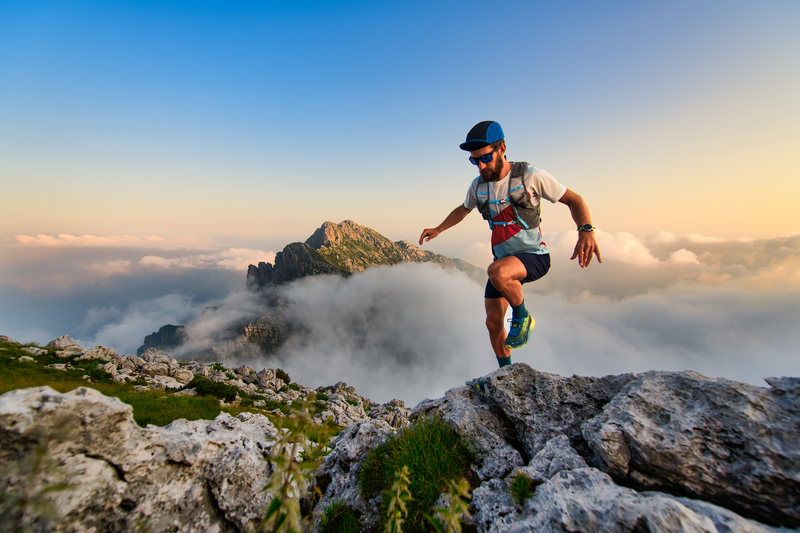
94% of researchers rate our articles as excellent or good
Learn more about the work of our research integrity team to safeguard the quality of each article we publish.
Find out more
ORIGINAL RESEARCH article
Front. Microbiol. , 08 April 2020
Sec. Microbial Symbioses
Volume 11 - 2020 | https://doi.org/10.3389/fmicb.2020.00476
Preterm birth (PTB) is defined as the birth of an infant before 37 weeks of gestational age. It is the leading cause of perinatal morbidity and mortality worldwide. In this study, we present a comprehensive meta-analysis of vaginal microbiome in PTB. We integrated raw longitudinal 16S rRNA vaginal microbiome data from five independent studies across 3,201 samples and were able to gain new insights into the vaginal microbiome state in women who deliver preterm in comparison to those who deliver at term. We found that women who deliver prematurely show higher within-sample variance in vaginal microbiome abundance, with the most significant difference observed during the first trimester. Modeling the data longitudinally revealed a number of microbial genera as associated with PTB, including several that were previously known and two newly identified by this meta-analysis: Olsenella and Clostridium sensu stricto. New hypotheses emerging from this integrative analysis can lead to novel diagnostics to identify women who are at higher risk for PTB and potentially inform new therapeutic interventions.
Preterm birth (PTB) is defined as a live birth before 37 weeks of gestational age. According to the Centers of Disease Control and prevention, one of every 10 infants born in the United States is born prematurely (Ferré, 2016). PTB and low birth weight are among the top causes for infant death in the United States (Mathews and MacDorman, 2010), and can cause complications to newborns (Ward and Beachy, 2003). Despite many attempts for PTB prevention, there is still an acute problem with prevalence rising according to the World Health Organization (Beck et al., 2010).
Spontaneous PTB, accounting for two-thirds of all PTBs (Goldenberg et al., 2008; Romero et al., 2014a), is considered a complex phenotype which can arise due to different causes. Risk factors for spontaneous preterm birth include a previous preterm birth, race, periodontal disease, low maternal body-mass index (Goldenberg et al., 2008), maternal stress (Copper et al., 1996), together with other demographic characteristics such as maternal age, low socioeconomic and educational status and marital status (Goldenberg et al., 2008). In a recent analysis carried out on a large cohort, maternal age of over 40 was associated with PTB (Fuchs et al., 2018). The mechanism behind PTB is unknown, but the phenomenon appears to be a collection of similar phenotypes with many different mechanisms. One proven mechanism is chorioamnionitis, a condition associated with microbial infection of the amniotic fluids (Romero et al., 2001). Unfortunately, intermittent antibiotic treatment of non-pregnant women who had an early spontaneous PTB did not significantly reduce subsequent PTBs (Andrews et al., 2006). Other suggested causes of PTB are progesterone deficiency, disruption of the immune tolerance of the mother toward the fetus and disruption of the vaginal microbial balance, causing an inflammatory process (Romero et al., 2014a), which is the focus of our study.
The human microbiome is becoming a major area of interest with several large consortiums such as the Human Microbiome Project releasing large reference datasets (Turnbaugh et al., 2007). The main focus currently is gut microbiota, but microbial communities from other body sites (oral, vaginal, and skin) are also being studied (Lloyd-Price et al., 2016). In general, the vaginal microbiome is less diverse in comparison to other body sites (Hyman et al., 2005), especially during pregnancy (Aagaard et al., 2012; Romero et al., 2014a). One main focus area of vaginal microbiome research is Bacterial vaginosis (BV), which is a risk factor for PTB (Nejad and Shafaie, 2008). A Lactobacilli-dominated microbiome is associated with a healthy state and BV is best described as a poly-bacterial instability state, with a shift toward higher concentrations of anaerobic bacteria. This high microbial diversity is associated with pro-inflammatory genital cytokines (Anahtar et al., 2015). Romero et al. (2014c) showed in a longitudinal study that pregnant women have a higher abundance of Lactobacilli compared to non-pregnant women. Others reported that the vaginal microbiome varies by gestational age and proximity to the cervix (Aagaard et al., 2012).
Studying the differences in vaginal microbiome during pregnancy with respect to birth timing may provide better understanding of the role of vaginal microbiome in PTB. Romero et al. (2014b) did not find any significant vaginal microbiome differences longitudinally. Hyman et al. (2014) found that uncultured bacteria are significantly different during term and PTB pregnancies, and that black patients have the most diverse microbial communities. DiGiulio et al. (2015) found several differences in abundance in the vaginal microbiome between women who deliver prematurely in comparison to those who deliver at term. They show that women with a Lactobacilli-poor community together with elevated Gardnerella or Ureaplasma abundances had a higher chance to deliver prematurely. Callahan et al. (2017) were able to confirm these associations in one of the cohorts that they examined. Stout et al. (2017) found that in a predominantly black population, there is higher vaginal microbial community richness and diversity in the first and second trimester of pregnancy in women who delivered prematurely when compared to women who delivered at term. Since the previous studies do not have consistent results and vary in the number of samples, subjects and ethnic backgrounds, we aimed to perform a meta-analysis to address these issues and see whether there is a common microbial signature associated with PTB.
