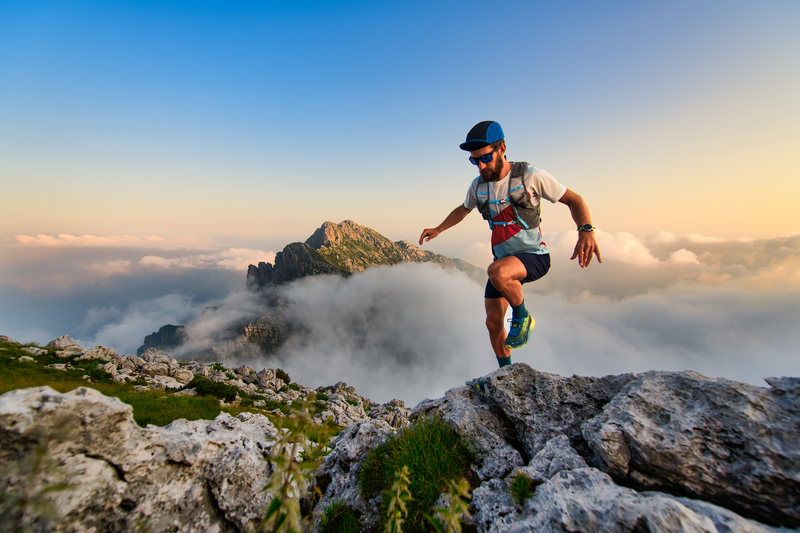
95% of researchers rate our articles as excellent or good
Learn more about the work of our research integrity team to safeguard the quality of each article we publish.
Find out more
ORIGINAL RESEARCH article
Front. Microbiol. , 14 January 2020
Sec. Microbial Physiology and Metabolism
Volume 10 - 2019 | https://doi.org/10.3389/fmicb.2019.02906
DNA damage response allows microorganisms to repair or bypass DNA damage and maintain the genome integrity. It has attracted increasing attention but the underlying influential factors affecting DNA damage response are still unclear. In this work, isobaric tags for relative and absolute quantification (iTRAQ)-based proteomic analysis was used to investigate the influence of carbon sources on the translational response of Acinetobacter baylyi ADP1 to DNA damage. After cultivating in a nutrient-rich medium (LB) and defined media supplemented with four different carbon sources (acetate, citrate, pyruvate, and succinate), a total of 2807 proteins were identified. Among them, 84 proteins involved in stress response were significantly altered, indicating the strong influence of carbon source on the response of A. baylyi ADP1 to DNA damage and other stresses. As the first study on the comparative global proteomic changes in A. baylyi ADP1 under DNA damage across nutritional environments, our findings revealed that DNA damage response in A. baylyi ADP1 at the translational level is significantly altered by carbon source, providing an insight into the complex protein interactions across carbon sources and offering theoretical clues for further study to elucidate their general regulatory mechanism to adapt to different nutrient environments.
Acinetobacter baylyi ADP1 is a well-established soil model microorganism for gene manipulation for its natural transformation ability. Its DNA damage response mechanism is not well-understood and remains unclear. A gel-free quantitative proteomic analysis (iTRAQ combined with LC/MS/MS) in this study explored the proteomic profiling of A. baylyi ADP1 with mitomycin C-induced DNA damage and cultivated with different carbon sources. This work illustrates a comprehensive picture of the dynamic changes in proteomics of A. baylyi ADP1 response to DNA damage stress, providing a deeper and broader understanding of the carbon source-dependent DNA damage response. Proteomics has great potential as an advanced technique for analyzing stress responses of microbes at the translational level.
A variety of sources can induce stress to bacterial cells, e.g., radiation (Sankaranarayanan, 1996), heat (Roncarati and Scarlato, 2017), salt (Egamberdieva et al., 2017), chemical mutagens (Abraham et al., 2006), carbon starvation (Handtke et al., 2018), and metabolites (Vainio et al., 1981; Kulling et al., 2002). To survive and thrive in these extreme conditions, microbes have a repertoire of genes that can be activated or silenced responding to stress (van der Veen and Abee, 2011). Although numerous works have attempted to unravel the changes in gene expression and mRNA transcription under stress response (Zhou et al., 2016; Kotrade et al., 2019; Qiao et al., 2019; Szabo et al., 2019), evidence has shown poor correlations between transcriptional and protein levels (Pascal et al., 2008). Accordingly, recent studies have employed proteomic analysis to investigate microbial global response to environmental stresses, e.g., antibiotic stress (Mathieu et al., 2016; Xiong et al., 2017), cold and light stress (Liu et al., 2018), oxidative stress (van Herwijnen et al., 2003), drying stress (Schott et al., 2017), thermal stress (Delgado-Baquerizo et al., 2018), and nitrate and phosphate depletion (Lin et al., 2017).
As a global response to DNA damage, the inducible DNA repair network was firstly found in Escherichia coli (Radman, 1975), named SOS response. SOS response network is highly conserved and widely present in bacteria, allowing them to repair or bypass DNA damage and maintain genome integrity (Friedman et al., 2005; Chaudhary et al., 2011). In addition, SOS response network is a key driving force for the evolution of bacterial genomes (Michel et al., 2004; Stavans, 2006), which is also linked to virulence and stress-induced mutagenesis (Sanchez-Alberola et al., 2012). Till now, SOS response network in E. coli is well studied that a LexA/RecA-dependent SOS response system consists of more than 40 enzymes performing diverse functions responding to DNA damage, e.g., homologous recombination, nucleotide excision repair (NER mechanism), and translesion DNA replication (Khan et al., 2001; Friedman et al., 2005; Meng and Zhu, 2011). Although other bacterial strains have similar DNA damage response mechanisms in comparison with that in E. coli (Rauch et al., 1996; Booth et al., 2001; Brezna et al., 2003; DeBruyn et al., 2012), different functional enzymes are found across species. For instance, a soil model microorganism Acinetobacter baylyi ADP1 has UmuDAb protein possessing a post-translational and LexA-like cleavage after DNA damage (Hare et al., 2012). Another recent study found a PafB/PafC-regulated DNA damage response network in Mycobacteria and other Actinobacteria strains (Olivencia et al., 2017). In addition, proteomics analysis has identified diverse proteins under DNA damage stress. For example, the proteomic response of Cryptococcus podzolicus Y3 to citrinin suggested that the up- and down-regulated proteins were associated with structural maintenance of chromosomes (DNA double-strand break repair Rad50 ATPase, etc.), cell apoptosis (cytochrome C), detoxification and energy metabolism (Glyco-syltransferase and malate dehydrogenase), and oxidative stress response (superoxide dismutase [Cu-Zn] and cysteine peroxiredoxin) (Wang et al., 2019).
DNA damage response, at the translational level, is reported to correlate with many environmental variables, e.g., pH (Wu et al., 2018), nutrient fluctuations (Lin et al., 2017), and carbon sources (Seo et al., 2013; Jiang et al., 2015). Among them, carbon source is essential for heterotrophic microorganisms and can influence protein profiles involved in carbohydrate transport and metabolism, energy metabolism, nucleotide metabolism, stress response, and protein biosynthesis (Halimaa et al., 2013; Moreno and Rojo, 2013; Siragusa et al., 2014; Liu et al., 2015; Mahar et al., 2016; Li et al., 2019).
Acinetobacter baylyi ADP1 is a well-established soil microorganism for gene manipulation owing to its natural transformation ability (Barbe et al., 2004) and tolerance to environmental stress (Al-Anizi et al., 2014). Many studies have constructed various bioreporters with A. baylyi ADP1 as hosts for genotoxicity assessment of environmental samples (Song et al., 2009, 2014; Zhang et al., 2013; Jiang et al., 2014, 2017; Jia et al., 2016). A previous study has documented that the DNA damage response in A. baylyi ADP1 was dependent on carbon source at both transcriptional and translational levels (Jiang et al., 2015). Such behavior might affect the performance of A. baylyi ADP1 bioreporters in environmental monitoring, but there is lack of detailed mechanisms of protein profiles change in response to genotoxins cultivated with different carbon sources. Therefore, a deeper insight into the carbon-dependent protein profiles gains our knowledge on the key proteins and processes involved in DNA damage response, benefiting the understanding of DNA damage response network in A. baylyi ADP1 and optimization of A. baylyi hosted bioreporters.
In this work, we studied the influence of carbon sources on proteomic profiles of A. baylyi ADP1 in response to DNA damage induced by mitomycin C. Besides a nutrient-rich medium Luria-Bertani Broth (LB), A. baylyi ADP1 cells were also cultivated in defined media with acetate, citrate, pyruvate, and succinate as sole carbon source, to explore the difference in proteomic profiles via isobaric tags for relative and absolute quantification (iTRAQ) coupled with liquid chromatography coupled with mass spectrometry (LC/MS/MS). Proteins associated with different functions and biological processes were compared, and up- or down-regulated proteins related to energy production and stress response were particularly addressed. A protein–protein network was built to elucidate the protein interactions. This work provides the first comprehensive discussion on DNA damage response of A. baylyi ADP1 under different carbon source conditions, aiming at a better understanding of DNA damage stress response and to improve the performance of A. baylyi ADP1 hosted bioreporters.
Unless specifically stated, all the reagents used in this study were purchased from Sigma-Aldrich (St. Louis, MO, United States) and of analytical grade. In this work, A. baylyi ADP1 was the model strain to evaluate the carbon-dependent proteome profiles in response to DNA damage. Nutrient-rich medium was LB medium, which consists of 5 g NaCl, 5 g yeast extract, and 10 g peptone in 1.0 L of sterilized water (pH adjusted to 7.0). Nutrient-deficient medium was prepared from minimal medium (MM), which consists of 0.5 g KH2PO4, 0.5 g NaCl, 0.1 g CaCl2, 0.2 g MgSO4⋅7H2O, 0.5 g FeSO4⋅7H2O, 0.5 g MnSO4, and 1.5 g (NH4)2SO4 in 1.0 L of sterilized water (Zhang et al., 2015). MM was then supplemented with different carbon sources (Zhang et al., 2011), and the final concentration of potassium acetate, sodium citrate, sodium pyruvate, and sodium succinate was 30 mM, designated as MMA, MMC, MMP, and MMS, respectively.
