- 1Department of Biodiversity, University of Duisburg Essen, Essen, Germany
- 2Department of Biology and Environmental Science, Linnaeus University, Kalmar, Sweden
- 3Research Department for Limnology, University of Innsbruck, Mondsee, Austria
Polynucleobacter (Burkholderiaceae, Betaproteobacteria) and Limnohabitans (Comamonadaceae, Betaproteobacteria) are abundant freshwater bacteria comprising large genetic and taxonomic diversities, with species adapted to physico-chemically distinct types of freshwater systems. The relative importance of environmental drivers, i.e., physico-chemistry, presence of microeukaryotes and geographic position for the diversity and prevalence has not been investigated for both taxa before. Here, we present the first pan-European study on this topic, comprising 255 freshwater lakes. We investigated Limnohabitans and Polynucleobacter using an amplicon sequencing approach of partial 16S rRNA genes along environmental gradients. We show that physico-chemical factors had the greatest impact on both genera. Analyses on environmental gradients revealed an exceptionally broad ecological spectrum of operational taxonomic units (OTUs). Despite the coarse resolution of the genetic marker, we found OTUs with contrasting environmental preferences within Polynucleobacter and Limnohabitans subclusters. Such an ecological differentiation has been characterized for PnecC and LimC before but was so far unknown for less well studied subclusters such as PnecA and PnecB. Richness and abundance of OTUs are geographically clustered, suggesting that geographic diversity patterns are attributable to region-specific physico-chemical characteristics (e.g., pH and temperature) rather than latitudinal gradients or lake sizes.
Introduction
The extent to which environmental factors constrain the distribution of bacterial freshwater taxa and promote their diversity is a critical subject in microbial ecology. For instance, ubiquitously distributed genera such as Polynucleobacter (Burkholderiaceae, Betaproteobacteria) and Limnohabitans (Comamonadaceae, Betaproteobacteria) comprise a broad diversity of species differentially adapted to physico-chemical parameters including pH and climate conditions (Kasalický et al., 2013; Hahn et al., 2015, 2016; Jezberová et al., 2017). The species diversity harbored by each of these two genera can phylogenetically be clustered into groups characterized by differences in their 16S rRNA genes (Hahn, 2003; Jezbera et al., 2011; Newton et al., 2011; Kasalický et al., 2013). The distribution of such genetic clusters is a function of (I) abiotic (physico-chemical), (II) biotic (e.g., co-occurrence with microeukaryotes) and (III) geographic factors, which may reflect their ecological adaptations, and eventually their potential for dispersal. While Polynucleobacter and Limnohabitans belong to the best-studied genera in freshwater ecosystems, the relative importance of each of the three factors for their prevalence and diversity has not been investigated in a single study so far.
Both, Polynucleobacter and Limnohabitans, are abundant in various freshwater ecosystems with diverse environmental conditions (Jezberová et al., 2010; Simek et al., 2010; Newton et al., 2011; Jezbera et al., 2012). For Polynucleobacter, five subclusters were described, namely PnecA, PnecB1, and PnecB2 (so far only investigated as PnecB in cultivation-independent studies), PnecC and PnecD (Hahn, 2003; Wu and Hahn, 2006a). PnecC is the best-studied subcluster so far. It comprises multiple described and presumably a large number of undescribed species with diverse ecophysiological and genomic traits, which contrasts their relatively low 16S rRNA gene sequence diversity (Hahn et al., 2016). On the contrary, PnecA is the least investigated subcluster and considered a rare taxon (Hahn et al., 2011). The Limnohabitans genus was as well divided into five subclusters LimA, LimB, LimC, LimD, and LimE (Kasalický et al., 2013). Ample knowledge on the ecology of Limnohabitans is based on studies employing the R-BT065 FISH probe, which targets bacteria affiliated to four of the subclusters (LimB, LimC, LimD, and LimE) (Simek et al., 2001; Jezbera et al., 2013).
In general, abiotic factors are considered to have the most important impact on bacterial communities (Crump et al., 2007). Several studies addressed the effects of chemical ecosystem features on the distribution of Polynucleobacter and Limnohabitans subgroups (Jezberová et al., 2010; Simek et al., 2010; Newton et al., 2011; Jezbera et al., 2013). The pH that is often the composition-controlling factor for bacteria in ecosystems (Lindström et al., 2005; Fierer and Jackson, 2006; Logue and Lindström, 2008) was observed to have a strong effect on the distribution of Polynucleobacter (Jezberová et al., 2010; Jezbera et al., 2011) and Limnohabitans (Simek et al., 2010, 2010; Jezbera et al., 2012). Other distribution limiting factors are hardness of water (conductivity) (Crump et al., 2007), which is often covarying with pH, temperature, and the concentration of dissolved organic carbon (DOC). The DOC composition is strongly coupled to the biological properties of lake ecosystems, as it summarizes external (e.g., humic substances from degraded plant material) and internal (e.g., primary production of phytoplankton) carbon substrate supplies (Moran and Hodson, 1990).
Among biotic factors, protists may shape bacterial communities through grazing (Grujcic et al., 2018) and exudation of organic matter (Horňák et al., 2017). Specifically, culture-based experiments demonstrated that Polynucleobacter and Limnohabitans strains (LimC, PnecC, and LimB) were preyed on preferentially by flagellates (Šimek et al., 2013; Grujcic et al., 2018). Limnohabitans strains from the R-BT cluster exhibited overall higher growth rates in grazing pressure experiments (Simek et al., 2006). Some Limnohabitans strains use algal derived organic material as substrate source and show specific substrate utilization characteristics in batch cultures (Simek et al., 2010; Kasalický et al., 2013). In growth experiments on organic matter derived from three axenic algae (genera Rhodomonas, Chlamydomonas, and Coelastrum), LimC strains grew on all sources, while a PnecB strain only grew on organic matter derived from Coelastrum (Horňák et al., 2017).
Biogeographical hypotheses have a long history in science and were tested on bacterioplankton community composition and their taxon richness with contradictory results (Horner-Devine et al., 2004; Schiaffino et al., 2013). Hypotheses such as the latitudinal diversity gradient and the species-area relationship postulate a higher richness of taxa with decreasing latitudinal grade and increasing habitat area, respectively. Studies focusing on biogeographic patterns of Polynucleobacter and Limnohabitans are rare, and most knowledge on this topic is available on the PnecC subcluster (Jezberová et al., 2010; Hahn et al., 2015). To our knowledge, except for PnecC the diversity of Polynucleobacter and Limnohabitans in relation to geography has not been studied before.
Here, we analyze the distribution and diversity of Limnohabitans and Polynucleobacter in a large geographical dataset comprising 255 lakes by using amplicon sequencing targeting the V2–V3 region of the 16S rRNA gene for taxonomic assignment. We followed a unique approach to analyze the effects of physico-chemical and geographic lake ecosystem properties, and the co-occurrence with microeukaryotes on the abundance of operational taxonomic units (OTUs) assigned to subclusters. One goal of the study was to assess the power of environmental variables to explain relative abundances of OTUs using variation partition. Further, we correlated relative abundances and diversity measures with environmental gradients to reveal so far uninvestigated ecological trends.
Experimental Procedures
Sampling
Sampling of 255 European freshwater lakes was conducted in August 2012, covering a broad latitudinal gradient ranging from Spain to the South of Scandinavia (Supplementary Table S1). The samples were taken by daylight from the shore of each lake or pond collecting epilimnial water up to 0.5 m depth (Supplementary Table S1). Samples were collected under a gradient setting, therefore, the lakes were sampled once and not in replicates. Samples for genomic DNA extraction were filtered onto 0.2 μm nucleopore filters. To obtain similar biomass per sampling site, water was filtered until the filters were blocked by biomass. Biomass filters were subsequently air dried and preserved below −80°C in a cryoshipper (Chart/MVE, Ball Ground, United States) to avoid DNA degradation.
Assessment of Physico-Chemical and Geographic Factors
Temperature, pH, and conductivity were determined directly in the field in triplicates by use of a Waterproof Tester “Combo” (Hanna Instruments, Vöhringen, Germany). To measure the surface area of each water body, we collected satellite images with corresponding scales from the sampling sites using Google Mymaps (Map data 2017 GeoBasis-DE/BKG (2009), Google). Area calculation of each lake or pond was conducted using ImageJ (v1.8.172; Schneider et al., 2012). The scales of the sampling site images were manually set and an automatic threshold from the 8-bit versions of the images was selected. The particle analysis tool was used to calculate the area in km2 of the thresholded images. Coordinates of the sampling sites were obtained with a GPS device.
DNA Extraction, PCR, and Sequencing
Genomic DNA was extracted from the biomass filters using the my-Budget DNA Mini Kit (Bio-Budget Technologies GmbH, Krefeld, Germany) following the protocol of the manufacturer with minor adaptations. We changed the protocol as follows: Filters were homogenized in 800 μl Lysis Buffer TLS within lysing Matrix E tubes (MP Biomedicals, Santa Ana, CA, United States) and homogenized three times for 45 s using FastPrep (MP Biomedicals, Santa Ana, CA, United States) at 6 m/s followed by incubation for 15 min at 55°C. The quality of the DNA was checked using a NanoDropTM ND-2000 UV–vis spectrophotometer (Thermo Fisher Scientific, Waltham, MA, United States).
PCR amplifications targeted the V2-V3 region of the 16S rRNA gene using the primers 104F (5′-GGC GVA CGG GTG MGT AA-3′) and 515R (5′-TTA CCG CGG CKG CTG GCA C-3′) (Lange et al., 2015). The selected forward primer contains two wobble positions to catch a broader spectrum of taxonomic groups. Primers with wobble positions containing guanine (G) and cytosine (C) clearly prefer the primer-template binding of Alphaproteobacteria DNA, while Betaproteobacteria are favored having adenosine (A) in the primer sequence. The binding strength of G-C pairs is higher than that of A-T pairs. Sequences of other taxa that match the primer variants with G or C at the wobble positions, that are Alphaproteobacteria, are therefore preferentially bound (Supplementary Table S2). Comparisons within as well as between Polynucleobacter and Limnohabitans are assumed to be valid, as all known sequences of isolated bacteria affiliated to these taxa exhibit identical sequences at the primer binding sites.
Each sample was amplified twice using primers with different sample identifiers following the AmpliconDuo protocol (A and B variant) (Lange et al., 2015). For the PCR reaction 1 μl of DNA template in 25 μl PCR reaction with 0.4 units of Phusion DNA polymerase (Thermo Fisher Scientific, Waltham, MA, United States), 0.25 μM primers, 0.4 mM dNTPs and 1 × Phusion buffer (Thermo Fisher Scientific, Waltham, MA, United States) were used. The PCR protocol consisted of 35 cycles, including a denaturation step at 98°C for 30 s, annealing step at 72°C for 45 s, and an elongation step at 72°C for 30 s. Finally, the PCR was completed by a final extension step at 72°C for 10 min. Samples were pooled in equimolar ratios and commercially sequenced using paired-end (2 × 300 bp) HiSeq 2500 Illumina sequencing in “rapid-run” mode (Fasteris, Geneva, Switzerland). Eukaryotic data for the same sites were processed as described in Boenigk et al. (2018).
