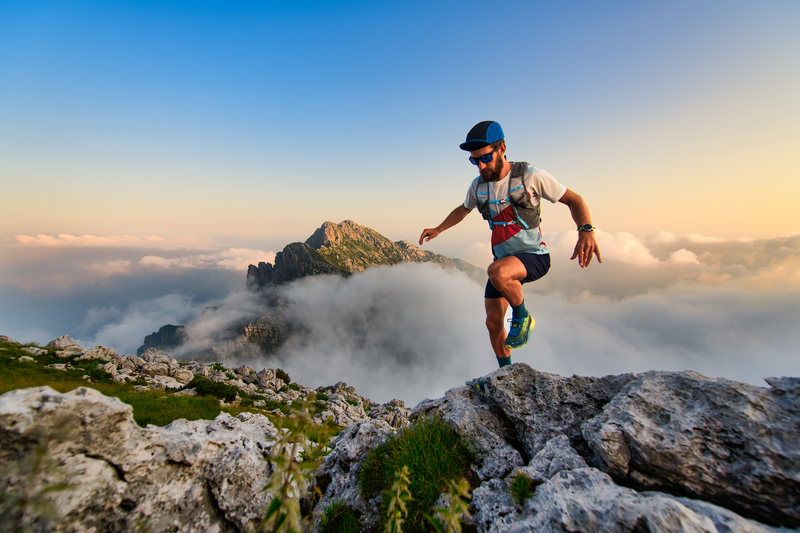
94% of researchers rate our articles as excellent or good
Learn more about the work of our research integrity team to safeguard the quality of each article we publish.
Find out more
ORIGINAL RESEARCH article
Front. Microbiol. , 04 September 2019
Sec. Systems Microbiology
Volume 10 - 2019 | https://doi.org/10.3389/fmicb.2019.02038
This article is part of the Research Topic Gut Microbiome Modulation in Ruminants: Enhancing Advantages and Minimizing Drawbacks View all 31 articles
This study aimed to investigate the effects of two practically relevant doses of menthol-rich plant bioactive lipid compounds (PBLC) on fermentation, microbial community composition, and their interactions in sheep rumen. Twenty-four growing Suffolk sheep were divided into three treatments and were fed hay ad libitum plus 600 g/d of concentrate containing no PBLC (Control) or PBLC at low dose (80 mg/d; PBLC-L) or high dose (160 mg/d; PBLC-H). After 4 weeks on the diets, samples of ruminal digesta were collected and analyzed for short-chain fatty acid (SCFA), ammonia, and microbiota; microbiota being analyzed in the solid and the liquid digesta fractions separately. Ruminal SCFA and ammonia concentrations were not affected by the PBLC treatments. The microbiota in the solid fraction was more diverse than that in the liquid fraction, and the relative abundance of most taxa differed between these two fractions. In the solid fraction, phylogenetic diversity increased linearly with increased PBLC doses, whereas evenness (lowest in PBLC-L) and Simpson diversity index (greatest in PBLC-H) changed quadratically. In the liquid fraction, however, the PBLC supplementation did not affect any of the microbial diversity measurements. Among phyla, Chloroflexi (highest in PBLC-L) and unclassified_bacteria (lowest in PBLC-L) were altered quadratically by PBLC. Lachnospiraceae, Bacteroidaceae (increased linearly), BS11 (increased in PBLC-L), Christensenellaceae (decreased in PBLC treatments), and Porphyromonadaceae (increased in PBLC treatments) were affected at the family level. Among genera, Butyrivibrio increased linearly in the solid fraction, YRC22 increased linearly in the liquid fraction, whereas Paludibacter increased and BF311 increased linearly with increasing doses of PBLC in both fractions. The PBLC treatments also lowered methanogens within the classes Thermoplasmata and Euryarchaeota. Correlation network analysis revealed positive and negative correlations among many microbial taxa. Differential network analysis showed that PBLC supplementation changed the correlation between some microbial taxa and SCFA. The majority of the predicted functional features were different between the solid and the liquid digesta fractions, whereas the PBLC treatments altered few of the predicted functional gene categories. Overall, dietary PBLC treatments had little influence on the ruminal fermentation and microbiota but affected the associations among some microbial taxa and SCFA.
Phytobiotics or plant bioactive molecules have been considered a new class of feed additives for livestock and poultry because of their several beneficial responses in animal production (Van Bibber-Krueger et al., 2016; Kumar et al., 2018; Szczechowiak et al., 2018). Depending upon the type and amount of bioactive compounds used, beneficial biological effects may include modulation of ruminal fermentation (Calsamiglia et al., 2007; Mirzaei-Alamouti et al., 2016; Kazemi-Bonchenari et al., 2018), inhibition of methane production and protein degradation (Cobellis et al., 2016; Patra et al., 2017; Soltan et al., 2018), decreased growth of pathogenic microorganisms in the intestines, boosting of immunity, augmentation of antioxidant activities in the animal tissues (Chowdhury et al., 2018; Kumar et al., 2018), regulation of gastrointestinal nutrient transport and barrier function (Patra et al., 2018), improvement of growth performance (Kazemi-Bonchenari et al., 2018) and body condition (Hausmann et al., 2017, 2018), and increased quantity and quality of milk and meat (Hausmann et al., 2018; Smeti et al., 2018). Based on their molecular structures, these secondary plant compounds can be separated into several classes, namely, saponins, tannins, flavonoids, alkaloids, organosulfur compounds, terpenoids, and phenylpropanoids (Wink, 2003; Patra, 2012). The so-called “essential oils” as a very important group of plant bioactive lipid compounds (PBLC) have been investigated widely in the diets of livestock and poultry due to their antimicrobial properties and specificity to certain microbes (Patra, 2011; Cobellis et al., 2016; Chowdhury et al., 2018). In ruminants, PBLC could inhibit some undesirable microbes, such as methanogenic archaea and protein-degrading bacteria, thus improving ruminal fermentation (McIntosh et al., 2003; Patra and Saxena, 2010; Cobellis et al., 2016).
In several previous trials, high doses of menthol-rich peppermint oil decreased methane production and altered the concentrations and proportions of short chain fatty acid (SCFA) in the ruminal fluid in vitro (up to 2 mL/L, Agarwal et al., 2009; up to 1 g/L, Patra and Yu, 2012; up to 0.6 g/L, Roy et al., 2015). In these in vitro studies, few select microbial populations (e.g., Ruminococcus spp., Fibrobacter succinogenes, methanogenic archaea, and protozoal numbers) were specifically affected by the menthol supplementation (Agarwal et al., 2009; Patra and Yu, 2012). In contrast, peppermint herbs fed at dosages of 50 g/kg DM to lactating Holstein cows (Hosoda et al., 2005) or at 200 g/d in steers (Ando et al., 2003) had no effect on the total concentration and molar proportions of SCFA. Assuming a menthol content of the applied peppermint supplements of ∼1% (Beigi et al., 2018), the menthol doses used in the latter in vivo studies were far lower than those used in vitro, however, they were yet higher than the doses that can be used economically and safely in routine practical feeding. Regarding safety, current EU regulations acknowledge that menthol supplementation is safe up to a concentration of 25 mg/kg complete feeding stuff and require detailed labeling where this concentration is exceeded (The European Commission, 2018).
The ruminal microbiota is highly dynamic and has diverse microbes associated with ruminal epithelium, digesta solids, or ruminal liquid (Mao et al., 2015; Klevenhusen et al., 2017). The three fractions have distinctive microbiota diversity, composition, and functions and perform different ecological and nutritional roles (Mao et al., 2015; Klevenhusen et al., 2017). It has been shown repeatedly that dietary PBLC including menthol can modulate ruminal fermentation by exerting antimicrobial actions (Calsamiglia et al., 2007; Patra and Saxena, 2009; Khiaosa-ard and Zebeli, 2013). To the best of our knowledge, however, no study has investigated the effects of any PBLC on microbiota in the different digesta fractions separately. We thus hypothesized that menthol might affect the microbiota in the solid and the liquid phase differently. Moreover, considering the specific antimicrobial actions of high doses of peppermint oil that lead to fermentation changes in vitro vs. an apparent absence of fermentation changes when menthol is supplemented at lower doses in vivo, it was further hypothesized that menthol effects on specific microbial populations may not result in detectable fermentation changes in vivo due to compensatory alterations in metabolic interactions among microbial species. To test the above hypotheses, this study was performed to investigate the effects of menthol-rich PBLC at two doses with practical relevance and yet justifiable safety on ruminal fermentation and its relation to the composition, structures, functions and interactions of microbiota in the solid and liquid fractions of ruminal digesta.
Twenty-four growing Suffolk sheep (15 females and 9 males) with initial body weight (BW) of 32.9 ± 3.44 kg and age of 121 ± 3.75 days were equally distributed into three experimental groups that either received no PBLC (Control; n = 8), 80 mg PBLC/d (PBLC-L; n = 8) or 160 mg PBLC/d (PBLC-H; n = 8). The experiment was performed in two feeding experimental runs with 12 sheep in the first run and 12 different sheep in the second run. Sheep were distributed to the three dietary treatment groups (Control, PBLC-L and PBLC-H) with the aim to achieve equal sex (5 females and 3 males, per group) and BW distribution by using a randomized block design. Each block consisted of one sheep per group with similar initial BW. This resulted in a total of four blocks of three sheep per run, which were accommodated to the four available pens. Each pen contained three separate automatic transponder-operated feeding stations with locking gates designed for small ruminants. Each sheep had access to only one specific feeder recognized by individual animal identification tag with an electronic transponder fitted to its neck collar.