Meta-analysis is a systematic approach to combine and integrate cohorts to study a biological question or a disease condition (Haidich, 2010). Meta-analysis provides enhanced statistical power due to a higher number of samples when combined. On the other hand, it requires care to ensure that data are comparable across cohorts and hence commonly utilizes methods such as batch correction (Chen et al., 2011) and mixed effect modeling (Stram, 1996). Meta-analysis has been carried out extensively in the fields of gene expression (Waldron and Riester, 2016) and genome-wide association studies (Evangelou and Ioannidis, 2013), where the robustness of the signatures across different studies is well established (Chen et al., 2014; Hughey and Butte, 2015). Meta-analysis across microbiome studies is much less common due to heterogeneity of the data and lack of analytical standards, though a few recent publications show that meta-analysis is possible and advantageous for microbiome data (Koren et al., 2013; Henschel et al., 2015; Duvallet et al., 2017; Mancabelli et al., 2017). In a recent paper by Duvallet et al. (2017), the authors demonstrate the importance of performing meta-analysis for gut microbiome in health and disease across a large number of studies and samples. Haque et al. (2017) present summary-level meta-analysis using pre-calculated abundance tables from the original studies to draw conclusions about the combined studies. They found that there is a difference in the variance of the vaginal microbiome in the first trimester between women who delivered prematurely and at term. In this study, we perform a comprehensive meta-analysis across 3,201 vaginal microbiome samples from 415 patients. We re-analyze all the samples with the same pipeline, correct for batch effects and apply a longitudinal modeling approach to demonstrate that publicly available data and robust computational approaches can be leveraged to identity new associations between bacterial genera and PTB. A web application with all the analysis results of this study is also available here: https://comphealth.ucsf.edu/app/ptb_microbiome_metaanalysis.
Raw data and metadata for the DiGiulio et al. (2015) cohort were downloaded from ImmPort (Bhattacharya et al., 2014), under Study SDY465 (Immport, 2011) in May 2016. Raw data and metadata for Romero et al. (2014b) cohort were downloaded from the Sequence Read Archive (Leinonen et al., 2011) under BioProject PRJNA242473 (NCBI Sequence Read Archive, 2014)1 in May 2016. Raw data for Hyman et al. (2014) cohort were received from the authors of the study (raw sequences and weeks of collection), metadata was downloaded from Supplementary Figure S1 of the paper. Raw data and metadata for the Callahan et al. (2017) cohort were downloaded from the Sequence Read Archive under BioProject PRJNA393472 (NCBI Sequence Read Archive, 2014)2 in January 2018. Raw data and metadata for the Stout et al. (2017) cohort were downloaded from the Sequence Read Archive under BioProject PRJNA294119 (NCBI Sequence Read Archive, 2014)3 in January 2018. The processed data was uploaded to ImmPort (Bhattacharya et al., 2014) under study SDY1162 (Immport, 2019) and to figshare (Kosti et al., 2019), together with relevant metadata. The DiGiulio cohort were collected from various locations within the United States. DiGiulio cohort was collected at Stanford University (California), the Callahan cohort was collected at Stanford University (California) and UAB (Alabama), the Romero cohort was collected at Wayne State University (Michigan), the Hyman cohort was collected at UCSF (California) and the Stout cohort was collected at Washington University in St. Louis (Missouri).
The raw sequences from the five studies mentioned above were filtered based on their metadata body site information, keeping only sequences from vaginal samples. For each cohort, the trimester of collection was defined by the following guidelines: samples taken in weeks 9–13 are defined as first trimester samples, samples taken in weeks 14–25 are defined as second trimester samples and weeks 26–36 are defined as third trimester samples. We exclude all samples before week 9 and after week 36 from our analysis, to assure similar distributions of sampling in PTB and term cohorts.
The pipeline receives as input raw 16S sequences collected from different studies as shown in Figure 1. It also receives the metadata from all cohorts according to shared properties: Sample and patient ID, week of specimen collection, body site of collected specimen, delivery outcome (term or PTB), trimester of pregnancy (as mentioned above), and patient’s race. The sequences are entered into a UPARSE OTU analysis pipeline using the USEARCH algorithm (Edgar, 2013). The following steps are carried out by USEARCH: (1) reads preparation, (2) reads de-replication by recognizing unique sequences, removing non-biological sequences and removing singletons, (3) taxonomy prediction using 16S reference set from RDP (Wang et al., 2007) (4) Generating an OTU table (5) tree creation by agglomerative clustering of reads. The reason to choose an OTU based method (over denoising methods) is the data in use in this meta-analysis. Earlier data, such as the Hyman cohort could only be found in FASTA format. To address the amplification by different primers, we carried out closed reference alignment of the data to ensure the robustness of the results and concordance of the studies. The closed-reference OTU picking process is the approach of choice if non-overlapping amplicons are compared.
Figure 1. Meta-analysis pipeline. The pipeline is divided into four sections: combining raw 16S rRNA data from five publicly available studies, processing the data using UPARSE, data normalization, and modeling using LMER to analyze with respect to birth timing as an outcome.
Our first effort was directed at processing the five input data sets to ensure these were compatible for cross-dataset analysis and integration. t-Distributed Stochastic Neighbor Embedding (t-SNE) of the OTUs revealed a bias in the data with samples clustering within the original studies (as shown in Figure 2A), when we look at the abundance of the OTUs (counts per million) and after applying a Log transformation (Figure 2B). To overcome this bias we applied the empirical Bayes algorithm ComBat (Johnson et al., 2007) from the sva R package (Leek et al., 2012) that was originally designed to remove batch effects from gene expression microarray measurements. The results of the parametric empirical Bayes data adjustment on the log transformed OTUs is shown in Figure 2C. We used gPCA R package, based on Reese et al. (2013), to show batch removal from the data after passing ComBat correction, using the test statistic delta (which describes batch effect magnitude).
Figure 2. t-SNE Plots of Term and Preterm OTUs. Coloring of the t-SNE Plots is by: (1) Data Origin for (A) raw data, (B) data after log transformation, (C) data after log transformation and ComBat modeling. (2) Pregnancy Outcome (D) data after log transformation and ComBat modeling.
The OTU table was filtered to exclude the bottom 30% of least abundant OTUs, resulting in 8,538 OTUs. This step was taken because including low abundance OTUs in the parallel model fitting procedure led to convergence failures. The threshold was chosen empirically using an analysis of progressively higher thresholds and stopping when the convergence problem was fixed. While the exclusion of OTUs below this quantile may lead to us missing promising rare bacteria, this data restriction does not increase type I error and was performed primarily for technical convenience rather than to enrich for discoveries. Omitting consistently low count OTU may also help remove OTUs that arise from biases in the input data or OTU identification procedure.
