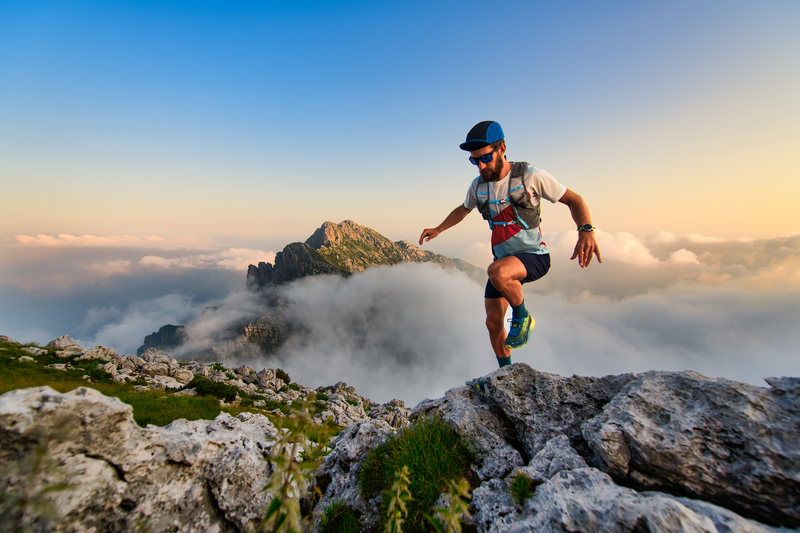
94% of researchers rate our articles as excellent or good
Learn more about the work of our research integrity team to safeguard the quality of each article we publish.
Find out more
ORIGINAL RESEARCH article
Front. Microbiol. , 02 August 2019
Sec. Aquatic Microbiology
Volume 10 - 2019 | https://doi.org/10.3389/fmicb.2019.01790
Diatoms are unicellular algae with a fundamental role in global biogeochemical cycles as major primary producers at the base of aquatic food webs. In recent years, chemical communication between diatoms and associated bacteria has emerged as a key factor in diatom ecology, spurred by conceptual and technological advancements to study the mechanisms underlying these interactions. Here, we use a combination of physiological, transcriptomic, and metabolomic approaches to study the influence of naturally co-existing bacteria, Maribacter sp. and Roseovarius sp., on the sexual reproduction of the biofilm inhabiting marine pennate diatom Seminavis robusta. While Maribacter sp. severely reduces the reproductive success of S. robusta cultures, Roseovarius sp. slightly enhances it. Contrary to our expectation, we demonstrate that the effect of the bacterial exudates is not caused by altered cell-cycle regulation prior to the switch to meiosis. Instead, Maribacter sp. exudates cause a reduced production of diproline, the sexual attraction pheromone of S. robusta. Transcriptomic analyses show that this is likely an indirect consequence of altered intracellular metabolic fluxes in the diatom, especially those related to amino acid biosynthesis, oxidative stress response, and biosynthesis of defense molecules. This study provides the first insights into the influence of bacteria on diatom sexual reproduction and adds a new dimension to the complexity of a still understudied phenomenon in natural diatom populations.
Diatoms are among the most productive and ecologically relevant unicellular algae on Earth. Their high genetic diversity and adaptive potential allowed them to diversify into hundreds of genera and over 100,000 species, occurring in freshwater, marine, and soil habitats globally (Malviya et al., 2016). Moreover, they are a fundamental link in global biogeochemical cycles, contributing up to 20% of the total primary production on Earth (Field et al., 1998) and being key players in oceanic silica cycling. While planktonic diatoms have been extensively studied, benthic diatoms often also dominate primary production in biofilms in the photic zone and play an important role in regulating nutrient fluxes in and out of sediments (Smith and Underwood, 1998).
In recent years, it has become increasingly clear that diatoms engage in multiple interactions with bacteria (Amin et al., 2012; Seymour et al., 2017). Many of these are confined to the so-called phycosphere (Bell and Mitchell, 1972), a zone surrounding the microalgal cell where diffusion controls transport of exuded chemicals (Seymour et al., 2017). While some bacteria promote the growth of diatoms or show mutualistic behavior (Seymour et al., 2017), for example by releasing nutrients (Helliwell et al., 2014) or growth hormones (Amin et al., 2015), other microbes suppress diatom growth (Meyer et al., 2017) by the production of algicidal compounds (Wang and Seyedsayamdost, 2017) or growth inhibiting factors (van Tol et al., 2017; Stock et al., 2019). Therefore, diatom–bacteria interactions control nutrient cycling at the base of the foodweb and act as regulators of algal blooms (Riemann et al., 2000; Seymour et al., 2017). Because of this, studying these interactions is fundamental for understanding the ecological importance of diatoms in biogeochemical cycles, as well as their evolutionary history (Azam and Malfatti, 2007; Ramanan et al., 2016). Despite the relevance of diatom–bacteria relationships, studies to unravel the underlying molecular mechanisms remain scarce (Durham et al., 2017).
