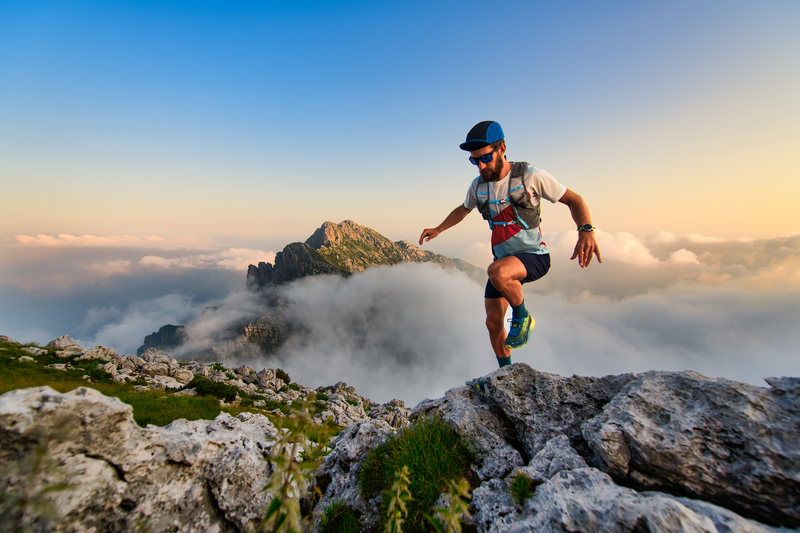
95% of researchers rate our articles as excellent or good
Learn more about the work of our research integrity team to safeguard the quality of each article we publish.
Find out more
ORIGINAL RESEARCH article
Front. Microbiol. , 15 April 2020
Sec. Extreme Microbiology
Volume 11 - 2020 | https://doi.org/10.3389/fmicb.2020.00569
This article is part of the Research Topic Polar and Alpine Microbiological and Biogeochemical Processes in the Warming World View all 13 articles
Snow algae blooms cover vast areas of summer snowfields worldwide, reducing albedo and increasing snow melt. Despite their global prevalence, little is known about the algae species that comprise these blooms. We used 18S and rbcL metabarcoding and light microscopy to characterize algae species composition in 31 snow algae blooms in the Coast Range of British Columbia, Canada. This study is the first to thoroughly document regional variation between blooms. We found all blooms were dominated by the genera Sanguina, Chloromonas, and Chlainomonas. There was considerable variation between blooms, most notably species assemblages above treeline were distinct from forested sites. In contrast to previous studies, the snow algae genus Chlainomonas was abundant and widespread in snow algae blooms. We found few taxa using traditional 18S metabarcoding, but the high taxonomic resolution of rbcL revealed substantial diversity, including OTUs that likely represent unnamed species of snow algae. These three cross-referenced datasets (rbcL, 18S, and microscopy) reveal that alpine snow algae blooms are more diverse than previously thought, with different species of algae dominating different elevations.
Each summer, vast areas of snow surface are colored red by snow algae blooms in polar and alpine snowfields worldwide. Red snowfields have been found on every continent (Marchant, 1982; Yoshimura et al., 1997; Duval et al., 1999; Segawa et al., 2018; Vimercati et al., 2019) as well as overlying Arctic sea ice (Gradinger and Nurnberg, 1996). Snow algae blooms can be quite extensive: in Alaska, remote sensing suggests snow algae covered up to one third of a 1,900 km2 icefield (Ganey et al., 2017). In recent years snow algae have received attention for their role in reducing snow surface albedo, which could substantially increase snow melt (Lutz et al., 2016; Ganey et al., 2017). Thus, snow algae could impact summer water supplies held in mountain snowpack, and reduce glacier mass balance. Snow algae blooms have been recorded throughout history since the time of the ancient Romans (Darwin, 1839; Elder, 1893), but we do not know whether the extent and duration of blooms are increasing with extended melt seasons due to global warming. Despite their potential impact on global albedo, we are only beginning to identify the algae species that comprise snow algae blooms.
Microscopy reveals a diversity of cell morphologies in snow algae blooms, but different species can look nearly identical, and the same species can look completely different depending on environmental conditions (Matsuzaki et al., 2019). The snow algae Chloromonas krienitzii are small green biflagellates in culture, but cells in field samples are nearly twice the diameter, with orange pigment, short spines, and thick cell walls (Matsuzaki et al., 2015). The environmental cues that trigger this transformation are not well understood, but increased light intensity and nitrogen deprivation can trigger secondary pigment accumulation in snow algae (Leya et al., 2009), and also in the freshwater algae Haematococcus pluvialis (Shah et al., 2016). Green blooms of snow algae are less frequently described in the literature than red blooms, and some researchers have suggested that green snow develops into red snow (Mueller et al., 2001). Metabarcoding studies have found green and red snow with distinct community compositions (Lutz et al., 2015; Terashima et al., 2017), but there are some OTUs that are found in both green and red snow (Lutz et al., 2017), leaving open the possibility that red snow develops from green beginnings.
