- 1Department of Biomedical Sciences, Rochester Institute of Technology, Rochester, NY, United States
- 2Thomas H. Gosnell School of Life Sciences, Rochester Institute of Technology, Rochester, NY, United States
Bovine trypanosomosis is a devastating disease that causes huge economic loss to the global cattle industry on a yearly basis. Selection of accurate biomarkers are important in early disease diagnosis and treatment. Of late, micro-RNAs (miRNAs) are becoming the most useful biomarkers for both infectious and non-infectious diseases in humans, but this is not the case in animals. miRNAs are non-coding RNAs that regulate gene expression through binding to the 3′-, 5′-untranslated regions (UTR) or coding sequence (CDS) region of one or more target genes. The molecular identification of miRNAs that regulates the expression of immune genes responding to bovine trypanosomosis is poorly defined, as is the possibility that these miRNAs could serve as potential biomarkers for disease diagnosis and treatment currently unknown. To this end, we utilized in silico tools to elucidate conserved miRNAs regulating immune response genes during infection, in addition to cataloging significant genes. Based on the p value of 1.77E-32, we selected 25 significantly expressed immune genes. Using prediction analysis, we identified a total of 4,251 bovine miRNAs targeting these selected genes across the 3′UTR, 5′UTR and CDS regions. Thereafter, we identified candidate miRNAs based on the number of gene targets and their abundance at the three regions. In all, we found the top 13 miRNAs that are significantly conserved targeting 7 innate immune response genes, including bta-mir-2460, bta-mir-193a, bta-mir-2316, and bta-mir-2456. Our gene ontology analysis suggests that these miRNAs are involved in gene silencing, cellular protein modification process, RNA-induced silencing complex, regulation of humoral immune response mediated by circulating immunoglobulin and negative regulation of chronic inflammatory response, among others. In conclusion, this study identifies specific miRNAs that may be involved in the regulation of gene expression during bovine trypanosomosis. These miRNAs have the potential to be used as biomarkers in the animal and veterinary research community to facilitate the development of tools for early disease diagnosis/detection, drug targeting, and the rational design of drugs to facilitate disease treatment.
Introduction
Every year, the Cattle industry is faced with huge economic losses due to diseases such as trypanosomosis. Bovine trypanosomosis is endemic in sub-Saharan Africa and some parts of The Americas, causing losses of several billion dollars annually (Abdi et al., 2017; Odeniran and Ademola, 2018). The disease is associated with multifactorial complex phenotype, requiring an integrative biological approach to elucidate the molecular networks involved and to identify significant markers that may be useful for disease diagnosis and treatment (Noyes et al., 2011; Smetko et al., 2015; Kim et al., 2017). Recently, major research efforts are focused on utilizing microRNA (miRNA) as diagnostic biomarkers or therapeutic targets due to their involvement in various disease phenotypes and malignancies (Lawless et al., 2014; Scheel et al., 2017; Ammah et al., 2018; da Silveira et al., 2018). MicroRNAs are short, non-coding RNAs, which are capable of post-transcriptionally modifying gene expression, and form complexes with argonaute proteins that hybridize to the target mRNAs leading to the degradation of mRNA and negatively impact protein expression (Bushati and Cohen, 2007; Bartel, 2009; Meister, 2013; Huberdeau et al., 2017).
Post-transcriptional modification of gene expression is becoming a major focus in disease studies (Liu and Wang, 2019), with several reports showing miRNAs as important players in post-transcriptional regulation of both innate and adaptive immune gene expression (O’Connell et al., 2010; Sandhu et al., 2012; Kramer et al., 2015; Mehta and Baltimore, 2016; Pichulik et al., 2016; Kumar et al., 2018; Awuah et al., 2019). miRNAs have also been implicated in the regulation of immune functions in different cell types, for example neutrophil senescence and naïve mouse B cells have been reported to be regulated by repertoires of miRNAs (Ward et al., 2011; Bronevetsky et al., 2013; Aalaei-Andabili and Rezaei, 2016; Kangas et al., 2017). While there are numerous miRNAs abundantly expressed in bovine tissues and the entire genome, there are very few studies elucidating their regulatory role in bovine immunity (Coutinho et al., 2007; Jin et al., 2009; Xu et al., 2009; Hanif et al., 2018; Li et al., 2019). MicroRNAs therefore, will play important and unique roles during immune response, including modification of gene expression during bovine trypanosomosis. For instance, mir-2284 and mir-2285 families have been associated with TLR genes, thereby regulating non-specific immune responses through the production of pro-inflammatory cytokines, suggesting a role in bovine disease tolerance (Das et al., 2016). Similarly, mir-2404, mir-2285, and mir-6522 have been reported to regulate CD8 alpha chain and T-cell surface glycoprotein, thus playing significant role in development of T and B cell, proliferation, and inflammatory process (Mahjoub et al., 2015; Hanif et al., 2018). Studies have shown that miRNAs repress the target genes through the 3′ untranslated region (3′UTR) of mRNAs, therefore the miRNA binding site in this region seems to be well characterized (Buza et al., 2014; Liu and Wang, 2019). However, comparative genome analysis has shown that both coding domain sequence (CDS) and 3′UTR sites are under selective pressure while many miRNA binding sites are conserved in both 3′UTR and CDS (Hausser et al., 2013; Brummer and Hausser, 2014). In fact, many investigators are suggesting an alternative mode of gene regulation, whereby miRNAs anneal within the CDS, 5′- and/or 3′-UTR regions of their targets thereby regulating their translation.
Hence, there remains an important need to identify possible miRNA binding sites within the complete sequence of a gene (i.e., 5′-UTR, CDS and 3′-UTR), which can serve as potential biomarkers for disease diagnosis and treatment. To date, there has not been a published study identifying potential miRNAs as biomarkers in the entire sequence of immune genes responding during bovine trypanosomosis, demonstrating the uniqueness of this study. Notably, there are many miRNA databases and algorithms, which are available to investigate significant pathways and transcriptional mechanisms within various interaction and expression datasets (Xie et al., 2013; Li et al., 2014; Hanif et al., 2018). There are also proven in silico tools and algorithms available for miRNA target prediction and for dissecting miRNA function (Kogelman et al., 2014; Scheel et al., 2017; Hanif et al., 2018). A quantitative measurement of miRNA expression in conjunction with their target gene expression would be an important means to deconvolute diverse biogenesis pathways and immune system regulation during bovine trypanosomosis. To this end, we have employed a combination of computational tools to identify and elucidate conserved miRNAs, their target interaction(s), and regulatory function(s) during bovine trypanosomosis. This study, we believe, is a proof of concept with the potential to guide future studies aiming to identify attractive and putative miRNA targets for the rationale design and/or discovery of drugs to combat diseases, including potential biomarkers for disease diagnosis.
Materials and Methods
Data Mining and Verification of Genes Associated With Bovine Trypanosomosis Pathway
In this study, we performed a data survey of genes that are reported to be significantly regulated during bovine trypanosomosis through publicly available databases. Briefly, we used LitInspector of Genomatix Literature Mining software (Version 3.10, Munich, Germany1) that uses proprietary literature data mining algorithms based on all available PubMed publications and their corresponding Medical Subject Headings (MeSH) (Berriz et al., 2003; Maier et al., 2005). This program gathers gene information and correlations with diseases and pathways from published literature. The gene sets are characterized based on annotation and literature including MeSH terms, gene ontology (GO) and pathways, as described (Scherf et al., 2005; Frisch et al., 2009). Out of the 3,738 disease terms found in the MeSH database, we selected only one term relevant for our study, “trypanosomosis” (MeSH-Term ID: c03.752.300.900), using bovine as our reference species. The list of individual genes in association with bovine trypanosomosis as found in the scientific literature was filtered for significance to avoid random matches (Buza et al., 2014). Based on the parameters defined above, we selected a total of 25 genes that are significantly regulated and associated with our disease term based on the MeSH p-value (p = 1.77E-32). The 25 gene sets were then used as targets for further analysis (gene functions and references in Supplementary Table S1).
Prediction and Extraction of Disease Associated Bovine miRNA
We searched for the identified 25 genes from our disease pathway and their locations within the bovine genome UMD 3.1 using the Ensembl BLAST/BLAT Genomic Sequence tool2. Complete sequence of the 25 genes were retrieved with their accession number: CD86 (XM_005201387), FcγR3A (NM_001077402), CD1A (NM_001105456), IL-6 (NM_173923), IFN-G (NM_174086), CD4 (NM_001103225), CXCL-8 (NM_173923), ICAM-1 (NM_174348), CSF2 (NM_174028), CD14 (XM_005209429), TLR-4 (NM_174198), LBP (NM_001038674), IL-10 (NM_174088), TLR-2 (NM_174197), CCL2 (NM_174006), MYD88 (NM_001014382), TNF (NM_173966), CD83(NM_001046590), CD80 (NM_001206439), IL-4 (NM_173921), IL-18 (NM_174091), LY96 (NM_001046517), ITGAM (NM_001039957), IL-12A (NM_174355), and MAPKAPK3 (NM_001034779). Thereafter, each gene was used as target to individually search the Bos taurus genome for possible miRNAs within their complete sequences (i.e., 3′-UTR, 5′-UTR and CDS) using miRWalk3; an online program which allows search for interactions between complete gene sequences and miRNAs using the TarPmiR algorithm. To avoid false positive miRNAs in our study, three other miRNA-target prediction software (TargetScan4, miRDB5 and miRBase6) were used to predict the miRNA and targets interaction for each gene. Only miRNAs that were confirmed in the three databases were included for further analysis. All miRNAs that were found from each gene target were partitioned into three regions; the 5′-UTR, CDS and 3′-UTR in order to examine the variation in miRNA abundance at each region of the targets. In order to identify common miRNAs among the three regions of each gene sequence, we sorted the matches using a web- based Venn diagram application7.
Network Analysis, Interaction of miRNA and Target Location of the Chromosome
Using the Ensembl BLAST/BLAT and the miRBase, we locate the position of each miRNA and their targets on the chromosomes within the bovine genome. All mature miRNAs sequences of our targets were retrieved; genes located on the same chromosome were pooled together for us to identify concomitant miRNAs co-regulating one or more targets with the aid of an online Venn diagram8. miRNA-target network was constructed with most significant genes in the disease pathway using miRNet; an online platform that integrate miRNAs, targets and their functions9 (Fan and Xia, 2018).