The raw OTU table was aggregated by the taxonomic assignment of UPARSE. For each genus, we sum all OTUs assigned to it, resulting in 690 genera. We than applied log transformation followed by ComBat on the data as mentioned above.
In order to show correlation of evenness with within-sample variance, both parameters were calculated on the non-normalized data, within each dataset.
The var function in R was used to calculate the within-sample variance in the following manner: the sum of squared deviations of every observation from the sample mean, divided by the degrees of freedom.
variance equation
Where s2is the variance, n is the number of samples, xi is the value per sample and is the sample mean.
Evenness (J) is defined as the diversity based on Simpson method (H) over total number of non-zero OTUs in a sample (S).
Evenness equation
The diversity was calculated using the vegan R package.
Within-sample variance of OTU abundance was calculated for overall term and preterm samples, and for term and preterm samples in the first, second and third trimesters separately using R’s var function. The within-sample variance calculation shows how variable are the normalized counts from the mean for that particular sample. The calculation was performed for each sample individually, on the ComBat normalized data.
For data from term and preterm pregnancies plotted by weeks a local polynomial regression was fitted, and the Kolmogorov–Smirnov statistic was used to test for significant differences between distributions. The data was also modeled to correct for difference between sampling strategies cohorts and racial composition using linear mixed effects regression from lme4 package in R (Bates et al., 2015).
For within-sample variance analysis by outcome we used the following model:
Trimester represents the sample’s trimester of collection, Outcome represents the sample’s phenotype (term or preterm), Race represents the race of the patient (white, black, asian, or other) and subjectID represents the subject ID for each sample. The p-values were obtained by Satterthwaite approximation from the model coefficients using the lmerTest R package (Kuznetsova et al., 2017).
Weighted linear mixed effects regression with the lme4 (v1.1-15) R package was used to test for differences in OTU abundance between term and preterm vaginal swab samples. For each of OTU the following model was fit:
Trimester represents the sample’s trimester of collection, Outcome represents the sample’s phenotype (term or preterm), Race represents the race of the patient (white, black, asian, or other), subjectID represents the subject ID for each sample and Source is the sample’s original cohort (DiGiulio, Romero, Callahan, Stout, or Hyman).
The weights used were obtained by running the voom function in limma (v3.30.13) (Ritchie et al., 2015) with a similar regression formula, minus the random effects. Contrasts of interest were tested with the emmeans package (v1.1), which allowed us to find group differences conditional on the trimester of observation and averaging over the effect of race. The log fold change we report represents the average change in logCPM when comparing term and preterm samples: log(CPM_preterm/CPM_term). The p-values from all trimesters and OTUs were then adjusted for multiple testing using the Benjamini-Hochberg procedure as implemented in the “p.adjust” function in R.
R custom code used to generate the figures and analysis in the meta-analysis can be found on figshare (Kosti et al., 2019). An Rdata file with computed summary counts tables and other necessary input files for the code (such as metadata) is also available on figshare (Kosti et al., 2019).
We searched the literature for raw 16S data from publicly available microbiome studies from vaginal samples of pregnant women who went on to deliver either preterm or at term. We excluded studies with only processed data or studies lacking metadata and outcome information. Five studies met our criteria: Hyman et al. (2014), Romero et al. (2014b), DiGiulio et al. (2015), Callahan et al. (2017), and Stout et al. (2017)(Table 1). To our knowledge there were no other publicly available PTB related microbiome studies that meet the above criteria at the time of study design. The prevalence of PTB in all five cohorts (ranging from 12.5 to 37%) is higher than in the general population, likely reflecting study design and clinical settings (Ferré, 2016). The experimental design and sampling strategy was different for each cohort (as listed in Table 1) yielding a different number of overall samples. For all three cohorts, the most samples were collected in the second and third trimesters (defined as 14–25 and 25–37 weeks of gestation, respectively). The five cohorts are different from one another not only in their sampling study design and number of patients but also in the racial composition of the cohorts (Table 1 and Supplementary Figure S1). In the Hyman et al. (2014) and DiGiulio et al. (2015) cohorts, most samples are from white patients, while in the Romero et al. (2014b), Callahan et al. (2017), and Stout et al. (2017) cohorts, most samples are from black patients (Supplementary Figure S1). By combining these groups together in a meta-analysis (see section “Materials and Methods”), we are able to capture variability across a more diverse population of patients. The combined data set contains vaginal 16S sequences and metadata for 3,201 samples from 415 pregnant women, mostly split between black and white individuals (44 and 34% accordingly).
Table 1. General properties of individual studies included in the meta-analysis: vaginal microbiome of five cohorts of term and preterm patients: Hyman et al. (2014), Romero et al. (2014b), DiGiulio et al. (2015), Callahan et al. (2017), and Stout et al. (2017).
We quantified the taxonomic composition of all vaginal microbiome samples with a consistent 16S pipeline that estimates the relative abundance of each species-level operational taxonomic unit (OTU) in each sample (Figure 1; see section “Materials and Methods”). As expected, samples cluster by cohort if no normalization is applied to the OTU abundances (Figure 2A) or if log transformation is applied (Figure 2B), but this bias is gone after batch normalization (Figure 2C; see section “Materials and Methods”). We also used a guided PCA analysis (see section “Materials and Methods”) after batch removal by ComBat, resulting in the test statistic delta of 0.05 (when 1 represents full batch effect) and p-value < 0.01. This result means no batch effect was indicated after ComBat. To confirm the successful removal of cohort bias, we fit a linear mixed effect model to the corrected data and did not observe any significant associations between individual species and a specific cohort. We observe a partial clustering of samples by outcome based on their overall OTU abundance profiles (Figure 2D), however, there was no obvious clustering by race or trimester of collection (Supplementary Figures S2A,B). We then aggregated the data by genera and taxonomic assignment to each OTU (see section “Materials and Methods”), and repeated the same normalization process to ensure that there is no bias toward original studies and observed comparable results (Supplementary Figure S3).