Mitomycin C is a bio-reductive alkylating agent causing DNA cross-linking and genotoxic effects (Abraham et al., 2006), viewed as a classic approach inducing DNA damage and used in this work to investigate the proteomic changes and global SOS response network in response to DNA damage in A. baylyi ADP1 among different carbon sources. In principle, A. baylyi ADP1 was firstly cultivated in nutrient-rich medium (LB) and then exposed to DNA damage induced by mitomycin C in both nutrient-rich medium (LB) and nutrient-deficient media (MMA, MMC, MMP, and MMS), respectively. To be more precise, A. baylyi ADP1 cells were inoculated in LB overnight at 30°C and harvested by centrifugation at 4000 × g for 10 min. After resuspended in the same volume of sterile deionized water, 1 ml of cell suspension was supplemented with 9 ml of fresh medium (LB, MMA, MMC, MMP, and MMS; carbon source final concentration, 27 mM, which falls in the range of 10–40 mM as conventional protocols for cell cultivation) (Selifonova et al., 1993; Zhang et al., 2012, 2013; Jebasingh et al., 2013; Borirak et al., 2015; Jia et al., 2016; Schmidt et al., 2016) and cultivated at 30°C until the early exponential phase when optical density at 600 nm (OD600) was 0.10. The suspensions were further added with mitomycin C to the final concentration of 1 μM to induce DNA damage. After 3-h exposure, cells were harvested by centrifugation at 6000 × g for 15 min at 4°C for further analysis. Although some previous studies have reported the application of 3 μM mitomycin C to induce A. baylyi cells, the concentration of mitomycin C used in the present study was set as 1 μM, because our previous work has reported a linear detection range of 0.1 nM to 1 μM for ADPWH_recA postexposure to mitomycin C (Jiang et al., 2017). Accordingly, mitomycin C concentration at 1 μM could induce the highest bioluminescent response of bioreporter cells, whereas higher concentration could significantly inhibit cell growth and might affect proteomic profiles (Song et al., 2009).
Cell pellets were suspended in a protein extraction buffer (urea [6.0 M], ethylenediamine tetra-acetic acid [EDTA, 0.5 mM], sodium dodecyl sulfate [SDS, 2%, w/v], and protease inhibitors cocktail mixture [Roche] in NH4HCO3 [100 mM] lysis buffer) (Borirak et al., 2015), followed by vortex and sonication (Branson Sonifier 450 D, United States). Sonication was set at 300 W in a pulse mode (10 s/10 s) for 20 min at ice bath until the cell suspension became clear. Protein concentration was measured by the Bradford assay (PierceTM Coomassie [Bradford] Protein Assay Kit, Thermo Scientific, United Kingdom).
Subsequently, around 100 μg of proteins was subjected to trypsin digestion as previously reported (Pereira et al., 2011). Briefly, 2 μl of Reducing Reagent (supplied in B-PERTM Complete Bacterial Protein Extraction Reagent, Thermo Scientific, United Kingdom) was added in protein suspension and incubated at 60°C for 1 h. Another 1 μl of Cysteine-Blocking Reagent was added, kept at room temperature for 10 min, and centrifuged for 20 min to discard the supernatant. Subsequently, 100 μl of Dissolution Buffer (supplied by iTRAQ® Reagent-8PLEX Multiplex Kit, Sigma-Aldrich, United States) was added and transferred to a new collection tube. Trypsin was added, incubated at 37°C overnight, and centrifuged for 20 min twice to collect the digested peptides. The quantity and quality of proteins and trypsin digestion efficiency were analyzed by 10% acrylamide gels.
The analysis of proteomics was performed using iTRAQ technology by Beijing Proteome Research Centre (Supplementary Figure S1). Briefly, 100 μg of digested peptides was labeled with amine-reactive isobaric tags (supplied in iTRAQ® Reagent-8PLEX Multiplex Kit, Sigma-Aldrich, United States), incubated at room temperature for 2 h, and stopped by 100 μl of water. Qualification and quantification of peptides were performed by LC/MS/MS. LC fractionation was performed in binary gradient buffers (buffer A and B). The labeled samples were resuspended in buffer A (10 mM NH4HCO3, 80% acetonitrile, pH 3.0). The binary gradient began with 5% B (10 mM NH4HCO3, 5% acetonitrile, pH 4.0) for 5 min, a linear ramp from 8% to 32% B for 59 min, an extended ramp from 32% to 95% B for 4 min, a further isocratic wash with 95% B for 5 min, and column re-equilibration with 5% B for 2 min. The injection volume was 100 μl and the chromatographic flow rate was constantly at 0.7 ml/min.
Each fragmented peptide tag produced distinct signature ions, which were detected by mass spectrometry (TripleTOFTM ABI-5600, Applied Biosystems, Wilmington, DE, United States) and distinguished by m/z value (Zilberstein, 2015). Data acquisition was in the positive ion mode with an accumulation time of 1 s. The selected mass detector ranged from 400 to 1250 m/z, and the precursor ion scan was performed within a range of 330–2000 m/z.
The original data were processed by ProteinPilotTM Software 4.5 (Applied Biosystems, Wilmington, DE, United States), using a database comprising all Acinetobacter protein sequences obtained from National Center for Biotechnology Information (NCBI) database. Acceptance threshold for peptide identification was length ≥ 6, z-score ≥ 5 and p-value ≤ 0.05. A decoy database created by reversing the sequences was used to calculate the false discovery rate (FDR), and proteins identified with at least two peptides and satisfying a 5% FDR were kept for quantitative analysis. The abundance of each peptide in LB treatment was set as the reference, and the relative abundance was calculated as the ratio of the abundance of each peptide in other treatments (MMA, MMC, MMP, and MMS) to that in LB.
Based on the well-accepted method to classify the up- and down-regulation using p-value in t-test (Lin et al., 2017; Fountain et al., 2018), peptides with relative abundance < 0.5 (p < 0.01), 0.5–0.83 (p < 0.05), 1.2–2.0 (p < 0.05), and >2.0 (p < 0.01) were designated in the present study to be significantly up-regulated or down-regulated. They were further classified according to the Gene Ontology (GO) function and GO biological process, via the Universal Protein Resource Database1 (O’Donovan and Apweiler, 2011; Lai et al., 2017) and PSORTdb 3.0.2 Network analysis of differentially expressed proteins was performed by String Software (V11.0): Protein–Protein Interaction Networks3. Statistical analysis was carried out by SPSS (version 21.0) to evaluate the correlations of the relative abundance of all the identified peptides between different carbon source treatments.
A total of 2807 proteins with function prediction were identified in all the treatments (Supplementary Figure S2). Analyzed by PSORTdb database and GO, they were categorized into 26 functions, and the most abundant ones included transferase (328 proteins, 11.7%), nucleic acid-binding protein (243 proteins, 8.6%), oxidoreductase (282 proteins, 10.0%), hydrolase (262 proteins, 9.3%), transporter (106 proteins, 3.8%), and lyase (93 proteins, 3.3%) (Supplementary Figure S2A). As for biological processes, most identified proteins were associated with biosynthesis process (257 proteins, 22.5%), metabolite process (219 proteins, 19.2%), transport (149 proteins, 13.1%), amino acid biosynthesis (91 proteins, 7.9%), regulator (73 proteins, 6.4%), and stress response (70 proteins, 6.1%) (Supplementary Figure S2B).
To investigate the distinct protein profiles in rich (LB) and defined media (MMA, MMC, MMP, and MMS), the numbers of up- or down-regulated proteins in MMA, MMC, MMP, and MMS treatments were calculated and listed in Table 1. Of all the 2807 identified proteins, the percentage of proteins with significant changes in MMA, MMC, MMP, and MMS was 52.1, 57.9, 51.1, and 48.2%, respectively. It indicated that the relative abundance of approximately half of the proteins was affected by carbon sources. Among the four defined media, the changes in peptide levels in MMC varied most greatly comparing to LB, with 29.5% proteins down-regulated and 28.3% proteins up-regulated, and MMS had 23.4% of proteins down-regulated and 24.7% of proteins up-regulated. However, the up- or down-regulated proteins are different across carbon sources, suggesting that the change of protein profiles is dependent on carbon sources.
Table 1. Number of proteins up- or down- regulated in defined media (MMA, MMC, MMP, and MMS) in comparison with LB.
The correlations of the relative abundance of all the identified peptides between different carbon source treatments are illustrated in Figure 1. The correlation coefficient (R), which is a statistical measure representing the strength of the relationship ranging from −1.0 and 1.0, is used to evaluate the similarity of protein profiles and a higher R value indicates a stronger positive relationship. Among the four treatments, the relative abundance of proteins in citrate treatment differed remarkably from that in acetate (R2 = 0.2432, Figure 1A), whereas the proteomic profiles were similar between acetate and pyruvate treatments (R2 = 0.5735, Figure 1B), and acetate and succinate treatments (R2 = 0.5697, Figure 1C). The proteomic profiles in pyruvate and succinate treatments showed the highest consistency (R2 = 0.8278, Figure 1F).
Figure 1. Correlation of relative abundance of proteins among carbon sources. (A) Acetate vs. citrate; (B) acetate vs. pyruvate; (C) acetate vs. succinate; (D) citrate vs. pyruvate; (E) citrate vs. succinate; (F) pyruvate vs. succinate.
