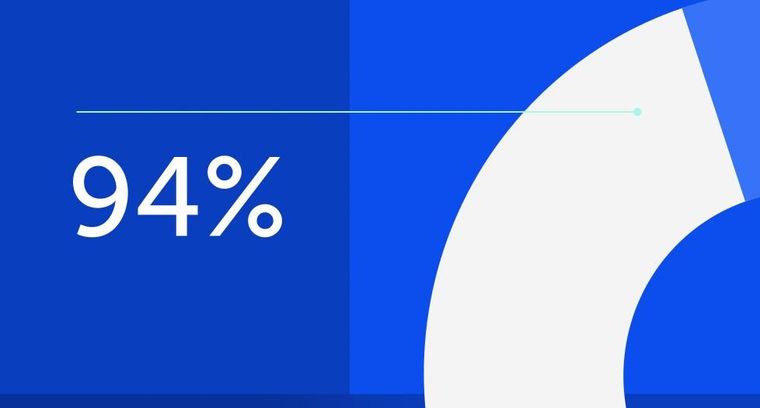
94% of researchers rate our articles as excellent or good
Learn more about the work of our research integrity team to safeguard the quality of each article we publish.
Find out more
ORIGINAL RESEARCH article
Front. Microbiol., 26 October 2020
Sec. Microbial Symbioses
Volume 11 - 2020 | https://doi.org/10.3389/fmicb.2020.565549
Although it is well-known that human skin aging is accompanied by an alteration in the skin microbiota, we know little about how the composition of these changes during the course of aging and the effects of age-related skin microbes on aging. Using 16S ribosomal DNA and internal transcribed spacer ribosomal DNA sequencing to profile the microbiomes of 160 skin samples from two anatomical sites, the cheek and the abdomen, on 80 individuals of varying ages, we developed age-related microbiota profiles for both intrinsic skin aging and photoaging to provide an improved understanding of the age-dependent variation in skin microbial composition. According to the landscape, the microbial composition in the Children group was significantly different from that in the other age groups. Further correlation analysis with clinical parameters and functional prediction in each group revealed that high enrichment of nine microbial communities (i.e., Cyanobacteria, Staphylococcus, Cutibacterium, Lactobacillus, Corynebacterium, Streptococcus, Neisseria, Candida, and Malassezia) and 18 pathways (such as biosynthesis of antibiotics) potentially affected skin aging, implying that skin microbiomes may perform key functions in skin aging by regulating the immune response, resistance to ultraviolet light, and biosynthesis and metabolism of age-related substances. Our work re-establishes that skin microbiomes play an important regulatory role in the aging process and opens a new approach for targeted microbial therapy for skin aging.
Skin is a complex barrier with a variety of biological functions. It provides an important surface for interactions with the external environment and protects the body from pathogenic, chemical, and physical assaults (Proksch et al., 2008). Skin microbiota (bacteria, fungi, and viruses) are indispensable parts of the skin barrier; they regulate inflammatory processes and provide innate and adaptive immunity (Zhai et al., 2018). Microecologically related research is a new direction in the study of skin aging. A range of cutaneous pathological states occurs when the compositional balance of the skin microbiome is broken due to factors such as aging (Kong et al., 2012; Schommer and Gallo, 2013; Prescott et al., 2017; Tett et al., 2017; Rocha and Bagatin, 2018). In addition, the composition of skin microbiomes varies depending on internal and external factors such as skin integrity and physiological status (Dreno et al., 2016), antibacterial therapy (Langdon et al., 2016), and demographic characteristics (Leung et al., 2016b). Skin aging is a process of alterations in the cutaneous structure and physiological changes (Lei et al., 2017; Bonté et al., 2019), accompanied by increases in species richness and changes in the dominant bacteria, according to some studies (Shibagaki et al., 2017). Therefore, we need to prepare a comprehensive picture of the variation in human skin microbiota associated with skin aging to reveal the patterns of interaction between the microbiota and the aging process.
Many studies (Shibagaki et al., 2017; Wilantho et al., 2017; Juge et al., 2018) have confirmed that the skin bacterial microbiomes differ between young women and older women; however, there are limited studies on the variation in the patterns of skin fungal microbiomes with aging. Additionally, existing studies have focused mainly on site-specific anatomic variation in the microbiomes, instead of differences in the aging process on the basis of exposure factors, i.e., intrinsic aging and photoaging. Intrinsic aging is basically the natural aging process of the skin and is determined by internal factors. Photoaging is accelerated aging of the skin caused by excessive exposure to the sun. In photoaging, the skin microbiome is characterized by ultraviolet (UV)-generated reactive oxygen species (ROS), which regulate gene expression related to collagen degradation and elastin accumulation. These ROS affect DNA or decrease protein tyrosine phosphatases, resulting in the upregulation of matrix metalloproteinase production, contributing to photooxidative skin aging and skin cancer (Wlaschek et al., 2001; Gonzaga, 2009). In short, intrinsic skin aging and photoaging are different processes. There is little evidence that microbiomes influence the aging process of the skin, and little is known about the specific variation patterns of microbiota and their functions in the two different aging processes. In an attempt to remedy this lack, we used 16S ribosomal DNA (rDNA) and internal transcribed spacer (ITS) rDNA sequencing to create profiles for exposed and non-exposed anatomical sites, respectively. We measured differences in the characteristics of microbial composition brought about by aging among four different age groups. Combined with clinical parameters, we focused on the functions of specific age-related microbial communities in each aging stage; these functions may be considered key regulators of skin aging. Combinations of microbial communities and age-related pathways in different age groups could affect UV-induced damage, immune barrier integrity, and the biosynthesis and metabolism of substances that alter the aging process. Our findings could be a milestone in targeted microbial anti-aging skin therapy, which would be a benefit to health care for aging-relevant cutaneous idiopathic diseases and wounds in different age groups.
Skin samples were collected from 80 healthy participants belonging to four age groups in Xi’an City (Shaanxi Province, China). Each group was composed of 20 individuals. The make-up of the individual groups, including age range and source of skin samples, was as follows: (1) 20 children, 3–7 years (cheek [CCHG], abdomen [ACHG]); (2) 20, youths, 19–23 years (cheek [CYHG], abdomen [AYHG]); (3) 20 middle-aged individuals, 37–42 years (cheek [CMAG], abdomen [AMAG]); and (4) 20 elderly individuals, 65–74 years (cheek [CELG], abdomen [AELG]). Each group was composed of 10 males and 10 females. The study was compliant with the Declaration of Helsinki Principles, and we obtained informed consent from participants or, in the case of the children, from their parents or other relatives, about the significance of the research and the process of implementation. All the participants were notified that the material they provided could identify their health status as that of having no current cutaneous disorders. Persons were excluded if they were receiving antimicrobial therapy or cleaning within 1 month. Participants were asked to avoid cleaning sampling sites or using cosmetics for at least 48 h before sampling. A strict procedure of superficial skin sampling was conducted for both cheeks (2 cm × 5 cm) and abdomen (5 cm × 8 cm) in a temperature- (22 ± 2°C) and humidity-controlled (40–60%) room. Samples were collected with sterile cotton-tipped swabs soaked in 0.15 mol/L sodium chloride and 0.1% Tween 20. Each cotton tip was cut, placed in a 2.0-ml sterile centrifuge tube, and frozen in liquid nitrogen immediately for 5 min, then stored at −80°C until DNA extraction.
