared spectroscopy for monitoring the shelf life of ham slices packed with probiotic supplemented edible films after treatment with high pressure processing. Food Res. Int. 106, 1061–1068. doi: 10.1016/j.foodres.2017.12.064
Pedregosa, F., Varoquaux, G., Gramfort, A., Michel, V., Thirion, B., Grisel, O., et al. (2011). Scikit-learn: machine learning in python. J. Mach. Learn. Res. 12, 2825–2830.
Pennacchia, C., Ercolini, D., and Villani, F. (2011). Spoilage-related microbiota associated with chilled beef stored in air or vacuum pack. Food Microbiol. 28, 84–93. doi: 10.1016/j.fm.2010.08.010
Pereira, P. M. d. C. C., and Vicente, A. F. d. R. B. (2013). Meat nutritional composition and nutritive role in the human diet. Meat Sci. 93, 586–592. doi: 10.1016/j.meatsci.2012.09.018
Probst, Y. (2009). Nutrient composition of chicken meat. RIRDC Publication No. 08/210. Available at: https://www.agrifutures.com.au/wp-content/uploads/publications/08-210.pdf (Accessed August 27, 2020).
Procura, F., Bueno, D. J., Bruno, S. B., and Rogé, A. D. (2019). Prevalence, antimicrobial resistance profile and comparison of methods for the isolation of Salmonella in chicken liver from Argentina. Food Res. Int. 119, 541–546. doi: 10.1016/j.foodres.2017.08.008
Rahman, U. u., Sahar, A., Pasha, I., ur Rahman, S., and Ishaq, A. (2018). Assessing the capability of Fourier transform infrared spectroscopy in tandem with chemometric analysis for predicting poultry meat spoilage. PeerJ 6:e5376. doi: 10.7717/peerj.5376
Rateni, G., Dario, P., and Cavallo, F. (2017). Smartphone-based food diagnostic technologies: a review. Sensors 17:1453. doi: 10.3390/s17061453
Rhoades, J., Kargiotou, C., Katsanidis, E., and Koutsoumanis, K. P. (2013). Use of marination for controlling Salmonella enterica and Listeria monocytogenes in raw beef. Food Microbiol. 36, 248–253. doi: 10.1016/j.fm.2013.06.010
Rivas, T., Herrera, A., and Yanguela, J. (1992). Microbial and organoleptic qualities of lamb liver during storage at 0 or 3°C. J. Food Prot. 55, 874–879. doi: 10.4315/0362-028X-55.11.874
Ropodi, A. I., Panagou, E. Z., and Nychas, G. -J. E. (2016). Data mining derived from food analyses using non-invasive/non-destructive analytical techniques; determination of food authenticity, quality and safety in tandem with computer science disciplines. Trends Food Sci. Technol. 50, 11–25. doi: 10.1016/j.jpgs.2016.01.011
Ropodi, A. I., Panagou, E. Z., and Nychas, G. -J. E. (2018). Rapid detection of frozen-then-thawed minced beef using multispectral imaging and Fourier transform infrared spectroscopy. Meat Sci. 135, 142–147. doi: 10.1016/j.meatsci.2017.09.016
Ross, T. (1996). Indices for performance evaluation of predictive models in food microbiology. J. Appl. Bacteriol. 81, 501–508. doi: 10.1111/j.1365-2672.1996.tb03539.x
Ross, T. (1999). Predictive food microbiology models in the meat industry. Sydney, Australia: Meat and livestock Australia.