Analysis of 7 Innate Immune Genes Associated With Bovine Trypanosomosis
We selected seven innate immune genes among the 25 that are responding in bovine trypanosomosis pathway for further analysis. Briefly, we used the accession numbers of TLR-4 (NM_174198), ITGAM (NM_001039957), ICAM-1 (NM_174348), CD14 (XM_005209429), TLR-2 (NM_174197), LBP (NM_001038674), and TNF-α (NM_173966) to query the databases of miRWalk10 and miRBase11 in order to retrieve the mature and precursor miRNA sequences within the targets for further analysis. We selected only common miRNAs from the two databases as candidates for further analysis. Based on the number of targets by a miRNA, we selected the top 10 miRNAs regulating four to five targets. It is assumed that a miRNA with multiple targets is probably a key miRNA in the innate immune system under study (Newman and Hammond, 2010; Wang, 2010). We also compared the miRNA abundance at 5′-UTR, CDS and 3′-UTR of the selected top 10. This is also necessary assuming the regions with the most miRNAs will be more regulated and have greater impact on disease outcome.
Evolutionary Trace and Phylogenetic Analysis of Conserved Bovine miRNA With Other Species
In order to determine the evolutionary trace and conservation of the identified bovine miRNA from the seven innate immune response genes, we selected the two miRNAs that showed the highest conservation from the 5′-UTR and 3′-UTR and 10 from the CDS being that it presented the most abundant miRNAs. Here, precursor miRNA sequences were retrieved from the miRBase database (see footnote 11); each bovine miRNA sequence was used for BLAST search with Ensembl BLAST/BLAT Genomic Sequence tool12. Homologous sequences from other species for each bovine miRNA were downloaded from the database for further analysis. Multiple sequence alignment (MAS) was performed using MEGA vs7 and Neighbor joining (NJ) phylogenetic tree was constructed with Cluster W to determine the nucleotide substitution and evolutionary divergence among the species. It is assumed that the most conserved miRNAs would be candidates for disease studies.
Gene Ontology and Functional Analysis of the Most Conserved Bovine miRNAs in Innate Immune System
We performed functional enrichment analysis using gene ontology (GO) term13 and DIANA tools14 for the topmost conserved miRNAs at the 3′UTR, 5′UTR and CDS of the 7 innate immune response genes to gain understanding of the possible biological processes and physiological pathways regulated by these miRNAs according to Paraskevopoulou et al. (2013). We used 13 topmost miRNAs from the 7 innate immune genes and 6 others that are significantly conserved from CXCL-8, TLR-4, and MAPKAPK3 (Supplementary Table S2), giving a total of 19 miRNAs to search the gene ontology database to collect the relevant biological processes and the GO terms. A higher stringency was imposed by considering only the GO terms with p < 0.001 as significantly enriched. Where GO terms were not found for certain bovine miRNAs, closely related and annotated miRNAs in other species were used as functional homologs.
Results
Identification of Candidate miRNAs and Gene Targets Associated With Bovine Trypanosomosis
Our data mining revealed a total of 25 genes which were significantly regulated during bovine trypanosomosis. From the prediction analysis, we identified 4,251 bovine miRNAs that target these 25 genes spanning the 3′UTR, 5′UTR and the CDS regions (Table 1). The number of miRNAs per gene ranges from 32 as seen in LY96 to 578 in MAPKAPK3 gene. As shown in Figure 1 and Table 1, about 8 other genes showed a higher density with over 200 miRNAs. It is observed that ICAM-1 and ITGAM genes had similar miRNA number (220).
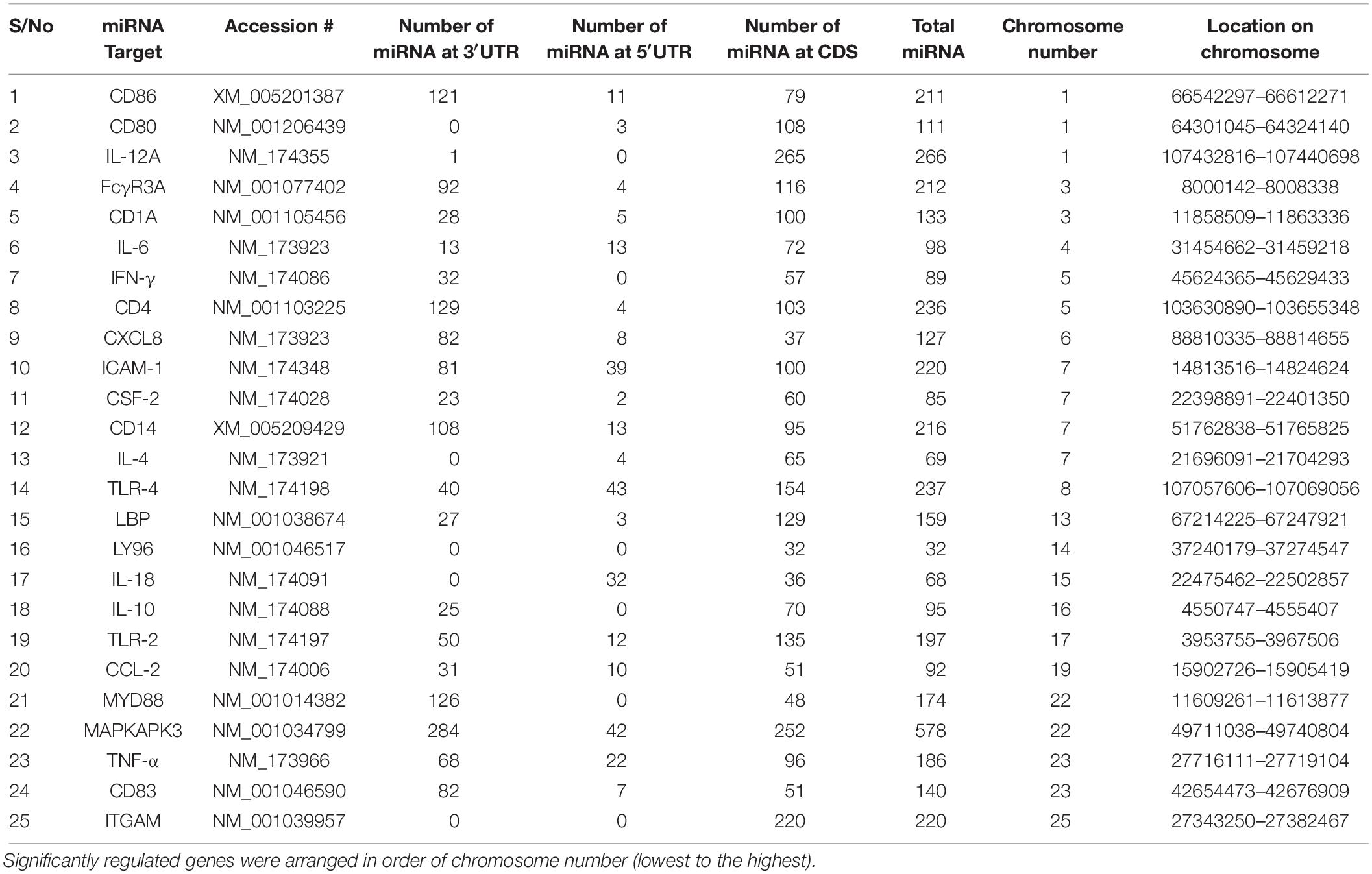
Table 1. List of significantly regulated genes during bovine trypanosomiasis and the number of miRNA at the 3′UTR, 5′UTR and CDS regions.
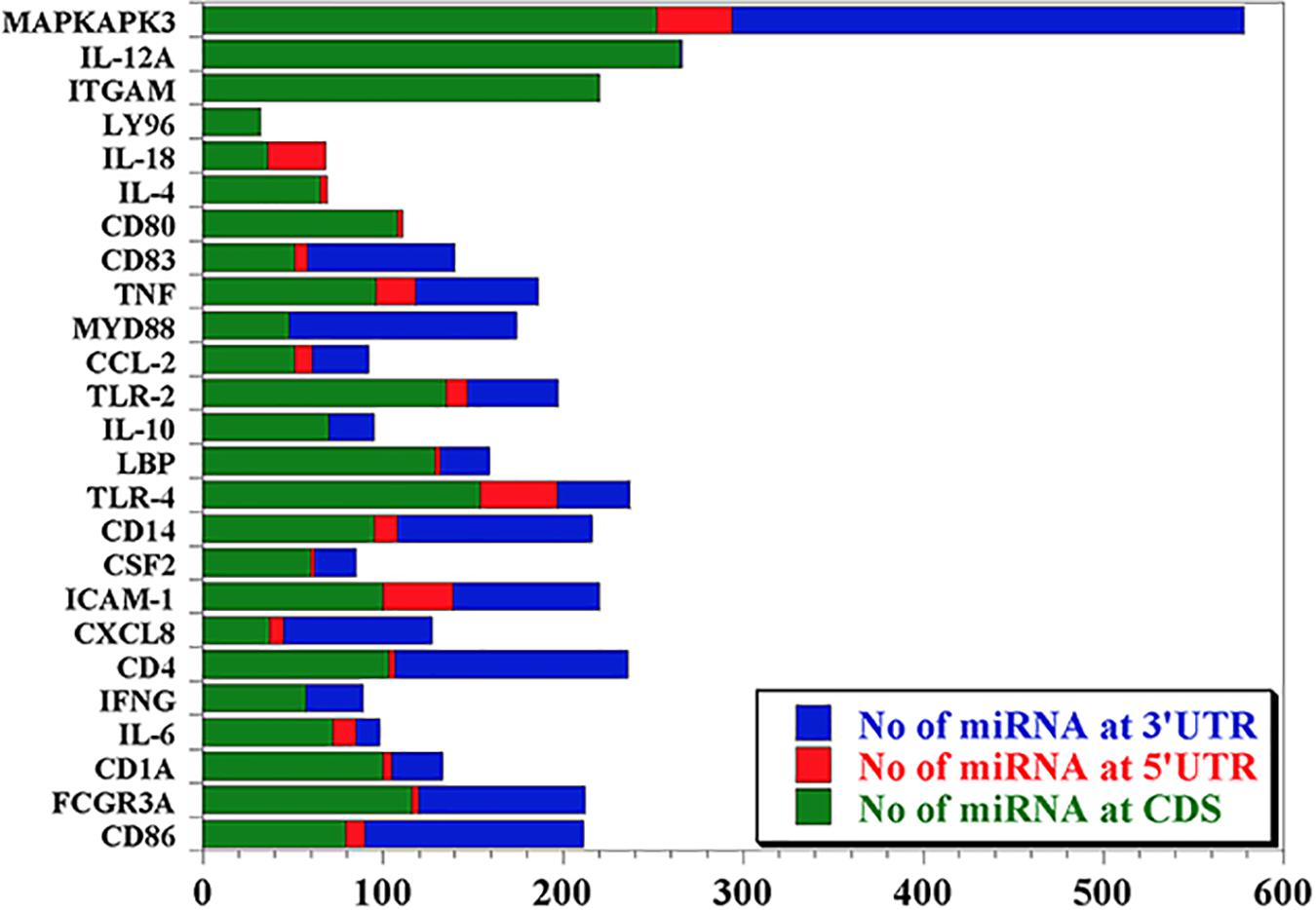
Figure 1. Predicted miRNA abundance targeting 25 genes significantly regulated during bovine trypanosomosis.
