- 1Zhengzhou Fruit Research Institute, Chinese Academy of Agricultural Sciences, Zhengzhou, China
- 2The Key Laboratory of Plant Immunity, College of Plant Protection, Nanjing Agricultural University, Nanjing, China
Grape white rot caused by Coniella diplodiella (Speg.) affects the production and quality of grapevine in China and other grapevine-growing countries. Despite the importance of C. diplodiella as a serious disease-causing agent in grape, the genome information and molecular mechanisms underlying its pathogenicity are poorly understood. To bridge this gap, 40.93 Mbp of C. diplodiella strain WR01 was de novo assembled. A total of 9,403 putative protein-coding genes were predicted. Among these, 608 and 248 genes are potentially secreted proteins and candidate effector proteins (CEPs), respectively. Additionally, the transcriptome of C. diplodiella was analyzed after feeding with crude grapevine leaf homogenates, which reveals the transcriptional expression of 9,115 genes. Gene ontology enrichment analysis indicated that the highly enriched genes are related with carbohydrate metabolism and secondary metabolite synthesis. Forty-three putative effectors were cloned from C. diplodiella, and applied for further functional analysis. Among them, one protein exhibited strong effect in the suppression of BCL2-associated X (BAX)-induced hypersensitive response after transiently expressed in Nicotiana benthamiana leaves. This work facilitates valuable genetic basis for understanding the molecular mechanism underlying C. diplodiella-grapevine interaction.
Introduction
Grapevine (Vitis vinifera L.) is an economically important fruit crop around the world. However, the widely planted Vitis vinifera cultivars are highly susceptible to various fungal pathogens (Armijo et al., 2016). Among them, the pathogenic fungus Coniella diplodiella (Speg.) Petr. and Syd, which causes white rot disease on the grapevine, can severely affect vine growth and grape production with an annual yield loss of 10–20% (Li et al., 2008). Coniella diplodiella is a necrotrophic fungal pathogen, which infects the injured grape berries, spikelets, young branches and leaves under high temperature and high humidity environments (Chethana et al., 2017; Zhang et al., 2017b). Several antifungal chemicals were used to control the white rot disease, however, the continuous application of chemical fungicides lead to the emergence of resistant pathogens and finally food safety and environmental problems (Han et al., 2015; Escribano-Viana et al., 2018).
Although the application of the fungicides is still one of the most effective ways to combat the C. diplodiella, efforts have been applied on the genetic basis of pathogen resistance and biological control reagents in recent years. Several candidate pathogenesis-related (PR) genes have been identified by comparative transcriptome analysis of susceptible and resistant grapevine species or cultivars challenged by C. diplodiella (Su et al., 2019; Zhang et al., 2019). Both salicylic acid (SA) and jasmonic acid (JA) synthesis signaling pathways may involve in host resistance against C. diplodiella. Association mapping in Chinese wild grapevines was performed to investigate potential quantitative trait loci (QTLs) involved in white rot disease resistance (Zhang et al., 2017c). However, molecular mechanism underlying pathogenicity of C. diplodiella on grapes has not been well understood so far, possibly due to the lack of genome information of this species.
The genus Coniella contains plenty of plant pathogens of economically important agricultural crops, including Vitis, Fragaria, and Punica (Alvarez et al., 2016). These Coniella species cause foliar, fruit, stem, and root diseases, bringing huge economic loss. However, the understanding of their pathogenicity mechanisms is very limited as there are no relevant genetic data available for these pathogens. Recently, the draft genome of Coniella lustricola, a new Coniella species isolated from submerged detritus, was reported (Raudabaugh et al., 2017). With the advantage of next generation sequencing (NGS) technologies, the number of sequenced fungal pathogen genomes are increasing rapidly. Genome annotation and comparison reveals the infection mechanism of plant pathogens of economically important crops, providing the genetic basis for understanding the plant-pathogen arm races (Moller and Stukenbrock, 2017; Raudabaugh et al., 2017).
Pathogens use secreted effectors to interfere with plant immunity, which are determinants of host-pathogen interaction (Dou and Zhou, 2012). Although some effectors have been characterized from the biotrophic and hemibiotrophic fungal pathogens, knowledge on the roles of effectors encoded by the necrotrophic fungi is still very limited (Lo Presti et al., 2015; Franceschetti et al., 2017). In addition to function as key virulence factors, effectors can be used for probing plant germplasm to seek resistance (R) genes in disease-resistant species or disease susceptibility (S) genes in disease-prone species (Vleeshouwers and Oliver, 2014; Xu et al., 2019). Wild Chinese Vitis species contain abundant and diverse gene resources for the genetic improvement of grapevine (Wan et al., 2007; Li et al., 2008). We have identified several grapevine accessions with conferred resistance to white rot disease (Zhang et al., 2017b). Furthermore, transcriptome analysis of the leaves of the resistant wild grapevine species Vitis davidii and the susceptible cultivar of V. vinifera “Manicure Finger” challenged by C. diplodiella identified more than 20 disease resistance-related genes (Zhang et al., 2019). However, the molecular mechanisms underlying the interaction of C. diplodiella with its host are still scarce.
In order to attain genetic information on the grape white rot fungus C. diplodiella, we sequenced the genome of C. diplodiella in this study. The 40.93 Mb genome contains 9,403 predicted genes coding for a large number of pathogenicity-related genes, including carbohydrate-active enzymes, secondary metabolite synthesis, effectors, and so on. These genes were differentially regulated by susceptible and resistant grapevine varieties. A preliminary screening of putative effector proteins revealed that one effector involved in the suppression of BCL2-associated X (BAX)-induced HR in tobacco, which may also important for the interaction between grapevine and C. diplodiella. Taken together, our results will improve the understanding of the pathogenicity of C. diplodiella on grapevine, and provide genome information for further comparison with other plant pathogens of the Coniella genus.
Materials and Methods
Fungal Strain and DNA Extraction
The Coniella diplodiella (Speg.) strain WR01 (from the Institute of Plant Protection, Chinese Academy of Agricultural Sciences) was cultured on PDA (Potato Dextrose Agar) medium at 28°C. Total DNA of C. diplodiella was isolated from the mycelia using QIAGEN® Genomic DNA kit following the standard procedures.
Genome Sequencing and Assembly
Qualified DNA was sheared using Covaris g-TUBE device. The fragmented DNA was repaired using PacBio Template Prep Kit (Pacific Biosciences, United States). The enrichment for long fragments was done by BluePippin size selection system (Sage Science) to construct a 20 kb library. After DNA purification using AMPure® PB beads (Pacific Biosciences, United States), the DNA fragments were ligated to the hairpins (SMRTbellTM templates). The library quality was checked by Agilent Bioanalyzer 2100 (Agilent Technologies, CA, United States) and Qubit 2.0 Fluorometer (Invitrogen, Life Technologies, CA, United States). The prepared SMRTbellTM templates was bound with magbead and loaded on a SMRT cell of PacBio Sequel platform. Single-molecule real-time (SMRT®) DNA sequencing (Berlin et al., 2015) was performed in Nextomics Biosciences Co., Ltd (Wuhan, China) according to the manufacturer’s protocol (Pacific Biosciences, CA, United States). Raw reads were processed by the SMRT Link v2.3.0 in the default mode to remove the adaptor sequences and low quality reads (below quality 0.8), and the filtered reads (6.3 G) were assembled to contigs with no gaps by CANU with default parameters (Koren et al., 2017). The sequencing data generated were deposited in NCBI Short Read Archive database (SRA accession: PRJNA649095).
Repetitive Elements and Non-coding RNA Analysis
Repetitive sequences were identified by aligning the assembly sequences with the known sequences in the Repbase database using RepeatProteinMasker and RepeatMasker (Bao et al., 2015) softwares, or by de novo prediction using three tools including RepeatModeler, LTRfinder (Xu and Wang, 2007), and PILER (Edgar and Myers, 2005) with default parameters. Tandem Repeats Finder (Benson, 1999) and MIcoSAtellite (Beier et al., 2017) were used to identify tandem repeat sequences and simple sequence repeats (SSR), respectively. tRNA sequences and its secondary structures were predicted using tRNAscan-SE (Schattner et al., 2005) software. rRNAs were predicted by RNAmmer (Lagesen et al., 2007) software.
Gene Prediction and Annotation
Protein coding gene models were predicted from the repeat-masked genome using three approaches: (1) ab initio gene prediction by SNAP (Korf, 2004) and Augustus (Stanke et al., 2004) using a transcriptome-based training set constructed by PASA (Haas et al., 2003); (2) protein homology-based prediction, where tBLASTn and GeneWise (Ter-Hovhannisyan et al., 2008) were used to align protein sequences from related species to the assembled genome; (3) transcriptome-based prediction, where transcripts were aligned to assembled genome using Exonerate software. Finally, EVidenceModeler (EVM) program was used to integrate all predicted gene models into a weighted consensus gene set (Haas et al., 2008).
All predicted gene models were annotated functionally by using several databases, including NCBI Non-Redundant (NR) and Swiss-Prot protein databases, Cluster of Orthologous Groups of proteins (COG), and Kyoto Encyclopedia of Genes and Genomes (KEGG) using BLASTp with E-value cut-off of 1e–05. Gene Ontology (GO) was performed using InterProScan.
Comparative Genomics
Orthologous groups of C. diplodiella and 12 other fungal genomes were determined by OrthoMCL (Li et al., 2003) software executed with the All-vs.-all BlASTp search with an E-value threshold of 1e–05. Amino acids of single-copy ortholog genes were aligned using MAFFT (Katoh and Standley, 2013). Gaps present in the alignments were removed by Gblocks (Castresana, 2000). The phylogenetic tree was constructed using RaxML (Stamatakis, 2014) with the GTRGAMMA model and 100 bootstrap replications, based on the concatenated alignments of single-copy ortholog families. Synteny analysis of C. diplodiella and its relative Coniella lustricola was performed by MUMmer (Kurtz et al., 2004). Mummerplot was used to produce the dot-blot of the MUMmer alignments.