The VISIA Complexion Analysis System (Canfield, Fairfield, NJ, United States) was used to take photographic images of participants to analyze the physiological characteristics of skin aging, quantitatively. Participants were asked to wash their face with clean water before this examination. Images were taken from the front and 45° to both lateral under the same conditions to ensure the sampling sites (both cheeks) were covered completely. The photographic images were of 10-megapixel resolution and were captured with standard, cross-polarized, parallel polarized, and UV light. Spots, wrinkles, texture, pores, UV spots, brown spots, red areas, and porphyrins indexes were all objectively evaluated by the system. Absolute scores were used to assess the actual condition of each evaluation index.
Total genomic DNA was extracted from the clipped swabs with the PowerSoil DNA isolation kit (MoBio Laboratories, Carlsbad, CA, United States) according to the manufacturer’s instructions. DNA concentration was measured with a NanoDrop spectrophotometer (Thermo Fisher Scientific). Hypervariable regions V3–V4 of the bacterial 16S rDNA were amplified with the primers 338F (ACTCCTACGGGAGGCAGCAG) and 806R (GGACTACHVGGGTWTCTAAT) (Munyaka et al., 2015). The fungal ITS region was amplified with the primers ITS1F (5-GGAAGTAAAAGTCGTAACAAGG-3) and ITS2 (5-TCCTCCGCTTATTGATATGC-3) (Zhang et al., 2015). Amplification was performed as described by Cao et al. (2018). The PCR products were purified and normalized using Agencourt AMPure XP magnetic beads (Beckman Coulter) according to the manufacturer’s protocol. Purified amplifications were quantitated with real-time PCR and pooled for Illumina sequencing. Deep sequencing was performed 2 × 300-bp paired-end on an Illumina MiSeq platform using MiSeq Reagent Kit v3 (600 cycles) (Illumina) according to the standard protocol.
Total DNA was isolated with AxyPrep Multisource Genomic DNA Kit (Axygen, Union City, CA, United States) according to the manufacturer’s instructions, and DNA purity was checked through the NanoPhotometer spectrophotometer (IMPLEN, Westlake Village, CA, United States). Microbial genera of Staphylococcus, Cutibacterium, Lactobacillus, and Corynebacterium were quantified by real-time quantitative PCR (qPCR) in different groups. The genus-specific primer pairs used for qPCR are shown in Supplementary Table 5 (Martineau et al., 2001; Rinttilä et al., 2004). The detailed protocol of the qPCR is shown in the Supplementary Protocol 1. Data were analyzed with ABI 7500 Real-Time PCR software version 2.0.5, and we used the second derivate maximum method, which calculated PCR efficiency in accordance with Pfaffl (2001). Sample DNA concentration was calculated by comparing the sample Cp value to the standard curve. Each group was tested in triplicate.
We assigned all reads to each specific sample on the basis of the barcode sequence. Reads were removed if they were < 200 bp, with an average low-quality score (≤20), containing ambiguous bases or not exactly matched to the universal primer. For chimera checking and taxonomy assignment of similar high-quality sequences, qualified reads were de novo clustered into operational taxonomic units (OTUs) at a similarity level of 97% (Edgar, 2013), and chimeric OTUs were identified by the UCHIME algorithm (v1.1.3) and removed. The Ribosomal Database Project Classifier tool was used to classify all qualified sequences into different taxonomic groups (Cole et al., 2009).
The taxonomy of each 16S rDNA and ITS rDNA sequence was analyzed by UCLUST with the corresponding Silva 16S rRNA database and Unite database, respectively. Chao1-estimated OTU number index was also used to assess species diversity. Beta diversity was evaluated by calculating UniFrac distance (weighted) and constructing from the OTU table principal component analysis between the samples in different groups. Spearman analysis was used to analyze the correlation between clinical data and dominant microbiomes, and the P-value was calculated with Mothur software, an OTU association program. The bacterial metabolic potentials were predicted by Tax4FUN software combined with the Kyoto Encyclopedia of Genes and Genomes (KEGG) database. FUNGuid software was used to predict fungal functions. All raw sequencing reads analyzed were deposited in the National Center for Biotechnology Information (NCBI) with the accession numbers PRJNA630834 and PRJNA630117.
According to different data types, various statistical strategies were used to determine statistical significance. Linear discriminant analysis effect size (LEfSe) analysis with a threshold of 3.0 was used to determine the significant microbial taxa among the four age groups. Wilcoxon’s rank-sum test analysis was used to evaluate the statistical differences in the relative abundance of microbial communities, species diversity, and pathway enrichment between the two groups. A Kruskal–Wallis rank-sum test was constructed to estimate the differences in microbial composition and species richness for multiple comparisons. In addition, we also used an analysis of similarities (ANOSIM) based on weighted UniFrac to calculate the differences in community structure. Spearman correlations were used to calculate the relationships between every two microbial genera. All these statistical analyses were performed using R software (v 3.5.0) and mothur software (v1.33.3). P-value < 0.05 was considered statistically significant.
We used the VISIA Complexion Analysis System to evaluate the following eight indicators for facial skin from 80 healthy participants in four age groups: spots, wrinkles, texture, pores, UV spots, brown spots, red areas, and porphyrins (see Table 1 and Supplementary Table 6). The absolute scores (ASs) of spots, UV spots, brown spots, red areas, wrinkles, and texture increased with increased age. However, the ASs of pores and porphyrins increased initially and then decreased during aging, and we detected peaks in the middle-aged and youth groups. These eight clinical parameters accurately reflect the changes of skin physiological state in aging in many respects, and they present the regular variation in patterns during aging. Thus, we used these parameters to quantitatively describe the different facial skin aging status and to perform an association analysis of microbiomes.
We extracted DNA from 160 samples of exposed sites (cheek) and non-exposed sites (abdomen) and compiled their rDNA sequences and OTUs (Table 2). We obtained 10,036,756 and 14,864,480 reads from 16S rDNA and ITS rDNA sequencing, respectively. Sequence assembly, chimera removal, and quality filtering resulted in 9,315,093 high-quality 16S rDNA sequences (average 58,219 reads/sample, from 26,245 reads/sample to 154,394 reads/sample) and 14,951,548 high-quality ITS rDNA sequences (average 92,903 reads/sample, from 26,370 reads/sample to 262,051 reads/sample). We clustered these data into 6,813 bacterial and 8,080 fungal OTUs with a ≥ 97% identity threshold. Forty-three phyla and 1,087 genera were annotated with taxonomic information based on bacterial OTUs, and 19 phyla and 975 genera were annotated based on fungal OTUs.
Table 2. Descriptive information and statistics for each group, i.e., children group (cheek [CCHG], abdomen [ACHG]), youth group (cheek [CYHG], abdomen [AYHG]), middle-aged group (cheek [CMAG], abdomen [AMAG]), and elder group (cheek [CELG], abdomen [AELG]).