Recently, it was shown that some bacteria are able to interfere with sexual reproduction of the benthic diatom Seminavis robusta (Cirri et al., 2018), a motile pennate diatom inhabiting coastal biofilm communities. S. robusta has a heterothallic mating system in which, once the cell size drops below the sexual size threshold (SST), both mating types (MT+ and MT–) release different sex inducing pheromones (SIP+ and SIP–, respectively). These SIPs induce a temporary arrest in the cell cycle of the opposite mating type in G1 phase to synchronize the switch to gametogenesis (Frenkel et al., 2014a; Moeys et al., 2016). Moreover, SIP+ induces the production of an attraction pheromone by MT– cells: a diketopiperazine consisting of two proline molecules called diproline (Gillard et al., 2013). This pheromone then attracts the MT+ cells, resulting in physical pairing of compatible cells and subsequent gametogenesis. Although diproline is stable in artificial seawater, in non-axenic cultures its concentration oscillates on a daily basis (Gillard et al., 2013; Frenkel et al., 2014b). It was recently shown that two bacteria associated with S. robusta (Maribacter sp. and Roseovarius sp.) are able to modulate extracellular diproline concentrations and that the exudates of both bacteria have different effects on the reproductive success of S. robusta (Cirri et al., 2018). Exudates of Maribacter sp. negatively affect the sexual reproduction of S. robusta, while Roseovarius sp. exudates slightly enhance it. Both bacterial isolates are able to degrade diproline, but only when severely nutrient-deprived. Experimental results suggest that bacterial metabolites interfere in a direct manner with the physiology of diatoms and attraction pheromone production, thereby influencing the reproductive success of S. robusta.
Here we combined physiological, metabolomic, and transcriptomic approaches to gain mechanistic insights into the effect of Roseovarius sp. and Maribacter sp. exudates on S. robusta and its sexual behavior. We analyzed the effect of both bacteria on the induced cell cycle arrest caused by SIP+, gene expression, and metabolic profiles in MT– cells. We show that neither of the bacterial exudates affect cell cycle arrest but they both trigger an oxidative stress response in the diatom. Moreover, we show that Maribacter sp. affects the metabolism of several amino- and fatty acids and thereby indirectly influences diproline production. Roseovarius sp. enhances the expression of enzymes that synthetize precursors of the attraction pheromone.
Seminavis robusta strains 85A (MT+) (BCCM: DCG0105) and 84A (MT–) (BCCM: DCG0104) were obtained from the diatom culture collection of the Belgian Coordinated Collection of Micro-organisms (BCCM/DCG1). Cultures of both mating types were grown separately under a 12 h:12 h dark/light regime (cool white light at an intensity of 50 μmol m–2 s–1) at 18°C in Guillard’s F/2 medium (Guillard, 1975). This medium was prepared by autoclaving 34.5 g/L Tropic Marin® BIO-ACTIF sea salt (Tropic Marin®, Wartenberg, Germany) and supplementing it with 20 mL/L Guillard’s (F/2) Marine Water Enrichment Solution (Sigma–Aldrich). Axenic cultures were prepared following the protocol of Cirri et al. (2018).
Stock cultures of Roseovarius sp. and Maribacter sp. isolated from S. robusta (for the method, see Cirri et al., 2018) were grown in DifcoTM Marine Broth medium at room temperature for 3 days before the experiment. Then 25 mL of the bacterial culture was transferred to a 50 mL Falcon tube, centrifuged for 3 min at 6,000 × g, washed three times with minimal medium (F/2 medium with 5 g/L glucose, 5 mL/L glycerol, and 1.5 g/L NH4NO3), and transferred to 500 mL of minimal medium. The cultures were grown for 10 days at room temperature until they reached the late exponential phase (OD600 = 0.1 measured with a Shimadzu® UV-1601 Spectrophotometer) before being sterile-filtered to harvest sterile bacterial exudates.
Seminavis robusta strain 85A (MT+) was grown at 18°C in CELLSTAR® Standard Cell Culture Flasks with a 175 cm2 surface area, filled with 200 mL Guillard’s F/2 medium under 12 h:12 h dark:light regime (50 μmol m–2 s–1 photons of cool white light). As a proxy for the biomass in the flasks, we measured the minimum fluorescence value (F0) after 15 min of dark-adaptation. Pulse-amplitude-modulation (PAM) fluorimetry measurements were performed using a MAXI Imaging PAM Fluorimeter, M-series (Walz, Effeltrich, Germany), equipped with an IMAG-K4 camera and mounted with an IMAG-MAX/F filter. F0 was measured using the following software settings: intensity 7, gain 3, and damping 2. When the culture reached an F0-value of ≈0.35, the medium was harvested, sterile-filtered using GF/F filters (ø 47 mm) on NalgeneTM reusable bottle top filters units (Thermo Fisher Scientific, Bremen, Germany) connected to sterile 250 mL Duran® bottles (Schott, Jena, Germany), aliquoted in 50 mL Falcon tubes, and stored at −20°C until usage. In total, 12 culture flasks (2,4-L SIP+-containing medium) were harvested.
Seminavis robusta strain 84A (MT–) was grown at 18°C in CELLSTAR§ Standard Cell Culture Flasks with a 175 cm2 surface area, filled with 200 mL Guillard’s F/2 medium under 12 h:12 h dark:light regime (50 μmol m–2 s–1 photons of cool white light). Once the cultures reached an F0-value of ≈0.30, the culture medium was renewed and the flasks were placed in complete darkness at 18°C for 24 h to synchronize the cell cycle in G1-phase (Moeys et al., 2016). After 21 h of darkness, sexuality was induced in MT– cultures by removing 20 mL medium and replacing it with 20 mL SIP+-containing medium to end up with a final dilution of 1:10 SIP+. Also, after 21 h of darkness, bacterial exudates were added to the flasks, diluted to a volume equivalent to the volume of a full bacterial culture at OD600 = 0.05, the cell density at which the effects on sexual reproduction of these bacteria were shown (Cirri et al., 2018). Addition of SIP+ and/or bacterial exudates was done in a dark room to prevent progression through the cell cycle. Control cultures, where no SIP+ or bacterial exudates were added, were also moved to the dark room and back to avoid any differences in light treatment between control and treatment cultures. After addition of SIP+ and/or bacterial exudates, the cultures were placed in complete darkness at 18°C for another 3 h before the light was switched on (50 μmol m–2 s–1 photons).