In order to understand the distribution of the miRNAs across the nucleotide sequence of each gene, we performed miRNA-target prediction for the 3′UTR, 5′UTR and the CDS regions using miRWalk. Generally, the CDS region presents the highest number of miRNAs for most genes followed by the 3′UTR, while the 5′UTR has the lowest (Figure 2). We observed that genes like LY96 and ITGAM have no miRNA at both 3′UTR and 5′UTR; CD80, IL-4 and IL-18 have no miRNA at the 3′UTR, with IL-12A, IFN-γ, IL-1- and MYD88 presenting no miRNA at the 5′UTR.
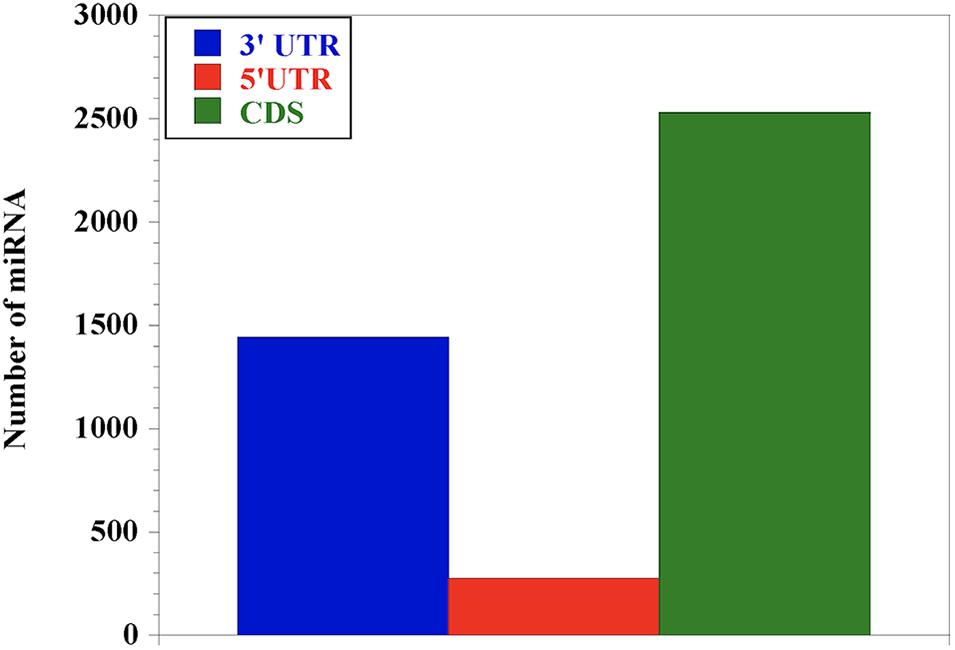
Figure 2. Distribution of miRNA abundance at the 3′UTR, 5′UTR and CDS of the 25 genes responding during bovine trypanosomosis. Our results show that miRNAs are relatively abundant in the coding sequence (CDS) region, indicating the importance of this region in gene regulation, followed by the 3′UTR. The 5′UTR had the least number of miRNAs.
To examine the most conserved miRNAs across the 2 or 3 regions, we compared the overlapping miRNAs for each gene, with the assumption that miRNA present at two or three regions will be conserved with important biological inferences. Notably, there are more matches of miRNAs between the 3′UTR and CDS region with FcγR3A, CD14 and MAPKAPK3 genes presenting 18, 20 and 99 matches respectively while CD80, IL-4, IL-18, LY96, ITGAM and IL-12A had no match of miRNA at the 3 regions (Table 2 and Supplementary Table S2). Overall, we observed that only CXCL-8, TLR-4, and MAPKAPK3 genes had conserved miRNAs present in their 3′UTR, 5′UTR and the CDS regions; bta-miR-2454-5p in CXCL-8, bta-miR-2328-3p in TLR-4, bta-miR-1777a, bta-miR-652 and bta-miR-7863 in MAPKAPK3 (Supplementary Table S2).
Conserved Bovine miRNAs With Different Chromosomes
We gathered miRNA from genes located on the same chromosome and we identified common miRNAs which may give biological relevance to our study. In all, we found a total of 15 out of 25 selected genes located on the same chromosome with chromosome 7 having 4 genes (CSF2, CD14, ICAM-1, and IL-4), followed by chromosome 1 possessing 3 genes (CD80, CD86, and IL-12A) while chromosomes 3, 5, 22 and 23 have 2 genes each in common (Figures 3A–F). MAPKAPK3 and MYD88 on chromosome 22 has the highest number of common miRNAs with a total of 130; while TNF-α and CD83 on chromosome 23 have 53 miRNAs in common. Thirty common miRNAs are presented between CD80, CD88, and IL-12A.
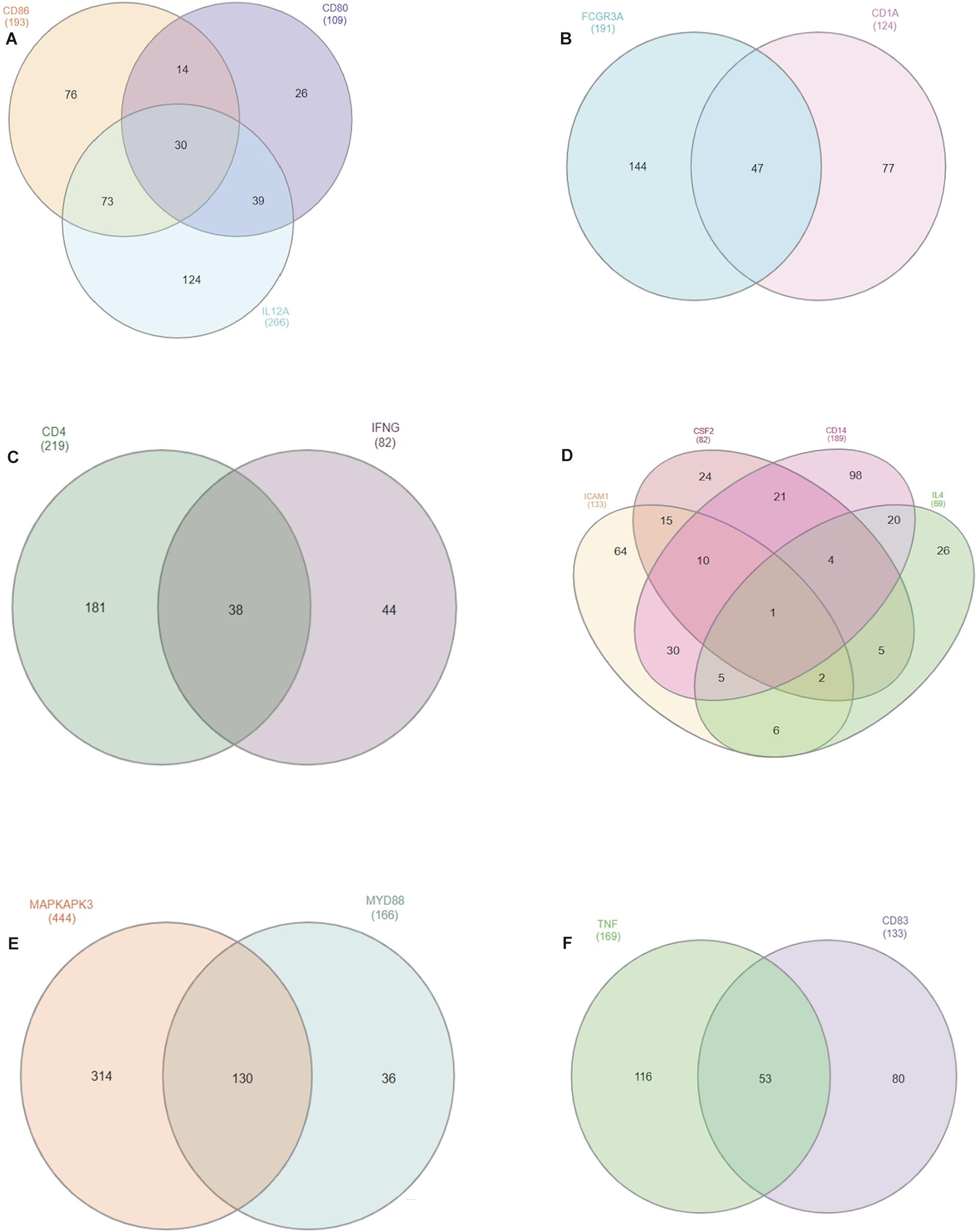
Figure 3. Venn diagram of conserved bovine miRNAs within different chromosomes (A: chromosome 1; B: chromosome 3; C: chromosome 5; D: chromosome 7; E: chromosome 22; F: chromosome 23). Overlapping regions in the Venn diagram represent the number of conserved miRNAs between one or two genes in the same chromosome.
Network Analysis of Bovine miRNA-Target Interactome During Bovine Trypanosomosis
miRNA-target network was constructed in order to identify significant association and connections between miRNAs and different targets during bovine trypanosomosis. Out of the 25 selected genes and 4,251 miRNAs that were subjected to network analysis, only 13 genes and 54 miRNAs were significant connected in our network (Figure 4). Overall, based on the size of each node, CD14, MYD88, TNF-α, and IL-10 were significant nodes detected from the network analysis. Likewise, bta-mir-2888, bta-mir-2394, bta-mir-1284, and bta-mir-2467-p were major miRNA nodes connecting two or more genes in the network.