Bioinformatic Analyses
We used the standardized workflow Natrix1 to analyze the amplicon data. Adapter removal, quality trimming, and demultiplexing using indexed sequences was performed by the sequencing company (Fasteris, Geneva, Switzerland). Thereupon, base quality of raw sequence reads was checked using the FastQC software (v0.11.8, Andrews, 2018). The raw sequences were quality filtered to remove reads with an average Phred quality score below 25 using PRINSEQ-lite (v0.20.4; Schmieder and Edwards, 2011). Additionally, all reads with at least one base with a Phred quality score below 15 were removed. The paired-end reads were assembled and quality filtered with the tool PANDASeq (v2.10; Masella et al., 2012). The remaining reads were dereplicated and chimeras were removed using UCHIME with default parameters (usearch v7.0.1090; Edgar et al., 2011). Finally, a split-sample filtering protocol for Illumina amplicon sequencing (AmpliconDuo) was used as described in Lange et al. (2015) for the removal of sequence artifacts. Sequences that were not found in both sample branches (A and B variant) were discarded resulting in 128,766,060 reads. Filtered reads were clustered in OTUs via the software SWARM using default settings (v2.2.2; Mahé et al., 2014) resulting in 145 759 OTUs. Taxonomic assignment was performed using the database SILVA (SILVA SSURef release 132). Betaproteobacteriales were reassigned to the class Betaproteobacteria.
As we focus in particular on Polynucleobacter (1,076,191 reads; 852 OTUs) and Limnohabitans lineages (729,321 reads; 1 230 OTUs), we assigned the respective OTUs to subclusters of these genera (PnecA, PnecB1, PnecB2, PnecC, and PnecD within Polynucleobacter and LimA, LimB, LimC, LimD, and LimE within Limnohabitans). In course of this assignment, specific chimera checks were applied to exclude chimeric sequences that had not been removed by UCHIME. To do so, the sequences were split in front and rear parts [front sequence (258 bp) and rear sequence (247 bp)] and blasted independently against the SILVA database. Sequences for which the taxonomic assignment of front and rear part did not match at the genus level were removed. Kept sequences were subjected to a further chimera check on the subcluster level. Front and rear sequences were independently assigned to the subclusters by phylogenetic placement (Janssen et al., 2018), and those sequences for which the assignment did not match were removed. The trees underlying the phylogenetic placement have been calculated as follows. 16S rRNA gene sequences of isolated Polynucleobacter and Limnohabitans strains were collected from GenBank (Clark et al., 2016). Solely two uncultured clones representing LimD were included, as no sequences of cultured representatives of this subcluster are publicly available. As outgroups, sequences of Cupriavidus metallidurans strain CH34T and Curvibacter gracilis strain 7-1T were included for Polynucleobacter and Limnohabitans, respectively. Sequences were aligned with muscle (Edgar, 2004) using the MEGA X software (Kumar et al., 2018). Alignments were trimmed to a length of 1384 bp for Polynucleobacter and 1322 bp for Limnohabitans. Shorter sequences as well as identical sequences were excluded from the alignment. Phylogenetic trees were calculated from these alignments using RAxML with the rapid hill-climbing algorithm and the GTRGAMMA nucleotide substitution model (Stamatakis, 2015). The trees including the phylogenetic placement of those OTUs that were finally kept are shown in Figures 1, 2. The applied filter method resulted in 25 Polynucleobacter OTUs accounting for 950,890 reads and 51 OTUs assigned to Limnohabitans subclusters with 631,159 reads. All OTUs and the respective number of reads are given in Supplementary Table S3. We have refrained from assigning OTUs on species level, as species within the selected genera cannot be resolved based on 16S rRNA gene sequences (Kasalický et al., 2013; Hahn et al., 2016). This is also evident in Figure 1 depicting two different PnecC species with identical sequences, which are thus represented by the same OTU (C_77).
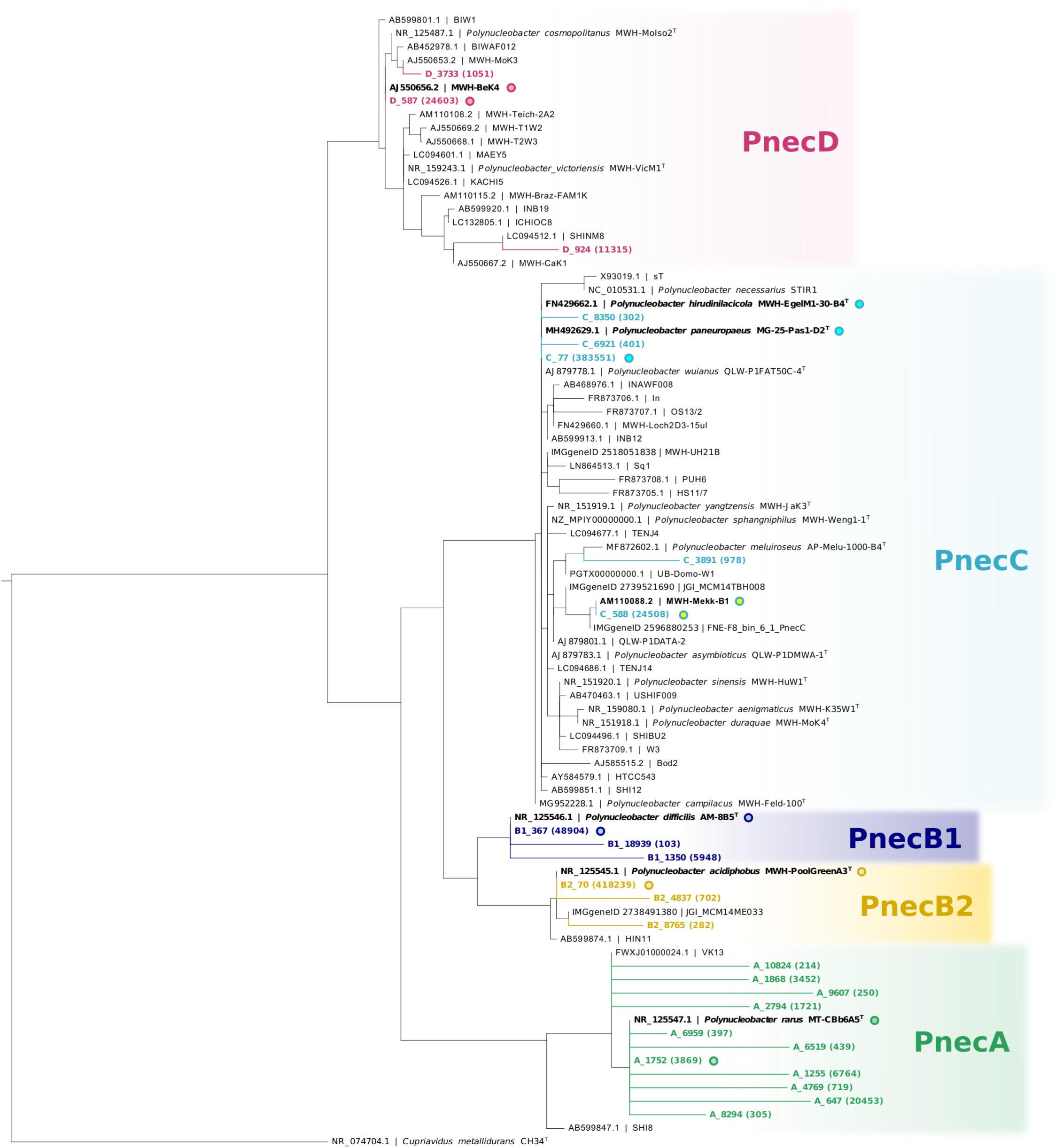
Figure 1. Phylogeny of Polynucleobacter OTUs with respect to 16S rRNA gene sequences of isolated strains. The centroid sequences (Mahé et al., 2014) representing all Polynucleobacter OTUs used in this study (in colors) were integrated into a RAxML backbone tree by phylogenetic placement. The backbone tree is based on the alignment of 16S rRNA gene sequences of isolated strains. Redundant reference sequences were excluded, except for two identical sequences within PnecC that represent different species. Designations of reference sequences consist of accession IDs before the vertical bar, and strain names after the vertical bar. Sequences are available either in GenBank or the IMG/ER database. Accession IDs referring to the latter database are designated accordingly. Sequence identities between OTUs and sequences of the backbone tree are highlighted by circles with common fill color. Numbers in brackets show the read count of each OTU in the whole dataset.
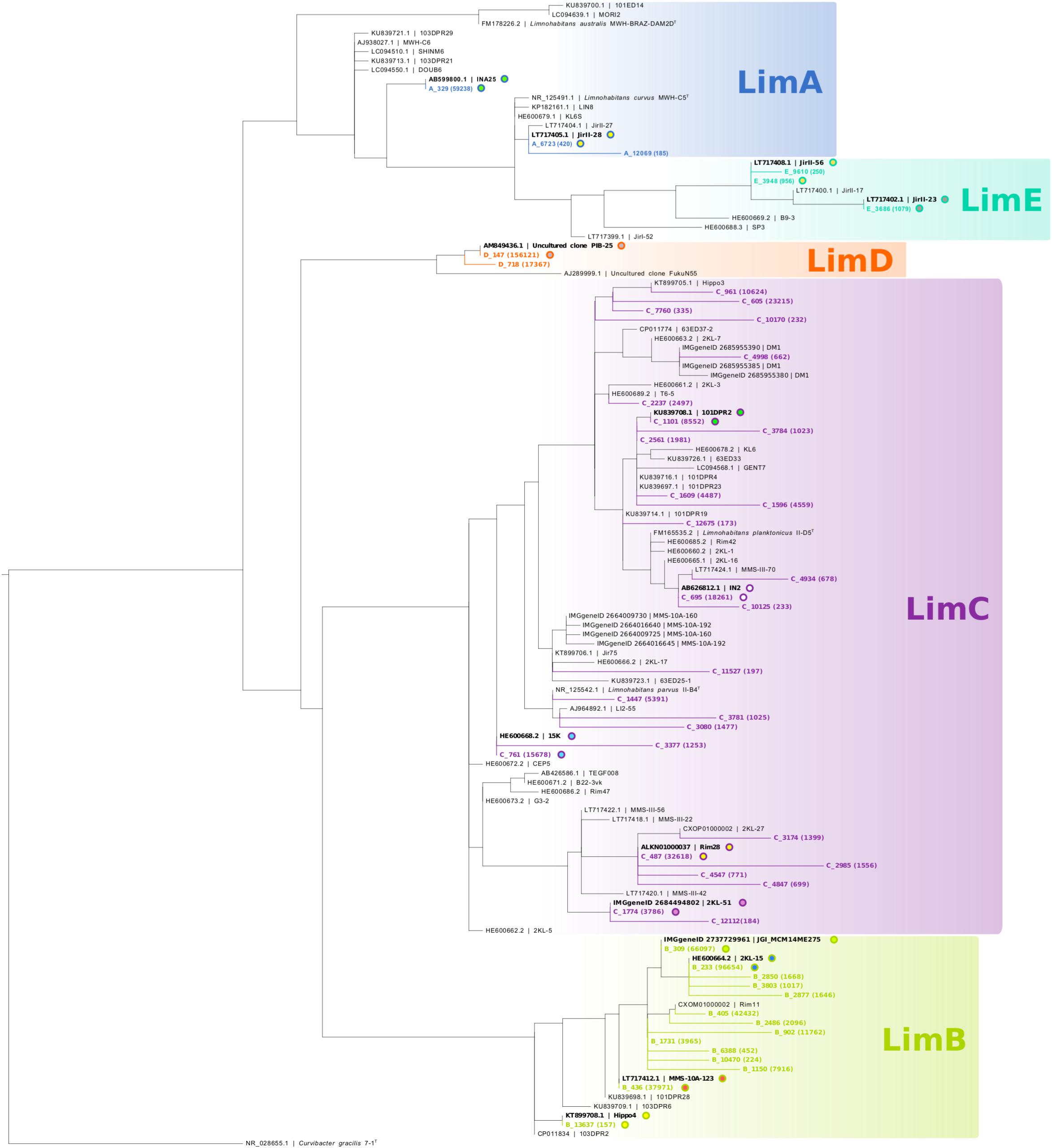
Figure 2. Phylogeny of Limnohabitans OTUs with respect to 16S rRNA gene sequences of isolated strains. The tree was generated analogous to Figure 1, i.e., all Limnohabitans OTUs (in colors) were used for phylogenetic placement, and the backbone tree is based on 16S rRNA gene sequences of isolated strains. Two sequences from uncultured clones of LimD were allowed in the backbone tree, as no cultured representatives of this subcluster are publicly available. Some Limnohabitans strains comprise multiple ribosomal operons, and are represented by multiple sequences in case of sequence differences among different 16S rRNA gene copies.