Recent attempts to compare microbiome diversity between women who deliver at term and preterm has resulted in mixed findings with some studies reporting a higher diversity in women who delivered preterm (Haque et al., 2017; Stout et al., 2017) and others showing no change in diversity (Romero et al., 2014b). We looked at the microbial variability across samples with different pregnancy outcomes. To explore this, we computed the within-sample variance of OTU abundances for each sample, as an extension of previous uses of variance. In general, variance is a commonly employed metric in gene expression analysis (Pritchard et al., 2001; Mar et al., 2011) and usually is in use to compare genes across samples. It has also been used in microbiome analysis to compare individuals with different clinical outcome (Reddy et al., 2018). The within-sample variance represents how much the normalized OTU abundances for that sample differ from the sample’s mean value (see section “Materials and Methods”). We chose to use within-sample variance to describe OTU abundance for several reasons. First, we seek to quantify how uniform abundances are across OTUs for a given sample, because evenness and other metrics of alpha diversity have associated with microbiome health (Turnbaugh et al., 2009). Supplementary Figure S4 shows the correlation between evenness and variance within each of the dataset, before batch removal. In all datasets we see a negative significant correlation between the two parameters, as expected. Second, the data transformation that we used to correct for batch effects produces an OTU matrix whose entries are no longer read counts. Therefore, richness and the Shannon index–commonly employed measures of alpha diversity–are not appropriate to use here.
We took advantage of the rich longitudinal cohort that we obtained and compared the within-sample variance across the three trimesters in women who delivered at term and preterm using a mixed linear effects regression (see section “Materials and Methods”). In this model, we take into account the trimester of collection, race and the delivery outcome, while correcting for the study and sampling frequency (see section “Materials and Methods”). The longitudinal trend of microbiome within-sample variance differs between samples from women who deliver at term and preterm (Figure 3A), with higher OTU variability across trimesters in the PTB group when compared to the term group throughout pregnancy [trimesters 1–3 (weeks 9–36); p-value < 2.2e-16, Two-sample Kolmogorov–Smirnov test] with the biggest difference in the first trimester (Figure 3B). We repeated the same analysis for the data aggregated by genera (see section “Materials and Methods”) and observed consistent results (Supplementary Figure S5). This finding also appears in four out of the five cohorts individually (Supplementary Figure S6, DiGiulio p-value < 2.2e-16, Romero p-value = 2.8e-9, Callahan p-value < 2.2e-16, and Stout p-value = 0.01, two-sample Kolmogorov–Smirnov test). The difference is not observed in Hyman et al. (2014) where we see very low within-sample variance in both sets, with patients who deliver at term having slightly higher within-sample variance (p-value = 1.1e-4, two-sample Kolmogorov–Smirnov test). It is important to note that the overall within-sample variance for this cohort is very low compared to the other cohorts and the magnitude of the difference is very small. Those results show that genus level trends are in agreement with species level trends although the V region targeted is different for the five original cohorts.
Figure 3. Analysis of modeled within-sample variance in PTB and term samples. (A) Modeled within-sample variance by weeks of gestation (trimesters 1–3). (B) Modeled within-sample variance by trimester of collection. The modeling takes into account the race, outcome, trimester of collection and the interaction between outcome and trimester of collection, while correcting for number of samples per patient.
We use the metadata of the five studies to subset the data into four racial groups: white, black, asian and other. Figure 4A shows that the trend of higher within-sample variance in the PTB group is true consistently across different racial groups. We chose to exclude two groups in this analysis: (1) the asian group that had no PTB samples in the first trimester (as can be seen in Table 1); (2) the “other” group, as the race composition in the group is unknown. We then plotted the results longitudinally. Our method shows that the higher within-sample variance trend in PTB is found across different racial groups. The results are shown in Figure 4B for black patients (p-value = 2.6E-4, two-sample Kolmogorov–Smirnov test) and Figure 4C for white patients (p-value = 1.7E-6, two-sample Kolmogorov–Smirnov test).
Figure 4. Analysis of modeled variance in different racial groups. (A) Modeled within-sample variance stratified by race (trimesters 1–3). (B) Modeled within-sample variance by weeks of gestation (trimesters 1–3) and outcome in black patients. (C) Modeled within-sample variance by weeks of gestation (trimesters 1–3) and outcome in white patients.
Finally, we performed an association meta-analysis comparing the abundance of the bacterial species in women who deliver preterm vs. at term. It is important to recognize that in our final cohort there is a bias arising from the difference in sampling time and study design between combined studies. The DiGiulio et al. (2015) and Callahan et al. (2017) cohorts are the largest cohorts, with the samples collected weekly from the patients whereas the Hyman et al. (2014) and Stout et al. (2017) are smaller, with one sample collected per trimester. In order to address the potential bias arising as a result of combining cohorts with different design and sampling strategy, we assessed the differences between term and preterm samples for each OTU by applying a weighted linear mixed effects regression model correcting for study, sampling bias, race (see section “Materials and Methods”) and adjusting the p-values by FDR.
Tables 2–4 show genera labels for OTUs that we identify as significantly associated with PTB in the first, second, and third trimesters, respectively. The abundance of these OTUs are shown in Supplementary Figure S7. Across all trimesters, there was only one OTU (Otu4172), Lactobacillus, that was more prevalent in patients who deliver at term. Other than this specific OTU, all the significant OTUs were more abundant in patients who delivered prematurely. In the first trimester, we were able to identify the association of a total number of six bacterial genera with PTB, shown in Table 2: Olsenella, Dialister, Prevotella, Megasphaera, Lactobacillus, and Atopobium. Five of the bacterial genera were reported previously in all or some of the original cohorts as having a higher abundance in those who deliver preterm. We also found one novel association in the first trimester using our method: Olsenella. Olsenella is a known oral bacterial genus (Dewhirst et al., 2010) and reported in the past as associated with BV (Srinivasan and Fredricks, 2008) but not PTB. In the second trimester, we were able to show the association of one bacterial genus with PTB, shown in Table 3: Lactobacillus. In the third trimester, we were able to show the association of three bacterial genera with PTB, shown in Table 3: Gardnerella, Lactobacillus, and Aerococcus. Our method now confirmed those genera as significantly associated with PTB. We also found one completely novel association using our method: Clostridium sensu stricto in the third trimester. There are reports of other strains of the Clostridiales order as involved in BV and PTB (Fredricks et al., 2005), but there is no report in the literature of the association of Clostridium sensu stricto with either phenotype.