All six treatments (control, SIP+-treated, Roseovarius sp.-treated, Maribacter sp.-treated, SIP+ + Roseovarius sp.-treated, and SIP+ + Maribacter sp.-treated) were cultured and harvested in five replicates.
After 10 h of light, 150 mL of the medium was poured over a GF/C filter (ø 47 mm) at 650 mbar on NalgeneTM reusable bottle top filters units (Thermo Fisher Scientific, Bremen, Germany) connected to sterile 250 mL Duran® bottles (Schott, Jena, Germany) without disturbing the cells. The filtrate was used for exometabolome extraction. The cells were then scraped from the surface of the culture flasks using a cell scraper and homogenized in the remaining medium (50 mL) by shaking. Ten milliliters of the cell suspension was used for flow cytometry analysis, while the remaining 40 mL of the suspension was used for RNA extraction.
Of each harvested culture, 10 mL was isolated in a 15 mL falcon tube. The samples were centrifuged for 5 min at 2,000 rcf. The supernatant was discarded and the cells were fixed by resuspending the pellet in 10 mL ice cold 75% ethanol. Samples were stored in the dark at 4°C until analysis.
Fixed cultures were centrifuged for 5 min at 3,000 rpm, after which the supernatant was replaced with 2 mL ice cold 75% ethanol. One milliliter of each sample was transferred to a 1.5-mL tube and washed three times with phosphate-buffered saline (PBS) buffer to remove all remaining ethanol. The fixed cells were treated with 1 μg/mL RNAse A for 20 min at 37°C and afterward stained with SYBR green (1× concentration, Life Technologies) in the dark for 20 min.
Samples were filtered through a cell strainer with pore size of 70 μm before feeding into the flow cytometer. Flow cytometry was carried out on a Bio-Rad S3e Cell Sorter (Bio-Rad Laboratories, Inc., Hercules, CA, United States), collecting 10,000 measurements for each sample and gating was carried out in the FSC and SSC channel to remove debris signals. An unstained control sample was run first to localize the cell population. After running the samples, G1 and G2 peaks were visually selected using ProSort 1.5 (Bio-Rad Laboratories, Inc., Hercules, CA, United States).
Data analysis was carried out in R (v3.4.32, R Development Core Team., 2008). Since the response variable is binary [i.e., the response of a cell is either “failure” (G1) or “success” (G2-M)] and we are interested in changes in the proportion of G2-M cells in the population, we adopted a generalized linear model (GLM) with binomial distribution and logit link using the R glm function to assess significance for the effect of SIP and bacterial exudates. Post hoc tests comparing all combinations of treatments were carried out using the glht function from the Multcomp package (Hothorn et al., 2008).
The cells for RNA extraction (40 mL suspension, see above) were harvested by filtration over a Versapor filter (3 μm pore size, 25 mm diameter, PALL). Immediately after filtration, the filters were put in Eppendorf Tubes, flash-frozen in liquid nitrogen, and stored at −80°C until RNA extraction.
RNA was extracted from all samples (six treatments, five replicates each) using the RNeasy® Plant Mini Kit (Qiagen). First, 1 mL RLT buffer and 10 μl β-mercaptoethanol were added to the Eppendorf tube containing the frozen filter. The cells were removed from the filter by pipetting up and down and using the pipet tip to scrape the filter. The filter was removed and silicon carbide beads (1 mm, BioSpec) were added to the Eppendorf Tube. The cells were lysed by silicon carbide beads beating on a beating mill (Retsch, 3 × 1 min at frequency 20 Hz, with 30 s on ice in between each run). The lysate was then transferred to a QIAshredder spin column (RNeasy§ kit) and the manufacturer’s instructions were followed from there. An on-column DNase treatment was performed using the RNase-free DNase set (Qiagen) according to the manufacturer’s instructions.
RNA quality was evaluated by spectrophotometry (NanodropTM) and Bioanalyzer (Agilent Technologies). For each treatment, the three replicates with the highest quality (high 260/230- and 280/260 ratio’s and high RIN-value) were selected for library preparation and sequencing.
The 18 sequencing libraries were prepared using Illumina§ TruSeq Stranded mRNA kit. The libraries were sequenced (2 × 75 bp) in one Illumina® NextSeq 500 H150 run. Library preparation and sequencing were performed by VIB Nucleomics Core (VIB, Leuven).
Paired-end reads were quality-trimmed using FastQ Quality Filter from the FastX Toolkit v. 0.0.133 using the following settings: −q 28, −p 30. Using the Salmon software tool in quasi-mapping mode (Patro et al., 2017), the quality-trimmed reads were mapped to an annotated genes model assembly of S. robusta. To generate the annotated assembly, Illumina paired-end reads and PacBio long reads were combined in a hybrid assembly approach and gene models were annotated using expression data as training for the BRAKER1 (Hoff et al., 2016) pipeline. Next, functional annotations for the S. robusta gene models were determined using three different strategies: (i) InterProScan v5.3 (Jones et al., 2014) was run to scan protein sequences for matches against the InterPro protein signature databases; (ii) eggNOG-mapper (Huerta-Cepas et al., 2017) was executed with DIAMOND mapping mode, based on eggNOG 4.5 orthology data (Huerta-Cepas et al., 2016); and (iii) AnnoMine (Vandepoele et al., 2013) was employed to retrieve consensus gene functional annotation from protein similarity searches [using DIAMOND v0.9.9.110 maximum (Buchfink et al., 2015), e-value 10e−05 against Swiss-Prot (Bairoch and Apweiler, 2000) database]. Gene ontology terms were retrieved from the results of the eggNOG-mapper.