Ross, T., Dalgaard, P., and Tienungoon, S. (2000). Predictive modelling of the growth and survival of Listeria in fishery products. Int. J. Food Microbiol. 62, 231–245. doi: 10.1016/S0168-1605(00)00340-8
Rouger, A., Remenant, B., Prévost, H., and Zagorec, M. (2017a). A method to isolate bacterial communities and characterize ecosystems from food products: validation and utilization in as a reproducible chicken meat model. Int. J. Food Microbiol. 247, 38–47. doi: 10.1016/j.ijfoodmicro.2016.04.028
Rouger, A., Tresse, O., and Zagorec, M. (2017b). Bacterial contaminants of poultry meat: sources, species, and dynamics. Microorganisms 5:50. doi: 10.3390/microorganisms5030050
Saenz-García, C. E., Castañeda-Serrano, P., Mercado Silva, E. M., Alvarado, C. Z., and Nava, G. M. (2020). Insights into the identification of the specific spoilage organisms in chicken meat. Foods 9:225. doi: 10.3390/foods9020225
Sahar, A., and Dufour, É. (2014). Use of Fourier transform-infrared spectroscopy to predict spoilage bacteria on aerobically stored chicken breast fillets. LWT-Food Sci. Technol. 56, 315–320. doi: 10.1016/j.lwt.2013.12.009
Schmutzler, M., Beganovic, A., Böhler, G., and Huck, C. W. (2015). Methods for detection of pork adulteration in veal product based on FT-NIR spectroscopy for laboratory, industrial and on-site analysis. Food Control 57, 258–267. doi: 10.1016/j.foodcont.2015.04.019
Seong, P. N., Cho, S. H., Park, K. M., Kang, G. H., Park, B. Y., Moon, S. S., et al. (2015). Characterization of chicken by-products by mean of proximate and nutritional compositions. Korean J. Food Sci. Anim. Resour. 35, 179–188. doi: 10.5851/kosfa.2015.35.2.179
Shelef, L. A. (1975). Microbial spoilage of fresh refrigerated beef liver. J. Appl. Bacteriol. 39, 273–280. doi: 10.1111/j.1365-2672.1975.tb00572.x
Shenoda, G. M. H., Darwish, M. I. S., Saleh, S. M. A., and Khalifa, H. A. A. (2019). Carcass characteristics and nutritional composition of some edible chicken by-products. Egypt J. Food Sci. 47, 81–90. doi: 10.21608/ejfs.2019.16364.1018
Silva, M. V. da. (2013). Poultry and poultry products - risks for human health. Poult. Dev. Rev. 11–21. Available at: http://www.fao.org/docrep/019/i3531e/i3531e03.pdf (Accessed September 5, 2020).
Silva, R. O., Rouxinol, M. I. F. C., and Patarata, L. A. S. C. (2020). Pork liver freshness evaluated through spoilage microbiota and a consumer test in shelf life extension experiment. J. Food Qual. 2020:1092865. doi: 10.1155/2020/1092865
Silva, S. Q., Santos, M. T., dos Paes, S. A., and Vanetti, M. C. D. (2016). Acid and low temperature treatments on Salmonella Enteritidis inoculated in pork and its subsequent survival in simulated gastric fluid. Ciência Rural 46, 530–535. doi: 10.1590/0103-8478cr20141582
Smadi, H., Sargeant, J. M., Shannon, H. S., and Raina, P. (2012). Growth and inactivation of Salmonella at low refrigerated storage temperatures and thermal inactivation on raw chicken meat and laboratory media: mixed effect meta-analysis. J. Epidemiol. Glob. Health 2, 165–179. doi: 10.1016/j.jegh.2012.12.001
Smola, A. J., and Scholkopf, B. (2004). A tutorial on support vector regression. Stat. Comput. 14, 199–222. doi: 10.1023/B:STCO.0000035301.49549.88
Socrates, G. (2001). Infrared and Raman characteristic group frequencies: Tables and charts. 3rd Edn. Chichester: Willey.
Torrione, P., Collins, L. M., and Morton, K. D. (2014). “Multivariate analysis, chemometrics, and machine learning in laser spectroscopy” in Laser spectroscopy for sensing: Fundamentals, techniques and applications. ed. M. Baudelet (Cambridge, UK: Woodhead Publishing Limited), 125–164.
