- 1Laboratorio de Biotecnología Aplicada, Facultad de Medicina Veterinaria, Universidad San Sebastián, Puerto Montt, Chile
- 2Chromatin and Disease Group, Cancer Epigenetics and Biology Programme (PEBC), Bellvitge Biomedical Research Institute (IDIBELL), Barcelona, Spain
- 3Epigenetics and Immune Disease Group, Josep Carreras Leukaemia Research Institute (IJC), Barcelona, Spain
- 4Departamento de Ingeniería Química y Bioprocesos, Escuela de Ingeniería, Pontificia Universidad Católica de Chile, Santiago, Chile
- 5Genomics and Health Area, Fundación para el Fomento de la Investigación Sanitaria y Biomédica de la Comunidad Valenciana (FISABIO)-Salud Pública, Valencia, Spain
- 6Integrative Systems Biology Institute, University of Valencia, CSIC, Valencia, Spain
- 7Biomedical Research Centre Network for Epidemiology and Public Health (CIBEResp), Madrid, Spain
Gut microbiota has been shown to have an important influence on host health. The microbial composition of the human gut microbiota is modulated by diet and other lifestyle habits and it has been reported that microbial diversity is altered in obese people. Obesity is a worldwide health problem that negatively impacts the quality of life. Currently, the widespread treatment for obesity is bariatric surgery. Interestingly, gut microbiota has been shown to be a relevant factor in effective weight loss after bariatric surgery. Since that the human gut microbiota of normal subjects differs between geographic regions, it is possible that rearrangements of the gut microbiota in dysbiosis context are also region-specific. To better understand how gut microbiota contribute to obesity, this study compared the composition of the human gut microbiota of obese and lean people from six different regions and showed that the microbiota compositions in the context of obesity were specific to each studied geographic location. Furthermore, we analyzed the functional patterns using shotgun DNA metagenomic sequencing and compared the results with other obesity-related metagenomic studies, we observed that microbial contribution to functional pathways were country-specific. Nevertheless, our study showed that although microbial composition of obese patients was country-specific, the overall metabolic functions appeared to be the same between countries, indicating that different microbiota components contribute to similar metabolic outcomes to yield functional redundancy. Furthermore, we studied the microbiota functional changes of obese patients after bariatric surgery, by shotgun metagenomics sequencing and observed that changes in functional pathways were specific to the type of obesity treatment. In all, our study provides new insights into the differences and similarities of obese gut microbiota in relation to geographic location and obesity treatments.
Introduction
It has been established that the composition of human gut microbiota greatly influences human health (Carding et al., 2015, reviewed by Hall et al., 2017). Although the microbiota composition of each individual is relatively stable across adult life, it varies widely between individuals (Lozupone et al., 2012; Kolde et al., 2018). It has been observed that the human gut microbiota is primarily dominated by the phyla Firmicutes and Bacteroidetes (Zhernakova et al., 2016), with lesser contribution from Actinobacteria, Proteobacteria and Verrucomicrobia (Qin et al., 2010). Nevertheless, the microbiota composition differs geographically, primarily based on a variety of factors including host genetics, dietary habits, age, geographic location and lifestyle (Yatsunenko et al., 2012; Chilton, 2014; Nishijima et al., 2016; Fujio-Vejar et al., 2017; Gupta et al., 2017). To date, most of the studies on human gut microbiota have focused on populations from North America and Europe and although several of studies have demonstrated associations between microbiota alterations and diseases, including obesity, the specific contribution of these alterations to treatment response and how they differ across geographic locations still need to be envisioned.
Worldwide, obesity has nearly tripled since 1980 (Stevens et al., 2012). Information published by the World Health Organization (2016) shows that more than 1.9 billion adults, 18 years and older, have a body mass index (BMI) above 25 kg/m2 among which, over 650 million have a BMI > 30 kg/m2, hence classifying them as obese. Overweight and obesity are defined as abnormal or excessive fat accumulation due to environmental and genetic factors (Angelakis et al., 2012). While overweight individuals haves a BMI range between 25 and less than 30 kg/m2, obesity is classified by BMI as: obesity grade 1 (30 to 35 kg/m2), grade 2 (35 to 40 kg/m2) and grade 3 (40 to 60 kg/m2) (NHLBI Expert Panel, 1998). Diverse studies have associated obesity with altered gut microbiota and reduced functional potential (Ley et al., 2006; Turnbaugh et al., 2006; Tremaroli and Backhed, 2012; Karlsson et al., 2013; Le Chatelier et al., 2013; Damms-Machado et al., 2015; Haro et al., 2016; Medina et al., 2017), which can be partially reversed after surgical intervention (Palleja et al., 2016). It has been originally observed that the relative abundance of Firmicutes and Bacteroidetes can be altered in obese patients, where an over-representation of Firmicutes is observed, in contrast to their lean counterparts (Ley et al., 2006; Turnbaugh et al., 2009; Million et al., 2012; Walters et al., 2014; Kasai et al., 2015; Haro et al., 2016). These taxonomic differences between lean and obese subjects may contribute to the development and perpetuation of obesity in several ways, including fat storage, regulation of energy metabolism, energy extraction from short chain fatty acids, increased low-grade inflammation and altered bile acid metabolism (Qin et al., 2012; Karlsson et al., 2013; Khan et al., 2016). However, a recent study focusing on the meta-analysis of previously published data using forest machine learning models showed no significant differences in gut microbiota composition between obese and healthy individuals (Sze and Schloss, 2016). Nevertheless, further research is required to unravel the exact role of gut microbiota composition in obesity context, and the factors, including geographical location, that may have an influence on not only differential microbial abundance but also long-term patient outcome.
Gastric surgical procedures, commonly known as bariatric surgery, have been successful in mediating long-term weight loss and reducing the incidence of related comorbidities (Sjöström, 2008; Eldar et al., 2011). Roux-en-Y gastric bypass (RYGB) is one of the most common bariatric surgery procedures in the United States (Smoot et al., 2006), where a small stomach pouch is connected to the proximal jejunum to directly bypass food to the small intestine, resulting in restrictive and malabsorptive nutrient intake (Tice et al., 2008; Tran et al., 2016). Another common bariatric surgery is sleeve gastrectomy (SG), where a significant portion of the stomach is removed to decrease its volume, leading to a significant reduction in the amount of food consumed (Gumbs et al., 2007). Since their invention, a number of studies have observed changes in obesity-associated microbiota and functional gene richness following different types of bariatric surgery (Zhang et al., 2009; Graessler et al., 2013; Kong et al., 2013; Tremaroli et al., 2015; Shao et al., 2016; Ilhan et al., 2017; Medina et al., 2017; Murphy et al., 2017). Specific changes following surgical intervention include an increase in Proteobacteria (Escherichia coli, Enterobacter spp.), changes in Bacteroides and Prevotella abundance, accompanied by an increase in Akkermansia and a decline in Clostridium genus, and global changes in Firmicutes/Bacteroidetes phyla ratios (Zhang et al., 2009; Furet et al., 2010; Li et al., 2011; Kong et al., 2013; Damms-Machado et al., 2015; Louis et al., 2016; Palleja et al., 2016; Ilhan et al., 2017; Medina et al., 2017). These microbial changes following bariatric surgery have shown to mediate different outcomes and, interestingly, such changes appear to depend on the type of surgery performed (Ilhan et al., 2017; Medina et al., 2017; Murphy et al., 2017). Specifically, metagenomic studies following RYGB showed an increase in pathways involving aerobic respiration and glutathione transfer and metabolism (Palleja et al., 2016). Both observations were in agreement with the increase in Proteobacteria phylum abundance, driven by facultative anaerobes, such as E. coli, as a result of lower gastric acid exposure during stomach transit. In the same study, the authors found an increase in the pathways that degrade putrescine to succinate to produce gamma-aminobutyric acid (GABA) as a byproduct. GABA is known to act on receptors in the hypothalamus to promote satiety and, additionally, is thought to promote GLP-1 release, a positive regulator of GABA production by pancreatic beta-cells, which provides further involvement of this pathway following RYGB (Palleja et al., 2016). In addition, a complementary study conducted 9 years after RYGB intervention showed that bacterial rearrangements were stable in the long run and surgically altered microbiota promoted reduced fat deposition in recipient mice (Tremaroli et al., 2015).
In this study, we compared the gut microbiota composition and functional patterns of obese subjects from Chile with published data from Italy, Denmark, United States, France, and Saudi Arabia, in order to better understand the microbiota contribution to obesity. We found obese subjects to show geographic specificity in regards to relative microbiota abundance, similar to what has been previously observed in healthy individuals. Interestingly, the gut microbiota of obese patients did not display differential enrichment of functional pathways between countries, indicating that the geography-specific microbial compositions converge to perform similar functions in obese individuals. Furthermore, this study analyzed the metagenomic profiles of Chilean patients subjected to gastric bypass and sleeve gastrectomy and observed changes in the functional capacity of gut microbiota after surgery. Specifically, we identified Akkermansia muciniphila as one of the bacteria that drives the change in metabolic pathways after surgical intervention for obesity in Chilean patients.
Materials and Methods
Sample and DNA Raw Data Collection
DNA raw data sequences from the stool of obese and lean subjects were obtained from studies carried out in Chile (Medina et al., 2017; Thomson et al., 2019), Italy (Tremaroli et al., 2015), Denmark (Palleja et al., 2016), United States (Ilhan et al., 2017), France and Saudi Arabia (Yasir et al., 2015), described in Table 1 and Supplementary Table S1. In addition, 12 DNA stool samples from Chilean obese patients before and after treatment obtained from a previous study (Medina et al., 2017) were sequenced using shotgun metagenomics. All experiments were conducted in accordance with the Declaration of Helsinki and approved by the Ethics Committee of the Faculty of Medicine, Pontifical Catholic University of Chile (n° 15-337).
Taxonomic Profiling From 16S rDNA Gene Amplicon Sequencing
Raw data of DNA sequences belonging to different studies were downloaded from ENA-EMBL or SRA-NCBI databases (Supplementary Table S1). To access the microbiota taxa and abundance, in this study we re-analyzed all raw data sequences using Microbiome Helper v2.3 OVA pipeline (Comeau et al., 2017). Briefly, MiSeq paired-end sequences were joined using PEAR (Zhang et al., 2014). Joined sequences were filtered by quality and length, in which demultiplexing and barcode depletion were performed using Microbiome Helper and QIIME v1.9.1 scripts (Caporaso et al., 2010; Navas-Molina et al., 2013; Comeau et al., 2017). Chimera sequences were filtered using the Vsearch tool (Rognes et al., 2016). Operational taxonomic units (OTUs) were picked by open-reference command and defined by clustering at 3% divergence (97% similarity) using the GreenGenes database release 08-2013 as reference (DeSantis et al., 2006; McDonald et al., 2012). Diversity analyses were performed using QIIME v1.9.1 scripts under Microbiome Helper v2.3 environment. The sequencing depth for even sub-sampling and maximum rarefaction depth was at least 10000 counts/sample, regard the minimum value obtained after OTU-picking for each data set (Supplementary Table S2). Predictive metagenomic functional profiling of microbial composition were performed using PICRUSt (Langille et al., 2013), following the instructions provided in their Metagenomic Prediction Tutorial. KEGG Orthology analyses were performed using the “ko_to_pathway_map” PICRUSt database to identify the enrichment of metabolic pathways.