Functional Annotation of Specific Gene Categories
Secretome was predicted using a combination of several tools. Signal peptides were predicted by both SignalP v4.1 (Petersen et al., 2011) and TargetP v1.1 (Emanuelsson et al., 2000). Transmembrane helices were analyzed using TMHMM v2.0 (Krogh et al., 2001). Glycosylphosphatidylinositol (GPI) modification sites were predicted by big-PI predictor (Eisenhaber et al., 1999). Extracellular secreted proteins were identified by a combination of characters including the presence of a functional signal peptide and the absence of a trans-membrane domain and GPI modifications (Sperschneider et al., 2015). Potentially secreted proteins with unknown functions (cannot be annotated by Swiss-Prot) were identified as putative effectors. Putative effectors which show no significant homology to known proteins from species outside the genus Coniella (BLASTp, E-value cut-off of 1e–05) were considered as Genus-specific candidate effectors.
Genes encoding carbohydrate-active enzymes were identified by the hmmscan program in the HMMER 3.0 package against the family-specific HMM profiles of CAZymes downloaded from the dbCAN database (Yin et al., 2012). The resulted file hmmscan was parsed by the hmmscan-parser scripts provided by dbCAN. Gene clusters related to secondary metabolite biosynthesis were identified using the antibiotics and secondary metabolite analysis shell database (antiSMASH v.4.0.2) (Weber et al., 2015).
Transcriptome Analysis and Quantitative RT-PCR
The agar plug (6 mm diameter) with C. diplodiella mycelium was inoculated onto PDA plates covered with or without the ground leaf homogenates from V. vinifera “Manicure Finger” or V. davidii accession 0940 under aseptic condition, and incubated at 28°C for 6 days (Figure 4). Then, fungal samples were harvested, immediately frozen in liquid nitrogen and stored at −80°C. Total RNA was extracted from six fungal samples with two biological replicates for each of the three different treatments using TRIzol reagent following manufacturer’s instructions (Invitrogen). The quality of RNA was evaluated using Agilent 2100 Bioanalyzer (Agilent, United States). The RNA-seq library construction and sequencing were performed in BGI company using Illumina HiSeq X Ten platform with 150 bp paired-end (PE) mode. Raw RNA-seq data was filtered to remove adaptor sequences and low-quality reads using a BGI internal software SOAPnuke (Chen et al., 2018). The clean reads were mapped to the C. diplodiella genome using Hisat2 software (Kim et al., 2015). Transcript assembly was performed using Bowtie 2 (Langmead and Salzberg, 2012) and gene expression levels were calculated based on fragments per kilobase of transcript per million fragments mapped (FPKM) using RSEM (Li and Dewey, 2011). Differential expression analysis was carried out using DEGseq2 (Wang et al., 2010).
For quantitative RT-PCR, the cDNAs were synthesized from 1 μg of total RNA using FastKing RT Kit with DNase (Tiangen Biotech, Beijing, PRC). The gene-specific primers were designed using Primer3Plus software and were listed in Supplementary Table S12. PCR reactions with the Roche FastStart DNA Master SYBR Green I reagent were performed on Roche LightCycler480 instruments with the following procedure: 95°C for 5 min, followed by 45 cycles of 95°C for 10 s, 60°C for 10 s, and 72°C for 20 s. The actin gene of C. diplodiella was used as the internal control. The relative gene expression level was analyzed by the 2–ΔΔCT method (Livak and Schmittgen, 2001). Primers used for qRT-PCR were listed in Supplementary Table S12. The data of RNA-seq has been deposited in NCBI Short Read Archive database (SRA accession: PRJNA657740).
Transient Expression Analysis of Candidate Effectors in Nicotiana benthamiana
Candidate effector genes (without putative signal peptide sequence, and an ATG start codon was added to initiate translation) were amplified from cDNA library using PrimeSTAR HS DNA Polymerase (Takara) and were ligated into the PVX vector pGR106. The primers used for vector construction were listed in Supplementary Table S13. Constructs verified by DNA sequencing were introduced into Agrobactrium tumefaciens (GV3101) cells containing the helper plasmid pJIC SA_Rep. Agroinfiltration assays were carried out according to the described method (Dou et al., 2008). Briefly, Agrobacterium cells carrying pGR106-effector or the respective control plasmids (empty pGR106 and pGR106-Bax) were cultured in LB (Luria-Bertani) medium containing kanamycin (50 μg/ml) overnight at 28°C with agitation. Bacterial cells were harvested by centrifugation (4,000 g, 2 min), washed with 10 mM MgCl2 twice and resuspended in MMA buffer (10 mM MgCl2, 10 mM MES, 100 μM acetosyringone, pH 5.6). The optical density of cell suspension was adjusted to an OD600 of 0.4 and incubated at room temperature for 2–3 h before infiltration. Fully expanded leaves from 5 to 6 weeks old Nicotiana benthamiana plants were used for agroinfiltration. Symptoms were observed in the following 3–7 days.
Results and Discussion
Genome Assembly and Annotation
The genome of C. diplodiella was sequenced by PacBio long-read single molecule real-time (SMRT) technologies to 145-fold coverage with an estimated genome size of 40.93 Mb and GC content of 49.79% (Table 1). The sequencing data (6,321,978,582 bp reads) were de novo assembled using CANU (Koren et al., 2017) into 13 contigs with an N50 length of 3.99 Mb, one of which corresponds to the mitochondrial genome with a length of 0.2 Mb. The other 12 contigs constitute the nuclear genome with a total size of 40.73 Mb. We identified 9,403 protein-coding gene models from genome assembly by combining ab initio gene prediction and homology-based methods. The average protein-coding gene length is 1,754 bp, with an average of 2.73 exons per gene and an average exon length of 571 bp. Furthermore, RNA-seq analysis of transcripts from in vitro C. diplodiella cultures supports the expression of 9,115 genes in the growth conditions tested (Figure 5). Both the estimated genome size and gene number of C. diplodiella are comparable to that of its relative Coniella lustricola. The completeness of the assembled gene space was evaluated by the benchmarking universal single-copy orthologs (BUSCO) method (Simão et al., 2015) using the Ascomycota dataset. This study identified 1,284 (97.6%) complete and single copy eukaryotic conserved protein-coding sequences (Supplementary Table S1), supporting a high completeness of the assembled C. diplodiella genome. Additionally, 311 tRNA and 57 rRNA genes were predicted in the genome (Table 1).
In total, 9,134 (97.14%) predicted genes could be annotated by homology search against multiple databases (Supplementary Tables S2, S3). Of them, 9,125 (97.04%) and 6,557 (69.73%) genes were annotated by NCBI non-redundant protein database (NR) and Swiss-Prot database, respectively. In addition, NR annotation showed 85.99% genes of C. diplodiella sharing homology with those of C. lustricola (Raudabaugh et al., 2017). Gene Ontology (GO) terms were assigned to 4,219 (44.87%) of the predicted protein-coding genes, including molecular function (3,386 genes), biological process (2,645 genes) and cellular component (1,293 genes) categories (Supplementary Table S3). The most enriched GO terms in the biological process were “single-organism process,” “metabolic process,” and “cellular process” terms (Supplementary Figure S1). KEGG analysis categorized 3,578 (38.05%) genes into 185 pathways (Supplementary Table S3). Among the 34 KEGG subclasses from 5 main classes, the “Global and overview maps,” “Carbohydrate Metabolism,” and “Translation” were the top three subclasses (Supplementary Figure S2). By the KOG (Eukaryotic Orthologous Groups) mapping, 2,870 (30.52%) genes were classified into 26 KOG categories (Supplementary Figure S3).
Repeat sequences were identified using several softwares. The length of total repetitive sequences was 5,182,685 bp, corresponding to 12.74% of the assembled C. diplodiella genome (Supplementary Table S4). The percentage of repetitive sequences in C. diplodiella is comparable to that of Valsa mali genome (14.05%) (Yin et al., 2015). The most abundant repetitive element is the long terminal repeat (LTR) element Gypsy (7.76%), followed by DNA transposons (1.75%), simple repeats (1.58%), non-LTR retrotransposon LINEs (0.49%) (Supplementary Table S5).
Orthologous Families and Phylogenetic Relationship Analysis
The predicted proteome of C. diplodiella was compared to 12 other filamentous fungi with different lifestyles. OrthoMCL analysis showed that C. diplodiella shared 8,823 orthologs with the other 12 fungi species, and 1,105 single-copy orthologous genes were conserved among all fungi analyzed (Figure 1A). The phylogenetic tree was constructed by RaxML using single-copy orthologous genes. Phylogenetic analysis revealed that C. diplodiella is evolutionally close to Coniella lustricola (Figure 1B; Raudabaugh et al., 2017), a non-pathogenic fungus that mainly feeds on plant detritus. Furthermore, we performed synteny comparison between C. diplodiella and C. lustricola. Genome colinearity comparison revealed a high sequence identity between them (Figure 2).