Green algae of class Chlorophyceae are predominant in many snow algae blooms, including the genera Sanguina, Chloromonas, and Chlainomonas. The genus Sanguina was only recently established and contains just two species; however, many sequences from red snow form a yet-unnamed sister clade to Sanguina (Procházková et al., 2019). Sanguina has been found in red and orange snow algae blooms worldwide (Procházková et al., 2019). Many species of snow algae have been assigned to Chloromonas, including at least twelve cultured representatives (Matsuzaki et al., 2019). Various Chloromonas species can form green, orange, or brown colored blooms on the snow surface, and are also found worldwide (Remias et al., 2013, 2018; Procházková et al., 2018b). Less is known about Chlainomonas, which has been found in central Europe, western USA, and New Zealand (Novis et al., 2008; Remias et al., 2016; Procházková et al., 2018a). The distinctive red-pigmented cells of this genus (nearly twice the diameter of Sanguina nivaloides) have only been reported from waterlogged snow overlying alpine lakes (Novis et al., 2008; Remias et al., 2016; Procházková et al., 2018b). 18S rDNA and rbcL sequences show that Chlainomonas is closely related to Chloromonas (Novis et al., 2008). While Chlorophyceae predominate in many snow algae blooms, other classes of snow algae have been reported: Chrysophyceae in yellow snow in Antarctica, the Alps, and Svalbard (Remias et al., 2019; Soto et al., 2020), and Trebouxiophyceae in green snow in Greenland (Lutz et al., 2015).
While many species of snow algae have been described on the basis of morphology and Sanger sequencing, metabarcoding studies have found red snow algae blooms are dominated by relatively few OTUs. Algae community composition was similar in 33 Arctic red snow samples, all of which were dominated by two OTUs of uncultured Chlamydomonadaceae, along with low relative abundance of Raphidonema nivale and Chloromonas polyptera (Lutz et al., 2016). Another study using ITS2 metabarcoding found 24 polar red snow sites contained similar algae assemblages, also dominated by two OTUs of uncultured Chlamydomonadaceae with secondary abundance of Raphidonema and Chloromonadinia (Segawa et al., 2018). Other studies using 18S metabarcoding were limited to class level taxonomic assignments of algae (Hamilton and Havig, 2017)—being highly conserved, short 18S reads cannot distinguish between closely related species or genera.
Based on how little is known about the regional variation in species composition of snow algae blooms, we set out to answer the following questions: what species of snow algae are found in our region? What patterns of co-occurrence exist between species? Which species are the most abundant? Are there distinct bloom types dominated by different species? To answer these questions, we assessed snow algae species composition in 33 samples from the Coast Range of British Columbia using light microscopy and 18S and rbcL (coding for Rubisco large subunit) metabarcoding. rbcL OTU richness was greater than 18S, revealing previously unknown diversity. By cross-referencing rbcL, 18S, and microscopy-based community composition we were able to account for some of the biases inherent in morphology-based identification and PCR-based metabarcoding. Our results show that snow algae species composition was highly variable from site to site, and blooms were dominated by different species at different elevations.
We collected 309 snow algae samples from alpine and subalpine sites in the Coast Range near Vancouver, British Columbia, Canada throughout the summer of 2018 (Supplementary Figure S1). We collected red, orange, and green snow samples from 13 different mountains from elevations ranging from 880 to 2,150 m above sea level (Supplementary Table S2). We collected samples from progressively higher elevations throughout the season as snow melted at lower elevations. We scooped samples from visibly colored snow into sterile 50 mL tubes, and kept samples cold during transport back to the lab by storing in snow. We melted each sample at room temperature on the lab bench, removed a 1 mL aliquot for light microscopy, and then stored the remaining sample at −20°C for up to eight months until DNA extraction.
We immediately fixed microscopy aliquots in 2% gluteraldehyde, which we stored at 4°C for up to 72 h. We quantified the relative abundance of morphospecies in 122 samples by identifying 100 cells on a haemocytometer under 400x light microscopy. We classified cell morphology based on similarity to published photographs of Sanguina nivaloides (Procházková et al., 2019), Chloromonas cf. nivalis (Procházková et al., 2018b), Chloromonas cf. brevispina (Matsuzaki et al., 2015), Chlainomonas krienitzii (Matsuzaki et al., 2015), Chlainomonas rubra (Novis et al., 2008). Cells that did not fall into one of these categories we classified as either “green cell” or “other.” We did not attempt to identify green cells, as different taxa can look highly similar and are therefore prone to misidentification by light microscopy.
We selected 33 samples for rbcL and 18S metabarcoding. We chose this subset to include samples from different mountains, elevations, and dates, including samples containing distinct or unfamiliar cell morphologies. To lyse the cells we freeze-dried samples and mini-pestled 5–20 mg at room temperature before incubation in CTAB lysis buffer (CTAB extraction buffer, 2009). We extracted DNA in small batches of 5–6 samples at a time using chloroform:isoamyl alcohol (Cubero et al., 1999), and purified DNA using ethanol and spin columns (Qiagen, Hilden) (Supplementary Protocol S3). As a negative control against cross-contamination we processed a tube of sterile distilled water alongside each batch, and tested this for DNA with a Qubit fluorometer (Thermo Fisher, Waltham, MA).