We detected different composition characteristics of skin microbiomes for the intrinsic aging process for unexposed sites in different aging stages. We found large differences in bacterial and fungal species diversity with Chao1 indices, particularly during the skin intrinsic aging process (Supplementary Table 7). Bacterial species richness increased gradually with advancing age, whereas the fungal communities in the middle-aged group showed the highest species diversity (Figures 1A,B). We further recorded the core species at the phylum and genus levels. Four major bacterial phyla and two fungal core phyla: Proteobacteria, Firmicutes, Actinobacteria, Bacteroidetes, Ascomycota, and Basidiomycota were dominant in the four age groups, accounting for > 78% of the total skin microbiomes. In addition, among the top five dominant bacterial species, we detected higher abundances of Cyanobacteria in the children group (4.67%) and Fusobacteria in the elder group (1.24%) compared with the other age groups. At the genus level, nine dominant bacterial genera (Streptococcus, Porphyromonas, Acinetobacter, Enhydrobacter, Comamonas, Staphylococcus, Cutibacterium, Corynebacterium, and Chryseobacterium) and eight dominant fungal genera (Candida, Malassezia, Penicillium, Wallemia, Aspergillus, and Cladosporium) were the top five species in at least one group, with average abundances of ≥ 1% (Supplementary Table 1 and Figures 1C,D).
Figure 1. Variation in patterns of skin microbiome during the intrinsic aging process. Bacterial (A) and fungal (B) species richness at each age group. Relative abundances of bacterial (C) and fungal (D) core species at the genus level in each group. Weighted UniFrac-PCoA plots of skin bacteria (E) and fungi (F) in each group. Bacterial (G) and fungal (H) phylogenetic distributions during intrinsic skin aging (LDA score > 3, P-value < 0.05). Based on linear discriminant analysis effect size (LEfSe), the phylogenetic distributions of distinct taxa are colored corresponding to the different classification (phyla to species were depicted by circles from the inside to outside). Circlize analysis shows the relative abundances of nine unique age-related functional taxa in each age group (I).
We used weighted UniFrac distance analysis to measure the overall structural similarity and differences in microbiomes associated with skin aging. Significant separations among clusters existed between different age groups with ANOSIM (P < 0.05) (Supplementary Table 2). Based on weighted UniFrac-principal coordinates analysis (PCoA), the microbial composition in the children group was obviously different from that in the other age groups. However, the structures of the microbial communities in the other three groups were similar (Figures 1E,F).
We next used LEfSe analysis to compare the differences in taxonomic profiles between the four age groups. At the phylum level, Proteobacteria (48.27%), Cyanobacteria (4.11%), and Fusobacteria (1.80%) were significantly enriched in the children group. In addition, the abundance of Actinobacteria (28.18%) was greater in the youth group compared with the other three age groups. Comparative analysis of genera on skin demonstrated that most age-related species predominated in the children group for both bacterial communities, such as Acinetobacter (14.33%), Streptococcus (8.50%), and Neisseria (2.41%), and fungal communities, such as Trichosporon (1.12%), Candida (6.71%), and Meyerozyma (2.18%). We also found significant enrichment of Staphylococcus in the youth (10.25%) and middle-aged (8.88%) groups and Corynebacterium in the youth (13.84%) group. In addition, Malassezia was uniquely more abundant in the youth (17.72%), middle-aged (14.99%), and elder groups (12.37%) (Supplementary Table 3 and Figures 1G,H).
We also performed qPCR for our focused Staphylococcus and Corynebacterium, closely related to the integrity of the skin immune barrier during skin intrinsic aging. The quantitative results were highly consistent with the results of the 16S rDNA sequencing. The details are shown in Supplementary Figure 2. We believe that the results obtained from the amplicons could well-reflect the real situation of the microbial variation patterns in skin aging processes, and the results are authentic and reliable.
Due to exogenous factors such as UV radiation, facial skin experiences excessive photoaging. Interestingly, we found that the cheek microbiomes during aging presented unique dynamic variation patterns different from intrinsic aging. The dynamic changes in species richness and core dominant phyla during photoaging were similar to the changes during intrinsic aging. With advancing age, bacterial alpha diversity increased monotonically, whereas the highest species diversity of fungal communities was detected in the middle-aged group (Figures 2A,B). Six major phyla were found by 16S rDNA sequencing, and ITS rDNA sequencing: Proteobacteria, Firmicutes, Actinobacteria, Bacteroidetes, Ascomycota, and Basidiomycota was dominant in all age groups. Cyanobacteria and Fusobacteriaon exposed skin were also dominant in the children group and elder group, respectively. For the genera on exposed skin, ten dominant bacterial genera (Streptococcus, Porphyromonas, Alloprevotella, Enhydrobacter, Haemophilus, Staphylococcus, Cutibacterium, Neisseria, Corynebacterium, and Chryseobacterium) and nine dominant fungal genera (Candida, Malassezia, Penicillium, Acremonium, Exophiala, Meyerozyma, Trichosporon, Aspergillus, and Cladosporium) were the top five dominant genuses in at least one group (Supplementary Table 1 and Figures 2C,D).
Figure 2. Variation in patterns of skin microbiome during photoaging. Bacterial (A) and fungal (B) species richness at each age group during photoaging. Relative abundances of bacterial (C) and fungal (D) core species at the genus level in each group. Weighted UniFrac-PCoA plots of skin bacteria (E) and fungi (F) in each group. Based on LEfSe, bacterial (G) and fungal (H) phylogenetic distributions during photoaging (LDA score > 3, P-value < 0.05). Circlize analysis shows the relative abundances of nine unique age-related functional taxa in each age group (I).
When analyzing the beta diversities of the facial skin microbiomes during photoaging, we found that, apart from the similar bacterial composition between the youth and middle-aged groups, significant separations among clusters with ANOSIM (P < 0.05) existed between different age groups (Supplementary Table 2). Based on weighted UniFrac-PCoA for both bacterial and fungal profiles, a great difference in microbial composition between children and the other age groups was also present during photoaging. Additionally, a significant difference existed between the youth and elder groups, whereas a similar microbial composition was identified between the middle-aged and both youth and elder groups (Figures 2E,F).
Differential analysis of taxa by LEfSe of the four age groups revealed that, at the phylum level, there was higher enrichment for Cyanobacteria in the children group (4.11%), Actinobacteria (26.47%) in the youth group, and Bacteroidetes (14.56%) in the elder group. Bacterial genus-level assignment similarly showed that more age-related species were dominant in the children group, including Neisseria (8.91%), Streptococcus (24.35%), and Haemophilus (5.15%) and fungal communities such as Candida (8.90%) and Trichosporon (2.36%). In contrast, Cutibacterium (15.13%; 12.12%) and Staphylococcus (19.22%; 19.48%) were enriched in both the youth and the middle-aged groups. Compared with the other three age groups, there was a higher proportion of Lactobacillus (1.15%) on exposed sites in the middle-aged group. Streptococcus, Leptotrichia, Alkanindiges, and Enhydrobacter were more likely to reside on cheeks in the elder group. Malassezia, the most abundant skin fungus, had a significantly higher proportion in the middle-aged (30.60%) and the elder (27.46%) groups (Supplementary Table 4 and Figures 2G,H). Similarly, quantitative analysis was performed with qPCR for skin immune barrier-related genus during photoaging processes, including Staphylococcus, Cutibacterium, and Lactobacillus, which were highly consistent with our results of 16S rDNA sequencing (Supplementary Figure 2).