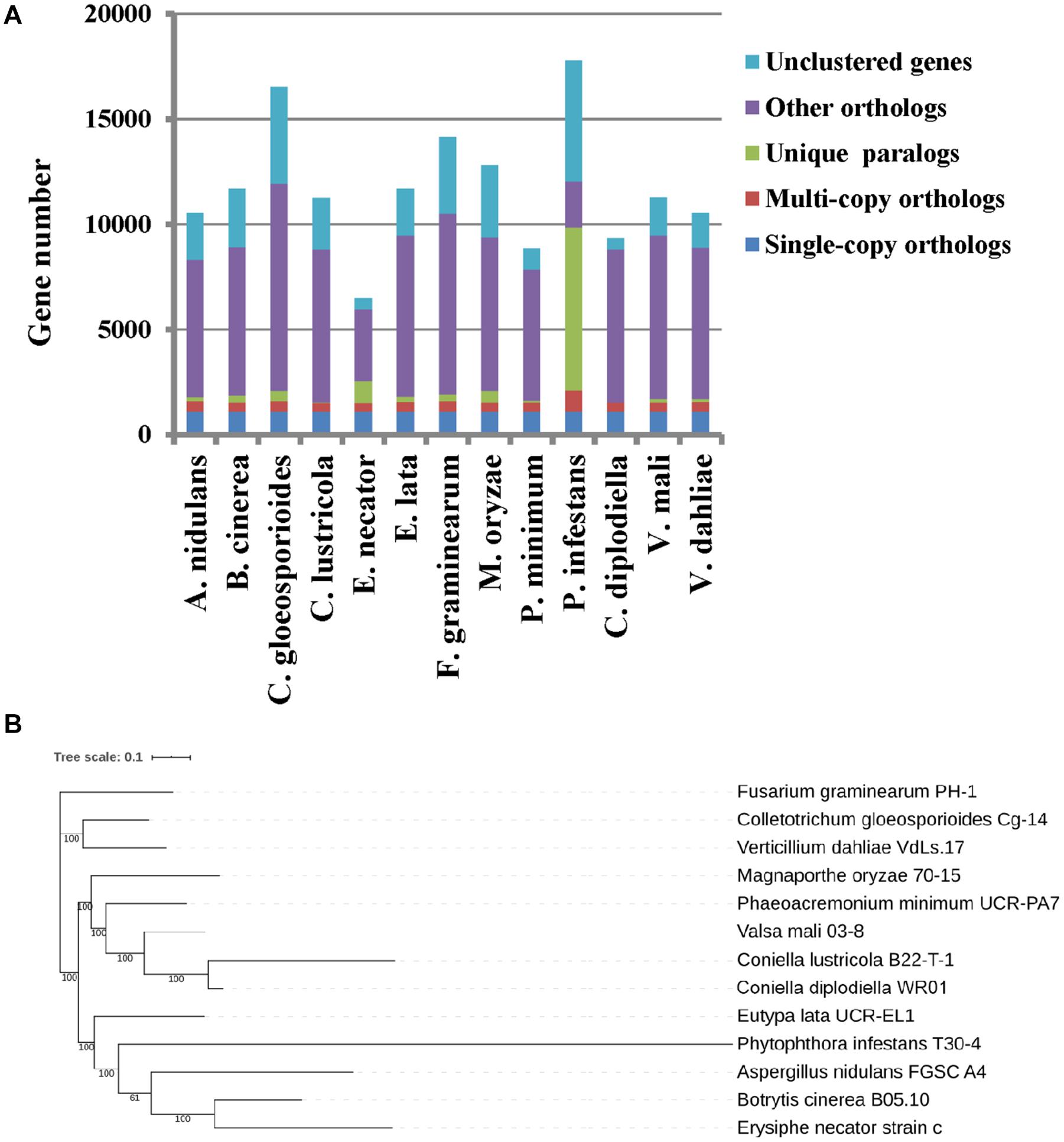
Figure 1. Orthologous gene family and phylogenetic relationship between C. diplodiella and 12 other fungi. (A) Statistic analysis of the shared and distinct orthologs. (B) A maximum likelihood phylogenetic tree was constructed by RaxML based on single-copy orthologous genes.
Secretome and Putative Effectors
The pathogenic fungi can deliver distinct sets of secreted proteins into the host-pathogen interface to facilitate its infection during plant-pathogen interactions. Using a combination of softwares including SignalP 4.1, TargetP 1.1, TMHMM 2.0, and big-PI Predictor (Sperschneider et al., 2015; Jones et al., 2018), we predicted 608 genes encoding putative secreted proteins, accounting for 6.47% of C. diplodiella proteome (Supplementary Table S6). The proportion of predicted secreted proteins relative to the total proteome is similar to those of other fungal pathogens (5–10%) (Lo Presti et al., 2015). Functional annotation showed that 88% of the secreted proteins have significant homologs outside the genus Coniella in GenBank NR database (E-value 1e–05) (Supplementary Table S3). Functional enrichment analysis showed that proteins involved in carbohydrate metabolism are significantly overrepresented in the C. diplodiella secretome (Supplementary Table S3). Some secreted proteins called effectors can function in the apoplast or translocate into plant cells to manipulate plant immunity (Dou and Zhou, 2012). Because most of the effectors identified so far have low sequence similarity to known proteins (Franceschetti et al., 2017), here candidate effectors proteins (CEPs) were defined as secreted proteins that have no homologs in Swiss-Prot database. Among the 608 secreted proteins of the C. diplodiella genome, we identified 246 CEPs, of which 72 proteins are Coniella-specific. Comparison analysis of amino acid sequences revealed that CEPs have shorter amino acid sequence length relative to other secreted proteins, with an average of 296 amino acids (Figure 3). In addition, they are cysteine-rich (2.18%) (Figure 3). These are the common properties for known fungal protein effectors (Jones et al., 2018). The identification of the effector repertoire of C. diplodiella will provide insights into its virulence function on host plants.
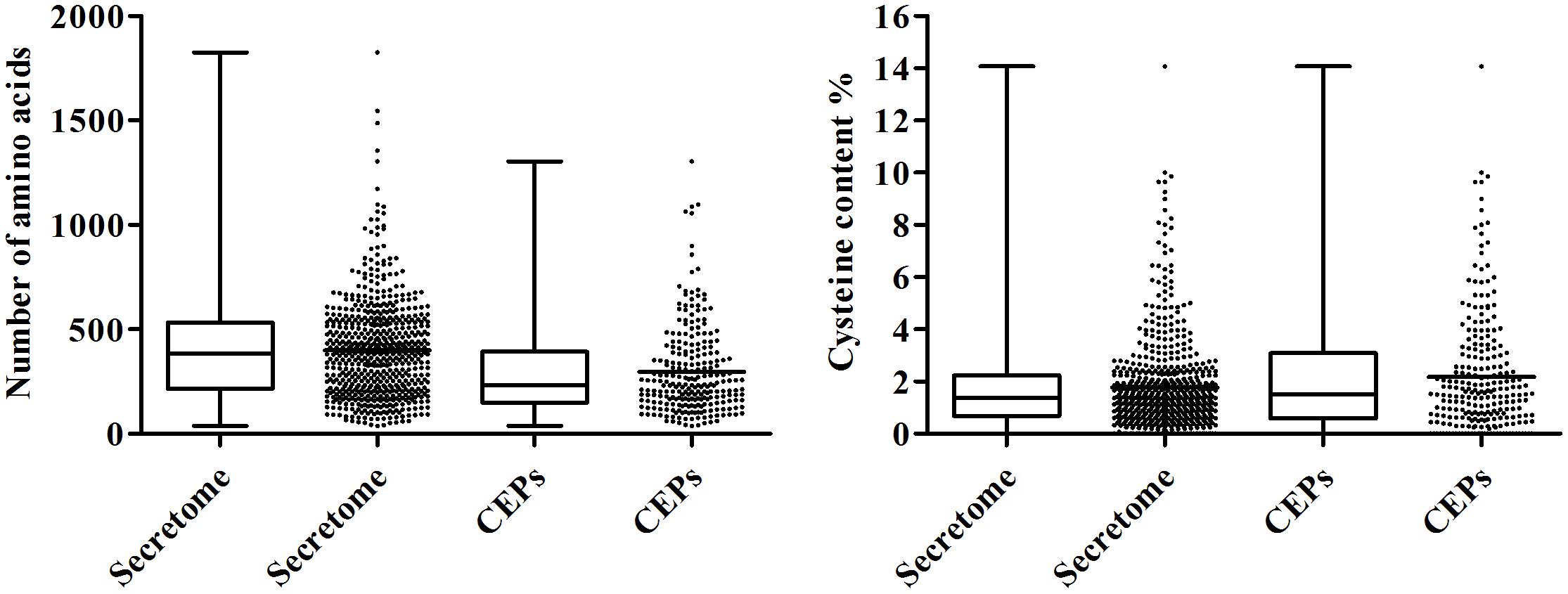
Figure 3. Box-plot shows sequence length and cysteine content of CEPs compared with those of the total secretome.
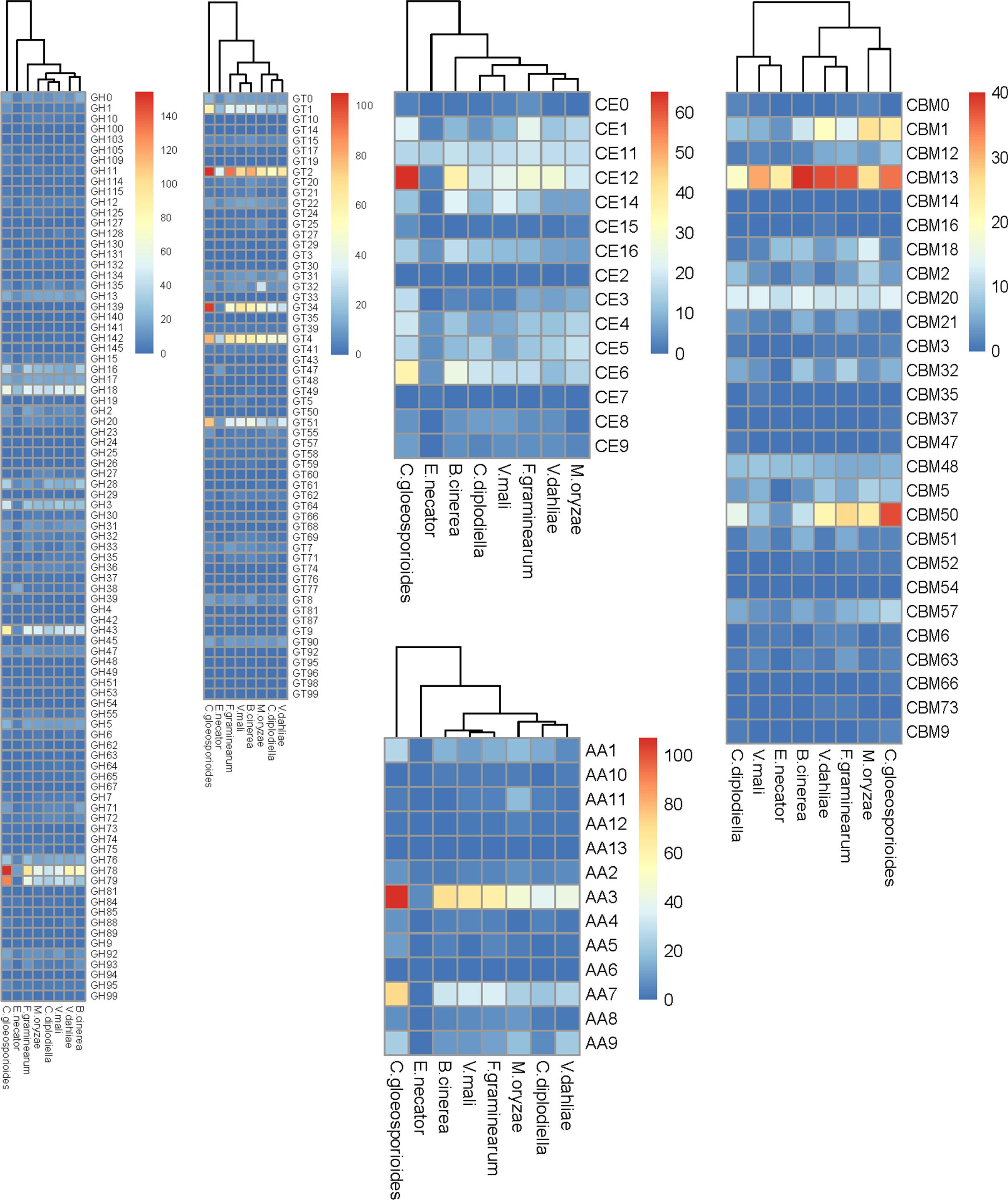
Figure 4. Comparison of carbohydrate-active enzymes between C. diplodiella and seven other fungal species. C. diplodiella, Coniella diplodiella; E. necator, Erysiphe necator; C. gloeosporioides, Colletotrichum gloeosporioides; B. cinerea, Botrytis cinerea; V. mali, Valsa mali; M. oryzae, Magnaporthe oryzae; F. graminearum, Fusarium graminearum; V. dahliae, Verticillium dahliae; GH, glycoside hydrolase; GT, glycosyltransferase; PL, polysaccharide lyase; CE, carbohydrate esterase; AA, auxiliary activity family; CBM, carbohydrate-binding module family.