The transcript-level abundances generated with Salmon were imported into R (v.3.4.4) and aggregated to gene level counts using the tximport package (Soneson et al., 2015). Genes with low overall counts [counts-per-million (CPM) < 1 in at least three samples] were removed from the libraries because they have little power for detecting differential expression (DE). Differences in sequencing depth and RNA population were corrected using a weighted trimmed mean of the log expression ratios (TMM) normalization (Robinson and Oshlack, 2010). Preliminary differences between expression profiles of different samples were explored with multi-dimensional scaling (MDS) plots based on the top 500 genes, generated using the plotMDS function included in the EdgeR package.
Differential expression analysis was performed using the R package edgeR 3.20.9 (Robinson et al., 2010). Negative binomial GLMs were fitted to model read counts for each gene in each sample and a dispersion parameter which accounts for variability between biological replicates was calculated (Lun et al., 2016). For DE analysis, nine comparisons (contrasts) were defined (SIP vs. C, M vs. C, R vs. C, SIP + M vs. SIP, SIP + R vs. SIP, SIP + R vs. R, SIP + M vs. M, SIP + M vs. SIP + R, see Figure 1 for experimental setup). A gene was considered differentially expressed (DE) if the false discovery rate (FDR) adjusted p-values were below 0.01 and the absolute log2 fold change (LFC) was equal or greater than 1. To confirm GTP specificity of the putative guanylate cyclases (GC), a multiple sequence alignment was carried out in MEGA 7 (Kumar et al., 2016) to check the presence of guanylate cyclase-specific motifs (Winger et al., 2008).
Figure 1. Experimental setup. Axenic MT– S. robusta cells were grown in F/2 medium until an F0-value of ≈0.3. Their cell-cycle was dark-synchronized for 24 h in the darkness. After 21 h, half of the samples were treated with sexual inducing pheromone (SIP+) previously harvested from MT+. Bacterial exudates either from Maribacter sp. or Roseovarius sp. were also added. All samples were kept in the darkness for an additional 3 h before switching on the light. After 10 h of light, both cells and exudates from the diatom cultures were harvested. Cells were used for RNA extraction and cell cycle analysis, the medium was analyzed with an untargeted metabolomics approach and a targeted approach to detect diproline and oxylipins.
For genes DE in one specific contrast, Gene Ontology enrichment for single comparisons was determined using a gene set enrichment approach (GSEA) as implemented in CAMERA (Wu and Smyth, 2012), included in the R package limma v.3.34.9 (Ritchie et al., 2015). Redundant GO terms were removed using REVIGO4 (Supek et al., 2011) using a low similarity value of 0.5. GO enrichment of genes that were DE in multiple contrasts was performed using Fisher’s exact test and the “weight” algorithm for GO group scoring as implemented in TopGO (Alexa and Rahnenführer, 2009). Venn diagrams were generated with the R package VennDiagram v. 1.6.20 and with the web-based application Venny v. 2.1 (Oliveros, 2007–20155).
A total of 150 mL of filtered medium from each culture flask was transferred to sterile and cleaned 250 mL Erlenmeyer flasks, which were covered immediately with aluminum foil and cooled down to 4°C before solid phase extraction. Roseovarius sp. and Maribacter sp. exudates (n = 4, diluted to an equivalent OD600 = 0.05 with minimal medium) were prepared and stored in the same way. Before extraction, 15 nmol of caffeine dissolved in methanol [HPLC grade, Sigma–Aldrich, Chromasolv®Plus (≥99.9%)] was added to each sample as an internal standard. The medium was extracted on 60 mg Oasis® HLB-SPE cartridges (Waters, Eschborn, Germany), following the manufacturer’s instructions. Gentle vacuum was applied to the cartridges with a VisiprepTM SPE Vacuum Manifold (Sigma–Aldrich) to have a flow-through of ca. 1 drop per second. The cartridges were eluted three times with 1 mL of methanol. The 3 mL of eluate was stored in 4 mL vial glass at −80°C until further analysis. Medium blanks (n = 3) were prepare in the same way by extracting sterile F/2 medium. 1.5 mL of the eluate from each sample was transferred to a clean vial, evaporated under a stream of nitrogen, and dissolved in 50 μl of methanol. Two quality control (QC) samples were prepared by pooling 5 μl from each sample in one clean vial.
After randomizing the measuring order list of the samples and including QC every 7 samples, 5 μl of each sample were analyzed by UHPLC Dionex UltiMate® 3000 (Thermo Fisher Scientific, Dreieich, Germany), coupled to an ESI-Orbitrap MS Q-Exactive Plus (Thermo Fisher Scientific, Dreieich, Germany).
Liquid chromatography was performed on an Accucore® C18 column (2.1 × 100mm, 2.6 μm particle size; Thermo Scientific, Dreieich, Germany). The composition of the mobile phase was set to 100% A (0.1% HCOOH and 2% ACN in H2O) for 0.2 min and ramped to 100% B (0.1% HCOOH in ACN) in a linear gradient within 9 min. The solvent composition was held at 100% B for 4 min, returned to 100% A in 0.1 min, and held at 100% A for 0.9 min. The flow rate ramped from 0.4 to 0.7 mL min–1 from 0.5 to 13.5 min.
Ionization was performed with a spray voltage of 3 kV and a capillary temperature of 360°C. Nitrogen was used as desolvation gas.
For monitoring, the scanned mass range was between 100 and 1,500 m/z, at a resolution m/Δm 280,000 full-width at half maximum (FWHM) (m/z 200) in positive mode, with automatic gain control (ACG) target 3 × 106, a maximum injection time (IT) of 200 ms.
For compound identification, full-scan MS/data-dependent MS/MS (ddMS2) experiment was performed on QC samples. Each experiment was composed of one full MS and up to 5 ddMS2. The five ions with the most intense signal detected in the full MS scan (intensity threshold 1.6 × 105) produced a specific MS/MS spectrum. For full MS, the settings were the ones described above, while for the data-dependent MS/MS the settings were the following: positive mode with a resolution of m/Δm 35,000 and an ACG target 1 × 105, a maximum IT of 50 ms, a stepped normalized collision energy (NCE, 15, 30, 45), an isolation window of 0.4 m/z.