Based on an (National Research Council [NRC], 2007) requirement for 300 g/d growth rate, all sheep were fed a pelleted concentrate (600 g/d) and ad libitum meadow hay (without chopping) for 4 weeks. Ingredients and chemical compositions of concentrates were the same except that the concentrates of the PBLC-L and PBLC-H groups were pelleted together with a PBLC premix (OAX17, PerformaNat GmbH, Germany; 13.33 g PBLC/kg of ground corn grains) to achieve the final target PBLC concentrations (133 or 267 mg/kg concentrate) (Table 1). Concentrates were pelleted below 50°C to avoid evaporative loss of PBLC during pelleting, and the concentrate pellets were stored in air-tight bags. The daily dose of PBLC was supplied with the concentrates at 07:00, 11:00, and 15:00 h in three equal portions (200 g each time). All sheep completely consumed the concentrate feed allowance. The commercial source of PBLC contained mainly menthol (900 g/kg) with other minor bioactive compounds. Drinking water was available at all times from push-button water troughs. Feed samples (hay and concentrate mixtures) were collected weekly and pooled for each treatment for chemical analyses.
The chemical composition of feed samples was analyzed following standard methods (Naumann et al., 2004). Briefly, content of dry matter was determined in a drying cabinet (VDLUFA MB III 3.1), crude ash in a muffle furnace at 550°C (VDLUFA III 8.1), ether extract by the Soxhlet method with hydrolysis (VDLUFA MB III 5.1.1), and crude protein by the incineration method (VDLUFA MB III 4.1.2). Acid detergent fiber (ADF) expressed exclusive of residual ash (ADFom) was determined using FibertecTM 8000 (FOSS, Hilleroed, Denmark; method, VDLUFA MB III 6.5.2). Neutral detergent fiber (NDF) content (comparable to the assay with heat-stable amylase and expressed exclusive of residual ash; aNDFom) was estimated using near-infrared spectroscopy according to the method VDLUFA MB III 31.2.
After the 4 weeks trial period, sheep were maintained on the assigned feeding regime until slaughter using penetrative captive bolt with subsequent exsanguination. Animals from the three groups were killed in the order of their block designation (i.e., block after block) over 6 consecutive days. Each day, one animal was killed 2 h after the morning feeding and a second animal was killed 2 h after the 11:00 h feeding. As group order of killing was kept constant for all blocks, exactly half of the animals in each group had been slaughtered 2 h after the morning feeding and the other half had been slaughtered 2 h after the 11:00 h feeding at the end of the trial. The pH in the ventral sac of the rumen was recorded immediately after killing using pH meter 3110 (Xylem Analytics Germany GmbH, Weilheim, Germany). One ruminal content sample of each sheep was filtered through two layers of sterile cheesecloth to separate solid and liquid fractions for microbiota analysis. These samples were immediately placed on ice and stored within 30 min at −20°C until DNA extraction. To determine the concentrations of short-chain fatty acids (SCFA) and ammonia, an aliquot of 8 mL of ruminal fluid from each sheep was immediately transferred into a 15 mL polypropylene centrifuge tube (TPP®, Trasadingen, Switzerland) containing 2 mL of metaphosphoric acid solution (250 g/L distilled water) to precipitate protein and stop fermentation. The tubes were kept at room temperature for 30 min and then placed on ice. The ruminal fluid was centrifuged at 5000 × g at 4°C for 15 min and the supernatant was stored at −20°C until analysis. Concentrations of SCFA (acetic, propionic, n-butyric, iso-butyric, n-valeric, and iso-valeric acids) and ammonia were analyzed as described by Pieper et al. (2014). Methane production was estimated from the stoichiometric relations between SCFA concentrations and methanogenesis (Moss et al., 2000).
Metagenomic DNA from the solid and liquid fractions of the rumen samples was extracted using the repeated bead beating (with a Homogenizer MM301, Retsch, Haan, Germany at a frequency up to 30 Hz) plus column purification method as described by Yu and Morrison (2004). The quality of extracted DNA was assessed based on the absorbance ratios of 260/280and 260/230 nm and quantified using a NanoDrop ND-2000 Spectrophotometer (Thermo Scientific, Wilmington, DE, United States). The DNA samples were dried under vacuum and sent to the DNA sequencing facility at The Ohio State University (United States) and stored at −20°C until analysis.
Amplicon libraries of 16S rRNA genes were prepared and sequenced at the Molecular and Cellular Imaging Center of The Ohio State University1. Briefly, dual index 16S rRNA gene amplicon libraries from both bacteria and archaea were prepared following the Illumina protocol (Fadrosh et al., 2014; Illumina 16S Metagenomic Sequencing Library Preparation guide – Part # 15044223 Rev. B) using 515F (5′-GTGCCAGCMGCCGCGGTAA-3′) and 806R (5′-GGACTACHVGGGTWTCTAAT-3′) specific primers (Caporaso et al., 2011) with a unique barcode for each DNA sample. The amplicon libraries were pooled equimolarly and sequenced on the MiSeq instrument in a 300 cycles paired-end run. The built-in plugins within QIIME2 (version 2018.6) (Bolyen et al., 2018) were used to analyze the 16S rRNA amplicon sequences. Briefly, DADA2 was first used to denoise the demultiplexed forward and the reverse reads with quality (Q > 25) filtering (Callahan et al., 2016). The denoised-feature table and amplicon sequence variants (ASVs) were used for taxonomic diversity analysis. The ASVs were taxonomically classified with the Greengenes 99% OTUs 16S rRNA gene reference sequences (version 13_8) using the naïve Bayesian taxonomic classifier (Wang et al., 2007). The relative abundance of a taxon was expressed as percentage of total sequences in respective samples. Assigned taxa were visualized through bar plots using the mean relative abundance of each group. Mitochondria sequences were removed in the final analysis. Raw 16S rRNA gene amplicon sequence data are available in NCBI Sequence Read Archive (SRA) under BioProject PRJNA529255.
The functional features of each sample were predicted using PICRUSt (Phylogenetic Investigation of Communities by Reconstruction of Unobserved States) (Langille et al., 2013). Species-level OTUs were picked using the q2-vsearch closed-reference OTU picking method against the Greengenes reference database of 16S rRNA gene sequences clustered at 97% sequence similarity (13_5 release). Copy number-based normalized classified OTUs table was used to link in between Greengenes IDs and KEGG orthologs by finding genome contents for each OTU from pre-calculated file followed by multiplying the OTU abundance by predicted functional abundance in the genome using PICRUSt.
The Mixed model procedure of SAS (2001) was used to analyze the relative abundance of phylum, family and genus, and KEGG gene abundance at subsystem levels 1, 2, and 3. Residuals were checked for normality using either Shapiro–Wilk or Kolmogorov–Smirnov test. When residuals did not show normal distribution, data were transformed (log, arcsine, sine, or square-root) to have normal distribution. If residuals did not follow normal distribution after various transformations, the non-parametric Wilcoxon test was used to compare solid vs. liquid fractions and Control vs. both PBLC treatments. Unequal variances among treatments, if any, were adjusted using the “Repeated” statement in the mixed model. The relative abundances of all the identified phyla and KEGG level 1 gene categories were analyzed, while only the relative abundance of the major families, genera, KEGG subsystem level 2 and level 3 categories (each with a relative abundance >0.5%) were comparatively analyzed because of the large numbers of taxa and functional categories identified. The model included treatment, block (i.e., initial BW of sheep), fractions of digesta, sex and fraction × treatment interaction. Linear and quadratic effects of PBLC doses (0, 80, and 160 mg/d) were assessed using polynomial contrasts. Contrasts between Control (0 mg/d of PBLC) vs. average of both PBLC groups (80 and 160 mg/d of PBLC) were also used to determine the overall effects of PBLC compared with the Control. When interaction was or tended to be significant, the SLICE option in the model was used to determine the effect in either the solid or liquid fraction. Variability of data was expressed as pooled SEM, and statistical significance was set at P ≤ 0.05, while a trend was considered at 0.05 < P ≤ 0.10.
Principal component analysis (PCA) was performed to investigate differences in the predicted functions at KEGG level 3 among the treatments and between the two digesta fractions using PROC PRINCOMP of SAS (2001) with the covariance matrix. The first three principal components were plotted to show the overall comparison of the treatments. The PCA loadings of the first three components were further analyzed using SAS (2001) by multivariate analysis of variance (MANOVA) to test for differences in the overall predicted functional features between digesta fraction, treatment and treatment × fraction interaction. Aided by an online tool2, linear discriminant analysis (LDA) effect size (LEfSe) and Kruskal-Wallis tests were used to find differentially abundant (P < 0.05 and LDA scores higher than 2; Segata et al., 2011) taxa and functional features.