Statistical Analyses
Prior to statistical analyses conducted using R v3.5.2 (R Core Team, 2018), each dataset was filtered for unreliable OTUs. To detect these OTUs, the samples were normalized by the total number of assigned reads per sample and each OTU in a sample was discarded with a relative abundance below a global threshold of 0.01%. The relative read abundances of Limnohabitans and Polynucleobacter OTUs among all bacteria were used to investigate their occurrence and frequency in presence of different environmental factors (temperature, pH, lake surface area, conductivity, and geographical position) visualized in jittered scatter plots (ggplot2 package, v3.1.0; Wickham, 2011). To explain the variation in the distribution of Polynucleobacter and Limnohabitans subclusters by (1) physico-chemical factors, (2) biotic factors (protistan community) and (3) geography on the distribution of Polynucleobacter and Limnohabitans subcluster, we applied variation partitioning (Blanchet et al., 2008). The response matrices are dissimilarity matrices (Bray–Curtis) of bacterial Hellinger-transformed community data (on basis of OTUs) of Polynucleobacter or Limnohabitans. The physico-chemical, biotic or geographical dataset was used accordingly as explanatory variable. The environmental dataset included five chemical and topographical parameters (Supplementary Table S1). The biotic dataset consisted of community data of microeukaryotes. The geographical dataset comprised Moran Eigenvector maps (MEMs) created by function dbmem, cf, distances ≤852 km (Blanchet et al., 2008), geographical latitude and longitude. MEMs decompose the spatial structure of a dataset into a large number of Eigenfunctions.
To investigate correlations of abiotic factors and the relative abundance of Polynucleobacter and Limnohabitans subclusters, Spearman rank correlations were calculated and visualized with the corrplot package v0.84 (Wei and Simko, 2017). To investigate relations between eukaryotic taxa and the subclusters of Polynucleobacter and Limnohabitans without the influence of covarying abiotic factors, we calculated Kendall partial rank correlations. Fungi, Metazoa, and mainly parasitic taxonomic groups were excluded from the eukaryotic dataset. Correlations with p-values below 0.05 were considered as significant. The correlations were visualized in a co-occurrence network using Cytoscape v.3.7.1 (Shannon et al., 2003). Correlation coefficients were represented as edges, and subcluster and eukaryotes were shown as nodes.
Further, we calculated diversity indices, i.e., OTU richness, Shannon index, Simpson index, and Evenness to analyze the effect of geography on diversity. We plotted the OTU richness and other diversity indices per sample and pie-charts depicting the subcluster composition on a map of Europe. To relate environmental factors to patterns of taxon richness and diversity indices, pH, area, and temperature of each sample were visualized with color gradients. The maps were generated with the rworldmap package v1.3-6 (South, 2011).
Data Availability
Raw amplicon sequences from the prokaryotic samples were deposited in the Sequence Read Archive at NCBI under Bioproject accession number PRJNA559862. Eukaryotic samples are available under Bioproject PRJNA414052.
Results
Influence of Environmental Factors
To analyze the influence of the environmental factors, i.e., geography, microeukaryotes and physico-chemistry on Polynucleobacter and Limnohabitans, we conducted variation partition analyses. The considered environmental factors explained overall 32.90% of the total variation of the Polynucleobacter community, while the same factors explained overall 15.70% of the total variation of the Limnohabitans community (Table 1). Of the total explained variation of Polynucleobacter, physico-chemical variables (pH, temperature, area, conductivity, and elevation) accounted for approximately 50%. The intersection of physico-chemistry and geography (latitude, longitude and MEMs) explained together 16.77% while geography alone explained 10.37%. The community composition of microeukaryotes explained with 3.66% a minor part of the variation. For Limnohabitans, physico-chemistry and the intersection of physico-chemistry and geography accounted for 26.32% each of the total variation. Geography and the community composition of microeukaryotes explained with 18.66 and 11.96%, respectively, a higher extent of the variation in Limnohabitans in comparison to Polynucleobacter.
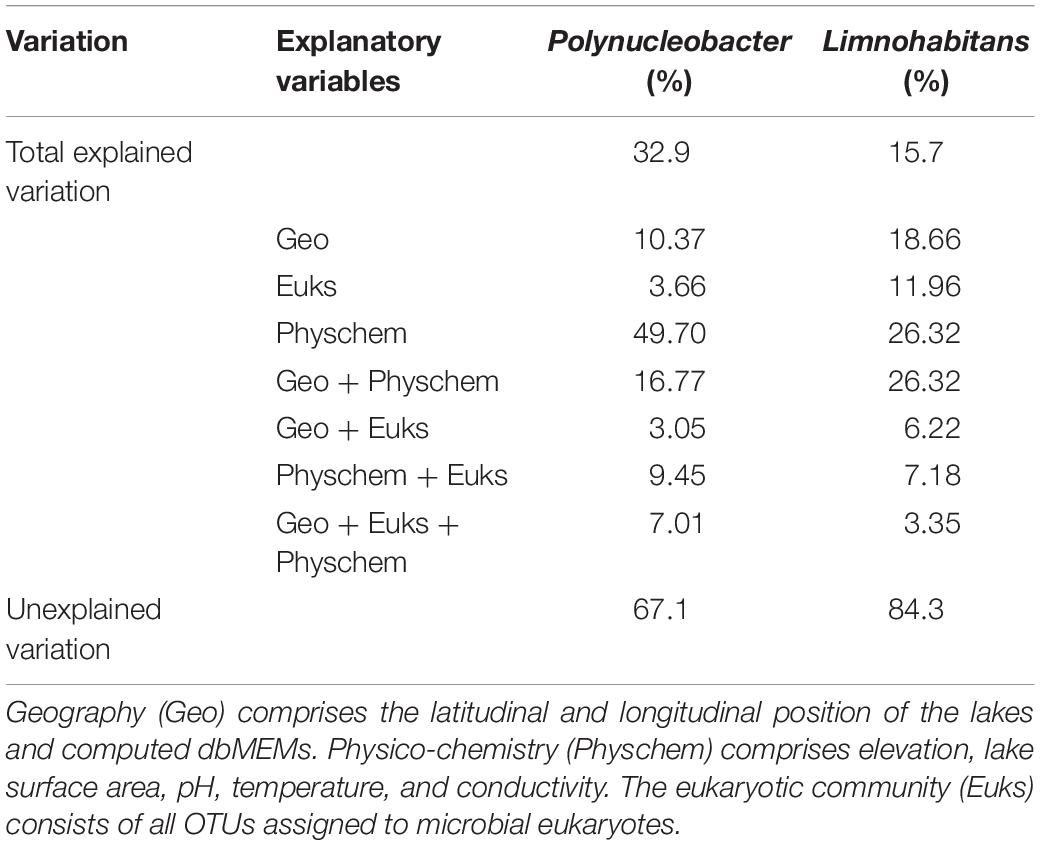
Table 1. Relative contribution of environmental variables, explaining the variation of Polynucleobacter and Limnohabitans.
Taxonomic Community Composition
Bacteroidetes, Alphaproteobacteria, and Cyanobacteria represented, despite site-specific variations, the major bacterial groups detected across the sampled lakes (38 ± 25, 19 ± 15, and 27 ± 22% of total bacterial reads). Betaproteobacteria contributed 3 ± 5% of all bacterial reads. Reads affiliated to Polynucleobacter accounted for 39 ± 55% and reads affiliated to Limnohabitans accounted for 19 ± 27% of all reads assigned to Betaproteobacteria (Table 2 and Supplementary Figure S1).
The bacterial dataset showed an unusual composition of the relative abundances of taxa in comparison to various other freshwater lake studies. Considering the major proteobacterial classes, Betaproteobacteria seem to be underrepresented, while Alphaproteobacteria might be overrepresented (Table 2). OTUs of the Limnohabitans genus were assigned to five subclusters. LimA (3 OTUs), LimB (14 OTUs), LimC (28 OTUs), LimD (2 OTUs), and LimE (3 OTUs). Subclusters LimB, LimD, and LimC were most abundant (47 ± 31, 24 ± 27, and 23 ± 31%, respectively), while subclusters LimA and LimE represented the minor fraction of the Limnohabitans genus across samples (Table 2). Yet, LimA comprised the second most abundant Limnohabitans OTU (A_329, 59,238 reads), which shares 100% sequence identity with strain INA25 (Figure 2). The most abundant OTU was D_147 with 156,121 reads, sharing sequence identity with the uncultured clone PIB-25 (Figure 2).
Operational taxonomic units of the Polynucleobacter genus were assigned to five subclusters. PnecA (11 OTUs), PnecB1 (3 OTUs), PnecB2 (3 OTUs), PnecC (4 OTUs), and PnecD (3 OTUs). PnecB2 (53 ± 42%), and PnecC (31 ± 39%) represented the major fraction within the Polynucleobacter genus across samples. Although PnecA contained the most OTUs, the PnecA subcluster had a comparably low relative abundance (4 ± 8%). Also the PnecD subcluster comprised a small relative fraction of reads (5 ± 15%). The most abundant Polynucleobacter OTUs were B2_70 with 418 239 reads (sequence identity with the type strain of P. acidiphobus) and C_77 with 383 551 reads (sequence identity with the type strains of P. paneuropaeus and P. hirudinilacicola) (Figure 1).
Relations of Polynucleobacter and Limnohabitans
Relative abundances of Polynucleobacter and Limnohabitans genera were not significantly related to each other along environmental gradients (Supplementary Figure S2). On subcluster level several subclusters were strongly correlated with one another (Supplementary Figure S3). In this respect, PnecA positively covaried with PnecC, LimB, and LimD. Both, PnecC and LimB had high correlation coefficients with LimD. PnecB2 had the highest negative correlation coefficient with PnecC.
Supplementary Figure S4 shows networks of correlations of OTUs based on relative read abundances. The network of positive correlations roughly separated into two pole-like clusters with strongly interconnected groups of OTUs (Supplementary Figure S4a). The left cluster is dominated by OTUs that are negatively correlated with pH, while the OTUs within the right cluster were predominantly associated with higher pH (Supplementary Figure S5). The two most abundant Polynucleobacter OTUs C_77 and B2_70 are located within the left and right cluster, respectively, and were strongly negatively correlated with each other (Supplementary Figure S4b). The OTU C_77 positively covaried with two of the most abundant Limnohabitans OTUs B_405 and D_147, while B2_70 was positively correlated with the LimB OTUs B_233, B_309 and B_436.
Composition in Dependency of Physico-Chemical Factors
PnecC exhibited the strongest associations with environmental factors (Figure 3 and Supplementary Figure S6). The relative read abundance of PnecC was negatively correlated with pH, conductivity, temperature, and lake surface area. Similarly, PnecA was associated with low pH, conductivity and temperature. Compared to PnecA and PnecC, PnecB2 exhibited inverse trends, i.e., this subcluster was preferentially detected at higher pH, conductivity, water temperature, and lake surface area. PnecB1 only correlated positively with latitude and negatively with temperature, and PnecD covaried positively with conductivity and area. Relations of the Limnohabitans subclusters LimA, LimB, LimD, and LimE were overall negative with pH, conductivity and except for LimB with temperature. LimB was positively correlated with area and LimE was negatively associated with area and positively with latitude. LimC exhibited no significant correlations to any tested environmental factor.