On the basis of differential analysis of taxa of the four age groups, we performed Spearman correlation analysis to describe the relationships between dominant facial microbial communities and age-related clinical parameters (based on the VISIA Complexion Analysis System). We found that the enrichment of Neisseria and Candida in the children and elder groups correlated positively with the ASs of brown spots and red areas. In the elder group, the higher abundance of Streptococcus showed a positive correlation with exacerbation of the progress of emerging (brown) spots, wrinkles, texture, and porphyrins, whereas we observed a nearly opposite trend for the children group. Because enrichment of Lactobacillus was present in the middle-aged group, except for UV spots, all remaining indicators declined to varying degrees. Similarly, the normal number of Cutibacterium was also considered a protective factor according to the skin parameters. Only the ASs of porphyrins and pores associated with sebum secretion increased with a higher abundance of Cutibacterium in the young and middle-aged groups. Most indices indicated a slowing of skin aging. In addition, we found remarkable downward trends in the ASs of UV spots and red areas due to the enrichment of Staphylococcus in the young and middle-aged groups. Malassezia was the most dominant fungi on human skin, especially for the middle-aged and elder groups. Most of the parameters suggested that skin was more likely to be in a senescent state when abundant Malassezia was present. At the phylum level, Cyanobacteria was a newly detected predominant phylum in the children group related to preventing UV-induced damage and pigmentation, including spots, UV spots, and brown spots (Figure 3 and Supplementary Figure 1). We ultimately identified nine microbial communities most closely associated with age-related clinical parameters and demonstrated their relative abundance in each age group in Figures 1I, 2I.
Figure 3. Correlation between clinical parameters and dominant bacterial communities. Heatmap of the Spearman correlation between clinical data and dominant bacterial communities in four age groups during photoaging (PA). **Represents the p value <0.01 for the Spearman correlation analysis.
Apparently, during intrinsic aging, different modes of action of skin microbiomes occur in different stages. During the transition from children to youth, we found higher enrichments in base excision repair, biosynthesis of amino acids, and biosynthesis of antibiotics and lower abundances of arginine and proline metabolism in the youth group. With the progression of aging, pantothenate and CoA biosynthesis and mismatch repair pathways gradually lost their dominance in the middle-aged group, and lower enrichment in D-arginine and D-ornithine metabolism and oxidative phosphorylation in the elder group may contribute to the intrinsic aging process. Remarkably, the abundance of vitamin B6 metabolism continually declined from youth to elder (Figure 4).
Figure 4. Functional prediction of skin microbiomes in skin aging. Comparison of potential metabolic functions in the Kyoto Encyclopedia of Genes and Genomes pathways between each two age groups during intrinsic skin aging (IA) and photoaging (PA).
During cheek photoaging, microbial alterations affect the aging process from children to youth, mainly by downregulating the MAPK signaling pathway, glutathione metabolism, photosynthesis, and pantothenate and CoA biosynthesis. In the subsequent aging stage, microbiomes in the middle-aged group decrease their metabolic capacities, such as glycerophospholipid metabolism, vitamin B6 metabolism, and metabolic pathways. We found that pathways associated with biosynthesis and lipid metabolism, i.e., biosynthesis of antibiotics, fatty acid biosynthesis, glycerol lipid metabolism, and porphyrin and chlorophyll metabolism gradually lost dominance in the elder group (Figure 4).
Physiological skin changes are thought to mirror aging. Because skin aging is prone to be affected by environmental factors, especially UV radiation, previous studies distinguished between intrinsic aging and photoaging (Bonté et al., 2019; Wang et al., 2019). Not only is skin aging associated with a decline in appearance and the occurrence of skin disorders, but also the bacterial composition of facial skin among women was strongly affected by aging (Makrantonaki et al., 2012; Blume-Peytavi et al., 2016; Shibagaki et al., 2017; Kim et al., 2019). In our research, for the first time, we comprehensively revealed the various age-dependent patterns in the composition of skin prokaryotic and eukaryotic communities. We also suggest that our findings represent a new stage in the understanding of the skin pan-microbiomes and characteristics of skin aging.
In aging, we detected different species diversities and compositional characteristics of skin microbiomes in different age groups. These differences could be ascribed to continuous self-regulation and self-renewal of skin microbiomes, related to individual’s residential environment and host selection pressures (e.g., local physiological status) (Kim et al., 2018; Dimitriu et al., 2019). Consistent with previous studies, higher species diversities of cutaneous bacterial communities were found with aging for both exposed and non-exposed sites (Leung et al., 2015, 2016a; Shibagaki et al., 2017). However, we found that, for both sampling sites, the fungal communities showed the highest species diversity in the middle-aged group (Figures 1A,B, 2A,B). In addition, we noted that the most conspicuous structural differences were found between the children and the other age groups (Figures 1E, 2E). With the onset of puberty, transition, and sexual maturation, the skin microbiomes would undergo a large shift, with the disappearance of several taxa. Because sex hormones stimulate remodeling of the local sebaceous environment, microorganisms become dominated by lipophilic communities such as Cutibacterium and Malassezia in the middle-aged and elder groups (Leyden et al., 1975; Oh et al., 2012; Belkaid and Tamoutounour, 2016).
We further identified several potentially functional skin age-related microbiotas combined with quantitative analysis. By exploring the specific distribution of these dominant communities and the proved functions associated with clinical characteristics, we hypothesized that microbial communities not only coexist with the skin barrier but might actually modify the process of skin aging, as proposed by current theories (Roth and James, 1988; Sanford and Gallo, 2013). Because diverse microbial compositions exist in different sites and different age groups, the potential effects of microorganisms on the skin are age- and site-specific (Figure 5). After identifying the nine microbial communities closely related to skin aging parameters, the potential relationships between these genera (including bacteria and fungi) were also expounded in the aging process (Supplementary Figure 3). Furthermore, our work also mainly focused on the effect of aging on the changes in abundance of certain genera and compositional contribution of the entire microbiomes, rather than the effects of aging on the changes of overall abundance or absolute quantity of microbiomes.
Figure 5. Overview of microbial action mode in skin aging. The “shield” before the microbial communities represents the protective factors, whereas the “sword” could be treated as unfavorable factors during skin aging. “+” followed by aging indicating potential functional pathways means that the abundance of a pathway increased with aging, whereas “–” indicates an opposite trend for the variation.
We observed a higher abundance of Cyanobacteria in the children group. As vital components of the photoprotective barrier, Cyanobacteria have developed protective mechanisms against ultraviolet radiation on the skin (Fuentes-Tristan et al., 2019). In correlation analysis with clinical data, enrichment of Cyanobacteria was greatly related to decreased UV-induced damage and pigmentation. In addition, significant enrichment of photosynthesis-related pathways indicated that Cyanobacteria were more active and had a more important function in the children group. On the basis of this evidence, we speculate that children should have a more complete anti-UV barrier.