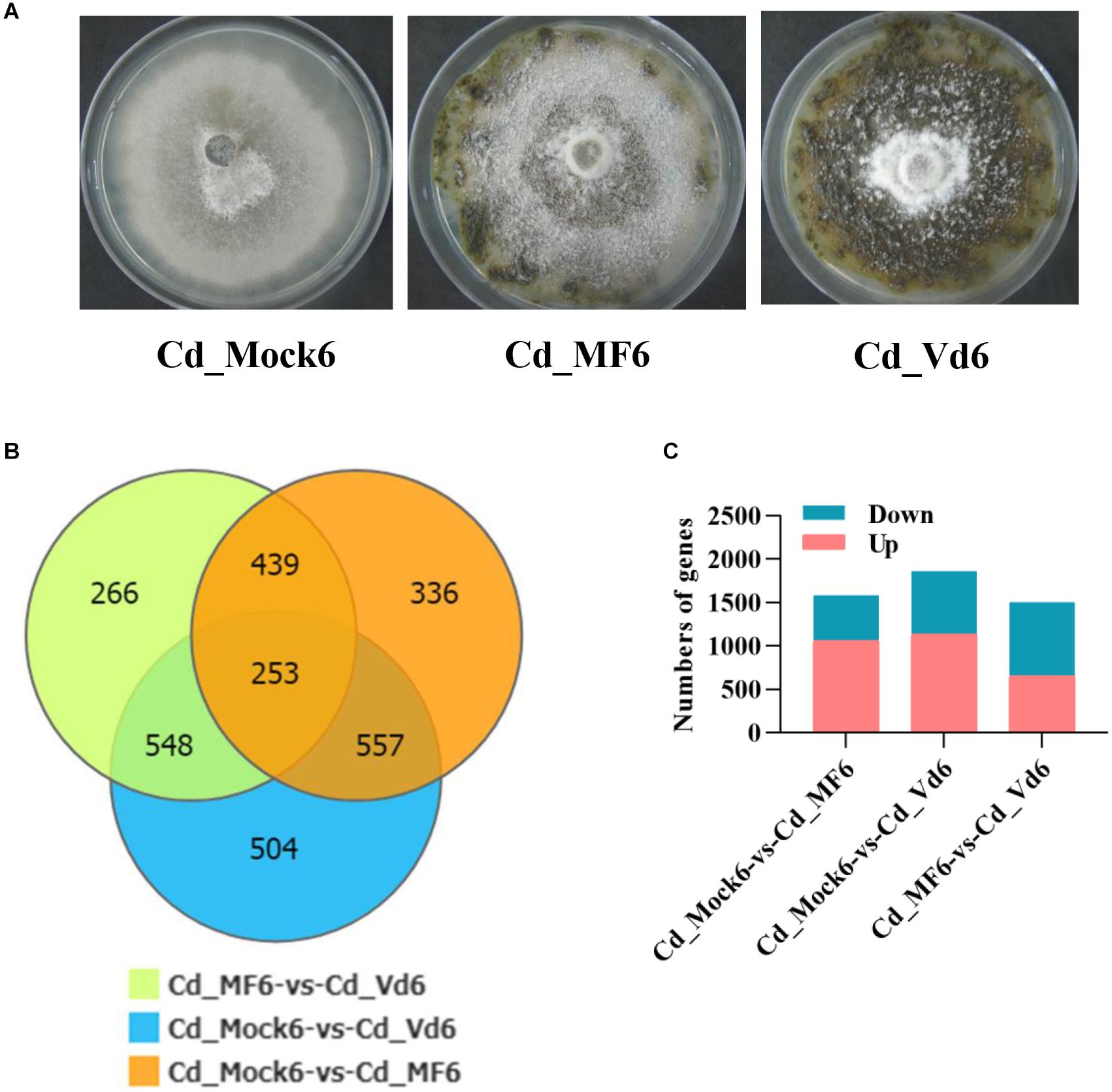
Figure 5. Statistics analysis of differentially expressed genes (DEGs) in C. diplodiella in response to susceptible and resistant grapevine host feeding. (A) Growth of C. diplodiella on potato dextrose agar (PDA) (Cd_Mock6), and PDA overlaid with leaf homogenates from susceptible V. vinifera “Manicure Finger” (Cd_MF6) or with that from resistant V. davidii accession 0940 (Cd_Vd6) for days at 28°C. (B) Venn diagram of DEGs of C. diplodiella with different treatments (Mock, MF, or Vd). (C) Number of up and down regulated genes of C. diplodiella with different treatments (Mock, MF, or Vd).
Secondary Metabolism Gene Clusters
Fungal secondary metabolites including non-ribosomal peptides, polyketides, NRPS-PKS hybrids, indole alkaloid, and terpenes are widely involved in the responses of fungus to environment stimuli and interaction with other organisms (Brakhage, 2013; Pusztahelyi et al., 2015). Biosynthetic pathways for secondary metabolites contain the backbone enzymes and several decorating enzymes and their corresponding genes tend to be co-regulated at the transcriptional level and clustered in the chromosome (Brakhage, 2013; Massonnet et al., 2018). The genome of C. diplodiella contains a total of 39 secondary metabolite gene clusters, including type 1 polyketide synthase (T1PKS, 15 clusters), non-ribosomal peptide synthetases (NRPS, three clusters), type 1 PKS-NRPS hybrids (four clusters), terpene synthases (three clusters), dimethylallyl tryptophan synthases (DMATS, one cluster), one T3 PKS and 13 other clusters (Supplementary Table S7). There are 21 and four genes predicted to encode polyketide synthase and non-ribosomal peptide synthetases, respectively. The numbers of PKS and NRPS genes in C. diplodiella are less than those in V. mali (Yin et al., 2015). Furthermore, many of these PKS and NRPS genes were surrounded by genes related to cytochrome P450 monooxygenase, dehydrogenase/reductase, alpha/beta-hydrolase and transporters (Supplementary Table S7). However, none of the predicted SM clusters showed high homology to the known clusters in other fungi. To our knowledge, there are no reports regarding bioactive secondary metabolite identified from Coniella-species, except for Coniella fragariae (Yu et al., 2018). Among the 423 putative secondary metabolism (SM) genes, the transcriptional expression of 414 SM genes was detected by RNA sequencing. Furthermore, comparing with that grown on PDA agar, 106 SM genes were significantly up-regulated in C. diplodiella mycelia by leaf tissue homogenates from the susceptible V. vinifera “Manicure Finger” grapevine (Supplementary Figure S4C), suggesting that these genes may participate in fungal infection. Of them, several up-regulated genes encode the polyketide synthases, including Cdip_03501, Cdip_04510, Cdip_04513, and Cdip_08045. They are considered as priority candidate genes for future study of secondary-metabolite biosynthesis. The PKS Cdip_08045 is orthologous to the conidial pigment polyketide synthase PfmaE in Pestalotiopsis fici, which is involved in melanin biosynthesis (Zhang et al., 2017a). Pigments produced by fungi can involve in fungal development, pathogenesis, and protect them from detrimental environments such as oxidative stress and UV irradiation. In Botrytis cinerea, deletions of PKS12 and PKS13 genes blocked spore pigmentation production (Schumacher, 2016). Similarly, deletion of PKSs in Alternaria alternata caused melanin deficiency and blocked spore formation (Wenderoth et al., 2017). The polyketide synthase gene cluster identification and gene expression profile analysis in this study support the possibility that melanin pigment may contribute to the C. diplodiella’s virulence. Moreover, many necrotrophic plant pathogens of the Dothideomycete class can produce phytotoxic metabolites that are required for pathogenicity (Stergiopoulos et al., 2013). The functional characterization of SM genes and the chemical entity of potential secondary metabolites from C. diplodiella await further investigation.
Carbohydrate-Active Enzymes
For successful colonization and infection, phytopathogenic fungi can produce an array of carbohydrate-active enzymes (CAZymes) to break down plant cell wall polysaccharides and derive nutrition from their hosts. The repertoire of CAZymes provides insights into its nutritional mode and infection mechanisms (Zhao et al., 2013). The C. diplodiella genome encoded 1,141 putative CAZymes. These CAZymes contain 507 glycoside hydrolases (GHs), 313 glycosyltransferases (GTs), 115 carbohydrate esterases (CEs), 98 auxiliary activities (AAs), 14 polysaccharide lyases (PLs) and 94 carbohydrate-binding modules (CBMs) and were categorized into 160 distinct families (Supplementary Table S8). Similarly, a total of 1,179 CAZymes including 531 GHs, 322 GTs, 125 CEs, 96 AAs, 13 PLs, and 92 CBMs were predicted in C. lustricola genome (Supplementary Table S8). Beside C. lustricola, the CAZyme profiles of C. diplodiella are close to that of other necrotrophic plant pathogens, such as Botrytis cinerea, Valsa mali, and Verticillium dahliae (Supplementary Table S8).