According to the classification of protein function, the percentages of proteins with significant fold changes in each carbon source treatment are illustrated in Figure 2. Proteins with each function had their unique profiles among carbon sources. The relative abundance of some proteins showed similar tendency in all treatments, e.g., transferase (24.4–27.7% up-regulated and 22.3–29.9% down-regulated), synthase (19.1–29.8% up-regulated and 21.3–25.5% down-regulated), and transporter (26.4–35.8% up-regulated and 25.5–34.9% down-regulated). The regulation of some other proteins varied significantly across treatments. For instance, 55% of receptor proteins were down-regulated in MMA treatment, whereas only 25, 20, and 30% of them were down-regulated in MMC, MMP, and MMS treatments, respectively. Some proteins even demonstrated entirely opposite patterns. Among them, DNA polymerase was up-regulated in MMC, MMP, and MMS treatments, but down-regulated in MMA treatment. Interestingly, the percentage of up-regulated proteins related to DNA replication was remarkably higher in MMC than that in other treatments, e.g., DNA polymerase (50.0% up-regulated, for DNA synthesis from a DNA template), helicase (48.0% up-regulated, for the unwinding of double-stranded helical structure of nucleic acids), nuclease (46.2% up-regulated, for hydrolysis of nucleic acids), as well as peptidase (54.5% up-regulated, for the breakdown of protein peptides).
Figure 2. Percentage of proteins with significant fold changes in each carbon source treatment compared to LB medium. (A) MMA; (B) MMC; (C) MMP; (D) MMS. Proteins are classified by functions, and the categories with numbers less than 10 are summed up as “others.”
Regarding biological processes, the percentages of up- and down-regulated proteins across different carbon source treatments are shown in Figure 3. Among them, proteins related to biosynthetic process, metabolic process, amino acid biosynthesis, and aromatic hydrocarbon catabolism showed similar patterns in all treatments. A significant repression effect on translation-related proteins was found in all treatments, in which 93.5% ± 1.9% of translation-related proteins were down-regulated. Proteins involved in ATP synthesis behaved differently between MMC (6.7% up-regulated and 40.0% down-regulated), MMA (40.0% up-regulated and 13.3% down-regulated), MMP (46.7% up-regulated and 13.3% down-regulated), and MMS (46.7% up-regulated and 20.0% down-regulated). Additionally, proteins associated with response in adverse conditions were mostly down-regulated, including proteins for stress response (30.4% ± 11.6%), virulence or antiviral defense (40.9% ± 5.3%), and antibiotic resistance (18.8% ± 12.5%).
Figure 3. Percentage of proteins with significant fold changes in each carbon source treatment compared to LB medium. (A) MMA; (B) MMC; (C) MMP; (D) MMS. Proteins are classified by biological process category.
Of the proteins with significant fold changes in comparison with LB, 174 and 210 proteins exhibited the same up- or down-regulation patterns across different carbon source treatments, respectively. Among them, 140 and 166 proteins were validated or had putative roles in metabolic processes (Table 2). Proteins associated with four types of functions were most significantly affected, including transferase (34 up-regulated and 27 down-regulated), hydrolase (11 up-regulated and 16 down-regulated), oxidoreductase (37 up-regulated and 12 down-regulated), and DNA/RNA-binding proteins (7 up-regulated and 60 down-regulated).
For biological processes, most up- or down-regulated proteins were related to metabolite pathways and amino acid synthesis. Phospho-2-dehydro-3-deoxyheptonate aldolase (Aro, biosynthetic process) and 5-methyltetrahydropteroyltrigluta mate-homocysteine methyltransferase (MetE, methionine formation) in all treatments were most up-regulated, showing an average relative abundance of 15.2 ± 10.2 and 9.8 ± 1.6, respectively. In contrast, enzymes responsible for biosynthesis, e.g., L-lactate dehydrogenase (LldD), pyruvate dehydrogenase (cytochrome, PoxB), and malate synthase G (GlcB), were remarkably down-regulated. Additionally, proteins associated with amino acid metabolism varied significantly across different carbon sources, such as alanine (MurC, PanC, and DadX), aspartate (PyrB, GltK, and AspA), and glutamate (ArgJ, MetE, GltK, Gdh, AstE, and HemL). Proteins involved in DNA replication and stress response showed a diverse profiling change. The up-regulated proteins included DNA polymerase Pol III (DnaX), DNA replication regulator (GlnG, BaeR), and Holliday junction DNA helicase (RuvB), whereas DNA replication regulator (LldR, Cbl), two-component regulatory system response regulator (OmpR), and heat shock proteins (HtpG and HslO) were obviously down-regulated. It is worth mentioning that the relative abundance of all identified ribosomal proteins, including twenty-eight 50S ribosomal proteins and eighteen 30S ribosomal proteins, remarkably decreased in comparison with those in LB treatments (Table 2).
As carbon source participates in metabolism and energy production, proteins involved in glycolysis, pyruvate metabolism, and tricarboxylic acid (TCA) cycle were investigated (Figure 4 and Table 2). Except for two enzymes in glycolysis and TCA cycle that were down-regulated, fruK and fum encoding enzymes for the conversion of fructose-6-P to fructose-1,6-BP and fumarate to malate, other enzymes were all up-regulated, including fructose-1,6-bisphosphate aldolase (Fda), phosphoglycerate kinase (Pgk), pyruvate dehydrogenase (AceE), isocitrate dehydrogenase (Icd), succinate dehydrogenase flavoprotein (SdhA), malate dehydrogenase (Mdh), and citrate synthase (GltA).
Figure 4. Proteins involved in glycolysis/gluconeogenesis and TCA cycle with significant fold changes in nutrient-deficient media compared to nutrient-rich LB medium. The down- and up-regulated proteins are highlighted in green and red, respectively. Glucose-1-P, glucose 1-phosphate; glucose-6-P, glucose 6-phosphate; fructose-6-P, fructose 6-phosphate; G-3-P, glyceraldehyde 3-phosphate; DHAP, dihydroxyacetone phosphate; 1,3-BP-glycerate, 1,3-bisphospho-D-glycerate; 3-P-glycerate, 3-phospho-D-glycerate; 2-P-glycerate, 2-phospho-D-glycerate; PEP, phosphoenolpyruvate.
The protein–protein interaction network for up-regulated proteins was primarily composed of two clusters (marked by red circles in Figure 5A), both closely related to metabolite processes. Among them, core proteins located in Cluster I were citrate synthase (GltA), malic enzyme (MaeA), methylmalonate-semialdehyde dehydrogenase (MmsA), NADH oxidase (PutA), etc. In Cluster II, core proteins included acetolactate synthase (IlvH), dihydroxy-acid dehydratase (IlvD1), ketol-acid reductoisomerase (IlvC), branched-chain amino acid aminotransferase (IlvE), isopropylmalate isomerase (LeuC and LeuD), bifunctional phosphoribosyl-AMP cyclohydrolase/phosphoribosyl-ATP pyrophosphatase (HisI), etc. In contrast, the protein–protein interaction network for down-regulated proteins had only one cluster (Figure 5B). Proteins related to translation process functioned in this cluster, including ribosomal proteins of rpl, rpm, and rps families.
Figure 5. Protein–protein interaction networks of proteins with fold change across carbon sources under mitomycin C-induced DNA damage predicted by STRING. (A) The protein–protein interaction network of up-regulated proteins in nutrient-deficient media and core proteins were mainly involved in metabolic pathways. (B) The protein–protein interaction network of down-regulated proteins in nutrient-deficient media and core proteins were involved in translation processes. The red circle labels the peptides clustered together in the interaction network with close biological process or similar functions.
As A. baylyi ADP1 cells are commonly used as bioreporter hosts for evaluating genotoxicity in environmental samples, the relative abundance and profiles of stress-related proteins across the four defined media were investigated. Heatmap listed the relative abundance of 80 proteins involved in stress response (Figure 6). It is worth noting that these 80 proteins might show different up- and down-regulation pattern across all four carbon source treatments, differing from those listed in Table 2. These proteins are classified into seven categories according to a previous study (Onnis-Hayden et al., 2009), including 12 general stress-related proteins responsible for keeping steady the biochemical and biophysical homeostasis of cells, 9 antibiotic resistance/detoxification-related proteins responsive to chemical/drug-induced stress or killing, 6 protein stress-related proteins related to oxidative stress, and heat- or chemical-induced protein damage, 3 electron transport/transport-related proteins involved in electron transport, 5 activators/repressors involved in stress response, 2 redox stress-related proteins associated with conditions altering the redox potential of cells, and 43 response/DNA repair-related proteins.
Figure 6. Fold changes of proteins involved in stress response network in relation to different stress response categories. Columns from left to right represent the ratio of proteins of MMA-to-LB, MMC-to-LB, MMP-to-LB, and MMS-to-LB, respectively. (A) General stress. (B) Antibiotic resistance/detoxification. (C) Protein stress. (D) Electron transport/transport. (E) Activator/repressor. (F) Redox stress. (G) SOS response/DNA repair. Red arrows refer to significantly down-regulated proteins with relative abundance < 0.83 (p < 0.05); green arrows represent significantly up-regulated proteins with relative abundance > 1.20 (p < 0.05); yellow arrows refer to proteins with insignificant relative abundance change from 0.83 to 1.20 (p > 0.05).
Among them, only 20 proteins were not influenced by carbon source, including 5 associated with general stress (activator of morphogenic pathway, universal stress protein, catabolite repression control protein, membrane protease subunit, small ubiquitous protein required for normal growth), 2 related to antibiotic resistance/detoxification (aminoglycoside phosphotransferase and tellurium resistance protein), 1 electron transport/transport (transthyretin-like protein), 1 activator/repressor (alternative sigma factor Rpoh), and 12 involved in SOS response/DNA repair [DNA starvation/stationary phase protection protein Dps, excinuclease ABC subunit B, holliday junction DNA helicase (RuvA), helicase, formamidopyrimidine-DNA glycosylase, transferase, DNA polymerase III subunits tau and gamma, DNA polymerase III subunit delta, recombination protein RecJ, enzyme in methyl-directed mismatch repair, protein used in recombination and DNA repair, and chaperone protein DnaJ].