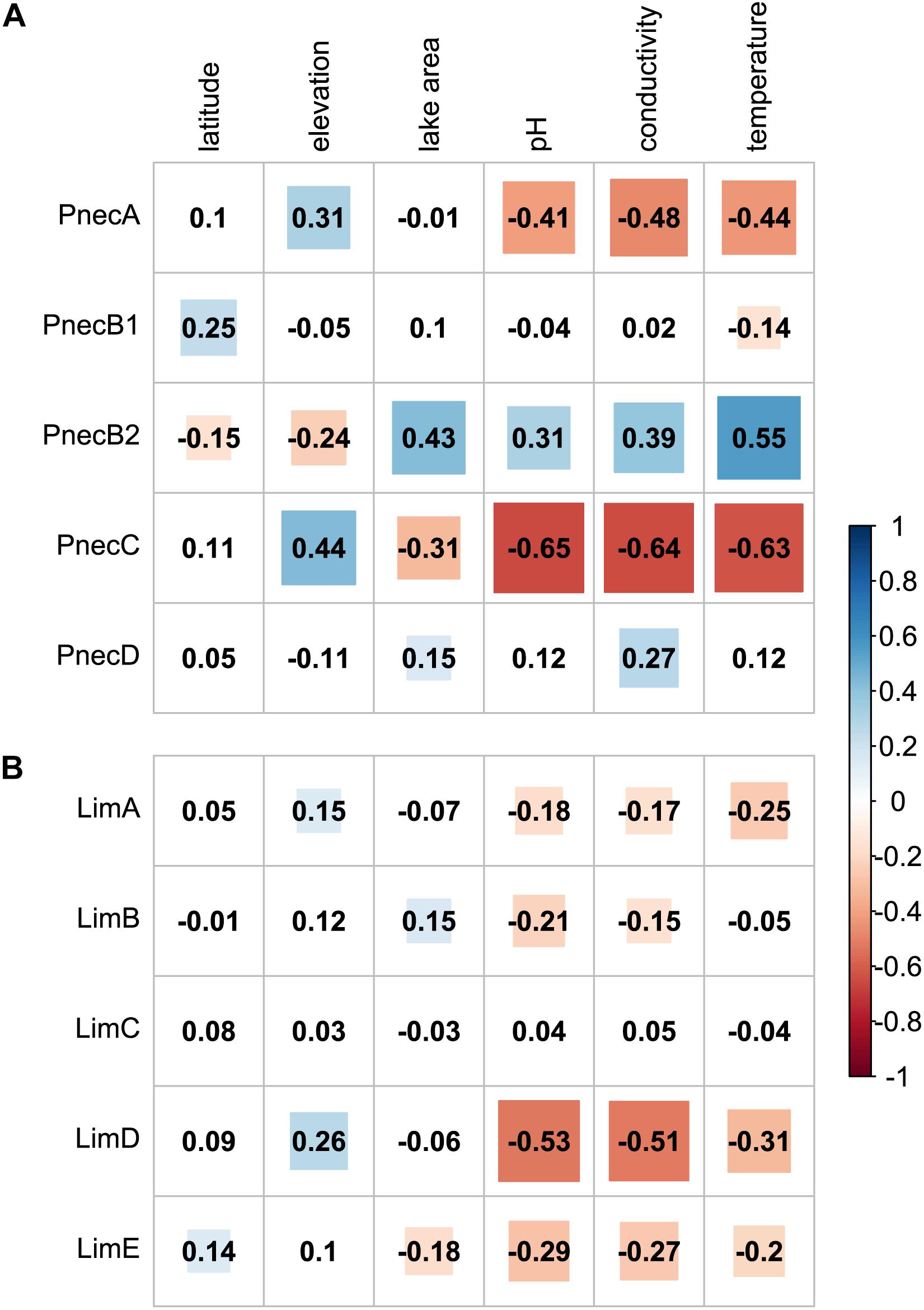
Figure 3. Spearman rank correlations of subcluster abundances with environmental parameters. Correlation coefficients for (A) Polynucleobacter and (B) Limnohabitans subclusters are provided for each correlation pair. Significant correlations (p < 0.05) are highlighted in blue for positive, and red for negative correlations.
The correlations of all OTUs with environmental parameters are presented in Supplementary Figure S5, which shows that not all OTUs associated to one subcluster exhibited coherent ecological signals. For example, LimB was negatively correlated with pH and conductivity, while OTU B_436 revealed positive correlations with these parameters. The abundance of Limnohabitans and Polynucleobacter OTUs as a function of pH, latitude and conductivity is shown in Figure 4. Only the thirteen most abundant OTUs per genus were included. The results for all OTUs are presented in Supplementary Figure S7. For Polynucleobacter, OTU C_77 shows the widest occurrence spectrum. It was merely absent in samples with pH > 10 and conductivity >1000 μS cm–1. The second PnecC OTU C_588 was detected in a narrower pH range (6–9) and seems to be preferentially abundant at the highest sampled latitudes (Southern Scandinavia). This geographic area, which comprises mostly acidic to circum-neutral lakes, was clearly dominated by the two PnecC OTUs in terms of read abundance.
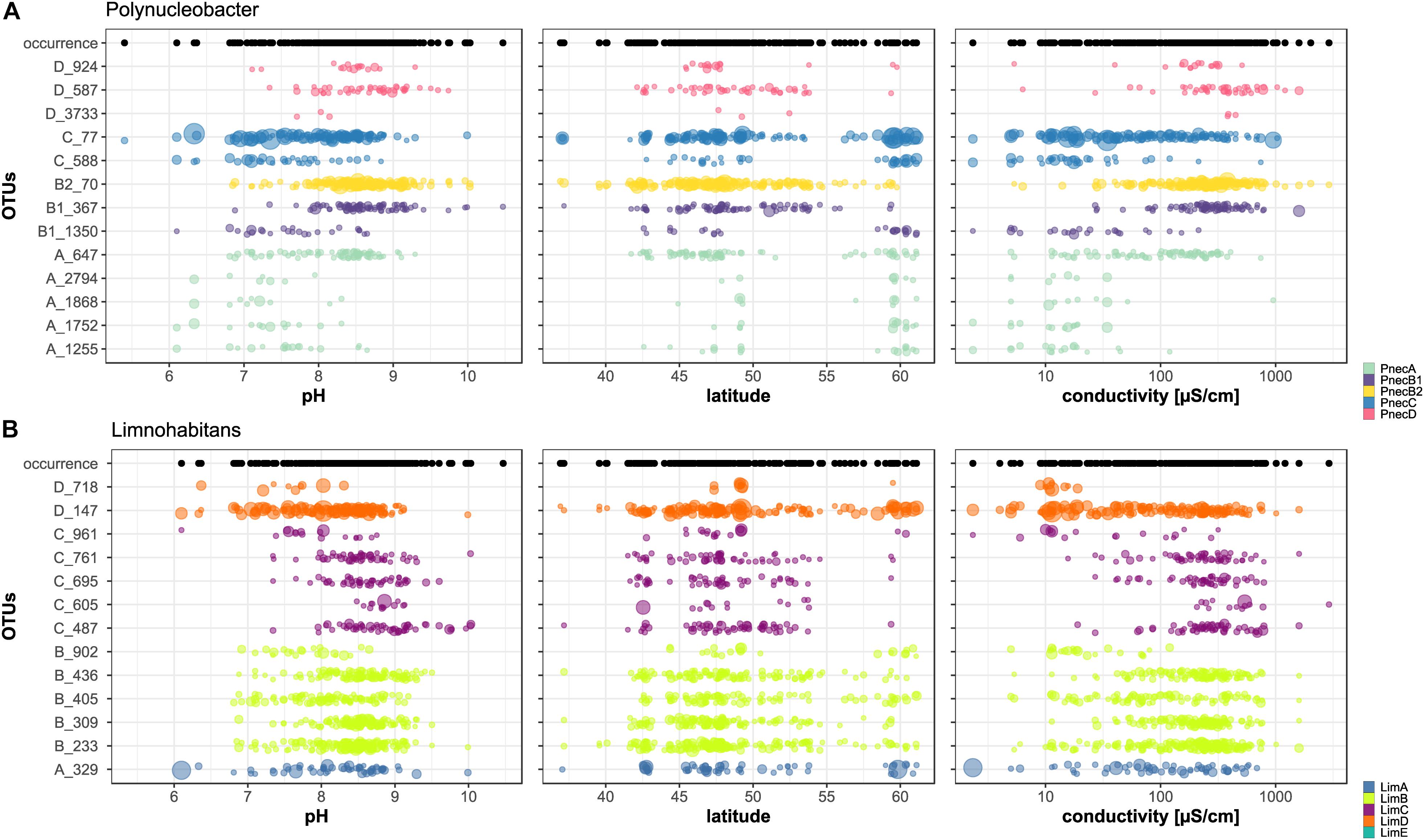
Figure 4. Abundances of OTUs across environmental gradients. Only the 13 most abundant OTUs assigned to (A) Polynucleobacter and (B) Limnohabitans are shown. The size of each dot is proportional to the OTU’s relative read abundance in the bacterial community. Black dots above each figure illustrate the occurrence of (A) Polynucleobacter and (B) Limnohabitans at the sampling sites.
The overall most abundant OTU B2_70 was the only Polynucleobacter OTU detected at the highest sampled conductivities (up to 2917 μS cm–1), but was restricted to pH > 6.8. Considering the geographic distribution of this OTU, it is conspicuous that it dominated many lakes in the Alps and its foothills. Generally, alpine lakes seem to be either dominated by OTU B2_70 [e.g., Lac de Annecy (Z163AC), Lac du Chevril (Z121CH), Kloentalersee (A281KL), Walensee (A272WA), Ammersee (A291AM), lake Wallersee (A102WA), lake Weissensee (A131WE), lake Erlaufsee (A042ER)] or C_77 [e.g., O271GA, Altausseer lake (A112AL), Obersee (A123OB), Morskie Oko (O041MO) and Popradske Pleso (O053PO)]. Exceptions are Rothsee (O261RO) and Plansee (A242PL), which contained a high relative abundance of PnecD reads (Supplementary Figure S8).
The two PnecB1 OTUs showed rather contrasting conductivity preferences, with B1_367 detection ranging from 27 to 1599 μS cm–1 and B1_1350 from 2 to 214 μS cm–1. PnecA and PnecD seem to consist of OTUs with coherent and more restricted habitat ranges than those of other subclusters. PnecA OTUs were preferentially detected at low conductivities, and those of PnecD at high conductivities. Interestingly, both PnecA and PnecD were not detected in Southern Europe (<42° latitude), yet, only 14 samples were analyzed from this region (Supplementary Figure S8).
Several Limnohabitans OTUs were not detected along the whole sampled pH gradient but restricted to relatively narrow pH ranges. For instance, no LimC OTU was detected at pH < 7.3, with only one exception (OTU C_961 at pH 6.1). The OTU C_605 revealed an exceptionally restricted pH range (8.4–9.2). Similarly, the distribution of LimB seems to be restricted by pH. All OTUs assigned to LimB showed a similar pH range, i.e., the subcluster was detected in only one sample with pH > 9.5, and not at all at pH < 6.8. On the contrary, LimA and LimD OTUs show a broader range toward low pH values, and were detected at a minimum pH of 6.1. LimD exhibited a high relative read abundance in the samples from Scandinavia. Conversely, LimB and LimC dominated the samples from Central and Eastern Europe (Supplementary Figure S8).