Taxonomic abundance and metagenomic prediction tables were exported to R environment (R Core Team, 2013) for statistical analysis and figures were represented using the package LSD Lots of Superior Depictions (Schwalb et al., 2011). Volcano plots were constructed using the adjusted FDR p-value obtained from the unpaired t-test comparisons and the fold change of each condition. The taxonomic processed data obtained from QIIME and the metadata for the raw data files used in this study are exhibited in Supplementary Table S2.
The PICRUSt functional prediction was validated comparing the KEGG Orthology abundances obtained with the Uniref90 metagenomic abundances from the HUMAnN2 output. For this, the metagenomic Uniref90 table was converted to KEGG Orthology using the script humann2_regroup_table from HUMAnN2 (Abubucker et al., 2012). Both KEGG Orthology datasets were merged by row and normalized using quantile normalization to compare linear dependence between each data set using Pearson and pairwise Spearman rank correlation in the R environment (R Core Team, 2013).
Functional Annotation and Metagenomic Profiling of Fecal DNA
A total of 12 DNA stool samples were sequenced using the Illumina HiSeq next-generation sequencing platform carried out at Genoma Mayor (Universidad Mayor, Chile) with an output of 2 × 100 pb and 20 × 106 paired-end reads per sample. The raw data produced from DNA sequencing in this study were stored at the ENA-EMBL database under the accession number PRJEB29060. To evaluate the taxa abundance and metagenomic composition, all metagenomic raw sequences were analyzed in parallel using the Microbiome Helper v2.3 environment following the metagenomics SOP v2 tutorial. This pipeline allowed the calculation of microbiota abundance using functions from MetaPhlAn2 (Truong et al., 2015) and functional profile using HUMAnN2 (Abubucker et al., 2012). DNA sequence quality control was performed using KneadData (McIver et al., 2018), in which low quality sequences were first removed by Trimmomatic (Bolger et al., 2014), followed by the application of Bowtie2 to screen out the contaminant DNA sequences mainly from human and viruses (Langmead and Salzberg, 2012). Both programs were run simultaneously for all samples using the GNU Parallel tool to repeat concatenate the entire process for each data set (First and Job, 2011). The paired-end files were merged using the script provided by the Microbiome Helper pipeline and then taxonomic and functional profiling were performed using MetaPhlAn2 and HUMAnN2, respectively. Gene family and pathway abundances were normalized for each sample and represented as a percentage. The resulting tables were exported to the R environment (R Core Team, 2013) for statistical analysis and figure representation. Metadata sets used for DNA shotgun sequencing to obtain stratified and unstratified metagenomic profiles are listed in Supplementary Table S3.
Data Comparison and Statistical Analysis
Statistical analyses were conducted using the R environment (R Core Team, 2013) version 3.4.4 (2018-03-15). Before calculations between countries, data sets were scaled proportionally, and using the R package preprocessCore (Bolstad, 2019), quantile normalization was performed to reduce batch effects between data belonging to different sources as was previously used for genome-wide analyses (Sun et al., 2011; Guo et al., 2014; Fei et al., 2018). For differential analysis of group variance between lean and obese microbiota abundance, we used Canonical Correspondence Analysis (CCA, a.k.a. constrained correspondence analysis) and Adonis tests, in which both were calculated using the R package vegan (Oksanen et al., 2018). The Adonis test was used for permutational multivariate analysis of variance using distance matrices, in which samples were fitted to linear models to calculate the whole compositional variability taking into account different sources of variation as well as the interactions between them. Spearman’s rank correlation analyses were used to assess taxa abundance or functional abundance associations between the samples groups. To analyze differences in group means between lean and obese microbiota abundance, we applied Wilcoxon sum rank test with FDR adjustment utilizing the Benjamini Hochberg (BH) method. This was performed using the R command p.adjust(), in which we considered tests with FDR < 0.05 to be significant. In order to identify differentially enriched biomarkers among the compared groups, we applied the LEfSe analytic method using the online interface Galaxy1 (Segata et al., 2011). Statistical differences between the KEGG Orthology abundances were calculated using the unpaired t-test and adjusted by BH FDR as described above. –log10 FDR was plotted against log2 ratio of each country in respect to Chile and represented using the R package LSD Lots of Superior Depictions (Schwalb et al., 2011).
Results
Gut Microbial Diversity Is Specific to Geographic Locations
The composition and function of the human gut microbiota represent one of most important factors involved in obesity and its treatment (Ley et al., 2006; Tremaroli and Backhed, 2012; Karlsson et al., 2013; Le Chatelier et al., 2013; Damms-Machado et al., 2015; Medina et al., 2017). It has been previously established that the human gut microbiota displays pronounced differences between individuals residing in distinct geographic locations (Yatsunenko et al., 2012; Fujio-Vejar et al., 2017; Gupta et al., 2017). It is therefore of great interest to compare the microbial diversity of obese individuals from different geographical locations around the world. In this line of research, this study compared the gut microbial diversity of obese and lean subjects from Chile (Medina et al., 2017; Thomson et al., 2019) with data published by other studies in different regions around the world, namely United States (Ilhan et al., 2017), France and Saudi Arabia (Yasir et al., 2015), described in Table 1. Taxonomic microbiota abundance were collected by sequencing the 16S rDNA hypervariable regions V3–V4 or V4–V5 using the Illumina MiSeq platform (Supplementary Table S1), and raw data was processed as described in materials and methods. Taxonomic abundance comparisons at the genus level from the data obtained showed significant diversity in microbiota composition in lean people as previously reported (Yatsunenko et al., 2012; Nishijima et al., 2016; Fujio-Vejar et al., 2017; Gupta et al., 2017), but also is country specific in gut microbiota of obese subjects. Specifically, data variance analysis using CCA and Adonis tests showed significant differences (p-value < 0.05) in the gut microbiota composition between Chile, United States, France, and Saudi Arabia, in which the lean and obese microbiota were clustered by country (Figures 1A,B). Utilizing a different approach, pairwise Spearman rank correlation, we further demonstrated that the taxonomic abundance correlation of obese gut microbiota differed between countries (Supplementary Figure S1A). Nevertheless, although obese microbiota composition is clustered by country, there is significant inter-individual variability between subjects, indicated by high dispersion patterns observed in data variance analyses (Figure 1). In this regard, although some proximity is observed between France and Saudi Arabia microbiota, CCA and Adonis test variance analyses between the two countries shows a significant statistical difference (Supplementary Figure S1B). In all, these comparisons indicate that the microbiota compositions of obese patients and lean subjects were specific to each analyzed geographic location and displayed significant differences between countries. Comparing taxonomic abundance of obese individuals with their lean counterparts from the same country we observed significant differences by multivariate variance analyses for Chile, France, and Saudi Arabia (CCA p-value < 0.05); however, no statistically significant differences were observed for United States (Supplementary Figure S2). Wilcoxon sum-rank tests showed significant differences (FDR < 0.05) between lean and obese microbiota in Chile and France data sets, but not in United States and Saudi Arabia. Interestingly, although CCA showed clustering by country in both cases, France and Chile shared some genus changes between lean and obese microbiota (Megasphaera, Veillonella, Adlercreutzia and Lachnospira) (Supplementary Table S2). In addition, heatmap clustering by complete linkage method and the stacked bar plot of taxonomic abundances showed differential patterns not only between obese and lean subjects, but also between countries, which again highlights our observation that taxonomic microbial distribution is specific to each country (Figure 2).
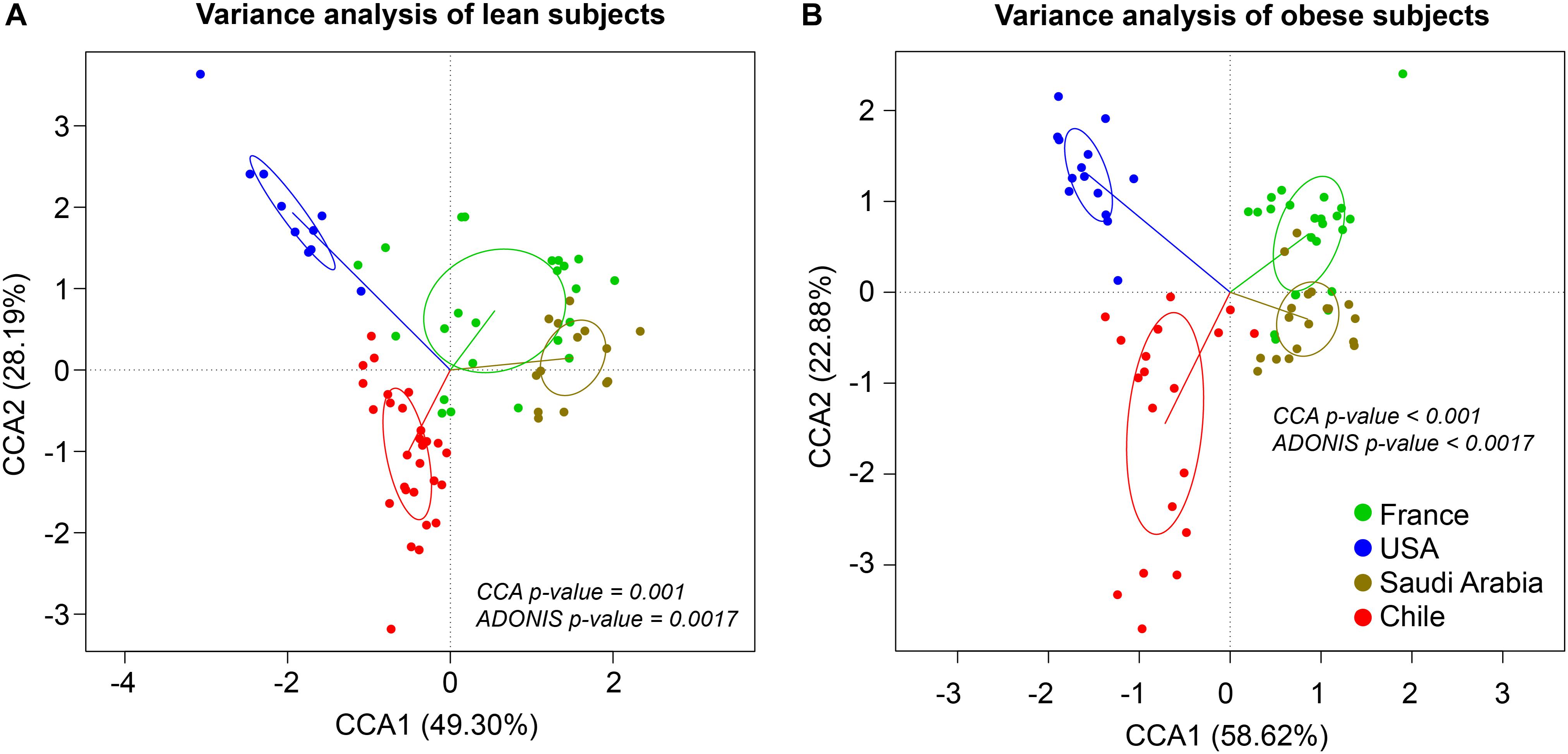
Figure 1. Taxonomic abundance comparison at genus level from 16S rDNA sequencing. Constrained Correspondence Analysis (CCA) and Adonis test were performed to assess the variance in microbiota profiles at the genus level in lean (A) and obese (B) subjects. Vectors represent quantitative explanatory variables with confidence circles depicted for each country. Corresponding p-values are shown for each analysis and p-value < 0.05 was considered statistically significant. x- and y-axis show CCA1 and CCA2 components, respectively. Green, blue, brown and red points indicate individuals from France, United States, Saudi Arabia, and Chile, respectively.