Proteins responsive to protein stress were mainly down-regulated (62.5%) or unchanged (33.3%). In this category, the relative abundance of heat shock protein 90 (0.6 ± 0.1) and heat shock protein HSP33 (0.5 ± 0.2) remarkably decreased in all treatments. Similarly, 40.0% and 55.0% of activators/repressors were down-regulated or unchanged, respectively. The relative abundance of DNA-binding transcriptional regulator HcaR declined to only 0.4 ± 0.1.
In contrast, proteins belonging to electron transporter/transporter were mostly up-regulated, e.g., electron transfer flavoprotein beta-subunit (1.7 ± 0.3), tryptophan synthase beta chain (1.5 ± 0.3), and oxidoreductase related to nitroreductase (2.0 ± 0.3). This was in accordance with the accelerated energy production rates in nutrient-deficient media (Figure 4), as electron transporters/transporters play critical roles in aerobic respiration and serve as the most typical way for bacteria to gain energy (Bjerg et al., 2018; Naradasu et al., 2019).
Across carbon source treatments, profiles of stress-related proteins in MMC treatment behaved significantly different in comparison with others. Nine proteins were down-regulated only in MMC treatment, which are general stress-related protein (Protease I), antibiotic resistance/detoxification-related proteins (tellurium resistance protein and RND efflux membrane fusion protein precursor), SOS response/DNA repair-related proteins (ATP-dependent helicase, endonuclease III, DNA ligase, DNA uptake protein, and/or related DNA-binding protein and rhombosortase), protein stress-related protein (bacteriolytic lipoprotein entericidin B), and redox stress-related catalase (hydroperoxidase II).
Forty-six proteins involved in SOS response/DNA repair were down-regulated in nutrient-deficient treatments (Figure 6G). Among them, three proteins were all down-regulated in all carbon source treatments, including DNA polymerase III subunit beta, protein RecA, and ATP-dependent dsDNA exonuclease (suppression of RecBC). Holliday junction DNA helicase (RuvB) was up-regulated in all nutrient-deficient treatments. The relative abundance of other proteins remained unchanged, e.g., DNA starvation/stationary phase protection protein Dps, excinuclease ABC subunit B, Holliday junction DNA helicase (RuvA), helicase, formamidopyrimidine-DNA glycosylase, transferase, DNA polymerase III subunits tau and gamma, DNA polymerase III subunit delta, recombination protein (RecJ), enzyme in methyl-directed mismatch repair, protein used in recombination and DNA repair, and Chaperone protein (DnaJ).
As a model soil microorganism with strong natural transformation competence, A. baylyi ADP1 has been fully sequenced (Barbe et al., 2004) and studied for decades (Hare and Gregg-Jolly, 2003; Metzgar et al., 2004; Zheng and Gregg-Jolly, 2004; Buchan and Ornston, 2005). Although A. baylyi ADP1 cells have been previously constructed as hosts for DNA damage responsive whole-cell bioreporters in environmental toxicity assessment (Ammerman and Azam, 1987; Barbe et al., 2004; Song et al., 2009; Jiang et al., 2014, 2017; Jia et al., 2016), the DNA damage response network in A. baylyi ADP1 is still not well-established. Additionally, previous studies addressing Acinetobacter-based bioreporters have demonstrated that carbon sources have significant impacts on their performance. For instance, MMC and MMS were preferred carbon sources to enhance the responsive ratio of ADPWH_recA after DNA damage, whereas MMA and rich medium (LB) did not achieve the optimal performance (Jiang et al., 2015). However, the underlying mechanisms influencing the behavior of ADP1 in response to toxins cultivated with different carbon sources, particularly on translational level, are still not clear and require further study.
From Figures 1, 2 and Supplementary Figure S1, carbon sources significantly affected the protein profiles in A. baylyi ADP1 responding to DNA damage by iTRAQ-based proteomics analysis, particularly those involved in metabolism, biosynthesis, transport, energy utilization, and stress response. Previous studies have reported some correlations between carbon source and proteomic profiles. Vandera et al. (2015) conducted a metabolic study on the cultivation of Arthrobacter phenanthrenivorans Sphe3 with phenanthrene, phthalate, glucose, or their combinations, suggesting that the influence of aromatic substrates in shaping the protein abundance was related to substrate and amino acid metabolism, as well as stress response. Giardina et al. investigated the influence of glucose starvation and re-feeding on the proteomic profiles of Saccharomyces cerevisiae, demonstrating that up-regulated proteins in response to glucose re-feeding included ribosomal subunits and plasma membrane ATPase, whereas those down-regulated included small heat shock proteins, mitochondrial proteins, and gluconeogenic enzymes (Licausi et al., 2013).
Particularly, across different carbon sources, the protein profiles in MMA, MMP, and MMS treatments were similar, all behaving different with those in MMC treatment (Table 2 and Figure 1). To be more precise, more proteins associated with DNA replication and stress were down-regulated in MMC treatment (Figures 2, 6). It might be explained by the ubiquitous roles of citrate as a key cellular intermediate readily assimilated through the central metabolic pathway. This possibly explains the previous observation in Jiang’s work that ADPWH_recA bioreporter cells had the best performance in responding to genotoxins in MMC treatment (Jiang et al., 2015).
The citrate-related proteomic profiles were also previously reported. By exploring the proteome of Pseudomonas putida F1, Mandalakis et al. (2013) found the enhancement of the Na+/H+ antiporter and carbonic anhydrase in citrate treatments compared to benzoate treatments, suggesting that citrate poses more challenges in maintaining pH homeostasis. Our work, for the first time, addressed the critical roles of citrate in shaping the DNA damage responsive proteomic profiles in A. baylyi ADP1.
As it is a key technique for exploring gene changes at the level of translation, proteomics has always been applied to reveal the responses of microbes to environmental stresses (McNair et al., 2007; Xu et al., 2007; Chuang et al., 2010; Tian et al., 2013). Transcriptome is another widely used technique in the investigation of microbial responses under stress, which is useful for identifying novel transcripts and analyzing gene expression (Jonas et al., 2007; Sun et al., 2015). The transcriptome of A. baylyi ADP1 in response to DNA damage was also explored. Hare et al. applied RNA-Seq to evaluate the DNA damage transcriptome in A. baylyi ADP1 induced by mitomycin C with MMS as the medium. Although 2% of genes (66) in the genome of A. baylyi ADP1 were inducible by DNA damage, only a few of them were differentially regulated, and most of them were repressed or absent (Hare et al., 2014). To be more precise, 38.4% of all genes in A. baylyi ADP1 were repressed in case of DNA damage induced by mitomycin C (Hare et al., 2014). Another study by Aranda et al. (2013) applied DNA microarray to investigate the transcriptional response of Acinetobacter baumannii to mitomycin C, confirming the roles of UmuDAb as a direct regulator of DNA damage response instead of RecA. Other studies addressed the transciptomic changes in A. baylyi postexposure to environmental stresses, such as low temperature (Ma et al., 2019), pesticides (Pi et al., 2017), and antibiotics (Heo et al., 2014). However, many studies have suggested a weak correlation or discrimination between the transcriptomic and proteomic changes (Barker et al., 2012; Lackner et al., 2012; Su et al., 2016; Fan et al., 2017; Bathke et al., 2019), which is possibly due to the key role that post-transcriptional processes play in the adaptation to stresses (Fan et al., 2017). Therefore, the investigation on the proteomic profiles of A. baylyi ADP1 responsive to DNA damage in different carbon sources provides significant molecular information and clue for further interpretation of the mechanism of DNA damage response in A. baylyi ADP1.
The present work addressed the different protein profiles in rich (LB) and nutrient-deficient media (MMA, MMC, MMP, and MMS), possibly explained by carbon catabolite repression (CCR). CCR was first reported in E. coli and defined as the repression of pathways or enzyme activities related to the use of secondary carbon sources in the presence of a preferred carbon source, which is an important mechanism allowing efficient carbon source utilization (Ishizuka et al., 1993; Gosset et al., 2004; Görke and Stülke, 2008). A recent study demonstrated that driven by CCR, E. coli showed time-series protein profile changes and 96 proteins were remarkably affected, including those responsible for amino acid biosynthesis, cell division and DNA replication, translation, transcription, and central carbon metabolism (Borirak et al., 2015).
For A. baylyi ADP1, Huang et al. found that some components in LB medium, e.g., yeast extract, aspartic acid, and asparagine, repressed the expression of Pu promoter, explained by CCR (Huang et al., 2008). In addition, CCR is previously reported in A. baylyi during the polycyclic aromatic hydrocarbon (PAH) degradation process, in which acetate and succinate exhibited the repression effect and catabolite repression control (Crc) protein was identified (Fischer et al., 2008; Bernard and Habash, 2009; Zimmermann et al., 2009). Accordingly, CCR could potentially influence the performance of Acinetobacter-based bioreporters owing to the down-regulated proteins related to adverse conditions in nutrient-deficient media.
It is worth pointing out that almost all translation-related proteins were down-regulated in nutrient-deficient treatments comparing to LB medium (Figure 3). This was consistent with the location of ribosomal proteins in the center of protein–protein interaction network for down-regulated proteins (Figure 5). Ribosomal proteins are reported to conjunct with rRNA and make up the ribosomal subunits involved in the cellular process of translation (Rodnina and Wintermeyer, 2011; Rainer Nikolay et al., 2015), and therefore are positively related to the protein synthesis. Our results indicated that the translation process in A. baylyi ADP1 was remarkably inhibited in nutrient-deficient medium, while ribosomal and translation-related proteins were up-regulated, resulting in increased protein synthesis and nutrient uptake in nutrient-rich medium, e.g., LB.