A previous study showed that the skin aging process could be regulated and even reversed by restoring the normal barrier functions (An et al., 2017), and, during photoaging, the immune–microbial alliance is considered a bridge between innate and adaptive immunity to preserve barrier integrity by microbiota. Association analysis with clinical parameters revealed that the specific enrichment of Staphylococcus, Cutibacterium, and Lactobacillus benefited some detected indicators (e.g., UV spots, red areas), and we believed that these microbiotas were likely to improve the skin barrier integrity. In the youth or middle-aged groups, higher enrichment of Staphylococcus could produce several antimicrobial proteins and proteases that protect the skin from pathogenic invasion and maintain skin microbiota homeostasis (Gallo and Hooper, 2012; Buffie and Pamer, 2013; Belkaid and Tamoutounour, 2016). Meanwhile, Cutibacterium could mediate the necessary immune response and suppress inflammation to further slow the aging process by modulating conjugated linoleic acid generation and control the expression of antimicrobial proteins by Staphylococcus (Liavonchanka et al., 2006). In the middle-aged group, the higher abundance of Lactobacillus may develop protective effects from aging for the following reasons. Lactobacillus can produce various antimicrobial substances and induce anti-inflammatory Treg cells to reduce inflammatory injury caused by UV radiation (Oh et al., 2006), potentially improving conditions for the delay in collagen synthesis and wrinkle formation (Landen et al., 2016), consistent with our skin parameters. The mentioned microbial functions are the specific and inherent characteristics of these three microbiotas, which could well-explain the results we obtained. Additionally, Lactobacillus could also act synergistically with Staphylococcus, which likely leads to enrichment in the biosynthesis of antibiotics, specifically in the middle-aged group, consistent with the result of the predicted functional pathway. Therefore, in the youth and middle-aged groups, we speculate that Staphylococcus, Cutibacterium, and Lactobacillus could promote the formation of mature and complete immune barriers or host defense, to further protect during photoaging.
On the basis of correlation analysis with the parameters, we regarded Streptococcus as a decelerating factor for skin aging in the children and youth groups, whereas an opposite behavior was observed in the other two groups. This interesting finding is potentially attributable to the alteration with the aging of the dominant species Streptococcus, from probiotic communities to the conditional pathogenic genera. Because the skin barriers of the children and elder groups exist in an immature and recessive status, respectively, we detected a higher abundance of some conditional pathogenic genera, such as Neisseria, Streptococcus (elder group), and Candida (Petry et al., 2012; Beatrous et al., 2017; Metin et al., 2018). According to the clinical parameters, these species could be regarded as accelerators (negative correlations) of aging. Due to long-term inflammatory stimulation, even association with various dermatological disorders, high enrichment of Malassezia could be considered an unfavorable factor, particularly in the middle-aged and elder groups according to clinical parameters. The analysis of the correlations between selected genera in the process of photoaging confirmed our inference, to some extent (Supplementary Figure 3).
We further depicted specific functional pathways exerted by the entire microbial community at different aging stages (aging-related landscape) with functional prediction analysis. The photoaging process is characterized mainly by variation of metabolic capacity. Due to higher hormone secretion, greater enrichment for pathways related to metabolism was observed in the youth compared with the middle-aged group, which indicated less protective effects of antioxidants (metabolism of cofactors and vitamins) (Martínez-Navarro et al., 2020) and decreased ability to provide energy, membrane integrity, and cell signaling (glycerophospholipid metabolism) (Cruickshank-Quinn et al., 2017) in the middle-aged group. As aging progresses, in the transition between the middle-aged and the elder groups, further reduction in lipid metabolism capacity (glycerolipid metabolism, fatty acid biosynthesis) (De Luca and Valacchi, 2010; Cruickshank-Quinn et al., 2017) and resistance to pathogens (biosynthesis of antibiotics) jointly promote photoaging. Interestingly, the bacterial communities have two nearly opposite effects on the skin at the children age. Decreasing antioxidant defense substances (glutathione metabolism) (Wolfle et al., 2014), epidermal homeostasis (pantothenate and CoA biosynthesis) (Kuehne et al., 2017), and UV-protection (photosynthesis) are considered unfavorable factors during skin aging. Conversely, less enrichment in the MAPK signaling pathway in the youth group could slow down the photoaging speed, with less degradation of the extracellular matrix (Shin et al., 2019). These predicted functions would help us to better understand the function of the whole skin microbiomes in the photoaging process. Although they cannot be the same as the actual situation, combined with the previous analysis, we can still obtain meaningful inferences.
Intrinsic skin aging is associated with the variation of immune homeostasis and defective barrier function (Zeng et al., 2019), so the immune–microbial alliance is also a vital factor that regulates the intrinsic aging process. We found significant enrichment of Staphylococcus in the youth and middle-aged groups, which would potentially maintain the integrity and healthy status of the skin immune barrier to further affect intrinsic aging. We also speculate that Corynebacterium is another age-related anti-aging factor, particularly Corynebacterium jeikeium (a resident bacteria on the skin), which usually produces several bacteriocin-like compounds against pathogens. Furthermore, the ability of manganese acquisition reduces oxidative damage to the epidermal tissue of non-exposed sites (Storz and Imlay, 1999; Cogen et al., 2008). In the youth group, according to the microbial inherent characteristics, Corynebacterium would act synergistically with Staphylococcus, where it would contribute to the enrichment of the biosynthesis of antibiotics. Because of the instability of the skin barrier in the children and elder groups, a higher proportion of pathogenic genera such as Neisseria, Streptococcus (elder group), and Candida are also regarded as unfavorable factors during intrinsic aging. Besides, we especially detected higher enrichment of Malassezia in the youth, middle-aged, and elder groups, which could be related to the long-term inflammatory stimulation to accelerate aging. The selected intrinsic skin aging-related six bacteria and fungi had significant correlations and relative variation patterns, as demonstrated in Supplementary Figure 3, which also could support the potential interactions between different microbial communities (including bacteria and fungi). We also found that, by the enrichment of specific signaling pathways, bacterial communities have different activities in the intrinsic process of skin aging.
The variations of predicted functions were also discussed in intrinsic aging progress. Sustained damage of DNA repair (mismatch repair, base excision repair) and antioxidant capacity (metabolism of cofactors and vitamins) during the youth to elder stages would accelerate intrinsic aging. In addition, compared with the middle-aged group, epidermal tissue is in a more healthy and stable state in the youth group (pantothenate and CoA biosynthesis) (Kuehne et al., 2017). In the elder group, a reduced energy supply to the host was suggested by the alteration of abundance in oxidative phosphorylation. The growth of children into adults is accompanied by increased amino acids synthesis (biosynthesis of amino acids) and resistance to pathogens (biosynthesis of antibiotics) and by decreased collagen synthesis (arginine and proline metabolism) (Kang et al., 2015) that is associated with facial wrinkles and texture. Therefore, significant differences in the variation of predicted functions for the skin microbiomes were present in the two aging processes. According to the functional prediction analysis, we found that photoaging was accompanied mainly by decreasing the biosynthesis and metabolism of age-related functional substances, whereas intrinsic skin aging was characterized by variation in the biological process.
Our study was limited mainly to function prediction analysis. In this section, we performed the function prediction analysis with Tax4fun software based on the database, which cannot be completely consistent with the actual function of the whole skin microbiomes. In addition, we just constructed the relationships between predicted results and potentially functionally dominant genera, whereas their real association cannot be determined. Therefore, additional metagenomic studies are needed to confirm our findings. In addition, we selected the cheek and abdomen as representative sites for study, although the microbial compositions in other body parts may be different. Based on fungal functional annotation and current microbiome research, skin fungal communities are recognized mainly as pathogens. More confirmed functional pathways related to microbiome during aging need experimental verification. Although we controlled the sampling process and area strictly, we still might obtain the different total population size for skin microbiomes of each sample. Therefore, the biological repetitions of multiple samples were conducted in each group. The results of qPCR were used to support our current results of relative abundance, to further ensure the reliability of our findings.