Operational Taxonomic Unit Diversity
Operational taxonomic unit richness, Shannon index (H), and Simpson index (SI) revealed overall similar correlations with environmental factors for the investigated samples. The OTU richness, H and SI of Polynucleobacter in the investigated samples was positively correlated with latitude and elevation, and negatively with pH, conductivity and temperature (Figure 5 and Supplementary Figure S9). The Evenness was positively correlated with latitude and negatively correlated with elevation and temperature. Surface area of lakes was not significantly correlated with OTU richness, H, SI and Evenness. In the sampled latitudinal gradient, the OTU richness, H and SI did not gradually increase but samples with high diversity were concentrated in two major regions (Figure 5 and Supplementary Figures S9, S10). The highest richness and diversity indices of Polynucleobacter communities were found in the northern area between 57° latitude and the northern edge of the entire sampling area at 63°comprising 17 sites. The second area with sites of high OTU richness and H were an alpine area between 45° and 49° latitude containing 11 sites (elevation: 427–1793 m). Exceptions from this pattern were two sampling sites in eastern Europe and two sites in the northern Alps also comprising high richness. The analysis of SI did not show high values in the alpine area. The Evenness of Polynucleobacter shows an inverted pattern compared to the richness and the Shannon index. The Evenness is low in the Scandinavian lakes and highest in the alpine region (Figure 5 and Supplementary Figure S10).
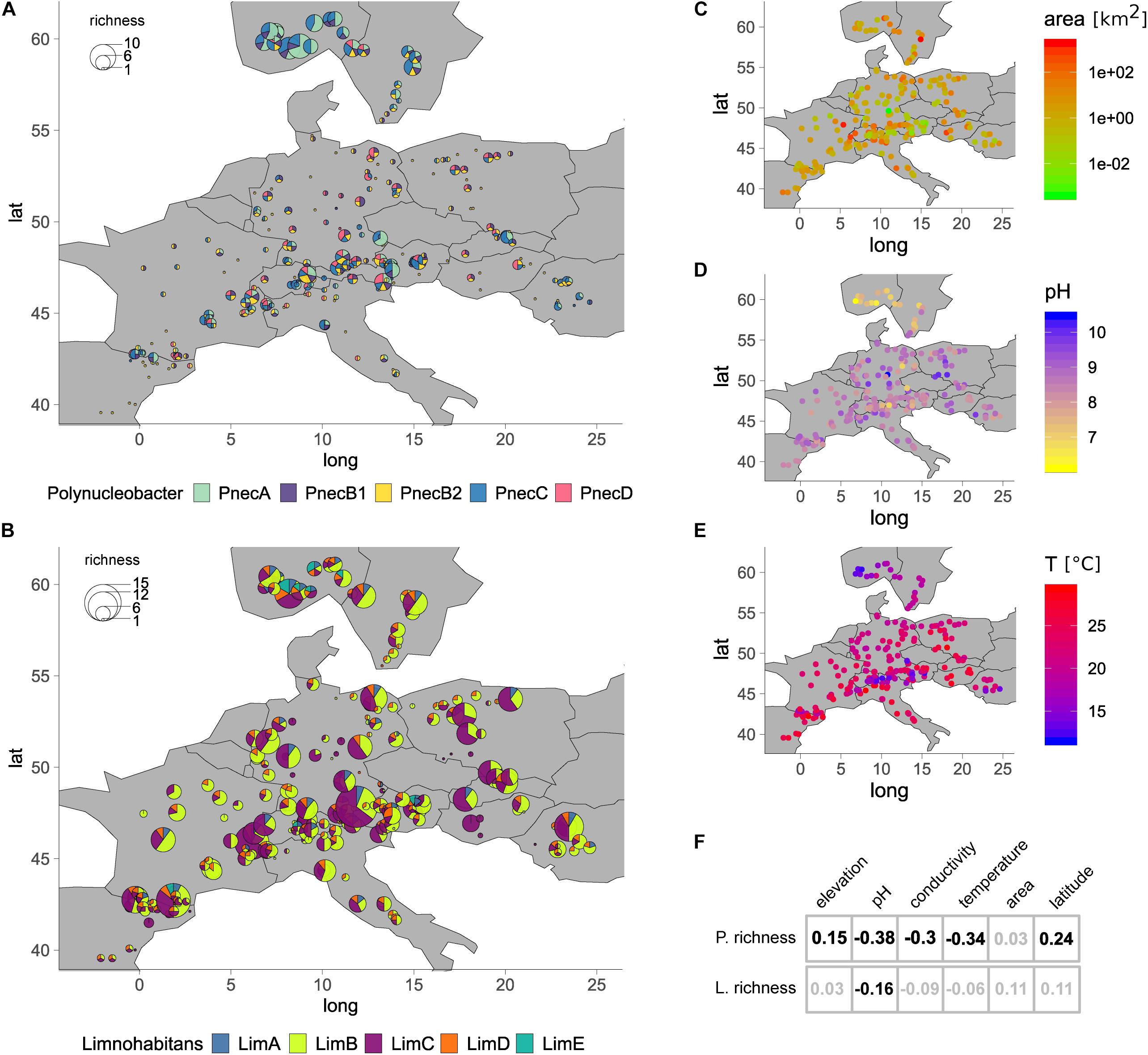
Figure 5. Geographic maps showing the OTU richness of each sample. The OTU richness of (A) Polynucleobacter and (B) Limnohabitans is represented by the size of each piechart. Piecharts are divided according to the number of OTUs associated to the different subclusters. The maps in panels (C–E) illustrate lake area, pH and temperature for each sample, respectively. The table in panel (F) shows Spearman rank correlation coefficients of environmental factors with OTU richness of Polynucleobacter and Limnohabitans. Significant correlations (p < 0.05) are shown in black, not significant correlations in gray font. Sites with higher Polynucleobacter OTU richness have lower temperature and acidic to circum-neutral pH.
The sites in the northern area were characterized by a high number of OTUs affiliated to PnecA. The lakes with highest richness were among the sites with lowest pH (6–7), conductivity and temperature (>20°C). The OTU richness of Limnohabitans was only negatively correlated with pH, and SI and H were positively correlated with lake area. The Evenness was positively correlated with pH and conductivity and negatively with elevation. OTU richness and diversity indices did not show an apparent geographical pattern (Figure 5 and Supplementary Figure S10).
Interactions of Bacteria and Microeukaryotes
The interaction network depicted in Figure 6 shows the partial correlations of eukaryotes with Polynucleobacter, and Limnohabitans subclusters. Partial correlations were calculated to exclude the co-variation effects of environmental factors (latitude, area, elevation, pH, temperature and conductivity) on protists and both genera. The network consists of eight nodes from Polynucleobacter and Limnohabitans, and sixteen nodes from eukaryotic groups. Out of 31 edges, 24 indicated a positive correlation, while 7 indicated negative correlations. PnecB2 and LimB have with seven and six connected edges to protists the most connecting edges among all tested subclusters. PnecB2 and Haptophyceae possessed with rk = 0.18, p = 1.47 × 10–4 the highest correlation coefficient within the Polynucleobacter genus (Supplementary Table S4). As revealed in Supplementary Figure S11, OTU B2_70 exhibits the most connections to protists among B2 OTUs and has the highest correlation coefficient with Haptophyeae [rk = 0.182, p = 1.45 × 10–4 (Supplementary Table S5)]. PnecB2 has connecting edges to Choanoflagellida, Cryptophyta, Haptophyta, Synurales, Euglenozoa, Ciliophora, and Katablepharidophyta. LimB and Synurales possessed with rk = 0.18, p = 1.28 × 10–4, the highest positive correlation coefficients within the Limnohabitans genus (Supplementary Table S4). The OTU network revealed that LimB was correlated with similar microeukaryotes compared to B2_70 with highest correlation coefficients between B_436 and Glaucocystophyceae (rk = 0.240, p = 1.11 × 10–6), and B_309 and Haptophyceae (rk = 0.212, p = 1.71 × 10–5). In general, LimB is connected through positive correlations with Chrysophyceae, Choanoflagellida, Cryptophyta, Haptophyta, Synurales, and Glaucocystophyceae (Figure 6).
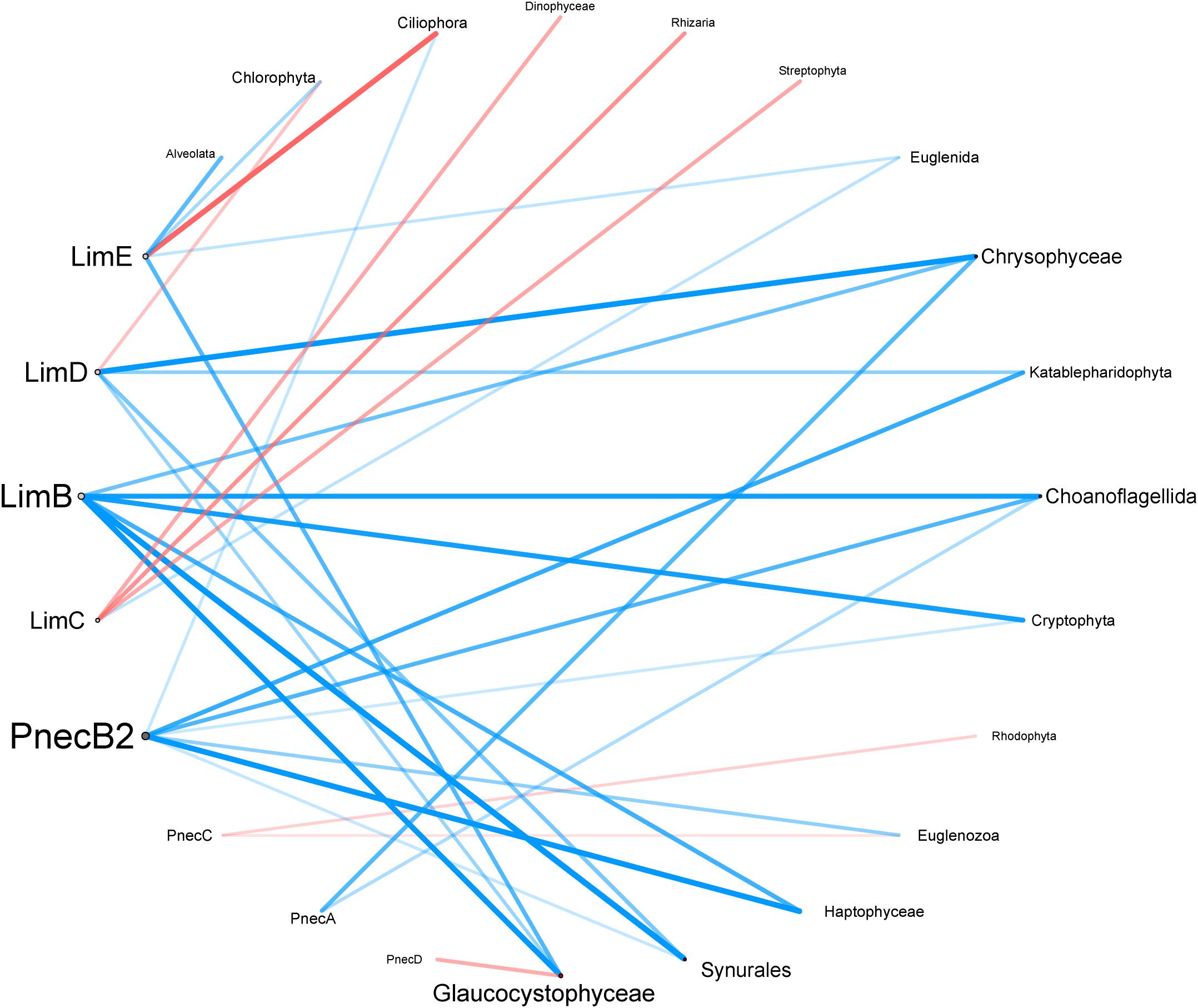
Figure 6. Correlation network of microeukaryotes with Polynucleobacter and Limnohabitans subclusters. Correlation coefficients are based on partial Kendall correlation analysis and represented by edge thickness. Positive and negative correlations are shown in blue and red, respectively. The number of edges connected to each node is visualized by the size of its name. Only significant correlations (p < 0.05) are shown.