Plant cell walls are composed primarily of pectins, celluloses, hemicelluloses, lignins, and proteins. The GHs are the largest family involved in the carbohydrate degradation process. The GH class contributes the most catalytic enzymes to the degradation of lignocelluloses. The most genes encoding for the lignocellulose degrading enzymes belong to the GH3, GH16, GH18, GH28, GH43, GH78, GH79 family in C. diplodiella genome (Figure 4 and Supplementary Table S9). Fungal pathogens usually encode a large number of pectin-degrading enzymes including pectinlyase, pectatelyase, pectinesterase, and polygalacturonase to efficiently digest pectin. Polygalacturonases (family GH28) and pectinesterases (family CE8) catalyze the de-esterification of pectin to pectate and methanol. Most fungi contain only a small number (no more than 8) of pectinesterases, which may play a critical role in pectin degradation during pathogen infection. The genome of C. diplodiella contains 25 polygalacturonase genes and nine pectinesterases genes, suggesting a potential ability for pectin degradation (Figure 4 and Supplementary Table S9). In addition, there are abundant GT classes related with hemicellulose (GT34) and chitin (GT2) degradation and pectin-degrading enzymes (PL1 and PL3) in C. diplodiella genome (Figure 4 and Supplementary Table S9).
The C. diplodiella contains no radical-copper oxidases of the family AA5, an essential enzyme for lignin degradation, while several AA5 genes exist in other necrotrophic and hemibiotrophic pathogens examined, except for V. mali (Figure 4 and Supplementary Table S9). There are fewer lytic polysaccharide monooxygenases (LPMOs) of the family AA9, which cleave cellulose chains in synergic with classical cellulases, and also smaller number of family CBM1 members in C. diplodiella genome, compared with the cereal pathogens C. graminicola and M. oryzae (Figure 4 and Supplementary Table S9). Plant cuticle composed of a cutin polymer matrix is an effective physical barrier against the majority of pathogens. Therefore, plant fungal pathogen needs to produce cutinases in the early infection stages to launch infection (Dickman et al., 1989; Lu et al., 2018). Considering that the initial infection of C. diplodiella often requires a pre-existing wound site, it is surprising that the high number of cutinase genes of family CE5 exists in C. diplodiella genome (Figure 4 and Supplementary Table S9). RNA-seq analysis showed that most the cutinase-encoding genes are expressed in very low level or not detected (Supplementary Table S11), suggesting that they don’t involve in initial infection, and their expression may require a special stimulus or only in special infection stage.
RNA-Seq Analysis of Transcriptome Changes of C. diplodiella in Response to Host Grapevine Feed
Because the successful infection of C. diplodiella usually require wounds on the grapevine, and its destructive necrotrophic lifestyle (Chethana et al., 2017), this makes it difficult to recover enough fungus samples for examining gene expression in planta. Alternatively, we incubated the fungus on PDA supplemented with resistant or susceptible grapevine leaf homogenates to partially mimic the physiological response of C. diplodiella to its host plant. The growth of C. diplodiella was inhibited by resistant Vitis davidii, compared with that grown on PDA or susceptible V. vinifera (Figure 5A).
For RNA-sequencing, about 6.6 Gb cleaned data and 44 Mb clean reads were generated for each cDNA library (Cd_Mock6-1, Cd_Mock6-2, Cd_MF6-1, Cd_MF6-2, Cd_Vd6-1, Cd_Vd6-2) (Supplementary Table S9). The clean reads Q30 value was about 91% and approximately 85.53–88.9% of the clean reads were mapped to the C. diplodiella genome (Supplementary Table S9). The expression levels of all the transcripts were estimated by FPKM (fragments per kilo-base of exon per million fragments mapped) using RSEM (Li and Dewey, 2011). Overall, a total of 2,861 DEGs and 253 common DEGs were identified among three different treatments (Figure 5B). There were 1,585 DEGs (528 up-regulated and 1,057 down-regulated) in Cd_Mock6-vs-Cd_MF6, 1,861 DEGs (727 up-regulated and 1,135 down-regulated) in Cd_Mock6-vs-Cd_Vd6, and 1,506 DEGs (854 up-regulated and 652 down-regulated) in Cd_MF6-vs-Cd_Vd6 (Figure 5C). Quantitative RT-PCR was performed with nine selected genes to validate the accuracy of the gene expression profiles derived from RNA-seq data. The relative expression levels of all the nine genes were overall consistent with those obtained from RNA-seq data (Supplementary Figure S5). Gene Ontology enrichment analysis of the up-regulated genes in Cd_Mock6-vs-Cd_MF6 showed that predominant DEGs are enriched in catalytic activity, membrane-related process and carbohydrate metabolic process (Figure 6A), while the riched GO terms of the up-regulated genes in Cd_Mock6-vs-Cd_Vd6 belong to the ribosome, DNA replication and extracellular region (Figure 6B). These results suggest that resistant V. davidii may possess special genes/metabolites for resisting C. diplodiella infection.
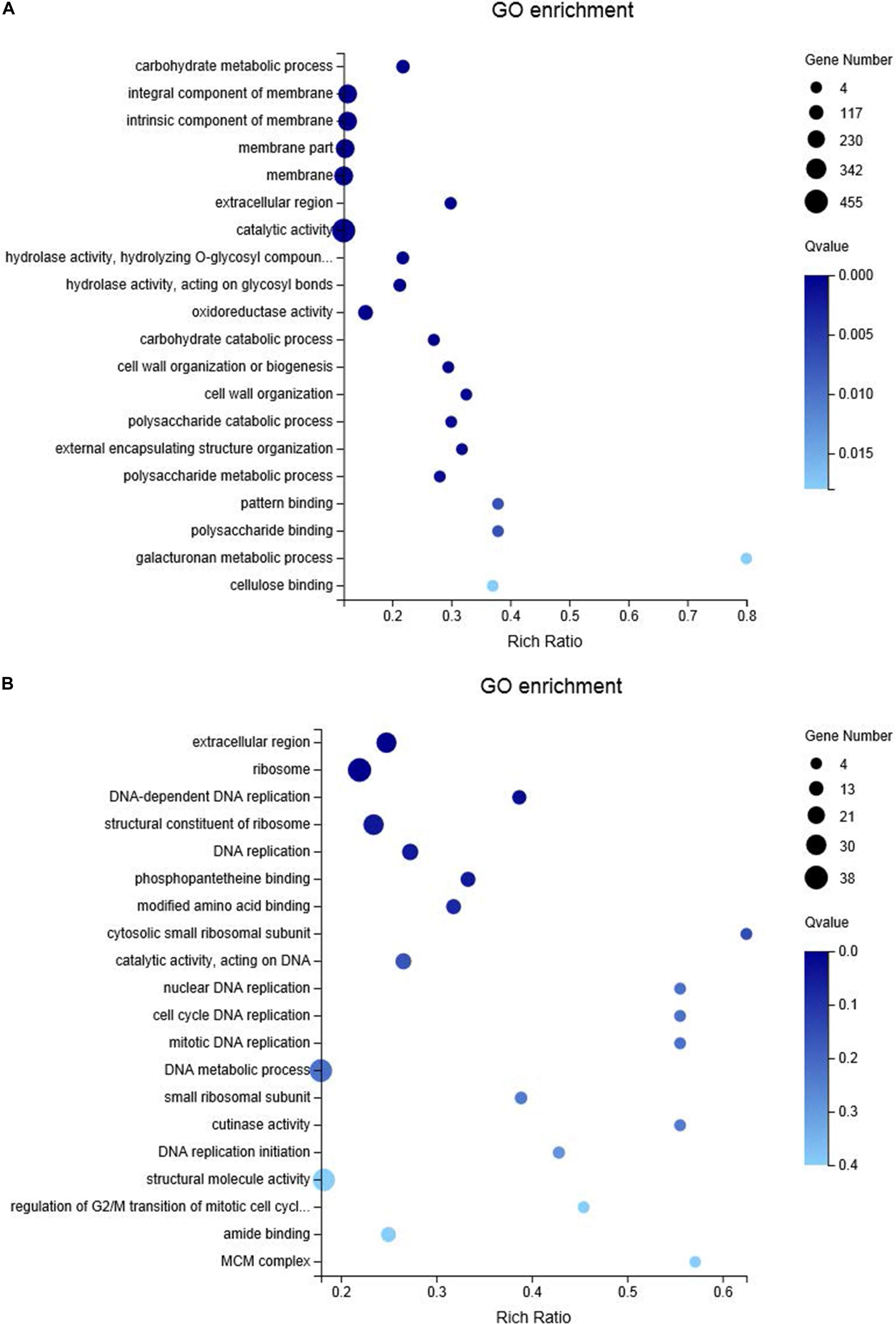
Figure 6. Bubble diagram of top 20 enriched GO terms of up-regulated genes in C. diplodiella in response to susceptible (A) and resistant (B) grapevine host feeding. X axis represents the Rich Ratio, which meaning the ratio of selected gene number annotated to a particular item to the total number of genes in this item in one species. The calculating formula is Rich Ratio = Term Candidate Gene Num/Term Gene Num. Y axis represents GO term. The size of the bubbles indicates the number of genes annotated to a GO term. And the color represents Q-value of enrichment. The deeper the color, the smaller the Q-value.