Our study was the first to systematically describe an entire landscape of skin age-related bacterial and fungal profiles (from children to elderly). Due to the changes in individual residential states of skin microbiomes during aging, specific age-related microbial compositions were presented in different age groups. Similar variation patterns of species diversity and compositional structures based on weighted UniFrac-PCoA were detected between intrinsic skin aging and photoaging. Conversely, we identified aging specific dominant microbes and predicted functional pathways for exposed and unexposed sites in each group (Figure 5). We found these potential age-related microbial factors in each group that were likely to affect the integrity of the skin immune and anti-UV barrier, biosynthesis and metabolism of age-related functional substances, and long-term inflammatory stimulation, to further regulate skin intrinsic aging and photoaging processes. Our work provides a new perspective on skin aging, which could be used to develop anti-aging cosmetics and skincare for different ages.
Our work provides a new strategy for future research and clinical application of skin microbiomes. First, by further expanding the research cohort and reducing age stratification, we can describe the variation patterns of skin microbial composition in the aging process more accurately. Then, some specific microbiota can be used as a biomarker to further evaluate skin aging status as a non-invasive auxiliary test. Second, further purification of specific anti-aging microbiota and related metabolites would promote the development of the products related to cosmetic medicine. In addition, based on our findings and further study, skin homeostasis regulators for different age groups can be developed to reduce the incidence of age-related skin diseases. Therefore, our research has great innovation and clinical value.
The datasets presented in this study can be found in online repositories. The names of the repository/repositories and accession number(s) can be found below: https://www.ncbi.nlm.nih.gov/, PRJNA630834; https://www.ncbi.nlm.nih.gov/, PRJNA630117.
Ethical review and approval was not required for the study on human participants in accordance with the local legislation and institutional requirements. Written informed consent to participate in this study was provided by the participants’ legal guardian/next of kin. Written informed consent was obtained from the individual(s), and minor(s)’ legal guardian/next of kin, for the publication of any potentially identifiable images or data included in this article.
DH, LS, ZZ, and ZL contributed to the study and experimental design. ZL, LL, and JY contributed to microbial sample collection. ZL, XY, and JL contributed to the 16S rDNA sequencing data analysis. ZL, YW, TH, and XW contributed to the ITS rDNA sequencing data analysis. ZL, HZ, HW, and KT contributed to the skin parameter collection. ZL and XB contributed to the microbial data analysis and statistics. ZL and TP contributed to the additional data analysis. ZL, XB, and TP wrote the manuscript. All authors read and approved the final manuscript.
This study was funded by the National Natural Science Foundation of China (nos. 81772071, 81971835, 81772072, 81201470, and 81501663). The Talent Support Program of Air Force Medical University “Project Ling Yun” to LS (no. cyjhsll).
The authors declare that the research was conducted in the absence of any commercial or financial relationships that could be construed as a potential conflict of interest.
We thank the following institutions for giving us powerful support for clinical parameters collection: Department of Dermatology in Xijing Hospital, Air Force Medical University, and Guangzhou Matrix Trading, Co. Ltd. Huan Jing and Qiao Zhang provided selfless assistance for picture capture. We thank Allwegene Co., Beijing, China for assisting in the experimentation, data processing, and bioinformatics. We also thank AiMi Academic Services (www.aimieditor.com) for English language editing and review services.
The Supplementary Material for this article can be found online at: https://www.frontiersin.org/articles/10.3389/fmicb.2020.565549/full#supplementary-material
Supplementary Figure 1 | Correlation between clinical parameters and dominant fungal communities.
Supplementary Figure 2 | The bar charts of DNA quantitative results according to qPCR.
Supplementary Figure 3 | The line charts and correlations of selected microbiotas.
Supplementary Table 1 | Relative abundance of dominant species.
Supplementary Table 2 | Comparing the similarity of skin microbial compositions and structures between different age groups.
Supplementary Table 3 | Differences in taxonomic profiles of skin microbiomes between age groups during intrinsic skin aging.
Supplementary Table 4 | Differences in taxonomic profiles of skin microbiomes between age groups during photoaging.
Supplementary Table 5 | The selected genus-specific primer pairs in our study.
Supplementary Table 6 | Multitest correction for the statistics (P-value) for clinical skin parameters of cheeks.
Supplementary Table 7 | Multitest correction for the statistics (P-value) for species richness indices (Chao 1 indices).
Supplementary Table 8 | Multitest correction for the statistics (P-value) for Spearman correlation analysis between skin photoaging related dominant genera.
Supplementary Table 9 | Multitest correction for the statistics (P-value) for Spearman correlation analysis between skin intrinsic aging related dominant genera.
Supplementary Protocol 1 | The protocol of Real-Time Quantitative PCR (qPCR).
An, S., Cha, H. J., Ko, J. M., Han, H., Kim, S. Y., Kim, K. S., et al. (2017). Kinetin improves barrier function of the skin by modulating keratinocyte differentiation markers. Ann. Dermatol. 29, 6–12. doi: 10.5021/ad.2017.29.1.6
Beatrous, S. V., Grisoli, S. B., Riahi, R. R., Matherne, R. J., and Matherne, R. J. (2017). Cutaneous manifestations of disseminated gonococcemia. Dermatol. Online J. 23:13030/qt33b24006.