Similar to our findings, previous studies also reported that translation-related proteins were significantly affected by carbon sources. However, no unique conclusion has been drawn whether the translation was promoted by nutritional downshift from nutrient-rich to nutrient-deficient medium. For instance, proteomic analysis deciphering the tacrolimus-overproduction mechanism of Streptomyces tsukubaensis revealed that soybean oil addition tuned the pathways of transcriptional regulation, translation, and stress response (Wang et al., 2017). Giardina et al. (2012) studied the proteomic changes in S. cerevisiae after transferring from glucose depletion to glucose-rich medium, and the proteins involved in translation including ribosomal subunits and plasma membrane ATPase were up-regulated in glucose-rich medium. A recent iTRAQ-based proteomic study investigated the adaptive strategies of Rubrivivax benzoatilyticus JA2 to glucose and demonstrated the down-regulation of proteins involved in DNA replication, translation, electron transport, and photosynthetic machinery (Gupta et al., 2019). These findings suggested that different carbon sources resulted in significant fold changes of translation-related proteins.
As A. baylyi ADP1 is commonly used as a bioreporter for genotoxicity assessment, the patterns of DNA damage responsive proteins were comprehensively analyzed. There were three down-regulated proteins (subunit β of DNA polymerase III, RecA, and ATP-dependent dsDNA exonuclease) and one up-regulated protein (Holliday junction DNA helicase RuvB) among all the proteins related to DNA damage response (Figure 6G).
Among the down-regulated proteins, DNA polymerase III is a primary enzyme complex involved in prokaryotic DNA replication, with proofreading capabilities to correct replication mistakes by means of exonuclease activity working at 3′ to 5′ (Kelman and O’Donnell, 1995). The subunit β of DNA polymerase III acts as sliding DNA clamps, keeping the polymerase bound to the DNA (Leshinsky, 2008). RecA is a highly conserved protein in prokaryotic organisms, and its role in SOS response in E. coli is binding to single-strand DNA and helping recombinational repair and cleavage of LexA (Michel, 2005; Schlacher et al., 2006; Chen et al., 2008). Although evidence has suggested that RecA is also involved in DNA repair in Acinetobacter, possibly responsible for the cleavage of LexA-like UmuDAb (Aranda et al., 2011; DeBruyn et al., 2012), its mechanisms in Acinetobacter are not clear. ATP-dependent dsDNA exonuclease participates in DNA replication process when forming the blockage of replication forks. The only up-regulated protein Holliday junction DNA helicase (RuvB) involves the removal of Holliday junction, created by the annealing of newly synthesized strands, from the end of DNA double strands (Fainstein, 2005).
As most proteins (55.3%) involved in SOS response/DNA repair were down-regulated (Figure 6G) in nutrient-deficient media, less DNA damage was repaired and therefore led to a higher induction ratio of ADPWH_recA to genotoxins. Thus, our results provided proteomic explanation that LB was not an ideal medium for genotoxicity assessment in Acinetobacter-based bioreporters (Jiang et al., 2015), offering in-depth clues on the roles of carbon sources in post-transcriptional regulation during DNA damage response in A. baylyi ADP1.
In this work, the impacts of carbon source on the proteomic profiles of A. baylyi ADP1 responding to DNA damage response were comprehensively explored and discussed by iTRAQ for the first time. Our results unraveled the significant difference in proteomic patterns between nutrient-rich and nutrient-deficient media. Transferase, hydrolase, oxidoreductase, and DNA/RNA-binding proteins were significantly affected by carbon sources, and they were mainly involved in the biological processes of metabolite pathways and amino acid synthesis. Particularly, 80 proteins involved in stress response were significantly altered, hinting the strong influence of carbon source on microbial response to DNA damage and other stresses. These findings provide important mechanistic insights into the adaptation of A. baylyi ADP1 to DNA damage stress under nutrient-deficient conditions, suggesting that nutrient-deficient medium, instead of LB, was ideal for genotoxicity assessment in Acinetobacter-based bioreporters. Our findings also offer technical tools and theoretical clues to unravel the SOS response network and influential factors in other model microorganisms.
The datasets generated for this study can be found in the http://www.ncbi.nlm.nih.gov/bioproject/549090.
BJ and DZ contributed to the conception and design of the study. BJ, NZ, LL, and GS did the lab work. DZ, YX, GL, and BJ performed the data analysis. DZ, BJ, NZ, and LL performed the statistical analysis. BJ wrote the first draft of the manuscript. DZ, GL, and YX revised the manuscript.
This study was financially supported by the National Natural Science Foundation of China (41807119), the Special Fund of State Key Joint Laboratory of Environment Simulation and Pollution Control (19K04ESPCT), Fundamental Research Funds for the Central Universities (FRF-TP-16-063A1), the China Postdoctoral Scientific Fund (2017M620626), and the Beijing Municipal Science and Technology Project (Grant Number: Z161100002716023). DZ also acknowledges the financial support of Chinese Government’s Thousand Talents Plan for Young Professionals and the help of Yiqing (Suzhou) Environmental Technology Co. Ltd. for data analysis.
The authors declare that the research was conducted in the absence of any commercial or financial relationships that could be construed as a potential conflict of interest.
The Supplementary Material for this article can be found online at: https://www.frontiersin.org/articles/10.3389/fmicb.2019.02906/full#supplementary-material
Abraham, L. M., Selva, D., Casson, R., and Leibovitch, I. (2006). Mitomycin: clinical applications in ophthalmic practice. Drugs 66, 321–340. doi: 10.2165/00003495-200666030-00005
Al-Anizi, A. A., Hellyer, M. T., and Zhang, D. (2014). Toxicity assessment and modelling of Moringa oleifera seeds in water purification by whole cell bioreporter. Water Res. 56, 77–87. doi: 10.1016/j.watres.2014.02.045
Ammerman, J. W., and Azam, F. (1987). Characteristics of cyclic AMP transport by marine bacteria. Appl. Environ. Microbiol. 53, 2963–2966.
Aranda, J., Bardina, C., Beceiro, A., Rumbo, S., Cabral, M. P., Barbe, J., et al. (2011). Acinetobacter baumannii RecA protein in repair of DNA damage, antimicrobial resistance, general stress response, and virulence. J. Bacteriol. 193, 3740–3747. doi: 10.1128/jb.00389-11
Aranda, J., Poza, M., Shingu-Vazquez, M., Cortes, P., Boyce, J. D., Adler, B., et al. (2013). Identification of a DNA-Damage-Inducible Regulon in Acinetobacter baumannii. J. Bacteriol. 195, 5577–5582. doi: 10.1128/JB.00853-13
Barbe, V., Vallenet, D., Fonknechten, N., Kreimeyer, A., Oztas, S., Labarre, L., et al. (2004). Unique features revealed by the genome sequence of Acinetobacter sp. ADP1, a versatile and naturally transformation competent bacterium. Nucleic Acids Res. 32, 5766–5779. doi: 10.1093/nar/gkh910
Barker, B. M., Kroll, K., Vodisch, M., Mazurie, A., Kniemeyer, O., and Cramer, R. A. (2012). Transcriptomic and proteomic analyses of the Aspergillus fumigatus hypoxia response using an oxygen-controlled fermenter. BMC Genomics 13:62. doi: 10.1186/1471-2164-13-62
Bathke, J., Konzer, A., Remes, B., Mcintosh, M., and Klug, G. (2019). Comparative analyses of the variation of the transcriptome and proteome of Rhodobacter sphaeroides throughout growth. BMC Genomics 20:358. doi: 10.1186/s12864-019-5749-3
Bernard, S. M., and Habash, D. Z. (2009). The importance of cytosolic glutamine synthetase in nitrogen assimilation and recycling. New Phytol. 182, 608–620. doi: 10.1111/j.1469-8137.2009.02823.x
Bjerg, J. T., Boschker, H. T. S., Larsen, S., Berry, D., Schmid, M., Millo, D., et al. (2018). Long-distance electron transport in individual, living cable bacteria. Proc. Natl. Acad. Sci. U.S.A. 115, 5786–5791. doi: 10.1073/pnas.1800367115
Booth, M. G., Jeffrey, W. H., and Miller, R. V. (2001). RecA expression in response to solar UVR in the marine bacterium Vibrio natriegens. Microbial Ecology 42, 531–539. doi: 10.1007/s00248-001-1009-5
Borirak, O., Rolfe, M. D., De Koning, L. J., Hoefsloot, H. C. J., Bekker, M., Dekker, H. L., et al. (2015). Time-series analysis of the transcriptome and proteome of Escherichia coli upon glucose repression. Biochim. Biophys. Acta Proteins Proteomics 1854, 1269–1279. doi: 10.1016/j.bbapap.2015.05.017
Brezna, B., Khan, A. A., and Cerniglia, C. E. (2003). Molecular characterization of dioxygenases from polycyclic aromatic hydrocarbon-degrading Mycobacterium spp. FEMS Microbiol. Lett. 223, 177–183. doi: 10.1016/s0378-1097(03)00328-8
Buchan, A., and Ornston, L. N. (2005). When coupled to natural transformation in Acinetobacter sp strain ADP1, PCR mutagenesis is made less random by mismatch repair. Appl. Environ. Microbiol. 71, 7610–7612. doi: 10.1128/aem.71.11.7610-7612.2005
Chaudhary, P., Sharma, R., Singh, S. B., and Nain, L. (2011). Bioremediation of PAH by Streptomyces sp. Bull. Environ. Contamin. Toxicol. 86, 268–271. doi: 10.1007/s00128-011-0211-5
Chen, Z., Yang, H., and Pavletich, N. P. (2008). Mechanism of homologous recombination from the RecA–ssDNA/dsDNA structures. Nature 453, 489–484.