Pearson correlations between SCFA and major OTUs each with a relative abundance >0.1% and among the OTUs each with a relative abundance >0.5% were calculated using SAS (2001). These cutoffs were used based on the assumption that the small OTUs with a relative abundance below these cutoffs would have minimal influence on the functionality of the entire microbiota. The force-directed correlation network layouts based on the numerical values of correlations coefficients were then created including nodes consisting of microbiota and SCFA pattern which had a P ≤ 0.01 using Cytoscape (Shannon et al., 2003). We chose the strong correlation (P ≤ 0.01) cutoffs to focus on substantial contributions in the interactions. These networks were created separately for the Control group and the PBLC groups, or the liquid fraction and the solid fraction. Correlation differences were also analyzed to evaluate the differential features between Control and PBLC treatments, and liquid and solid fractions, and these networks were visualized in Cytoscape (Shannon et al., 2003).
Ruminal fermentation characteristics including pH, total SCFA concentrations and molar proportion of acetate, propionate, butyrate and other minor acids, and acetate to propionate ratio were not affected by the dietary PBLC supplementation (Table 2). Ammonia concentrations in ruminal fluid were not changed by PBLC. The estimated methane production in sheep was also not influenced by PBLC feeding (Table 2).
Table 2. Effect of two doses of dietary menthol-rich plant bioactive lipid compounds (PBLC) on ruminal pH and short-chain fatty acids (SCFA) in sheep.
On average, 37,359 quality checked sequences were obtained from each sample, which allows for >99% depth coverage. As shown in Table 3, all the determined alpha diversity measurements, except for Simpson diversity index (P = 0.103) and evenness (P = 0.69), were greater in the solid fraction than in the liquid fraction. In the solid fraction, the number of observed OTU and Chao 1 richness estimate were not affected (P > 0.10) by PBLC, but phylogenetic diversity (P = 0.028) increased linearly with PBLC doses; whereas evenness (lowest in PBLC-L), and Simpson diversity index (greatest in PBLC-H) were quadratically changed by PBLC (P = 0.006–0.001). In the liquid fraction, however, none of the determined alpha diversity measurements was influenced by the PBLC supplementation (P > 0.10) except for Simpson index (increased linearly, P = 0.094). Interactions between treatment and digesta fraction were not significant (P > 0.10) for any of the alpha diversity measurements.
Table 3. Effect of dietary menthol-rich plant bioactive lipid compounds (PBLC) on ruminal microbiota diversity measurements in the solid and the liquid fractions of sheep ruminal digesta.
In the solid fraction, 104 species-level OTUs were shared among all the treatments, but 5, 7, and 11 OTUs were unique to the Control PBLC-L, and PBLC-H, respectively. In the liquid fraction, the number of shared OTUs was 93, whereas 7, 11, and 6 OTUs were unique to Control, PBLC-L and PBLC-H, respectively (Supplementary Figure S1).
Twenty-one different phyla were identified in all the samples, and all differed in relative abundance between the solid and liquid fractions, except for Tenericutes, Chloroflexi, Euryarchaeota, Proteobacteria, TM7, WPS-2, and Armatimonadetes. Firmicutes, Fibrobacteres, Spirochetes, SR1, Planctomycetes, and Actinobacteria were more predominant (P ≤ 0.05) in the solid fraction than in the liquid fraction, while Bacteroidetes, Verrucomicrobia, Synergistetes, Cyanobacteria, Lentisphaerae, and LD1 showed an opposite distribution (P ≤ 0.05) (Figure 1 and Supplementary Table S1). Interaction effects between treatment and digesta fraction were not evident (P ≥ 0.10) for any of the phyla. Among the phyla, Firmicutes (quadratic response; P = 0.065; lowest in PBLC-L), Chloroflexi (quadratic response; P = 0.004; highest in PBLC-L), Planctomycetes (decreased in both PBLC treatments vs. Control; P = 0.062), UP_bacteria (quadratic response; P = 0.007; lowest in PBLC-L), SR1 (tended to increase linearly; P = 0.051), and Euryarchaeota (decreased in both PBLC treatments vs. Control; P = 0.009) were influenced by the PBLC treatments.
Figure 1. Community barplot analysis depicting the average relative abundance of phyla detected in solid and liquid fractions of the ruminal digesta of different treatment groups. Control, PBLC-L, and PBLC-H, treatment groups supplemented with menthol-rich plant bioactive lipid compounds at 0, 80, and 160 mg/d, respectively. In the square brackets, symbols † and ‡ indicate greater (P ≤ 0.05) abundances in the solid and the liquid fractions, respectively, while uppercase letters indicate significant (P ≤ 0.05) treatment effect (T; Control vs. both PBLC-L and PBLC-H) or dose effect (L for linear, Q for quadratic) of PBLC; whereas, lowercase letters (t for treatment, and l and q for linear and quadratic dose effect, respectively) indicate a trend (0.05 < P ≤ 0.10). No interactions between treatment and digesta fraction were present (P ≥ 0.10).
Ninety-one different families were found from at least one sample, and 21 families including four unclassified families (UF) had a relative abundance of >0.5% in at least one of the fractioned samples. All these major families were significantly different (P = 0.03 to < 0.001) in relative abundance between the solid and the liquid fractions, except for BS11 (P = 0.10) (Figure 2 and Supplementary Table S2). Overall, the solid fraction had a greater relative abundance of UF_Clostridiales 1, Ruminococcaceae, Lachnospiraceae, S24-7, UF_Bacteroidales 2, Clostridiaceae, Christensenellaceae, Fibrobacteraceae, UF_Clostridiales 2, Mogibacteriaceae, and Spirochaetaceae, but a lower relative abundance of Prevotellaceae, UF_Bacteroidales 1, Paraprevotellaceae, Veillonellaceae, Bacteroidaceae, RF16, Erysipelotrichaceae, RFP12, Dethiosulfovibrionaceae, and Porphyromonadaceae than the liquid fraction (P ≤ 0.05). Lachnospiraceae (P = 0.019), Bacteroidaceae (P = 0.026), and UF_Clostridiales 2 (P = 0.060) tended to increase or increased linearly with increasing doses of PBLC. A trend in fraction × treatment interaction (P = 0.075) was noted for Erysipelotrichaceae, which was not affected by the PBLC treatments in the solid fraction but increased linearly in the liquid fraction. BS11 increased quadratically (P = 0.012) with the highest relative abundance in the PBLC-L, while Christensenellaceae decreased (P = 0.010) and Porphyromonadaceae increased (Wilcoxon test, P = 0.008) in both PBLC groups.
Figure 2. Community barplot analysis depicting the average relative abundance of the major families (each representing ≥0.5% total sequences) detected in solid and liquid fractions of the rumen digesta of different treatment groups. Control, PBLC-L, and PBLC-H, treatment groups supplemented with menthol-rich plant bioactive lipid compounds at 0, 80, and 160 mg/d, respectively. In the square brackets, symbols † and ‡ indicate grater (P ≤ 0.05) abundances in the solid and the liquid fractions, respectively, while uppercase letters indicate significant (P ≤ 0.05) treatment effect (T; Control vs. both PBLC-L and PBLC-H) or dose effect (L for linear, Q for quadratic) of PBLC; whereas, lowercase letters (t for treatment, and l and q for linear and quadratic dose effect, and i for interaction effect between treatment and digesta fraction) indicate a trend (0.05 < P ≤ 0.10).
The present study identified 144 genera in at least one sample, and many of them were unclassified genera (UG). Twenty-eight genera had relative abundances >0.5%, and all these genera significantly differed in relative abundance between the solid and the liquid fractions (P = 0.03 to < 0.001), except for UG_BS11 (P = 0.10), YRC22 (P = 0.76), and Paludibacter (P = 0.14) (Figure 3 and Supplementary Table S3). Ruminococcus, Clostridium, Fibrobacter, Butyrivibrio, Succiniclasticum, Treponema, several unclassified genera within the families Ruminococcaceae, S24-7, Lachnospiraceae, Prevotellaceae, Christensenellaceae, and Mogibacteriaceae, or orders Clostridiales and Bacteroidales were more predominant in the solid fraction than in the liquid fraction (P ≤ 0.05), whereas Prevotella, CF231, BF311, TG5, UG_Bacteroidales 1, UG_Paraprevotellaceae, UG_RF16, UG_Veilonellaceae, and UG_RFP12 were more predominant in the liquid fraction than in the solid fraction (P ≤ 0.05).
Figure 3. Community barplot analysis depicting the average relative abundance of the major genera (each representing ≥0.5% total sequences) detected in the solid and the liquid fractions of the rumen digesta of different treatment groups. Control, PBLC-L, and PBLC-H, treatment groups supplemented with menthol-rich plant bioactive lipid compounds at 0, 80, and 160 mg/d, respectively. In the square brackets, symbols † and ‡ indicate greater (P ≤ 0.05) abundances in the solid and the liquid fractions, respectively, while uppercase letters indicate significant (P ≤ 0.05) treatment effect (T; Control vs. both PBLC-L and PBLC-H) or dose effect (L for linear, Q for quadratic) of PBLC; whereas, lowercase letters (t for treatment, and l and q for linear and quadratic dose effect, and i for interaction effect between treatment and digesta fraction) indicate a trend (0.05 < P ≤ 0.10).
