- 1School of Life Sciences, Arizona State University, Tempe, AZ, United States
- 2Center for Fundamental and Applied Microbiomics, The Biodesign Institute, Arizona State University, Tempe, AZ, United States
- 3Swette Center for Environmental Biotechnology, The Biodesign Institute, Arizona State University, Tempe, AZ, United States
- 4Natural Resources and Environmental Management, University of Hawai‘i at Mânoa, Honolulu, HI, United States
- 5Tropical Plant and Soil Sciences, University of Hawai‘i at Mânoa, Honolulu, HI, United States
- 6College of Integrative Sciences and Arts, Arizona State University, Mesa, AZ, United States
The functions and interactions of individual microbial populations and their genes in agricultural soils amended with biochar remain elusive but are crucial for a deeper understanding of nutrient cycling and carbon (C) sequestration. In this study, we coupled DNA stable isotope probing (SIP) with shotgun metagenomics in order to target the active community in microcosms which contained soil collected from biochar-amended and control plots under napiergrass cultivation. Our analyses revealed that the active community was composed of high-abundant and low-abundant populations, including Actinobacteria, Proteobacteria, Gemmatimonadetes, and Acidobacteria. Although biochar did not significantly shift the active taxonomic and functional communities, we found that the narG (nitrate reductase) gene was significantly more abundant in the control metagenomes. Interestingly, putative denitrifier genomes generally encoded one gene or a partial denitrification pathway, suggesting denitrification is typically carried out by an assembly of different populations within this Oxisol soil. Altogether, these findings indicate that the impact of biochar on the active soil microbial community are transient in nature. As such, the addition of biochar to soils appears to be a promising strategy for the long-term C sequestration in agricultural soils, does not impart lasting effects on the microbial functional community, and thus mitigates un-intended microbial community shifts that may lead to fertilizer loss through increased N cycling.
Introduction
Modern agriculture faces multiple challenges: it must produce more food and fiber to feed a growing global population, adopt more efficient and sustainable management strategies for production, and adapt to climate change (FAO, 2017). These challenges require action by restructuring agroecosystems in order to increase food production from existing farm land while concomitantly achieving major reductions in environmental impacts (Garnett et al., 2013; Campbell et al., 2014). In this regard, incorporation of biochar into soils is a promising management strategy to address the reduction of greenhouse gas (GHG) emissions by enhancing carbon (C) sequestration in agricultural soils, while concurrently improving soil fertility (Jha et al., 2010; Lehmann et al., 2011; Paustian et al., 2016). Biochar is a carbonaceous product of biomass pyrolysis, which contains large portions of aromatic compounds that influence its stability and the spatial organization of C within soil particles (Wiedemeier et al., 2015; Hernandez-Soriano et al., 2016). In addition to carbon sequestration, biochar is intended for use as a soil conditioner to improve soil properties relevant to crop productivity (Jeffery et al., 2011). The hypothesized mechanisms for positive improvements to soil fertility are often explained by increased cation exchange capacity (CEC) (Liang et al., 2006), porosity, liming capacity, as well as enhanced water and nutrient retention (Lehmann et al., 2011; Laghari et al., 2016), and its influence on soil structure (Jien and Wang, 2013). However, the utility of biochar for any particular application is dependent on the soils it is added to and the biochars inherent properties, which are a function of feedstock and pyrolysis temperature. Previous studies have shown that feedstock type largely determines the CEC, mineral elements concentration and total organic carbon (OC) (Mukherjee et al., 2011; Barrow, 2012; Zhao et al., 2013). Enriched biochar can also be made by mixing biochars with minerals, clays and manure and heating the mixture at low temperatures to increase the concentration of exchangeable cations and available phosphorus (Chia et al., 2014). While parameters that most affect pH, surface chemistry, recalcitrance, and volatile matter content in biochar are mainly influenced by pyrolysis temperature, with recalcitrance increasing and volatile matter content decreasing with higher pyrolysis temperatures (Bruun et al., 2011; Zhao et al., 2013; Suliman et al., 2016). The ability to tailor biochar through feedstock and pyrolysis manipulations offers considerable opportunities for the use of biochar as a soil conditioner or carbon negative technology.
Despite the widespread interest in the application of biochar as a sustainable management practice, the effects of biochar on the soil microbiome still remain relatively underexplored due to the vast metabolic and phylogenetic diversity of the microorganisms present in soils. Soil microbial communities are complex and play key roles in sustaining soil function due to their significant role in regulating global nutrient and biogeochemical cycling via fundamental ecological processes such as mineralization and decomposition. The addition of biochar to soils can modify the soil environment which can influence soil microbial activity, abundance, and community composition. However, the microbial response to biochar is dependent on numerous factors such as soil type, properties of the biochar and cropping system (Anders et al., 2013; Docherty et al., 2015; Jenkins et al., 2017; Yu et al., 2018). In short-term experiments lasting less than 1 year, biochar produced at lower pyrolysis temperatures have been reported to increase soil respiration (Luo et al., 2011; Wang et al., 2012), while longer-term experiments (up to 4 years) have reported no significant difference in soil respiration but significantly increased soil microbial biomass (Zhang et al., 2014; Zheng et al., 2016). Other short-term studies have reported changes in the soil microbial community after biochar amendment, with increased microbial biomass after the application of biochars produced at lower pyrolysis temperatures (Anders et al., 2013; Chen et al., 2016), while others have found no significant change or decreased microbial biomass with the addition of biochar produced at higher temperatures (Elzobair et al., 2016; Li et al., 2018). Previous studies have indicated that biochar significantly altered microbial community composition, however, biochar effects have been reported with some contradictory findings on the significant changes in the relative abundance of bacterial groups. Several studies focused on shifts in the bacterial community have observed increased soil pH, water holding capacity, and nitrogen (N) mineralization and increases in the relative abundance of Actinobacteria, Bacteroidetes, and Proteobacteria (Anderson et al., 2011; Xu et al., 2016; Zheng et al., 2016). In pot-experiments examining root-associated microbial communities, biochar addition increased the relative abundance of Bacteroidetes and decreased Proteobacteria (Kolton et al., 2011). In soils that contained natural or added biochar increase soil respiration and increased relative abundance of Gemmatimonadetes and Actinobacteria have also been reported (Khodadad et al., 2011). In part, the difference observed between biochar studies may reflect the differences in soils, plant cover and the land management. Jenkins et al. (2017) examined the effects of biochar application across three site in Europe under different land management and observed that biochar increased relative abundance of Gemmatimonadetes and Proteobacteria and decreased Acidobacteria under an Italian grassland, while Acidobacteria and Gemmatimonadetes relative abundance increased under short rotation coppice in the United Kingdom. Other studies have found distinct bacterial communities on the surfaces of biochar or mineral enriched biochar which can support chemolithotrophic processes (Ye et al., 2017). Overall, various changes in the microbial community have been reported after biochar application though these effects are not uniform and depend strongly on soil type, biochar feedstock, application rate and cropping system (Steinbeiss et al., 2009; Khodadad et al., 2011; Lehmann et al., 2011; Gomez et al., 2014; Thies et al., 2015; Yu et al., 2018; Zhang et al., 2019). It is crucially important to understand the effects of biochar on soil microbial communities in order to predict the potential for C sequestration and nutrient cycling under large-scale agricultural production.
The ecology of soil microbial communities and changes in these communities due to biochar addition has principally been investigated using molecular techniques. These studies have primarily focused on the composition and diversity of the total community derived from soil genomic DNA, which may or may not be active. Examination of active microbial populations can provide insights into how communities respond to changing environmental conditions and contribute to nutrient cycling, C stabilization and storage. In order to predict the impact of the microbiome on soil ecosystem function, it is critical to specifically target members of the active soil microbial community. To this end, DNA stable isotope probing (SIP) is a cultivation-independent technique that can be used to link microbial activity to the identity within environmental samples (Chen and Murrell, 2010; Lee et al., 2011; Verastegui et al., 2014; Coyotzi et al., 2016). It relies on the incorporation of stable isotope labels into microbial DNA during growth on the labeled substrate, thus acting as a filter to enrich the DNA of active populations. DNA-SIP has been coupled with shotgun metagenomic sequencing to identify new functional and adaptive traits of microbial taxa and to directly link microbial populations with ecological processes (Eyice et al., 2015; Ziels et al., 2018). Metagenomic techniques have been used to study these highly complex and diverse ecosystems, providing descriptions of the taxonomic and functional potential of natural microbial communities. Additionally, assembling and binning of sequences from metagenomes has allowed for the recovery of genomes of abundant and rare microbial populations (i.e., metagenomic assembled genomes or MAGs) from various environments. However, there have been no previously reported studies coupling DNA-SIP with shotgun metagenomics to recover population MAGs from biochar-amended soils.
In the present study, we performed DNA-SIP coupled with shotgun metagenomics to investigate the active populations of the soil microbiome of a tropical Oxisol that experienced 2 years of a low-volatile matter biochar amendment under napiergrass cultivation. The objectives of this study were: (1) to identify and explore the effects of biochar amendment on active soil microbial populations, and (2) to gain insight into the functional aspects of the active community. Targeting the active community allowed for the recovery of higher quality MAGs, which enabled the characterization of gene content to test the conclusions concerning individual capabilities and metabolisms. We hypothesized that the impact of biochar on the soil microbial communities would still be apparent after 2 years following a single application of biochar. Furthermore, we hypothesized that soil microbial communities would exhibit a significant change, due to biochar addition, in the composition and abundance of the metabolically active populations and their functional responses in terms of both their nutrient cycling potential and their use of biochar-derived recalcitrant (e.g., aromatic) carbon substrates in these Oxisol soils under Napiergrass cultivation. We expected that, based on the previous metagenomic analyses (Yu et al., 2019), that biochar amendment would increase the relative abundances of two bacterial phyla in the active population, Bacteroidetes and Proteobacteria. We also expected that the active population of biochar-amended soils would exhibit an increased genetic potential for denitrification.
Materials and Methods
Overview of Sites, Biochar and Sample Collection
The Oxisol soils used in this study were collected from a field experiment on the island of Oahu, HI, United States at the Poamoho agricultural research station, which was managed by the College of Tropical Agriculture and Human Resources, University of Hawaii Manoa (21°32′30″N, 158°01′15″W). The soil is highly weathered with low CEC, slightly acidic, and contains about 44% clay rich in kaolinite and iron oxides (NRCS Web Soil Survey). Detailed descriptions of the field experiment, biochar type and biochar application rate were described in a previous study (Yu et al., 2018). Briefly, the biochar used in this experiment was produced by Diacarbon Inc1. The feedstock was 20% anaerobic digester sewage sludge and 80% spruce, pine and fir wood chips, which underwent pyrolysis in a continuous flow reactor at about 600°C. The biochar is 74% carbon, 1.03% nitrogen, and 1.93% hydrogen and has a pH of 10.53 and an electrical conductivity of 444.5 μS/cm. For proximate analysis, the biochar is 29.73% volatile matter, 56.72% fixed C and 15.31% ash. Samples were collected from plots under napiergrass (Pennisetum purpureum var. green bana) cultivation approximately 2 years after a single addition of biochar. Ratoon harvest was the harvest technique used for the crop, which is a zero-tillage system that retains the below-ground environment. Soil samples were collected on November 2015 from four replicate plots from biochar-amended and control soils prior to harvest. Each plot was split in half and from each half-plot three 0 – 10 cm depth cores were taken randomly and then mixed to create a composite. Four composite samples were taken per half plot for a total of 8 replicates per plot. Samples transported on dry ice to the laboratory and were frozen at −80°C without addition of any protective agent until ready for further processing. Soil chemical properties were determined as previously described (Yu et al., 2018, 2019), and are summarized in Supplementary Table S1.
Preparation of Stable Isotope Probing Soil Microcosms
The soils, previously frozen field-moist, were thawed and soil from each plot were sieved together through a 2 mm sieve, sample replicates were composited based on the respective plot from which the soils were collected. Microcosms were prepared by adding 10 g of soil in 150 ml serum bottles and pre-incubated at 4°C open to the ambient atmosphere in the dark for 7 days to allow the soils to equilibrate. Uniformly labeled (>97 atom % 13C) 13C-perennial ryegrass (Lolium perenne – aboveground biomass) (IsoLife, Wageningen, Netherlands) was powdered using a mortar and pestle, 0.5% (w/w) was added to soils before bottles were sealed and capped with butyl rubber septa. Each microcosm with 13C-labeled perennial ryegrass was paired with an identical ‘12C-control’ microcosm amended with the corresponding unlabeled 12C-perennial ryegrass (∼1.1% atom 13C). 12C-control microcosms were used to control for background presence of GC-rich DNA in higher density CsCl gradient fractions (Youngblut and Buckley, 2014). All microcosms were maintained at 23°C for 14 days in the dark. Soil respiration as a proxy for activity was measured in parallel microcosms prepared using 5 g of soil and 0.05% (w/w) 13C-perennial ryegrass, soil microcosms were not continuously aerated. For determination of cumulative CO2 and N2O, 200 μl of headspace from each microcosm was sampled in triplicate using a gas-tight syringe (VICI Precision Sampling, Baton Rouge, LA, United States). Headspace CO2 and N2O content was measured on a GC-ECD-FID (SRI 8610C) after microcosms were set up (day 0) and after 1, 3, 5, 7, 10, and 14 days of incubation. A standard curve was generated prior to measurement for each time point, each standard curve contained four points ranging from 250 to 5000 ppm CO2 for day 0 and day 1 measurements and later from 2500 to 25,000 ppm CO2 for remaining gas measurements. Similarly, a four-point standard curve for was generated to determine N2O concentration ranging from 0.5 to 25 ppm for all time points. Cumulative gas concentrations were calculated for each microcosm by summing the aggregate gas production over the 14-day incubation.