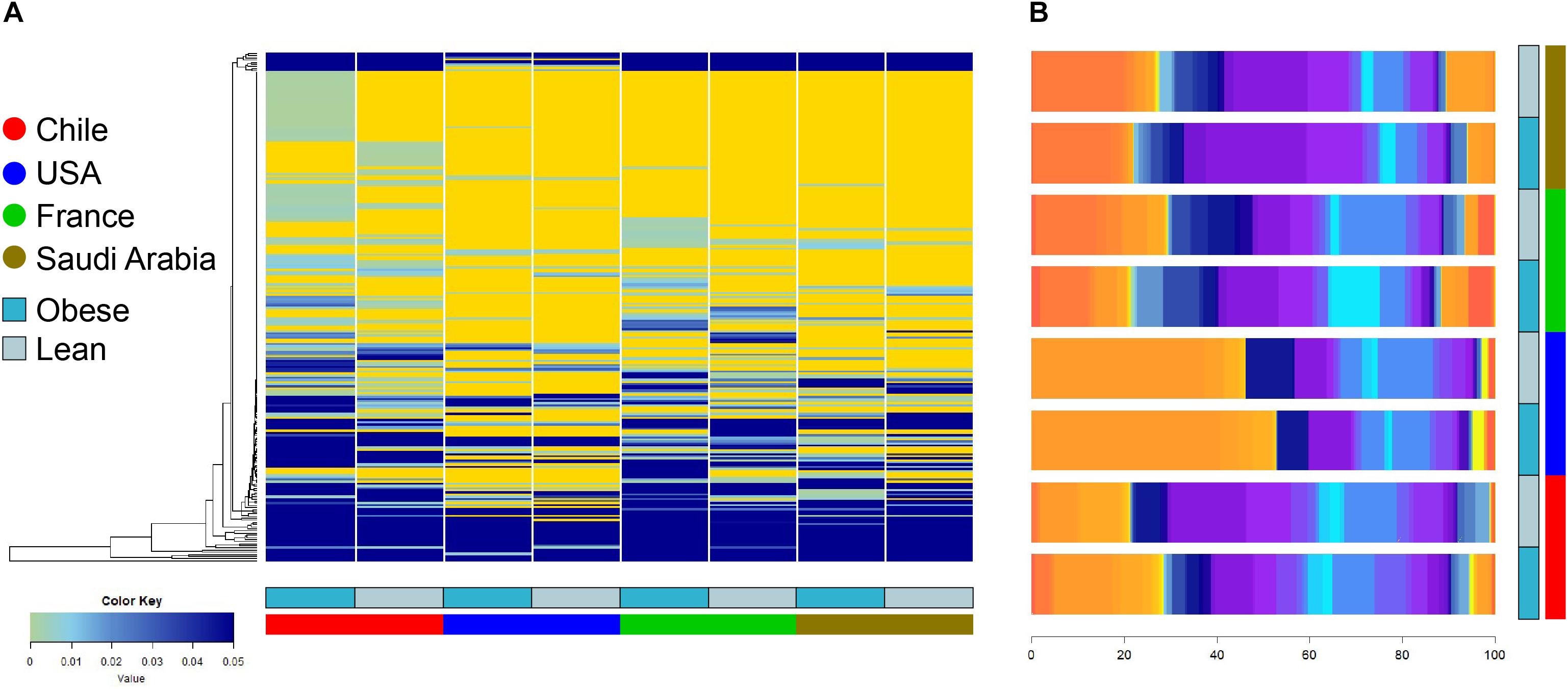
Figure 2. Heatmap clustering and stacked bar plot of taxonomic abundances of lean and obese subjects at genus level. (A) Taxonomy abundance at genus level between obese and lean subjects were clustered using complete linkage method. Values indicate abundance of microbiota at the genus level. (B) Average taxonomy abundance at genus level were represented as stacked bar plot to of lean and obese subject of each country. x-axis depicts percentage of abundance of each taxonomy. Dark and light blue represent obese and lean subjects, respectively. Red, blue, green, and brown represent individuals from Chile, United States, France, and Saudi Arabia, respectively.
Next, using the PICRUSt tool (Langille et al., 2013) designed to infer the functional patterns from taxonomic profile, we compared predicted KEGG Orthology (KO) enrichment of obese subjects from United States, France, and Saudi Arabia with the KO obtained from the obese Chilean data as reference in order to identify potential functional differences. Here, we observed significant differences (FDR < 0.05) in enriched pathways for all the countries studied with respect to Chile (Figure 3). Downregulated pathways in Saudi Arabia displayed an enrichment in translation and energy metabolism, whereas the United States and France showed a downregulation in membrane transport. For upregulated pathways, United States showed a specific enrichment in cellular processing and signaling and amino acid metabolism, whereas France and Saudi Arabia were found to enrich in pathways involving membrane transport (Supplementary Figure S3). In summary, the differences observed by functional prediction suggested that the human gut metagenome of obese subjects, like their healthy counterparts (Yatsunenko et al., 2012), may differ according to the geographical location.
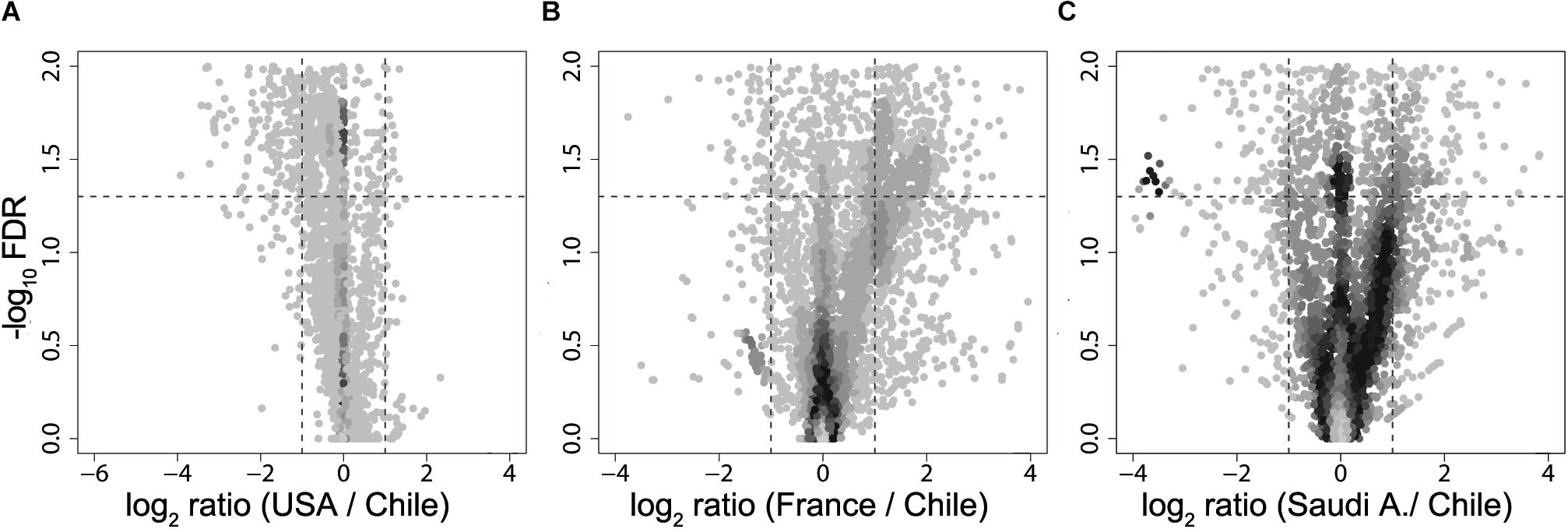
Figure 3. Volcano plot of KEGG Orthology abundances obtained from metagenomic simulation. Fold change of United States (A), France (B), and Saudi Arabia (C) of KO abundance with regard to Chile are plotted against –log10 of FDR. Dotted lines in the x-axis denotes a two-fold change, while dotted lines in the y-axis delimit a FDR value of 0.05.