Trends for interaction effects between treatment × digesta fraction were noted for the relative abundance of YRC22 (P = 0.077) and Butyrivibrio (P = 0.088). With the increased doses of PBLC, Butyrivibrio increased their relative abundance linearly (P = 0.015) in the solid fraction, while YRC22 did the same (P = 0.007) but in the liquid fraction. The relative abundance of UG_Clostridiales 1 tended to decrease (P = 0.098) and that of UG_Christensenellaceae (Wilcoxon P = 0.080) and UG_Paraprevotellaceae (P = 0.036) tended to decrease or decreased linearly in response to the PBLC supplementation irrespective of fractions. On the contrary, Paludibacter increased (Wilcoxon test, P = 0.043) and the relative abundances of three other taxa (UG_Lachnospiraceae 1, P = 0.018; BF311, P = 0.025; and UG_Clostridiales 2, P = 0.057) tended to increase linearly or increased linearly with increased PBLC doses in both fractions. The PBLC treatments quadratically affected the relative abundance of UG_BS11 (P = 0.012; highest in PBLC-L), UG_Ruminococcaceae 2 (P = 0.047; lowest in PBLC-L), and UG_Lachnospiraceae 2 (P = 0.050; lowest in PBLC-L).
LEfSe analysis showed that some bacteria and archaea were enriched in the solid fraction of the PBLC treatments, including Dehalobacteriaceae, Mycoplasmataceae, UG_Lachnospiraceae, unclassified species (US)_Dehalobacterium, and US_Desulfovibrio, whereas Christensenellaceae, UG_Paraprevotellaceae, Euryarchaeota, US_Methanosphaera, US_Prevotella, LD1-PB3, and UG_LD1-PB3 were significantly more predominant in the solid fraction of the Control than of the PBLC treatments (P ≤ 0.05; Figure 4). In the liquid fraction, WCHB1-25, US_WCHB1-25, Bacteroidaceae, US_BF311, and US_YRC22 had a greater relative abundance in the PBLC groups than in the Control, whereas Christensenellaceae, UG_Christensenellaceae, Thermoplasmata, Methanomassiliicoccaceae, and vadinCA11, US_Blautia 2 and UC_Proteobacteria were significantly greater in the Control than in the PBLC treatments (P ≤ 0.05; Figure 4).
Figure 4. Linear discriminant analysis effect size (LEfSe) and their cladograms of biomarker genera and species in the solid (A,B) or liquid (C,D) fractions of the rumen digesta. Nodes represent position of microbiota in taxa with circular and axial lines indicate the level of taxa and number of taxa at the lower level. Control and PBLC, sheep supplemented with 0 and 80 or 160 mg/d menthol-rich plant bioactive lipid compounds.
Irrespective of PBLC supplementation, the concentrations of all six determined SCFAs were correlated (P ≤ 0.05) with at least one of the 17 solid-associated OTUs, and there were 29 significant correlations between individual SCFA and OTUs, of which nine were positive (Figure 5A). Only 14 correlations (P ≤ 0.05) were found among four SCFAs (all the six SCFA except acetate and propionate) and 10 liquid-associated OTUs, out of which seven were positive (Figure 5B). Between the solid and liquid fractions, an overlap was noted in the correlations of OTU4, OTU14, and OTU19 with butyrate and OTU16 with valerate. Consequently, 25 correlations were unique for the solid-associated OTUs and 10 correlations were unique for the liquid-associated OTUs (Supplementary Figure S2).
Figure 5. Correlation network showing the correlation between SCFAs in the ruminal fluid and the major OTUs (each representing ≥0.1% total sequences) in the solid (A) and liquid fractions of digesta (B). Dotted lines indicate positive correlations whereas solid lines represent negative correlations. The shorter the distances between two nodes, the stronger the correlation between them. See Supplementary Table S5 for the taxonomic assignment of the OTUs.
In the Control group, 11 OTUs from both digesta fractions were correlated with the concentration of at least one of the six detected SCFAs (9 OTUs negatively and 2 OTUs positively; P ≤ 0.05). A total of 31 individual correlations were noted within the correlation network, out of which 29 correlations were negative and two (US_BS11 with propionate and US_CF231 with valerate) were positive (Figure 6A). In the PBLC groups, five OTUs from both digesta fractions were associated (P ≤ 0.05) to at least one of five SCFAs (all the detected SCFAs except propionate), and of the 12 correlations, seven were negative (Figure 6B). Although PBLC did not influence the concentrations of any of the SCFAs (Table 2), it was striking that, with the exception of a single correlation between OTU14 and butyrate, there was no further overlap in the OTU-SCFA correlation networks between the Control and PBLC treatments. Thus, differential network analysis for unique correlations in the Control group (Supplementary Figure S3A) largely mirrored the set of the all identified correlations in that group (Figure 6A), whereas differential network analysis for unique correlations in both PBLC groups (Supplementary Figure S3B) largely mirrored the total set of correlations identified in the PBLC groups (Figure 6B).
Figure 6. Correlation network showing the correlation between major OTUs (each representing ≥0.1% of total sequences) in the digesta and SCFAs of ruminal fluid in the Control group (A) and both PBLC-L and PBLC-H groups (B). Control, PBLC-L, and PBLC-H, treatment groups supplemented with menthol-rich PBLC at 0, 80, and 160 mg/d, respectively. Dotted lines indicate positive correlations, whereas solid lines represent negative correlations. The shorter the distance between two nodes, the stronger the correlation between them. See Supplementary Table S5 for the taxonomic assignment of the OTUs.
Network analysis revealed that some ruminal microbes extensively correlated among themselves. A total of 106 correlations were found among the 22 OTUs in the correlation network of the Control group (P < 0.01; r > 0.62 or r <−0.62) either positively (n = 64) or negatively (n = 42) (Figure 7A). In the PBLC groups, 20 OTUs were correlated (P < 0.001; r > 0.62 or r < −0.62) to other OTUs forming 74 correlations, out of which 34 were negative (Figure 7B). The OTU-OTU correlations between the microbiota of the solid vs. liquid fractions were less prominent (Supplementary Figure S4). Differential network analysis revealed that 62 correlations (mostly positive correlations) among the 22 OTUs were exclusively present in the Control group compared with the PBLC groups (Supplementary Figure S5A). Alternatively, 19 OTUs in the PBLC groups were involved in 30 unique correlations (17 positive and 13 negative correlations) that were not present in the Control group (Supplementary Figure S5B). A merged network depicting all the OTU-OTU and the OTU-SCFA correlations is shown in Supplementary Figure S6 for completeness.
Figure 7. Correlation networks showing correlations between major OTUs (each representing ≥0.5% total sequences) in the rumen of the Control group (A) and both the plant bioactive lipid compound groups (B). Dotted lines indicate positive correlations, while solid lines represent negative correlations. The shorter the distance between two nodes, the stronger the correlation between them. See Supplementary Table S5 for the taxonomic assignment of the OTUs.
At the KEGG level 1, all the functional categories had very small but significant differences (P ≤ 0.05) between the solid and the liquid fractions of the ruminal digesta (Supplementary Figure S7). The predicted genes for “metabolism” had the highest relative abundance (>52% of total functions in each fraction), which was significantly greater in the liquid than the solid fractions. The predicted “genetic information processing” was also more predominant for the liquid (24.1%) than for the solid (23.8%) fractions. However, “environmental information processing” (10.9% vs. 8.92%) and “cellular processes” (3.09% vs. 2.49%) were more predominant in the solid than in the liquid fractions. No significant difference in the predicted functional categories was noted at KEGG level 1 between the Control and the PBLC treatments (P > 0.05).
At KEGG level 2, a total of 64 KEGG ortholog groups were predicted. Among them, 30 gene families had a relative abundance >0.5%, and all of them were different between the solid and liquid fractions (P ≤ 0.05), except for the genes assigned to “translation proteins” (Figure 8 and Supplementary Table S4). The majority of the functions were involved in (for solid vs. liquid fractions) “amino acid metabolism” (10.4% vs. 10.8%), “carbohydrate metabolism” (10.1% vs. 10.3%), “replication and repair” (9.74% vs. 10.0%), “membrane transport” (9.28% vs. 7.54%), “translation” (6.30% vs. 6.47%), and “energy metabolism” (6.17% vs. 6.33%). Among other predicted functional categories each with a relative abundance >1%, “signal transduction,” “xenobiotics biodegradation and metabolism,” “cell motility,” “transcription,” and “lipid metabolism” were more prevalent in the solid than in the liquid fractions. The PBLC treatments altered a number of functions (P < 0.05), including “membrane transport” (quadratic effect; lowest in PBLC-L), “glycan biosynthesis and metabolism” (quadratic effect; highest in PBLC-L), “folding, sorting and degradation” (quadratic effect; highest in PBLC-L), “transcription” (quadratic effect; lowest in PBLC-L), “metabolism of other amino acids” (increased in PBLC groups), “biosynthesis of other secondary metabolites” (quadratic effect; highest in PBLC-L), “translation proteins” (decreased linearly), and “membrane and intracellular structural molecules” (quadratic effect; highest in PBLC-L).