DNA Extraction and Density-Gradient Centrifugation
Soil samples were collected for DNA extraction from 13C-ryegrass fed microcosms after 14 days of incubation. Soil DNA was extracted from 5 g of soil using the DNeasy PowerMax Soil kit (Qiagen Company, Hilden, Germany) as described previously (Yu et al., 2018). An initial extraction, followed by a second successive extraction, was conducted on each sample to improve DNA extract yield. A successive extraction involved adding new aliquots of bead solution, 0.5M Tris buffer (pH 9), 0.2M phosphate buffer (pH 8) were and solution C1 to the soil pellet after initial lysis, centrifugation, and removal of supernatant containing crude DNA extract. Lysis and centrifugation steps were then repeated. The DNA extracts from the initial and successive extraction was pooled and concentrated using a DNA120 SpeedVac (Thermo Savant) and was quantified using the Qubit dsDNA high-sensitivity kit (ThermoFisher Scientific, Waltham, MA, United States) using the Qubit 3.0 (ThermoFisher Scientific, Waltham, MA, United States).
DNA extracts (3 μg DNA) were subjected to density-gradient centrifugation and fractionation (Dunford and Neufeld, 2010). Briefly, DNA extracts were mixed with gradient buffer (0.1M Tris-HCl, 0.1M KCl, and 1 mM EDTA) and 7.163M CsCl solution and loaded into 4.8ml polypropylene Quick-Seal tubes (Beckman Coulter, Brea, CA, United States). Density gradient centrifugation was performed with a VTi 65.2 rotor at 55,000 rpm at 20°C for 60 h in an OptimaMax ultracentrifuge (Beckman Coulter, Brea, CA, United States) with the vacuum on, maximum acceleration, and no brake on deceleration. Gradients were displaced with mineral oil (Johnson Johnson) pumped into the top of the Optiseal tube using a syringe pump (KD Scientific), and approximately 250 μl fractions were collected dropwise from a needle in the bottom of the tube. The temperature corrected refractive index (nD-TC 20°C) of each gradient fraction was immediately measured using an AR200 digital refractormeter (Reichart, Ithaca, NY, United States), and buoyant density was calculated from the refractive index using the equation
where ρ is the density of the CsCl (g ml–1), η is the measured refractive index, and a and b are coefficient values of 10.9276 and 13.593, respectively, for CsCl at 20°C (Birnie, 1978). DNA was precipitated from each fraction as described by Dunford and Neufeld (2010). The pellet was suspended in sterile TE buffer and the final concentration of DNA in each fraction was measured using the Qubit dsDNA high-sensitivity kit (ThermoFisher Scientific, Waltham, MA, United States) using the Qubit 3.0 (ThermoFisher Scientific, Waltham, MA, United States).
Quantitative PCR
To further detect differences in buoyant density values between the 12C- and 13C-incubated microcosms, quantitative PCR was conducted on DNA from gradient fractions with buoyant densities ranging from 1.682 to 1.719 g ml–1. The qPCR targeted the 16S rRNA gene fragment using the 341F/797R primer pair (Nadkarni et al., 2002). qPCR was performed using the QuantStudio3 (Applied Biosystems). Each 20 μl reaction mix contained 1 μl DNA template, 500 nM of each forward and reverse primers, 7 μl PCR-grade water, 10 μl PowerUp SYBR green Master Mix (2X, Applied Biosystems). The amplification procedure for all qPCR assays consisted of an initial denaturation at 95°C for 3 min, followed by 40 cycles of denaturation at 95°C for 45 s, annealing at 60°C for 45 s, and extension at 72°C for 1min, and a final extension at 72°C for 7 min. All samples were analyzed in duplicate, no-template controls were included on each qPCR run. Plasmid standards for qPCR were prepared by cloning the 16S rRNA PCR amplicon fragment from E. coli K-12 into a pCR4-TOPO plasmid using the TA TOPO cloning kit (Invitrogen, Carlsbad, CA, United States). Plasmids containing the target PCR amplicon sequence were quantified by Qubit. Gene copy numbers were calculated from the measured DNA concentration and the molecular weight of the ligated plasmid containing the PCR amplicon insert. Calibration standards included 108, 107, 106, 105, 104, 103, 102 gene copies per reaction and included in triplicate in each qPCR run. The average slope of the calibration curve was −3.4051 (97.45% PCR efficiency) and the R2 value was 0.988.
Metagenomic Sequencing, Assembly and Binning
Pooled volumetric fractions from heavy DNA (Figure 1) from density-gradient centrifugation were used to generate an Illumina sequence library with an average insert size of 400 bp that was sequenced on an Illumina NextSeq 500 with paired-end 150 bp reads at the DNASU Core Facility at Arizona State University. The metagenomic sequencing produced an average of 49.4M reads for biochar-amended samples and 40.9M reads for control samples. Estimates of average coverage and sequence diversity for each metagenomic data set were carried out with Nonpareil 3 using default settings (Rodriguez-R and Konstantinidis, 2014; Rodriguez-R et al., 2018). The raw sequencing reads were quality filtered and trimmed to remove Illumina adaptors using Trimmomatic version 0.36 (Bolger et al., 2014), paired-end reads were interleaved using the interleave-reads.py script from khmer version 2.1.1 (Crusoe et al., 2015) before assembly. The assembly of metagenomes was carried out using SPAdes version 3.11.1 (Bankevich et al., 2012) with default parameters and the kmer list: 27, 37, 47, 57, 67, 77, 87. The assembled contigs were quality checked by mapping the raw reads to contigs using Bowtie2 version 2.2.5 (Langmead et al., 2009; Langmead and Salzberg, 2012). SAMtools version 1.8 (Li et al., 2009) was used to sort and index the mapping files and extract contig coverage information. Coverage of the assembled contigs was calculated using BEDTools2 version 2.24.0 (Quinlan and Hall, 2010), assembled contigs quality filtered to remove contigs with <90% coverage. The quality of the filtered assemblies was assessed with QUAST version 3.0 (Gurevich et al., 2013).
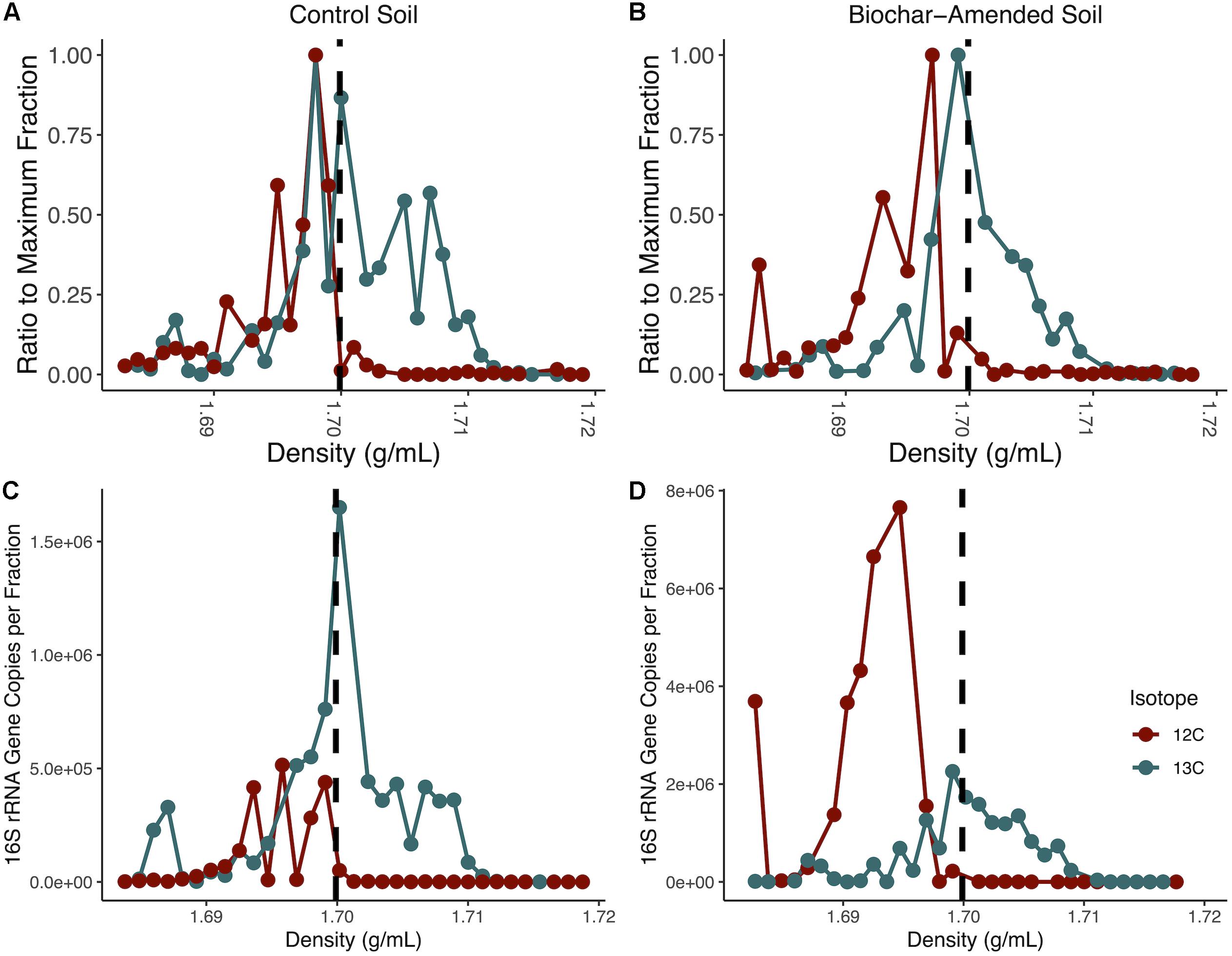
Figure 1. Isopycnic separation of DNA from density-gradient fractionation. Normalized DNA concentration in each fraction recovered after isopycnic separation of DNA from the 13C-incubated microcosms and 12C-controls for control soil microcosms (A) and biochar-amended (B). DNA was measured with Qubit for each density gradient fraction, and divided by the maximum fraction value. Each point represents an average of four replicates. Gradient fractions from 13C-incubated microcosms were subsequently pooled for metagenomic sequencing. Total copies of 16S rRNA genes measured by qPCR for each density-gradient fraction recovered from isopycnic separation of DNA from 13C-incubated microcosms and 12C-controls for control soil microcosms (C) and biochar-amended microcosms (D). Density gradient fractions > 1.70 g/ml pooled for subsequent metagenomic sequencing. Each point represents an average of four biological replicates.
The mapping data and coverage information were used to bin contigs into population genome bins separately for each 13C-metagenome with MetaBat version 2.12.1 (Kang et al., 2015) using a minimum contig length of 2000 bp. CheckM version 1.0.11 (Parks et al., 2015) was used to evaluate the level of bin completeness and contamination based on domain-level single-copy genes. Genome bins (i.e., MAGs) with over 50% completion according to CheckM were imported in to Anvio version 6.1 (Eren et al., 2015) to be manually curated, which typically improved bin quality by reduction of contamination level. The quality of refined MAGs was assessed by running CheckM and MAGs with >50% completeness and <10% contamination were used for downstream analysis.
Metagenomic Annotation and 16S rRNA Gene Analysis
Taxonomic classification for each MAG was carried out using GTDB-Tk (Chaumeil et al., 2019) against the Genome Taxonomy Database (GTDB) (Parks et al., 2018, 2019). MAG abundance was calculated using the “bin_coverage_individualassembly.pl” script2. For the assessment of taxonomic composition of each metagenome, 16S rRNA gene fragments were first recovered from metagenomes using Barrnap version 0.93. Average coverage of 16S rRNA gene fragments were determined by mapping metagenomic libraries using Bowtie2 version 2.2.5 (Langmead et al., 2009; Langmead and Salzberg, 2012) and mean depth calculated using the jgi_summarize_bam_contig_depths script in MetaBat version 2.12.1 (Kang et al., 2015). To assess community structure, Barrnap output sequences were parsed to remove 23S and 5S gene fragments then input in the RDP classifier (Wang et al., 2007) with confidence cutoff of 80%. The resulting output was used to generate a Bray–Curtis distance matrix in Rstudio v. 3.3.2 using the phyloseq package (McMurdie and Holmes, 2013). Protein-coding genes within the MAGs were identified using Prodigal version 2.6.3 (Hyatt et al., 2010) and functional annotation was carried out using GHOSTKOALA (Kanehisa et al., 2016). We specifically focused on the effect of each treatment on the presence or absence of genes for the catabolic processes of various C-complexes with different decomposability, ranging from the highly recalcitrant aromatic compounds to the more labile monosaccharides, sugar acids and sugar alcohols, more attention was also given to the genes for N metabolism. Statistics were performed using Rstudio v. 3.3.2 with general dependency on the following packages: ggplot2 (Wickham, 2009), dplyr (Wickham et al., 2019), cowplot (Wilke, 2017) and reshape2 (Wickham, 2007). The vegan R-package (Oksanen et al., 2018) provided tools to calculate non-metric multi-dimensional scaling on Bray-Curtis distance matrix (vegdist) and significant differences in the functional and taxonomic communities between treatments were tested with permutational analysis of variance (PERMANOVA) (Anderson and Walsh, 2013) and analysis of similarity (ANOSIM) (Clarke et al., 2008). To identify genes that were differentially present between active community of control and biochar-amended samples, DESeq2 package was employed (Anders and Huber, 2010). A count table of functional annotations was generated using the Kyoto Encyclopedia of Genes and Genomes (KEGG) orthology (KO) terms. Each column represented a metagenome and each element was the count of reads from the metagenome assigned to the KO term. DESeq2 was used with default settings to estimate the effective size library and variance to normalize the counts prior to the detection of difference between biochar-amended and control metagenomes for each KO term.