Chuang, A. S., Jin, Y. O., Schmidt, L. S., Li, Y. L., Fogel, S., Smoler, D., et al. (2010). Proteomic analysis of ethene-enriched groundwater microcosms from a Vinyl chloride-contaminated site. Environ. Sci. Technol. 44, 1594–1601. doi: 10.1021/es903033r
DeBruyn, J. M., Mead, T. J., and Sayler, G. S. (2012). Horizontal transfer of PAH catabolism genes in Mycobacterium: evidence from comparative genomics and isolated Pyrene-degrading bacteria. Environ. Sci. Technol. 46, 99–106. doi: 10.1021/es201607y
Delgado-Baquerizo, M., Oliverio, A. M., Brewer, T. E., Benavent-Gonzalez, A., Eldridge, D. J., Bardgett, R. D., et al. (2018). A global atlas of the dominant bacteria found in soil. Science 359, 320–325. doi: 10.1126/science.aap9516
Egamberdieva, D., Wirth, S. J., Shurigin, V. V., Hashem, A., and Abd Allah, E. F. (2017). Endophytic bacteria improve plant growth, symbiotic performance of chickpea (Cicer arietinum L.) and induce suppression of root rot caused by Fusarium solani under salt stress. Front. Microbiol. 8:1887. doi: 10.3389/fmicb.2017.01887
Fainstein, S. S. (2005). Cities and diversity - Should we want it? Can we plan for it? Urb. Affairs Rev. 41, 3–19. doi: 10.1177/1078087405278968
Fan, M., Sun, X., Xu, N., Liao, Z., Li, Y., Wang, J., et al. (2017). Integration of deep transcriptome and proteome analyses of salicylic acid regulation high temperature stress in Ulva prolifera. Sci. Rep. 7:11052. doi: 10.1038/s41598-017-11449-w
Fischer, R., Bleichrodt, F. S., and Gerischer, U. C. (2008). Aromatic degradative pathways in Acinetobacter baylyi underlie carbon catabolite repression. Microbiology 154, 3095–3103. doi: 10.1099/mic.0.2008/016907-0
Fountain, J. C., Koh, J., Yang, L., Pandey, M. K., Nayak, S. N., Bajaj, P., et al. (2018). Proteome analysis of Aspergillus flavus isolate-specific responses to oxidative stress in relationship to aflatoxin production capability. Sci. Rep. 8:3430. doi: 10.1038/s41598-018-21653-x
Friedman, N., Vardi, S., Ronen, M., Alon, U., and Stavans, J. (2005). Precise temporal modulation in the response of the SOS DNA repair network in individual bacteria. PLoS Biology 3:e238. doi: 10.1371/journal.pbio.0030238
Giardina, B. J., Stanley, B. A., and Chiang, H. L. (2012). Comparative proteomic analysis of transition of Saccharomyces cerevisiae from glucose-deficient medium to glucose-rich medium. Proteome Sci. 10:40. doi: 10.1186/1477-5956-10-40
Görke, B., and Stülke, J. (2008). Carbon catabolite repression in bacteria: many ways to make the most out of nutrients. Nat. Rev. Microbiol. 6:613. doi: 10.1038/nrmicro1932
Gosset, G., Zhang, Z., Nayyar, S., Cuevas, W. A., and Saier, M. H. (2004). Transcriptome analysis of crp-dependent catabolite control of gene expression in Escherichia coli. J. Bacteriol. 186, 3516–3524. doi: 10.1128/jb.186.11.3516-3524.2004
Gupta, D., Mohammed, M., Mekala, L. P., Chintalapati, S., and Chintalapati, V. R. (2019). iTRAQ-based quantitative proteomics reveals insights into metabolic and molecular responses of glucose-grown cells of Rubrivivax benzoatilyticus JA2. J. Proteomics 194, 49–59. doi: 10.1016/j.jprot.2018.12.027
Halimaa, P., Lin, Y. F., Ahonen, V. H., Blande, D., Clemens, S., Gyenesei, A., et al. (2013). Gene expression differences between Noccaea caerulescens ecotypes help to identify candidate genes for metal phytoremediation. Environ. Sci. Technol. 48, 3344–3353. doi: 10.1021/es4042995
Handtke, S., Albrecht, D., Otto, A., Becher, D., Hecker, M., and Voigt, B. (2018). The proteomic response of Bacillus pumilus cells to glucose starvation. Proteomics 18:1700109. doi: 10.1002/pmic.201700109
Hare, J. M., Adhikari, S., Lambert, K. V., Hare, A. E., and Grice, A. N. (2012). The Acinetobacter regulatory UmuDAb protein cleaves in response to DNA damage with chimeric LexA/UmuD characteristics. FEMS Microbiol. Lett. 334, 57–65. doi: 10.1111/j.1574-6968.2012.02618.x
Hare, J. M., Ferrell, J. C., Witkowski, T. A., and Grice, A. N. (2014). Prophage induction and differential RecA and UmuDAb transcriptome regulation in the DNA damage responses of Acinetobacter baumannii and Acinetobacter baylyi. PLoS One 9:e93861. doi: 10.1371/journal.pone.0093861
Hare, J. M., and Gregg-Jolly, L. A. (2003). UmuD is not involved in the response to DNA damage of Acinetobacter sp. strain ADP1. Abstracts of the general meeting of the. Am. Soc. Microbiol. 103:K–031.
Heo, A., Jang, H.-J., Sung, J.-S., and Park, W. (2014). Global transcriptome and physiological responses of Acinetobacter oleivorans DR1 exposed to distinct classes of antibiotics. PLoS One 9:e110215. doi: 10.1371/journal.pone.0110215
Huang, W. E., Singer, A. C., Spiers, A. J., Preston, G. M., and Whiteley, A. S. (2008). Characterizing the regulation of the Pu promoter in Acinetobacter baylyi ADP1. Environ. Microbiol. 10, 1668–1680. doi: 10.1111/j.1462-2920.2008.01583.x
Ishizuka, H., Hanamura, A., Kunimura, T., and Aiba, H. (1993). A lowered concentration of cAMP receptor protein caused by glucose is an important determinant for catabolite repression in Escherichia coli. Mol. Microbiol. 10, 341–350. doi: 10.1111/j.1365-2958.1993.tb01960.x
Jebasingh, S. E. J., Lakshmikandan, M., Rajesh, R. P., and Raja, P. (2013). Biodegradation of acrylamide and purification of acrylamidase from newly isolated bacterium Moraxella osloensis MSU11. Int. Biodeterior. Biodegrad. 85, 120–125. doi: 10.1016/j.ibiod.2013.06.012
Jia, J., Li, H., Zong, S., Jiang, B., Li, G., Ejenavi, O., et al. (2016). Magnet bioreporter device for ecological toxicity assessment on heavy metal contamination of coal cinder sites. Sensors Actuat. B 222, 290–299. doi: 10.1016/j.snb.2015.08.110
Jiang, B., Li, G. H., Xing, Y., Zhang, D. Y., Jia, J. L., Cui, Z. S., et al. (2017). A whole-cell bioreporter assay for quantitative genotoxicity evaluation of environmental samples. Chemosphere 184, 384–392. doi: 10.1016/j.chemosphere.2017.05.159
Jiang, B., Song, Y., Zhang, D., Huang, W. E., Zhang, X., and Li, G. (2015). The influence of carbon sources on the expression of the recA gene and genotoxicity detection by an Acinetobacter bioreporter. Environ. Sci. 17, 835–843. doi: 10.1039/c4em00692e
Jiang, B., Zhu, D., Song, Y., Zhang, D., Liu, Z., Zhang, X., et al. (2014). Use of a whole-cell bioreporter. Acinetobacter baylyi, to estimate the genotoxicity and bioavailability of chromium(VI)-contaminated soils. Biotechnol. Lett. 37, 343–348. doi: 10.1007/s10529-014-1674-3
Jonas, C., Xe, Isabelle, G.-M., Ger, J. J., and Catherine, B. (2007). Response of the transcriptome of the intertidal red seaweed Chondrus crispus to controlled and natural stresses. New Phytol. 176, 45–55. doi: 10.1111/j.1469-8137.2007.02152.x
Kelman, Z., and O’Donnell, M. (1995). DNA polymerase III holoenzyme: structure and function of a chromosomal replicating machine. Ann. Rev. Biochem. 64, 171–200. doi: 10.1146/annurev.biochem.64.1.171
Khan, A. A., Wang, R. F., Cao, W. W., Doerge, D. R., Wennerstrom, D., and Cerniglia, C. E. (2001). Molecular cloning, nucleotide sequence, and expression of genes encoding a polcyclic aromatic ring dioxygenase from Mycobacterium sp strain PYR-1. Appl. Environ. Microbiol. 67, 3577–3585. doi: 10.1128/aem.67.8.3577-3585.2001
Kotrade, P., Sehr, E. M., Wischnitzki, E., and Brueggemann, W. (2019). Comparative transcriptomics-based selection of suitable reference genes for normalization of RT-qPCR experiments in drought-stressed leaves of three European Quercus species. Tree Genet. Genomes 15, 38.