Metagenomic Variations Across Regional Location in the Context of Obesity
Our initial analysis utilizing 16S rDNA amplicon sequencing showed marked differences between the compositions of the gut microbiota of obese subjects from different countries, therefore, to further validate our initial observation, we analyzed stool samples of 6 Chilean pre-treatment obese patients obtained from a previous study (Medina et al., 2017) by shotgun DNA sequencing in order to dissect the contribution of metabolic functional changes and microbiota compositions. Additionally, we compared the results obtained from the Chilean cohort with similar studies carried out from patients in Italy (Tremaroli et al., 2015) and Denmark (Palleja et al., 2016), described in Table 1. All raw data were analyzed using the Metagenomic SOAP v2 from Microbiome Helper (Comeau et al., 2017) as described in “Materials and Methods.” First, we compared the functional composition of obese subjects from Chile (n = 6), Italy (n = 7) and Denmark (n = 7) before any medical intervention utilizing CCA, Adonis tests and pairwise Spearman rank correlation. Initially, we performed an unstratified analysis, which corresponds to the analysis of metabolic pathway enrichment without taking into account microbial composition, and observed no statistically significant differences between the three countries using CCA analysis and Adonis tests (p-value > 0.05), in which the variance plot displayed little dispersion between the samples from different countries (Figure 4A). Furthermore, the pairwise Spearman rank correlation showed overall good correlation between the samples of all datasets (Figure 4B). Hence, in contrast to PICRUSt prediction, these results showed that the overall microbial functionality had no differences between obese subjects belonging to different world regions. Subsequently, we performed a stratified data analysis, a type of analysis that distinguishes the functional contribution of each bacterial species to the overall metabolic pathways. Interestingly, stratified data, unlike unstratified analysis, showed significant dispersion between the countries (Figure 4C), and no correlation between datasets was observed (Figure 4D), indicating that individual microbial metagenomic contribution to overall functional pathways is different between countries, in agreement with functional inferences obtained by PICRUSt (Figure 3). Also, we use the Spearman rank correlation values obtained to compare statistically into-countries and cross-country associations in both unstratified and stratified data, finding no statistical differences between Spearman values (p-value FDR adjusted of 0.75 and 1, respectively). Utilizing linear discriminant analysis (LDA) to quantify phylogenetic and functional diversity, pathways over 1.5 LDA score were found to be differentially enriched between countries in both stratified and unstratified approaches (Figure 5). In addition, we validated the PICRUSt functional prediction comparing KEGG Orthology obtained with HUMAnN2 output from metagenomic data using Pearson and pairwise Spearman rank Correlation, with both showing linear dependence between 3590 common KEGG Orthology families (Supplementary Figure S4 and Supplementary Table S5), which proved the relationship between in silico predictions and our biological findings as was previously demonstrated by PICRUSt authors (Langille et al., 2013). Altogether, these results suggested that microbial contribution to functional pathways was country-specific, indicating that there was redundancy in the functions of these distinct microbial species found in obese subjects from different countries, in which different microbiota components contribute to obtain similar metabolic outcomes.
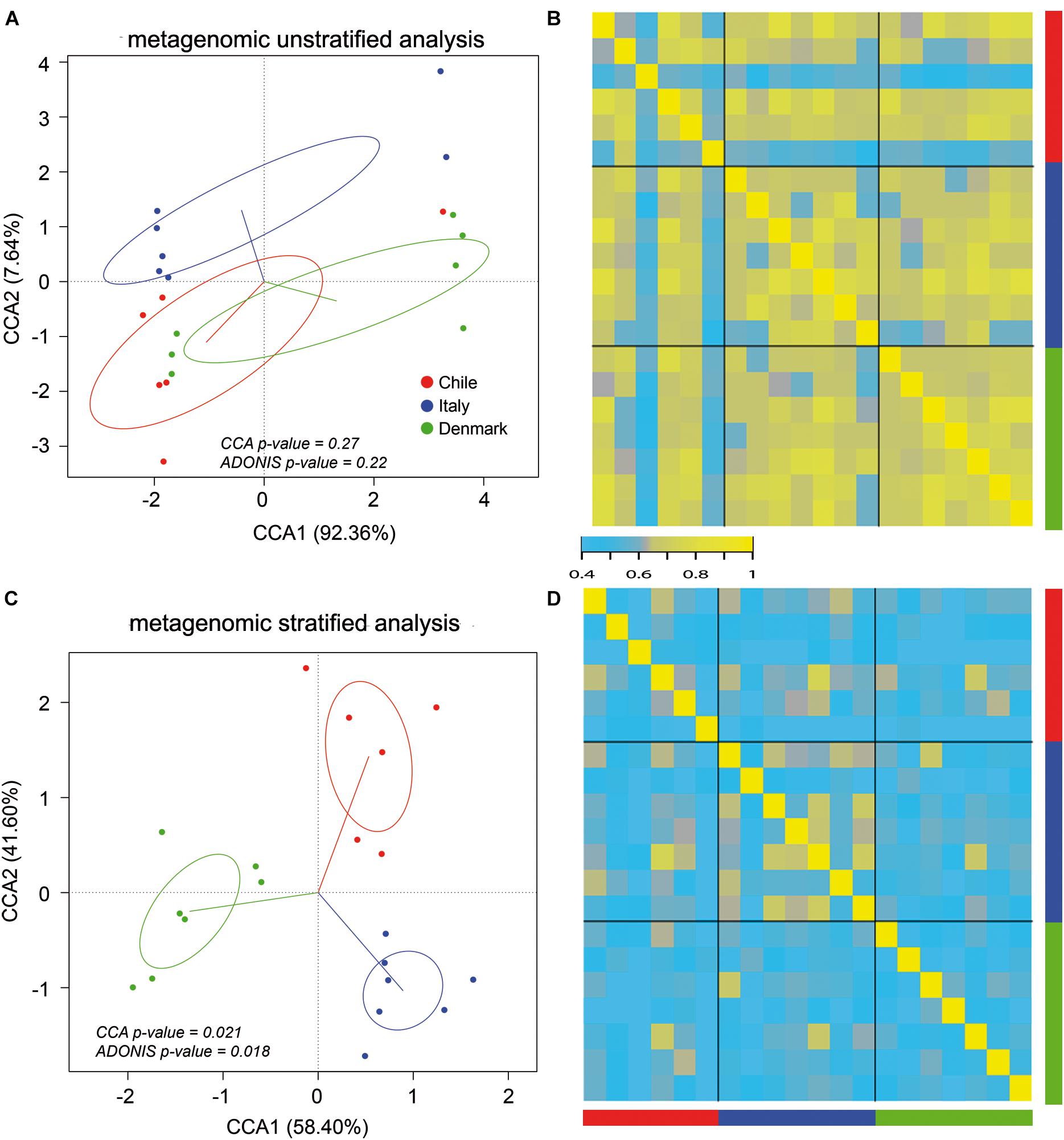
Figure 4. Stratified and unstratified functional profiles of obese gut microbiota from three different countries. Unstratified (A,B) and stratified (C,D) analyses of obese gut microbiota abundance for Chile (red), Italy (blue) and Denmark (green). Scatterplots (A,C) represent CCA and Adonis test analysis, and heatmap (B,D) represent pairwise Spearman rank correlation of functional microbial abundance between countries.
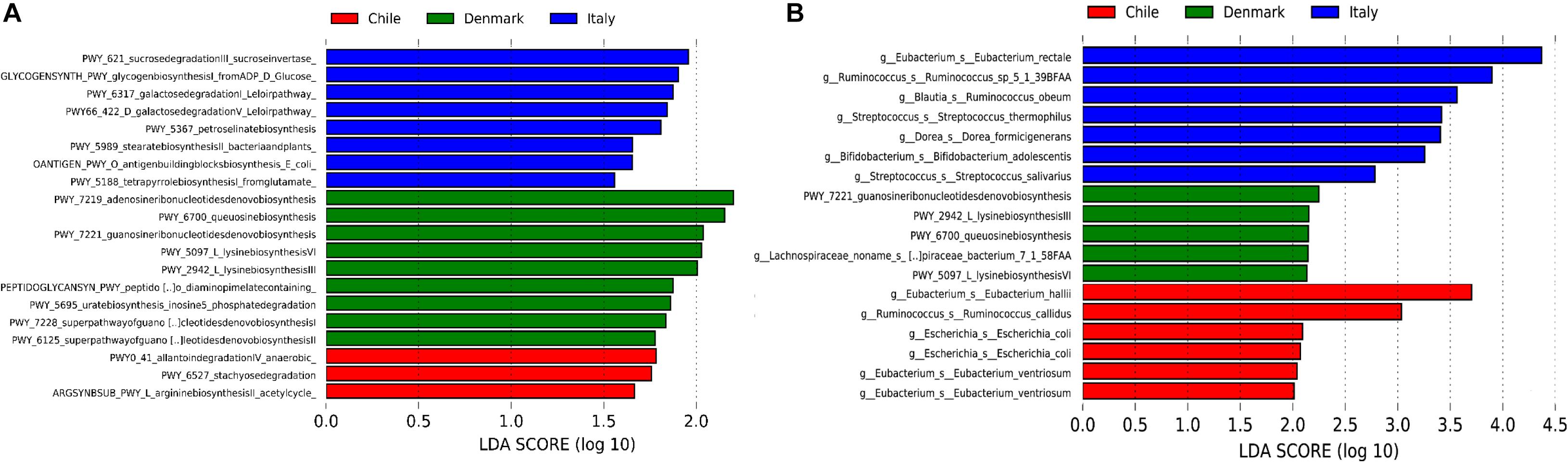
Figure 5. Linear discriminant analysis (LDA) effect size (LEfSe) of metabolic pathways. Unstratified (A) and stratified (B) functional enrichment analyses from microbiome of obese subjects belonging to Chile (red, n = 6), Denmark (green, n = 7) and Italy (blue, n = 7). Pathways with LDA scores higher than 1.5 (A) or 2 (B) are shown. For stratified functional enrichment (B), unmapped/unintegrated reads are denoted as the bacterial taxa in which the pathways belong to.
Furthermore, microbial taxonomy abundance data obtained from shotgun DNA sequencing showed that Chilean microbiota composition from obese subjects was different compared to Italian and Danish cohorts (Tremaroli et al., 2015; Palleja et al., 2016). CCA analysis and Adonis tests showed significant differences between the microbiota of analyzed subjects at species level (Supplementary Figure S5A), which strengthens our previous observations obtained from the 16S rDNA sequencing taxonomic comparison at genus level (Figure 1B). In addition, LDA analysis showed differences in species abundance between the three microbial datasets species with and LDA scores higher than 2, reinforcing the observation that the microbiota under dysbiosis has different compositions specific to each geographical location (Supplementary Figure S5B).