Results
Enrichment of 13C-DNA and Statistics of Metagenomes
Our study focused on soils collected 2 years after the initial addition of biochar to an Oxisol soil under napiergrass cultivation (Yu et al., 2018). Eight samples from the biochar-amended and control plots, collected prior to harvest, were used to determine soil chemical characteristics. Between plots, few exhibited significant differences in elemental concentration and nutrient status (Supplementary Table S1). Mean C concentration (C%) of biochar-amended soils was previously shown to be significantly higher compared to soils from control plots (Yu et al., 2019). Similar to our previous findings, no statistical differences between soil plots were observed in soil base cations (calcium [Ca2+], sodium [Na–], magnesium [Mg2+], and potassium [K+]), pH or total nitrogen concentration. Comparison of the active community between biochar-amended and control soil microcosms was based on the respiration, or cumulative gas production. The rate of CO2 production was not significantly different between biochar-amended and control soil microcosms (Supplementary Figure S1). After 14 days of incubation, CO2 concentration comprised approximately 15% of the headspace. In our previous comparative metagenomic study, we found significantly higher copies of genes involved in denitrification (Yu et al., 2019), therefore in the current experiment we measured N2O gas to further explore this. However, no significant difference was found between N2O production rates in the biochar-amended and control microcosms (Supplementary Figure S1).
Total DNA concentrations were measured in 23 density gradient fractions to detect buoyant density shifts after the mineralization of 12C- or 13C-labeled perennial ryegrass after 14 days. The heavy density fractions had a buoyant density between 1.70 and 1.717g ml–1. In the control soil microcosms the heavy density fractions contained between 58-times to 2.9-times more DNA than the fractions from the soil microcosms that contained the 12C- labeled perennial ryegrass. Biochar-amended soil microcosms heavy density fractions contained between 166.8-times to 16.4-times more DNA than the DNA fractions from the soil microcosms that contained the 12C- labeled perennial ryegrass (Figure 1). To further confirm the enrichment of 13C-labeled DNA, the 16S rRNA gene was quantified in density fractions between 1.683 and 1.718 g ml–1. The heavy density-gradient factions with buoyant density ranging from 1.701 to 1.711g ml–1 contained over 100-times more 16S rRNA gene copies in 13C-incubated samples than in the 12C-controls for both control and biochar-amended microcosms (Figures 1A,B). Heavy gradient fractions from biochar-amended and control microcosms containing at least 100-times higher levels of 13C incorporation were thereafter pooled for each microcosm for subsequent shotgun metagenomic sequencing.
13C-labeled DNA was sequenced from four replicate samples representing the biochar-amended soils (Plots 1, 3, 4, 8) and four samples representing the control soils (Plots 2, 5, 6, 7), yielding approximately 24–53 megabytes of short paired-end sequence data per sample. The Nonpareil algorithm that was used to estimated coverage based on the read redundancy value revealed an average coverage of approximately 0.65 and 0.54 for metagenomes obtained from biochar-amended and control Oxisol samples, respectively (Supplementary Figure S2). On average, the coverage of the DNA-SIP metagenomes (0.60, this study) was much higher compared to our previous study using whole community metagenomes (0.32, Yu et al., 2019) of the same soils. The sequence diversity values, a measure of alpha-diversity derived from Nonpareil curves, exhibited no differences between the biochar-amended (average, 21.23) and control soils (average, 21.04). Metagenomes from 13C-labeled biochar-amended and control soil were assembled and quality checked to produce contigs for binning. The quality assembled contigs in biochar-amended and control metagenomes amounted to 361,137 and 258,035 bp within contigs longer than 1.5 kb, respectively. The N50 values averaged 580 and 592 bp from biochar-amended and control metagenomes. Sequence statistics for each treatment plot were summarized in Table 1.
Active Community Taxonomic Composition and Functional Diversity
The taxonomic affiliations of recovered 16S rRNA gene fragments (metagenome-derived) showed little to no differences between the biochar-amended and control metagenomes (Figure 2). The active bacterial community in biochar-amended and control metagenomes were primarily represented by the phyla Actinobacteria at 71.5 and 80.0%, followed by Proteobacteria at 15.7 and 6.5%, respectively (Figure 2). The next most abundant bacterial phyla in the biochar-amended metagenomes were the Bacteroidetes and Gemmatimonadetes which represented 1.6 and 1.5% of the community, respectively. In the control metagenomes, Gemmatimonadetes composed 1.0% of the community and Bacteroidetes represented 0.4%. The remaining bacterial phyla represented less than 1% of the community. The active archaeal phyla in the biochar-amended and control metagenomes were represented by Crenarchaeota at 4.9 and 5.3%, followed by Woesearchaeota at 4.4 and 4.5%, of the community respectively. In the control metagenomes, Euryarchaeota represented 1.7% of the community, but were <1% in the biochar-amended metagenomes.
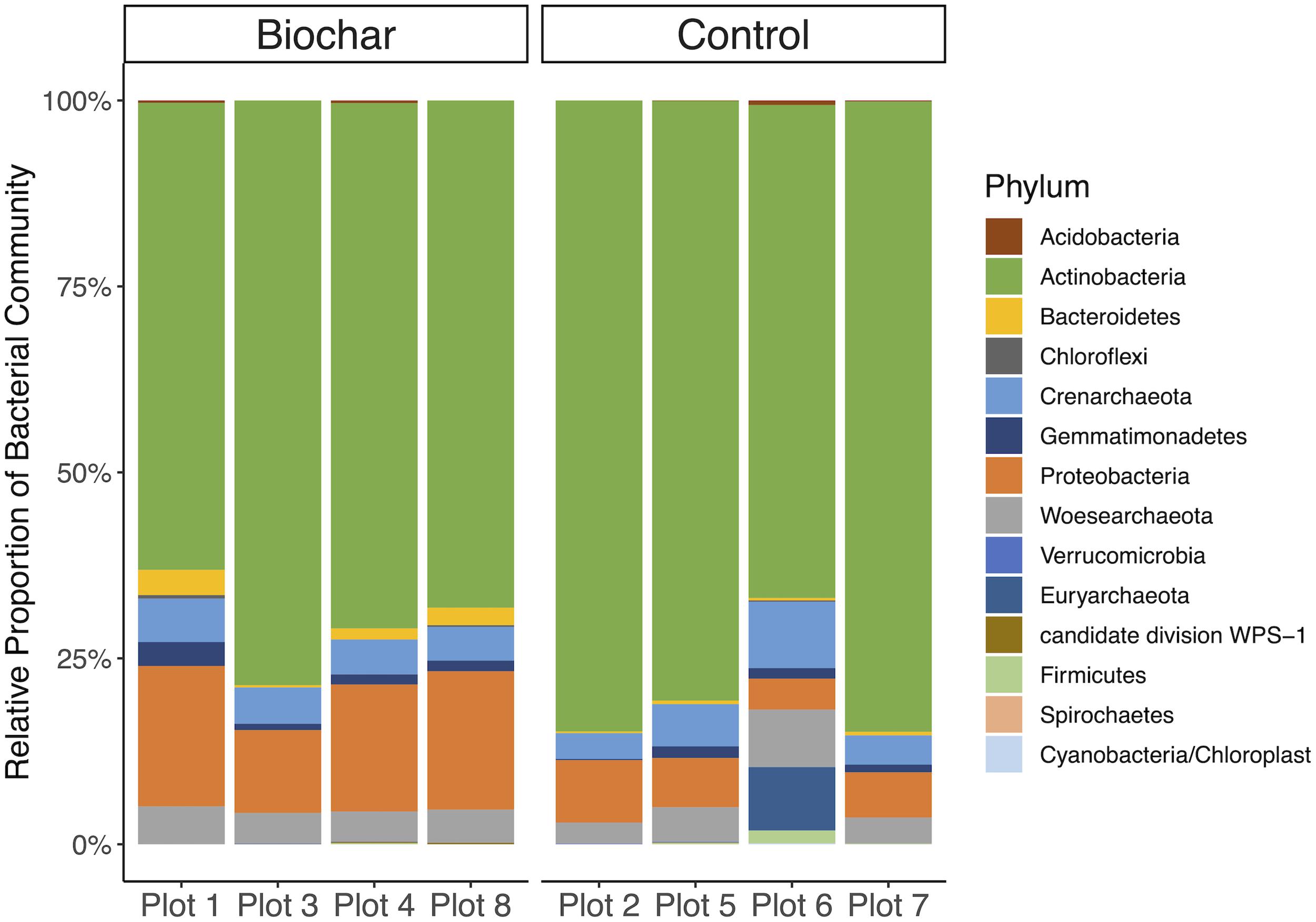
Figure 2. Taxonomic affiliation of recovered 16S rRNA gene fragments. Mean relative abundance of bacterial phyla for each plot for biochar-amended and control treatments. Underlying data is based on average coverage depth of 16S rRNA gene-encoding fragments recovered from metagenomic datasets.
Biochar had no significant effect the relative abundance of most bacterial phyla, though Proteobacteria and Bacteroidetes were significantly enriched in the biochar-amended metagenomes (two-tailed t-test, P < 0.05). Biochar also did not cause a significant shift in taxonomic β-diversity, based on Bray–Curtis distances of phylum-level active community composition (ANOSIM, P = 0.66) (Figure 3A). In addition, biochar amendment generally did not significantly shift the functional gene content of the active community, summarized as KO terms (ANOSIM, P = 0.16) (Figure 3B). However, of the 6097 KO terms with abundances adequate for P-value assignment in DESeq2, three KO terms differed significantly (adjusted P < 0.05) and another eight differed nearly significantly (adjusted P < 0.1) between control and biochar-amended metagenomes. DESeq2 analysis revealed a statistically significant decrease in nitrate reductase abundance (KO00370 narG; narZ; nxrA) in biochar-amended metagenomes and a significant enrichment of genes involved in bacterial motility-pilus systems and type VI secretion systems (Supplementary Table S2).
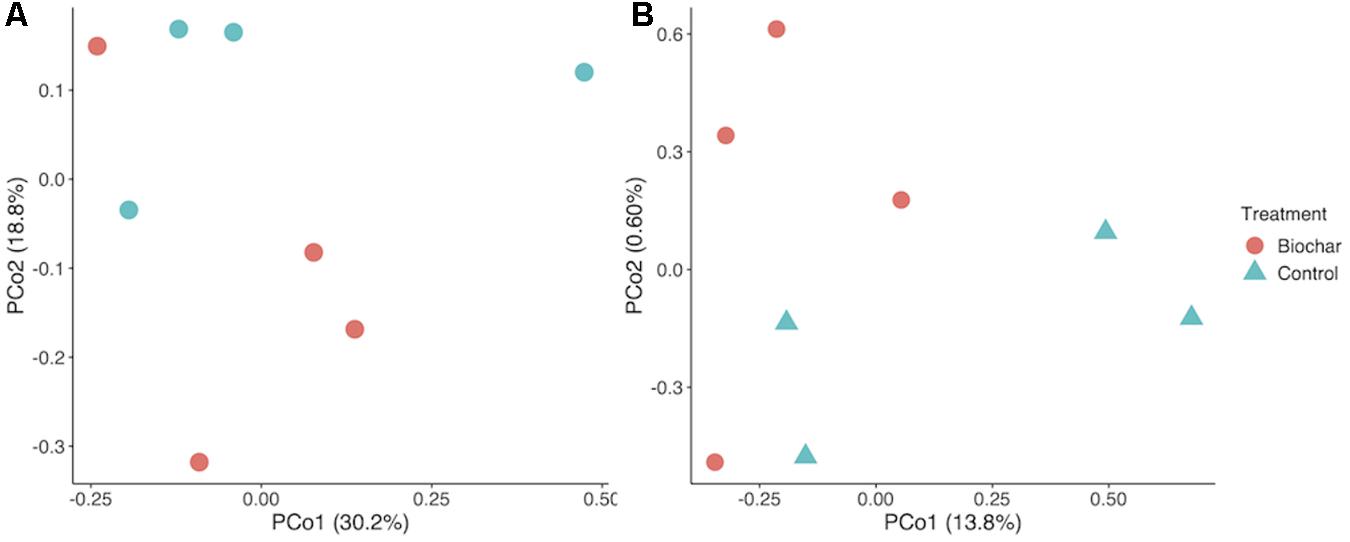
Figure 3. Taxonomic and functional shifts as an effect of biochar amendment. (A) PCoA plot of taxonomic community composition. (B) Principle coordinate analysis (PCoA) Plot of KO term annotations. Underlying data are based on Bray–Curtis distance matrix derived from a KO term count matrix. Underlying data are a Bray–Curtis distance matrix of 16S rRNA gene-encoding fragments recovered with Barrnap and processed in the RDP classifier.