We designed custom primers to target a hypervariable region of snow algae rbcL. This gene is an established barcode for green algae, and is highly differentiated at the species level (Hall et al., 2010). We designed primers with the Eurofins primer design tool (https://eurofinsgenomics.eu/en/ecom/tools/pcr-primer-design/) based on the consensus of 20 GenBank snow algae sequences, targeting a 400 bp section of rbcL (Supplementary Figure S5). Sanguina sequences were not included because they were not available at the time. For 18S we used the universal primers Euk1181 and Euk1624 targeting the V7-V8 hypervariable regions (Wang et al., 2014) (Supplementary Figure S4). Primer sequences are available in Supplementary Figure S6.
We constructed our 18S and rbcL amplicon libraries using a standard two-step PCR protocol (Supplementary Protocol S7). The two-step PCR consists of an initial amplification of the region of interest, followed by a secondary amplification that attaches a barcode marker to each oligonucleotide, allowing samples to be pooled for high-throughput sequencing (Meyer and Kircher, 2010). We purified PCR product using Agencourt AMPure XP kit (Beckman Coulter, Brea, CA). We then standardized DNA concentration with Qubit, pooled samples, and sequenced our library on an Illumina MiSeq platform using the V3 kit (Illumina, San Diego, CA).
We demultiplexed reads with CUTADAPT (Martin, 2011), and followed the default pipeline of DADA2 to filter, trim, denoise, dereplicate, merge paired-end reads, and remove chimeras (Callahan et al., 2016). We assigned taxonomy to amplicon sequence variants (ASVs) using IDTaxa, discarding assignments with a confidence score of 50% or lower. This is within IDTaxa's recommended settings of 40–60% (Murali et al., 2018). Because snow algae are not well represented on databases such as SILVA, we made custom databases for both 18S and rbcL using all available snow algae sequences on GenBank. Additionally, we classified 18S ASV taxonomy with SILVA, using the same 50% confidence cutoff as before (Quast et al., 2013).
We visualized rbcL ASV genetic distance using t-SNE (van der Maaten and Hinton, 2008), and then clustered ASVs by sequence similarity into OTUs using DBSCAN (Hahsler et al., 2019) with the epsilon parameter set equal to 4. The output of t-SNE depends on a user-specified parameter “perplexity,” which determines whether the algorithm pays more attention to local or global clustering patterns (Wattenberg et al., 2016). To ensure that our results were not an artifact of this parameter selection, we ran the algorithm with a range of values from 10 to 50 and found no effect on the results. To validate this unconventional OTU clustering method (t-SNE and DBSCAN) we overlaid these OTU clusters on phylogenetic trees using IQTree (Nguyen et al., 2015) (Supplementary Figures S8, S9).
All scripts used in this analysis are freely available at https://github.com/cengstro/bc_snow_algae_amplicon.
We observed morphologically distinct snow algae blooms at different elevations (Figure 1; Supplementary Figure S10). We observed green, orange, and red snow as early as May 18 in forested areas, but did not observe snow algae above treeline (approximately 1,500 m in our study region) until June 20 (Supplementary Table S2). Red snow was prevalent in areas of high solar exposure above treeline, and most of these sites were dominated by cell morphologies we classified as Sanguina cf. nivaloides. Cells resembling Chlainomonas rubra were common at all elevations, often as the dominant cell type. Below treeline, the dominant cell morphologies were classified as Chloromonas cf. brevispina, Chloromonas cf. nivalis, and green cells that we did not attempt to classify.
Figure 1. Representative photographs of snow algae in the Coast Mountains of British Columbia. (A) Bronze colored snow algae blooms below conifer canopy. (B) Dark snow runnels containing snow algae (samples S2.2 and S9.2, Supplementary Table S2). (C) Red snow bloom above treeline at sample site G1.1. (D) Photomicrograph of bronze snow containing Chloromonas cf. brevispina and Chloromonas cf. nivalis. All photomicrographs taken with DIC light microscopy at 630x magnification, all scale bars 30 μm. (E) Photomicrograph of orange snow from the surface of a runnel containing Chloromonas krienitzii and Chloromonas cf. nivalis. (F) Photomicrograph of red snow containing Sanguina nivaloides and Chlainomonas rubra cell morphologies.
Both 18S and rbcL amplicon libraries were dominated by reads assigned to Chlorophyta (Figure 2). We detected 68 algae amplicon sequence variants (ASVs) using 18S: 50 Chlorophyceae, 11 Trebouxiophyceae, and 7 Chrysophyceae. Our rbcL library detected 644 ASVs: 603 Chlorophyta and 41 Trebouxiophyceae. We found seven distinct rbcL ASV clusters (which we define here as OTUs) compared with just three 18S-defined algal OTUs (Supplementary Figure S9). In rbcL, the most abundant genera were Chloromonas, Chlainomonas, and Sanguina. Although the majority of ASVs were not assigned to genus level, our clustering showed that most ASVs were genetically similar to one of these three genera (Figure 2). OTUs “D” and “F” were closely related to Chloromonas, but they did not match any known species on GenBank. OTU “E” was not assigned to genus level, and the ten best BLAST matches included six different genera within Chlamydomonadaceae (86–87% sequence match), two of which were Chloromonas snow algae (LC012735).