We carried out a comprehensive longitudinal meta-analysis of vaginal microbiome in PTB, merging five independent studies totaling 3,201 samples from 415 pregnant women that delivered at term or prematurely. Overall, looking at the t-SNE plot of normalized OTUs, partial clustering of the phenotypic groups was observed on a global scale. We found that the microbial within-sample variance in patients who deliver preterm is significantly different from those who deliver at term, with a higher microbial within-sample variance in the group that went on to deliver preterm. We looked at within-sample variance using a measure of sample variance. In general, variance is a method used in the gene expression field. This measure is also used by new emerging tools for microbiome analysis (Bradley and Pollard, 2017). The observed difference in within-sample variance agrees with two of the original studies (Hyman et al., 2014; Stout et al., 2017), whereas the others do not report differences in variance between patients who go on deliver preterm in comparison to those who deliver at term. DiGiulio et al. (2015) for example did not find changes in the variance throughout the pregnancy, but did find change between the pregnancy period to the post-partum period. Differences in vaginal composition between black and white non-pregnant women has been shown in the past (Fettweis et al., 2014). In our analysis we show that the higher within-sample variance in women who delivered prematurely is found in both populations, showing that this finding holds in different ethnic and racial groups.
We were also able to identify bacterial genera that are significantly associated with birth timing across all trimesters using linear mixed effects regression. First, an OTU representing Lactobacillus is found to be more abundant in patients who deliver at term across all trimesters. Since this finding is only at a genera level, further experiments are required here to carry out species and strain level identification. This finding is in agreement with other studies showing that the absence of Lactobacillus species can be used as a predictor for PTB (Usui et al., 2002; Petricevic et al., 2014). Second, we found several bacterial genera that are associated with PTB. Two of those, Olsenella and Clostridium sensu stricto, were not reported in the original studies we used in this meta-analysis. Olsenella is shown to be involved in BV, but not in PTB, and Clostridium sensu stricto was not reported as associated with BV or PTB.
An interesting attempt to predict PTB in the first trimester vaginal microbiome data was performed in a publication by Donders et al. (2009). The authors were able to show that women with normal vaginal microbiome had a 75% lower risk for PTB and that the absence of Lactobacilli, rather than BV, was the strongest risk factor. Based on the associations that we observe, with Lactobacillus as a protective genus found to be significantly higher in term patients across pregnancy trimesters, we would suggest that the lack of Lactobacillus, in combination with other anaerobic bacteria could be used as a stronger predictor for PTB when being tested across pregnancy trimesters.
There are a few limitations in this study. The first one reflects differences in 16S rDNA amplification. DiGiulio et al. (2015) the amplification was targeted toward the V3–V5 and V4 region of the 16S rRNA gene, in Callahan (Callahan et al., 2017) the V4 region was amplified, in Romero et al. (2014b), and Hyman et al. (2014) the V1–V3 region was amplified, and in Stout et al. (2017) both V1–V3 and V3–V5 regions were amplified. This may lead to unevenness in amplification of certain bacteria. One example of such known mismatch is amplification of Gardnerella (Hyman et al., 2005) and in both Romero et al. (2014b) and DiGiulio et al. (2015) the authors added primers to correct for this known bias. In our meta-analysis, we address this issue in several ways. The first is processing the data with a closed reference alignment method to address the amplification by different primers. In a closed-reference OTU picking process, reads are clustered against a reference sequence collection and any reads which do not hit a sequence in the reference sequence collection are excluded from downstream analyses and is the approach of choice if non-overlapping amplicons are compared. This way we limit the results only to known bacteria that is found in RDP, and the chance for mismatches that may arise from open-reference or de novo OTU picking decrease. Also, using a trustworthy reference database with full or almost full coverage to many of the 16S rRNA molecules, we decrease the chance of not identifying the same molecule by 2 different amplified regions from different studies. In addition, we carry out data normalization using ComBat to account for potential batch effects (Johnson et al., 2007). We show the removal of those batch effects by using gPCA and t-SNE plots. We apply linear mixed effects regression that takes into account patient-specific and cohort-specific effects (see section “Materials and Methods”) when carrying out association analysis. Finally, we have merged the OTUs into taxa to reduce variability and repeated the within-sample variance analysis. Those results show that genus level trends are in agreement with species level trends although the V region targeted is different for the five original cohorts.
Another potential limitation is the use of within-sample variance as the metric of difference between women who delivered at term vs. preterm. We are aware that this metric is not commonly employed microbiome studies, though it has been useful in gene expression analysis. Due to the nature of the OTU data we sought to model and the complexity of the normalization done by ComBat, we could not use any of the traditional metrics of alpha diversity. It is not a measure of richness, although it does quantify a particular notion of evenness because it is the sum of deviations of normalized OTU abundances from the mean abundance of a given sample. Furthermore, within-sample variance has a mathematical relationship to beta-diversity and provides a potentially useful measure of how unique a sample is in the data set. This within-sample variance approach produced results that are in agreement with the results in the individual studies we analyzed.
A last possible limitation is patient selection criteria. Patients in the combined studies come from different backgrounds in terms of geography, diet, lifestyle and other characteristics such as height, weight, medical conditions that may affect the results.
We included all available metadata we had in our models, but couldn’t account for missing information in the five studies we combined. While it is clear that other important variables such as prior medical conditions, prior pregnancies information, maternal age, periodontal disease, low maternal body-mass index, low socioeconomic and educational status and single marital status (Goldenberg et al., 2008) and maternal stress (Copper et al., 1996) have a huge impact on PTB, in most cohorts we used those variables do not exist. Also, we lack information about the mode of delivery (vaginal or c-section), about infections and other conditions during the pregnancy and have only partial information on medications given during pregnancy in a few of the studies. Having access to detailed phenotypic data can improve the models and allow us to explore other relevant questions in association with the microbial genera. Finally, the clinical definition of PTB may be different depending on the exact obstetrical definition that was used in the individual studies, however, the majority of the cohorts we investigated focused on spontaneous PTB and the signals that are robust despite the potential patient heterogeneity are more likely to be real as we have seen from prior gene expression meta-analysis studies (Dudley et al., 2009; Haynes et al., 2017; Sweeney et al., 2017). As more data becomes publicly available, we hope that additional standardization and meta-data availability will help address some of the aforementioned issues.
We have shown that meta-analysis of the vaginal microbiome can shed light on outcomes of pregnancy, such as PTB. We first showed that there is a significant difference between the microbial within-sample variance in women who delivered prematurely when compared to patients who deliver at term. We then showed that this differential within-sample variance between women who deliver preterm in comparison to those who deliver at term is observed across pregnancy trimesters and is consistent across different racial or ethnic backgrounds. We also found that while Lactobacillus abundance is associated with term delivery, several genera are associated with PTB. Among those, two associations are novel. This result can inform future diagnostics and help in monitoring pregnancies with a simple swab done in the first trimester of pregnancy, and may in the future decrease the rates of PTB around the world.