All data were acquired and processed with the software XcaliburTM version 3.0.63 (Thermo Fisher Scientific, Bremen, Germany).
XcaliburTM raw data files were imported into Thermo Compound Discoverer 2.1.0.398 (Thermo Fisher Scientific, Bremen, Germany) and analyzed following a standard pipeline for untargeted metabolomics for high resolution spectra. The important values for features extraction are the following: precursor ion deviation 5 ppm, maximum retention time shift 0.5 min, signal-to-noise threshold (S/N) 3, minimum peak intensity for peak selection 1 × 106 au, retention time shift for grouping 0.5 min, and relative intensity tolerance for isotope search 30%. The exact masses of unknown compounds found in the samples were compared to online databases (PubChem, ChemSpider, mzCloud) and to an in-house library of 650 natural compounds (mass tolerance = 5 ppm) for identification.
After the analysis, a table with putative compound names and the molecular formula, exact masses, retention times, and chromatographic area for each sample was exported for further processing. All features found in the medium blank samples were removed from the samples. Data were then filtered based on QCs coefficient of variation (CV): only features with CV < 20% were retained (Dunn et al., 2011).
Finally, data were normalized on diatom biomass (using F0 as a proxy) and Pareto scaled to obtain normally distributed data. The obtained .csv table was used to perform statistical analysis with MetaboAnalystR (Chong and Xia, 2018). Principal component analysis (PCA) was performed to detect grouping and outliers in the samples. Significant features were selected from the results of one-way ANOVA analysis (FDR-adjusted p-value cutoff = 0.05, Fisher LSD post hoc analysis), which were visualized by heatmaps (distance measure = euclidean, clustering algorithm = Ward).
After statistical analysis, significant features were selected in the Thermo Compound Discoverer molecule list and exported to SIRIUS v. 4.0 (Böcker et al., 2009) to confirm features identity. Default settings for Orbitrap High Resolution Mass Spectrometry were used (ppm = 5), choosing all the possible adducts as candidates. For structural evaluation of compounds, CSI:FingerID (Dührkop et al., 2015) was used to compare to PubMed spectral database.
Targeted detection of oxylipins was based on a method by Rettner et al. (2018). Briefly, measurements were performed on AcquityTM UPLC system (Waters, Milford, MA, United States) coupled with a QTrap® 5500 (ABSciex, Darmstadt, Germany). We used an ACQUITY UPLC® BEH C18 column for separation (1.7 μm, 2.1 × 100 mm; Waters, Eschborn, Germany) kept at 50°C. The QTrap 5500 was operated in negative ionization mode only using scheduled multiple reaction monitoring (MRM). The scheduled MRM window was 60 s, and each oxylipin parameter was optimized individually (CE, EP, DP, CXP). The investigated oxylipins are the same analyzed by Rettner et al. (2018). Following instrument settings were used: curtain gas 35, collision gas medium (MRM); ion spray voltage −4,000, temperature 500°C, ion source gas 1 and 2 40. Solvent used were A: 100% H2O + 0.01% CH3COOH and B: 100% CH3OH + 0.01% CH3COOH with a solvent flow 0.3 mL * min–1. Injection volumes 10 μL. The gradient started at 42% B, ramped to 86% B at 12.5 min, then 98% B at 15.5 min, returned to 42% B in 0.5 min and re-equilibrated for 1 min. Manual integration of corresponding peaks was carried out using the Analyst software version 1.6. To confirm their presence in all samples, arachidonic acid and 15-hydroxyeicosatetraenoic acid (HETE) were additionally measured in negative mode on a UHPLC Dionex UltiMate® 3000 (Thermo Fisher Scientific, Dreieich, Germany), coupled to an ESI-Orbitrap MS Q-Exactive Plus (Thermo Fisher Scientific, Dreieich, Germany), following the method mentioned in the previous paragraph. Identity of the compounds was confirmed by comparison with an external standard.
Diproline was quantified following the method of Gillard et al. (2013). One microliter of the extract was injected into an ISQ Trace GC Ultra GC/MS system (Thermo Fisher, Dreieich, Germany) equipped with a 0.25 μm × 0.25 mm × 30 m DB-5MS + DG column (Agilent, Böblingen, Germany). Helium 5.0 (Linde AG, Pullach, Germany) was used as carrier gas with a constant flow of 1.2 mL min–1. The split ratio was 1:8. The initial oven temperature of 155°C was held for 3 min, ramped to 210°C with 25°C min–1, then to 255°C with 7°C min–1, and finally to 315°C with 25°C min–1, which was held for 3 min. The injector temperature was kept at 300°C during the entire run. The scanned mass range was between 50 and 400 m/z with a scan rate of 0.5 scans s–1 and an inter-scan delay of 0.04 s–1. Electron ionization was carried out at 70 eV.
To quantify diproline, the peak area of both diproline and internal standard (caffein, 15 nmol) was determined with the function Quan Browser included in the software XcaliburTM version 3.0.63 (Thermo Fisher Scientific, Bremen, Germany) and Microsoft Office Excel (Microsoft®, United States). All results were normalized to the diatom biomass. A one-way ANOVA followed by Bonferroni’s multiple comparisons tests was performed using GraphPad Prism version 7.00 for Windows (GraphPad Software, La Jolla, CA, United States6).