Figure 8. Relative abundances of different predicted functional features (at KEGG level 2) of the rumen microbiota compared between the solid and the liquid fractions of the ruminal digesta and among the three treatment groups. Control, PBLC-L, and PBLC-H, treatment groups supplemented with menthol-rich PBLC at 0, 80, and 160 mg/d, respectively. In the square brackets, symbols † and ‡ indicate grater (P ≤ 0.05) abundances in the solid and the liquid fractions, respectively, while uppercase letters indicate significant (P ≤ 0.05) treatment effects (T; Control vs. both PBLC-L and PBLC-H) or dose effect (L for linear, Q for quadratic) of PBLC; whereas, lowercase letters (t for treatment, and l and q for dose) indicate a trend (0.05 < P ≤ 0.10).
At KEGG level 3, a total of 70 predicted functional categories each with a relative abundance >0.5% were noted in the samples (data not shown), and all these categories were different (P ≤ 0.05) in relative abundance between the two digesta fractions, with the exception of “ribosome biogenesis,” “glycolysis/gluconeogenesis,” “translation proteins_unclassified,” and “valine, leucine and isoleucine biosynthesis.” As evaluated using MANOVA analysis, the PCA plot also showed clear separation (Pillai’s trace, P < 0.001) between the two fractions (Figure 9). The PBLC treatments affected the relative abundance of “transporters” (quadratic effect; lowest in PBLC-L), “amino acid related enzymes” (quadratic effect; lowest in Control), “amino sugar and nucleotide sugar metabolism” (increased in PBLC groups), “transcription factors” (quadratic effect; lowest in PBLC-L), “chaperones and folding catalysts” (quadratic effect; highest in PBLC-L), “nitrogen metabolism” (increased in the PBLC groups), “membrane and intracellular structural molecules_unclassified” (quadratic effect; highest in PBLC-L), “protein export” (quadratic effect; lowest in Control), and “butanoate metabolism” (quadratic effect; lowest in PBLC-L), but the magnitudes of the changes were very small (Table 4). MANOVA analysis revealed that no separation was noted between the Control and the PBLC treatments (Pillai’s trace, P = 0.55) on the PCA plot (Figure 9). Furthermore, no significant interaction between the treatment × digesta fractions (Pillai’s trace, P = 0.47) was noted for the overall predicted functional features.
Table 4. Effect of supplementation of menthol-rich plant bioactive compounds (PBLC) on predicted gene function at KEGG level 3 in the solid and the liquid fractions of ruminal digesta in sheep.
Figure 9. Principal component analysis of the predicted functional features (at KEGG level 3) of the ruminal microbiota between the solid and the liquid fractions of the ruminal digesta and among the three treatment groups. Control, PBLC-L, and PBLC-H, treatment groups supplemented with menthol-rich PBLC at 0, 80, and 160 mg/d, respectively. Statistical comparison of the scores of the first three principal components using MANOVA showed that solid vs. liquid fractions of digesta were different (P < 0.001). Treatment (P = 0.55) and interaction among treatment × digesta fraction (P = 0.47) were not significant for the overall predicted functional features.
Various PBLC can influence the ruminal microbiota with consequences for fermentation, which may include an alteration of SCFA profile, inhibition of methane production, suppression of protein degradation and ammonia production, modification of fatty acid biohydrogenation, and mitigation of ruminal acidosis (Hutton et al., 2009; Patra, 2011; Cobellis et al., 2016). High doses of peppermint oil that contains menthol as a major component also changed ruminal SCFA profile (i.e., increased acetate and lowered propionate molar proportions), methane production and microbial abundances in vitro (Agarwal et al., 2009; Patra and Yu, 2012). However, the results from in vitro studies may not agree with those of in vivo studies (Flachowsky and Lebzien, 2012). Thus, we investigated how menthol-containing PBLC might affect ruminal microbiota and fermentation in vivo. The PBLC did not affect SCFA and ammonia concentrations or estimated methane production at the two doses tested. Our results are in line with a previous study that showed no effect of feeding peppermint herb (50 g/kg DM) to lactating Holstein cows on total SCFA concentration or molar proportion of acetate, propionate, and butyrate though digestibility of nutrients was lowered (Hosoda et al., 2005). Ando et al. (2003) also found no changes in ruminal SCFA concentration or molar SCFA proportion in steers supplemented with 200 g dried peppermint herb (equivalent to 29 g/kg diet). Assuming a menthol content of the applied peppermint supplements of ∼1% (Beigi et al., 2018), the PBLC doses used in those two previous studies were likely ∼5 times higher than the two doses used in our study. Hence, menthol does not appear to markedly change ruminal SCFA production when applied at dosages that have any practical relevance in vivo.
According to previous studies (Larue et al., 2005; Pitta et al., 2010), the solid and the liquid fractions of rumen digesta have different microbiota. This can be explained by the fact that types and availability of substrates differ between the solid and the liquid fractions of the ruminal content, causing differences in the microbiota composition between these two fractions (de Menezes et al., 2011; Petri et al., 2012; Klevenhusen et al., 2017).
Supplementation of PBLC increased the microbial phylogenetic diversity and the number of observed species-level OTUs numerically (P = 0.14) in the solid fraction. This seems surprising since most studies revealed inhibitory or no effects of different PBLC on ruminal microbiota (Patra and Yu, 2012, 2015a; Schären et al., 2017, 2018). The inhibitory effect was also noted for menthol-containing peppermint oil that decreased the abundances of total bacteria, archaea, and select bacterial species including Ruminococcus albus, Ruminococcus flavefaciens, and Fibrobacter succinogenes in ruminal cultures in vitro (Patra and Yu, 2012). In agreement with the present study, however, peppermint oil increased species richness in a previous in vitro study, while garlic oil decreased richness when added at the same dose (0.5 g/L) (Patra and Yu, 2015b). Collectively, menthol at low or medium doses may increase diversity in the rumen by inhibiting some dominant bacteria.
The PBLC treatments altered the relative abundance of only a few taxa, and most of them were unclassified genera or species of bacteria. Among the classified genera, Paludibacter and BF311 were increased or increased linearly by PBLC. Paludibacter (e.g., P. propionicigenes) can ferment soluble sugars, soluble starch, and glycogen to predominantly propionate and acetate, but cannot utilize cellulose, xylan, fumarate, malate, lactate, succinate or pyruvate (Ueki et al., 2006). Little is known about the substrates and fermentation products of BF311, which has no cultured representative, but our correlation analysis suggests that this genus might interact with Prevotella, a major genus in the rumen (Bi et al., 2018).
Our initial hypothesis was that PBLC could have differential effects on the microbiota in the solid vs. the liquid fractions because those fractions have different microbiota (Belanche et al., 2017; Klevenhusen et al., 2017). However, we did not find much differential effect of PBLC between the two fractions except for YRC22 and Butyrivibrio, with the former increasing its relative abundance in the liquid fraction while the latter expanding its relative abundance in the solid fraction with the increased doses of PBLC. This is different to our previous study in vitro (Patra and Yu, 2014) where Butyrivibrio fibrisolvens, a Gram-positive butyrate-producing species, was unaffected by peppermint oil but inhibited by oregano oil at the same dose. The discrepancy may be explained either by not having separate data for solid and liquid fractions or by the much higher dose of menthol-rich PBLC in the previous study performed in vitro. The metabolism of YRC22 is not known because there is no known cultured representative, but it was predominant in the rumen of cattle (Klevenhusen et al., 2017) and increased due to dietary propionate supplementation (Yao et al., 2017) and decreased due to 1% nitrate addition (Zhao et al., 2015). Taken together, PBLC affected the abundance of certain ruminal bacteria, but these changes were rather small and were only marginally fraction-specific.
Ruminal archaeal taxa, i.e., Euryarchaeota and US_Methanosphaera in the solid fraction, Thermoplasmata and its family Methanomassiliicoccaceae and genus vadinCA11 in the liquid fraction, were significantly decreased in the PBLC groups. Thermoplasmata is a class of methylotrophic methanogens, and it was also inhibited by rapeseed oil in lactating cows (Poulsen et al., 2013). These changes in archaea might suggest decreased methanogenesis by these taxa. Peppermint oil decreased methane production and total archaeal number in the ruminal fluid when used at very high concentrations in vitro (Agarwal et al., 2009; Patra and Yu, 2012) and acted similarly at lower doses in vivo in lactating dairy cattle (50 g/kg peppermint herbs; Hosoda et al., 2005). The relative proportions of individual SCFA have been shown to vary when methane production is altered (Patra, 2010), which has been used to estimate methane production from SCFA profiles in some studies (Wolin, 1960; Moss et al., 2000). The methane production estimated from the SCFA concentrations, however, did not change in response to the PBLC treatments. Albeit those estimates have to be interpreted with great care, they may suggest that changes in archaea do not necessarily cause an alteration in methane production, possibly due to compensation by the other methanogenic archaea (Ohene-Adjei et al., 2008).