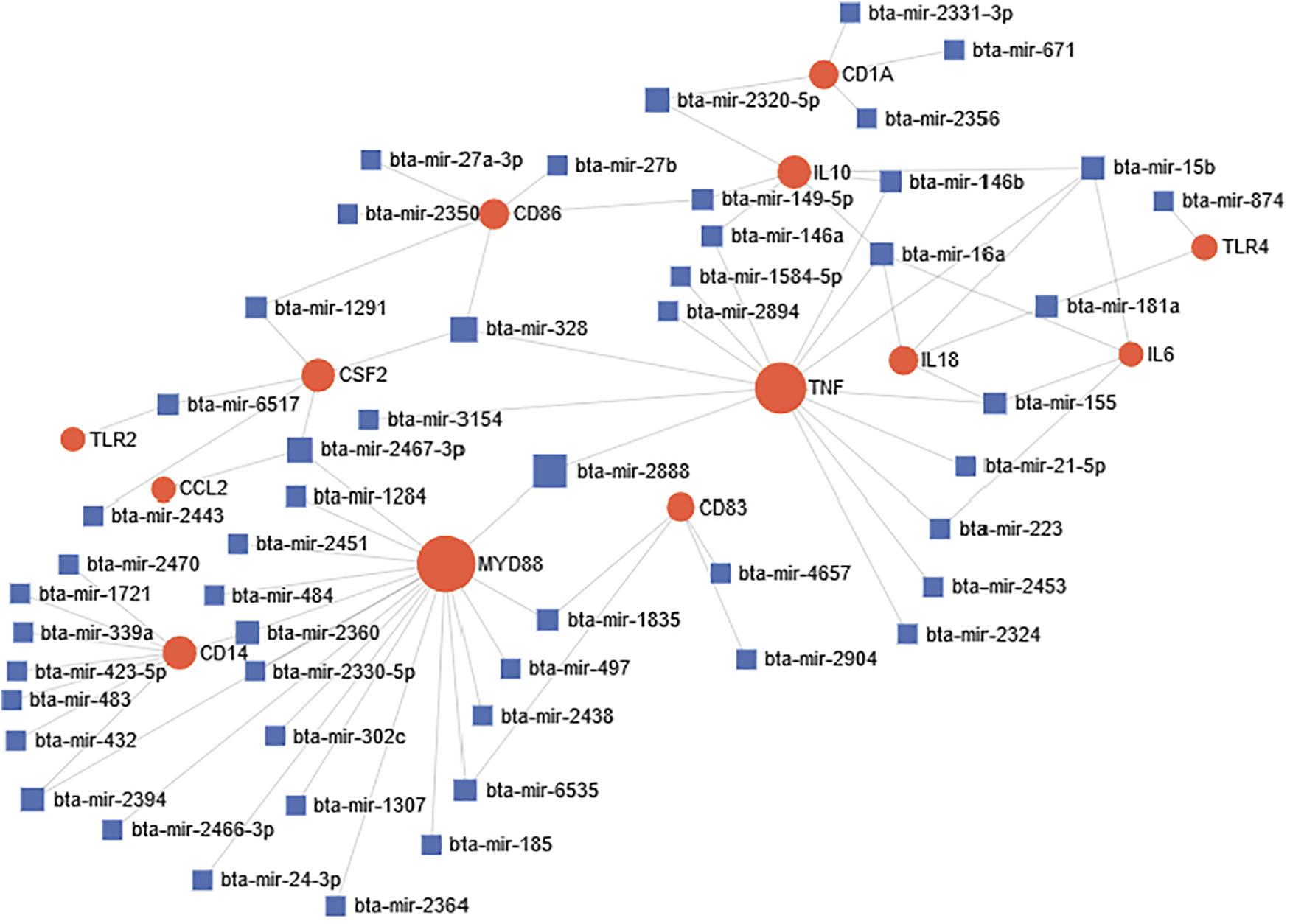
Figure 4. Predicted miRNA-target interactome network during bovine trypanosomosis. Circular node shape with red color represents all significantly connected genes within the network during the disease condition. Node size simulates the number of interactions, which is directly proportional. Each miRNA in the network is represented by square shape and color blue. Most important miRNA nodes joined one or two genes together thereby affecting their expression simultaneously.
Prediction of Conserved miRNAs-Target Interaction in 7 Innate Immune Response Genes
We focused on identifying miRNAs that are concomitantly present in the 7 innate immune response genes among the list of 25 genes that are significantly responding to bovine trypanosomosis. Here, we postulate that bovine miRNAs targeting the greatest number of genes will be potential candidates as biomarkers for disease diagnosis or treatment. From Tables 3–5, we present the prediction analysis of miRNA-target interactions and their matured sequences. We showed the top 10 bovine miRNAs and their targets based on the 3′UTR, 5′UTR, and the CDS regions. Our selection is based on the miRNAs that target between 3-5 genes out of the selected 6 innate immune response genes. We observe that the CDS region has miRNAs that target the most genes; 5 out of the 7 genes at a time. For example, bta-miR-2349 targets CD14, ITGAM, TLR-2, TLR-4, and TNF-α while bta-miR-193a-5p targets ICAM-1, ITGAM, LBP, TLR-2, and TNF-α (Table 3). Following the CDS region is the 3′UTR, while the 5′UTR has the least number of miRNAs, targeting less than 4 genes (Tables 4, 5). bta-miR-2460 from the 3′UTR is demonstrated to target CD14, ICAM-1, TLR-4, and TNF-α genes, while bta-miR-2374 are targeting CD14, ICAM-1, LBP, and TLR-4 gene sets (Table 4), indicating they are the topmost in this region. Only bta-miR-2392 is targeting ICAM-1, TLR-2, TLR-4 and TNF-α from the 5′UTR (Table 5).
Table 6 shows the number of miRNAs common to a gene pair from each region. Our analyses reveal that the highest number of bovine miRNAs common between two genes are located at the CDS region. There are 55 miRNAs shared between TLR-4 and ITGAM, 54 between LBP and ITGAM, 52 between TLR-2 and ITGAM, 44 between ITGAM and ICAM-1, and 40 between TNF-α and ITGAM. Notably, CD14 and TNF-α have the highest common miRNA (15) at the 3′UTR followed by 10 between CD14 and TLR-4 (Table 6).
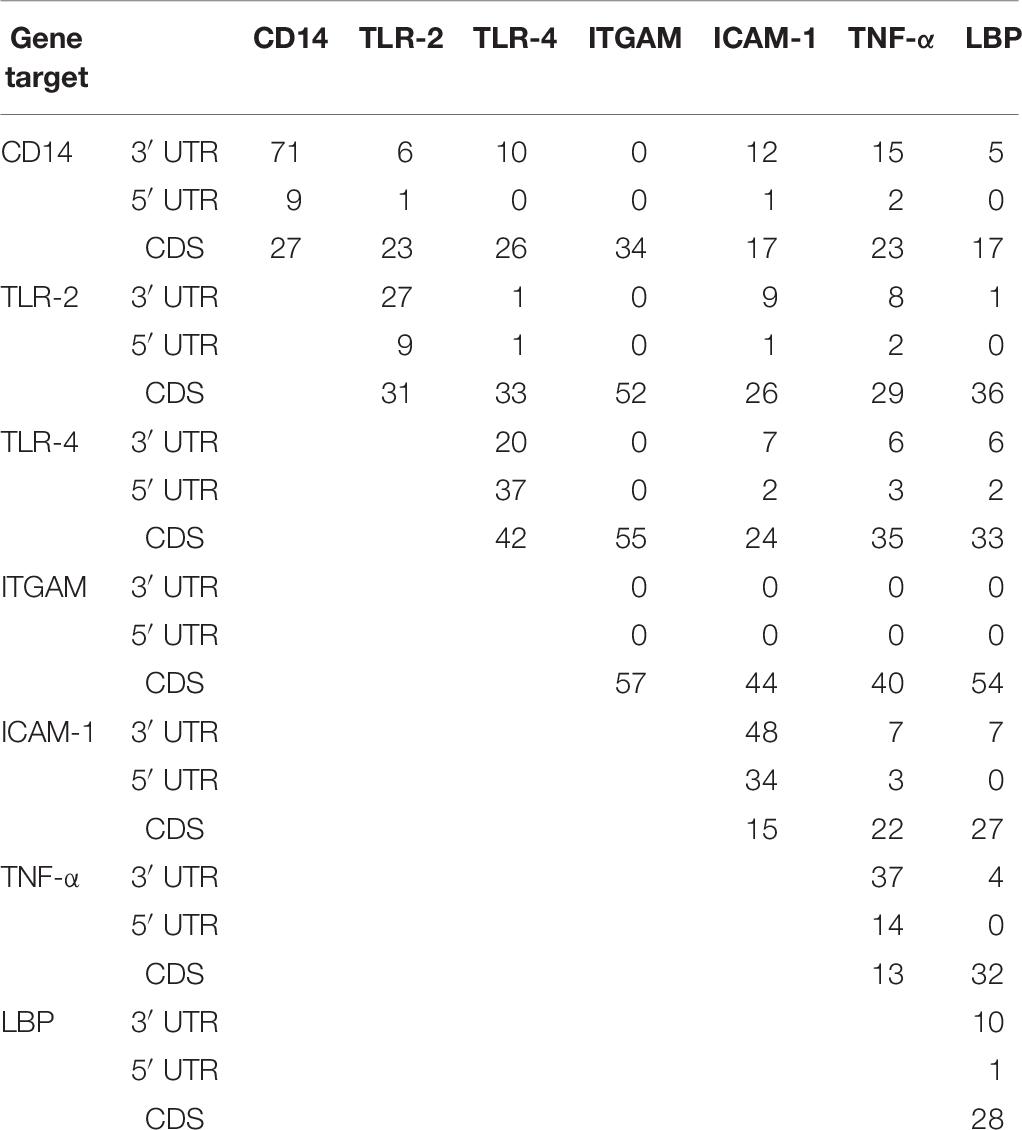
Table 6. Number of concomitant miRNAs at the 3′UTR, 5′UTR and CDS regions of seven innate immune response genes.