Figure 2. (A) Multidimensional scaling (MDS) plot showing genetic distances between rbcL ASVs. Taxonomic assignments are indicated by color, and point size is proportional to total relative abundance. Dotted ellipses indicate OTU clusters. Stress = 0.13. (B) t-Distributed Stochastic Neighbor Embedding (t-SNE) dimensionality reduction of snow algae rbcL ASVs, with perplexity = 30. Dotted lines indicate OTU clustering by DBSCAN with eps=4.
18S and rbcL taxonomic composition varied with elevation. Low elevation samples were similar as shown by low rbcL UniFrac distances (Lozupone and Knight, 2005), while generally high elevation samples were more compositionally distinct (Figure 3). Samples collected latest in the season had the highest diversity (Supplementary Figure S11). Although there was no statistically significant trend between Shannon diversity and date, there was a weak correlation between Faith's phylogenetic diversity and date (Pearson's r = 0.36, p = 0.04). Sanguina predominated above 1,500 m, but was absent below this elevation (Figure 4). High-elevation samples contained one OTU of Chloromonas that was absent from low-elevation sites (Figure 3 OTU “F”). Raphidonema was restricted to three samples from high-elevation snow overlying glaciers (best BLAST match to Raphidonema longiseta, KM462868.1). Most snow algae blooms above treeline were red, but we did collect two green snow samples from above treeline (N1.5, G1.4), both of which were dominated by Chloromonas. Chlainomonas was highly abundant at all elevations, in both high and low relative abundance. Chloromonas krienitzii was predominant around 1,200 m in clearings or sparse trees.
Figure 3. Non-metric multidimensional scaling (NMDS) showing rbcL UniFrac distances between samples. Each sample is labeled by sample ID and colored by elevation.
Figure 4. Stacked barplots showing snow algae relative abundance, as measured by: (top) rbcL OTUs, (middle) 18S taxonomic assignment with custom snow algae database, and (bottom) cell morphology. Samples are arranged from low to high elevation left to right. Green triangles (bottom) indicate samples collected from sites below conifer canopy, and blue squares indicate samples collected from snow overlying glacier.
At one site, we observed the snow transition from green to orange (Supplementary Figure S12). In May, the surface snow was white, with green snow 2–5 cm below the surface of a runnel (sample S2.2). On subsequent visits in June, the same runnel was orange on the surface with the green snow remaining below the surface (samples S4.6, S6.1, S9.2, S11.2). The green snow contained predominantly green cells, including cells with 2 or 4 flagella, while the orange snow contained predominantly orange spherical cells resembling Chloromonas krienitzii (Matsuzaki et al., 2015). Both green and orange snow were dominated by rbcL reads assigned to C. krienitzii, although the orange surface snow contained slightly higher abundance of Chlainomonas (Supplementary Figure S12).
Snow algae blooms are a widespread and globally important phenomenon, yet until now the distribution of distinct blooms within a region has not been well-documented. We present multiple data sets demonstrating elevational patterns in alpine snow algae bloom species composition. Most dramatically, Sanguina was dominant in red snow above treeline, while green and orange blooms of C. krienitzii were dominant in runnels at lower elevations. We found unexpected diversity within rbcL that we did not detect using 18S, including yet-unnamed species.
Community composition was consistent between 18S and rbcL libraries. However, in some cases we were able to distinguish taxa by rbcL that were not distinguished with 18S. For example, Chlainomonas was absent from our 18S dataset (Figure 4), likely because 18S of this genus is nearly identical to that of the closely-related Chloromonas. Because of this similarity, previous metabarcoding studies using 18S may have missed this genus. Indeed, our rbcL data suggest Chlainomonas may be more widely distributed than previously thought. Although previous work suggested that Chlainomonas is restricted to waterlogged snow overlying lakes (Novis et al., 2008; Procházková et al., 2018a), we did not find this to be the case. Only one Chlainomonas-dominant sample was located in waterlogged snow at the edge of a melt pool (sample S8.11), while the other Chlainomonas-dominant sample sites were not notably wetter than the surrounding snow, nor were they located over frozen lakes.
We noted that Chlainomonas was found in consistently higher relative abundance in our rbcL dataset than in cell counts (Figure 3). One possible explanation is that rbcL could have overestimated Chlainomonas due to higher rbcL copy number. rbcL is located in the plastid genome, and Chlainomonas rubra has multiple parietal chloroplasts per cell (Procházková et al., 2018b). Thus, Chlainomonas could have more copies of the plastid genome than genera with only one chloroplast such as Sanguina (Procházková et al., 2019). However, Chlamydomonas reinhardtii plastid genome copy number can vary depending on growth conditions (Eberhard et al., 2002). Accounting for the discrepancy between cell counts and rbcL relative abundance could prove challenging.