Recovery of MAGs and Diversity of MAGs Involved in C and N Cycling
To precisely identify and quantify individual populations in the active community, we performed genome binning analysis of the individually assembled metagenomic data sets. Between 20 and 48 bins were recovered through binning for each individual metagenome. Due to low completeness of some MAGs, a threshold of 50% completeness and less than 10% contamination, based on the presence of 71 single-copy bacterial genes, was established for further analysis. These medium- to high-quality MAGs collectively recruited about 4.11 and 4.81% of the short reads, on average, for the both13C-biochar-amended and 13C-control, respectively. After refining genomic bins, 84 population MAGs (49 and 35 MAGs from 13C-biochar-amended and 13C-control) remained, these represented ∼25% of the total MAGs obtained. Assigned taxonomies at the family-level and genomic characteristics of genome bins used in this study are summarized in Table 2 (Supplementary Table S3). Genome size ranged from 1.84 to 11.9 Mbp, and G+C% content varied from 58.3% to 73.1% (Supplementary Table S3). Inferred taxonomy revealed that MAGs recovered from the active community represented members of Acidobacteria, Actinobacteria, Gemmatimonadetes, and Proteobacteria (Alphaproteobacteria, Betaproteobacteria, and Gammaproteobacteria) in both soil metagenomes, whereas Myxococcota (Deltaproteobacteria) were characteristic of biochar-amended soils (Supplementary Table S3).
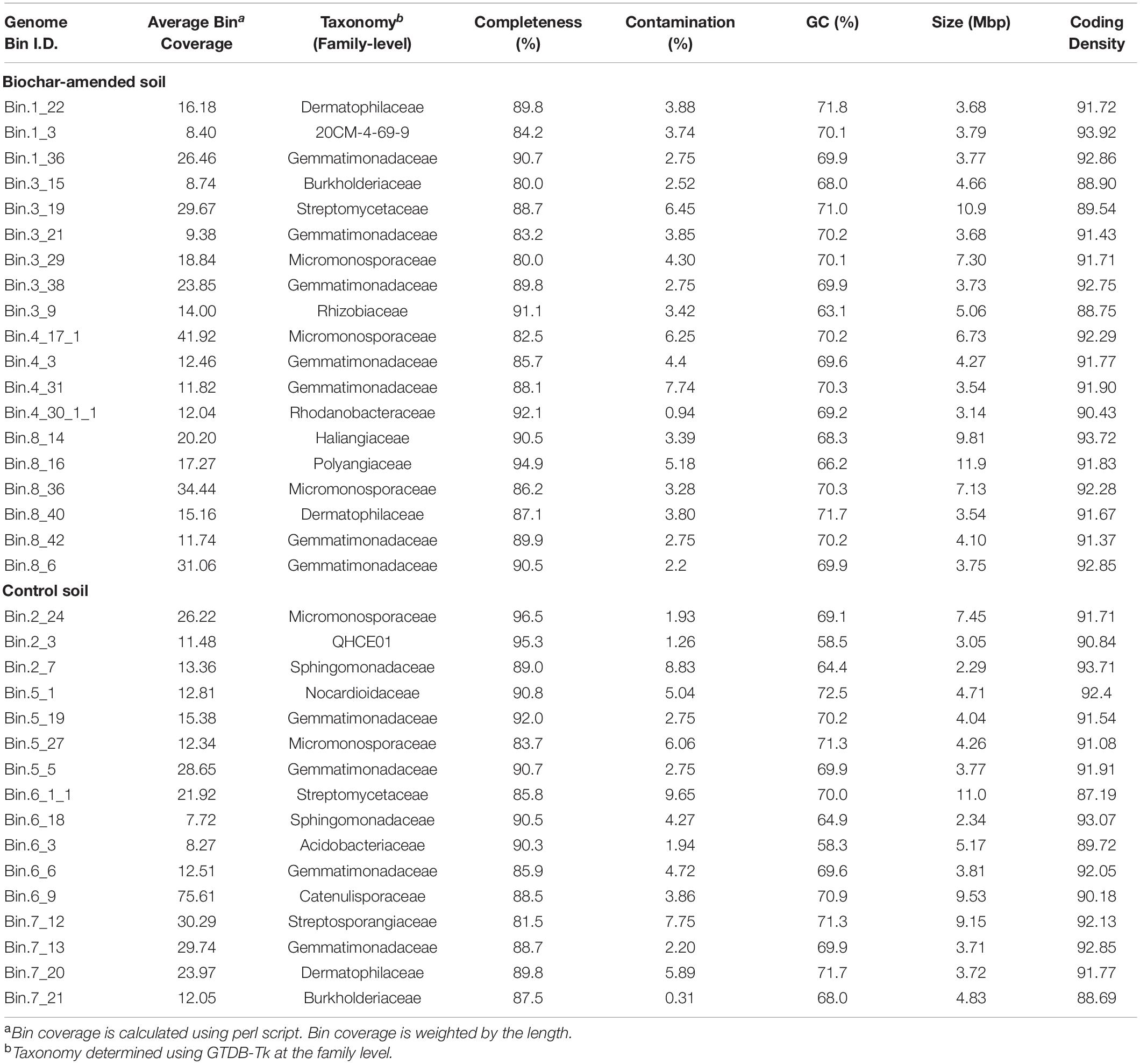
Table 2. Taxonomic classification and characteristics of genome bins that were at least 80% completeness calculated from CheckM.
Estimates of MAG abundance within a metagenome was calculated by normalizing average bin coverage by the contig lengths. The majority of recovered MAGs in both the 13C-biochar-amended and 13C-control metagenomes belonged to phylum Actinobacteria (Figure 4), represented by Actinomycetales (avg. coverage of metagenome: 5% biochar-amended, 11.6% control), Mycobacteriales (avg. coverage of metagenome: 13.3% biochar-amended, 13.7% control), Propionibacteriales (avg. coverage of metagenome: 1.7% biochar-amended, 1.4% control), Streptosporangiales (avg. coverage of metagenome: 7% biochar-amended, 10.1% control), 20CM-4-69-9 (avg. coverage of metagenome: 0.8% biochar-amended, 0.8% control), and Streptomycetales (avg. coverage of metagenome: 43.6% biochar-amended, 48.1% control) (Figure 4). Approximately 20% (10/49) of MAGs recovered from 13C-biochar-amended and 13C-control soils were taxonomically assigned at the order-level to Streptomycetales. The next most abundant MAGs recovered were assigned to phylum Gemmatimonadetes which represented an average coverage of 11.9% (7 MAGs) and 10% (5 MAGs) of 13C-biochar-amended and 13C-control metagenomes, respectively (Figure 4A). MAGs assigned to phylum Proteobacteria, represented by order Burkholderiales (avg. coverage of metagenome: 0.8% biochar-amended, 1.3% control) and Sphingomonadales (avg. coverage of metagenome: 1.8% biochar-amended, 2.2% control), were recovered from both 13C-biochar-amended and 13C-control metagenomes. Proteobacterial orders Rhizobiales and Xanthomonadales MAGs were only recovered from the biochar-amended metagenomes and comprised on average 2.9 and 0.8% coverage of the 13C-biochar-amended metagenomes, respectively. Additionally, the Myxococcota were only recovered from one 13C-biochar-amended metagenomes, further classified as Haliangiales and Polyangiales with 1.9% and 2.4% of coverage of the Plot 8 metagenome, respectively (Figure 4B). Acidobacteria MAGs recovered from 13C-biochar-amended and 13C-control metagenomes were assigned to order Vicinamibacterales and Acidobacteriales, respectively, and represented 0.9% of the coverage their respective metagenome (Figure 4).
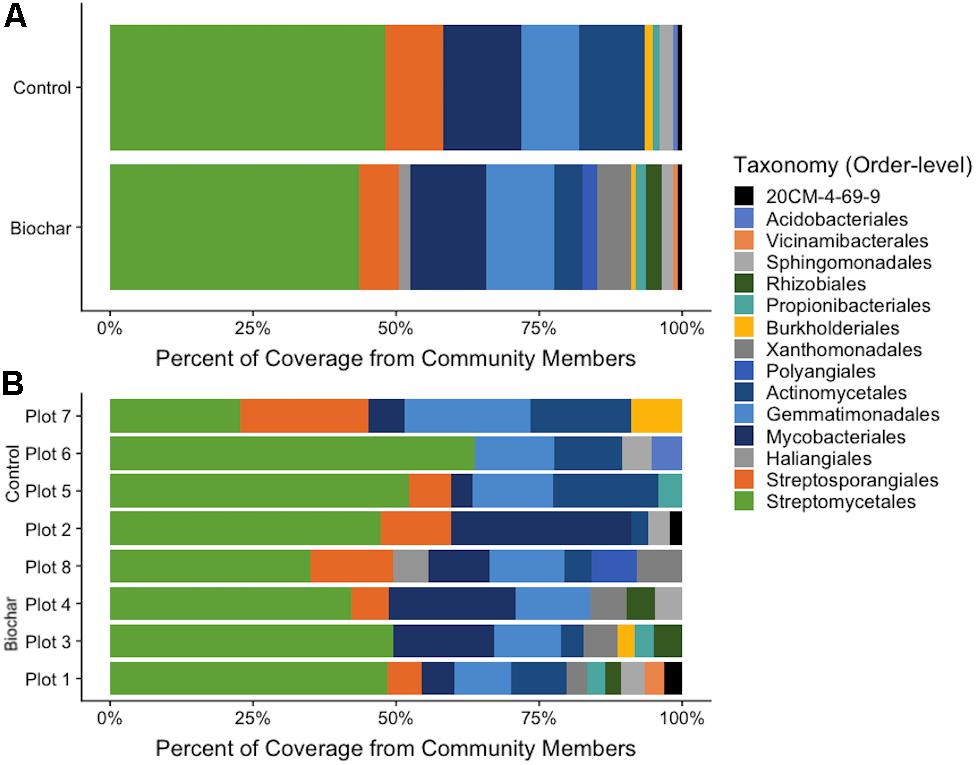
Figure 4. Proportion of abundance of recovered populations from metagenomes. (A) Proportion of MAG abundance from biochar-amended and control metagenomes. (B) Proportion of MAG coverage from each plot. Abundance was calculated as bin coverage normalized by contig lengths. Taxonomic classification is based on GTDB-Tk database.
The examination of agriculturally relevant N-cycling genes showed that 46 MAGs, representing different bacterial phyla, possessed at least one gene involved in denitrification. Genes involved in nitrification and nitrogen fixation were not observed in the recovered MAGs. Among MAGs that possessed denitrification genes, 25 and 21 MAGs were recovered from 13C-biochar-amended and 13C-control metagenomes, respectively. MAGs obtained from both soils contained a gene involved in single steps of the denitrification pathway. Those that contained genes involved in denitrification generally belonged to the phyla Actinobacteria and Proteobacteria. Of the 34 Actinobacteria MAGs that possessed denitrification genes, four MAGs recovered from biochar-amended soils and six MAGs recovered from control soils contained at least one copy of nirK and narG genes (Figures 5A,B). Nearly all MAGs assigned to Streptomycetales and Streptosporangiales had at least one copy of narG. Two Alphaproteobacteria MAGs recovered biochar-amended soil contained one copy of either nirK or narG genes, however, Alphaproteobacteria MAGs recovered from the control soil did not possess denitrification genes. Both Burkholderiales MAGs possessed all necessary genes to perform complete denitrification (i.e., reduction of NO3– or NO2– to N2) (Figure 5). At least one copy of nirK and norB genes were found in a Gammaproteobacteria (Bin3.6) and Deltaproteobacteria (Bin8.14) MAG recovered from biochar-amended soil. Nearly half of Gemmatimonadetes MAGs contained a copy of nirK, of these four MAGs, all recovered from biochar-amended metagenomes, also contained a copy of nosZ. In addition, the Acidobacteria MAG recovered from the biochar-amended soil (Bin 1.33) possessed the nosZ gene, while nosZ was not observed in the Acidobacteria MAG recovered from the control metagenomes. Other N-cycling genes observed in recovered MAGs included a gene for a cytochrome c nitrite reductase (nrfA) associated with dissimilatory nitrate reduction to ammonium (DNRA), which was found in two MAGs recovered from biochar-amended metagenomes (Bin1.21 and Bin8.9_1_1). All recovered Gemmatimonadetes MAGs also possessed nagB (ammonia from amino sugars) (Figures 5A,B).