In order to maximize sexual reproduction success, diatoms need to finely synchronize their cell cycle. When a suitable mating partner is present, S. robusta cell cycle is temporarily arrested in G1 phase by the SIP of the opposite mating type, resulting in a synchronized switch from a mitotic to meiotic cell cycle in both partners (Gillard et al., 2013; Moeys et al., 2016). Some studies reported that algicidal bacteria can have an effect on microalgal cell cycle progression (Pokrzywinski et al., 2017). We therefore tested if the effect of bacterial exudates on sexual reproduction observed by Cirri et al. (2018) is due to an interference with the regulation of the cell cycle during the initial sexual stages.
The relative number of MT– cells in G1- and S/G2-phase of the cell cycle was measured in six different treatments after dark-synchronization in G1 phase (Figure 1): control (non-induced, axenic, C), induced cultures (axenic, SIP), Roseovarius sp. exudates + non-induced cultures (R), Maribacter sp. exudates + non-induced cultures (M), Roseovarius sp. exudates + induced cultures (SIP + R), and Maribacter sp. exudates + induced cultures (SIP + M).
Ten hours after re-illumination, the percentage of cells in S/G2 phase was significantly lower (p < 0.001) in the SIP-induced cultures compared to non-induced controls, confirming that SIP+ arrests cell cycle progression of MT– in G1 phase (Moeys et al., 2016; Figure 2). The presence of exudates only (without SIP+ induction) did not reduce the peak in S/G2-phase cells, suggesting that the bacterial exudates did not affect the cell cycle progression in mitotic cells. More importantly, post hoc contrasts (SIP vs. SIP + M and SIP vs. SIP + R) did not show significant effects of exudates on cell cycle progression compared with SIP+-treated cultures (p = 0.8 and p = 0.91, respectively). Therefore, we conclude that the effect of bacterial exudates on sexual reproduction in S. robusta is not due to interference with the SIP+-induced cell cycle arrest.
Figure 2. Cell cycle analysis. Flow cytometric measurements of the percentages of cells that have progressed through S-phase for all six experimental treatments. C is the axenic, non-induced control; M is the non-induced control + Maribacter sp. exudates; R is the non-induced control + Roseovarius sp. exudates; SIP is the induced axenic control; SIP + M is the induced culture + Maribacter sp. Exudates; SIP + R is the induced control + Roseovarius sp. exudates. Proportion post-S-phase cells differed significantly between all non-conditioned cells (“a”) and SIP+-conditioned cells (“b”). *p < 0.05, ∗∗p < 0.01, and ∗∗∗p <0.001.
To study the transcriptional changes in S. robusta MT– cells in response to the presence of bacterial exudates, we extracted mRNA of induced and non-induced diatom cultures; both untreated and treated with bacterial exudates after 24 h dark-synchronization followed by 10 h of illumination (Figure 1). We obtained expression data for 25,557 genes. 4,225 unique genes (16.6% of the expressed genes) were DE in at least one treatment (Table 1, |LFC| > 1, FDR < 0.05) and more than half of these genes were functionally annotated (>59% in each comparison).
Table 1. Summary of the number of significantly differentially expressed genes in different comparisons.
A MDS plot of the differences in gene expression profiles between RNA-seq samples (Figure 3A) showed that the strongest difference in gene expression between samples was caused by the induction of sexuality (SIP+-treatment). This was confirmed by the high number of DE genes in induced cultures compared to non-induced cultures (SIP vs. C, SIP + M vs. M, and SIP + R vs. R: Table 1 and Supplementary Tables S1, S2). Moreover, in the comparisons of non-induced control cultures (C), non-induced cultures treated with Maribacter sp. exudates (M), and non-induced cultures treated with Roseovarius sp. exudates (R) with their SIP+-treated equivalents (SIP, SIP + M and SIP + R, respectively), high amounts of genes that were up- or downregulated in response to SIP+ were shared in all three comparisons (28% of the total upregulated genes and 40.1% of the total downregulated genes are shared in all three comparisons, Figures 3B,C).
Figure 3. (A) Multi-dimensional scaling (MDS) plot for the obtained transcriptomes. Distance between samples is based on log2 fold changes. C is the axenic non-induced control; M is the non-induced control + Maribacter sp. exudates; R is the non-induced control + Roseovarius sp. exudates; SIP is the induced axenic control; SIP + M is the induced culture + Maribacter sp. exudates; SIP + R is the induced control + Roseovarius sp. exudates. (B,C) Venn diagrams of SIP+-induced up- (B) and downregulated (C) S. robusta genes. The up- and downregulated genes thresholds are: log2 fold change (LFC) = 1, false discovery rate (FDR) = 0.05.
Of this shared set of 329 genes that are SIP+-upregulated despite bacterial exudates presence (Figure 3B), some are associated to early meiosis-related processes (Table 2), especially dsDNA break repair, DNA duplex unwinding, and DNA replication (Supplementary Table S1: GO enrichment results). In conclusion, we show that of the known SIP+-triggered processes, early meiosis is not significantly affected by either bacterium.
Table 2. Upregulated genes involved in sexual reproduction and diproline production shared by all SIP+-induced cultures compared to non-induced controls (SIP vs. C, SIP + M vs. M, and SIP + R vs. R).