Our findings highlight the remaining unexplored diversity in the snow algae microbiome. Many ASVs were closely related to Chloromonas. Two Chloromonas OTUs “D” and “F” did not match any known species in GenBank (Figure 2). The majority of Chloromonas rbcL ASVs did not form distinct clusters. There were at least three species present in this group, however there could be more. One possibility is the 18S of OTU “E” is identical to other algae 18S. Indeed, rbcL diversity could be higher within Chloromonas than other genera because most Chloromonas species lack a pyrenoid (Nozaki et al., 2002), an organelle involved in the carbon-concentrating mechanism which contains high concentrations of cross-linked Rubisco. Perhaps due to the absence of a pyrenoid, many species of Chloromonas have high concentrations of non-synonymous mutations in the region of rbcL that codes for binding Rubisco together (Nozaki et al., 2002). While rbcL is a poor indicator of Chloromonas phylogeny (Nozaki et al., 2002), it nonetheless is highly differentiated between species and therefore is an effective barcode (Hall et al., 2010). Future studies could use ITS2, because compensatory base changes (CBCs) in this region correlate with species boundaries (Wolf et al., 2013).
The variation in species composition we observed could be due to differences in environmental conditions. Notably, Sanguina and Chloromonas “C” were only found in late summer samples from high alpine sites above 1,500 m (Figure 3). The lack of canopy cover at high elevations could select for light-tolerant species, but many low-elevation blooms were found in clearings that also received full sunlight (Figure 4). Nutrients could also impact snow algae species composition: garden fertilizer applied to experimental plots in Alaska stimulated snow algae growth (Ganey et al., 2017), and conifer litter was found to enhance the growth of the cultures of Chloromonas pichinchae, but not Raphidonema nivale (Hoham, 1976). Intriguingly, Chloromonas was dominant in low elevation sites with canopy cover, while we only found Raphidonema at high-elevation glacier sites. In Svalbard, R. nivale abundance increased on glacier surface snow following wind storms, and the authors concluded that Raphidonema is a soil algae that can opportunistically colonize snow via wind (Stibal and Elster, 2005). Given the aerial dispersal capabilities of microalgae (Tesson et al., 2016) and genetic overlap between Arctic and Antarctic snow algae populations (Segawa et al., 2018) it seems unlikely that geographic distance would pose a barrier to snow algae distribution on a regional scale. Day length could also explain some of the seasonal variation we observed: peak snowmelt would coincide with longer day length at our high elevation sites, whereas snowmelt coincides with shorter days at lower elevations. Site topography could also potentially influence species distribution: two sites dominated by C. krienitzii were in runnels overlying ephemeral streams, which could influence snow moisture and nutrient availability. However, measuring these environmental parameters is more challenging than it might initially appear. For example, depending on the time of day and weather we could visit the same bloom and get completely different measurements of temperature, irradiance, or snow moisture. The need for environmental data preceding the blooms is one of several issues that will be addressed in future work.
Previous work has shown that Chloromonas krienitzii undergoes distinct green and orange morphologies (Matsuzaki et al., 2015), but our study is the first to document this transition in the field. The transition occurred from May to June, suggesting that this process is mediated by seasonal changes. Although Chlainomonas rbcL was abundant in orange surface samples, our microscopic observations suggest that this was not the species responsible for the orange coloration, as orange C. krienitzii far outnumbered the red Chlainomonas. Secondary pigments likely protect snow algae from the damaging effects of intense solar irradiation at the snow surface (Bidigare et al., 1993), which could be why green cells were concentrated a few centimeters below the snow surface.
In conclusion, snow algae blooms contain diverse species assemblages, with different species occurring at different elevations. Blooms were dominated by three genera, Chloromonas, Chlainomonas, and Sanguina. We report substantially more species-level diversity than previous studies based on morphology or 18S sequence. Our work provides insight into the diversity and distribution of snow algae, the primary producers in a poorly understood yet globally important microbiome.
All raw sequence data are available under European Nucleotide Archive accession PRJEB34539. All scripts used in this study are available at https://github.com/cengstro/bc_snow_algae_amplicon.
CE, LQ, and KY designed this study. Samples collected by CE with assistance from KY and LQ. KY and CE prepared sequence libraries. CE and KY completed the bioinformatic analysis. CE wrote the manuscript with major input from LQ and KY. All authors discussed the results and contributed to the final manuscript.
This project was funded with a Sector Innovation Grant from Genome BC (SIP016), and a NSERC Individual Discovery Grant, both awarded to LQ.
The authors declare that the research was conducted in the absence of any commercial or financial relationships that could be construed as a potential conflict of interest.
We thank Leah Tooman (Simon Fraser University) for assistance with sequencing, and Chris Rushton (Simon Fraser University) for assistance with bioinformatics.