Publicly available datasets were analyzed in this study. This data can be found here: SDY465 https://www.immport.org/shared/study/SDY465, PRJNA242473; https://www.ncbi.nlm.nih.gov/bioproject/PRJNA242473, PRJNA294119; https://www.ncbi.nlm.nih.gov/bioproject/PRJNA294119, PRJNA393472; https://www.ncbi.nlm.nih.gov/bioproject/PRJNA393472. The processed data was uploaded to ImmPort under study SDY1162 and to figshare (Kosti et al., 2019), together with relevant metadata.
This study used publicly available data. The studies involving human participants were reviewed and approved at the time of original publications (please see references and data availability statement). Written informed consent was not necessary to obtain for this study.
IK, MS, and AB conceived and designed the analysis. IK refined and normalized the data, created the pipeline, and performed the data analysis and modeling. SL and KP designed the data modeling and association analysis. SL performed data modeling and association analysis. All authors contributed to writing and editing the manuscript.
This project was in part supported by the March of Dimes Prematurity Research Center at Stanford and the Stanford Child Health Research Institute, K01LM012381, Burroughs Wellcome Fund Grant, NIAID R21 grant AI108953 and the Gladstone Institutes. AB was supported in part by the NIAID Bioinformatics Integration Support Contract (BISC) HHSN316201200036W. The content is solely the responsibility of the authors and does not necessarily represent the official views of the National Institutes of Health.
The authors declare that the research was conducted in the absence of any commercial or financial relationships that could be construed as a potential conflict of interest.
We acknowledge Linda Giudice, Kim Chi, Brittni Boyd, Richard Hyman, Eula Fung Molly Stout, and Methodius Tuuli for help accessing the data. We acknowledge Linda Giudice, Jacques Ravel, Silvia Pineda, Hagay Enav, Ben Callahan, and Dan DiGiulio for helpful discussions and comments on the manuscript. We also acknowledge Boris Oskotsky for technical support.
The Supplementary Material for this article can be found online at: https://www.frontiersin.org/articles/10.3389/fmicb.2020.00476/full#supplementary-material
Aagaard, K., Riehle, K., Ma, J., Segata, N., Mistretta, T.-A., Coarfa, C., et al. (2012). A metagenomic approach to characterization of the vaginal microbiome signature in pregnancy. PLoS One 7:e36466. doi: 10.1371/journal.pone.0036466
Anahtar, M. N., Byrne, E. H., Doherty, K. E., Bowman, B. A., Yamamoto, H. S., Soumillon, M., et al. (2015). Cervicovaginal bacteria are a major modulator of host inflammatory responses in the female genital tract. Immunity 42, 965–976. doi: 10.1016/j.immuni.2015.04.019
Andrews, W. W., Goldenberg, R. L., Hauth, J. C., Cliver, S. P., Copper, R., and Conner, M. (2006). Interconceptional antibiotics to prevent spontaneous preterm birth: a randomized clinical trial. Am. J. Obstet. Gynecol. 194, 617–623. doi: 10.1016/j.ajog.2005.11.049
Bates, D., Mächler, M., Bolker, B., and Walker, S. (2015). Fitting linear mixed-effects models using lme4. J. Stat. Softw. 67, 1–48. doi: 10.18637/jss.v067.i01
Beck, S., Wojdyla, D., Say, L., Pilar Bertran, A., Meraldi, M., Harris Requejo, J., et al. (2010). The worldwide incidence of preterm birth: a systematic review of maternal mortality and morbidity. Bull. World Health Organ. 88, 31–38. doi: 10.2471/BLT.08.062554
Bhattacharya, S., Andorf, S., Gomes, L., Dunn, P., Schaefer, H., Pontius, J., et al. (2014). ImmPort: disseminating data to the public for the future of immunology. Immunol. Res. 58, 234–239. doi: 10.1007/s12026-014-8516-1
Bradley, P. H., and Pollard, K. S. (2017). Proteobacteria explain significant functional variability in the human gut microbiome. Microbiome 5:36. doi: 10.1186/s40168-017-0244-z
Callahan, B. J., DiGiulio, D. B., Goltsman, D. S. A., Sun, C. L., Costello, E. K., Jeganathan, P., et al. (2017). Replication and refinement of a vaginal microbial signature of preterm birth in two racially distinct cohorts of US women. Proc. Natl. Acad. Sci. U.S.A. 114, 9966–9971. doi: 10.1073/pnas.1705899114
Chen, C., Grennan, K., Badner, J., Zhang, D., Gershon, E., Jin, L., et al. (2011). Removing batch effects in analysis of expression microarray data: an evaluation of six batch adjustment methods. PLoS One 6:e17238. doi: 10.1371/journal.pone.0017238
Chen, R., Khatri, P., Mazur, P. K., Polin, M., Zheng, Y., Vaka, D., et al. (2014). A meta-analysis of lung cancer gene expression identifies PTK7 as a survival gene in lung adenocarcinoma. Cancer Res. 74, 2892–2902. doi: 10.1158/0008-5472.CAN-13-2775
Copper, R. L., Goldenberg, R. L., Das, A., Elder, N., Swain, M., Norman, G., et al. (1996). The preterm prediction study: maternal stress is associated with spontaneous preterm birth at less than thirty-five weeks’ gestation. Am. J. Obstet. Gynecol. 175, 1286–1292. doi: 10.1016/S0002-9378(96)70042-X
Dewhirst, F. E., Chen, T., Izard, J., Paster, B. J., Tanner, A. C. R., Yu, W.-H., et al. (2010). The human oral microbiome. J. Bacteriol. 192, 5002–5017. doi: 10.1128/JB.00542-10
DiGiulio, D. B., Callahan, B. J., McMurdie, P. J., Costello, E. K., Lyell, D. J., Robaczewska, A., et al. (2015). Temporal and spatial variation of the human microbiota during pregnancy. Proc. Natl. Acad. Sci. U.S.A. 112, 11060–11065. doi: 10.1073/pnas.1502875112
Donders, G. G., Van Calsteren, K., Bellen, G., Reybrouck, R., Van den Bosch, T., Riphagen, I., et al. (2009). Predictive value for preterm birth of abnormal vaginal flora, bacterial vaginosis and aerobic vaginitis during the first trimester of pregnancy. BJOG Int. J. Obstet. Gynaecol. 116, 1315–1324. doi: 10.1111/j.1471-0528.2009.02237.x