A correlation network existed between SCFA and some of the detected microbial taxa. Propionate and acetate showed correlations with one (i.e., US_RF39) and three (US_Mogibacteriaceae, US_RF39, and Anaerostipes sp.) OTUs, respectively, in the solid fraction, whereas those acids were not correlated to any OTUs in the liquid fraction. This might imply that acetate and propionate production is highly redundant in the ruminal microbiota. In fact, acetate and propionate concentrations in ruminal fluid are higher compared to the other SCFA, and many diverse ruminal microbes are involved in the production of these SCFA in their energy yielding pathways (Russell and Wallace, 1997). Butyrate had the most complex correlation network in both the solid- and liquid-associated microbiota. Moreover, three OTUs (US_Ruminococcaceae 1, Ruminococcus sp., and US_Clostridiales 2) all showed negative correlations to butyrate concentration in both the solid and the liquid fractions. This suggests that a rather limited number of microbes may be involved in the regulation of the production of butyrate (Diez-Gonzalez et al., 1999). Furthermore, the more complex networks in the solid fraction may imply that microbiota therein may be of greater importance for SCFA production in general and butyrate production in particular (Petri et al., 2012; Klevenhusen et al., 2017).
One striking finding of the present study was that the correlation networks between ruminal microbial OTUs and SCFA had almost no overlap between the Control group and the two PBLC-treated groups. Whereas propionate correlated with four microbial OTUs (i.e., US_BS11, US_Prevotellaceae, US_Paraprevotellaceae, and Pseudobutyrivibrio sp.) in the Control group, it was not correlated to any microbial taxa in the PBLC groups. Relationships between the other five measured SCFA and microbial taxa were also rather sparse in the PBLC groups compared to the Control. Importantly, the negative correlation between OTU14 (Ruminococcus spp.) and butyrate was the only correlation that occurred in both the Control and the PBLC groups. All other correlations were different between the Control and the PBLC treatments. This indicates that PBLC profoundly altered the correlation networks among OTUs and SCFA even though the relative abundance of only a few microbes was altered by PBLC and SCFA concentrations were unchanged. Previous studies had shown that diet could alter the metabolic networks in the rumen (Tapio et al., 2017; Wolff et al., 2017), however, we are not aware of any study that showed such effect on the correlation networks among OTUs and SCFA induced by PBLC.
We also used co-occurrence networks to evaluate how PBLC might influence interactions among different taxa of ruminal microbes. We noted that most of the predominant taxa extensively interacted in both the Control and the PBLC groups. There were many unique interactions in the PBLC groups, which were absent in the Control group and vice versa. Thus, the PBLC treatment might extensively affect the microbial interactions despite the minor effects on rumen microbiota composition and relative abundance of major taxa and no detectable changes in SCFA production. The mechanistic explanations of these interactions are not known and neither it is known how they are affected by PBLC. The influence of diet on microbial interactions is at a similarly descriptive state (Henderson et al., 2015; Tapio et al., 2017). Thus, further research is warranted to better understand the physiology of the ruminal microbiota using metagenomics and metatranscriptomics in combination with fermentation profile analyses (Tapio et al., 2017).
As expected, most of the predicted functions differed between the solid- and the liquid-associated microbiota, which was further confirmed by PCA that separated solid and liquid fractions by the first two principal components. The differential functions between the two fractions were consistent with the finding of the study of Mao et al. (2015). Overall, although a few functional categories were affected by PBLC, no distinct separation among the treatments was seen on the PCA plot, suggesting that PBLC had little effect on the metabolic function of the microbiota. This once again highlights the functional redundancy of the ecosystem where the microbiota can adapt to fulfill its metabolic functions under varying environmental conditions.
Menthol-rich PBLC at practically relevant dosages (80–160 mg/d; equivalent to 57–114 mg/kg diet DM) elicits a few distinct changes in microbiota composition, including a reduction of certain methanogens and an increase in microbiota diversity, especially among the particle-colonizing bacteria. However, the menthol-rich PBLC did not change ruminal microbial fermentation. Considering the changes in microbial composition and diversity, it appears surprising that PBLC rich in menthol did not exert a significant effect on ruminal fermentation. By demonstrating changes in the microbial and metabolic networks during PBLC supplementation, the present study indicates that stable fermentation is maintained during minor microbial alterations as a result of metabolic redundancy of the ruminal ecosystem. These findings suggest that correlation network analysis can add novel dimensions to the understanding of ruminal microbial interactions, which may be of practical relevance for targeted manipulation of ruminal fermentation. Further research is needed to elucidate and confirm these interactions.
The datasets generated for this study can be found in NCBI Sequence Read Archive (SRA), PRJNA529255.
The study was performed with ethical approval by the local authorities, the Landesamt für Gesundheit und Soziales, Berlin, Germany (LAGeSo, filed under G0141/17).
AP, SG, H-SB, and JA designed and conducted the experiment. AP and RP designed the feeding. TP, RP, and JA took responsibility for the microbiota, SCFA and ammonia analyses, respectively. AP analyzed all final data sets and created tables and figures. AP wrote the manuscript together with JA, ZY, and TP. H-SB, AP, and JA obtained funding for this study. All authors reviewed, provided comments and approved the final version of the manuscript.
This work was supported by a fellowship from the Alexander von Humboldt Foundation to AP and accessory bench fees. The study received further financial support from PerformaNat GmbH. Open Access Funding was provided by the Freie Universität Berlin.
H-SB is a member of PerformaNat GmbH, Germany, which funded parts of the study. This fact had no influence on the acquisition and interpretation of results and does not affect authors’ adherence to the publishing policies of Frontiers.
The remaining authors declare that the research was conducted in the absence of any commercial or financial relationships that could be construed as a potential conflict of interest.
We gratefully acknowledge the support by the barn staff of the animal facility. We further acknowledge support by the Open Access Publication Initiative of Freie Universität Berlin.
The Supplementary Material for this article can be found online at: https://www.frontiersin.org/articles/10.3389/fmicb.2019.02038/full#supplementary-material
Agarwal, N., Shekhar, C., Kumar, R., Chaudhary, L. C., and Kamra, D. N. (2009). Effect of peppermint (Mentha piperita) oil on in vitro methanogenesis and fermentation of feed with buffalo rumen liquor. Anim. Feed Sci. Technol. 148, 321–327. doi: 10.1016/j.anifeedsci.2008.04.004
Ando, S., Nishida, T., Ishida, M., Hosoda, K., and Bayaru, E. (2003). Effect of peppermint feeding on the digestibility, ruminal fermentation and protozoa. Livest. Prod. Sci. 82, 245–248. doi: 10.1016/s0301-6226(03)00012-5
Beigi, M., Torki-Harchegani, M., and Pirbalouti, A. G. (2018). Quantity and chemical composition of essential oil of peppermint (Mentha × piperita L.) leaves under different drying methods. Int. J. Food Prop. 21, 282–291.
Belanche, A., Newbold, C. J., Lin, W., Rees Stevens, P., and Kingston-Smith, A. H. (2017). A systems biology approach reveals differences in the dynamics of colonization and degradation of grass vs. hay by rumen microbes with minor effects of vitamin E supplementation. Front. Microbiol. 8:1456. doi: 10.3389/fmicb.2017.01456
Bi, Y., Zeng, S., Zhang, R., Diao, Q., and Tu, Y. (2018). Effects of dietary energy levels on rumen bacterial community composition in Holstein heifers under the same forage to concentrate ratio condition. BMC Microbiol. 18:69. doi: 10.1186/s12866-018-1213-9
Bolyen, E., Rideout, J. R., Dillon, M. R., Bokulich, N. A., Abnet, C., Al-Ghalith, G. A., et al. (2018). QIIME 2: reproducible, interactive, scalable, and extensible microbiome data science. PeerJ Preprints 6:e27295v2.