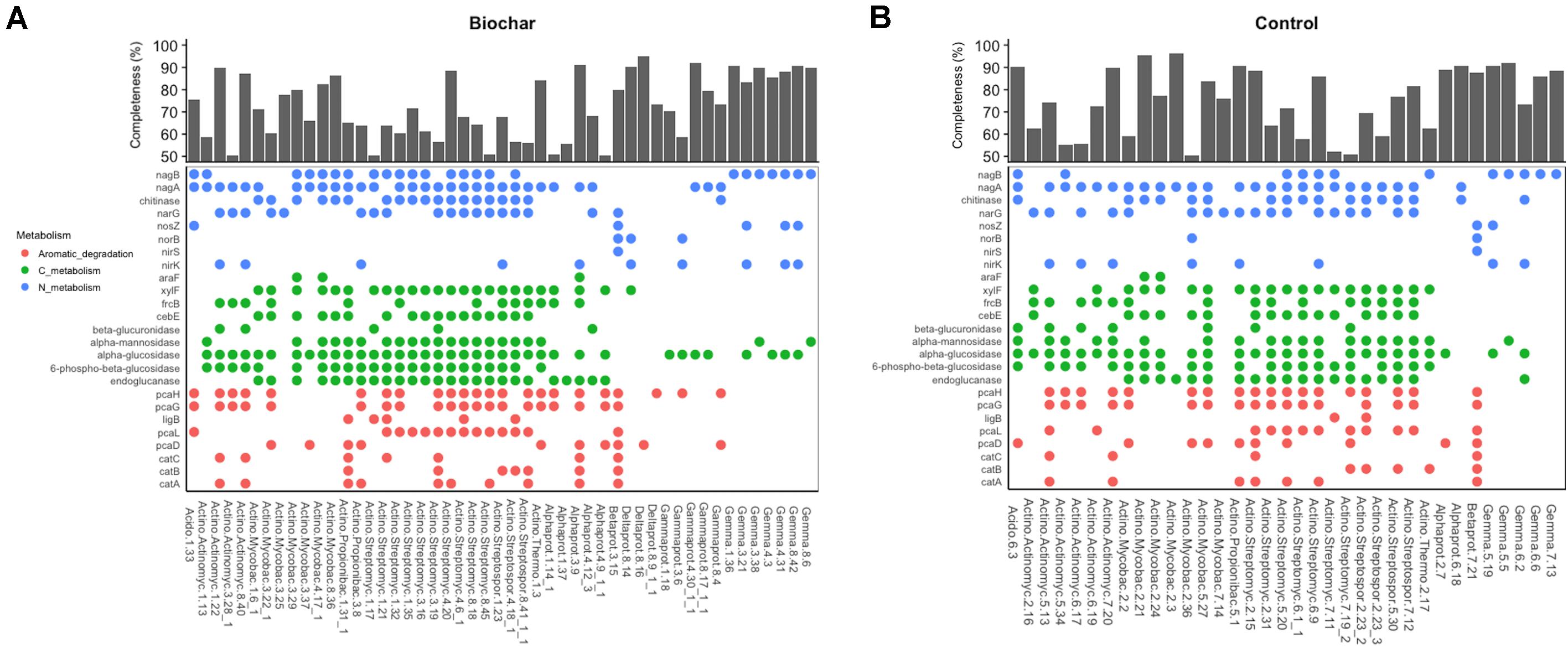
Figure 5. Metabolic features of medium- and high-quality MAGs recovered from biochar-amended and control metagenomes. (A) Presence/absence of gene in MAGs recovered from biochar-amended metagenomes and completeness of biochar MAGs and taxonomic classification at phylum-level. (B) Presence/absence of gene in MAGs recovered from control metagenomes and completeness of control MAGs and taxonomic classification at phylum-level.
In addition to N-cycling, we sought to identify genes in the recovered MAGs that encode for enzymes directly involved in the decomposition of plant organic carbon via hydrolysis of glycosidic bonds that target cellulose (e.g., endoglucanases), hemi-cellulose (e.g., xylanases), cellobiose (e.g., beta-glucosidase), and ring-opening enzymes involved in degradation of aromatic compounds prevalent in soils. Most Gemmatimonadetes MAGs contained genes involved in degradation of labile carbohydrates such as endoglucanase, alpha-glucosidase and alpha-mannosidase but did not have genes for aromatic degradation (Figures 5A,B). On the other hand, both Betaproteobacteria (i.e., Burkholderiales) MAGs possessed key genes in the beta-ketoadipate pathway including genes for catechol ortho-cleavage to 3-oxoadipate (i.e., catABC and pcaDL) and the ring-opening step of protocatechuate degradation (i.e., pcaGH). However, genes for the degradation of more labile compounds were not observed in these MAGs. Alphaproteobacteria and Gammaproteobacteria MAGs possessed genes involved in a single step of the beta-ketoadipate pathway and genes associated with degradation of labile carbohydrates and sugar transport systems. Overall, Actinobacteria MAGs possessed multiple copies of gene associated with the degradation of plant biomass C, such as cellulose, hemi-cellulose and cellobiose (i.e., endoglucanases, beta-glucosidases, alpha-glucosidases, alpha-mannosidase), binding proteins involved in sugar transport, ring-opening enzymes or a partial beta-ketoadipate pathway (Figures 5A,B).
Discussion
Biochar Had Negligible Effects on the Active Soil Community
In this study, we examined the active community of a tropical Oxisol 2 years after the initial addition of biochar under napiergrass cultivation. We investigated the impact of biochar amendment on the genomic diversity and functional potential of active soil bacterial community using DNA-SIP shotgun metagenomics and MAGs (see below). Here, we did not observe a significant shift in the composition of the active community in response to biochar. This finding contrasts with our previous study, based on 16S rRNA amplicon analysis, which found significant changes in the community composition and alpha diversity in response to a month and a year after biochar addition (Yu et al., 2018). However, this is consistent with our previous comparative metagenomic study on the total community, which showed that biochar did not have a significant effect on the community composition 2 years after biochar addition (Yu et al., 2019). Altogether, this may indicate that the soil microbial community is resilient to a single biochar addition as we were unable to detect compositional changes in the whole and active community as time from the initial disturbance increased.
The strength of environmental disturbances and the frequency it is applied can have an effect on the resilience of the microbial composition (Allison and Martiny, 2009). Here and in our previous studies, a low-volatile matter biochar was only added at the initiation of the experiment. In addition, the microbial community was initially sensitive to perturbations related to the addition of biochar but the strongest determinant of the community composition was soil type, as well as the degree of biochar-related changes in composition determined by soil type (Yu et al., 2018). Although examination of 16S rRNA gene fragments extracted from each individual metagenome did show a significant increase in Proteobacteria and Bacteroidetes abundance in the biochar metagenomes, it is important to note that the number of recovered 16S rRNA genes per metagenome was extremely low compared to the number of gene-encoding sequences. This may reflect difficulties presented by the massive data volume of metagenomes, high sequence similarity of 16S rRNA genes and skewed species abundance which make rRNA recovery from metagenomic datasets difficult (Yuan et al., 2015).
In addition, the similarity of the active communities between biochar-amended and control soils may reflect the conditions of the experimental set up. For instance, sieved soils resulting in different size fractions have been shown to support distinct microbial communities (Bach et al., 2018; Fox et al., 2018) and the input of fresh organic matter has been shown to stimulate a select group of bacteria (Pascault et al., 2013). Previous studies using 13C-DNA-SIP showed that the C assimilating bacterial phyla found in heavy-fraction soil DNA enriched with maize and wheat residue were primarily distributed among phyla Actinobacteria, Proteobacteria, and Firmicutes, and the quality of plant material has a strong influence on the composition of the degrading communities (Bernard et al., 2007; Pascault et al., 2013; Fan et al., 2014; Su et al., 2017). Here, the major bacterial phyla recovered from the active community were primarily distributed among known plant biomass degrading Actinobacteria suborders, such as Actinomycetales, Streptomycetales, Propionibacteriales, Mycobacteriales, with genomes known to be particularly enriched in carbohydrate-active enzyme genes (Lewin et al., 2016).
Previous studies have examined how short- and longer-term biochar application affects soil communities (Anders et al., 2013; Noyce et al., 2015; Jenkins et al., 2017; Zhang et al., 2019). They principally revealed that biochar amendment is accompanied by significant shifts in soil chemistry and the soil microbial community. Other studies have observed negligible biochar effects on soil community structure, GHG production, and plant productivity or that biochar effects were transient and showed no long-term effects (1–3 years) on microbial growth rates in agricultural soils (Rousk et al., 2013; Meschewski et al., 2019; Azeem et al., 2020). Our results concur with the latter in that biochar amendment did not significantly shift the active taxonomic or functional communities, at least over a period of 2 years.
These findings contradict the results of our previous study on the same soils (Yu et al., 2019), which observed significantly higher relative abundances of Proteobacteria and Bacteroidetes and an enrichment of genes involved in pathways, such as denitrification, respiration and metabolism of aromatic compounds with biochar amendment. We also failed to find support of our initial hypothesis, based on our earlier findings, that biochar would have a significant positive effect on genes involved in denitrification in the active microbial community. The results of our current study showed that the narG gene, encoding nitrate reductase, was significantly higher in the control soil metagenomes, while no significant differences were observed for other denitrification genes, such as nirK/nirS, norB, and nosZ. This was not expected since previous studies focused on biochar effects on denitrification found that biochar increased the abundance of nitrite reductase genes (nirK/nirS) (Ducey et al., 2013; Liu et al., 2018), and nitrous oxide reductase genes (nosZ) (Xu et al., 2014; Harter et al., 2016) in soil. Overall, our results showed that biochar addition did not affect the active denitrifying community, which may suggest that long-term effects of biochar application do not alter the potential for microbially-mediated N loss from these agricultural soils.
We also observed a significant increase in genes for an outer membrane usher protein (fimD) and type VI secretion system protein (impL) associated with biochar, which may indicate bacterial movement and communication (Gallique et al., 2017; Yang and Dirk van Elsas, 2018). However, whether this is impacted by biochar or reflect indirect effects of the microcosm experiment remain unresolved and outside the scope of this study. Based on these results we conclude that even if the agricultural application of biochar impacts the soil microbial community in the short-term the effects are not lasting in the active community. These findings may suggest that the active soil microbial community is functionally resilient to a single biochar application, and biochar effects may be overwritten by the other factors, such as land management or cropping system (Hardy et al., 2019; Azeem et al., 2020).
Recovery of Populations of the Active Community
By coupling SIP with shotgun metagenomics we selected for the active community and improved resolution within the high diversity environment of the soil and demonstrated the ability to assemble several high quality MAGs. By assembling MAGs of active populations, we have gained a new depth of insight into the putative nutrient cycling and life strategies of some Oxisol agricultural soil microorganisms that was not possible with only metagenomics. We recovered 12 Gemmatimonadetes MAGs from 13C-DNA with an average coverage of about 11%, which composed a higher proportion of the active community than expected. Gemmatimonadetes were better represented in the recovered MAGs than compared to our previous studies based on 16S rRNA amplicon and rRNA gene fragments (metagenome-derived), which composed approximately 1–1.5% of the Oxisol soil communities (Yu et al., 2018, 2019). This contrast with other SIP studies, which have generally recovered Gemmatimonadetes sequences from the unlabeled light fraction, suggesting this group may be oligotrophic and likely correspond to K-strategies (Bernard et al., 2007; Pascault et al., 2013). Our finding highlights that low abundant soil bacteria can be fast-growing (i.e., sufficient growth within 14 days) and metabolically versatile. For instance, Gemmatimonadetes MAGs encoded the genes involved in labile C (e.g., starch) metabolism and organic N cycling (e.g., N-acetyl glucosaminidase). In addition, four of the Gemmatimonadetes MAGs encoded enzymes necessary for the reduction of NO2 and N2O (i.e., nirK and nosZ), which is consistent with studies that have reported Gemmatimonadetes as nirK denitrifiers and have N2O reduction ability (Helen et al., 2016; Park et al., 2017). Interestingly, this finding contrasts somewhat with earlier studies that have previously shown that the genetic linkage of nirS and nosZ is the predominant pattern of denitrification genes (Graf et al., 2014). This may suggest that less abundant microorganisms may play an important role in increasing functional redundancy, which can enhance the ability of soil communities to counteract environmental disturbances. The functional importance of low-abundant microbes may be due to effects that are disproportionately large given their abundance (i.e., keystone species) or as a provision of the insurance effect, that rare or low abundance species may offer a pool of genetic resources that can be activated when the appropriate conditions are met (Jousset et al., 2017). Shade et al. (2014) estimated that conditionally rare taxa, those which are rare in most conditions but become dominant occasionally, comprised 1.5–28% of all microbes.