Study of Evolutionarily Conserved Bovine miRNA Among the 7 Innate Immune Response Genes and Other Species
Phylogenetic analyses were performed to examine the evolutionarily conserved bovine miRNAs with other species. To be more stringent, only miRNAs that target 4 out of 7 innate immune response genes were included in the analysis (one from the 5′UTR, 2 from 3′UTR and 10 from the CDS). Analysis of all the 10 miRNAs at the CDS with their homologous sequences from other species identified bta-mir-193a as highly conserved with human, monkey, dog, pig, chicken, gorilla, mouse, rat, rabbit, and alligator (Figure 5). In the same manner, bovine bta-mir-4657 clusters with a human homolog hsa-mir-4657, bta-mir-2888-2 with hsa-mir-6803 and bta-mir-2349 with hsa-mir-4769 (Figure 5). Furthermore, we individually searched for the conserved homologs of the 13 miRNAs selected for stringency (one from the 5′UTR, 2 from 3′UTR, and 10 from the CDS) in order to determine their evolutionary trace with other species. Figures 6A–M show different clusters of each miRNA with their homologs from other species. Based on the number of homologs found with other species, bta-mir-2888-1 (Figure 6J) with 14 homologs has the highest followed by bta-mir-193a with 13 (Figure 6A), bta-mir-2888-2, bta-mir-2392, and bta-mir-2349 have 11, 9 and 8 homologs respectively (Figures 6E,G,K). bta-mir-2422 presented the least homolog with rat (rno-mir-327) and mouse (mmu-mir-327) (Figure 6H).
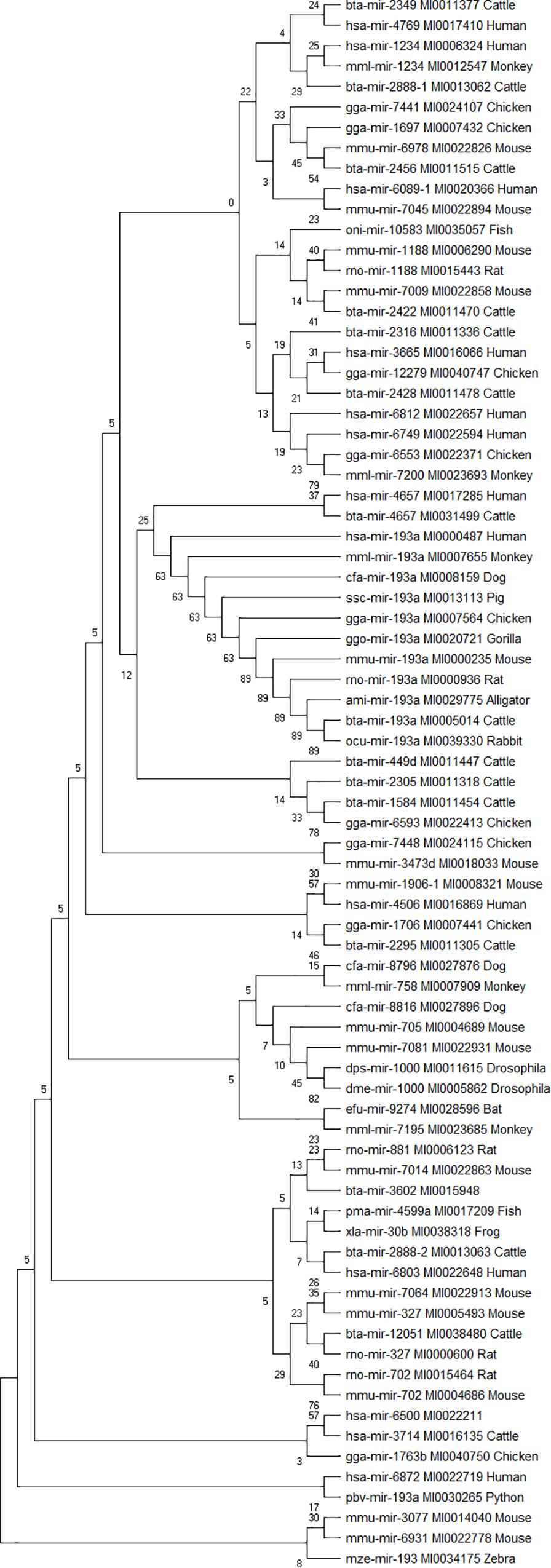
Figure 5. Phylogenetic tree of 13 bovine miRNA and their homologs in other species. The evolutionary trace was inferred using the Neighbor-Joining method. The tree is drawn to scale, with branch lengths in the same units as those of the evolutionary distances used to infer the phylogenetic tree. The evolutionary distances were computed using the p-distance method and are in the units of the number of nucleotide sequence differences per site. The analysis involved 77 nucleotide sequences.
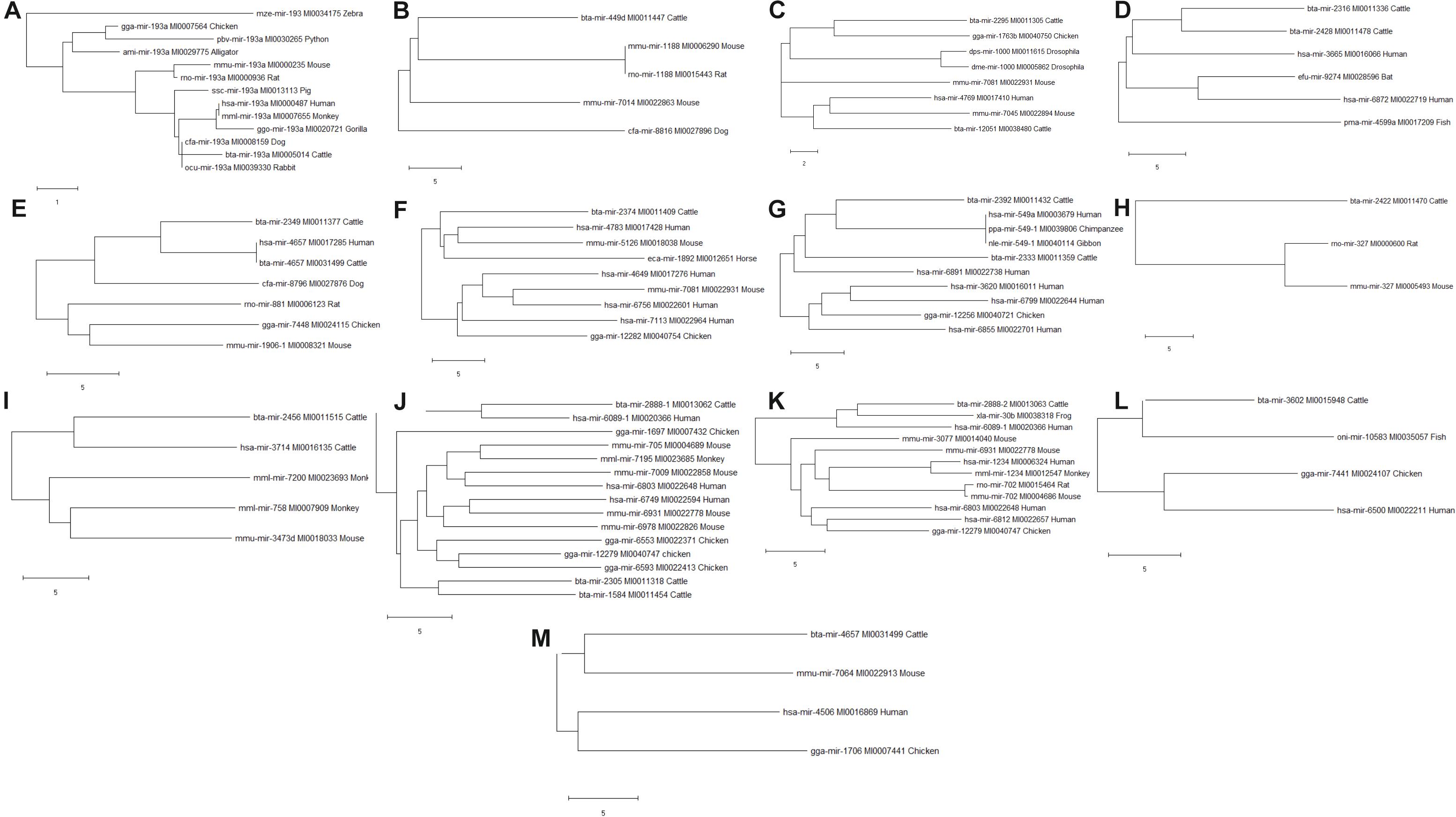
Figure 6. Evolutionarily trace of conserved bovine miRNA among the 7 innate immune genes and other species (A: bta-mir-193a; B: bta-mir-449d; C: bta-mir-2295; D: bta-mir-2316; E: bta-mir-2349; F: bta-mir-2374; G: bta-mir-2392; H: bta-mir-2422; I: bta-mir-2456; J: bta-mir-2888-1; K: bta-mir-2888-2; L: bta-mir-3602; M: bta-mir-4657).
Enrichment Analysis and GO Terms of the Conserved miRNAs
In order to better elucidate the possible biological and physiological processes regulated by the identified bovine miRNAs, 19 predicted conserved miRNAs were subjected to GO term analysis using the sub-term biological process as the focus. Topmost GO terms were selected for each tested miRNA as significantly enriched based on the p-value of less than 0.001. From the analysis, we found only 10 out of the 19 bovine miRNAs being functionally annotated with GO terms (Table 7). We infer the functional annotation of the remaining 9 bovine miRNAs from closely related species (in parentheses) based on the homology between 95-100%. We found that bta-mir-2460, bta-mir-193a, bta-mir-2328-3p, bta-mir-652, bta-mir-2392, and bta-mir-2454 are significantly involved with gene silencing (GO:0035195) and RNA-induced silencing complex (GO:0016442); bta-mir-2295 is involved with signal transduction in response to DNA damage (GO:0042770); bta-mir-2422 is involved with regulation of humoral immune response mediated by circulating immunoglobulin (GO:0002923), and bta-mir-2888-2 is involved with 2-aminobenzenesulfonate metabolic process (GO:0018868), among others (Table 7).
Discussion
Identification of molecular biomarkers associated with diseases is important in early disease diagnosis, drug target and treatment. miRNAs are increasingly emerging as molecular biomarkers because of their essential role in gene regulation both in animals and plants during disease condition (Buza et al., 2014; Agarwal et al., 2015; Scheel et al., 2017). In this study, we identified and elucidated conserved miRNAs from a list of gene targets important in innate immune response during bovine trypanosomosis through computational prediction analysis. Previous studies have focused on the 3′UTR region to study the miRNA-target interactions while few others suggested that miRNAs can anneal within the 3′UTR, 5′UTR or CDS regions of their target to regulate gene expression (Erhard et al., 2014; Yang et al., 2017; da Silveira et al., 2018). This study however brings a unique perspective through identification of conserved miRNAs targeting immune genes responding during bovine trypanosomosis by analyzing the complete sequence of the gene which include the 3′-, 5′ UTRs and the CDS. Our study showed a significantly higher density of miRNA binding sites at the CDS region for all genes, identifying it as an important region which may play a significant regulatory role in expression during the disease condition. Studies have shown that target genes having many miRNA binding sites at the CDS region can be easily degraded (Schnall-Levin et al., 2011; Hausser and Zavolan, 2014). Likewise, Ott et al. (2011) and Chi et al. (2009) discovered that there are increasing miRNA target sites at the CDS region of mammalian transcripts during immunoprecipitation studies. This observation is in line with our results, suggesting that CDS sites may have specific function in regulating gene expression rather than the previous focus on the 3′UTR (Hausser et al., 2013). Brummer and Hausser (2014) stated that the CDS sites may enhance the repertoire of miRNA regulation than the 3′UTR sites because of their interaction with alternative polyadenylation, alternative splicing and the general gene architecture.