Gut Microbiota Functionality Changes Following Bariatric Surgery in Chilean Subjects
Several studies have shown that the regulation of energy and fat storage is influenced by intestinal microbes, and this composition may contribute to obesity (Qin et al., 2012; Karlsson et al., 2013). In this regard, it has been reported that human gut microbiota composition varies between obese patients who underwent different types of surgical intervention (Zhang et al., 2009; Furet et al., 2010; Schloss, 2016; Ilhan et al., 2017; Medina et al., 2017; Murphy et al., 2017). Moreover, several of these studies describe functional changes after treatment and its prevalence across time (Tremaroli et al., 2015; Palleja et al., 2016). More specifically, they report an increase in Proteobacteria abundance and facultative anaerobes, such as E. coli, and an enrichment in microbial pathways involving aerobic respiration, glutathione and gamma-aminobutyric acid metabolism. Similarly, in a previous study from our laboratory using Chilean obese patients, we observed significant changes in the human gut microbiota, and these changes were specific to the type of surgery performed (Medina et al., 2017). Here, utilizing the same cohort, we performed shotgun DNA sequencing of stool samples before and after surgical intervention in order to analyze changes in taxonomic composition (Medina et al., 2017). Although no statistical testing was performed due to insufficient cohort number (n = 2 for each group), our results suggested that the two kinds of bariatric surgery mediated different rearrangements of functional pathways. We found stratified and unstratified functional changes of several folds, 6 months following either Roux-en-Y gastric bypass (RYGB) or sleeve gastrectomy (SG) interventions (Figure 6). More specifically, unstratified functional changes were more pronounced in RYGB–treated patients compared to SG-treated patients, represented by higher data variance with an increase in log2 fold change (Figures 6B,D). Functional changes in unstratified RYGB data suggested an increase in pathways related with acetyl-CoA biosynthesis, trehalose degradation, GABA shunt and phospholipid remodeling. Contrastingly, changes observed in SG intervention included an increase in metabolic pathways such as fatty acid β-oxidation, TCA cycle and acetyl-CoA biosynthesis (Supplementary Table S4). Top stratified functional changes after RYGB were mainly driven by A. muciniphila, E. coli, Bacteroides vulgatus, Eubacterium siraeum and Streptococcus salivarius, while in SG functional changes were driven by Bacteroides cellulosilyticus, S. salivarius, Eubacterium eligens, Lactococcus lactis, Alistipes finegoldii, E. coli and A. muciniphila (Supplementary Table S4). Altogether, these results suggested that bariatric surgery in Chilean patients also caused functional rearrangement in microbiota, in concordance to previous metagenomic studies (Graessler et al., 2013; Tremaroli et al., 2015; Palleja et al., 2016), and these changes appeared to be specific to the surgery performed.
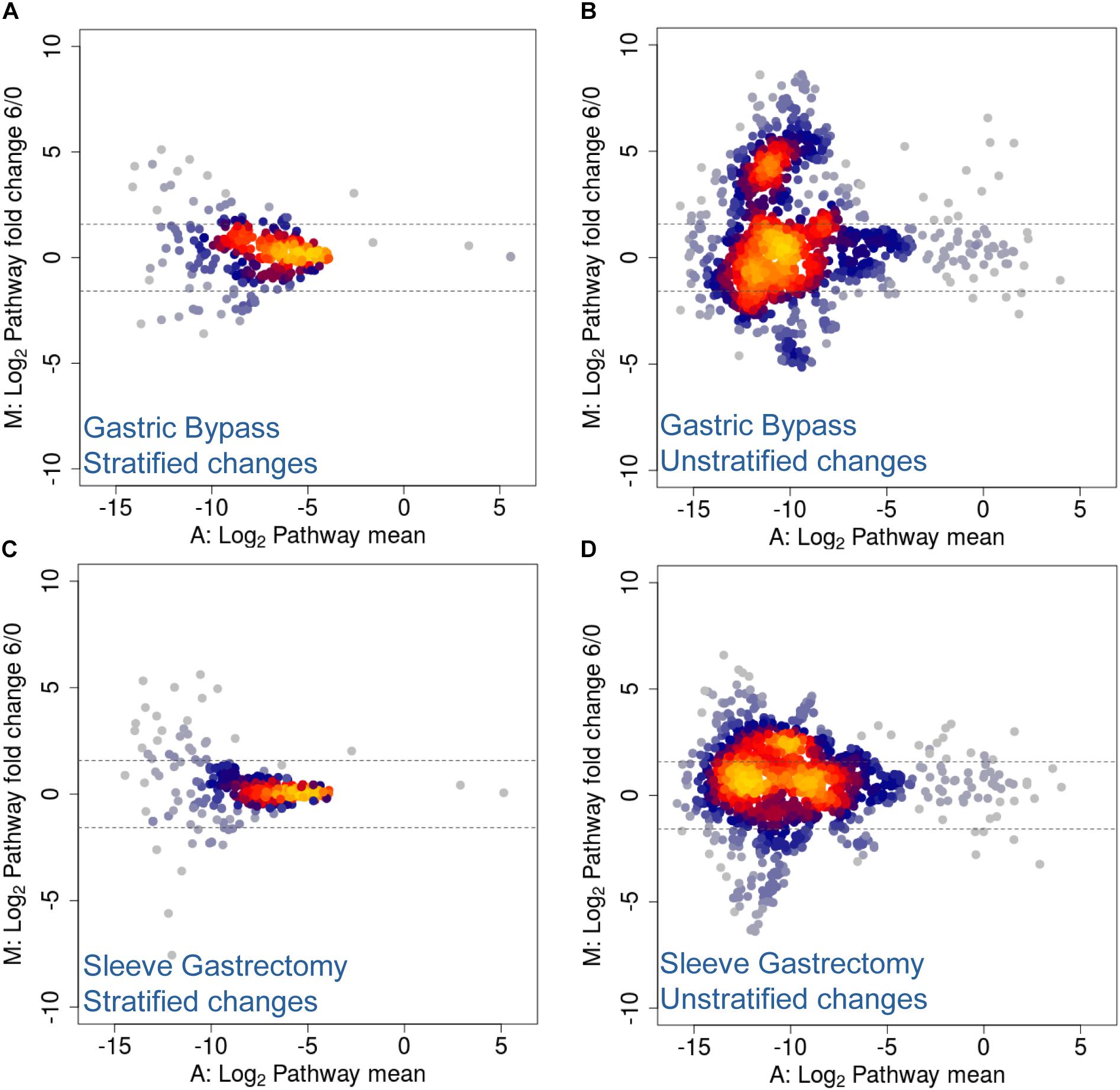
Figure 6. MA Plots for stratified and unstratified functional changes after bariatric surgery in Chilean patients. (A,B) Gastric bypass and (C,D) sleeve gastrectomy stratified (A,C) and unstratified (B,D) functional changes 6 months post-surgery. Values are expressed as mean (x-axis) and fold change (y-axis), and represented as log2 values. Dotted lines denote a two-fold change.
Discussion
Obesity is a world-wide health problem whose global prevalence has increased at an accelerated rate since 1980 (Stevens et al., 2012). It has been previously described that the composition and functionality of human gut microbiota plays a crucial role in mediating both disease and recovery following medical intervention (Turnbaugh et al., 2006; Tremaroli and Backhed, 2012; Karlsson et al., 2013; Le Chatelier et al., 2013; Damms-Machado et al., 2015). This study compared the gut microbiota compositions of obese subjects from Chile with previously published data from the United States, France, and Saudi Arabia using 16S rDNA Illuminia MiSeq, as well as from Italy and Denmark using Illumina HiSeq shotgun metagenomics, and found that the microbiota composition differs significantly between obese and lean subjects from these different countries, showing country-specific clustering. It has previously been described that a number of factors can modulate the composition of intestinal microbiota, one of which is geographic origin (reviewed by Rojo et al., 2017). A previous study compared the microbiota composition of a group of healthy subjects from France with Saudi Arabia by 16S rDNA sequencing and suggested that the dietary habits of different regions or countries can generate changes in intestinal microbiota (Yasir et al., 2015). This idea was reinforced by other studies suggesting that different types of diets directly change microbiota composition (Wu et al., 2011; Fava et al., 2013). In fact, mice subjected to obesogenic diets displayed significant functional and taxonomic modifications in their gut microbiota (Tran et al., 2019). Other studies show that genetics play a secondary role compared to environmental factors (Gupta et al., 2017; Jones et al., 2018; Rothschild et al., 2018). However, twin studies revealed more highly correlated microbiota compositions between monozygotic twins than dizygotic twins (Goodrich et al., 2014). Given subjects belonging to close location have less genetic variance than subjects from distant regions, this may also contribute to the differences in microbiota composition observed between geographical origins.
Our work attempted to indicate the need for the global study of human microbiota for a proper assessment of the microbial contribution in respect to geographic distribution. In this context, we compared the taxonomic gut microbiota abundance of lean and obese at genus level using multivariate variance analysis and non-parametric mean comparison. Although, using Wilcoxon rank test, we found different statistical changes in France and Chile gut microbiota, this was, however, not the case for United States and Saudi Arabia. Our results suggest a country-specific signature for both lean and obese microbiota. Nevertheless, we cannot discard the possibility that our observations may be influenced by technical and methodological factors. First, it is known that non-parametric tests have less chance of detecting a true effect where one exists (Whitley and Ball, 2002). Hence, utilizing a different statistical approach to analyze differences in variance (CAA and Adonis test) between lean and obese subjects in France, Chile, and Saudi Arabia, the subsequent results support the notion that obese versus lean microbiota is different between countries. Second, we cannot discard that this observation may be relatively influenced by the methodology differences between studies and also may be confounded by large interpersonal variation. A meta-analysis of the association between differences in the microbiome and obesity status made between 10 published studies found that is difficult to classify a subject as obese by its microbiota composition, suggesting the possibility that each individual has their own bacterial signatures (Sze and Schloss, 2016). Another meta-analysis that compared lean and obese subject from five published studies also found that signatures of obesity were not consistent between studies, as just one of the 5 studies analyzed showed diversity differences between lean and obese subject (Walters et al., 2014). Nevertheless, the authors declared that it was possible to assess differences between lean and obese subjects when they changed the method to identify OTUs (from “close reference” to “pick de novo”) or used supervised learning tools to categorize subjects according to lean and obese states with considerable accuracy (Walters et al., 2014). Altogether, these observations suggest that geographical differences between lean and obese subjects requires additional validation using an international cohort that not only takes into account confounding factors but maintain the same experimental design.
Our study performed a functional inference analysis based on the data obtained by 16S rDNA sequencing and observed significant differences in KEGG Orthology enrichment in samples obtained from France, United States, and Saudi Arabia compared to Chile. These results suggested the microbial differences observed were associated with changes in functional pathways, however, this kind of analysis was unable to differentiate the microbial contribution of each microorganism species to the identified functional pathways. Therefore, a more detailed comparison of metagenomic DNA sequencing data was used, which was obtained using the HiSeq platform to analyze stool samples of obese patients from Chile, Italy and Denmark. First, we performed an unstratified analysis, a type of metabolic pathway enrichment analysis without taking into account microbial contribution, and revealed that the functionality in obesity context did not show statistical differences (in both CCA and Adonis testing) between patients from Chile, Italy and Denmark, suggesting that gut microbiota metabolic pathways do not change according to geographic origin. Conversely, using a stratified approach that could identify specific microbial composition and their individual contribution to metabolic pathways, significant differences between countries were identified, in agreement with our initial PICRUSt metabolic inference, because both methods takes in account taxonomic composition to assess metabolic pathways abundance. Altogether, our observations indicate while overall metabolic functions do not change, microbial functional contribution to obesity are specific to each country.