Dudley, J. T., Tibshirani, R., Deshpande, T., and Butte, A. J. (2009). Disease signatures are robust across tissues and experiments. Mol. Syst. Biol. 5:307. doi: 10.1038/msb.2009.66
Duvallet, C., Gibbons, S. M., Gurry, T., Irizarry, R. A., and Alm, E. J. (2017). Meta-analysis of gut microbiome studies identifies disease-specific and shared responses. Nat. Commun. 8:1784. doi: 10.1038/s41467-017-01973-8
Edgar, R. C. (2013). UPARSE: highly accurate OTU sequences from microbial amplicon reads. Nat. Methods 10, 996–998. doi: 10.1038/nmeth.2604
Evangelou, E., and Ioannidis, J. P. A. (2013). Meta-analysis methods for genome-wide association studies and beyond. Nat. Rev. Genet. 14, 379–389. doi: 10.1038/nrg3472
Ferré, C. (2016). Effects of maternal age and age-specific preterm birth rates on overall preterm birth rates – United States, 2007 and 2014. MMWR Morb. Mortal. Wkly. Rep. 65, 1181–1184. doi: 10.15585/mmwr.mm6543a1
Fettweis, J. M., Brooks, J. P., Serrano, M. G., Sheth, N. U., Girerd, P. H., Edwards, D. J., et al. (2014). Differences in vaginal microbiome in African American women versus women of European ancestry. Microbiology 160, 2272–2282. doi: 10.1099/mic.0.081034-0
Fredricks, D. N., Fiedler, T. L., and Marrazzo, J. M. (2005). Molecular identification of bacteria associated with bacterial vaginosis. N. Engl. J. Med. 353, 1899–1911. doi: 10.1056/NEJMoa043802
Fuchs, F., Monet, B., Ducruet, T., Chaillet, N., and Audibert, F. (2018). Effect of maternal age on the risk of preterm birth: a large cohort study. PLoS One 13:e0191002. doi: 10.1371/journal.pone.0191002
Goldenberg, R. L., Culhane, J. F., Iams, J. D., and Romero, R. (2008). Epidemiology and causes of preterm birth. Lancet 371, 75–84. doi: 10.1016/S0140-6736(08)60074-4
Haque, M. M., Merchant, M., Kumar, P. N., Dutta, A., and Mande, S. S. (2017). First-trimester vaginal microbiome diversity: a potential indicator of preterm delivery risk. Sci. Rep. 7:16145. doi: 10.1038/s41598-017-16352-y
Haynes, W. A., Vallania, F., Liu, C., Bongen, E., Tomczak, A., Andres-Terrè, M., et al. (2017). Empowering multi-cohort gene expression analysis to increase reproducibility. Pac. Symp. Biocomput. 22, 144–153. doi: 10.1142/9789813207813_0015
Henschel, A., Anwar, M. Z., and Manohar, V. (2015). Comprehensive meta-analysis of ontology annotated 16s rRNA profiles identifies beta diversity clusters of environmental bacterial communities. PLoS Comput. Biol. 11:e1004468. doi: 10.1371/journal.pcbi.1004468
Hughey, J. J., and Butte, A. J. (2015). Robust meta-analysis of gene expression using the elastic net. Nucleic Acids Res. 43:e79. doi: 10.1093/nar/gkv229
Hyman, R. W., Fukushima, M., Diamond, L., Kumm, J., Giudice, L. C., and Davis, R. W. (2005). Microbes on the human vaginal epithelium. Proc. Natl. Acad. Sci. U.S.A. 102, 7952–7957. doi: 10.1073/pnas.0503236102
Hyman, R. W., Fukushima, M., Jiang, H., Fung, E., Rand, L., Johnson, B., et al. (2014). Diversity of the vaginal microbiome correlates with preterm birth. Reprod. Sci. 21, 32–40. doi: 10.1177/1933719113488838
Immport (2011). Available online at https://www.immport.org/shared/study/SDY465 (accessed January 1, 2011).
Immport (2019). Available online at https://www.immport.org/shared/study/SDY1162 (accessed May 1, 2016).
Johnson, W. E., Li, C., and Rabinovic, A. (2007). Adjusting batch effects in microarray expression data using empirical Bayes methods. Biostat. Oxf. Engl. 8, 118–127. doi: 10.1093/biostatistics/kxj037
Koren, O., Knights, D., Gonzalez, A., Waldron, L., Segata, N., Knight, R., et al. (2013). A guide to enterotypes across the human body: meta-analysis of microbial community structures in human microbiome datasets. PLoS Comput. Biol. 9:e1002863. doi: 10.1371/journal.pcbi.1002863
Kosti, I., Lyalina, S., Pollard, K. S., Butte, A., and Sirota, M. (2019). Meta-Analysis of Vaginal Microbiome Data Provides New Insights into Preterm Birth (Figshare). Available online at: https://figshare.com/s/1a57942d9c8f72023ebc (accessed June 17, 2019).
Kuznetsova, A., Brockhoff, P. B., and Christensen, R. H. B. (2017). lmerTest package: tests in linear mixed effects models. J. Stat. Softw. 82, 1–26. doi: 10.18637/jss.v082.i13
Leek, J. T., Johnson, W. E., Parker, H. S., Jaffe, A. E., and Storey, J. D. (2012). The sva package for removing batch effects and other unwanted variation in high-throughput experiments. Bioinformatics 28, 882–883. doi: 10.1093/bioinformatics/bts034
Leinonen, R., Sugawara, H., and Shumway, M. (2011). The sequence read archive. Nucleic Acids Res. 39, D19-D21. doi: 10.1093/nar/gkq1019
Lloyd-Price, J., Abu-Ali, G., and Huttenhower, C. (2016). The healthy human microbiome. Genome Med. 8:51. doi: 10.1186/s13073-016-0307-y
Mancabelli, L., Milani, C., Lugli, G. A., Turroni, F., Ferrario, C., van Sinderen, D., et al. (2017). Meta-analysis of the human gut microbiome from urbanized and pre-agricultural populations. Environ. Microbiol. 19, 1379–1390. doi: 10.1111/1462-2920.13692
Mar, J. C., Matigian, N. A., Mackay-Sim, A., Mellick, G. D., Sue, C. M., Silburn, P. A., et al. (2011). Variance of gene expression identifies altered network constraints in neurological disease. PLoS Genet. 7:e1002207. doi: 10.1371/journal.pgen.1002207
Mathews, T. J., and MacDorman, M. F. (2010). Infant mortality statistics from the 2006 period linked birth/infant death data set. Natl. Vital Stat. Rep. 58, 1–31.
Nejad, V. M., and Shafaie, S. (2008). The association of bacterial vaginosis and preterm labor. J. Pak. Med. Assoc. 58, 104–106.