Tougan, P. U., Dahouda, M., Salifou, C. F. A., Ahounou Ahounou, S. G., Kpodekon, M. T., Mensah, G. A., et al. (2013). Conversion of chicken muscle to meat and factors affecting chicken meat quality: a review. Int. J. Agron. Agric. Res. 3, 1–20. doi: 10.6084/m9.figshare.1476821.v1
Tsakanikas, P., Fengou, L. C., Manthou, E., Lianou, A., Panagou, E. Z., and Nychas, G. -J. E. (2018). A unified spectra analysis workflow for the assessment of microbial contamination of ready-to-eat green salads: comparative study and application of non-invasive sensors. Comput. Electron. Agric. 155, 212–219. doi: 10.1016/j.compag.2018.10.025
Tsakanikas, P., Karnavas, A., Panagou, E. Z., and Nychas, G. -J. (2020). A machine learning workflow for raw food spectroscopic classification in a future industry. Sci. Rep. 10, 1–11. doi: 10.1038/s41598-020-68156-2
Tsakanikas, P., Pavlidis, D., and Nychas, G. -J. (2015). High throughput multispectral image processing with applications in food science. PLoS One 10:e0140122. doi: 10.1371/journal.pone.0140122
Tsakanikas, P., Pavlidis, D., Panagou, E., and Nychas, G. -J. (2016). Exploiting multispectral imaging for non-invasive contamination assessment and mapping of meat samples. Talanta 161, 606–614. doi: 10.1016/j.talanta.2016.09.019
Vapnik, V., Golowich, S. E., and Smola, A. (1997). “Support vector method for function approximation, regression estimation, and signal processing” in Advances in neural information processing systems. eds. M. C. Mozer, M. I. Jordan, and T. Petsche (Cambridge: MA, MIT Press), 281–287.
Vasconcelos, H., and Saraiva, C., and de Almeida, J. M. M. M. (2014). Evaluation of the spoilage of raw chicken breast fillets using Fourier transform infrared spectroscopy in tandem with chemometrics. Food Bioprocess Technol. 7, 2330–2341. doi: 10.1007/s11947-014-1277-y
Wood, J. D. (2017). “Meat composition and nutritional value” in Lawrie’s meat science. 8th Edn. ed. F. Toldra. (Duxford, UK: Woodhead Publishing), 635–659.
Woolthuis, C. H., Caspar, H. J., Mossel, D. A. A., Van Logtestijn, J. G., De Kruijf, J. M., and Smulders, F. J. M. (1984). Microbial decontamination of porcine liver with lactic acid and hot water. J. Food Prot. 47, 220–226. doi: 10.4315/0362-028X-47.3.220
Yu, X., Wang, J., Wen, S., Yang, J., and Zhang, F. (2019). A deep learning based feature extraction method on hyperspectral images for nondestructive prediction of TVB-N content in Pacific white shrimp (Litopenaeus vannamei). Biosyst. Eng. 178, 244–255. doi: 10.1016/j.biosystemseng.2018.11.018
Keywords: chicken liver, poultry, spoilage, Salmonella, Fourier-transform infrared spectroscopy, machine learning, support vector regression
Citation: Dourou D, Grounta A, Argyri AA, Froutis G, Tsakanikas P, Nychas G-JE, Doulgeraki AI, Chorianopoulos NG and Tassou CC (2021) Rapid Microbial Quality Assessment of Chicken Liver Inoculated or Not With Salmonella Using FTIR Spectroscopy and Machine Learning. Front. Microbiol. 11:623788. doi: 10.3389/fmicb.2020.623788
Edited by:
Javier Carballo, University of Vigo, SpainReviewed by:
Luca Roscini, University of Perugia, ItalyElisavet Stavropoulou, Centre Hospitalier Universitaire Vaudois (CHUV), Switzerland
Copyright © 2021 Dourou, Grounta, Argyri, Froutis, Tsakanikas, Nychas, Doulgeraki, Chorianopoulos and Tassou. This is an open-access article distributed under the terms of the Creative Commons Attribution License (CC BY). The use, distribution or reproduction in other forums is permitted, provided the original author(s) and the copyright owner(s) are credited and that the original publication in this journal is cited, in accordance with accepted academic practice. No use, distribution or reproduction is permitted which does not comply with these terms.
*Correspondence: Anthoula A. Argyri, YW50aGkuYXJneXJpQGdtYWlsLmNvbQ==; Chrysoula C. Tassou, Y3Rhc3NvdUBuYWdyZWYuZ3I=
†These authors have contributed equally to this work