In both the biochar-amended and control metagenomes, MAGs that contain a nitrite reductase gene predominantly harbored nirK, which encodes the copper-containing nitrite reductase. The cytochrome cd1 nitrite reductase encoded by the nirS gene was only found in the Burkholderiales MAGs, which also were the only MAGs that encoded all enzymes required to perform complete denitrification. Variable abundance ratios of nirK and nirS genes have previously been reported, with a trend of nirK abundances to be more sensitive to nutrient changes and higher in bulk soil, and nirS abundance to be higher in the rhizosphere (Henry et al., 2004; Kandeler et al., 2006; Bárta et al., 2010). This would be consistent with our soil collection (i.e., bulk soil) although we did not observe significant differences in soil chemical data. These findings are consistent with previous studies that have proposed a modular assembly for denitrification pathways in soils and suggested shared regulatory mechanisms that may constrain the loss of nor and nos in nirS-type denitrifiers (Graf et al., 2014; Orellana et al., 2014). In addition, nine MAGs related to several actinobacterial suborders had nirK and narG genes, though whether there are co-occurrence patterns between nirK and narG remain unclear. In fact, majority of Actinobacteria MAGs from both metagenomic datasets contained at least one copy of narG gene. Earlier studies of narG diversity in soil environments have identified sequences related to those from Actinobacteria (Philippot et al., 2002; Palmer and Horn, 2012), which may highlight the importance of Actinobacteria in the nitrate reducing community of Oxisol soils. Altogether, these findings highlight the importance of accounting for the different organisms and their interactions to better understand denitrification processes in soils, as the reduction of nitrate or nitrite to N2 would require the combined participation of different N-reducing bacteria.
We explored the impact of active microbial populations in the potential breakdown and recycling of plant biomass in soils, by surveying genes associated with biomass and aromatic degradation in the recovered MAGs. Overall, the Actinobacteria MAGs encoded the greatest number and variety of enzymes involved in the degradation of plant biomass, which was expected since this taxonomic group has many representatives that have been characterized for their ability to degrade a variety of labile and recalcitrant organic compounds. For example, Actinomycetes can compete with fungi for lignin degradation (De Boer et al., 2005), Mycobacteria can degrade polycyclic aromatic hydrocarbons under oligotrophic conditions (Uyttebroek et al., 2006), and aerobic cellulose degradation has been demonstrated by a number of Actinobacteria species (Anderson et al., 2012). In the biochar-amended soil metagenomes we recovered more diverse Proteobacteria MAGs, which was not surprising due to the higher amount of total C% in the biochar-amended soils. The Rhizobiales MAGs contained the genetic potential to degrade a variety plant organic C and complete or partial β-ketoadipate pathway, similar to many related Rhizobiales (MacLean et al., 2006). The β-ketoadipate pathway is present in many members of the Rhizobiaceae family, emphasizing the importance of aromatic acid catabolism in this family (Parke and Ornston, 1986). In addition to the denitrification pathway, Burkholderiales MAGs also had the complete set of genes in the β-ketoadipate pathway but did not have gene for degradation or transport of labile plant C compounds. This finding was not surprising as the representative of the Burkholderiales order (genus Cupriavidus) have been reported to degrade recalcitrant C and aromatic compounds including lignin (Shi et al., 2013) and phenoxy herbicides (Cuadrado et al., 2010). From a functional point of view, the active population was composed of a small diversity of species that harbored different degradation capabilities, which may suggest the different trophic behaviors with copiotrophic Actinobacteria (Ho et al., 2017) and Rhizobiales (Bastida et al., 2015) and oligotrophic Acidobacteria (Fierer et al., 2007; Ho et al., 2017) and Burkholderiales (Nicolitch et al., 2019) with the genetic potential to degrade labile (e.g., cellulose and hemicellulose) or more refractory compounds like aromatics, respectively.
Conclusion
The combination of metagenome and MAG analysis allowed for an increased understanding of the potential biological functions of the active soil microbial community as altered by biochar addition. Potential carbon cycling pathways in both datasets appeared to not be significantly altered by biochar, especially related to complex carbon sources. In addition, we observed no difference in genes involved in the denitrification pathway. However narG was significantly higher in the control metagenomes of the active communities. In summary, this study demonstrated the application of DNA-SIP combined with shotgun metagenomics and genomic binning to identify active populations in a tropical Oxisol soil under biochar amendment. The results indicated that the taxonomic and functional composition of the active community was not significantly affected by biochar amendment. Finally, we were able to recover high quality MAGs of low-abundance populations using DNA-SIP to target the active community. These results may suggest that application of biochar may influence the microbial communities and their function soon after application, however, the effects on the microbial community are not lasting. Although biochar did not have lasting effects on the active soil community, it may still be a promising strategy for the intended purpose of biochar in agricultural soil. For example, biochar addition can result in long-term sequestration of C without a significant long-term influence on the soil microbial community which may lead to unexpected nutrient losses from the soil through biotic processes, such as denitrification.
Data Availability Statement
The datasets for the biochar-amended and control metagenomes raw sequences and assembled MAGs can be found in GenBank deposited under PRJNA622594. This data can be found here: (https://www.ncbi.nlm.nih.gov/bioproject/PRJNA622594).
Author Contributions
JY conducted the soil incubations and library preparation and wrote up the manuscript. JY, MP, and CP analyzed the data and discussed the results. LD, SC, and JD designed the field experiment, collected the soil samples, and carried out the chemical characterization of soils. All the authors contributed to the article and approved the submitted version.
Funding
This project was supported by grants from the United States Department of Agriculture National Institute of Food and Agriculture (USDA-NIFA) award number 2012-67020-30234, USDA-NIFA Hatch project HAW01130-H managed by the College of Tropical Agriculture and Human Resources, and graduate student funding from the Arizona State University School of Life Sciences Completion Fellowship.
Conflict of Interest
The authors declare that the research was conducted in the absence of any commercial or financial relationships that could be construed as a potential conflict of interest.
Acknowledgments
The authors wish to thank Susan Migita and Poamoho Research Station, Stephen Romaniello, and Natasha Zolotova from the W.M. Keck Foundation Laboratory for Environmental Biochemistry, and Diacarbon Energy, Inc. for providing the biochar.
Supplementary Material
The Supplementary Material for this article can be found online at: https://www.frontiersin.org/articles/10.3389/fmicb.2020.587972/full#supplementary-material
Footnotes
- ^ www.diacarbon.com
- ^ http://github.com/seanmcallister/bin_coverage_tools.git
- ^ https:github.com/tseemann/barrnap
References
Allison, S. D., and Martiny, J. B. H. (2009). Resistance, resilience, and redundancy in microbial communities/arttitle. Light Evol. 2, 149–166. doi: 10.17226/12501
Anders, E., Watzinger, A., Rempt, F., Kitzler, B., Wimmer, B., Zehetner, F., et al. (2013). Biochar affects the structure rather than the total biomass of microbial communities in temperate soils. Agric. Food Sci. 22, 404–423. doi: 10.23986/afsci.8095
Anders, S., and Huber, W. (2010). Differential expression analysis for sequence count data. Genome Biol. 11:R106. doi: 10.1186/gb-2010-11-10-r106
Anderson, C. R., Condron, L. M., Clough, T. J., Fiers, M., Stewart, A., Hill, R. A., et al. (2011). Biochar induced soil microbial community change: implications for biogeochemical cycling of carbon, nitrogen and phosphorus. Pedobiologia 54, 309–320. doi: 10.1016/j.pedobi.2011.07.005
Anderson, I., Abt, B., Lykidis, A., Klenk, H. P., Kyrpides, N., and Ivanova, N. (2012). Genomics of aerobic cellulose utilization systems in actinobacteria. PLoS One 7:e39331. doi: 10.1371/journal.pone.0039331
Anderson, M. J., and Walsh, D. C. I. (2013). PERMANOVA, ANOSIM, and the Mantel test in the face of heterogeneous dispersions: what null hypothesis are you testing? Ecol. Monogr. 83, 557–574. doi: 10.1890/12-2010.1
Azeem, M., Sun, D., Crowley, D., Hayat, R., Hussain, Q., Ali, A., et al. (2020). Crop types have stronger effects on soil microbial communities and functionalities than biochar or fertilizer during two cycles of legume-cereal rotations of dry land. Sci. Total Environ. 715:136958. doi: 10.1016/j.scitotenv.2020.136958
Bach, E. M., Williams, R. J., Hargreaves, S. K., Yang, F., and Hofmockel, K. S. (2018). Greatest soil microbial diversity found in micro-habitats. Soil Biol. Biochem. 118, 217–226. doi: 10.1016/j.soilbio.2017.12.018
Bankevich, A., Nurk, S., Antipov, D., Gurevich, A. A., Dvorkin, M., Kulikov, A. S., et al. (2012). SPAdes: a new genome assembly algorithm and its applications to single-cell sequencing. J. Comput. Biol. 19, 455–477. doi: 10.1089/cmb.2012.0021
Barrow, C. J. (2012). Biochar: potential for countering land degradation and for improving agriculture. Appl. Geogr. 34, 21–28. doi: 10.1016/j.apgeog.2011.09.008
Bárta, J., Melichová, T., Vaněk, D., Picek, T., and Šantrůčková, H. (2010). Effect of pH and dissolved organic matter on the abundance of nirK and nirS denitrifiers in spruce forest soil. Biogeochemistry 101, 123–132. doi: 10.1007/s10533-010-9430-9
Bastida, F., Selevsek, N., Torres, I. F., Hernández, T., and García, C. (2015). Soil restoration with organic amendments: linking cellular functionality and ecosystem processes. Sci. Rep. 5:15550. doi: 10.1038/srep15550
Bernard, L., Mougel, C., Maron, P. A., Nowak, V., Lévêque, J., Henault, C., et al. (2007). Dynamics and identification of soil microbial populations actively assimilating carbon from 13C-labelled wheat residue as estimated by DNA- and RNA-SIP techniques. Environ. Microbiol. 9, 752–764. doi: 10.1111/j.1462-2920.2006.01197.x
Birnie, G. D. (1978). Centrifugal Separations in Molecular and Cell Biology. Boston, MA: Butterworth & Co Publishers Ltd.
Bolger, A. M., Lohse, M., and Usadel, B. (2014). Trimmomatic: a flexible trimmer for Illumina sequence data. Bioinformatics 30, 2114–2120. doi: 10.1093/bioinformatics/btu170
Bruun, E. W., Hauggaard-Nielsen, H., Ibrahim, N., Egsgaard, H., Ambus, P., Jensen, P. A., et al. (2011). Influence of fast pyrolysis temperature on biochar labile fraction and short-term carbon loss in a loamy soil. Biomass Bioenergy 35, 1182–1189. doi: 10.1016/j.biombioe.2010.12.008
Campbell, B. M., Thornton, P., Zougmoré, R., Van Asten, P., Lipper, L., Struik, P. C., et al. (2014). Sustainable intensification: what is its role in climate smart agriculture? Curr. Opin. Environ. Sustain. 8, 39–43. doi: 10.1016/j.cosust.2014.07.002
Chaumeil, P.-A., Mussig, A. J., Hugenholtz, P., and Parks, D. H. (2019). GTDB-Tk: a toolkit to classify genomes with the genome taxonomy database. Bioinformatics doi: 10.1093/bioinformatics/btz848 [Epub ahead of print].