Functional Analysis of Putative Effectors
Effector proteins secreted by plant pathogens are key virulence factors that can suppress plant defense responses and modulate host cell physiology to promote infection (Lo Presti et al., 2015). When transiently expressed in plant cells, the pro-apoptotic mouse protein BAX (BCL2-associated X) can trigger programmed cell death (PCD) that closely resemble hypersensitive response in plants (Lacomme and Santa Cruz, 1999). Therefore, the ability to suppress BAX-triggered PCD has been used as a powerful tool for initial screening of candidate effectors (Wang et al., 2011; Li et al., 2015). To gain information on the function of putative effectors in plant-pathogen interaction, we randomly selected 43 candidate effector genes (the detailed information was listed in Supplementary Table S6) for transient expression in N. benthamiana. When co-infiltrated with BAX, Cdip00651 (here we named as Coniella diplodiella effector 1, CdE1) shows significant suppression of BAX-triggered PCD, but all other 42 candidate effectors do not (Figure 7), suggesting that CdE1 involves in plant immune suppression. CdE1 encodes a 91 amino acid protein with a predicted secretory signal peptide (amino acids 1–31) and contains no recognizable functional domain. The possible role of CdE1 in virulence and/or avirulence function needs to be explored by constructing CdE1 gene deletion and overexpression C. diplodiella mutants. In general, biotrophic and hemibiotrophic pathogens deliver effectors to interfere with PCD. In contrast, necrotrophic pathogens secrete effectors to promote plant cell death (Wang et al., 2014). For instance, the ToxA protein of P. tritici-repentis triggers cell death in wheat by targeting the host chloroplastic protein ToxABP1 (Manning et al., 2007). In this study, we identified one effector that suppresses BAX-triggered PCD among the 43 candidate effectors tested, but no one was found to induce cell death on non-host N. benthamiana. Given that necrotrophic effectors are usually host-specific, the necrosis-inducing activity of these CEPs needs to be further determined on hosts. As more effectors from necrotrophic pathogens were identified, the effectors with cell death-suppressing activity have been found. Several effectors of V. mali were found to suppresses Bax-induced PCD, in which both VmEP1 and VmPxE1 were demonstrated to contribute to the virulence of V. mali (Li et al., 2015; Zhang et al., 2018). Phytotoxin oxalic acid (OA) is a key virulence factor in the necrotrophic fungus Sclerotinia sclerotiorum and host interaction (Kabbage et al., 2013). OA-deficient mutants display non-pathogenic phenotype and trigger autophagic cell death, indicating that autophagy act in host defense against this pathogen and OA contributes to the suppression of autophagy (Kabbage et al., 2013). Therefore, we can assume that necrotrophic pathogens also employ effectors to suppress defense responses manifested as an PCD. Functional characterization of the host targets of CdE1 in grapevine will help to ascertain the roles of CdE1 in C. diplodiella-grapevine interaction.
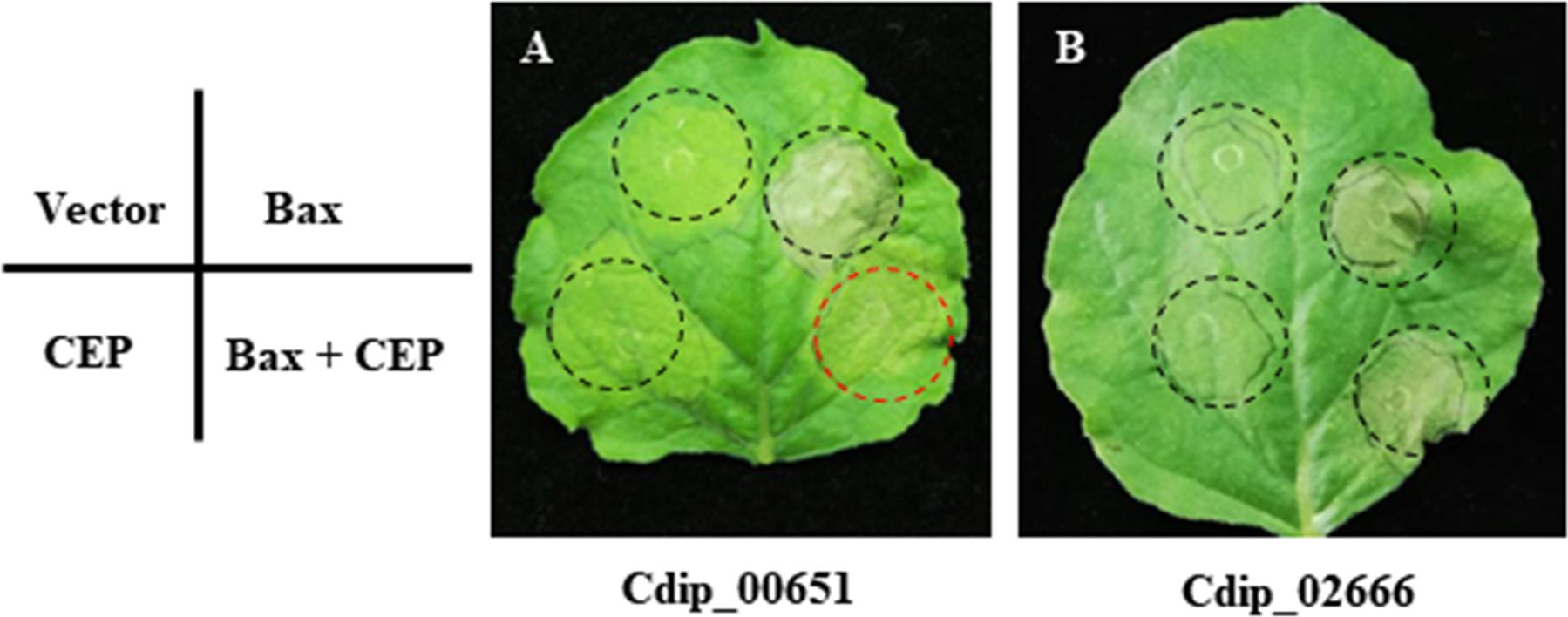
Figure 7. Effects of transient expression of candidate effector proteins on Bax-induced programmed cell death (PCD) in N. benthamiana using agroinfiltration. N. benthamiana leaves were infiltrated with Agrobacterium tumefaciens cells carrying pGR106 empty vector or the Bax gene, or candidate effector genes. For cell death suppression assays, co-expression of CEPs and Bax by mixing an equal volume of cells (OD600 = 0.8). Both Cdip_00651 (A) suppressed Bax-induced PCD, while other CEPs tested, such as Cdip_02666 (B), failed to block Bax-induced PCD. One representative result was showed in figure, and two independent experiments with six biological replicates for each experiment were performed with similar results.
Conclusion
Although grape white rot is one of the main fungal diseases in viticulture regions in China and widely distributed among most grape cultivation areas of the world, there is limited knowledge of its pathogenic mechanisms. The genome sequence and annotation of C. diplodiella revealed the genomic characteristics. Genome comparative analysis provided evidence for evolutionary relationships between C. diplodiella and C. lustricola. C. diplodiella contains a large number of genes encoding carbohydrate-active enzymes, consistent with other necrotrophic pathogens and its relative C. lustricola. As an essential feature for the necrotrophic pathogens is the use of special secondary metabolites as virulence factors, we identified numerous secondary biosynthetic gene clusters. None of them share similarity with known biosynthetic gene clusters, indicating that C. diplodiella may produce novel secondary metabolites. During plant-pathogen interaction, fungal effectors are crucial regulator of both pathogen virulence and plant immunity, therefore, identification of the target of effectors in plant host will provide insights into the way how pathogen interfere with host immunity for the success of infection. Further functional analysis of the pathogenesis-related candidate genes will improve our understanding of the interaction between C. diplodiella and grapevine.
Data Availability Statement
The data of RNA-seq has been deposited in NCBI Short Read Archive database (SRA accession: PRJNA657740).
Author Contributions
YZ, CL, and RL conceived and designed the experiments. RL, YZ, and LS analyzed the data. YW, JJ, and XF helped to prepare biological materials and data analysis. RL and PL did the RT-PCR, gene cloning, and gene transient expression. RL wrote the draft manuscript. YZ, YW, and CL revised the manuscript. All authors have read and approved the final manuscript for submission.
Funding
This work was supported financially by the National Natural Science Foundation of China (31872057), the China Agriculture Research System (CARS-29), Henan Key Laboratory of Fruit and Cucurbit Biology (HNS-201907-01), and the Agricultural Science Technology Innovation Program (CAAS-ASTIP-ZFRI).
Conflict of Interest
The authors declare that the research was conducted in the absence of any commercial or financial relationships that could be construed as a potential conflict of interest.
Acknowledgments
We thank Jian Guo for his patient help in the analysis of data.
Supplementary Material
The Supplementary Material for this article can be found online at: https://www.frontiersin.org/articles/10.3389/fmicb.2020.599150/full#supplementary-material
Supplementary Figure 1 | GO classification of total predicted genes in C. diplodiella.
Supplementary Figure 2 | KEGG pathway classification of total predicted genes in C. diplodiella.
Supplementary Figure 3 | KOG function classification of total predicted genes in C. diplodiella.
Supplementary Figure 4 | The expression pattern of total DEGs (A) and genes for carbohydrate enzymes (B), and secondary metabolite synthesis (C) in response to susceptible and resistant grapevine host feeding.
Supplementary Figure 5 | RT-PCR validation of expression levels of 9 DEGs identified by RNA-seq. Values in left vertical axis represents relative expression levels of selected DEGs quantified by qRT-PCR in three different treatments. The right vertical axis shows the fragments per kilo-base per million mapped reads (FPKM) values from RNA-seq. For qRT-PCR data, the means and SD of two biological replicates were shown.
Supplementary Table 1 | BUSCO analysis of C. diplodiella contigs.
Supplementary Table 2 | Statistics of C. diplodiella gene annotation.
Supplementary Table 3 | Summary of C. diplodiella gene annotation.
Supplementary Table 4 | Repeatitive sequence statistic of C. diplodiella.
Supplementary Table 5 | Summary of repeat element types in the C. diplodiella genome.
Supplementary Table 6 | Characteristics of secretome in C. diplodiella.
Supplementary Table 7 | Inventory of secondary metabolism gene clusters in C. diplodiella.
Supplementary Table 8 | Statistics of CAZymes family in different fungi.
Supplementary Table 9 | The gene numbers of CAZymes subfamily in different fungi.
Supplementary Table 10 | The overall quality and mapping of RNA-seq reads from C. diplodiella with three different treatments.
Supplementary Table 11 | Expression of putative cutinase-coding genes in C. diplodiella.
Supplementary Table 12 | Primers used for qRT-PCR.