In addition, our study identified significant overlaps of miRNA binding sites between the 3′UTR and CDS regions, indicating possible evolutionary conservation of these regulatory elements between the two regions (Hurst, 2006; Gaidatzis et al., 2007; Forman et al., 2008). Published reports have shown that miRNAs simultaneously targeting both 3′UTR and CDS regions of mRNAs are more regulated than those targeted by 3′UTR only (Fang and Rajewsky, 2011; Bazzini et al., 2012). Therefore, we adjudge that conserved miRNAs found targeting both regions have undergone selective pressure, and are therefore evolutionarily conserved, which may induce mRNA translational inhibition and degradation of immune gene response during bovine trypanosomosis.
Furthermore, we found significant miRNAs cluster for genes located on the same chromosome, the most indication suggesting evolutionary conservation and functional roles on their targets. Thus, there is the possibility that expression of two or more genes on the same chromosome can be co-regulated by a single miRNA, and this observation might be important for quantifying gene regulation during disease condition or as targets for treatment. Pande et al. (2018) and Hanif et al. (2018) support the finding that miRNAs clusters across cattle genome have variable functions through large evolutionary distance.
Additionally, our study showed networks between miRNAs and target genes, which are significantly connected during bovine trypanosomosis. From our network interactome, CD14, TLR-2, TLR-4, IL10 MYD88, TNF-α, bta-mir-2360, bta-mir-1835, bta-mir-2888, bta-mir-146b and bta-mir-2320-5p amongst others are important drivers and connectors within the network, indicating they are major players during disease condition. Studies have shown that molecular interactions between genes, proteins, RNA and other biological molecules contribute to cellular performance and homeostasis (Govindaraju et al., 2012; Ji et al., 2018; Wang et al., 2018). The co-expression of these miRNAs within the network may regulate the interaction of certain gene sets such as MYD88 and TNF-α, CD14 and TLR-2, IL-18, and TLR-4, among others. Studies to date have demonstrated the importance of CD14 and TLR gene family in facilitating pattern recognition of pathogen-associated molecules and to elicit innate immune response against viruses, bacteria, fungi, and protozoa (Seabury et al., 2010; Ojurongbe et al., 2019; Morenikeji and Thomas, 2019b). Likewise, MYD88 is a signal transduction adaptor, transferring signals from interleukin-1 (IL-1) receptors and Toll-like receptors, and driving macrophage release of cytokines, imperative in innate immune response to exogenous pathogenic stimuli. Therefore, a significant overlap of miRNAs targeting these genes altogether could work synergistically as putative markers for disease discovery, characterization and therapeutic manipulations for bovine trypanosomiasis treatment.
Moreover, among the 7 innate immune response genes considered in this study, our functional analysis show that bta-mir-2460, bta-mir-193a, bta-mir-2349, bta-mir-3602, bta-mir-2454, and bta-mir-652 are significantly enriched within biological processes such as gene silencing, RNA-induced silencing complex, and cellular protein modification process. These miRNAs can potentially regulate CD14, ITGAM, LBP, TLR-2, TLR-4, and TNF-α genes and their expression might be key regulatory factors that determine disease susceptibility, tolerance or resistance in cattle. We have shown that CD14 is particularly implicated in disease tolerance among cattle with bovine trypanosomosis, with significant expression among trypanotolerant animals and the reverse among trypanosusceptible ones (Morenikeji and Thomas, 2019a). Notably, some studies have also shown that bta-mir-2460, bta-mir-193a, and bta-mir-652 are significantly involved in biological regulation, cellular and metabolic process, cell death, establishment of localization, and growth, thereby supporting their significant role in disease development (Jin et al., 2013; Schanzenbach et al., 2017; Li et al., 2018).
The significant number of targets by each miRNA depicts evidence of their evolutionary conservation with the implication that they play an essential role in gene regulation during bovine trypanosomosis. Yang et al. (2012) and Zheng et al. (2014) have reported the significant role of miRNAs in fine-tuning innate immune gene expression in Holstein cows during heat stress. Remarkably, bta-mir-2888-1 and bta-mir-2888-2 together targets ICAM-1, ITGAM, LBP, TLR-2, and TNF-α to respond to dihydrosphingosine-1-P pathway and 2-aminobenzenesulfonate metabolic process, which are important regulators of physiological biosynthesis, cell survival, proliferation, and cell-cell interactions (Bu et al., 2008). Previous studies have shown that ICAM-1, TLR-4 and ITGAM were implicated in early inflammation and signaling in critical pathways regulating bovine innate immunity (Boulougouris et al., 2019). As seen from our study therefore, bta-mir-2888-1 and bta-mir-2888 would be significant biomarkers that regulate the expression of these genes during disease condition and could serve as potential therapeutic targets for bovine trypanosomosis.
Our phylogenetic analysis revealed bta-mir-193a to be highly conserved across different species ranging from human to gorilla, monkey, mouse, rat, rabbit, chicken, pig, dog, python, and zebra. This shows that bta-mir-193a has a long history of evolution among others and would be a molecular marker of choice in bovine disease studies, and as such require additional in vitro validation studies. Reports from other studies have shown that bta-miR-193a regulates the expression of many proinflammatory cytokines, promote apoptosis and inhibition of bacteria in mice, goat and cattle (Dilda et al., 2011; Rosenberger et al., 2012; Lawless et al., 2013; Li et al., 2018). The fact that our results show bta-mir-193a targeting CD14, ITGAM, TLR-2, TLR-4, and TNF-α strongly supports its roles in transcriptional activation of proinflammatory response and innate immune gene expression during bovine trypanosomosis. This provides compelling evidence that this miRNA could serve as a significant biomarker in disease diagnosis and treatment. Many in vitro studies have elucidated the significant roles of miRNAs in regulating bovine immune responses during disease conditions (Dilda et al., 2011; Lawless et al., 2013; Wang et al., 2017; Ammah et al., 2018). The fact that TLR-2 and TLR-4 are part of bta-miR-193a target suggests its involvement in Toll-like receptor signaling during host response to trypanosomosis infection.
Conclusion
Our study provides computational evidence that certain miRNAs are conserved within the bovine genome and may be associated with regulation of immune response during bovine trypanosomosis. We propose that miRNAs targeting more genes will play greater roles in immune regulation and as such exert significant impact on disease phenotype. We also show that the CDS region has abundant miRNA binding site for all genes in this study demonstrating the importance of this region and requiring further elucidation in bovine disease studies. Additionally, we demonstrate that miRNA that co-target 3′UTR and CDS are important and capable of impacting target gene expressions in a complementary manner. Finally, our study identified some microRNAs including bta-mir-193a, bta-mir-2460, bta-mir-2349, and bta-mir-2888 amongst others, which might be important regulatory markers for diagnosis of bovine trypanosomosis as well as treatment and drug targets.
Data Availability
All datasets generated for this study are included in the manuscript and/or the Supplementary Files.
Author Contributions
OM and MH performed the experiment. OM, MH, AH, and BT analyzed the data. OM, AH, and BT wrote the manuscript. All authors reviewed and approved the final manuscript.
Funding
We acknowledge the intramural funding through a Laboratory and Faculty Development Award, College of Health Sciences and Technology, Rochester Institute of Technology (BT). OM is supported through the American Association of Immunologists Careers in Immunology Fellowship Program. AH acknowledge the College of Science and The Thomas H. Gosnell School of Life Sciences for ongoing support. The funders had no role in study design, data collection and analysis, decision to publish, or preparation of the manuscript.
Conflict of Interest Statement
The authors declare that the research was conducted in the absence of any commercial or financial relationships that could be construed as a potential conflict of interest.