Although the lack of differences observed in metagenomic unstratified analysis may be due to small cohort numbers, our comparisons suggest that the gut microbiota of obese patients from different countries have functional convergence, where the same essential metabolic functions are carried out by related and unrelated bacterial species. Nevertheless, it is not surprising that bacterial compositions of obese individuals differ between counties due to both genetic and environmental factors, as previously described by others (reviewed by Gupta et al., 2017), however, our observations relating to convergent metabolic functions provide new insights into how the gut microbiota shape common disease phenotypes. The concept of intestinal functional redundancy has been previously explored, in which taxonomic diversity appears to be irrelevant for the inference of functional traits (reviewed by Moya and Ferrer, 2016; Rojo et al., 2017). One such example was demonstrated by the Human Microbiome Project Consortium (Huttenhower et al., 2012), where the authors observed the microbial metabolism to remain constant across individuals over time despite high variability in composition. One possible explanation of microbial functional redundancy can be due to the evolutionary convergence of unrelated taxa, in which variable combinations of species from different phyla can at least partially fulfill the metabolic functions of another, resulting in different species of bacteria behaving similarly (Moya and Ferrer, 2016; Rojo et al., 2017).
Bariatric surgeries have become a frequent choice of treatment for obesity patients over the last years, being SG the most frequent procedure in the world (Angrisani et al., 2017; reviewed by ASMBS, 2018). Several studies have shown that the intestinal microbiota composition changes following surgical intervention and these changes vary between patients who have undergone different kind of surgical intervention such as RYGB, SG and laparoscopic adjustable gastric banding (LAGB) (Tremaroli et al., 2015; Palleja et al., 2016; Ilhan et al., 2017; Medina et al., 2017). One of the limitations of our metagenomic study after bariatric surgery is our small cohort number (n = 2 for each group), and therefore, further studies with larger cohort are required to confirm that different treatments mediate differentially metagenomic rearrangements. However, this study suggest that the microbial functionality of patients changed 6 months following surgical treatment. In agreement with previous studies, it also observed specificity in the changes regarding to the type of surgery performed, where functional changes were mainly mediated by A. muciniphila, E. coli, B. vulgatus, E. siraeum and S. salivarius after RYGB, and by B. cellulosilyticus, S. salivarius, E. eligens, L. lactis, A. finegoldii, E. coli and A. muciniphila species after SG. Although it is impossible to determine from this study whether the microbiota composition changes were a consequence of dietary changes or weight modification, our results not only hint at a microbiota signature for different bariatric surgeries, but also suggest the need for further microbiota meta-analyses at world-wide levels to study metabolic disorders such as obesity.
A common key factor in obesity is the low abundance of A. muciniphila (Schneeberger et al., 2015; Palleja et al., 2016; Medina et al., 2017; Seck et al., 2018), a mucin-degrading bacterium that resides in the human gut mucus layer and whose abundance in healthy subjects represents 3–5% of the residential microbial community (Derrien et al., 2004). Interestingly, it has been shown that A. muciniphila prevents inflammation and adipose tissue alterations in mice (Schneeberger et al., 2015). The administration of A. muciniphila grown under mucin-depleted conditions is effective in reducing obesity and improve intestinal barrier integrity in obese mice (Shin et al., 2019), controls fat mass storage and glucose homeostasis in obese and type 2 diabetic mice (Everard et al., 2013), and it has been previously described that bariatric surgery improves its abundance (Damms-Machado et al., 2015; Tremaroli et al., 2015; Palleja et al., 2016; Medina et al., 2017). Furthermore, overweight and obese individuals with higher A. muciniphila abundance is associated with a healthier metabolic status compared with lower abundance (Dao et al., 2016). Therefore, the enrichment of A. muciniphila provides a possible therapy in the treatment of obesity, and this possibility has been explored in a recent study (Sheng et al., 2018). Here, we identified A. muciniphila as one of the bacteria that drove the changes in metabolic pathways after surgical intervention of Chilean obese patients, an important observation considering that gut microbiota of healthy Chilean subjects has high abundance of Verrucomicrobia bacteria, including A. muciniphila (Fujio-Vejar et al., 2017). Further studies to understand the underlying mechanisms involving A. muciniphila as a target of bacto-therapy to treat obesity are required.
Our results, together with previously published studies, highlight the need to consider region-specific analysis of the gut microbiota in order to fully understand the bacterial basis for the development of such diseases as obesity and the response to surgical and non-surgical treatment, opening to the possibility that probiotic development to treat different kinds of dysbiosis should be country-specific.
Conclusion
This study identified significant differences in the human gut microbiota of obese patients from around the world, and found that functional dissimilarities were mediated by differences in taxonomic microbiota composition, which were region-specific, rather than alterations in metabolic pathways. This indicates the presence of functional metabolic redundancy between the microbiota of obese patients despite the bacterial differences and geographic origin. Furthermore, functional changes in gut microbiota following bariatric surgery were observed to be specific to the type of treatment received, providing new insights into the role of the gut microbiome in treatment strategies.
Ethics Statement
All experiments were conducted in accordance with the Declaration of Helsinki and approved by the Ethics Committee of the Faculty of Medicine, Pontifical Catholic University of Chile (n° 15–337). DNA samples used here for metagenomic belongs from previous published studies (Medina et al., 2017). The 16S and metagenomic raw data sequences used belongs from previous published studies (Tremaroli et al., 2015; Yasir et al., 2015; Palleja et al., 2016; Ilhan et al., 2017; Medina et al., 2017; Thomson et al., 2019).
Author Contributions
DM conceived, designed, performed the comparisons, analyzed the data, and wrote the manuscript. TL wrote and edited the figures and manuscript. PT contributed to the discussion. AA provided the scripts to do the multivariate data analysis. VP-B and AM critically read and edited the manuscript.
Funding
This work was supported by CONICYT-Chile through the FONDECYT [n° 3160525] (DM) and by Universidad San Sebastián, and by grants to AM from the Spanish Ministry of Economy and Competitiveness (projects SAF2012-31187, SAF2013-49788-EXP, SAF2015-65878-R), Carlos III Health Institute (Projects PIE14/00045 and AC15/00022), Generalitat Valenciana (Project PrometeoII/2014/065 and Prometeo/2018/A/133), Asociación Española Contra el Cancer (Project AECC 2017-1485) and co-financed by the European Regional Development Fund (ERDF).
Conflict of Interest
The authors declare that the research was conducted in the absence of any commercial or financial relationships that could be construed as a potential conflict of interest.
Acknowledgments
We thank Daniel Garrido from Pontificia Universidad Católica de Chile for kindly providing the DNA samples belonging to a previous study (Medina et al., 2017) to be used here for shotgun DNA sequencing. We also thank the Departamento de Ingeniería Química y Bioprocesos of the Pontificia Universidad Católica de Chile for their contribution.
Supplementary Material
The Supplementary Material for this article can be found online at: https://www.frontiersin.org/articles/10.3389/fmicb.2019.02346/full#supplementary-material
Footnotes
References
Abubucker, S., Segata, N., Goll, J., Schubert, A. M., Izard, J., Cantarel, B. L., et al. (2012). Metabolic reconstruction for metagenomic data and its application to the human microbiome. PLoS Comput. Biol. 8:e1002358. doi: 10.1371/journal.pcbi.1002358
Angelakis, E., Armougom, F., Million, M., and Raoult, D. (2012). The relationship between gut microbiota and weight gain in humans. Future Microbiol. 7, 91–109. doi: 10.2217/fmb.11.142
Angrisani, L., Santonicola, A., Iovino, P., Vitiello, A., Zundel, N., Buchwald, H., et al. (2017). Bariatric surgery and endoluminal procedures: IFSO worldwide survey 2014. Obes. Surg. 27, 2279–2289. doi: 10.1007/s11695-017-2666-x
ASMBS (2018). American Society for Metabolic and Bariatric Surgery: Estimate of Bariatric Surgery Numbers, 2011–2016. Available at: https://asmbs.org/resources/estimate-of-bariatric-surgery-numbers.
Bolger, A. M., Lohse, M., and Usadel, B. (2014). Trimmomatic: a flexible trimmer for illumina sequence data. Bioinformatics 30, 2114–2120. doi: 10.1093/bioinformatics/btu170
Bolstad, B. (2019). Preprocesscore: A Collection of Pre-Processing Functions. Avalaible at: https://github.com/bmbolstad/preprocessCore.