Supplementary Table 13 | Primers used for gene cloning of selected candidate effector genes.
References
Alvarez, L. V., Groenewald, J. Z., and Crous, P. W. (2016). Revising the Schizoparmaceae: Coniella and its synonyms Pilidiella and Schizoparme. Stud. Mycol. 85, 1–34. doi: 10.1016/j.simyco.2016.09.001
Armijo, G., Schlechter, R., Agurto, M., Munoz, D., Nunez, C., and Arce-Johnson, P. (2016). Grapevine pathogenic microorganisms: understanding infection strategies and host response scenarios. Front. Plant Sci. 7:382. doi: 10.3389/fpls.2016.00382
Bao, W., Kojima, K. K., and Kohany, O. (2015). Repbase update, a database of repetitive elements in eukaryotic genomes. Mob. DNA 6:11. doi: 10.1186/s13100-015-0041-9
Beier, S., Thiel, T., Munch, T., Scholz, U., and Mascher, M. (2017). MISA-web: a web server for microsatellite prediction. Bioinformatics 33, 2583–2585.
Benson, G. (1999). Tandem repeats finder: a program to analyze DNA sequences. Nucleic Acids Res. 27, 573–580. doi: 10.1093/bioinformatics/btx198
Berlin, K., Koren, S., Chin, C. S., Drake, J. P., Landolin, J. M., and Phillippy, A. M. (2015). Assembling large genomes with single-molecule sequencing and locality-sensitive hashing. Nat. Biotechnol. 33, 623–630. doi: 10.1038/nbt.3238
Brakhage, A. A. (2013). Regulation of fungal secondary metabolism. Nat. Rev. Microbiol. 11, 21–32. doi: 10.1038/nrmicro2916
Castresana, J. (2000). Selection of conserved blocks from multiple alignments for their use in phylogenetic analysis. Mol. Biol. Evol. 17, 540–552. doi: 10.1093/oxfordjournals.molbev.a026334
Chen, Y., Chen, Y., Shi, C., Huang, Z., Zhang, Y., Li, S., et al. (2018). SOAPnuke: a MapReduce acceleration-supported software for integrated quality control and preprocessing of high-throughput sequencing data. Gigascience 7, 1–6. doi: 10.1093/gigascience/gix120
Chethana, K. W. T., Zhou, Y., Zhang, W., Liu, M., Xing, Q. K., Li, X. H., et al. (2017). Coniella vitis sp. nov. is the common pathogen of white rot in chinese vineyards. Plant Dis. 101, 2123–2136. doi: 10.1094/PDIS-12-16-1741-RE
Dickman, M. B., Podila, G. K., and Kolattukudy, P. E. (1989). Insertion of cutinase gene into a wound pathogen enables it to infect intact host. Nature 342, 446–448. doi: 10.1038/342446a0
Dou, D., Kale, S. D., Wang, X., Chen, Y., Wang, Q., Wang, X., et al. (2008). Conserved C-terminal motifs required for avirulence and suppression of cell death by Phytophthora sojae effector Avr1b. Plant Cell 20, 1118–1133. doi: 10.1105/tpc.107.057067
Dou, D., and Zhou, J. M. (2012). Phytopathogen effectors subverting host immunity: different foes, similar battleground. Cell Host Microbe 12, 484–495. doi: 10.1016/j.chom.2012.09.003
Edgar, R. C., and Myers, E. W. (2005). PILER: identification and classification of genomic repeats. Bioinformatics 21(Suppl. 1) i152–i158. doi: 10.1093/bioinformatics/bti1003
Eisenhaber, B., Bork, P., and Eisenhaber, F. (1999). Prediction of potential GPI-modification sites in proprotein sequences. J. Mol. Biol. 292, 741–758. doi: 10.1006/jmbi.1999.3069
Emanuelsson, O., Nielsen, H., BrBrunak, S., and von Heijne, G. (2000). Predicting subcellular localization of proteins based on their N-terminal amino acid sequence. J. Mol. Biol. 300, 1005–1016. doi: 10.1006/jmbi.2000.3903
Escribano-Viana, R., Lopez-Alfaro, I., Lopez, R., Santamaria, P., Gutierrez, A. R., and Gonzalez-Arenzana, L. (2018). Impact of chemical and biological fungicides applied to grapevine on grape biofilm, must, and wine microbial diversity. Front. Microbiol. 9:59. doi: 10.3389/fmicb.2018.00059
Franceschetti, M., Maqbool, A., Jimenez-Dalmaroni, M. J., Pennington, H. G., Kamoun, S., and Banfield, M. J. (2017). Effectors of filamentous plant pathogens: commonalities amid diversity. Microbiol. Mol. Biol. Rev. 81:e00066–16. doi: 10.1128/MMBR.00066-16
Haas, B. J., Delcher, A. L., Mount, S. M., Wortman, J. R., Smith, R. K. Jr., Hannick, L. I., et al. (2003). Improving the Arabidopsis genome annotation using maximal transcript alignment assemblies. Nucleic Acids Res. 31, 5654–5666. doi: 10.1093/nar/gkg770
Haas, B. J., Salzberg, S. L., Zhu, W., Pertea, M., Allen, J. E., Orvis, J., et al. (2008). Automated eukaryotic gene structure annotation using EVidenceModeler and the program to assemble spliced alignments. Genome Biol. 9:R7. doi: 10.1186/gb-2008-9-1-r7
Han, J., Chen, D., Huang, J., Li, X., Zhou, W. W., Gao, W., et al. (2015). Antifungal activity and biocontrol potential of Paenibacillus polymyxa HT16 against white rot pathogen (Coniella diplodiella Speq.) in table grapes. Biocontrol Sci. Technol. 25, 1120–1132. doi: 10.1080/09583157.2015.1036003
Jones, D. A., Bertazzoni, S., Turo, C. J., Syme, R. A., and Hane, J. K. (2018). Bioinformatic prediction of plant-pathogenicity effector proteins of fungi. Curr. Opin. Microbiol. 46, 43–49. doi: 10.1016/j.mib.2018.01.017
Kabbage, M., Williams, B., and Dickman, M. B. (2013). Cell death control: the interplay of apoptosis and autophagy in the pathogenicity of Sclerotinia sclerotiorum. PLoS Pathog. 9:e1003287. doi: 10.1371/journal.ppat.1003287
Katoh, K., and Standley, D. M. (2013). MAFFT multiple sequence alignment software version 7: improvements in performance and usability. Mol. Biol. Evol. 30, 772–780. doi: 10.1093/molbev/mst010
Kim, D., Langmead, B., and Salzberg, S. L. (2015). HISAT: a fast spliced aligner with low memory requirements. Nat. Methods 12, 357–360. doi: 10.1038/nmeth.3317
Koren, S., Walenz, B. P., Berlin, K., Miller, J. R., Bergman, N. H., and Phillippy, A. M. (2017). Canu: scalable and accurate long-read assembly via adaptive k-mer weighting and repeat separation. Genome Res. 27, 722–736. doi: 10.1101/gr.215087.116
Korf, I. (2004). Gene finding in novel genomes. BMC Bioinformatics 5:59. doi: 10.1186/1471-2105-5-59
Krogh, A., Larsson, B., von Heijne, G., and Sonnhammer, E. L. (2001). Predicting transmembrane protein topology with a hidden Markov model: application to complete genomes. J. Mol. Biol. 305, 567–580. doi: 10.1006/jmbi.2000.4315
Kurtz, S., Phillippy, A., Delcher, A. L., Smoot, M., Shumway, M., Antonescu, C., et al. (2004). Versatile and open software for comparing large genomes. Genome Biol. 5:R12. doi: 10.1186/gb-2004-5-2-r12
Lacomme, C., and Santa Cruz, S. (1999). Bax-induced cell death in tobacco is similar to the hypersensitive response. Proc. Natl. Acad. Sci. U.S.A. 96:79567961. doi: 10.1073/pnas.96.14.7956
Lagesen, K., Hallin, P., Rodland, E. A., Staerfeldt, H. H., Rognes, T., and Ussery, D. W. (2007). RNAmmer: consistent and rapid annotation of ribosomal RNA genes. Nucleic Acids Res. 35, 3100–3108. doi: 10.1093/nar/gkm160
Langmead, B., and Salzberg, S. L. (2012). Fast gapped-read alignment with Bowtie 2. Nat. Methods 9, 357–359. doi: 10.1038/nmeth.1923
Li, B., and Dewey, C. N. (2011). RSEM: accurate transcript quantification from RNA-Seq data with or without a reference genome. BMC Bioinformatics 12:323. doi: 10.1186/1471-2105-12-323
Li, D., Wan, Y. Z., Wang, Y. J., and He, P. C. (2008). Relatedness of resistance to anthracnose and to white rot in Chinese wild grapes. Vitis 47, 213–215.