Supplementary Material
The Supplementary Material for this article can be found online at: https://www.frontiersin.org/articles/10.3389/fmicb.2019.02010/full#supplementary-material
Footnotes
- ^ https://www.genomatix.de/solutions/genomatix-software-suite.html
- ^ http://www.ensembl.org/Tools/Blast/GenomicSeq
- ^ http://mirwalk.umm.uni-heidelberg.de
- ^ http://www.targetscan.org/vert_72/
- ^ http://mirdb.org/
- ^ http://www.mirbase.org/index.shtml
- ^ http://bioinfogp.cnb.csic.es/tools/venny/
- ^ http://bioinformatics.psb.ugent.be/software/details/Venn-Diagrams
- ^ https://www.mirnet.ca/miRNet/faces/upload/GeneUploadView.xhtml
- ^ http://mirwalk.umm.uni-heidelberg.de/search_genes/
- ^ http://www.mirbase.org/index.shtml
- ^ http://www.ensembl.org/Tools/Blast/GenomicSeq
- ^ http://geneontology.org/
- ^ http://diana.imis.athena-innovation.gr/DianaTools/index.php
References
Aalaei-Andabili, S. H., and Rezaei, N. (2016). MicroRNAs (MiRs) precisely regulate immune system development and function in immunosenescence process. Int. Rev. Immunol. 35, 57–66. doi: 10.3109/08830185.2015.1077828
Abdi, R. D., Agga, G. E., Aregawi, W. G., Bekana, M., Leeuwen, T. V., Delespaux, V., et al. (2017). A systematic review and metanalysis of trypanosome prevalence in tsetse flies. BMC Vet. Res. 13:100. doi: 10.1186/s12917-017-1012-9
Agarwal, V., Bell, G. W., Nam, J. W., and Bartel, D. P. (2015). Predicting effective microRNA target sites in mammalian mRNAs. eLife 4:e05005. doi: 10.7554/eLife.05005
Ammah, A. A., Do, D. N., Bissonnette, N., Gévry, N., and Ibeagha-Awemu, E. M. (2018). Co-expression network analysis identifies miRNA–mRNA networks potentially regulating milk traits and blood metabolites. Int. J. Mol. Sci. 19:2500. doi: 10.3390/ijms19092500
Awuah, D., Alobaid, M., Latif, A., Salazar, F., Emes, R. D., and Ghaemmaghami, A. M. (2019). The cross-talk between miR-511-3p and C-Type lectin receptors on dendritic cells affects dendritic cell function. J. Immunol. 203, 148–157. doi: 10.4049/jimmunol.1801108
Bartel, D. P. (2009). MicroRNAs: target recognition and regulatory functions. Cell 136, 215–233. doi: 10.1016/j.cell.2009.01.002
Bazzini, A. A., Lee, M. T., and Giraldez, A. J. (2012). Ribosome profiling shows that miR-430 reduces translation before causing mRNA decay in zebrafish. Science 336, 233–237. doi: 10.1126/science.1215704
Berriz, G. F., King, O. D., Bryant, B., Sander, C., and Roth, F. P. (2003). Characterizing gene sets with FuncAssociate. Bioinformatics 19, 2502–2504. doi: 10.1093/bioinformatics/btg363
Boulougouris, X., Rogiers, C., Van Poucke, M., De Spiegeleer, B., Peelman, L. J., Duchateau, L., et al. (2019). Distinct neutrophil C5a receptor inflammatory events in cows initiated by chemoattractant C5a and lipopolysaccharide around parturition and in mid lactation. J. Dairy Sci. 102, 1457–1472. doi: 10.3168/jds.2018-15356
Bronevetsky, Y., Villarino, A. V., Eisley, C. J., Barbeau, R., Barczak, A. J., Heinz, G. A., et al. (2013). T cell activation induces proteasomal degradation of argonaute and rapid remodeling of the microRNA repertoire. J. Exp. Med. 210, 417–432. doi: 10.1084/jem.20111717
Brummer, A., and Hausser, J. (2014). MicroRNA binding sites in the coding region of mRNAs: extending the repertoire of post-transcriptional gene regulation. Bioessays 36, 617–626. doi: 10.1002/bies.201300104
Bu, S., Kapanadze, B., Hsu, T., and Trojanowska, M. (2008). Opposite effects of Dihydrosphingosine 1-Phosphate and Sphingosine 1-Phosphate on transforming growth factor-β/Smad signaling are mediated through the PTEN/PPM1A-dependent pathway. J. Biol. Chem. 283, 19593–19602. doi: 10.1074/jbc.M802417200
Bushati, N., and Cohen, S. M. (2007). microRNA functions. Annu. Rev. Cell Dev. Biol. 23, 175–205. doi: 10.1146/annurev.cellbio.23.090506.123406
Buza, T., Arick, M., Wang, H., and Peterson, D. G. (2014). Computational prediction of disease microRNAs in domestic animals. BMC Res. Notes 7:403. doi: 10.1186/1756-0500-7-403
Chi, S. W., Zang, J. B., Mele, A., and Darnell, R. B. (2009). Argonaute HITS-CLIP decodes microRNA-mRNA interaction maps. Nature 460, 479–486. doi: 10.1038/nature08170
Coutinho, L. L., Matukumalli, L. K., Sonstegard, T. S., Van Tassell, C. P., Gasbarre, L. C., Capuco, A. V., et al. (2007). Discovery and profiling of bovine microRNAs from immune-related and embryonic tissues. Physiol. Genomics. 29, 35–43. doi: 10.1152/physiolgenomics.00081.2006
da Silveira, W. A., Renaud, L., Simpson, J., Glen, W. B., Hazard, E. S., Chung, D., et al. (2018). miRmapper: a tool for interpretation of miRNA–mRNA interaction networks. Genes 9:E458. doi: 10.3390/genes9090458
Das, K., Garnica, O., and Dhandayuthapani, S. (2016). Modulation of host miRNAs by intracellular bacterial pathogens. Front. Cell. Infect. Microbiol. 6:79. doi: 10.3389/fcimb.2016.00079
Dilda, F., Gioia, G., Pisani, L., Restelli, L., Lecchi, C., Albonico, F., et al. (2011). Escherichia coli lipopolysaccharides and Staphylococcus aureus enterotoxin B differentially modulate inflammatory microRNAs in bovine monocytes. Vet. J. 192, 514–516. doi: 10.1016/j.tvjl.2011.08.018
Erhard, F., Haas, J., Lieber, D., Malterer, G., Jaskiewicz, L., Zavolan, M., et al. (2014). Widespread context dependency of microRNA-mediated regulation. Genome Res. 24, 906–919. doi: 10.1101/gr.166702.113
Fan, Y., and Xia, J. (2018). miRNet—functional analysis and visual exploration of miRNA–target interactions in a network context. Methods Mol. Biol. 1819, 215–233. doi: 10.1007/978-1-4939-8618-7_10
Fang, Z., and Rajewsky, N. (2011). The impact of miRNA target sites in coding sequences and in 3’UTRs. PLoS One 6:e18067. doi: 10.1371/journal.pone.0018067
Forman, J. J., Legesse-Miller, A., and Coller, H. A. (2008). A search for conserved sequences in coding regions reveals that the let-7 microRNA targets Dicer within its coding sequence. PNAS 105, 14879–14884. doi: 10.1073/pnas.0803230105
Frisch, M., Klocke, B., Haltmeier, M., and Frech, K. (2009). LitInspector: literature and signal transduction pathway mining in PubMed abstracts. Nucleic Acids Res. 37, W135–W140. doi: 10.1093/nar/gkp303
Gaidatzis, D., van Nimwegen, E., Hausser, J., and Zavolan, M. (2007). Inference of miRNA targets using evolutionary conservation and pathway analysis. BMC Bioinform. 8:69. doi: 10.1186/1471-2105-8-69
Govindaraju, A., Uzun, A., Robertson, L., Atli, M. O., Kaya, A., Topper, E., et al. (2012). Dynamics of microRNAs in bull spermatozoa. Reprod. Biol. Endocrinol. 10:82. doi: 10.1186/1477-7827-10-82
Hanif, Q., Farooq, M., Amin, I., Mansoor, S., Zhang, Y., and Khan, Q. M. (2018). In silico identification of conserved miRNAs and their selective target gene prediction in indicine (Bos indicus) cattle. PLoS One 13:e0206154. doi: 10.1371/journal.pone.0206154
Hausser, J., Syed, A. P., Bilen, B., and Zavolan, M. (2013). Analysis of CDS-located miRNA target sites suggests that they can effectively inhibit translation. Genome Res. 23, 604–615. doi: 10.1101/gr.139758.112
Hausser, J., and Zavolan, M. (2014). Identification and consequences of miRNA-target interactions–beyond repression of gene expression. Nat. Rev. Genet. 15, 599–612. doi: 10.1038/nrg3765
Huberdeau, Q., Zeitler, D. M., Hauptmann, J., Bruckmann, A., Fressigné, L., and Danner, J. (2017). Phosphorylation of argonaute proteins affects mRNA binding and is essential for microRNA-guided gene silencing in vivo. EMBO J. 36, 2088–2106. doi: 10.15252/embj.201696386
Hurst, L. D. (2006). Preliminary assessment of the impact of microRNA-mediated regulation on coding sequence evolution in mammals. J. Mol. Evol. 63, 174–182. doi: 10.1007/s00239-005-0273-2
Ji, K., Fan, R., Zhang, J., Yang, S., and Dong, C. (2018). Long non-coding RNA expression profile in Cdk5-knockdown mouse skin. Gene 672, 195–201. doi: 10.1016/j.gene.2018.05.120
Jin, H. Y., Oda, H., Lai, M., Skalsky, R. L., Bethel, K., Shepherd, J., et al. (2013). MicroRNA-17~92 plays a causative role in lymphomagenesis by coordinating multiple oncogenic pathways. EMBO J. 32, 2377–2391. doi: 10.1038/emboj.2013.178
Jin, W., Grant, J. R., Stothard, P., Moore, S. S., and Guan, L. L. (2009). Characterization of bovine miRNAs by sequencing and bioinformatics analysis. BMC Mol. Biol. 10:90. doi: 10.1186/1471-2199-10-90
Kangas, R., Tormakangas, T., Fey, V., Pursiheimo, J., Miinalainen, I., Alen, M., et al. (2017). Aging and serum exomiR content in women-effects of estrogenic hormone replacement therapy. Sci. Rep. 1:42702. doi: 10.1038/srep42702
Kim, S. J., Ka, S., Ha, J. W., Kim, J., Yoo, D., Kim, K., et al. (2017). Cattle genome-wide analysis reveals genetic signatures in trypanotolerant N’Dama. BMC Genomics 18:371. doi: 10.1186/s12864-017-3742-2
Kogelman, L. J. A., Pant, S. D., Fredholm, M., and Kadarmideen, H. N. (2014). Systems genetics of obesity in an F2 pig model by genome-wide association, genetic network and pathway analyses. Front. Genet. 5:214. doi: 10.3389/fgene.2014.00214
Kramer, N. J., Wang, W. L., Reyes, E. Y., Kumar, B., Chen, C. C., Ramakrishna, C., et al. (2015). Altered lymphopoiesis and immunodeficiency in miR-142 null mice. Blood 125, 3720–3730. doi: 10.1182/blood-2014-10-603951
Kumar, V., Kumar, V., Kumar, A., Donald, W. C., McGuire, C. T., and Mahato, R. I. (2018). Impact of miRNA-mRNA Profiling and their correlation on Medulloblastoma Tumorigenesis. Mol. Ther. Nucleic Acids 12, 490–503. doi: 10.1016/j.omtn.2018.06.004
Lawless, N., Foroushani, A. B., McCabe, M. S., O’Farrelly, C., and Lynn, D. J. (2013). Next generation sequencing reveals the expression of a unique miRNA profile in response to a Gram-positive bacterial infection. PLoS One 8:e57543. doi: 10.1371/journal.pone.0057543
Lawless, N., Vegh, P., O’Farrelly, C., and Lynn, D. J. (2014). The role of microRNAs in bovine infection and immunity. Front. Immunol. 5:611. doi: 10.3389/fimmu.2014.00611
Li, L., Song, Y., Shi, X., Liu, J., Xiong, S., Chen, W., et al. (2018). The landscape of miRNA editing in animals and its impact on miRNA biogenesis and targeting. Genome Res. 28, 132–143. doi: 10.1101/gr.224386.117
Li, Y., Carrillo, J. A., Ding, Y., He, Y., Zhao, C., Liu, J., et al. (2019). DNA methylation, microRNA expression profiles and their relationships with transcriptome in grass-fed and grain-fed Angus Cattle rumen tissue. bioRxiv 581421. doi: 10.1101/581421
Li, Y., Qiu, C., Tu, J., Geng, B., Yang, J., Jiang, T., et al. (2014). HMDD v2.0: a database for experimentally supported human microRNA and disease associations. Nucleic Acids Res. 42, D1070–D1074. doi: 10.1093/nar/gkt1023
Liu, W., and Wang, X. (2019). Prediction of functional microRNA targets by integrative modeling of microRNA binding and target expression data. Genome Biol. 20:18. doi: 10.1186/s13059-019-1629-z
Mahjoub, N., Dhorne-Pollet, S., Fuchs, W., Endale Ahanda, M. L., Lange, E., Klupp, B., et al. (2015). A 2.5-kilobase deletion containing a cluster of nine microRNAs in the latency-associatedtranscript locus of the pseudorabies virus affects the host response of porcine trigeminal ganglia during established latency. J. Virol. 89, 428–442. doi: 10.1128/JVI.02181-14
Maier, H., Döhr, S., Grote, K., O’Keeffe, S., Werner, T., Hrabé de Angelis, M., et al. (2005). LitMiner and WikiGene: identifying problem-related key players of gene regulation using publication abstracts. Nucleic Acids Res. 33, W779–W782. doi: 10.1093/nar/gki417
Mehta, A., and Baltimore, D. (2016). MicroRNAs as regulatory elements in immune system logic. Nat. Rev. Immunol. 16, 279–294. doi: 10.1038/nri.2016.40
Meister, G. (2013). Argonaute proteins: functional insights and emerging roles. Nat. Rev. Genet. 14, 447–459. doi: 10.1038/nrg3462
Morenikeji, O. B., and Thomas, B. N. (2019a). Disease tolerance and immune response in bovine trypanosomiasis is mediated by CD14 promoter gene. J. Immunol. 73(1 Suppl.), 17.