Chen, J., Sun, X., Li, L., Liu, X., Zhang, B., Zheng, J., et al. (2016). Change in active microbial community structure, abundance and carbon cycling in an acid rice paddy soil with the addition of biochar. Eur. J. Soil Sci. 67, 857–867. doi: 10.1111/ejss.12388
Chen, Y., and Murrell, J. C. (2010). When metagenomics meets stable-isotope probing: progress and perspectives. Trends Microbiol. 18, 157–163. doi: 10.1016/j.tim.2010.02.002
Chia, C. H., Singh, B. P., Joseph, S., Graber, E. R., and Munroe, P. (2014). Characterization of an enriched biochar. J. Anal. Appl. Pyrolysis 108, 26–34. doi: 10.1016/j.jaap.2014.05.021
Clarke, K. R., Somerfield, P. J., and Gorley, R. N. (2008). Testing of null hypotheses in exploratory community analyses: similarity profiles and biota-environment linkage. J. Exp. Mar. Biol. Ecol. 366, 56–69. doi: 10.1016/j.jembe.2008.07.009
Coyotzi, S., Pratscher, J., Murrell, J. C., and Neufeld, J. D. (2016). Targeted metagenomics of active microbial populations with stable-isotope probing. Curr. Opin. Biotechnol. 41, 1–8. doi: 10.1016/j.copbio.2016.02.017
Crusoe, M. R., Alameldin, H. F., Awad, S., Boucher, E., Caldwell, A., Cartwright, R., et al. (2015). The khmer software package: enabling efficient nucleotide sequence analysis. F1000Res. 4:900. doi: 10.12688/f1000research.6924.1
Cuadrado, V., Gomila, M., Merini, L., Giulietti, A. M., and Moore, E. R. B. (2010). Cupriavidus pampae sp. nov., a novel herbicide-degrading bacterium isolated from agricultural soil. Int. J. Syst. Evol. Microbiol. 60, 2606–2612. doi: 10.1099/ijs.0.018341-0
De Boer, W., Folman, L. B., Summerbell, R. C., and Boddy, L. (2005). Living in a fungal world: impact of fungi on soil bacterial niche development. FEMS Microbiol. Rev. 29, 795–811. doi: 10.1016/j.femsre.2004.11.005
Docherty, K. M., Borton, H. M., Espinosa, N., Gebhardt, M., Gil-Loaiza, J., Gutknecht, J. L. M., et al. (2015). Key edaphic properties largely explain temporal and geographic variation in soil microbial communities across four biomes. PLoS One 10:e0135352. doi: 10.1371/journal.pone.0135352
Ducey, T. F., Ippolito, J. A., Cantrell, K. B., Novak, J. M., and Lentz, R. D. (2013). Addition of activated switchgrass biochar to an aridic subsoil increases microbial nitrogen cycling gene abundances. Appl. Soil Ecol. 65, 65–72. doi: 10.1016/j.apsoil.2013.01.006
Dunford, E. A., and Neufeld, J. D. (2010). DNA stable-isotope probing (DNA-SIP) video link. J. Vis. Exp. 42:e2027. doi: 10.3791/2027
Elzobair, K. A., Stromberger, M. E., Ippolito, J. A., and Lentz, R. D. (2016). Contrasting effects of biochar versus manure on soil microbial communities and enzyme activities in an Aridisol. Chemosphere 142, 145–152. doi: 10.1016/j.chemosphere.2015.06.044
Eren, A. M., Esen, O. C., Quince, C., Vineis, J. H., Morrison, H. G., Sogin, M. L., et al. (2015). Anvi’o: an advanced analysis and visualization platform for ’omics data. PeerJ 3:e1319. doi: 10.7717/peerj.1319
Eyice, Ö., Namura, M., Chen, Y., Mead, A., Samavedam, S., and Schäfer, H. (2015). SIP metagenomics identifies uncultivated Methylophilaceae as dimethylsulphide degrading bacteria in soil and lake sediment. ISME J. 9, 2336–2348. doi: 10.1038/ismej.2015.37
Fan, F., Yin, C., Tang, Y., Li, Z., Song, A., Wakelin, S. A., et al. (2014). Probing potential microbial coupling of carbon and nitrogen cycling during decomposition of maize residue by13C-DNA-SIP. Soil Biol. Biochem. 70, 12–21. doi: 10.1016/j.soilbio.2013.12.002
Fierer, N., Bradford, M. A., and Jackson, R. B. (2007). Toward an ecological classification of soil bacteria. Ecology 88, 1354–1364. doi: 10.1890/05-1839
Fox, A., Ikoyi, I., Torres-Sallan, G., Lanigan, G., Schmalenberger, A., Wakelin, S., et al. (2018). The influence of aggregate size fraction and horizon position on microbial community composition. Appl. Soil Ecol. 127, 19–29. doi: 10.1016/j.apsoil.2018.02.023
Gallique, M., Bouteiller, M., and Merieau, A. (2017). The type VI secretion system: a dynamic system for bacterial communication? Front. Microbiol. 8:1454. doi: 10.3389/fmicb.2017.01454
Garnett, T., Appleby, M. C., Balmford, A., Bateman, I. J., Benton, T. G., Bloomer, P., et al. (2013). Sustainable intensification in agriculture: premises and policies. Science 341, 33–34. doi: 10.1126/science.1234485
Gomez, J. D., Denef, K., Stewart, C. E., Zheng, J., and Cotrufo, M. F. (2014). Biochar addition rate influences soil microbial abundance and activity in temperate soils. Eur. J. Soil Sci. 65, 28–39. doi: 10.1111/ejss.12097
Graf, D. R. H., Jones, C. M., and Hallin, S. (2014). Intergenomic comparisons highlight modularity of the denitrification pathway and underpin the importance of community structure for N2O emissions. PLoS One 9:e114118. doi: 10.1371/journal.pone.0114118
Gurevich, A., Saveliev, V., Vyahhi, N., and Tesler, G. (2013). QUAST: quality assessment tool for genome assemblies. Bioinformatics 29, 1072–1075. doi: 10.1093/bioinformatics/btt086
Hardy, B., Sleutel, S., Dufey, J. E., and Cornelis, J.-T. (2019). The long-term effect of biochar on soil microbial abundance, activity and community structure is overwritten by land management. Front. Environ. Sci. 7:110. doi: 10.3389/fenvs.2019.00110
Harter, J., Weigold, P., El-Hadidi, M., Huson, D. H., Kappler, A., and Behrens, S. (2016). Soil biochar amendment shapes the composition of N2O-reducing microbial communities. Sci. Total Environ. 562, 379–390. doi: 10.1016/j.scitotenv.2016.03.220
Helen, D., Kim, H., Tytgat, B., and Anne, W. (2016). Highly diverse nirK genes comprise two major clades that harbour ammonium-producing denitrifiers. BMC Genomics 17:155. doi: 10.1186/s12864-016-2465-0
Henry, S., Baudoin, E., Ló Pez-Gutiérrez, J. C., Martin-Laurent, F., Brauman, A., and Philippot, L. (2004). Quantification of denitrifying bacteria in soils by nirK gene targeted real-time PCR. J. Microbiol. Methods 59, 327–335. doi: 10.1016/j.mimet.2004.07.002
Hernandez-Soriano, M. C., Kerré, B., Kopittke, P. M., Horemans, B., and Smolders, E. (2016). Biochar affects carbon composition and stability in soil: a combined spectroscopy-microscopy study. Sci. Rep. 6:25127. doi: 10.1038/srep25127
Ho, A., Di Lonardo, D. P., and Bodelier, P. L. E. (2017). Revisiting life strategy concepts in environmental microbial ecology. FEMS Microbiol. Ecol. 93:fix006. doi: 10.1093/femsec/fix006
Hyatt, D., Chen, G. L., LoCascio, P. F., Land, M. L., Larimer, F. W., and Hauser, L. J. (2010). Prodigal: prokaryotic gene recognition and translation initiation site identification. BMC Bioinformatics 11:119. doi: 10.1186/1471-2105-11-119
Jeffery, S., Verheijen, F. G. A., van der Velde, M., and Bastos, A. C. (2011). A quantitative review of the effects of biochar application to soils on crop productivity using meta-analysis. Agric. Ecosyst. Environ. 144, 175–187. doi: 10.1016/j.agee.2011.08.015
Jenkins, J. R., Viger, M., Arnold, E. C., Harris, Z. M., Ventura, M., Miglietta, F., et al. (2017). Biochar alters the soil microbiome and soil function: results of next-generation amplicon sequencing across Europe. Glob. Change Biol. Bioenergy 9, 591–612. doi: 10.1111/gcbb.12371
Jha, P., Biswas, A. K., Lakaria, B. L., and Subba Rao, A. (2010). Biochar in agriculture - prospects and related implications. Curr. Sci. 99, 1218–1225. doi: 10.2307/24068517
Jien, S. H., and Wang, C. S. (2013). Effects of biochar on soil properties and erosion potential in a highly weathered soil. Catena 110, 225–233. doi: 10.1016/j.catena.2013.06.021
Jousset, A., Bienhold, C., Chatzinotas, A., Gallien, L., Gobet, A., Kurm, V., et al. (2017). Where less may be more: how the rare biosphere pulls ecosystems strings. ISME J. 11, 853–862. doi: 10.1038/ismej.2016.174
Kandeler, E., Deiglmayr, K., Tscherko, D., Bru, D., and Philippot, L. (2006). Abundance of narG, nirS, nirK, and nosZ genes of denitrifying bacteria during primary successions of a glacier foreland. Appl. Environ. Microbiol. 72, 5957–5962. doi: 10.1128/AEM.00439-06
Kanehisa, M., Sato, Y., and Morishima, K. (2016). BlastKOALA and GhostKOALA: KEGG tools for functional characterization of genome and metagenome sequences. J. Mol. Biol. 428, 726–731. doi: 10.1016/j.jmb.2015.11.006
Kang, D. D., Froula, J., Egan, R., and Wang, Z. (2015). MetaBAT, an efficient tool for accurately reconstructing single genomes from complex microbial communities. PeerJ 3:e1165. doi: 10.7717/peerj.1165
Khodadad, C. L. M., Zimmerman, A. R., Green, S. J., Uthandi, S., and Foster, J. S. (2011). Taxa-specific changes in soil microbial community composition induced by pyrogenic carbon amendments. Soil Biol. Biochem. 43, 385–392. doi: 10.1016/j.soilbio.2010.11.005
Kolton, M., Harel, Y. M., Pasternak, Z., Graber, E. R., Elad, Y., and Cytryn, E. (2011). Impact of biochar application to soil on the root-associated bacterial community structure of fully developed greenhouse pepper plants. Appl. Environ. Microbiol. 77, 4924–4930. doi: 10.1128/AEM.00148-11
Laghari, M., Naidu, R., Xiao, B., Hu, Z., Mirjat, M. S., Hu, M., et al. (2016). Recent developments in biochar as an effective tool for agricultural soil management: a review. J. Sci. Food Agric. 96, 4840–4849. doi: 10.1002/jsfa.7753
Langmead, B., and Salzberg, S. L. (2012). Fast gapped-read alignment with Bowtie 2. Nat. Methods 9, 357–359. doi: 10.1038/nmeth.1923
Langmead, B., Trapnell, C., Pop, M., and Salzberg, S. L. (2009). Ultrafast and memory-efficient alignment of short DNA sequences to the human genome. Genome Biol. 10:R25. doi: 10.1186/gb-2009-10-3-r25
Lee, T. K., Lee, J., Sul, W. J., Iwai, S., Chai, B., Tiedje, J. M., et al. (2011). Novel biphenyl-oxidizing bacteria and dioxygenase genes from a Korean tidal mudflat. Appl. Environ. Microbiol. 77, 3888–3891. doi: 10.1128/AEM.00023-11
Lehmann, J., Rillig, M. C., Thies, J., Masiello, C. A., Hockaday, W. C., and Crowley, D. (2011). Biochar effects on soil biota - A review. Soil Biol. Biochem. 43, 1812–1836. doi: 10.1016/j.soilbio.2011.04.022
Lewin, G. R., Carlos, C., Chevrette, M. G., Horn, H. A., McDonald, B. R., Stankey, R. J., et al. (2016). Evolution and ecology of actinobacteria and their bioenergy applications. Annu. Rev. Microbiol. 70, 235–254. doi: 10.1146/annurev-micro-102215-095748
Li, H., Handsaker, B., Wysoker, A., Fennell, T., Ruan, J., Homer, N., et al. (2009). The sequence alignment/map format and SAMtools. Bioinformatics 25, 2078–2079. doi: 10.1093/bioinformatics/btp352
Li, Q., Lei, Z., Song, X., Zhang, Z., Ying, Y., and Peng, C. (2018). Biochar amendment decreases soil microbial biomass and increases bacterial diversity in Moso bamboo (Phyllostachys edulis) plantations under simulated nitrogen deposition. Environ. Res. Lett. 13:044029. doi: 10.1088/1748-9326/aab53a
Liang, B., Lehmann, J., Solomon, D., Kinyangi, J., Grossman, J., O’Neill, B., et al. (2006). Black carbon increases cation exchange capacity in soils. Soil Sci. Soc. Am. J. 70, 1719–1730. doi: 10.2136/sssaj2005.0383
Liu, Y., Zhu, J., Ye, C., Zhu, P., Ba, Q., Pang, J., et al. (2018). Effects of biochar application on the abundance and community composition of denitrifying bacteria in a reclaimed soil from coal mining subsidence area. Sci. Total Environ. 625, 1218–1224. doi: 10.1016/j.scitotenv.2018.01.003
Luo, Y., Durenkamp, M., De Nobili, M., Lin, Q., and Brookes, P. C. (2011). Short term soil priming effects and the mineralisation of biochar following its incorporation to soils of different pH. Soil Biol. Biochem. 43, 2304–2314. doi: 10.1016/j.soilbio.2011.07.020
MacLean, A. M., MacPherson, G., Aneja, P., and Finan, T. M. (2006). Characterization of the β-ketoadipate pathway in Sinorhizobium meliloti. Appl. Environ. Microbiol. 72, 5403–5413. doi: 10.1128/AEM.00580-06
McMurdie, P. J., and Holmes, S. (2013). phyloseq: an R package for reproducible interactive analysis and graphics of microbiome census data. PLoS One 8:e61217. doi: 10.1371/journal.pone.0061217
Meschewski, E., Holm, N., Sharma, B. K., Spokas, K., Minalt, N., and Kelly, J. J. (2019). Pyrolysis biochar has negligible effects on soil greenhouse gas production, microbial communities, plant germination, and initial seedling growth. Chemosphere 228, 565–576. doi: 10.1016/j.chemosphere.2019.04.031
Mukherjee, A., Zimmerman, A. R., and Harris, W. (2011). Surface chemistry variations among a series of laboratory-produced biochars. Geoderma 163, 247–255. doi: 10.1016/j.geoderma.2011.04.021
Nadkarni, M. A., Martin, F. E., Jacques, N. A., and Hunter, N. (2002). Determination of bacterial load by real-time PCR using a broad-range (universal) probe and primers set. Microbiology 148, 257–266. doi: 10.1099/00221287-148-1-257
Nicolitch, O., Feucherolles, M., Churin, J. L., Fauchery, L., Turpault, M. P., and Uroz, S. (2019). A microcosm approach highlights the response of soil mineral weathering bacterial communities to an increase of K and Mg availability. Sci. Rep. 9:14403. doi: 10.1038/s41598-019-50730-y
Noyce, G. L., Basiliko, N., Fulthorpe, R., Sackett, T. E., and Thomas, S. C. (2015). Soil microbial responses over 2 years following biochar addition to a north temperate forest. Biol. Fertil. Soils 51, 649–659. doi: 10.1007/s00374-015-1010-7
Oksanen, J., Blanchet, F. G., Friendly, M., Kindt, R., Legendre, P., Mcglinn, D., et al. (2018). Package “vegan.” Available online at: https://cran.r-project.org/web/packages/vegan/index.html (accessed June 11, 2020).