Caporaso, J. G., Kuczynski, J., Stombaugh, J., Bittinger, K., Bushman, F. D., Costello, E. K., et al. (2010). QIIME allows analysis of high-throughput community sequencing data. Nat. Publ. Gr. 7, 335–336. doi: 10.1038/nmeth.f.303
Carding, S., Verbeke, K., Vipond, D. T., Corfe, B. M., and Owen, L. J. (2015). Dysbiosis of the gut microbiota in disease. Microb. Ecol. Health Dis. 26:26191. doi: 10.3402/mehd.v26.26191
Chilton, S. N. (2014). The effects of diet and the microbiome on reproduction and longevity: a comparative review across 5 continents. J. Nutr. Food Sci. 5:364. doi: 10.4172/2155-9600.1000364
Comeau, A. M., Douglas, G. M., and Langille, M. G. (2017). Microbiome helper: a custom and streamlined workflow for microbiome. mSystems 3:2. doi: 10.1128/mSystems.00127-16
Damms-Machado, A., Mitra, S., Schollenberger, A. E., Kramer, K. M., Meile, T., Königsrainer, A., et al. (2015). Effects of surgical and dietary weight loss therapy for obesity on gut microbiota composition and nutrient absorption. Biomed Res. Int. 2015:806248. doi: 10.1155/2015/806248
Dao, M. C., Everard, A., Aron-Wisnewsky, J., Sokolovska, N., Prifti, E., Verger, E. O., et al. (2016). Akkermansia muciniphila and improved metabolic health during a dietary intervention in obesity: relationship with gut microbiome richness and ecology. Gut 65, 426–436. doi: 10.1136/gutjnl-2014-308778
Derrien, M., Vaughan, E. E., Plugge, C. M., and de Vos, W. M. (2004). Akkermansia municiphila gen. nov., sp. nov., a human intestinal mucin-degrading bacterium. Int. J. Syst. Evol. Microbiol. 54(Pt 5), 1469–1476. doi: 10.1099/ijs.0.02873-0
DeSantis, T. Z., Hugenholtz, P., Larsen, N., Rojas, M., Brodie, E. L., Keller, K., et al. (2006). Greengenes, a chimera-checked 16S rRNA gene database and workbench compatible with ARB. Appl. Environ. Microbiol. 72, 5069–5072. doi: 10.1128/AEM.03006-5
Eldar, S., Heneghan, H. M., Brethauer, S. A., and Schauer, P. R. (2011). Bariatric surgery for treatment of obesity. Int. J. Obes. 35(Suppl. 3), S16–S21. doi: 10.1038/ijo.2011.142
Everard, A., Belzer, C., Geurts, L., Ouwerkerk, J. P., Druart, C., Bindels, L. B., et al. (2013). Cross-talk between Akkermansia muciniphila and intestinal epithelium controls diet-induced obesity. Proc. Natl. Acad. Sci. U.S.A. 110, 9066–9071. doi: 10.1073/pnas.1219451110
Fava, F., Gitau, R., Griffin, B. A., Gibson, G. R., Tuohy, K. M., and Lovegrove, J. A. (2013). The type and quantity of dietary fat and carbohydrate alter faecal microbiome and short-chain fatty acid excretion in a metabolic syndrome “at-risk” population. Int. J. Obes. 37, 216–223. doi: 10.1038/ijo.2012.33
Fei, T., Zhang, T., Shi, W., and Yu, T. (2018). Mitigating the adverse impact of batch effects in sample pattern detection. Bioinformatics 34, 2634–2641. doi: 10.1093/bioinformatics/bty117
First, Y., and Job, P. (2011). GNU parallel: the command-line power tool. USENIX Mag. 3, 42–47. doi: 10.5281/zenodo.16303
Fujio-Vejar, S., Vasquez, Y., Morales, P., Magne, F., Vera-Wolf, P., Ugalde, J. A., et al. (2017). The gut microbiota of healthy chilean subjects reveals a high abundance of the phylum verrucomicrobia. Front. Microbiol. 8:1221. doi: 10.3389/fmicb.2017.01221
Furet, J., Kong, L., Tap, J., Poitou, C., Basdevant, A., Bouillot, J. L., et al. (2010). Differential adaptation of human gut microbiota to bariatric surgery-induced weight loss. Diabetes Metab. Res. Rev. 59, 3049–3057. doi: 10.2337/db10-0253
Goodrich, J. K., Waters, J. L., Poole, A. C., Sutter, J. L., Koren, O., Blekhman, R., et al. (2014). Human genetics shape the gut microbiome. Cell 159, 789–799. doi: 10.1016/j.cell.2014.09.053
Graessler, J., Qin, Y., Zhong, H., Zhang, J., Licinio, J., Wong, M. L., et al. (2013). Metagenomic sequencing of the human gut microbiome before and after bariatric surgery in obese patients with type 2 diabetes: correlation with inflammatory and metabolic parameters. Pharmacogenomics J. 13, 514–522. doi: 10.1038/tpj.2012.43
Gumbs, A. A., Gagner, M., Dakin, G., and Pomp, A. (2007). Sleeve gastrectomy for morbid obesity. Obes. Surg. 17, 962–969. doi: 10.1007/s11695-007-9151-x
Guo, Y., Zhao, S., Su, P. F., Li, C. I., Ye, F., Flynn, C. R., et al. (2014). Statistical strategies for microRNAseq batch effect reduction. Transl. Cancer Res. 3, 260–265. doi: 10.3978/j.issn.2218-676X.2014.06.05
Gupta, V. K., Paul, S., and Dutta, C. (2017). Geography, ethnicity or subsistence-specific variations in human microbiome composition and diversity. Front. Microbiol. 8:1162. doi: 10.3389/fmicb.2017.01162
Hall, A. B., Tolonen, A. C., and Xavier, R. J. (2017). Human genetic variation and the gut microbiome in disease. Nat. Rev. Genet. 18, 690–699. doi: 10.1038/nrg.2017.63
Haro, C., Rangel-Zúñiga, O. A., Alcalá-Díaz, J. F., Gómez-Delgado, F., Pérez-Martínez, P., Delgado-Lista, J., et al. (2016). Intestinal microbiota is influenced by gender and body mass index. PLoS One 2:11. doi: 10.1371/journal.pone.0154090
Huttenhower, C., Gevers, D., Knight, R., Abubucker, S., Badger, J. H., Chinwalla, A. T., et al. (2012). Structure, function and diversity of the healthy human microbiome. Nature 486, 207–214. doi: 10.1038/nature11234
Ilhan, Z. E., Dibaise, J. K., Isern, N. G., Hoyt, D. W., Marcus, A. K., Kang, D. W., et al. (2017). Distinctive microbiomes and metabolites linked with weight loss after gastric bypass, but not gastric banding. ISME J. 11, 2047–2058. doi: 10.1038/ismej.2017.71
Jones, R. B., Zhu, X., Moan, E., Murff, H. J., Ness, R. M., Seidner, D. L., et al. (2018). Inter-niche and inter-individual variation in gut microbial community assessment using stool, rectal swab, and mucosal samples. Sci. Rep. 8:4139. doi: 10.1038/s41598-018-22408-4
Karlsson, F., Tremaroli, V., Nielsen, J., and Backhed, F. (2013). Assessing the human gut microbiota in metabolic diseases. Diabetes Metab. Res. Rev. 62, 3341–3349. doi: 10.2337/db13-0844
Kasai, C., Sugimoto, K., Moritani, I., Tanaka, J., Oya, Y., Inoue, H., et al. (2015). Comparison of the gut microbiota composition between obese and non-obese individuals in a Japanese population, as analyzed by terminal restriction fragment length polymorphism and next-generation sequencing. BMC Gastroenterol. 15:100. doi: 10.1186/s12876-015-0330-2
Khan, M. J., Gerasimidis, K., Edwards, C. A., and Shaikh, M. G. (2016). Role of gut microbiota in the aetiology of obesity: proposed mechanisms and review of the literature. J. Obes. 2016, 1–27. doi: 10.1155/2016/7353642
Kolde, R., Franzosa, E. A., Rahnavard, G., Hall, A. B., Vlamakis, H., Stevens, C., et al. (2018). Host genetic variation and its microbiome interactions within the human microbiome project. Genome Med. 10:6. doi: 10.1186/s13073-018-0515-8
Kong, L. C., Tap, J., Aron-Wisnewsky, J., Pelloux, V., Basdevant, A., Bouillot, J. L., et al. (2013). Gut microbiota after gastric bypass in human obesity: increased richness and associations of bacterial genera with adipose tissue genes. Am. Soc. Nutr. 98, 16–24. doi: 10.3945/ajcn.113.058743
Langille, M. G. I., Zaneveld, J., Caporaso, J. G., McDonald, D., Knights, D., Reyes, J. A., et al. (2013). Predictive functional profiling of microbial communities using 16S rRNA marker gene sequences. Nat. Biotechnol. 31, 814–821. doi: 10.1038/nbt.2676
Langmead, B., and Salzberg, S. L. (2012). Fast gapped-read alignment with bowtie 2. Nat. Methods 9:357. doi: 10.1038/nmeth.1923
Le Chatelier, E., Nielsen, T., Qin, J., Prifti, E., Hildebrand, F., Falony, G., et al. (2013). Richness of human gut microbiome correlates with metabolic markers. Nature 500, 541–546. doi: 10.1038/nature12506
Ley, R. E., Turnbaugh, P. J., Klein, S., and Gordon, J. I. (2006). Microbial ecology: human gut microbes associated with obesity. Nature 444, 1022–1023. doi: 10.1038/nature4441021a
Li, J. V., Ashrafian, H., Bueter, M., Kinross, J., Sands, C., and le Roux, C. W. (2011). Metabolic surgery profoundly influences gut microbial-host metabolic cross-talk. Gut 60, 1214–1223. doi: 10.1136/gut.2010.234708
Louis, S., Tappu, R. M., Damms-Machado, A., Huson, D. H., and Bischoff, S. C. (2016). Characterization of the gut microbial community of obese patients following a weight-loss intervention using whole metagenome shotgun sequencing. PLoS One 11:1–18. doi: 10.1371/journal.pone.0149564
Lozupone, C. A., Stombaugh, J. I., Gordon, J. I., Jansson, J. K., and Knight, R. (2012). Diversity, stability and resilience of the human gut microbiota. Nature 489, 220–230. doi: 10.1038/nature11550
McDonald, D., Price, M. N., Goodrich, J., Nawrocki, E. P., DeSantis, T. Z., Probst, A., et al. (2012). An improved Greengenes taxonomy with explicit ranks for ecological and evolutionary analyses of bacteria and archaea. ISME J. 6, 610–618. doi: 10.1038/ismej.2011.139
McIver, L. J., Abu-Ali, G., Franzosa, E. A., Schwager, R., Morgan, X. C., Waldron, L. et al. (2018). bioBakery: a meta’omic analysis environment. Bioinformatics 34, 1235–1237. doi: 10.1093/bioinformatics/btx754
Medina, D. A., Pedreros, J. P., Turiel, D., Quezada, N., Pimentel, F., Escalona, A., et al. (2017). Distinct patterns in the gut microbiota after surgical or medical therapy in obese patients. PeerJ 5:e3443. doi: 10.7717/peerj.3443
Million, M., Maraninch, M., Henry, M., Armougom, F., Richet, H., Carrieri, P., et al. (2012). Obesity-associated gut microbiota is enriched in Lactobacillus reuteri and depleted in Bifidobacterium animalis and Methanobrevibacter smithii. Int. J. Obes. 36, 817–825. doi: 10.1038/ijo.2011.153
Moya, A., and Ferrer, M. (2016). Functional redundancy-induced stability of gut microbiota subjected to disturbance. Trends Microbiol. 24, 402–413. doi: 10.1016/j.tim.2016.02.002
Murphy, R., Tsai, P., Jüllig, M., Liu, A., Plank, L., and Booth, M. (2017). Differential changes in gut microbiota after gastric bypass and sleeve gastrectomy bariatric surgery vary according to diabetes remission. Obes. Surg. 27, 917–925. doi: 10.1007/s11695-016-2399-2
Navas-Molina, J. A., Peralta-Sanchez, J. M., Gonza, Lez, A., McMurdie, P. J., Vázquez-Baeza, Y., et al. (2013). Advancing our understanding of the human microbiome using QIIME. Methods Enzymol. 531, 371–444. doi: 10.1016/B978-0-12-407863-5.00019-8
NHLBI Expert Panel (1998). Clinical guidelines on the identification, evaluation, and treatment of overweight and obesity in adults - the evidence report. Obes. Res. 6(Suppl. 2), 51S–209S.
Nishijima, S., Suda, W., Oshima, K., Kim, S.-W., Hirose, Y., Morita, H., et al. (2016). The gut microbiome of healthy Japanese and its microbial and functional uniqueness. DNA Res. 23, 125–133. doi: 10.1093/dnares/dsw002
Oksanen, J., Blanchet, F. G., Friendly, M., Kindt, R., Legendre, P., McGlinn, D., et al. (2018). Vegan: Community Ecology Package. Available at: https://cran.r-project.org/package=vegan.