The Supplementary Material for this article can be found online at: https://www.frontiersin.org/articles/10.3389/fmicb.2020.00569/full#supplementary-material
Bidigare, R. R., Ondrusek, M. E., Kennicutt, M. C., Iturriaga, R., Harvey, H. R., Hoham, R. W., et al. (1993). Evidence a photoprotective for secondary carotenoids of snow algae. J. Phycol. 29, 427–434. doi: 10.1111/j.1529-8817.1993.tb00143.x
Callahan, B. J., McMurdie, P. J., Rosen, M. J., Han, A. W., Johnson, A. J. A., and Holmes, S. P. (2016). DADA2: High-resolution sample inference from Illumina amplicon data. Nat. Methods 13, 581–583. doi: 10.1038/nmeth.3869
Cubero, O. F., Crespo, A., Fatehi, J., and Bridge, P. D. (1999). DNA extraction and PCR amplification method suitable for fresh, herbarium-stored, lichenized, and other fungi. Plant Syst. Evol. 216, 243–249. doi: 10.1007/BF01084401
Darwin, C. (1839). The Voyage of the Beagle. London; New York, NY: Dent; Dutton. Available online at: https://search.library.wisc.edu/catalog/999467080402121
Duval, B., Duval, E., and Hoham, R. W. (1999). Snow algae of the Sierra Nevada, Spain, and High Atlas mountains of Morocco. Int. Microbiol. 2, 39–42.
Eberhard, S., Drapier, D., and Wollman, F.-A. (2002). Searching limiting steps in the expression of chloroplast-encoded proteins: relations between gene copy number, transcription, transcript abundance and translation rate in the chloroplast of Chlamydomonas reinhardtii. Plant J. 31, 149–160. doi: 10.1046/j.1365-313X.2002.01340.x
Elder, P. (1893). “Chap. 57 (56.) Showers of milk, blood, flesh, iron, wool, and baked tiles” in The Natural History of Pliny, Vol. 1 (London: George Bell & Sons). Available online at: https://books.google.ca/books?id=MZYVAAAAYAAJ&printsec=frontcover&dq=Showers+of+milk,+blood,+flesh,+iron,+wool,+and+baked+tiles%27%27+in+The+Natural+History+of+Pliny,+Volume+1.+Bohn%27s+Classical+Library&hl=en&sa=X&ved=0ahUKEwiB_sqO1cXoAhUkJDQIHR7KA8QQ6AEIKDAA#v=onepage&q&f=false
Ganey, G. Q., Loso, M. G., Burgess, A. B., and Dial, R. J. (2017). The role of microbes in snowmelt and radiative forcing on an Alaskan icefield. Nat. Geosci. 10, 754–759. doi: 10.1038/ngeo3027
Gradinger, R., and Nurnberg, D. (1996). Snow algal communities on arctic pack ice floes dominated by Chlamydomonas nivalis (Bauer) Wille. Proc. NIPR Symp. Polar Biol. 9, 35–43.
Hahsler, M., Piekenbrock, M., and Doran, D. (2019). Dbscan: fast density-based clustering with R. J. Stat. Softw. 91, 1–29. doi: 10.18637/jss.v091.i01
Hall, J. D., Fu, K., Lo, C., Lewis, L. A., and Karol, K. G. (2010). An assessment of proposed DNA barcodes in freshwater green algae. Cryptog. Algol. 31, 529–555.
Hamilton, T. L., and Havig, J. (2017). Primary productivity of snow algae communities on stratovolcanoes of the Pacific Northwest. Geobiology 15, 280–295. doi: 10.1111/gbi.12219
Hoham, R. W. (1976). The effect of coniferous litter and different snow meltwaters upon the growth of two species of snow algae in axenic culture. Arct. Alpine Res. 8, 377–386. doi: 10.1080/00040851.1976.12003886
Leya, T., Rahn, A., Lütz, C., and Remias, D. (2009). Response of arctic snow and permafrost algae to high light and nitrogen stress by changes in pigment composition and applied aspects for biotechnology: pigment change in snow algae. FEMS Microbiol. Ecol. 67, 432–443. doi: 10.1111/j.1574-6941.2008.00641.x
Lozupone, C., and Knight, R. (2005). UniFrac: a new phylogenetic method for comparing microbial communities. Appl. Environ. Microbiol. 71, 8228–8235. doi: 10.1128/AEM.71.12.8228-8235.2005
Lutz, S., Anesio, A. M., Edwards, A., and Benning, L. G. (2017). Linking microbial diversity and functionality of arctic glacial surface habitats. Environ. Microbiol. 19, 551–565. doi: 10.1111/1462-2920.13494
Lutz, S., Anesio, A. M., Field, K., and Benning, L. G. (2015). Integrated “Omics”, targeted metabolite and single-cell analyses of arctic snow algae functionality and adaptability. Front. Microbiol. 6:1323. doi: 10.3389/fmicb.2015.01323
Lutz, S., Anesio, A. M., Raiswell, R., Edwards, A., Newton, R. J., Gill, F., et al. (2016). The biogeography of red snow microbiomes and their role in melting arctic glaciers. Nat. Commun. 7:11968. doi: 10.1038/ncomms11968