LimC has three connecting edges that show negative correlations to Dinophyceae, Rhizaria, and Streptophyta and one positive correlation to Euglenida. LimC OTUs show overall the strongest negative correlations with protists, in particular with Katablepharidophyta and Haptophyceae (Supplementary Figure S11b and Supplementary Table S5). Further, groups of LimC OTUs positively correlated with similar protists. For example, C_4547, C_4847, C_1774, and C_3784 positively correlated with Ciliophora, and C_605, C_487, C_1609, C_695, C_1101, C_2561, and C_12575 with Euglenida. The strongest positive correlation is visible between Apusozoa and C_2985 with rk = 0.694, p = 614 × 10–45. PnecC and PnecA have two connecting edges each. While PnecA positively correlates with Chrysophyceae and Choanoflagellida, PnecC negatively correlates with Rhodophyta and Euglenozoa.
Among eukaryotes, Glaucocystophyceae (four edges) and Chrysophyceae, Choanoflagellida, and Synurales (three edges) have the most connecting edges to bacterial subclusters. Chlorophyta, Ciliophora, Euglenida, Katablepharidophyta, Cryptophyta, Euglenozoa, and Haptophyta have two connecting edges each.
Discussion
Here, we investigated for the first time the joint effects of physico-chemical factors, presence of microeukaryotes, and geography on two abundant freshwater taxa, Polynucleobacter and Limnohabitans. Our analyses clearly indicate physico-chemical factors having the strongest influence on subclusters and OTUs of both taxa (Table 1). In this context, we could corroborate known ecological preferences of subclusters, most notably with respect to pH and conductivity. In accordance with previous studies, abundances of PnecA (Newton et al., 2011), PnecC (Jezberová et al., 2010; Jezbera et al., 2011, 2012; Newton et al., 2011; Watanabe et al., 2012), LimA (LhabA3 in Newton et al., 2011; Shabarova et al., 2017), and LimD (Lhab-A2 in Newton et al., 2011) were negatively correlated with pH (Figure 3 and Supplementary Figure S6). We also found associations with low pH for LimB and LimE, which to our knowledge have not been analyzed as separate groups in previous cultivation-independent studies. Interestingly, most studies employing the R-BT FISH probe, which targets LimB, LimC, LimD, and LimE, reported positive relations with pH (Simek et al., 2010; Jezbera et al., 2012), while we did not find a significant positive correlation for any Limnnohabitans subcluster. Among Polynucleobacter, the only subcluster preferentially abundant at high pH was PnecB2, which conforms to earlier studies investigating the broader PnecB group that included PnecB1 and PnecB2 (Wu and Hahn, 2006a, b; Newton et al., 2011; Jezbera et al., 2012), Relations of subcluster abundances with conductivity were similar to those with pH, as a corrolary of the positive correlation between the two physico-chemical parameters (Supplementary Figure S12).
The assignment of OTUs beyond the subcluster level yet suggests intra-subcluster differentiation and diversity that has only been demonstrated for PnecC (Hahn et al., 2016) and LimC (Jezbera et al., 2013; Kasalický et al., 2013) before. We found that OTUs within subclusters showed partly contrasting preferences, and even single OTUs exhibited broad ecological preferences along environmental gradients (Figure 4). These results suggest that ecological diversification is not only limited to the PnecC subcluster, but pronounced in other subclusters as well. Geography had the second largest impact on Limnohabitans and Polynucleobacter community composition. While a noticeable geographic pattern for Polynucleobacter resulted from a pronounced PnecA richness in Scandinavian lakes, we did not observe a geographic pattern for Limnohabitans. The investigated taxa were least affected by the composition of microeukaryotes. The weakness of the signal is probably caused by partially opposing effects of complex interactions between bacteria and microeukaryotes.
Ecological Diversification
Among Polynucleobacter, PnecC is to date the best characterized subcluster considering diversity, ecophysiology, and genomic traits. However, it was demonstrated that the 16S rRNA gene does not reflect the diversity of PnecC yet rather conceals its taxon richness, which is revealed by extensive genomic differences of strains with similar 16S sequence (Hahn et al., 2016). The coarse resolution of the 16S rRNA gene and indications for a strong diversification in PnecC are also supported by our analyses on OTUs assigned to this subcluster. The phylogenetic analysis of the partial 16S rRNA sequences revealed that the OTU C_77 of the subcluster PnecC matches the sequences of two described PnecC species (Figure 1). Further, C_77 exhibited exceptionally broad ecological preferences, specifically in latitudinal distribution, conductivity, and pH (Figure 4). While a pan-European distribution was determined for Polynucleobacter paneuropeaus (Hoetzinger et al., 2019) that has a matching 16S rRNA gene sequence with C_77, the covered conductivity and pH ranges of C_77 likely exceed the niche this species might be adapted to. Six strains of P. paneuropeaus were isolated from habitats with conductivities and pH values ranging from 15–55 μS cm–1 and 4.6–7.5, respectively (Hoetzinger et al., 2019), which cover only a small part of the environmental range C_77 occured in. The type strain of Polynucleobacter hirudinilacicola, also comprised in the OTU C_77, was isolated from a pond with relatively high conductivity (353 μS cm–1) and a pH of 8.0 (Hahn et al., 2018). Possibly, this or other yet undescribed species may contribute to detections at higher conductivity and pH values of the OTU.
Although PnecC was considered an exception regarding ecological differentiation, we found indications for similar differentiation in two more Polynucleobacter subclusters. PnecB shows divergent ecological signals among the subclusters B2 and B1, and within B1 itself. Specifically, the subcluster B2 matched previously observed features of PnecB, i.e., positive correlations with pH and its absence from acidic habitats (Wu and Hahn, 2006b; Newton et al., 2011), while PnecB1 indicated a weak relation to physico-chemical factors except for latitude and temperature. The vague ecological signal of PnecB1 most probably results from OTUs assigned to this subcluster having different ecological preferences as indicated by different pH and conductivity ranges for the two assigned OTUs (Figure 4). While B1_367 preferentially occurs in lakes with alkaline pH and a measured conductivity between 100 and 1000 μS cm–1, B1_1350 occurred in circum-neutral lakes with low conductivity up to 200 μS cm–1. This observation suggests that subcluster B1 comprises at least two differentially adapted species. Thus, PnecB is composed of highly divergent taxa whose unique ecological adaptations are concealed by the coarse subcluster level.
Another example for ecological diversification was revealed in PnecA. Although PnecA was overall the rarest subcluster in the dataset, which corroborates estimations from previous studies (Hahn et al., 2011; Newton et al., 2011), it comprised significantly more OTUs than the other Polynucleobacter subclusters. These findings suggest that the PnecA subcluster comprises an exceptionally high 16S rRNA sequence diversity and significantly contributes to the Polynucleobacter diversity, in particular in Scandinavian samples (Figure 5). More sampling at these sites during different seasons and from more northern lakes would help to validate the high 16S rRNA sequence diversity of PnecA in this region. Despite the high number of PnecA OTUs, most of them exhibit similar occurrence patterns, suggesting a coherent ecology of most PnecA taxa compared to PnecC and PnecB. An exception was the most abundant OTU (A_647) occurring at a broader ecological range (Figure 4). Interestingly, this OTU shares sequence identity with the type strain of the only so far described PnecA species, Polynucleobacter rarus (Hahn et al., 2011), and was most abundant in lakes with alkaline pH between 8 and 9. In contrast, the type strain of P. rarus was isolated from the acidic Crystal Bog Lake (pH ∼ 4.5) located in North America (Newton et al., 2006). This may indicate ecological diversification within OTU A_647, similarly as described above for OTU C_77. Hence, ecologically distinct species with similar 16S rRNA might be also present in other Polynucleobacter subclusters than PnecC.
Limnohabitans has been shown to comprise bacteria with larger genomes (Kasalický et al., 2018), bigger cell sizes (Kasalický et al., 2013), and presumably higher overall growth rates (Simek et al., 2006) as compared to Polynucleobacter (Hahn et al., 2017). Thus, a more opportunistic lifestyle has been proposed for Limnohabitans bacteria, which might also be reflected in more generalist adaptations (Kasalický et al., 2013). Nevertheless, extensive phenotypic and ecological diversification has been demonstrated within the subcluster LimC as well (Jezbera et al., 2013; Kasalický et al., 2013), which might be comparable to the diversification within PnecC discussed above. A difference to Polynucleobacter is a higher diversity of Limnohabitans on 16S rRNA sequence level, as evident from 51 OTUs assigned to Limnohabitans subclusters opposed to 25 Polynucleobacter OTUs (Figures 1, 2). Most OTUs were assigned to LimC (27 OTUs) and LimB (14 OTUs). Within LimC, for instance OTUs C_605 and C_961 show opposing preferences regarding pH and conductivity. The former is more abundant at alkaline pH (8.5–9.2) and high conductivities, while the latter was preferentially detected at lower pH (7.5–8.1) and low conductivities.
Also within certain Limnohabitans OTUs, broad environmental preferences may indicate cryptic diversity rather than generalist adaptations. For example, OTU A_329 was abundant in the most acidic lake sampled (Lake Černé Jezero, pH 5.4), and rare or absent in circum-neutral lakes, but showed relatively high abundances in lakes with pH > 7.5. Such a distribution pattern would be unlikely for a single generalist phenotype.
Especially interesting is the most abundant Limnohabitans OTU D_147, identical in partial 16S sequence with Uncultured clone PIB-25 (Figure 2). This OTU shows surprisingly similar distribution patterns to the Polynucleobacter OTU C_77, which comprises at least two different species (see above). Unfortunately, no isolates affiliated to LimD are available to date, and it can thus only be speculated if this subcluster comprises a species cluster comparable to PnecC. In summary, our study indicates extensive ecological diversification within all Polynucleobacter and Limnohabitans subclusters. Cultivation as well as cultivation-independent studies with sequences providing higher phylogenetic resolution would be necessary to better characterize this diversification.
Diversity and Geography
The magnitude and large geographic range of sampling sites allowed us to investigate Polynucleobacter and Limnohabitans diversity, i.e., OTU richness and diversity indices, in light of biogeography. In this context, we focused on the latitudinal diversity gradient and the species-area relationship. Briefly, the latitudinal diversity gradient suggests that species richness declines from the tropics to the poles (Hillebrand, 2004). The species-area relationship assumes a positive correlation between ecosystem size and observed number of species (MacArthur and Wilson, 1967). While Reche et al. (2005) found a positive species-area relationship for bacterial communities using 16S rRNA OTUs as diversity unit, we observed no significant correlation of lake area with OTU richness in our study, neither for Polynucleobacter nor for Limnohabitans (Figure 5 and Supplementary Figures S9, S10). Yet, Shannon and Simpson index of Limnohabitans showed a weak positive correlation with lake area (Supplementary Figure S9). Regarding the latitudinal diversity gradient hypothesis, Limnohabitans diversity indices did not significantly correlate with temperature and latitude. However, Polynucleobacter diversity indices were negatively correlated with temperature and positively with latitude. This contradicts the assumptions of the latitudinal diversity gradient hypothesis. However, diversity did not increase gradually with latitude but the positive correlation was mainly caused by a particularly high diversity in the most northern region of our sampling area (Figure 5).