Palleja, A., Kashani, A., Allin, K. H., Nielsen, T., Zhang, C., Li, Y., et al. (2016). Roux-en-Y gastric bypass surgery of morbidly obese patients induces swift and persistent changes of the individual gut microbiota. Genome Med. 8, 67. doi: 10.1186/s13073-016-0312-1
Qin, J., Li, R., Raes, J., Arumugam, M., Burgdorf, K. S., Manichanh, C., et al. (2010). A human gut microbial gene catalogue established by metagenomic sequencing. Nature 464, 59–65. doi: 10.1038/nature08821
Qin, J., Li, Y., Cai, Z., Li, S., Zhu, J., Zhang, F., et al. (2012). A metagenome-wide association study of gut microbiota in type 2 diabetes. Nature 490, 55–60. doi: 10.1038/nature11450
R Core Team (2013). R Core Team. R A Lang. Environ. Stat. Comput. R Found. Stat. Comput. Vienna: R Core Team.
Rognes, T., Flouri, T., Nichols, B., Quince, C., and Mahé, F. (2016). VSEARCH: a versatile open source tool for metagenomics. PeerJ 4:e2584. doi: 10.7717/peerj.2584
Rojo, D., Méndez-García, C., Raczkowska, B. A., Bargiela, R., Moya, A., Ferrer, M., et al. (2017). Exploring the human microbiome from multiple perspectives: factors altering its composition and function. FEMS Microbiol. Rev. 41, 453–478. doi: 10.1093/femsre/fuw046
Rothschild, D., Weissbrod, O., Barkan, E., Kurilshikov, A., Korem, T., Zeevi, D., et al. (2018). Environment dominates over host genetics in shaping human gut microbiota. Nature 555, 210–215. doi: 10.1038/nature25973
Schloss, P. D. (2016). Looking for a signal in the noise: revisiting obesity and the. 7, 1–9. doi: 10.1128/mBio.01018-16.Editor
Schneeberger, M., Everard, A., Gómez-Valadés, A. G., Matamoros, S., Ramírez, S., Delzenne, N. M., et al. (2015). Akkermansia muciniphila inversely correlates with the onset of inflammation, altered adipose tissue metabolism and metabolic disorders during obesity in mice. Sci. Rep. 5:16643. doi: 10.1038/srep16643
Schwalb, B., Tresch, A., Torkler, P., Duemcke, S., Demel, C., Ripley, B., et al. (2011). LSD: Lots of Superior Depictions. Available at: http://cran.r-project.org/web/packages/LSD/index.html
Seck, E. H., Senghor, B., Merhej, V., Bachar, D., Cadoret, F., Robert, C., et al. (2018). Salt in stools is associated with obesity, gut halophilic microbiota and Akkermansia muciniphila depletion in humans. Int. J. Obes. 43, 862–871. doi: 10.1038/s41366-018-0201-3
Segata, N., Izard, J., Waldron, L., Gevers, D., Miropolsky, L., Garrett, W. S., et al. (2011). Metagenomic biomarker discovery and explanation. Genome Biol. 12:R60. doi: 10.1186/gb-2011-12-6-r60
Shao, Y., Ding, R., Xu, B., Hua, R., Shen, Q., He, K., et al. (2016). Alterations of gut microbiota after roux-en-Y gastric bypass and sleeve gastrectomy in sprague-dawley rats. Obes. Surg. 27, 295–302. doi: 10.1007/s11695-016-2297-7
Sheng, L., Jena, P. K., Liu, H.-X., Hu, Y., Nagar, N., Bronner, D. N., et al. (2018). Obesity treatment by epigallocatechin-3-gallate–regulated bile acid signaling and its enriched Akkermansia muciniphila. FASEB J. doi: 10.1096/fj.201800370R [Epub ahead of print]
Shin, J., Noh, J.-R., Chang, D.-H., Kim, Y.-H., Kim, M. H., Lee, E. S., et al. (2019). Elucidation of akkermansia muciniphila probiotic traits driven by mucin depletion. Front. Microbiol. 10:1137. doi: 10.3389/fmicb.2019.01137
Sjöström, L. (2008). Bariatric surgery and reduction in morbidity and mortality: experiences from the SOS study. Int. J. Obes. 32(Suppl. 7), S93–S97. doi: 10.1038/ijo.2008.244
Smoot, T. M., Xu, P., Hilsenrath, P., Kuppersmith, N. C., and Singh, K. P. (2006). Gastric bypass surgery in the united states, 1998-2002. Am. J. Public Health 96, 1187–1189. doi: 10.2105/AJPH.2004.060129
Stevens, G. A., Singh, G. M., Lu, Y., Danaei, G., Lin, J. K., Finucane, M. M., et al. (2012). National, regional, and global trends in adult overweight and obesity prevalences. Popul Health Metr. 10:22. doi: 10.1186/1478-7954-10-22
Sun, Z., Chai, H. S., Wu, Y., White, W. M., Donkena, K. V., Klein, C. J., et al. (2011). Batch effect correction for genome-wide methylation data with illumina infinium platform. BMC Med Genom. 16:84. doi: 10.1186/1755-8794-4-84
Sze, M. A., and Schloss, P. D. (2016). Looking for a signal in the noise: revisiting obesity and the microbiome. MBio 23, 7. doi: 10.1128/mBio.01018-6
Thomson, P., Santibañez, R., Aguirre, C., Galgani, J., and Garrido, D. (2019). Short-term impact of sucralose consumption on the metabolic response and gut microbiome of healthy adults. Br. J. Nutr. 1, 1–23. doi: 10.1017/S0007114519001570
Tice, J. A., Karliner, L., Walsh, J., Petersen, A. J., and Feldman, M. D. (2008). Gastric banding or bypass? a systematic review comparing the two most popular bariatric procedures. Am. J. Med. 121, 885–893. doi: 10.1016/j.amjmed.2008.05.036
Tran, D. D., Nwokeabia, I. D., Purnell, S., Zafar, S. N., Ortega, G., Hughes, K., et al. (2016). Revision of roux-en-y gastric bypass for weight regain: a systematic review of techniques and outcomes. Obes. Surg. 26, 1627–1634. doi: 10.1007/s11695-016-2201-5
Tran, H. Q., Mills, R. H., Peters, N. V., Holder, M. K., de Vries, G. J., Knight, R. et al. (2019). Associations of the fecal microbial proteome composition and proneness to diet-induced obesity. Mol. Cell. Proteomics. 18, 1864–1879. doi: 10.1074/mcp.ra119.001623
Tremaroli, V., and Backhed, F. (2012). Functional interactions between the gut microbiota and host metabolism. Nature 489, 242–249. doi: 10.1038/nature11552
Tremaroli, V., Karlsson, F., Werling, M., Ståhlman, M., Kovatcheva-Datchary, P., Olbers, T., et al. (2015). Roux-en-Y gastric bypass and vertical banded gastroplasty induce long-term changes on the human gut microbiome contributing to fat mass regulation. Cell Metab. 22, 228–238. doi: 10.1016/j.cmet.2015.07.009
Truong, D. T., Franzosa, E. A., Tickle, T. L., Scholz, M., Weingart, G., Pasolli, E., et al. (2015). MetaPhlAn2 for enhanced metagenomic taxonomic profiling. Nat. Methods 12, 902–903. doi: 10.1038/nmeth.3589
Turnbaugh, P. J., Hamady, M., Yatsunenko, T., Cantarel, B. L., Duncan, A., Ley, R. E., et al. (2009). A core gut microbiome in obese and lean twins. Nature 457, 480–484. doi: 10.1038/nature07540
Turnbaugh, P. J., Ley, R. E., Mahowald, M. A., Magrini, V., Mardis, E. R., and Gordon, J. I. (2006). An obesity-associated gut microbiome with increased capacity for energy harvest. Nature 444, 1027–1131. doi: 10.1038/nature05414
Walters, W. A., Xu, Z., and Knight, R. (2014). Meta-analyses of human gut microbes associated with obesity and IBD. FEBS Lett. 588, 4223–4233. doi: 10.1016/j.febslet.2014.09.039
Whitley, E., and Ball, J. (2002). Statistics review 6: nonparametric methods. Crit. Care 6, 509–513. doi: 10.1186/cc1820
Wu, G. D., Chen, J., Hoffmann, C., Bittinger, K., Chen, Y. Y., Keilbaugh, S. A., et al. (2011). Linking long-term dietary patterns with gut microbial enterotypes. Science 7 334, 105–108. doi: 10.1126/science.1208344
Yasir, M., Angelakis, E., Bibi, F., Azhar, E. I., Bachar, D., Lagier, J. C., et al. (2015). Comparison of the gut microbiota of people in france and saudi arabia. Nutr Diabetes 27:e153. doi: 10.1038/nutd.2015.3
Yatsunenko, T., Rey, F. E., Manary, M. J., Trehan, I., Dominguez-Bello, M. G., Contreras, M., et al. (2012). Human gut microbiome viewed across age and geography. Nature 486, 222–227. doi: 10.1038/nature11053
Zhang, H., DiBaise, J. K., Zuccolo, A., Kudrna, D., Braidotti, M., Yeisoo, Y., et al. (2009). Human gut microbiota in obesity and after gastric bypass. Proc. Natl. Acad. Sci. U. S. A. 106, 2365–2370. doi: 10.1073/pnas.0812600106
Zhang, J., Kobert, K., Flouri, T., and Stamatakis, A. (2014). PEAR: a fast and accurate illumina paired-end reAd mergeR. Bioinformatics 30, 614–620. doi: 10.1093/bioinformatics/btt593
Keywords: human gut microbiota, bariatric surgery, obesity, functional redundancy, metagenomic, functional convergence
Citation: Medina DA, Li T, Thomson P, Artacho A, Pérez-Brocal V and Moya A (2019) Cross-Regional View of Functional and Taxonomic Microbiota Composition in Obesity and Post-obesity Treatment Shows Country Specific Microbial Contribution. Front. Microbiol. 10:2346. doi: 10.3389/fmicb.2019.02346
Received: 29 October 2018; Accepted: 26 September 2019;
Published: 17 October 2019.
Edited by:
Qi Zhao, Shenyang Aerospace University, ChinaReviewed by:
Alexander V. Tyakht, Institute of Gene Biology (RAS), RussiaSofia Forslund, Max Delbrück Center for Molecular Medicine, Germany
Copyright © 2019 Medina, Li, Thomson, Artacho, Pérez-Brocal and Moya. This is an open-access article distributed under the terms of the Creative Commons Attribution License (CC BY). The use, distribution or reproduction in other forums is permitted, provided the original author(s) and the copyright owner(s) are credited and that the original publication in this journal is cited, in accordance with accepted academic practice. No use, distribution or reproduction is permitted which does not comply with these terms.
*Correspondence: Daniel A. Medina, daniel.medina@uss.cl