Callahan, B. J., McMurdie, P. J., Rosen, M. J., Han, A. W., Johnson, A. J. A., and Holmes, S. P. (2016). DADA2: high-resolution sample inference from Illumina amplicon data. Nat. Methods 13, 581–583. doi: 10.1038/nmeth.3869
Calsamiglia, S., Busquet, M., Cardozo, P. W., Castillejos, L., and Ferret, A. (2007). Invited review: essential oils as modifiers of rumen microbial fermentation. J. Dairy Sci. 90, 2580–2595. doi: 10.3168/jds.2006-644
Caporaso, J. G., Lauber, C. L., Walters, W. A., Berg-Lyons, D., Lozupone, C. A., Turnbaugh, P. J., et al. (2011). Global patterns of 16S rRNA diversity at a depth of millions of sequences per sample. Proc. Natl. Acad. Sci. U.S.A. 108, 4516–4522. doi: 10.1073/pnas.1000080107
Chowdhury, S., Mandal, G. P., Patra, A. K., Samanta, I., Pradhan, S., Kumar, P., et al. (2018). Different essential oils in diets of broiler chickens: 2. Gut microbes and morphology, immune response, and some blood profile and antioxidant enzymes. Anim. Feed Sci. Technol. 236, 39–47. doi: 10.1016/j.anifeedsci.2017.12.003
Cobellis, G., Trabalza-Marinucci, M., and Yu, Z. (2016). Critical evaluation of essential oils as rumen modifiers in ruminant nutrition: a review. Sci. Total Environ. 545-546, 556–568. doi: 10.1016/j.scitotenv.2015.12.103
de Menezes, A. B., Lewis, E., O’Donovan, M., O’Neill, B. F., Clipson, N., and Doyle, E. M. (2011). Microbiome analysis of dairy cows fed pasture or total mixed ration diets. FEMS Microbiol. Ecol. 78, 256–265. doi: 10.1111/j.1574-6941.2011.01151.x
Diez-Gonzalez, F., Bond, D. R., Jennings, E., and Russell, J. B. (1999). Alternative schemes of butyrate production in Butyrivibrio fibrisolvens and their relationship to acetate utilization, lactate production and phylogeny. Arch. Microbiol. 171, 324–330. doi: 10.1007/s002030050717
Fadrosh, D. W., Ma, B., Gajer, P., Sengamalay, N., Ott, S., Brotman, R. M., et al. (2014). An improved dual-indexing approach for multiplexed 16S rRNA gene sequencing on the Illumina MiSeq platform. Microbiome 2:6. doi: 10.1186/2049-2618-2-6
Flachowsky, G., and Lebzien, P. (2012). Effects of phytogenic substances on rumen fermentation and methane emissions: a proposal for a research process. Anim. Feed Sci. Technol. 176, 70–77. doi: 10.1016/j.anifeedsci.2012.07.009
Hausmann, J., Deiner, C., Immig, I., Pieper, R., Starke, A., and Aschenbach, J. R. (2017). Effects of combined supplementation with plant bioactive lipid compounds and biotin on ruminal fermentation, body condition and energy metabolism in transition dairy cows. Anim. Feed Sci. Technol. 225, 27–37. doi: 10.1016/j.anifeedsci.2017.01.009
Hausmann, J., Deiner, C., Patra, A. K., Immig, I., Starke, A., and Aschenbach, J. R. (2018). Effects of a combination of plant bioactive lipid compounds and biotin compared with monensin on body condition, energy metabolism and milk performance in transition dairy cows. PLoS One 13:e0193685. doi: 10.1371/journal.pone.0193685
Henderson, G., Cox, F., Ganesh, S., Jonker, A., and Young, W., Global Rumen Census Collaborators, et al. (2015). Rumen microbial community composition varies with diet and host, but a core microbiome is found across a wide geographical range. Sci. Rep. 5:14567. doi: 10.1038/srep14567
Hosoda, K., Nishida, T., Park, W.-Y., and Eruden, B. (2005). Influence of Mentha×piperita L. (peppermint) supplementation on nutrient digestibility and energy metabolism in lactating dairy cows. Asian-Australas. J. Anim. Sci. 18, 1721–1726. doi: 10.5713/ajas.2005.1721
Hutton, P., White, C. L., Durmic, Z., and Vercoe, P. E. (2009). Eremophila glabra is an Australian plant that reduces lactic acid accumulation in an in vitro glucose challenge designed to simulate lactic acidosis in ruminants. Animal 3, 1254–1263. doi: 10.1017/S1751731109004789
Kazemi-Bonchenari, M., Falahati, R., Poorhamdollah, M., Heidari, S. R., and Pezeshki, A. (2018). Essential oils improved weight gain, growth and feed efficiency of young dairy calves fed 18 or 20% crude protein starter diets. J. Anim. Physiol. Anim. Nutr. 102, 652–661. doi: 10.1111/jpn.12867
Khiaosa-ard, R., and Zebeli, Q. (2013). Meta-analysis of the effects of essential oils and their bioactive compounds on rumen fermentation characteristics and feed efficiency in ruminants. J. Anim. Sci. 91, 1819–1830. doi: 10.2527/jas.2012-5691
Klevenhusen, F., Petri, R. M., Kleefisch, M. T., Khiaosa-Ard, R., Metzler-Zebeli, B. U., and Zebeli, Q. (2017). Changes in fibre-adherent and fluid-associated microbial communities and fermentation profiles in the rumen of cattle fed diets differing in hay quality and concentrate amount. FEMS Microbiol. Ecol. 93, doi: 10.1093/femsec/fix100
Kumar, P., Patra, A. K., Mandal, G. P., and Debnath, B. C. (2018). Carcass characteristics, chemical and fatty acid composition and oxidative stability of meat from broiler chickens fed black cumin (Nigella sativa) seeds. J. Anim. Physiol. Anim. Nutr. 102, 769–777. doi: 10.1111/jpn.12880
Langille, M. G., Zaneveld, J., Caporaso, J. G., McDonald, D., Knights, D., Reyes, J. A., et al. (2013). Predictive functional profiling of microbial communities using 16S rRNA marker gene sequences. Nat. Biotechnol. 31, 814–821. doi: 10.1038/nbt.2676
Larue, R., Yu, Z., Parisi, V. A., Egan, A. R., and Morrison, M. (2005). Novel microbial diversity adherent to plant biomass in the herbivore gastrointestinal tract, as revealed by ribosomal intergenic spacer analysis and rrs gene sequencing. Environ. Microbiol. 7, 530–543. doi: 10.1111/j.1462-2920.2005.00721.x
Mao, S., Zhang, M., Liu, J., and Zhu, W. (2015). Characterising the bacterial microbiota across the gastrointestinal tracts of dairy cattle: membership and potential function. Sci. Rep. 5:16116. doi: 10.1038/srep16116
McIntosh, F. M., Williams, P., Losa, R., Wallace, R. J., Beever, D. A., and Newbold, C. J. (2003). Effects of essential oils on ruminal microorganisms and their protein metabolism. Appl. Environ. Microbiol. 69, 5011–5014. doi: 10.1128/AEM.69.8.5011-5014.2003
Mirzaei-Alamouti, H., Moradi, S., Shahalizadeh, Z., Razavian, M., Amanlou, H., Harkinezhad, T., et al. (2016). Both monensin and plant extract alter ruminal fermentation in sheep but only monensin affects the expression of genes involved in acid-base transport of the ruminal epithelium. Anim. Feed Sci. Technol. 219, 132–143. doi: 10.1016/j.anifeedsci.2016.06.009
Moss, A. R., Jouany, J. P., and Newbold, J. (2000). Methane production by ruminants: its contribution to global warming. Ann. Zootechnie 49, 231–253. doi: 10.1051/animres:2000119
National Research Council [NRC] (2007). Nutrient Requirements of Small Ruminants: Sheep, Goats, Cervids, and New World Camelids. Washington, DC: The National Academies Press, doi: 10.17226/11654
Naumann, K., Bassler, R., Seibold, R., and Barth, K. (2004). Die Chemische Untersuchung von Futtermitteln. Darmstadt: VDLUFA-Verlag.