Kulling, S. E., Lehmann, L., and Metzler, M. (2002). Oxidative metabolism and genotoxic potential of major isoflavone phytoestrogens. J. Chromatogr. B 777, 211–218. doi: 10.1016/s1570-0232(02)00215-5
Lackner, D. H., Schmidt, M. W., Wu, S., Wolf, D. A., and Bähler, J. (2012). Regulation of transcriptome, translation, and proteome in response to environmental stress in fission yeast. Genome Biol. 13:R25. doi: 10.1186/gb-2012-13-4-r25
Lai, C., Huang, L., Chen, L., Chan, M., and Shaw, J. (2017). Genome-wide analysis of GDSL-type esterases/lipases in Arabidopsis. Plant Mol. Biol. 95, 181–197. doi: 10.1007/s11103-017-0648-y
Leshinsky, R. (2008). Knowing the social in urban planning law decision-making. Urb. Policy Res. 26, 415–427. doi: 10.1080/08111140802084767
Li, H., Martin, F. L., Jones, K. C., and Zhang, D. (2019). Interrogating the transient selectivity of bacterial chemotaxis-driven affinity and accumulation of carbonaceous substances via raman microspectroscopy. Front. Microbiol. 10:2215. doi: 10.3389/fmicb.2019.02215
Licausi, F., Ohme-Takagi, M., and Perata, P. (2013). APETALA2/Ethylene Responsive Factor (AP2/ERF) transcription factors: mediators of stress responses and developmental programs. New Phytol. 199, 639–649. doi: 10.1111/nph.12291
Lin, Q., Liang, J. R., Huang, Q. Q., Luo, C. S., Anderson, D. M., and Bowler, C., et al. (2017). Differential cellular responses associated with oxidative stress and cell fate decision under nitrate and phosphate limitations in Thalassiosira pseudonana: comparative proteomics. PLoS One 12:e0184849. doi: 10.1371/journal.pone.0184849
Liu, J.-Y., Chang, M.-C., Meng, J.-L., Feng, C.-P., and Wang, Y. (2018). A comparative proteome approach reveals metabolic changes associated with Flammulina velutipes mycelia in response to cold and light stress. J. Agricult. Food Chem. 66, 3716–3725. doi: 10.1021/acs.jafc.8b00383
Liu, W., Xie, Y., Ma, J., Luo, X., Nie, P., Zuo, Z., et al. (2015). IBS: an illustrator for the presentation and visualization of biological sequences. Bioinformatics 31, 3359–3361. doi: 10.1093/bioinformatics/btv362
Ma, Y., Zhang, S., Meng, L., and Li, W. (2019). Transcriptomic responses of Acinetobacter harbinensis HITLi 7(T) at low temperatures. Biomed. Environ. Sci. 32, 371–375.
Mahar, A., Wang, P., Ali, A., Awasthi, M. K., Lahori, A. H., Wang, Q., et al. (2016). Challenges and opportunities in the phytoremediation of heavy metals contaminated soils: a review. Ecotoxicol. Environ. Safety 126, 111–121. doi: 10.1016/j.ecoenv.2015.12.023
Mandalakis, M., Panikov, N., Dai, S., Ray, S., and Karger, B. L. (2013). Comparative proteomic analysis reveals mechanistic insights into Pseudomonas putida F1 growth on benzoate and citrate. Amb. Express 3:64. doi: 10.1186/2191-0855-3-64
Mathieu, A., Fleurier, S., Frenoy, A., Dairou, J., Bredeche, M.-F., Sanchez-Vizuete, P., et al. (2016). Discovery and function of a general core hormetic stress response in E-coli induced by sublethal concentrations of antibiotics. Cell Rep. 17, 46–57. doi: 10.1016/j.celrep.2016.09.001
McNair, K., Broad, J., Riedel, G., Davies, C. H., and Cobb, S. R. (2007). Global changes in the hippocampal proteome following exposure to an enriched environment. Neuroscience 145, 413–422. doi: 10.1016/j.neuroscience.2006.12.033
Meng, L., and Zhu, Y.-G. (2011). Pyrene biodegradation in an industrial soil exposed to simulated rhizodeposition: how does it affect functional microbial abundance? Environ. Sci. Technol. 45, 1579–1585. doi: 10.1021/es102995c
Metzgar, D., Bacher, J. M., Pezo, V., Reader, J., Doring, V., Schimmel, P., et al. (2004). Acinetobacter sp ADP1: an ideal model organism for genetic analysis and genome engineering. Nucleic Acids Res. 32, 5780–5790. doi: 10.1093/nar/gkh881
Michel, B. (2005). After 30 years of study, the bacterial SOS response still surprises us. PLoS Biol. 3:e255. doi: 10.1371/journal.pbio.0030255
Michel, B., Grompone, G., Flores, M. J., and Bidnenko, V. (2004). Multiple pathways process stalled replication forks. Proc. Natl. Acad. Sci. U.S.A. 101, 12783–12788. doi: 10.1073/pnas.0401586101
Moreno, R., and Rojo, F. (2013). The contribution of proteomics to the unveiling of the survival strategies used by Pseudomonas putida in changing and hostile environments. Proteomics 13, 2822–2830. doi: 10.1002/pmic.201200503
Naradasu, D., Miran, W., Sakamoto, M., and Okamoto, A. (2019). Isolation and characterization o f human gut bacteria capable of extracellular electron transport by electrochemical techniques. Front. Microbiol. 9:3267. doi: 10.3389/fmicb.2018.03267
O’Donovan, C., and Apweiler, R. (2011). “A guide to uniprot for protein scientists,” in Bioinformatics for Comparative Proteomics, eds C. H. Wu and C. Chen (Totowa, NJ: Humana Press), 25–35. doi: 10.1007/978-1-60761-977-2_2
Olivencia, B. F., Muller, A. U., Roschitzki, B., Burger, S., Weber-Ban, E., and Imkamp, F. (2017). Mycobacterium smegmatis PafBC is involved in regulation of DNA damage response. Sci. Rep. 7.
Onnis-Hayden, A., Weng, H., He, M., Hansen, S., Ilyin, V., Lewis, K., et al. (2009). Prokaryotic real-time gene expression profiling for toxicity assessment. Environ. Sci. Technol. 43, 4574–4581. doi: 10.1021/es803227z
Pascal, L. E., True, L. D., Campbell, D. S., Deutsch, E. W., Risk, M., Coleman, I. M., et al. (2008). Correlation of mRNA and protein levels: cell type-specific gene expression of cluster designation antigens in the prostate. BMC Genomics 9:246. doi: 10.1186/1471-2164-9-246
Pereira, S. B., Ow, S. Y., Barrios-Llerena, M. E., Wright, P. C., Moradas-Ferreira, P., and Tamagnini, P. (2011). iTRAQ-based quantitative proteomic analysis of Gloeothece sp. PCC 6909: comparison with its sheathless mutant and adaptations to nitrate deficiency and sulfur limitation. J. Proteomics 75, 270–283. doi: 10.1016/j.jprot.2011.09.007
Pi, B. R., Yu, D. L., Hua, X. T., Ruan, Z., and Yu, Y. S. (2017). Genomic and transcriptome analysis of triclosan response of a multidrug-resistant Acinetobacter baumannii strain, MDR-ZJ06. Arch. Microbiol. 199, 223–230. doi: 10.1007/s00203-016-1295-4
Qiao, W., Zhang, Y., Xie, Z., Luo, Y., Zhang, X., Sang, C., et al. (2019). Toxicity of perfluorooctane sulfonate on Phanerochaete chrysosporium: growth, pollutant degradation and transcriptomics. Ecotoxicol. Environ. Safety 174, 66–74. doi: 10.1016/j.ecoenv.2019.02.066
Radman, M. (1975). “SOS repair hypothesis: phenomenology of an inducible DNA repair which is accompanied by mutagenesis,” in Molecular Mechanisms for Repair of DNA, eds P. Hanawalt and R. Setlow (New York, NY: Springer), 355–367. doi: 10.1007/978-1-4684-2895-7_48
Rainer Nikolay, R., Van Den Bruck, D., Achenbach, J., and Nierhaus, K. H. (2015). Ribosomal Proteins: Role in Ribosomal Functions. Chichester: John Wiley & Sons Ltd.
Rauch, P. J., Palmen, R., Burds, A. A., Gregg-Jolly, L. A., Van Der Zee, J. R., and Hellingwerf, K. J. (1996). The expression of the Acinetobacter calcoaceticus recA gene increases in response to DNA damage independently of RecA and of development of competence for natural transformation. Microbiology 142(Pt 4), 1025–1032. doi: 10.1099/00221287-142-4-1025
Rodnina, M. V., and Wintermeyer, W. (2011). The ribosome as a molecular machine: the mechanism of tRNA–mRNA movement in translocation. Biochem. Soc. Trans. 39, 658–662. doi: 10.1042/BST0390658
Roncarati, D., and Scarlato, V. (2017). Regulation of heat-shock genes in bacteria: from signal sensing to gene expression output. FEMS Microbiol. Rev. 41, 549–574. doi: 10.1093/femsre/fux015
Sanchez-Alberola, N., Campoy, S., Barbe, J., and Erill, I. (2012). Analysis of the SOS response of Vibrio and other bacteria with multiple chromosomes. BMC Genomics 13:58. doi: 10.1186/1471-2164-13-58
Sankaranarayanan, K. (1996). Environmental chemical mutagens and genetic risks: lessons from radiation genetics. Environm. Mol. Mutagenesis 28, 65–70. doi: 10.1002/(sici)1098-2280(1996)28:2<65::aid-em1>3.0.co;2-d
Schlacher, K., Cox, M. M., Woodgate, R., and Goodman, M. F. (2006). RecA acts in trans to allow replication of damaged DNA by DNA polymerase V. Nature 442, 883–887. doi: 10.1038/nature05042
Schmidt, A., Kochanowski, K., Vedelaar, S., Ahrne, E., Volkmer, B., Callipo, L., et al. (2016). The quantitative and condition-dependent Escherichia coli proteome. Nat. Biotechnol. 34, 104–110. doi: 10.1038/nbt.3418
Schott, A.-S., Behr, J., Geißler, A. J., Kuster, B., Hahne, H., and Vogel, R. F. (2017). Quantitative proteomics for the comprehensive analysis of stress responses of Lactobacillus paracasei subsp. paracasei F19. J. Proteome Res. 16, 3816–3829. doi: 10.1021/acs.jproteome.7b00474
Selifonova, O., Burlage, R., and Barkay, T. (1993). Bioluminescent sensors for detection of bioavailable Hg(II) in the environment. Appl. Environ. Microbiol. 59, 3083–3090.