Li, L., Stoeckert, C. J., and Roos, D. S. (2003). OrthoMCL: identification of ortholog groups for eukaryotic genomes. Genome Res. 13, 2178–2189. doi: 10.1101/gr.1224503
Li, Z., Yin, Z., Fan, Y., Xu, M., Kang, Z., and Huang, L. (2015). Candidate effector proteins of the necrotrophic apple canker pathogen Valsa mali can suppress BAX-induced PCD. Front. Plant Sci. 6:579. doi: 10.3389/fpls.2015.00579
Livak, K. J., and Schmittgen, T. D. (2001). Analysis of relative gene expression data using real-time quantitative PCR and the 2-ΔΔCT method. Methods 25, 402–408. doi: 10.1006/meth.2001.1262
Lo Presti, L., Lanver, D., Schweizer, G., Tanaka, S., Liang, L., Tollot, M., et al. (2015). Fungal effectors and plant susceptibility. Annu. Rev. Plant Biol. 66, 513–545. doi: 10.1146/annurev-arplant-043014-114623
Lu, L., Rong, W., Massart, S., and Zhang, Z. (2018). Genome-wide identification and expression analysis of cutinase gene family in Rhizoctonia cerealis and functional study of an active cutinase rccut1 in the fungal-wheat interaction. Front. Microbiol. 9:1813. doi: 10.3389/fmicb.2018.01813
Manning, V. A., Hardison, L. K., and Ciuffetti, L. M. (2007). Ptr ToxA interacts with a chloroplast-localized protein. Mol. Plant Microbe. Interact. 20, 168–177. doi: 10.1094/MPMI-20-2-0168
Massonnet, M., Morales-Cruz, A., Minio, A., Figueroa-Balderas, R., Lawrence, D. P., Travadon, R., et al. (2018). Whole-genome resequencing and pan-transcriptome reconstruction highlight the impact of genomic structural variation on secondary metabolite gene clusters in the grapevine esca pathogen Phaeoacremonium minimum. Front. Microbiol. 9:1784. doi: 10.3389/fmicb.2018.01784
Moller, M., and Stukenbrock, E. H. (2017). Evolution and genome architecture in fungal plant pathogens. Nat. Rev. Microbiol. 15, 756–771. doi: 10.1038/nrmicro.2017.76
Petersen, T. N., Brunak, S., von Heijne, G., and Nielsen, H. (2011). SignalP 4.0: discriminating signal peptides from transmembrane regions. Nat. Methods 8, 785–786. doi: 10.1038/nmeth.1701
Pusztahelyi, T., Holb, I. J., and Pocsi, I. (2015). Secondary metabolites in fungus-plant interactions. Front. Plant Sci. 6:573. doi: 10.3389/fpls.2015.00573
Raudabaugh, D. B., Iturriaga, T., Carver, A., Mondo, S., Pangilinan, J., Lipzen, A., et al. (2017). Coniella lustricola, a new species from submerged detritus. Mycol. Prog. 17, 191–203. doi: 10.1007/s11557-017-1337-6
Schattner, P., Brooks, A. N., and Lowe, T. M. (2005). The tRNAscan-SE, snoscan and snoGPS web servers for the detection of tRNAs and snoRNAs. Nucleic Acids Res. 33, W686–W689. doi: 10.1093/nar/gki366
Schumacher, J. (2016). DHN melanin biosynthesis in the plant pathogenic fungus Botrytis cinerea is based on two developmentally regulated key enzyme (PKS)-encoding genes. Mol. Microbiol. 99, 729–748. doi: 10.1111/mmi.13262
Simão, F. A., Waterhouse, R. M., Ioannidis, P., Kriventseva, E. V., and Zdobnov, E. M. (2015). BUSCO: assessing genome assembly and annotation completeness with single-copy orthologs. Bioinformatics 31, 3210–3212. doi: 10.1093/bioinformatics/btv351
Sperschneider, J., Williams, A. H., Hane, J. K., Singh, K. B., and Taylor, J. M. (2015). Evaluation of secretion prediction highlights differing approaches needed for oomycete and fungal effectors. Front. Plant Sci. 6:1168. doi: 10.3389/fpls.2015.01168
Stamatakis, A. (2014). RAxML version 8: a tool for phylogenetic analysis and post-analysis of large phylogenies. Bioinformatics 30, 1312–1313. doi: 10.1093/bioinformatics/btu033
Stanke, M., Steinkamp, R., Waack, S., and Morgenstern, B. (2004). AUGUSTUS: a web server for gene finding in eukaryotes. Nucleic Acids Res. 32, W309–W312. doi: 10.1093/nar/gkh379
Stergiopoulos, I., Collemare, J., Mehrabi, R., and De Wit, P. J. (2013). Phytotoxic secondary metabolites and peptides produced by plant pathogenic Dothideomycete fungi. FEMS Microbiol. Rev. 37, 67–93. doi: 10.1111/j.1574-6976.2012.00349.x
Su, K., Guo, Y., Zhao, Y., Gao, H., Liu, Z., Li, K., et al. (2019). Candidate genes for grape white rot resistance based on SMRT and Illumina sequencing. BMC Plant Biol. 19:501. doi: 10.1186/s12870-019-2119-x
Ter-Hovhannisyan, V., Lomsadze, A., Chernoff, Y. O., and Borodovsky, M. (2008). Gene prediction in novel fungal genomes using an ab initio algorithm with unsupervised training. Genome Res. 18, 1979–1990. doi: 10.1101/gr.081612.108
Vleeshouwers, V. G., and Oliver, R. P. (2014). Effectors as tools in disease resistance breeding against biotrophic, hemibiotrophic, and necrotrophic plant pathogens. Mol. Plant Microbe. Interact. 27, 196–206. doi: 10.1094/MPMI-10-13-0313-IA
Wan, Y., Schwaninger, H., He, P., and Wang, Y. (2007). Comparison of resistance to powdery mildew and downy mildew in Chinese wild grapes. Vitis 46, 132–136. doi: 10.1088/0253-6102/48/3/010
Wang, L., Feng, Z., Wang, X., Wang, X., and Zhang, X. (2010). DEGseq: an R package for identifying differentially expressed genes from RNA-seq data. Bioinformatics 26, 136–138. doi: 10.1093/bioinformatics/btp612
Wang, Q., Han, C., Ferreira, A. O., Yu, X., Ye, W., Tripathy, S., et al. (2011). Transcriptional programming and functional interactions within the Phytophthora sojae RXLR effector repertoire. Plant Cell 23, 2064–2086. doi: 10.1105/tpc.111.086082
Wang, X., Jiang, N., Liu, J., Liu, W., and Wang, G. L. (2014). The role of effectors and host immunity in plant-necrotrophic fungal interactions. Virulence 5, 722–732. doi: 10.4161/viru.29798
Weber, T., Blin, K., Duddela, S., Krug, D., Kim, H. U., Bruccoleri, R., et al. (2015). antiSMASH 3.0-a comprehensive resource for the genome mining of biosynthetic gene clusters. Nucleic Acids Res. 43, W237–W243. doi: 10.1093/nar/gkv437
Wenderoth, M., Pinecker, C., Voß, B., and Fischer, R. (2017). Establishment of CRISPR/Cas9 in Alternaria alternata. Fungal Genet. Biol. 101, 55–60. doi: 10.1016/j.fgb.2017.03.001
Xu, Z., and Wang, H. (2007). LTR_FINDER: an efficient tool for the prediction of full-length LTR retrotransposons. Nucleic Acids Res. 35, W265–W268. doi: 10.1093/nar/gkm286
Xu, Z., Xu, X., Gong, Q., Li, Z., Li, Y., Wang, S., et al. (2019). Engineering broad-spectrum bacterial blight resistance by simultaneously disrupting variable TALE-binding elements of multiple susceptibility genes in rice. Mol. Plant 12, 1434–1446. doi: 10.1016/j.molp.2019.08.006
Yin, Y., Mao, X., Yang, J., Chen, X., Mao, F., and Xu, Y. (2012). dbCAN: a web resource for automated carbohydrate-active enzyme annotation. Nucleic Acids Res. 40, W445–W451. doi: 10.1093/nar/gks479
Yin, Z., Liu, H., Li, Z., Ke, X., Dou, D., Gao, X., et al. (2015). Genome sequence of Valsa canker pathogens uncovers a potential adaptation of colonization of woody bark. New Phytol. 208, 1202–1216. doi: 10.1111/nph.13544
Yu, H., Sperlich, J., Mandi, A., Kurtan, T., Dai, H., Teusch, N., et al. (2018). Azaphilone derivatives from the fungus coniella fragariae inhibit NF-kappa B activation and reduce tumor cell migration. J. Nat. Prod. 81, 2493–2500. doi: 10.1021/acs.jnatprod.8b00540
Zhang, M., Feng, H., Zhao, Y., Song, L., Gao, C., Xu, X., et al. (2018). Valsa mali pathogenic effector VmPxE1 contributes to full virulence and interacts with the host peroxidase MdAPX1 as a potential target. Front. Microbiol. 9:821. doi: 10.3389/fmicb.2018.00821
Zhang, P., Wang, X., Fan, A., Zheng, Y., Liu, X., Wang, S., et al. (2017a). A cryptic pigment biosynthetic pathway uncovered by heterologous expression is essential for conidial development in Pestalotiopsis fici. Mol. Microbiol. 105, 469–483. doi: 10.1111/mmi.13711
Zhang, Y., Fan, X., Sun, H., Jiang, J., and Liu, C. (2017b). Identification and evaluation of resistance to white rot in grape resources. J. Fruit Sci. 34, 1095–1105. doi: 10.13925/j.cnki.gsxb.20160281
Zhang, Y., Feng, L., Fan, X., Jiang, J., Zheng, X., Sun, H., et al. (2017c). Genome-wide assessment of population structure, linkage disequilibrium and resistant QTLs in Chinese wild grapevine. Sci. Hortic. 215, 59–64. doi: 10.1016/j.scienta.2016.12.014
Zhang, Y., Yao, J. L., Feng, H., Jiang, J., Fan, X., Jia, Y. F., et al. (2019). Identification of the defense-related gene VdWRKY53 from the wild grapevine Vitis davidii using RNA sequencing and ectopic expression analysis in Arabidopsis. Hereditas 156:14. doi: 10.1186/s41065-019-0089-5
Keywords: Coniella diplodiella, grapevine, genome assembly, transcriptome, pathogenicity, effector
Citation: Liu R, Wang Y, Li P, Sun L, Jiang J, Fan X, Liu C and Zhang Y (2021) Genome Assembly and Transcriptome Analysis of the Fungus Coniella diplodiella During Infection on Grapevine (Vitis vinifera L.). Front. Microbiol. 11:599150. doi: 10.3389/fmicb.2020.599150
Received: 26 August 2020; Accepted: 14 December 2020;
Published: 11 January 2021.
Edited by:
Paloma Melgarejo, Ministerio de Agricultura, Alimentación y Medio Ambiente, SpainReviewed by:
K. W. Thilini Chethana, Mae Fah Luang University, ThailandNian Wang, Huazhong Agricultural University, China
Copyright © 2021 Liu, Wang, Li, Sun, Jiang, Fan, Liu and Zhang. This is an open-access article distributed under the terms of the Creative Commons Attribution License (CC BY). The use, distribution or reproduction in other forums is permitted, provided the original author(s) and the copyright owner(s) are credited and that the original publication in this journal is cited, in accordance with accepted academic practice. No use, distribution or reproduction is permitted which does not comply with these terms.
*Correspondence: Chonghuai Liu, liuchonghuai@caas.cn; Ying Zhang, zhangying05@caas.cn