Morenikeji, O. B., and Thomas, B. N. (2019b). In silico analyses of CD14 molecule reveal significant evolutionary diversity, potentially associated with speciation and variable immune response in mammals. Peer J. 7:e7325. doi: 10.7717/peerj.7325
Newman, M. A., and Hammond, S. M. (2010). Emerging paradigms of regulated microRNA processing. Genes Dev. 24, 1086–1092. doi: 10.1101/gad.1919710
Noyes, H., Brass, A., Obara, I., Anderson, S., Archibald, A. L., Bradley, D. G., et al. (2011). Genetic and expression analysis of cattle identifies candidate genes in pathways responding to Trypanosoma congolense infection. Proc. Natl. Acad. Sci. U.S.A. 108, 9304–9309. doi: 10.1073/pnas.1013486108
O’Connell, R. M., Kahn, D., Gibson, W. S., Round, J. L., Scholz, R. L., Chaudhuri, A. A., et al. (2010). MicroRNA-155 promotes autoimmune inflammation by enhancing inflammatory T cell development. Immunity 33, 607–619. doi: 10.1016/j.immuni.2010.09.009
Odeniran, P. O., and Ademola, I. O. (2018). A meta-analysis of the prevalence of African animal trypanosomiasis in Nigeria from 1960 to 2017. Paras. Vect. 11:280. doi: 10.1186/s13071-018-2801-0
Ojurongbe, O., Funwei, R. I., Snyder, T. J., Aziz, N., Li, Y., Falade, C. O., et al. (2019). Genetic diversity of CD14 promoter gene polymorphism (rs2569190) is associated with regulation of parasitemia but not susceptibility to Plasmodium falciparum infection. Infect. Dis. 10, 117863361772678. doi: 10.1177/1178633617726781
Ott, C. E., Grünhagen, J., Jäger, M., Horbelt, D., Schwill, S., Kallenbach, K., et al. (2011). MicroRNAs differentially expressed in postnatal aortic development downregulate elastin via 3’ UTR and coding-sequence binding sites. PLoS One 6:e16250. doi: 10.1371/journal.pone.0016250
Pande, H. O., Tesfaye, D., Hoelker, M., Gebremedhn, S., Held, E., Neuhoff, C., et al. (2018). MicroRNA-424/503 cluster members regulate bovine granulosa cell proliferation and cell cycle progression by targeting SMAD7 gene through activin signaling pathway. J Ovarian Res. 11:34. doi: 10.1186/s13048-018-0410-3
Paraskevopoulou, M. D., Georgakilas, G., Kostoulas, N., Reczko, M., Maragkakis, M., Dalamagas, T. M., et al. (2013). DIANA-LncBase: experimentally verified and computationally predicted microRNA targets on long non-coding RNAs. Nucleic Acids Res. 41, D239–D245. doi: 10.1093/nar/gks1246
Pichulik, T., Khatamzas, E., Liu, X., Brain, O., Delmiro Garcia, M., Leslie, A., et al. (2016). Pattern recognition receptor mediated downregulation of microRNA-650 fine-tunes MxA expression in dendritic cells infected with influenza A virus. Eur. J. Immunol. 46, 167–177. doi: 10.1002/eji.201444970
Rosenberger, C. M., Podyminogin, R. L., Navarro, G., Zhao, G. W., Askovich, P. S., Weiss, M. J., et al. (2012). miR-451 regulates dendritic cell cytokine responses to influenza infection. J. Immunol. 189, 5965–5975. doi: 10.4049/jimmunol.1201437
Sandhu, K. S., Hagely, K., and Neff, M. M. (2012). Genetic interactions between brassinosteroid-inactivating P450s and photomorphogenic photoreceptors in Arabidopsis thaliana. G3 2, 1585–1593. doi: 10.1534/g3.112.004580
Schanzenbach, C. I., Kirchner, B., Ulbrich, S. E., and Pfaffl, M. W. (2017). Can milk cell or skim milk miRNAs be used as biomarkers for early pregnancy detection in cattle? PLoS One 12:e0172220. doi: 10.1371/journal.pone.0172220
Scheel, T. K. H., Moore, M. J., Luna, J. M., Nishiuchi, E., Fak, J., Darnell, R. B., et al. (2017). Global mapping of miRNA-target interactions in cattle (Bos taurus). Sci. Rep. 7:8190. doi: 10.1038/s41598-017-07880-8
Scherf, M., Epple, A., and Werner, T. (2005). The next generation of literature analysis: integration of genomic analysis into text mining. Brief. Bioinform. 6, 287–297. doi: 10.1093/bib/6.3.287
Schnall-Levin, M., Rissland, O. S., Johnston, W., Perrimon, N., Bartel, D. P., and Berger, B. (2011). Unusually effective microRNA targeting within repeat-rich coding regions of mammalian mRNAs. Genome Res. 21, 1395–1403. doi: 10.1101/gr.121210.111
Seabury, C. M., Seabury, P. M., Decker, J. E., Schnabel, R. D., Taylor, J. F., and Womack, J. E. (2010). Diversity and evolution of 11 innate immune genes in Bos taurus taurus and Bos taurus indicus cattle. Proc. Natl. Acad. Sci. U.S.A. 107, 151–156. doi: 10.1073/pnas.0913006107
Smetko, A., Soudre, A., Silbermayr, K., Müller, S., Brem, G., Hanotte, O., et al. (2015). Trypanosomosis: potential driver of selection in African cattle. Front. Genet. 6:137. doi: 10.3389/fgene.2015.00137
Wang, H., Zhong, J., Chai, Z., Zhu, J., and Xin, J. (2018). Comparative expression profile of micro RNA s and pi RNA s in three ruminant species testes using next-generation sequencing. Reprod. Domest. Anim. 53, 963–970. doi: 10.1111/rda.13195
Wang, L. L., Liu, Y., Chung, J. J., Wang, T., Gaffey, A. C., Lu, M., et al. (2017). Sustained miRNA delivery from an injectable hydrogel promotes cardiomyocyte proliferation and functional regeneration after ischaemic injury. Nat. Biomed. Eng. 1, 983–992. doi: 10.1038/s41551-017-0157-y
Ward, J. R., Heath, P. R., Catto, J. W., Whyte, M. K., Milo, M., and Renshaw, S. A. (2011). Regulation of neutrophil senescence by microRNAs. PLoS One 6:e15810. doi: 10.1371/journal.pone.0015810
Xie, B., Ding, Q., Han, H., and Wu, D. (2013). miRCancer: a microRNA-cancer association database constructed by text mining on literature. Bioinformatics 29, 638–644. doi: 10.1093/bioinformatics/btt014
Xu, N., Papagiannakopoulos, T., Pan, G., Thomson, J. A., and Kosik, K. S. (2009). MicroRNA-145 regulates OCT4, SOX2, and KLF4 and represses pluripotency in human embryonic stem cells. Cell 137, 647–658. doi: 10.1016/j.cell.2009.02.038
Yang, G., Yang, L., Zhao, Z., Wang, J., and Zhang, X. (2012). Signature miRNAs involved in the innate immunity of invertebrates. PLoS One 7:e39015. doi: 10.1371/journal.pone.0039015
Yang, P., Zhang, M., Liu, X., and Pu, H. (2017). MicroRNA-421 promotes the proliferation and metastasis of gastric cancer cells by targeting claudin-11. Exp. Ther. Med. 14, 2625–2632. doi: 10.3892/etm.2017.4798
Keywords: cattle, miRNA-target, expression, trypanosomosis, evolution, immune response
Citation: Morenikeji OB, Hawkes ME, Hudson AO and Thomas BN (2019) Computational Network Analysis Identifies Evolutionarily Conserved miRNA Gene Interactions Potentially Regulating Immune Response in Bovine Trypanosomosis. Front. Microbiol. 10:2010. doi: 10.3389/fmicb.2019.02010
Received: 28 June 2019; Accepted: 16 August 2019;
Published: 28 August 2019.
Edited by:
Jude Ezeh Uzonna, University of Manitoba, CanadaReviewed by:
Jata Shankar, Jaypee University of Information Technology, IndiaMaryam Dadar, Razi Vaccine and Serum Research Institute, Iran
Copyright © 2019 Morenikeji, Hawkes, Hudson and Thomas. This is an open-access article distributed under the terms of the Creative Commons Attribution License (CC BY). The use, distribution or reproduction in other forums is permitted, provided the original author(s) and the copyright owner(s) are credited and that the original publication in this journal is cited, in accordance with accepted academic practice. No use, distribution or reproduction is permitted which does not comply with these terms.
*Correspondence: Bolaji N. Thomas, Ym50c2JpQHJpdC5lZHU=