We also found upregulation of genes involved in cGMP biosynthesis (GC) and breakdown (phosphodiesterases, PDE) (Table 2 and Supplementary Table S1). It has been shown that cGMP signaling likely plays an important role as a secondary messenger during the onset of sexual reproduction in pennate diatoms (Moeys et al., 2016; Basu et al., 2017). The upregulation of these genes was not uniform across the experimental treatments (Table 2), with some GC and PDE genes showing higher upregulation in axenic conditions (Sro991_g228730, LFC = 4.09) while others being more upregulated either in presence of Roseovarius sp. exudates (Sro1233_g254830) or in presence of Maribacter sp. exudates (Sro218_g090200, Sro1656_g289030). Interestingly, expression of several receptor-type GCs with PDE activities (GC/PDEs) was triggered by Maribacter sp. exudates (upregulation of seven GCs SIP + M vs. SIP, two of which contain a PDE domain, Supplementary Table S7). These receptor-type GCs were not DE in axenic conditions or in presence of Roseovarius sp. exudates, suggesting a role for specific cGMP-related signaling pathways during the perception of Maribacter sp. It has been shown that cyclic nucleotide signaling is crucial for an array of physiological processes in diatoms, from regulation of silicon cycle (Aline et al., 1984; Smith et al., 2016) to acclimation to CO2 (Hennon et al., 2015). Moreover, this mechanism was also suggested to be involved during the onset of the sexual reproduction in the diatom Pseudo-nitzschia multistriata (Basu et al., 2017). In plants, signaling by cyclic nucleotides (cGMP and cAMP) is well studied (Isner and Maathuis, 2018) and cAMPs were suggested to play a role in plant–bacteria interactions (Tian et al., 2012). In diatoms or other algae, a similar role of cGMP in inter-kingdom crosstalk has not been described so far. Our results suggest that these pathways may be involved in either the diatom/bacteria recognition process, or in the negative modulation of reproduction by Maribacter sp.
The second major separation in gene expression profiles of S. robusta observed in the MDS plot corresponds to the presence or absence of bacterial exudates in MT– cultures (Figure 3A). The replicates of induced samples treated with bacterial exudates (SIP + M and SIP + R) clustered together more closely compared to the replicates of non-induced samples (M and R), suggesting that the transcriptional changes caused by the bacterial exudates were more coherent when SIP+ is present. Additionally, the number of DE genes in response to the bacterial exudates was higher in the presence of SIP+ (Table 1: compare M vs. C, 331 DE genes with SIP + M vs. SIP, 530 DE genes; and compare R vs. C, 107 DE genes with SIP + R vs. SIP, 190 DE genes). Moreover, there is only limited overlap between genes that are DE in response to bacterial exudates in presence and absence of SIP+ (Supplementary Figure S2).
Because Maribacter sp. and Roseovarius sp. affect sexual reproduction of S. robusta, albeit in opposite directions (Cirri et al., 2018), we next focused on transcriptional changes observed in induced S. robusta in the presence and absence of bacterial exudates (SIP + M vs. SIP and SIP + R vs. SIP). Venn diagrams showing the numbers of shared and unique up- and downregulated genes between SIP + M vs. SIP and SIP + R vs. SIP are, respectively, shown in Figures 4A,B, while Venn diagrams in Figures 4C,D display up- and downregulated genes in M vs. C and R vs. C, respectively. A detail description of up- and downregulated genes in the different treatments of induced S. robusta cultures is reported in Supplementary Tables S3, S5, S7, S8, S10.
Figure 4. Venn diagrams showing overlap between Maribacter sp. (M)- and Roseovarius sp. (R)-induced DE genes in presence (A,B) and absence (B,C) of SIP+. (A,B) Upregulated (A) and downregulated (B) genes in response to Maribacter sp. (SIP + M) and Roseovarius sp. (SIP + R) treatments in presence of SIP+. (C,D) Upregulated (C) and downregulated (D) genes in response to Maribacter sp. (M) and Roseovarius sp. (R) treatments in absence of SIP+. The up- and downregulated genes thresholds are: log2 fold change (LFC) = 1, false discovery rate (FDR) = 0.05.
Both in induced and in non-induced cultures, Maribacter sp. exudates triggered significantly more DE genes compared to Roseovarius sp. exudates (Table 1, Figure 4, and Supplementary Figures S1, S2). This indicates that Maribacter sp. has a stronger effect on the physiology of S. robusta compared to the Roseovarius sp.
One of our main research questions is whether the positive effect of Roseovarius and negative effect of Maribacter on sexual reproduction is linked to a change in diproline biosynthesis by the diatom. Moeys et al. (2016) hypothesized that the upregulation of proline biosynthesis is crucial for diproline synthesis, thereby increasing the proline pool that can be used for diproline production.
Δ1-Pyrroline-5-carboxylate synthetase (P5CS, Sro2012_g310890), a key enzyme in proline biosynthesis (Hu et al., 1992), was upregulated in SIP vs. C, SIP + M vs. M, and SIP + R vs. R, but the strongest upregulation was observed in presence of Roseovarius sp. exudates (LFC = 6.89, FDR < 10–6), while upregulation was less strong in axenic conditions (LFC = 3.64, FDR < 10–4) or in presence of Maribacter sp. exudates (LFC = 4.94, FDR < 10–5) (Table 2). Another gene of this pathway, Δ1-pyrroline-5-carboxylate reductase (PC5, Sro216_g089310), was also upregulated in all three comparisons. Here too, upregulation was stronger in the presence of Roseovarius sp. exudates (LFC = 4.46, FDR < 10–5) compared to Maribacter sp. exudates (LFC = 3.15, FDR < 10–3) or axenic conditions (LFC = 3.84, FDR < 10–4) (Table 2). To check if this gene regulation affects diproline production, we measured the attraction pheromone concentration in the medium of induced cultures both in presence and absence of the Maribacter sp. and Roseovarius sp. exudates (Figure 5A). These measurements confirmed that diproline is only produced in induced cultures. In absence of SIP+, the bacterial exudates did not trigger diproline production. Furthermore, as suggested by the transcriptome data, an increase in diproline concentration after treatment with Roseovarius sp. exudates occurs; however, the increase is small and not significant (Figure 5A). More interestingly, we observed that the diproline production in induced cultures was significantly lower in the presence of Maribacter sp. exudates (p < 0.012) compared to axenic cultures. Our transcriptomic data reveal that the proline biosynthetic pathway was strongly upregulated in all induced cultures. Since conditioning with SIP+ induces diproline production, this upregulation in response to all treatments with SIP+ supports the hypothesis by Moeys et al. (2016) that an increased proline production feeds into the diproline production pathway. Interestingly, diproline biosynthetic genes are upregulated most strongly in the presence of Roseovarius sp. exudates, which is in accordance with the slightly higher concentration of diproline and the enhanced reproduction success observed by Cirri et al. (2018). On the other hand, induced cultures in presence of Maribacter sp. exudates did not show a decrease in gene expression of these genes. Thus, the lower concentration of diproline and the negative effect of Maribacter sp. on sexual reproduction cannot be explained through this pathway.