Marchant, H. J. (1982). Snow algae from the Australian snowy mountains. Phycologia 21, 178–184. doi: 10.2216/i0031-8884-21-2-178.1
Martin, M. (2011). Cutadapt removes adapter sequences from high-throughput sequencing reads. EMBnet J. 17, 10–12. doi: 10.14806/ej.17.1.200
Matsuzaki, R., Kawai-Toyooka, H., Hara, Y., and Nozaki, H. (2015). Revisiting the taxonomic significance of aplanozygote morphologies of two cosmopolitan snow species of the genus Chloromonas (Volvocales, Chlorophyceae). Phycologia 54, 491–502. doi: 10.2216/15-33.1
Matsuzaki, R., Nozaki, H., Takeuchi, N., Hara, Y., and Kawachi, M. (2019). Taxonomic re-examination of “Chloromonas nivalis (Volvocales, Chlorophyceae) zygotes” from Japan and description of C. muramotoi sp. nov. PLoS ONE 14:e0210986. doi: 10.1371/journal.pone.0210986
Meyer, M., and Kircher, M. (2010). Illumina sequencing library preparation for highly multiplexed target capture and sequencing. Cold Spring Harb. Protoc. 2010:pdb.prot5448. doi: 10.1101/pdb.prot5448
Mueller, T., Leya, T., and Fuhr, G. (2001). Persistent snow algal fields in spitsbergen: field observations and a hypothesis about the annual cell circulation. Arctic Antarct. Alpine Res. 33, 42–51. doi: 10.2307/1552276
Murali, A., Bhargava, A., and Wright, E. S. (2018). IDTAXA: A novel approach for accurate taxonomic classification of microbiome sequences. Microbiome 6:140. doi: 10.1186/s40168-018-0521-5
Nguyen, L.-T., Schmidt, H. A., and Haeseler, A. von, Minh, B. Q. (2015). IQ-TREE: a fast and effective stochastic algorithm for estimating maximum-likelihood phylogenies. Mol. Biol. Evol. 32, 268–274. doi: 10.1093/molbev/msu300
Novis, P. M., Hoham, R. W., Beer, T., and Dawson, M. (2008). Two snow species of the quadriflagellate green alga Chlainomonas (Chlorophyta, Volvocales): ultrastructure and phylogenetic position within the Chloromonas clade. J. Phycol. 44, 1001–1012. doi: 10.1111/j.1529-8817.2008.00545.x
Nozaki, H., Onishi, K., and Morita, E. (2002). Differences in pyrenoid morphology are correlated with differences in the rbcL genes of members of the chloromonas lineage (Volvocales, Chlorophyceae). J. Mol. Evol. 55, 414–430. doi: 10.1007/s00239-002-2338-9
Procházková, L., Leya, T., Křížková, H., and Nedbalová, L. (2019). Sanguina nivaloides and Sanguina aurantia gen. Et spp. Nov. (Chlorophyta): the taxonomy, phylogeny, biogeography and ecology of two newly recognised algae causing red and orange snow. FEMS Microbiol. Ecol. 95:fiz064. doi: 10.1093/femsec/fiz064
Procházková, L., Remias, D., Holzinger, A., Řezanka, T., and Nedbalová, L. (2018a). Ecophysiological and morphological comparison of two populations of Chlainomonas sp. (Chlorophyta) causing red snow on ice-covered lakes in the High Tatras and Austrian Alps. Eur. J. Phycol. 53, 230–243. doi: 10.1080/09670262.2018.1426789
Procházková, L., Remias, D., Rezanka, T., and Nedbalova, L. (2018b). Chloromonas nivalis subsp. Tatrae, subsp. Nov. (Chlamydomonadales, Chlorophyta): re-examination of a snow alga from the High Tatra Mountains (Slovakia). Fottea 18, 1–18. doi: 10.5507/fot.2017.010
Quast, C., Pruesse, E., Yilmaz, P., Gerken, J., Schweer, T., Yarza, P., et al. (2013). The SILVA ribosomal RNA gene database project: improved data processing and web-based tools. Nucleic Acids Res. 41, D590–D596. doi: 10.1093/nar/gks1219
Remias, D., Pichrtová, M., Pangratz, M., Lütz, C., and Holzinger, A. (2016). Ecophysiology, secondary pigments and ultrastructure of Chlainomonas sp. (Chlorophyta) from the European Alps compared with Chlamydomonas nivalis forming red snow. FEMS Microbiol. Ecol. 92:fiw030. doi: 10.1093/femsec/fiw030
Remias, D., Procházková, L., Holzinger, A., and Nedbalová, L. (2018). Ecology, cytology and phylogeny of the snow alga Scotiella cryophila K-1 (Chlamydomonadales, Chlorophyta) from the Austrian Alps. Phycologia 57, 581–592. doi: 10.2216/18-45.1
Remias, D., Procházková, L., Nedbalová, L., Andersen, R. A., and Valentin, K. (2019). Two new Kremastochrysopsis species, K. austriaca sp. Nov. And K. americana sp. Nov. (Chrysophyceae). J. Phycol. jpy.12937. doi: 10.1111/jpy.12937