Ohene-Adjei, S., Chaves, A. V., McAllister, T. A., Benchaar, C., Teather, R. M., and Forster, R. J. (2008). Evidence of increased diversity of methanogenic archaea with plant extract supplementation. Microb. Ecol. 56, 234–242. doi: 10.1007/s00248-007-9340-0
Patra, A. K. (2010). Meta-analyses of effects of phytochemicals on digestibility and rumen fermentation characteristics associated with methanogenesis. J. Sci. Food Agric. 90, 2700–2708. doi: 10.1002/jsfa.4143
Patra, A. K. (2011). Effects of essential oils on rumen fermentation, microbial ecology and ruminant production. Asian J. Anim. Vet. Adv. 6, 416–428. doi: 10.3923/ajava.2011.416.428
Patra, A. K. (2012). “An overview of antimicrobial properties of different classes of phytochemicals,” in Diet Phytochemicals and Microbes, ed. A. K. Patra (Dorrecht: Springer), 1–32. doi: 10.1007/978-94-007-3926-0_1
Patra, A. K., Amasheh, S., and Aschenbach, J. R. (2018). Modulation of gastrointestinal barrier and nutrient transport function in farm animals by natural plant bioactive compounds - a comprehensive review. Crit. Rev. Food Sci. Nutr. 11, 1–30. doi: 10.1080/10408398.2018.1486284
Patra, A. K., Park, T., Kim, M., and Yu, Z. (2017). Rumen methanogens and mitigation of methane emission by anti-methanogenic compounds and substances. J. Anim. Sci. Biotechnol. 8:13. doi: 10.1186/s40104-017-0145-9
Patra, A. K., and Saxena, J. (2009). Dietary phytochemicals as rumen modifiers: a review of the effects on microbial populations. Antonie van Leeuwenhoek 96, 363–375. doi: 10.1007/s10482-009-9364-1
Patra, A. K., and Saxena, J. (2010). A new perspective on the use of plant secondary metabolites to inhibit methanogenesis in the rumen. Phytochemistry 71, 1198–1222. doi: 10.1016/j.phytochem.2010.05.010
Patra, A. K., and Yu, Z. (2012). Effects of essential oils on methane production and fermentation by, and abundance and diversity of, rumen microbial populations. Appl. Environ. Microbiol. 78, 4271–4280. doi: 10.1128/AEM.00309-12
Patra, A. K., and Yu, Z. (2014). Effects of vanillin, quillaja saponin, and essential oils on in vitro fermentation and protein-degrading microorganisms of the rumen. Appl. Microbiol. Biotechnol. 98, 897–905. doi: 10.1007/s00253-013-4930-x
Patra, A. K., and Yu, Z. (2015a). Effects of adaptation of in vitro rumen culture to garlic oil, nitrate, and saponin and their combinations on methanogenesis, fermentation, and abundances and diversity of microbial populations. Front. Microbiol. 6:1434. doi: 10.3389/fmicb.2015.01434
Patra, A. K., and Yu, Z. (2015b). Essential oils affect populations of some rumen bacteria in vitro as revealed by microarray (RumenBactArray) analysis. Front. Microbiol. 6:297. doi: 10.3389/fmicb.2015.00297
Petri, R. M., Forster, R. J., Yang, W., McKinnon, J. J., and McAllister, T. A. (2012). Characterization of rumen bacterial diversity and fermentation parameters in concentrate fed cattle with and without forage. J. Appl. Microbiol. 112, 1152–1162. doi: 10.1111/j.1365-2672.2012.05295.x
Pieper, R., Boudry, C., Bindelle, J., Vahjen, W., and Zentek, J. (2014). Interaction between dietary protein content and the source of carbohydrates along the gastrointestinal tract of weaned piglets. Arch. Anim. Nutr. 68, 263–280. doi: 10.1080/1745039X.2014.932962
Pitta, D. W., Pinchak, E., Dowd, S. E., Osterstock, J., Gontcharova, V., Youn, E., et al. (2010). Rumen bacterial diversity dynamics associated with changing from bermudagrass hay to grazed winter wheat diets. Microb. Ecol. 59, 511–522. doi: 10.1007/s00248-009-9609-6
Poulsen, M., Schwab, C., Jensen, B. B., Engberg, R. M., Spang, A., Canibe, N., et al. (2013). Methylotrophic methanogenic Thermoplasmata implicated in reduced methane emissions from bovine rumen. Nat. Commun. 4:1428. doi: 10.1038/ncomms2432
Roy, D., Tomar, S. K., and Kumar, V. (2015). Rumen modulatory effect of thyme, clove and peppermint oils in vitro using buffalo rumen liquor. Vet. World 8, 203–207. doi: 10.14202/vetworld.2015.203-7
Russell, J. B., and Wallace, R. J. (1997). “Energy yielding and consuming reactions,” in The Rumen Microbial Ecosystem, eds P. N. Hobson and C. S. Stewart (London: Blackie Academic & Professional), 246–282. doi: 10.1007/978-94-009-1453-7_6
Schären, M., Drong, C., Kiri, K., Riede, S., Gardener, M., Meyer, U., et al. (2017). Differential effects of monensin and a blend of essential oils on rumen microbiota composition of transition dairy cows. J. Dairy Sci. 100, 2765–2783. doi: 10.3168/jds.2016-11994
Schären, M., Frahm, J., Kersten, S., Meyer, U., Hummel, J., Breves, G., et al. (2018). Interrelations between the rumen microbiota and production, behavioral, rumen fermentation, metabolic and immunological attributes of dairy cows. J. Dairy Sci. 101, 4615–4637. doi: 10.3168/jds.2017-13736
Segata, N., Izard, J., Waldron, L., Gevers, D., Miropolsky, L., Garrett, W. S., et al. (2011). Metagenomic biomarker discovery and explanation. Genome Biol. 12:R60. doi: 10.1186/gb-2011-12-6-r60
Shannon, P., Markiel, A., Ozier, O., Baliga, N. S., Wang, J. T., Ramage, D., et al. (2003). Cytoscape: a software environment for integrated models of biomolecular interaction networks. Genome Res. 13, 2498–2504. doi: 10.1101/gr.1239303
Smeti, S., Hajji, H., Mekki, I., Mahouachi, M., and Atti, N. (2018). Effects of dose and administration form of rosemary essential oils on meat quality and fatty acid profile of lamb. Small Rumin. Res. 158, 62–68. doi: 10.1016/j.smallrumres.2017.10.007
Soltan, Y. A., Natel, A. S., Araujo, R. C., Morsy, A. S., and Abdalla, A. L. (2018). Progressive adaptation of sheep to a microencapsulated blend of essential oils: ruminal fermentation, methane emission, nutrient digestibility, and microbial protein synthesis. Anim. Feed Sci. Technol. 237, 8–18. doi: 10.1016/j.anifeedsci.2018.01.004
Szczechowiak, J., Szkudelska, K., Szumacher-Strabel, M., Sadkowski, S., Gwozdz, K., El-Sherbiny, M., et al. (2018). Blood hormones, metabolic parameters and fatty acid proportion in dairy cows fed condensed tannins and oils blend. Ann. Anim. Sci. 18, 155–166. doi: 10.1515/aoas-2017-0039
Tapio, I., Fischer, D., Blasco, L., Tapio, M., Wallace, R. J., Bayat, A. R., et al. (2017). Taxon abundance, diversity, co-occurrence and network analysis of the ruminal microbiota in response to dietary changes in dairy cows. PLoS One 12:e0180260. doi: 10.1371/journal.pone.0180260
The European Commission (2018). Commission implementing regulation (EU) 2018/245 of 15 February 2018 concerning the authorisation of menthol, d-carvone, menthyl acetate, d,l-isomenthone, 3-methyl- 2-(pent-2(cis)-enyl)cyclopent-2-en-1-one, 3,5,5-trimethylcyclohex-2-en-1-one, d-fenchone, fenchyl alcohol, carvyl acetate, dihydrocarvyl acetate and fenchyl acetate as feed additives for all animal species. Official J. Eur. Union L 53, 87–104.
Ueki, A., Akasaka, H., Suzuki, D., and Ueki, K. (2006). Paludibacter propionicigenes gen. nov., sp. nov., a novel strictly anaerobic, Gram-negative, propionate-producing bacterium isolated from plant residue in irrigated rice-field soil in Japan. Int. J. Syst. Evol. Microbiol. 56, 39–44. doi: 10.1099/ijs.0.63896-0
Van Bibber-Krueger, C. L., Miller, K. A., Aperce, C. C., Alvarado-Gilis, C. A., Higgins, J. J., and Drouillard, J. S. (2016). Effects of crystalline menthol on blood metabolites in Holstein steers and in vitro volatile fatty acid and gas production. J. Anim. Sci. 94, 1170–1178. doi: 10.2527/jas.2015-8779
Wang, Q., Garrity, G. M., Tiedje, J. M., and Cole, J. R. (2007). Naive Bayesian classifier for rapid assignment of rRNA sequences into the new bacterial taxonomy. Appl. Environ. Microbiol. 73, 5261–5267. doi: 10.1128/aem.00062-07
Wink, M. (2003). Evolution of secondary metabolites from an ecological and molecular phylogenetic perspective. Phytochemistry 64, 3–19. doi: 10.1016/s0031-9422(03)00300-5
Wolff, S. M., Ellison, M. J., Hao, Y., Cockrum, R. R., Austin, K. J., Baraboo, M., et al. (2017). Diet shifts provoke complex and variable changes in the metabolic networks of the ruminal microbiome. Microbiome 5:60. doi: 10.1186/s40168-017-0274-6
Wolin, M. J. (1960). A theoretical rumen fermentation balance. J. Dairy Sci. 43, 1452–1459. doi: 10.3168/jds.s0022-0302(60)90348-9
Yao, Q., Li, Y., Meng, Q., and Zhou, Z. (2017). The effect of calcium propionate on the ruminal bacterial community composition in finishing bulls. Asian-Australas. J. Anim. Sci. 30, 495–504. doi: 10.5713/ajas.16.0469
Yu, Z., and Morrison, M. (2004). Improved extraction of PCR-quality community DNA from digesta and fecal samples. Biotechniques 36, 808–812. doi: 10.2144/04365st04
Keywords: correlation network analysis, microbiota composition, menthol, ruminal fermentation, sheep
Citation: Patra AK, Park T, Braun H-S, Geiger S, Pieper R, Yu Z and Aschenbach JR (2019) Dietary Bioactive Lipid Compounds Rich in Menthol Alter Interactions Among Members of Ruminal Microbiota in Sheep. Front. Microbiol. 10:2038. doi: 10.3389/fmicb.2019.02038
Received: 04 May 2019; Accepted: 19 August 2019;
Published: 04 September 2019.
Edited by:
Emilio M. Ungerfeld, Institute of Agricultural Research, ChileReviewed by:
Anusorn Cherdthong, Khon Kaen University, ThailandCopyright © 2019 Patra, Park, Braun, Geiger, Pieper, Yu and Aschenbach. This is an open-access article distributed under the terms of the Creative Commons Attribution License (CC BY). The use, distribution or reproduction in other forums is permitted, provided the original author(s) and the copyright owner(s) are credited and that the original publication in this journal is cited, in accordance with accepted academic practice. No use, distribution or reproduction is permitted which does not comply with these terms.
*Correspondence: Jörg R. Aschenbach, am9lcmcuYXNjaGVuYmFjaEBmdS1iZXJsaW4uZGU=
Disclaimer: All claims expressed in this article are solely those of the authors and do not necessarily represent those of their affiliated organizations, or those of the publisher, the editors and the reviewers. Any product that may be evaluated in this article or claim that may be made by its manufacturer is not guaranteed or endorsed by the publisher.
Research integrity at Frontiers
Learn more about the work of our research integrity team to safeguard the quality of each article we publish.