Seo, J. S., Keum, Y. S., and Li, Q. X. (2013). Metabolomic and proteomic insights into carbaryl catabolism by Burkholderia sp C3 and degradation of ten N-methylcarbamates. Biodegradation 24, 795–811. doi: 10.1007/s10532-013-9629-2
Siragusa, S., De Angelis, M., Calasso, M., Campanella, D., Minervini, F., Di Cagno, R., et al. (2014). Fermentation and proteome profiles of Lactobacillus plantarum strains during growth under food-like conditions. J. Proteomics 96, 366–380. doi: 10.1016/j.jprot.2013.11.003
Song, Y., Jiang, B., Tian, S., Tang, H., Liu, Z., Li, C., et al. (2014). A whole-cell bioreporter approach for the genotoxicity assessment of bioavailability of toxic compounds in contaminated soil in China. Environ. Pollut. 195, 178–184. doi: 10.1016/j.envpol.2014.08.024
Song, Y., Li, G., Thornton, S. F., Thompson, I. P., Banwart, S. A., Lerner, D. N., et al. (2009). Optimization of bacterial whole cell bioreporters for toxicity assay of environmental samples. Environ. Sci. Technol. 43, 7931–7938. doi: 10.1021/es901349r
Stavans, J. (2006). The SOS Response of Bacteria to DNA Damage - A Single Cell Study. Dordrecht: Springer.
Su, Y., Jiang, X. Z., Wu, W. P., Wang, M. M., Hamid, M. I., Xiang, M. C., et al. (2016). Genomic, transcriptomic, and proteomic analysis provide insights into the cold adaptation mechanism of the obligate psychrophilic fungus Mrakia psychrophila. G3-Genes Genomes Genet. 6, 3603–3613. doi: 10.1534/g3.116.033308
Sun, P., Mao, Y., Li, G., Cao, M., Kong, F., Wang, L., et al. (2015). Comparative transcriptome profiling of Pyropia yezoensis (Ueda) MS Hwang & HG Choi in response to temperature stresses. BMC Genomics 16:463. doi: 10.1186/s12864-015-1586-1
Szabo, B., Lang, Z., Bakonyi, G., Marien, J., Roelofs, D., Van Gestel, C. A. M., et al. (2019). Transgenerational and multigenerational stress gene responses to the insecticide etofenprox in Folsomia candida (Collembola). Ecotoxicol. Environ. Safety 175, 181–191. doi: 10.1016/j.ecoenv.2019.03.052
Tian, X. X., Chen, L., Wang, J. X., Qiao, J. J., and Zhang, W. W. (2013). Quantitative proteomics reveals dynamic responses of Synechocystis sp PCC 6803 to next-generation biofuel butanol. J. Proteomics 78, 326–345. doi: 10.1016/j.jprot.2012.10.002
Vainio, H., Norppa, H., Hemminki, K., and Sorsa, M. (1981). Metabolism and genotoxicity of styrene. Adv. Exp. Med. Biol. 136(Pt A), 257–274. doi: 10.1007/978-1-4757-0674-1_15
van der Veen, S., and Abee, T. (2011). Bacterial SOS response: a food safety perspective. Curr. Opin. Biotechnol. 22, 136–142. doi: 10.1016/j.copbio.2010.11.012
van Herwijnen, R., Wattiau, P., Bastiaens, L., Daal, L., Jonker, L., Springael, D., et al. (2003). Elucidation of the metabolic pathway of fluorene and cometabolic pathways of phenanthrene, fluoranthene, anthracene and dibenzothiophene by Sphingomonas sp LB126. Res. Microbiol. 154, 199–206. doi: 10.1016/s0923-2508(03)00039-1
Vandera, E., Samiotaki, M., Parapouli, M., Panayotou, G., and Koukkou, A. I. (2015). Comparative proteomic analysis of Arthrobacter phenanthreniuorans Sphe3 on phenanthrene, phthalate and glucose. J. Proteomics 113, 73–89. doi: 10.1016/j.jprot.2014.08.018
Wang, J., Liu, H. H., Huang, D., Jin, L. N., Wang, C., and Wen, J. P. (2017). Comparative proteomic and metabolomic analysis of Streptomyces tsukubaensis reveals the metabolic mechanism of FK506 overproduction by feeding soybean oil. Appl. Microbiol. Biotechnol. 101, 2447–2465. doi: 10.1007/s00253-017-8136-5
Wang, K. L., Lin, Z., Zhang, H. Y., Zhang, X. Y., Zheng, X. F., Zhao, L. N., et al. (2019). Investigating proteome and transcriptome response of Cryptococcus podzolicus Y3 to citrinin and the mechanisms involved in its degradation. Food Chem. 283, 345–352. doi: 10.1016/j.foodchem.2019.01.052
Wu, H., Zhao, Y., Du, Y., Miao, S., Liu, J., Li, Y., et al. (2018). Quantitative proteomics of Lactococcus lactis F44 under cross-stress of low pH and lactate. J. Dairy Sci. 101, 6872–6884. doi: 10.3168/jds.2018-14594
Xiong, L., Liao, D., Lu, X., Yan, H., Shi, L., and Mo, Z. (2017). Proteomic analysis reveals that a global response is induced by subinhibitory concentrations of ampicillin. Bioengineered 8, 732–741. doi: 10.1080/21655979.2017.1373532
Xu, S. Y., Chen, Y. X., Wu, W. X., Zheng, S. J., Xue, S. G., Yang, S. Y., et al. (2007). Protein changes in response to pyrene stress in maize (Zea mays L.) leaves. J. Integr. Plant Biol. 49, 187–195. doi: 10.1111/j.1744-7909.2007.00284.x
Zhang, D., Berry, J. P., Zhu, D., Wang, Y., Chen, Y., Jiang, B., et al. (2015). Magnetic nanoparticle-mediated isolation of functional bacteria in a complex microbial community. ISME J. 9, 603–614. doi: 10.1038/ismej.2014.161
Zhang, D., Ding, A., Cui, S., Hu, C., Thornton, S. F., Dou, J., et al. (2013). Whole cell bioreporter application for rapid detection and evaluation of crude oil spill in seawater caused by Dalian oil tank explosion. Water Res. 47, 1191–1200. doi: 10.1016/j.watres.2012.11.038
Zhang, D., He, Y., Wang, Y., Wang, H., Wu, L., Aries, E., et al. (2012). Whole-cell bacterial bioreporter for actively searching and sensing of alkanes and oil spills. Microb. Biotech. 5, 87–97. doi: 10.1111/j.1751-7915.2011.00301.x
Zhang, D. Y., Fakhrullin, R. F., Ozmen, M., Wang, H., Wang, J., Paunov, V. N., et al. (2011). Functionalization of whole-cell bacterial reporters with magnetic nanoparticles. Microb. Biotechnol. 4, 89–97. doi: 10.1111/j.1751-7915.2010.00228.x
Zheng, Q., and Gregg-Jolly, L. A. (2004). Genes regulated in response to DNA damage in Acinetobacter sp strain ADP. Abstr. Gen. Meet. Am. Soc. Microbiol. 104:293.
Zhou, H., Wang, H., Huang, Y., and Fang, T. (2016). Characterization of pyrene degradation by halophilic Thalassospira sp strain TSL5-1 isolated from the coastal soil of Yellow Sea. China. Int. Biodeterior. Biodegrad. 107, 62–69. doi: 10.1016/j.ibiod.2015.10.022
Zilberstein, D. (2015). “Proteomic analysis of posttranslational modifications using iTRAQ in leishmania,” in Parasite Genomics Protocols, ed. C. Peacock (New York, NY: Springer), 261–268. doi: 10.1007/978-1-4939-1438-8_16
Keywords: Acinetobacter baylyi ADP1, DNA damage response, proteomics, carbon source, iTRAQ
Citation: Jiang B, Xing Y, Li G, Zhang N, Lian L, Sun G and Zhang D (2020) iTRAQ-Based Comparative Proteomic Analysis of Acinetobacter baylyi ADP1 Under DNA Damage in Relation to Different Carbon Sources. Front. Microbiol. 10:2906. doi: 10.3389/fmicb.2019.02906
Received: 20 June 2019; Accepted: 02 December 2019;
Published: 14 January 2020.
Edited by:
Biswarup Mukhopadhyay, Virginia Tech, United StatesReviewed by:
Veronica Godoy, Northeastern University, United StatesCopyright © 2020 Jiang, Xing, Li, Zhang, Lian, Sun and Zhang. This is an open-access article distributed under the terms of the Creative Commons Attribution License (CC BY). The use, distribution or reproduction in other forums is permitted, provided the original author(s) and the copyright owner(s) are credited and that the original publication in this journal is cited, in accordance with accepted academic practice. No use, distribution or reproduction is permitted which does not comply with these terms.
*Correspondence: Yi Xing, eGluZ3lpQHVzdGIuZWR1LmNu; Dayi Zhang, emhhbmdkYXlpQHRzaW5naHVhLm9yZy5jbg==; emhhbmdkYXlpQHRzaW5naHVhLmVkdS5jbg==
Disclaimer: All claims expressed in this article are solely those of the authors and do not necessarily represent those of their affiliated organizations, or those of the publisher, the editors and the reviewers. Any product that may be evaluated in this article or claim that may be made by its manufacturer is not guaranteed or endorsed by the publisher.
Research integrity at Frontiers
Learn more about the work of our research integrity team to safeguard the quality of each article we publish.