Belkaid, Y., and Tamoutounour, S. (2016). The influence of skin microorganisms on cutaneous immunity. Nat. Rev. Immunol. 16, 353–366. doi: 10.1038/nri.2016.48
Blume-Peytavi, U., Kottner, J., Sterry, W., Hodin, M. W., Griffiths, T. W., Watson, R. E., et al. (2016). Age-associated skin conditions and diseases: current perspectives and future options. Gerontologist 56, (Suppl. 2), S230–S242. doi: 10.1093/geront/gnw003
Bonté, F., Girard, D., Archambault, J. C., and Desmoulière, A. (2019). Skin changes during ageing. Subcell. Biochem. 91, 249–280. doi: 10.1007/978-981-13-3681-2_10
Buffie, C. G., and Pamer, E. G. (2013). Microbiota-mediated colonization resistance against intestinal pathogens. Nat. Rev. Immunol. 13, 790–801. doi: 10.1038/nri3535
Cao, Y., Qiao, M., Tian, Z., Yu, Y., Xu, B., Lao, W., et al. (2018). Comparative analyses of subgingival microbiome in chronic periodontitis patients with and without IgA nephropathy by high throughput 16S rRNA sequencing. Cell Physiol. Biochem. 47, 774–783. doi: 10.1159/000490029
Cogen, A. L., Nizet, V., and Gallo, R. L. (2008). Skin microbiota: a source of disease or defence? Br. J. Dermatol. 158, 442–455. doi: 10.1111/j.1365-2133.2008.08437.x
Cole, J. R., Wang, Q., Cardenas, E., Fish, J., Chai, B., Farris, R. J., et al. (2009). The ribosomal database project: improved alignments and new tools for rRNA analysis. Nucleic Acids Res. 37, D141–D145. doi: 10.1093/nar/gkn879
Cruickshank-Quinn, C., Armstrong, M., Powell, R., Gomez, J., Elie, M., and Reisdorph, N. (2017). Determining the presence of asthma-related molecules and salivary contamination in exhaled breath condensate. Respir. Res. 18:57. doi: 10.1186/s12931-017-0538-5
De Luca, C., and Valacchi, G. (2010). Surface lipids as multifunctional mediators of skin responses to environmental stimuli. Mediators Inflamm. 2010:321494. doi: 10.1155/2010/321494
Dimitriu, P. A., Iker, B., Malik, K., Leung, H., Mohn, W. W., and Hillebrand, G. G. (2019). New insights into the intrinsic and extrinsic factors that shape the human skin microbiome. mBio 10:e00839-19. doi: 10.1128/mBio.00839-19
Dreno, B., Araviiskaia, E., Berardesca, E., Gontijo, G., Sanchez Viera, M., Xiang, L. F., et al. (2016). Microbiome in healthy skin, update for dermatologists. J. Eur. Acad. Dermatol. Venereol. 30, 2038–2047. doi: 10.1111/jdv.13965
Edgar, R. C. (2013). UPARSE: highly accurate OTU sequences from microbial amplicon reads. Nat. Methods 10, 996–998. doi: 10.1038/nmeth.2604
Fuentes-Tristan, S., Parra-Saldivar, R., Iqbal, H. M. N., and Carrillo-Nieves, D. (2019). Bioinspired biomolecules: mycosporine-like amino acids and scytonemin from Lyngbya sp. with UV-protection potentialities. J. Photochem. Photobiol. B 201:111684. doi: 10.1016/j.jphotobiol.2019.111684
Gallo, R. L., and Hooper, L. V. (2012). Epithelial antimicrobial defence of the skin and intestine. Nat. Rev. Immunol. 12, 503–516. doi: 10.1038/nri3228
Gonzaga, E. R. (2009). Role of UV light in photodamage, skin aging, and skin cancer: importance of photoprotection. Am. J. Clin. Dermatol. 10, (Suppl. 1), 19–24. doi: 10.2165/0128071-200910001-00004
Juge, R., Rouaud-Tinguely, P., Breugnot, J., Servaes, K., Grimaldi, C., Roth, M. P., et al. (2018). Shift in skin microbiota of Western European women across aging. J. Appl. Microbiol. 125, 907–916. doi: 10.1111/jam.13929
Kang, D., Shi, B., Erfe, M. C., Craft, N., and Li, H. (2015). Vitamin B12 modulates the transcriptome of the skin microbiota in acne pathogenesis. Sci. Transl. Med. 7:293ra103. doi: 10.1126/scitranslmed.aab2009
Kim, H. J., Kim, H., Kim, J. J., Myeong, N. R., Kim, T., Park, T., et al. (2018). Fragile skin microbiomes in megacities are assembled by a predominantly niche-based process. Sci. Adv. 4:e1701581. doi: 10.1126/sciadv.1701581
Kim, H. J., Kim, J. J., Myeong, N. R., Kim, T., Kim, D., An, S., et al. (2019). Segregation of age-related skin microbiome characteristics by functionality. Sci. Rep. 9:16748. doi: 10.1038/s41598-019-53266-3
Kong, H. H., Oh, J., Deming, C., Conlan, S., Grice, E. A., Beatson, M. A., et al. (2012). Temporal shifts in the skin microbiome associated with disease flares and treatment in children with atopic dermatitis. Genome Res. 22, 850–859. doi: 10.1101/gr.131029.111
Kuehne, A., Hildebrand, J., Soehle, J., Wenck, H., Terstegen, L., Gallinat, S., et al. (2017). An integrative metabolomics and transcriptomics study to identify metabolic alterations in aged skin of humans in vivo. BMC Genomics 18:169. doi: 10.1186/s12864-017-3547-3
Landen, N. X., Li, D., and Stahle, M. (2016). Transition from inflammation to proliferation: a critical step during wound healing. Cell Mol. Life Sci. 73, 3861–3885. doi: 10.1007/s00018-016-2268-0
Langdon, A., Crook, N., and Dantas, G. (2016). The effects of antibiotics on the microbiome throughout development and alternative approaches for therapeutic modulation. Genome Med. 8:39. doi: 10.1186/s13073-016-0294-z
Lei, L., Zeng, Q., Lu, J., Ding, S., Xia, F., Kang, J., et al. (2017). MALAT1 participates in ultraviolet B-induced photo-aging via regulation of the ERK/MAPK signaling pathway. Mol. Med. Rep. 15, 3977–3982. doi: 10.3892/mmr.2017.6532
Leung, M. H., Chan, K. C., and Lee, P. K. (2016a). Skin fungal community and its correlation with bacterial community of urban Chinese individuals. Microbiome 4:46. doi: 10.1186/s40168-016-0192-z
Leung, M. H., Wilkins, D., and Lee, P. K. (2016b). Erratum: insights into the pan-microbiome: skin microbial communities of Chinese individuals differ from other racial groups. Sci. Rep. 6:21355. doi: 10.1038/srep21355
Leung, M. H., Wilkins, D., and Lee, P. K. (2015). Insights into the pan-microbiome: skin microbial communities of Chinese individuals differ from other racial groups. Sci. Rep. 5:11845. doi: 10.1038/srep11845
Leyden, J. J., Mcginley, K. J., Mills, O. H., and Kligman, A. M. (1975). Age-related changes in the resident bacterial flora of the human face. J. Invest. Dermatol. 65, 379–381. doi: 10.1111/1523-1747.ep12607630
Liavonchanka, A., Hornung, E., Feussner, I., and Rudolph, M. G. (2006). Structure and mechanism of the Propionibacterium acnes polyunsaturated fatty acid isomerase. Proc. Natl. Acad. Sci. U.S.A. 103, 2576–2581. doi: 10.1073/pnas.0510144103
Makrantonaki, E., Bekou, V., and Zouboulis, C. C. (2012). Genetics and skin aging. Dermatoendocrinol 4, 280–284. doi: 10.4161/derm.22372
Martineau, F., Picard, F. J., Ke, D., Paradis, S., Roy, P. H., Ouellette, M., et al. (2001). Development of a PCR assay for identification of staphylococci at genus and species levels. J. Clin. Microbiol. 39, 2541–2547. doi: 10.1128/JCM.39.7.2541-2547.2001