Petricevic, L., Domig, K. J., Nierscher, F. J., Sandhofer, M. J., Fidesser, M., Krondorfer, I., et al. (2014). Characterisation of the vaginal Lactobacillus microbiota associated with preterm delivery. Sci. Rep. 4:5136. doi: 10.1038/srep05136
Pritchard, C. C., Hsu, L., Delrow, J., and Nelson, P. S. (2001). Project normal: defining normal variance in mouse gene expression. Proc. Natl. Acad. Sci. U.S.A. 98, 13266–13271. doi: 10.1073/pnas.221465998
Reddy, R. M., Weir, W. B., Barnett, S., Heiden, B. T., Orringer, M. B., Lin, J., et al. (2018). Increased variance in oral and gastric microbiome correlates with esophagectomy anastomotic leak. Ann. Thorac. Surg. 105, 865–870. doi: 10.1016/j.athoracsur.2017.08.061
Reese, S. E., Archer, K. J., Therneau, T. M., Atkinson, E. J., Vachon, C. M., de Andrade, M., et al. (2013). A new statistic for identifying batch effects in high-throughput genomic data that uses guided principal component analysis. Bioinformatics 29, 2877–2883. doi: 10.1093/bioinformatics/btt480
Ritchie, M. E., Phipson, B., Wu, D., Hu, Y., Law, C. W., Shi, W., et al. (2015). limma powers differential expression analyses for RNA-sequencing and microarray studies. Nucleic Acids Res. 43:e47. doi: 10.1093/nar/gkv007
Romero, R., Dey, S. K., and Fisher, S. J. (2014a). Preterm labor: one syndrome, many causes. Science 345, 760–765. doi: 10.1126/science.1251816
Romero, R., Gómez, R., Chaiworapongsa, T., Conoscenti, G., Cheol Kim, J., and Mee Kim, Y. (2001). The role of infection in preterm labour and delivery. Paediatr. Perinat. Epidemiol. 15, 41–56. doi: 10.1046/j.1365-3016.2001.00007.x
Romero, R., Hassan, S. S., Gajer, P., Tarca, A. L., Fadrosh, D. W., Bieda, J., et al. (2014b). The vaginal microbiota of pregnant women who subsequently have spontaneous preterm labor and delivery and those with a normal delivery at term. Microbiome 2:18. doi: 10.1186/2049-2618-2-18
Romero, R., Hassan, S. S., Gajer, P., Tarca, A. L., Fadrosh, D. W., Nikita, L., et al. (2014c). The composition and stability of the vaginal microbiota of normal pregnant women is different from that of non-pregnant women. Microbiome 2:4.
Srinivasan, S., and Fredricks, D. N. (2008). The human vaginal bacterial biota and bacterial vaginosis. Interdiscip. Perspect. Infect. Dis. 2008, 1–22. doi: 10.1155/2008/750479
Stout, M. J., Zhou, Y., Wylie, K. M., Tarr, P. I., Macones, G. A., and Tuuli, M. G. (2017). Early pregnancy vaginal microbiome trends and preterm birth. Am. J. Obstet. Gynecol.217, 356.e1–356.e18. doi: 10.1016/j.ajog.2017.05.030
Stram, D. O. (1996). Meta-analysis of published data using a linear mixed-effects model. Biometrics 52, 536–544. doi: 10.2307/2532893
Sweeney, T. E., Haynes, W. A., Vallania, F., Ioannidis, J. P., and Khatri, P. (2017). Methods to increase reproducibility in differential gene expression via meta-analysis. Nucleic Acids Res. 45:e1. doi: 10.1093/nar/gkw797
Turnbaugh, P. J., Hamady, M., Yatsunenko, T., Cantarel, B. L., Duncan, A., Ley, R. E., et al. (2009). A core gut microbiome in obese and lean twins. Nature 457, 480–484. doi: 10.1038/nature07540
Turnbaugh, P. J., Ley, R. E., Hamady, M., Fraser-Liggett, C., Knight, R., and Gordon, J. I. (2007). The human microbiome project: exploring the microbial part of ourselves in a changing world. Nature 449, 804–810. doi: 10.1038/nature06244
Usui, R., Ohkuchi, A., Matsubara, S., Izumi, A., Watanabe, T., Suzuki, M., et al. (2002). Vaginal lactobacilli and preterm birth. J. Perinat. Med. 30, 458–466. doi: 10.1515/JPM.2002.072
Waldron, L., and Riester, M. (2016). Meta-Analysis in gene expression studies. Methods Mol. Biol. 1418, 161–176. doi: 10.1007/978-1-4939-3578-9_8
Wang, Q., Garrity, G. M., Tiedje, J. M., and Cole, J. R. (2007). Naive Bayesian classifier for rapid assignment of rRNA sequences into the new bacterial taxonomy. Appl. Environ. Microbiol. 73, 5261–5267. doi: 10.1128/aem.00062-07
Keywords: microbiome, vaginal microbiome, preterm birth, longitudinal analysis, meta-analysis, public data analysis
Citation: Kosti I, Lyalina S, Pollard KS, Butte AJ and Sirota M (2020) Meta-Analysis of Vaginal Microbiome Data Provides New Insights Into Preterm Birth. Front. Microbiol. 11:476. doi: 10.3389/fmicb.2020.00476
Received: 13 December 2019; Accepted: 04 March 2020;
Published: 08 April 2020.
Edited by:
Alfonso Benítez-Páez, Institute of Agrochemistry and Food Technology (IATA), SpainReviewed by:
Gregory A. Buck, Virginia Commonwealth University, United StatesCopyright © 2020 Kosti, Lyalina, Pollard, Butte and Sirota. This is an open-access article distributed under the terms of the Creative Commons Attribution License (CC BY). The use, distribution or reproduction in other forums is permitted, provided the original author(s) and the copyright owner(s) are credited and that the original publication in this journal is cited, in accordance with accepted academic practice. No use, distribution or reproduction is permitted which does not comply with these terms.
*Correspondence: Marina Sirota, bWFyaW5hLnNpcm90YUB1Y3NmLmVkdQ==
†These authors have contributed equally to this work
Disclaimer: All claims expressed in this article are solely those of the authors and do not necessarily represent those of their affiliated organizations, or those of the publisher, the editors and the reviewers. Any product that may be evaluated in this article or claim that may be made by its manufacturer is not guaranteed or endorsed by the publisher.
Research integrity at Frontiers
Learn more about the work of our research integrity team to safeguard the quality of each article we publish.