Remias, D., Wastian, H., Lütz, C., and Leya, T. (2013). Insights into the biology and phylogeny of Chloromonas polyptera (Chlorophyta), an alga causing orange snow in Maritime Antarctica. Antarct. Sci. 25, 648–656. doi: 10.1017/S0954102013000060
Segawa, T., Matsuzaki, R., Takeuchi, N., Akiyoshi, A., Navarro, F., Sugiyama, S., et al. (2018). Bipolar dispersal of red-snow algae. Nat. Commun. 9:3094. doi: 10.1038/s41467-018-05521-w
Shah, M. M. R., Liang, Y., Cheng, J. J., and Daroch, M. (2016). Astaxanthin-producing green microalga Haematococcus pluvialis: from single cell to high value commercial products. Front. Plant Sci. 7:531. doi: 10.3389/fpls.2016.00531
Soto, D. F., Fuentes, R., Huovinen, P., and Gómez, I. (2020). Microbial composition and photosynthesis in Antarctic snow algae communities: integrating metabarcoding and pulse amplitude modulation fluorometry. Algal Res. 45:101738. doi: 10.1016/j.algal.2019.101738
Stibal, M., and Elster, J. (2005). Growth and morphology variation as a response to changing environmental factors in two Arctic species of Raphidonema (Trebouxiophyceae) from snow and soil. Polar Biol. 28, 558–567. doi: 10.1007/s00300-004-0709-y
Terashima, M., Umezawa, K., Mori, S., Kojima, H., and Fukui, M. (2017). Microbial community analysis of colored snow from an alpine snowfield in Northern Japan reveals the prevalence of betaproteobacteria with snow algae. Front. Microbiol. 8:1481. doi: 10.3389/fmicb.2017.01481
Tesson, S. V. M., Skjøth, C. A., S?^antl-Temkiv, T., and Löndahl, J. (2016). Airborne microalgae: insights, opportunities, and challenges. Appl. Environ. Microbiol. 82, 1978–1991. doi: 10.1128/AEM.03333-15
van der Maaten, L., and Hinton, G. (2008). Visualizing data using t-SNE. J. Mach. Learn. Res. 9, 2579–2605.
Vimercati, L., Solon, A. J., Krinsky, A., Arán, P., Porazinska, D. L., Darcy, J. L., et al. (2019). Nieves penitentes are a new habitat for snow algae in one of the most extreme high-elevation environments on Earth. Arctic Antarct. Alpine Res. 51, 190–200. doi: 10.1080/15230430.2019.1618115
Wang, Y., Tian, R. M., Gao, Z. M., Bougouffa, S., and Qian, P.-Y. (2014). Optimal eukaryotic 18S and universal 16S/18S ribosomal RNA primers and their application in a study of symbiosis. PLoS ONE 9:e90053. doi: 10.1371/journal.pone.0090053
Wattenberg, M., Viégas, F., and Johnson, I. (2016). How to use t-SNE effectively. Distill 1:e2. doi: 10.23915/distill.00002
Wolf, M., Chen, S., Song, J., Ankenbrand, M., and Müller, T. (2013). Compensatory base changes in ITS2 secondary structures correlate with the biological species concept despite intragenomic variability in ITS2 sequences – a proof of concept. PLoS ONE 8:e66726. doi: 10.1371/journal.pone.0066726
Keywords: snow, algae, microbiome, amplicon, rbcL, 18S, alpine, metabarcoding
Citation: Engstrom CB, Yakimovich KM and Quarmby LM (2020) Variation in Snow Algae Blooms in the Coast Range of British Columbia. Front. Microbiol. 11:569. doi: 10.3389/fmicb.2020.00569
Received: 21 January 2020; Accepted: 15 March 2020;
Published: 15 April 2020.
Edited by:
David Anthony Pearce, Northumbria University, United KingdomReviewed by:
Stefanie Lutz, Agroscope, SwitzerlandCopyright © 2020 Engstrom, Yakimovich and Quarmby. This is an open-access article distributed under the terms of the Creative Commons Attribution License (CC BY). The use, distribution or reproduction in other forums is permitted, provided the original author(s) and the copyright owner(s) are credited and that the original publication in this journal is cited, in accordance with accepted academic practice. No use, distribution or reproduction is permitted which does not comply with these terms.
*Correspondence: Lynne M. Quarmby, cXVhcm1ieUBzZnUuY2E=
Disclaimer: All claims expressed in this article are solely those of the authors and do not necessarily represent those of their affiliated organizations, or those of the publisher, the editors and the reviewers. Any product that may be evaluated in this article or claim that may be made by its manufacturer is not guaranteed or endorsed by the publisher.
Research integrity at Frontiers
Learn more about the work of our research integrity team to safeguard the quality of each article we publish.