We suppose two underlying causes for this geographic pattern. Firstly, the regional characteristics in the sampled Scandinavian area may promote Polynucleobacter abundance (Supplementary Figure S8), and consequently its diversity. Logue and Lindström (2008) state the importance of regional factors for the diversity and biogeography of bacterioplankton in inland waters. In their concept, lakes are regarded as parts of larger units (i.e., their drainage areas), and thus the region the lakes are located in affects their local physico-chemical characteristics (e.g., pH). As these characteristics in turn affect the local bacterial communities, they are referred to as indirect regional effects on community composition. In addition, the import of bacteria from the regional metacommunity poses direct effects on the local communities (Logue and Lindström, 2008). The importance of such dispersal effects is exemplified by the island biogeography theory (Connor and McCoy, 1979), if lakes are viewed as islands within a terrestrial landscape (Dodson, 1992). Due to a high density and connectivity of lakes in Scandinavian areas, both indirect and direct regional influences might be particularly important for resident bacterial communities. Accordingly, Logares et al. (2013) observed similar physico-chemical characteristics for different lakes from a region in Scandinavia, and estimated high dispersal among these lakes. The Scandinavian lakes in our study are also characterized by similar environmental features, i.e., low pH and temperature (Supplementary Table S1), which favor the abundance of PnecC and PnecA (Figure 3 and Supplementary Figures S5, S8). Large population sizes and high dispersal rates may consequently account for high Polynucleobacter diversities within single lakes.
A second factor accounting for the high OTU richness observed in this area is the specifically high contribution of PnecA to the regional metacommunity. PnecA is characterized by a high 16S rRNA gene diversity compared to the other Polynucleobacter subclusters (Figure 1). Thus, the OTU richness in this region may be disproportionally high due to the used marker and does not necessarily represent an equally higher species richness.
Although we could not find a latitudinal gradient and no clear species-area relationship by analyzing 16S rRNA gene amplicons, we cannot exclude that such patterns would become evident when using phylogenetic markers with higher resolution. The 16S rRNA gene in this respect suffers from limitations to resolve species diversity, as discussed above. According to calibrated molecular clocks from endosymbiotic bacteria, the maximum evolutionary rate of the 16S rRNA gene is 0.091% sequence divergence per million year (Kuo and Ochman, 2009). This suggests that possible patterns caused by recent temporal events (e.g., the last glacial period c. 115 000 – c. 11 700 years ago) cannot be detected with this marker.
Microbial Interactions of Microeukaryotes and Both Taxa
The effect of eukaryotes on the composition of Polynucleobacter and Limnohabitans was the lowest in comparison to geography and physico-chemical factors (Table 1). The overall low correlation coefficients might suggest varying trophic interactions of eukaryotes and bacteria among sites (Figure 6). Remarkably, Limnohabitans subcluster show an overall stronger interconnection in the network compared to Polynucleobacter suggesting members of Limnohabitans being highly interactive. In this respect, LimB seems to play a major role in the network. For example, LimB shows a comparably strong connection to the groups of eukaryotic flagellates Cryptophyta, Choanoflagellida, and Chrysophyta. These flagellates increased in relative abundance in grazing experiments with LimB (Grujcic et al., 2018). Interconnections with these and other eukaryotes may also harbor different qualities of interactions besides predator-prey relationships, such as utilization of eukaryotic exudates by bacteria (Simek et al., 2011; Horňák et al., 2017). For PnecB, which shows the strongest interconnection of the Polynucleobacter subclusters in our network, it was demonstrated that they grew on organic matter derived by chlorophyte taxa, i.e., Coelastrum reticulatum (Horňák et al., 2017). Our results do not show a connection of PnecB2 and Chlorophyta but with other eukaryotic groups that comprise exudate releasing taxa. It is possible that PnecB2 species specifically profit from exudates of other, so far not tested eukaryotes. Additionally, seasonal effects and grazing pressure by zooplankton, as demonstrated by Jezberová et al. (2017), affect the abundance of microeukaryotes and therefore the correlations with bacteria. Summarized, the analyzed co-occurrences might indicate direct interrelations, however, we cannot exclude the presence of unobserved interactions. For instance, interactions among bacteria and eukaryotes with opposing effects on bacterial abundance, i.e., grazing and exudate utilization, may mitigate signals of interrelation. Diverging exudate preferences of different taxa within bacterial subclusters may also contribute to the weakening of correlations.
Conclusion
Our study emphasizes the importance of physico-chemical factors on the distribution of bacterial taxa affiliated to Polynucleobacter and Limnohabitans in comparison to composition of microeukaryotes and geographical ecosystem properties. Along those physico-chemical gradients, we could demonstrate that the ecological diversification within PnecC and LimC is not an exception within the genera Polynucleobacter and Limnohabitans, but likely applicable for the other subclusters as well. We identified geographic patterns in OTU richness and abundance for both taxa that indicate the importance of region-specific environmental conditions for local community composition. A relationship between area or latitudinal gradient and taxon richness could not be identified, however, due to the limited phylogenetic resolution of the 16SrRNA gene we cannot exclude the presence of such relationships. Finally, observed co-occurrence patterns between bacterial and microeukaryotic taxa provide a basis for future research to test for specific bacteria-protist interactions in freshwater ecosystems.
Data Availability Statement
The datasets generated for this study can be found in the NCBI Sequence Read Archive BioProjects PRJNA559862 and PRJNA414052.
Author Contributions
All authors contributed to the study design, read, and approved the final version of the manuscript. JN analyzed the sequencing data and performed the statistical analyses. MH conducted the phylogenetic analyses. JN and MH wrote the manuscript. JB, MWH, and DB contributed to the final stages of writing.
Funding
We thank the German Research Foundation (DFG) for the funding of the projects BO 3245/19 and BO3245/17. Further, we thank the team for publication services of the library of the University of Duisburg – Essen for providing funds for the open access publication fee.
Conflict of Interest
The authors declare that the research was conducted in the absence of any commercial or financial relationships that could be construed as a potential conflict of interest.
Acknowledgments
We thank Manfred Jensen for helping with the procession of raw reads. We are also thankful to the entire Department of Biodiversity for their effort in sampling and sample extraction. Finally, we thank the reviewers for reviewing the manuscript.
Supplementary Material
The Supplementary Material for this article can be found online at: https://www.frontiersin.org/articles/10.3389/fmicb.2020.00154/full#supplementary-material
Footnotes
References
Andrews, S. (2018). FastQC: A Quality Control Tool for High Throughput Sequence Data. Available at http://www.bioinformatics.babraham.ac.uk/projects/fastqc (accessed October 04, 2018).
Blanchet, F. G., Legendre, P., and Borcard, D. (2008). Forward selection of explanatory variables. Ecology 89, 2623–2632. doi: 10.1890/07-0986.1
Boenigk, J., Wodniok, S., Bock, C., Beisser, D., Hempel, C., Grossmann, L., et al. (2018). Geographic distance and mountain ranges structure freshwater protist communities on a European scalå. Metabarcoding and Metagenom. 2:e21519. doi: 10.3897/mbmg.2.21519
Clark, K., Karsch-Mizrachi, I., Lipman, D. J., Ostell, J., and Sayers, E. W. (2016). GenBank. Nucleic Acids Res. 44, D67–D72. doi: 10.1093/nar/gkv1276
Connor, E. F., and McCoy, E. D. (1979). The statistics and biology of the species-area relationship. Am. Nat. 113, 791–833. doi: 10.1086/283438
Crump, R. C., Adams, H. E., Hobbie, J. E., and Kling, G. W. (2007). Biogeography of bacterioplankton in lakes and streams of an Arctic tundra catchment. Ecology 88, 1365–1378. doi: 10.1890/06-0387
Dodson, S. (1992). Predicting crustacean zooplankton species richness. Limnol. Oceanogr. 37, 848–856. doi: 10.4319/lo.1992.37.4.0848
Edgar, R. C. (2004). MUSCLE: multiple sequence alignment with high accuracy and high throughput. Nucleic Acids Res. 32, 1792–1797. doi: 10.1093/nar/gkh340
Edgar, R. C., Haas, B. J., Clemente, J. C., Quince, C., and Knight, R. (2011). UCHIME improves sensitivity and speed of chimera detection. Bioinformatics 27, 2194–2200. doi: 10.1093/bioinformatics/btr381
Fierer, N., and Jackson, R. B. (2006). The diversity and biogeography of soil bacterial communities. Proc. Natl. Acad. Sci. U.S.A. 103, 626–631. doi: 10.1073/pnas.0507535103
Grujcic, V., Nuy, J. K., Salcher, M. M., Shabarova, T., Kasalicky, V., Boenigk, J., et al. (2018). Cryptophyta as major bacterivores in freshwater summer plankton. ISME J. 12, 1668–1681. doi: 10.1038/s41396-018-0057-5
Hahn, M. W. (2003). Isolation of strains belonging to the cosmopolitan Polynucleobacter necessarius cluster from freshwater habitats located in three climatic zones. Appl. Environ. Microbiol. 69, 5248–5254. doi: 10.1128/aem.69.9.5248-5254.2003
Hahn, M. W., Jezberová, J., Koll, U., Saueressig-Beck, T., and Schmidt, J. (2016). Complete ecological isolation and cryptic diversity in Polynucleobacter bacteria not resolved by 16S rRNA gene sequences. ISME J. 10, 1642–1655. doi: 10.1038/ismej.2015.237
Hahn, M. W., Koll, U., Jezberová, J., and Camacho, A. (2015). Global phylogeography of pelagic Polynucleobacter bacteria: restricted geographic distribution of subgroups, isolation by distance and influence of climate. Environ. Microbiol. 17, 829–840. doi: 10.1111/1462-2920.12532
Hahn, M. W., Koll, U., Schmidt, J., Huymann, L. R., Karbon, G., and Lang, E. (2018). Polynucleobacter hirudinilacicola sp. nov. and Polynucleobacter campilacus sp. nov., both isolated from freshwater systems. Int. J. Syst. Evol. Microbiol. 68, 2593–2601. doi: 10.1099/ijsem.0.002880
Hahn, M. W., Lang, E., Tarao, M., and Brandt, U. (2011). Polynucleobacter rarus sp. nov., a free-living planktonic bacterium isolated from an acidic lake. Int. J. Syst. Evol. Microbiol. 61(Pt 4), 781–787. doi: 10.1099/ijs.0.017350-0