Orellana, L. H., Rodriguez-R, L. M., Higgins, S., Chee-Sanford, J. C., Sanford, R. A., Ritalahti, K. M., et al. (2014). Detecting nitrous oxide reductase (nosZ) genes in soil metagenomes: method development and implications for the nitrogen cycle. mBio 5:e01193-14. doi: 10.1128/mBio.01193-14
Palmer, K., and Horn, M. A. (2012). Actinobacterial nitrate reducers and proteobacterial denitrifiers are abundant in N2O-metabolizing palsa peat. Appl. Environ. Microbiol. 78, 5584–5596. doi: 10.1128/AEM.00810-12
Park, D., Kim, H., and Yoon, S. (2017). Nitrous oxide reduction by an obligate aerobic bacterium, Gemmatimonas aurantiaca strain T-27. Appl. Environ. Microbiol. 83:e00502-17. doi: 10.1128/AEM.00502-17
Parke, D., and Ornston, L. N. (1986). Enzymes of the β-ketoadipate pathway are inducible in Rhizobium and Agrobacterium spp. and constitutive in Bradyrhizobium spp. J. Bacteriol. 165, 288–292. doi: 10.1128/jb.165.1.288-292.1986
Parks, D. H., Chuvochina, M., Chaumeil, P.-A., Rinke, C., Mussig, A. J., and Hugenholtz, P. (2019). Selection of representative genomes for 24,706 bacterial and archaeal species clusters provide a complete genome-based taxonomy. bioRxiv [Preprint]. doi: 10.1101/771964
Parks, D. H., Chuvochina, M., Waite, D. W., Rinke, C., Skarshewski, A., Chaumeil, P. A., et al. (2018). A standardized bacterial taxonomy based on genome phylogeny substantially revises the tree of life. Nat. Biotechnol. 36, 996–1004. doi: 10.1038/nbt.4229
Parks, D. H., Imelfort, M., Skennerton, C. T., Hugenholtz, P., and Tyson, G. W. (2015). CheckM: assessing the quality of microbial genomes recovered from isolates, single cells, and metagenomes. Genome Res. 25, 1043–1055. doi: 10.1101/gr.186072.114
Pascault, N., Ranjard, L., Kaisermann, A., Bachar, D., Christen, R., Terrat, S., et al. (2013). Stimulation of different functional groups of bacteria by various plant residues as a driver of soil priming effect. Ecosystems 16, 810–822. doi: 10.1007/s10021-013-9650-7
Paustian, K., Lehmann, J., Ogle, S., Reay, D., Robertson, G. P., and Smith, P. (2016). Climate-smart soils. Nature 532, 49–57. doi: 10.1038/nature17174
Philippot, L., Piutti, S., Martin-Laurent, F., Hallet, S., and Germon, J. C. (2002). Molecular analysis of the nitrate-reducing community from unplanted and maize-planted soils. Appl. Environ. Microbiol. 68, 6121–6128. doi: 10.1128/AEM.68.12.6121-6128.2002
Quinlan, A. R., and Hall, I. M. (2010). BEDTools: a flexible suite of utilities for comparing genomic features. Bioinformatics 26, 841–842. doi: 10.1093/bioinformatics/btq033
Rodriguez-R, L. M., Gunturu, S., Tiedje, J. M., Cole, J. R., and Konstantinidis, K. T. (2018). Nonpareil 3: fast estimation of metagenomic coverage and sequence diversity. mSystems 3:e00039-18. doi: 10.1128/mSystems.00039-18
Rodriguez-R, L. M., and Konstantinidis, K. T. (2014). Nonpareil: a redundancy-based approach to assess the level of coverage in metagenomic datasets. Bioinformatics 30, 629–635. doi: 10.1093/bioinformatics/btt584
Rousk, J., Dempster, D. N., and Jones, D. L. (2013). Transient biochar effects on decomposer microbial growth rates: evidence from two agricultural case-studies. Eur. J. Soil Sci. 64, 770–776. doi: 10.1111/ejss.12103
Shade, A., Jones, S. E., Caporaso, J. G., Handelsman, J., Knight, R., Fierer, N., et al. (2014). Conditionally rare taxa disproportionately contribute to temporal changes in microbial diversity. mBio 5:e01371-14. doi: 10.1128/mBio.01371-14
Shi, Y., Chai, L., Tang, C., Yang, Z., Zhang, H., Chen, R., et al. (2013). Characterization and genomic analysis of kraft lignin biodegradation by the beta-proteobacterium Cupriavidus basilensis B-8. Biotechnol. Biofuels 6:1. doi: 10.1186/1754-6834-6-1
Steinbeiss, S., Gleixner, G., and Antonietti, M. (2009). Effect of biochar amendment on soil carbon balance and soil microbial activity. Soil Biol. Biochem. 41, 1301–1310. doi: 10.1016/j.soilbio.2009.03.016
Su, P., Lou, J., Brookes, P. C., Luo, Y., He, Y., and Xu, J. (2017). Taxon-specific responses of soil microbial communities to different soil priming effects induced by addition of plant residues and their biochars. J. Soils Sediments 17, 674–684. doi: 10.1007/s11368-015-1238-8
Suliman, W., Harsh, J. B., Abu-Lail, N. I., Fortuna, A. M., Dallmeyer, I., and Garcia-Perez, M. (2016). Influence of feedstock source and pyrolysis temperature on biochar bulk and surface properties. Biomass Bioenergy 84, 37–48. doi: 10.1016/j.biombioe.2015.11.010
Thies, J. E., Rillig, M. C., and Graber, E. R. (2015). “Biochar effects on the abundance, activity and diversity of the soil biota,” in Biochar for Environmental Management: Science, Technology and Implementation, 2nd Edn, eds J. Lehmann and S. Joseph (London: Routledge), 327–390.
Uyttebroek, M., Breugelmans, P., Janssen, M., Wattiau, P., Joffe, B., Karlson, U., et al. (2006). Distribution of the Mycobacterium community and polycyclic aromatic hydrocarbons (PAHs) among different size fractions of a long-term PAH-contaminated soil. Environ. Microbiol. 8, 836–847. doi: 10.1111/j.1462-2920.2005.00970.x
Verastegui, Y., Cheng, J., Engel, K., Kolczynski, D., Mortimer, S., Lavigne, J., et al. (2014). Multisubstrate isotope labeling and metagenomic analysis of active soil bacterial communities. mBio 5:e01157-14. doi: 10.1128/mBio.01157-14
Wang, J., Pan, X., Liu, Y., Zhang, X., and Xiong, Z. (2012). Effects of biochar amendment in two soils on greenhouse gas emissions and crop production. Plant Soil 360, 287–298. doi: 10.1007/s11104-012-1250-3
Wang, Q., Garrity, G. M., Tiedje, J. M., and Cole, J. R. (2007). Naïve Bayesian classifier for rapid assignment of rRNA sequences into the new bacterial taxonomy. Appl. Environ. Microbiol. 73, 5261–5267. doi: 10.1128/AEM.00062-07
Wickham, H. (2007). Reshaping data with the reshape package. J. Stat. Softw. 21, 1–20. doi: 10.18637/jss.v021.i12
Wickham, H., François, R., Henry, L., and Müller, K. (2019). dplyr: A Grammar of Data Manipulation. R Packag. version 0.8.1.
Wiedemeier, D. B., Abiven, S., Hockaday, W. C., Keiluweit, M., Kleber, M., Masiello, C. A., et al. (2015). Aromaticity and degree of aromatic condensation of char. Org. Geochem. 78, 135–143. doi: 10.1016/j.orggeochem.2014.10.002
Wilke, C. O. (2017). cowplot: Streamlined Plot Theme and Plot Annotations for “ggplot2.” Available online at: https://CRAN.R-project.org/package=cowplot (accessed June 11, 2020).
Xu, H.-J., Wang, X.-H., Li, H., Yao, H.-Y., Su, J.-Q., and Zhu, Y.-G. (2014). Biochar impacts soil microbial community composition and nitrogen cycling in an acidic soil planted with rape. Environ. Sci. Technol. 48, 9391–9399. doi: 10.1021/es5021058
Xu, N., Tan, G., Wang, H., and Gai, X. (2016). Effect of biochar additions to soil on nitrogen leaching, microbial biomass and bacterial community structure. Eur. J. Soil Biol. 74, 1–8. doi: 10.1016/j.ejsobi.2016.02.004
Yang, P., and Dirk van Elsas, J. (2018). Mechanisms and ecological implications of the movement of bacteria in soil. Appl. Soil Ecol. 129, 112–120. doi: 10.1016/j.apsoil.2018.04.014
Ye, J., Joseph, S. D., Ji, M., Nielsen, S., Mitchell, D. R. G., Donne, S., et al. (2017). Chemolithotrophic processes in the bacterial communities on the surface of mineral-enriched biochars. ISME J. 11, 1087–1101. doi: 10.1038/ismej.2016.187
Youngblut, N. D., and Buckley, D. H. (2014). Intra-genomic variation in G + C content and its implications for DNA stable isotope probing. Environ. Microbiol. Rep. 6, 767–775. doi: 10.1111/1758-2229.12201
Yu, J., Deem, L. M., Crow, S. E., Deenik, J., and Ryan Penton, C. (2019). Comparative metagenomics reveals enhanced nutrient cycling potential after 2 years of biochar amendment in a tropical oxisol. Appl. Environ. Microbiol. 85:e02957-18. doi: 10.1128/AEM.02957-18
Yu, J., Deem, L. M., Crow, S. E., Deenik, J. L., and Penton, C. R. (2018). Biochar application influences microbial assemblage complexity and composition due to soil and bioenergy crop type interactions. Soil Biol. Biochem. 117, 97–107. doi: 10.1016/j.soilbio.2017.11.017
Yuan, C., Lei, J., Cole, J., and Sun, Y. (2015). Reconstructing 16S rRNA genes in metagenomic data. Bioinformatics 31, i35–i43. doi: 10.1093/bioinformatics/btv231
Zhang, M., Riaz, M., Zhang, L., El-Desouki, Z., and Jiang, C. (2019). Biochar induces changes to basic soil properties and bacterial communities of different soils to varying degrees at 25 mm rainfall: more effective on acidic soils. Front. Microbiol. 10:1321. doi: 10.3389/fmicb.2019.01321
Zhang, Q. Z., Dijkstra, F. A., Liu, X. R., Wang, Y. D., Huang, J., and Lu, N. (2014). Effects of biochar on soil microbial biomass after four years of consecutive application in the north China Plain. PLoS One 9:e102062. doi: 10.1371/journal.pone.0102062
Zhao, L., Cao, X., Mašek, O., and Zimmerman, A. (2013). Heterogeneity of biochar properties as a function of feedstock sources and production temperatures. J. Hazard. Mater. 256–257, 1–9. doi: 10.1016/j.jhazmat.2013.04.015
Zheng, J., Chen, J., Pan, G., Liu, X., Zhang, X., Li, L., et al. (2016). Biochar decreased microbial metabolic quotient and shifted community composition four years after a single incorporation in a slightly acid rice paddy from southwest China. Sci. Total Environ. 571, 206–217. doi: 10.1016/j.scitotenv.2016.07.135
Keywords: biochar amendment, isopycnic centrifugation, carbon sequestration, denitrification, Nextseq sequencing, metagenomic assembled genomes, active bacterial populations
Citation: Yu J, Pavia MJ, Deem LM, Crow SE, Deenik JL and Penton CR (2020) DNA-Stable Isotope Probing Shotgun Metagenomics Reveals the Resilience of Active Microbial Communities to Biochar Amendment in Oxisol Soil. Front. Microbiol. 11:587972. doi: 10.3389/fmicb.2020.587972
Received: 27 July 2020; Accepted: 08 October 2020;
Published: 17 November 2020.
Edited by:
Jeanette M. Norton, Utah State University, United StatesReviewed by:
Stephen David Joseph, University of New South Wales, AustraliaXiaoyu Liu, Nanjing Agricultural University, China
Copyright © 2020 Yu, Pavia, Deem, Crow, Deenik and Penton. This is an open-access article distributed under the terms of the Creative Commons Attribution License (CC BY). The use, distribution or reproduction in other forums is permitted, provided the original author(s) and the copyright owner(s) are credited and that the original publication in this journal is cited, in accordance with accepted academic practice. No use, distribution or reproduction is permitted which does not comply with these terms.
*Correspondence: Julian Yu, julianyu@asu.edu