Martínez-Navarro, F. J., Martínez-Morcillo, F. J., López-Muñoz, A., Pardo-Sánchez, I., Martínez-Menchón, T., Corbalán-Vélez, R., et al. (2020). The vitamin B6-regulated enzymes PYGL and G6PD fuel NADPH oxidases to promote skin inflammation. Dev. Comp. Immunol. 108:103666. doi: 10.1016/j.dci.2020.103666
Metin, A., Dilek, N., and Bilgili, S. G. (2018). Recurrent candidal intertrigo: challenges and solutions. Clin. Cosmet. Investig. Dermatol. 11, 175–185. doi: 10.2147/CCID.S127841
Munyaka, P. M., Eissa, N., Bernstein, C. N., Khafipour, E., and Ghia, J. E. (2015). Antepartum antibiotic treatment increases offspring susceptibility to experimental colitis: a role of the gut microbiota. PLoS One 10:e0142536. doi: 10.1371/journal.pone.0142536
Oh, J., Conlan, S., Polley, E. C., Segre, J. A., and Kong, H. H. (2012). Shifts in human skin and nares microbiota of healthy children and adults. Genome Med. 4:77. doi: 10.1186/gm378
Oh, S., Kim, S. H., Ko, Y., Sim, J. H., Kim, K. S., Lee, S. H., et al. (2006). Effect of bacteriocin produced by Lactococcus sp. HY 449 on skin-inflammatory bacteria. Food Chem. Toxicol. 44, 1184–1190. doi: 10.1016/j.fct.2005.08.008
Petry, V., Bessa, G. R., Poziomczyck, C. S., Oliveira, C. F., Weber, M. B., Bonamigo, R. R., et al. (2012). Bacterial skin colonization and infections in patients with atopic dermatitis. An. Bras. Dermatol. 87, 729–734. doi: 10.1590/s0365-05962012000500010
Pfaffl, M. W. (2001). A new mathematical model for relative quantification in real-time RT-PCR. Nucleic Acids Res. 29:e45. doi: 10.1093/nar/29.9.e45
Prescott, S. L., Larcombe, D. L., Logan, A. C., West, C., Burks, W., Caraballo, L., et al. (2017). The skin microbiome: impact of modern environments on skin ecology, barrier integrity, and systemic immune programming. World Allergy Organ J. 10:29. doi: 10.1186/s40413-017-0160-5
Proksch, E., Brandner, J. M., and Jensen, J. M. (2008). The skin: an indispensable barrier. Exp. Dermatol. 17, 1063–1072. doi: 10.1111/j.1600-0625.2008.00786.x
Rinttilä, T., Kassinen, A., Malinen, E., Krogius, L., and Palva, A. (2004). Development of an extensive set of 16S rDNA-targeted primers for quantification of pathogenic and indigenous bacteria in faecal samples by real-time PCR. J. Appl. Microbiol. 97, 1166–1177. doi: 10.1111/j.1365-2672.2004.02409.x
Rocha, M. A., and Bagatin, E. (2018). Skin barrier and microbiome in acne. Arch. Dermatol. Res. 310, 181–185. doi: 10.1007/s00403-017-1795-3
Roth, R. R., and James, W. D. (1988). Microbial ecology of the skin. Annu. Rev. Microbiol. 42, 441–464. doi: 10.1146/annurev.mi.42.100188.002301
Sanford, J. A., and Gallo, R. L. (2013). Functions of the skin microbiota in health and disease. Semin. Immunol. 25, 370–377. doi: 10.1016/j.smim.2013.09.005
Schommer, N. N., and Gallo, R. L. (2013). Structure and function of the human skin microbiome. Trends Microbiol. 21, 660–668. doi: 10.1016/j.tim.2013.10.001
Shibagaki, N., Suda, W., Clavaud, C., Bastien, P., Takayasu, L., Iioka, E., et al. (2017). Aging-related changes in the diversity of women’s skin microbiomes associated with oral bacteria. Sci. Rep. 7:10567. doi: 10.1038/s41598-017-10834-9
Shin, E. J., Lee, J. S., Hong, S., Lim, T. G., and Byun, S. (2019). Quercetin directly targets JAK2 and PKCdelta and prevents UV-Induced photoaging in human skin. Int J. Mol. Sci. 20:5262. doi: 10.3390/ijms20215262
Storz, G., and Imlay, J. A. (1999). Oxidative stress. Curr. Opin. Microbiol. 2, 188–194. doi: 10.1016/s1369-5274(99)80033-2
Tett, A., Pasolli, E., Farina, S., Truong, D. T., Asnicar, F., Zolfo, M., et al. (2017). Unexplored diversity and strain-level structure of the skin microbiome associated with psoriasis. NPJ Biofilms Microbiomes 3:14. doi: 10.1038/s41522-017-0022-5
Wang, Y., Wang, L., Wen, X., Hao, D., Zhang, N., He, G., et al. (2019). NF-kappaB signaling in skin aging. Mech. Ageing Dev. 184:111160. doi: 10.1016/j.mad.2019.111160
Wilantho, A., Deekaew, P., Srisuttiyakorn, C., Tongsima, S., and Somboonna, N. (2017). Diversity of bacterial communities on the facial skin of different age-group Thai males. PeerJ 5:e4084. doi: 10.7717/peerj.4084
Wlaschek, M., Tantcheva-Poór, I., Naderi, L., Ma, W., Schneider, L. A., Razi-Wolf, Z., et al. (2001). Solar UV irradiation and dermal photoaging. J. Photochem. Photobiol. B Biol. 63, 41–51. doi: 10.1016/s1011-1344(01)00201-9
Wolfle, U., Seelinger, G., Bauer, G., Meinke, M. C., Lademann, J., and Schempp, C. M. (2014). Reactive molecule species and antioxidative mechanisms in normal skin and skin aging. Skin Pharmacol. Physiol. 27, 316–332. doi: 10.1159/000360092
Zeng, Q., Jiang, J., Wang, J., Zhou, Q., and Zhang, X. (2019). N-Terminal Acetylation and C-Terminal amidation of spirulina platensis-derived hexapeptide: anti-photoaging activity and proteomic analysis. Mar. Drugs 17:520. doi: 10.3390/md17090520
Zhai, W., Huang, Y., Zhang, X., Fei, W., Chang, Y., Cheng, S., et al. (2018). Profile of the skin microbiota in a healthy Chinese population. J. Dermatol. 45, 1289–1300. doi: 10.1111/1346-8138.14594
Keywords: skin microbiomes, intrinsic skin aging, photoaging, VISIA, skin immune regulation
Citation: Li Z, Bai X, Peng T, Yi X, Luo L, Yang J, Liu J, Wang Y, He T, Wang X, Zhu H, Wang H, Tao K, Zheng Z, Su L and Hu D (2020) New Insights Into the Skin Microbial Communities and Skin Aging. Front. Microbiol. 11:565549. doi: 10.3389/fmicb.2020.565549
Received: 28 May 2020; Accepted: 28 September 2020;
Published: 26 October 2020.
Edited by:
Amparo Latorre, University of Valencia, SpainCopyright © 2020 Li, Bai, Peng, Yi, Luo, Yang, Liu, Wang, He, Wang, Zhu, Wang, Tao, Zheng, Su and Hu. This is an open-access article distributed under the terms of the Creative Commons Attribution License (CC BY). The use, distribution or reproduction in other forums is permitted, provided the original author(s) and the copyright owner(s) are credited and that the original publication in this journal is cited, in accordance with accepted academic practice. No use, distribution or reproduction is permitted which does not comply with these terms.
*Correspondence: Linlin Su, bGlubGluc3VAZm1tdS5lZHUuY24=; Dahai Hu, aHVkaGFpQGZtbXUuZWR1LmNu
†These authors have contributed equally to this work
Disclaimer: All claims expressed in this article are solely those of the authors and do not necessarily represent those of their affiliated organizations, or those of the publisher, the editors and the reviewers. Any product that may be evaluated in this article or claim that may be made by its manufacturer is not guaranteed or endorsed by the publisher.
Research integrity at Frontiers
Learn more about the work of our research integrity team to safeguard the quality of each article we publish.