Hahn, M. W., Schmidt, J., Asiyo, G. S., Kyrpides, N. C., Woyke, T., and Whitman, W. B. (2017). Reclassification of a Polynucleobacter cosmopolitanus strain isolated from tropical Lake Victoria as Polynucleobacter victoriensis sp. nov. Int. J. Syst. Evol. Microbiol. 67, 5087–5093. doi: 10.1099/ijsem.0.002421
Hillebrand, H. (2004). On the generality of the latitudinal diversity gradient. Am. Nat. 163, 192–211. doi: 10.1086/381004
Hoetzinger, M., Schmidt, J., Pitt, A., Koll, U., Lang, E., and Hahn, M. W. (2019). Polynucleobacter paneuropaeus sp. nov., characterized by six strains isolated from freshwater lakes located along a 3000 km north-south cross-section across Europe. Int. J. Syst. Evol. Microbiol. 69, 203–213. doi: 10.1099/ijsem.0.003130
Horňák, K., Kasalický, V., Šimek, K., and Grossart, H.-P. (2017). Strain-specific consumption and transformation of alga-derived dissolved organic matter by members of the Limnohabitans-C and Polynucleobacter-B clusters of Betaproteobacteria. Environ. Microbiol. 19, 4519–4535. doi: 10.1111/1462-2920.13900
Horner-Devine, M. C., Lage, M., Hughes, J. B., and Bohannan, B. J. M. (2004). A taxa-area relationship for bacteria. Nature 432, 750–753. doi: 10.1038/nature03073
Janssen, S., McDonald, D., Gonzalez, A., Navas-Molina, J. A., Jiang, L., Xu, Z. Z., et al. (2018). Phylogenetic placement of exact Amplicon sequences improves associations with clinical information. mSystems 3:e00021-18. doi: 10.1128/mSystems.00021-18
Jezbera, J., Jezberová, J., Brandt, U., and Hahn, M. W. (2011). Ubiquity of Polynucleobacter necessarius subspecies asymbioticus results from ecological diversification. Environ. Microbiol. 13, 922–931. doi: 10.1111/j.1462-2920.2010.02396.x
Jezbera, J., Jezberová, J., Kasalický, V., Šimek, K., and Hahn, M. W. (2013). Patterns of Limnohabitans microdiversity across a large set of freshwater habitats as revealed by reverse line blot hybridization. PloS One 8:e58527. doi: 10.1371/journal.pone.0058527
Jezbera, J., Jezberová, J., Koll, U., Horňák, K., Šimek, K., and Hahn, M. W. (2012). Contrasting trends in distribution of four major planktonic betaproteobacterial groups along a pH gradient of epilimnia of 72 freshwater habitats. FEMS Microbiol. Ecol. 81, 467–479. doi: 10.1111/j.1574-6941.2012.01372.x
Jezberová, J., Jezbera, J., Brandt, U., Lindström, E. S., Langenheder, S., and Hahn, M. W. (2010). Ubiquity of Polynucleobacter necessarius ssp. asymbioticus in lentic freshwater habitats of a heterogeneous 2000 km area. Environ. Microbiol. 12, 658–669. doi: 10.1111/j.1462-2920.2009.02106.x
Jezberová, J., Jezbera, J., Znachor, P., Nedoma, J., Kasalický, V., and Šimek, K. (2017). The Limnohabitans genus harbors generalistic and opportunistic subtypes: evidence from spatiotemporal succession in a canyon-shaped reservoir. Appl. Environ. Microbiol. 83:e01530-17. doi: 10.1128/AEM.01530-17
Kasalický, V., Jezbera, J., Hahn, M. W., and Šimek, K. (2013). The diversity of the Limnohabitans genus, an important group of freshwater bacterioplankton, by characterization of 35 isolated strains. PloS One 8:e58209. doi: 10.1371/journal.pone.0058209
Kasalický, V., Zeng, Y., Piwosz, K., Šimek, K., Kratochvilová, H., and Koblížek, M. (2018). Aerobic anoxygenic photosynthesis is commonly present within the genus Limnohabitans. Appl. Environ. Microbiol. 84:e02116-17. doi: 10.1128/AEM.02116-17
Kumar, S., Stecher, G., Li, M., Knyaz, C., and Tamura, K. (2018). MEGA X: molecular evolutionary genetics analysis across computing platforms. Mol. Biol. Evol. 35, 1547–1549. doi: 10.1093/molbev/msy096
Kuo, C.-H., and Ochman, H. (2009). Inferring clocks when lacking rocks: the variable rates of molecular evolution in bacteria. Biol. Direct 4:35. doi: 10.1186/1745-6150-4-35
Lange, A., Jost, S., Heider, D., Bock, C., Budeus, B., Schilling, E., et al. (2015). AmpliconDuo: a split-sample filtering protocol for high-throughput amplicon sequencing of microbial communities. PloS One 10:e0141590. doi: 10.1371/journal.pone.0141590
Lindström, E. S., Kamst-Van Agterveld, M. P., and Zwart, G. (2005). Distribution of typical freshwater bacterial groups is associated with pH, temperature, and lake water retention time. Appl. Environ. Microbiol. 71, 8201–8206. doi: 10.1128/AEM.71.12.8201-8206.2005
Logares, R., Lindström, E. S., Langenheder, S., Logue, J. B., Paterson, H., Laybourn-Parry, J., et al. (2013). Biogeography of bacterial communities exposed to progressive long-term environmental change. ISME J. 7, 937–948. doi: 10.1038/ismej.2012.168
Logue, J. B., and Lindström, E. S. (2008). Biogeography of bacterioplankton in inland waters. Freshw. Rev. 1, 99–114. doi: 10.1608/FRJ-1.1.9
MacArthur, R. H., and Wilson, E. O. (1967). The Theory of Islandbiogeography. Princeton, NJ: Princeton University Press.
Mahé, F., Rognes, T., Quince, C., de Vargas, C., and Dunthorn, M. (2014). Swarm: robust and fast clustering method for amplicon-based studies. PeerJ 2:e593. doi: 10.7717/peerj.593
Masella, A. P., Bartram, A. K., Truszkowski, J. M., Brown, D. G., and Neufeld, J. D. (2012). PANDAseq: paired-end assembler for illumina sequences. BMC Bioinformatics 13:31. doi: 10.1186/1471-2105-13-31
Moran, M. A., and Hodson, R. E. (1990). Bacterial production on humic and nonhumic components of dissolved organic carbon. Limnol. Oceanogr. 35, 1744–1756. doi: 10.4319/lo.1990.35.8.1744
Newton, R. J., Jones, S. E., Eiler, A., McMahon, K. D., and Bertilsson, S. (2011). A guide to the natural history of freshwater lake bacteria. Microbiol. Mol. Biol. Rev. 75, 14–49. doi: 10.1128/MMBR.00028-10
Newton, R. J., Kent, A. D., Triplett, E. W., and McMahon, K. D. (2006). Microbial community dynamics in a humic lake: differential persistence of common freshwater phylotypes. Environ. Microbiol. 8, 956–970. doi: 10.1111/j.1462-2920.2005.00979.x
R Core Team (2018). R: A Language and Environment for Statistical Computing. Available at https://www.R-project.org/ (accessed December 20, 2018).
Reche, I., Pulido-Villena, E., Morales-Baquero, R., and Casamayor, E. O. (2005). Does ecosystem size determine aquatic bacterial richness? Ecology 86, 1715–1722. doi: 10.1890/04-1587
Schiaffino, R. M., Gasol, J. M., Izaguirre, I., and Unrein, F. (2013). Picoplankton abundance and cytometric group diversity along a trophic and latitudinal lake gradient. Aquat. Microb. Ecol. 68, 231–250. doi: 10.3354/ame01612
Schmieder, R., and Edwards, R. (2011). Quality control and preprocessing of metagenomic datasets. Bioinformatics 27, 863–864. doi: 10.1093/bioinformatics/btr026
Schneider, C. A., Rasband, W. S., and Eliceiri, K. W. (2012). NIH Image to imagej: 25 years of image analysis. Nat. Methods 9, 671–675. doi: 10.1038/nmeth.2089
Shabarova, T., Kasalický, V., Šimek, K., Nedoma, J., Znachor, P., Posch, T., et al. (2017). Distribution and ecological preferences of the freshwater lineage LimA (genus Limnohabitans) revealed by a new double hybridization approach. Environ. Microbiol. 19, 1296–1309. doi: 10.1111/1462-2920.13663
Shannon, P., Markiel, A., Ozier, O., Baliga, N. S., Wang, J. T., Ramage, D., et al. (2003). Cytoscape: a software environment for integrated models of biomolecular interaction networks. Genome Res. 13, 2498–2504. doi: 10.1101/gr.1239303
Simek, K., Hornák, K., Jezbera, J., Nedoma, J., Vrba, J., Straskrábová, V., et al. (2006). Maximum growth rates and possible life strategies of different bacterioplankton groups in relation to phosphorus availability in a freshwater reservoir. Environ. Microbiol. 8, 1613–1624. doi: 10.1111/j.1462-2920.2006.01053.x
Šimek, K., Kasalický, V., Jezbera, J., Horňák, K., Nedoma, J., Hahn, M. W., et al. (2013). Differential freshwater flagellate community response to bacterial food quality with a focus on Limnohabitans bacteria. ISME J. 7, 1519–1530. doi: 10.1038/ismej.2013.57
Simek, K., Kasalicky, V., Jezbera, J., Jezberová, J., Hejzlar, J., and Hahn, M. W. (2010). Broad habitat range of the phylogenetically narrow R-BT065 cluster, representing a core group of the Betaproteobacterial genus Limnohabitans. Appl. Environ. Microbiol. 76, 631–639. doi: 10.1128/AEM.02203-09
Simek, K., Kasalický, V., Zapomelová, E., and Hornák, K. (2011). Alga-derived substrates select for distinct Betaproteobacterial lineages and contribute to niche separation in Limnohabitans strains. Appl. Environ. Microbiol. 77, 7307–7315. doi: 10.1128/AEM.05107-11
Simek, K., Pernthaler, J., Weinbauer, M. G., Hornák, K., Dolan, J. R., Nedoma, J., et al. (2001). Changes in bacterial community composition and dynamics and viral mortality rates associated with enhanced flagellate grazing in a mesoeutrophic reservoir. Appl. Environ. Microbiol. 67, 2723–2733. doi: 10.1128/AEM.67.6.2723-2733.2001
South, A. (2011). rworldmap: a new R package for mapping global data. R J. 3, 35–43. doi: 10.32614/RJ-2011-006
Stamatakis, A. (2015). Using RAxML to infer phylogenies. Curr. Protoc. Bioinformatics 51, 6.14.1–6.14.14.
Watanabe, K., Komatsu, N., Kitamura, T., Ishii, Y., Park, H.-D., Miyata, R., et al. (2012). Ecological niche separation in the Polynucleobacter subclusters linked to quality of dissolved organic matter: a demonstration using a high sensitivity cultivation-based approach. Environ. Microbiol. 14, 2511–2525. doi: 10.1111/j.1462-2920.2012.02815.x
Wei, T., and Simko, V. (2017). R Package “corrplot”: Visualization of a Correlation Matrix (Version 0.84).
Wickham, H. (2011). ggplot2. Wiley Interdiscip. Rev. Comput. Stat. 3, 180–185. doi: 10.1002/wics.147
Wu, Q. L., and Hahn, M. W. (2006a). Differences in structure and dynamics of Polynucleobacter communities in a temperate and a subtropical lake, revealed at three phylogenetic levels. FEMS Microbiol. Ecol. 57, 67–79. doi: 10.1111/j.1574-6941.2006.00105.x
Keywords: freshwater bacteria, Polynucleobacter, Limnohabitans, biogeography, ecological differentiation, physicochemical characteristics, microbial eukaryotes, 16S
Citation: Nuy JK, Hoetzinger M, Hahn MW, Beisser D and Boenigk J (2020) Ecological Differentiation in Two Major Freshwater Bacterial Taxa Along Environmental Gradients. Front. Microbiol. 11:154. doi: 10.3389/fmicb.2020.00154
Received: 04 November 2019; Accepted: 22 January 2020;
Published: 13 February 2020.
Edited by:
Télesphore Sime-Ngando, Centre National de la Recherche Scientifique (CNRS), FranceReviewed by:
Karel Hornak, University of Zurich, SwitzerlandVojtech Kasalicky, Academy of Sciences of the Czech Republic, Czechia
Copyright © 2020 Nuy, Hoetzinger, Hahn, Beisser and Boenigk. This is an open-access article distributed under the terms of the Creative Commons Attribution License (CC BY). The use, distribution or reproduction in other forums is permitted, provided the original author(s) and the copyright owner(s) are credited and that the original publication in this journal is cited, in accordance with accepted academic practice. No use, distribution or reproduction is permitted which does not comply with these terms.
*Correspondence: Julia K. Nuy, Julia.nuy@uni-due.de