Figure 5. (A) Diproline and (B) arachidonic acid relative concentration. Arachidonic acid is normalized to diatom biomass. Diproline is normalized to diatom biomass and internal standard. Internal standard (IS) is caffeine (15 nmol). Significance was tested with a one-way ANOVA (adjusted p-value after Bonferroni’s correction for multiple comparisons = 0.05). SIP+ axenic treatment is taken as control for assessing significant differences in diproline concentration in SIP + M and SIP + R treatments. C is the axenic, non-induced control; M is the non-induced control + Maribacter sp. exudates; R is the non-induced control + Roseovarius sp. exudates; SIP is the induced axenic control; SIP + M is the induced culture + Maribacter sp. exudates; SIP + R is the induced control + Roseovarius sp. exudates. *p < 0.05, ∗∗p < 0.01, and ∗∗∗p <0.001.
Considering that the exact mechanism of the attraction pheromone biosynthesis remains unknown and that a significant amount of S. robusta genes lack a good annotation, it is possible that other but yet unknown pathways related to diproline production are involved.
Gene ontology enrichment of a set of genes that is upregulated in induced culture only when Maribacter sp. exudates are present (SIP + M vs. SIP, Supplementary Table S7) showed a downregulation of several amino acid catabolic pathways, especially those of tyrosine (two genes), arginine (two genes), and phenylalanine (two genes) (Supplementary Table S9). The downregulation of these pathways was stronger in presence of SIP+ (SIP + M vs. SIP, Table 4): four downregulated genes involved in tyrosine metabolism, four for phenylalanine catabolism, and two for arginine catabolism. Downregulation in response to Maribacter sp. exudates was strongest for a tyrosine aminotransferase (Sro379_g130480) and two fumarylacetoacetase (Sro341_g121520 and Sro341_g121510) (LFC < −3.9, LFC < −3.4, and LFC < −3.33, respectively, in SIP + M vs. SIP, Supplementary Table S8). Both are involved in phenylalanine catabolism: the former enzyme catalyzes the conversion of tyrosine to 4-hydroxyphenylpyruvate, the latter breaks down fumarylacetoacetate into fumarate and acetoacetate (Santucci et al., 2017), thus influencing the TCA cycle. Interestingly, the phenylalanine-to-tyrosine pathway was one of the processes that was actively upregulated by SIP+ (Supplementary Table S1: phenylalanine 4-monooxygenase activity). In higher plants, phenylalanine and tyrosine are produced via the shikimate pathway (Tzin and Galili, 2010) and it has been suggested that downstream products like tyramine are involved in defense responses (Trezzini et al., 1993). In diatoms, less is known about the importance of the metabolism of these two amino acids. However, their biosynthesis is strongly connected to the biosynthetic pathway of tryptophan (Bromke, 2013), an amino acid that has a fundamental role in algae–bacteria interactions (Amin et al., 2015).
Interestingly, in cultures treated with SIP+ and Maribacter sp. exudates, a total of 40 genes associated with photosynthetic functions and the light-harvesting complex (LHC) were upregulated compared to the SIP+ only treatment (SIP + M vs. SIP), many of which were downregulated in SIP vs. Control (Table 3 and Supplementary Table S7). Twenty-two of these were fucoxanthin-chlorophyll a–c binding proteins (FCPs, Supplementary Table S7), intrinsic proteins of the thylakoid membrane that bind chlorophyll a and c and that are responsible for the absorption of the blue–green wavelengths in aquatic environments (Schellenberger Costa et al., 2012; Kuczynska et al., 2015). FCPs are also involved in non-photochemical quenching (NPQ) (Kuczynska et al., 2015), a mechanism that protects plants and algae from high light stress (Horton and Ruban, 2004; Dong et al., 2016). So far, nothing was known about possible effects of bacteria on diatom FCPs or NPQ, and the biological significance of this observation requires more in-depth photophysiological studies. Next to the FCP genes, we identified four genes involved in carotenoid and chlorophyll biosynthesis which are upregulated in SIP + M vs. SIP: a carotene desaturase (Sro536_g162170), a glutamate tRNA ligase (Sro20_g014070), and two glutamate-1-semialdehyde 2,1-aminomutases (Sro479_g151140 and Sro1597_g284880) (Supplementary Table S7). The strong upregulation of these enzymes, combined with the downregulation of the arginine catabolic pathway (Table 4), could diminish the availability of glutamate and arginine, two important substrates for proline biosynthesis in diatoms (Bromke, 2013).
Table 3. GO enrichment of genes upregulated by Maribacter sp. in the presence of SIP+ (SIP + M vs. SIP).
Table 4. GO enrichment of genes downregulated by Maribacter sp. in the presence of SIP+ (SIP + M vs. SIP).
Taking these results into account, it appears that treatment with Maribacter sp. exudates has a strong influence on gene expression of amino acid metabolism and LHC genes. We observed that Maribacter sp. exudates do not negatively influence the sexual reproduction of S. robusta by directly targeting proline production. Instead, we hypothesize that the upregulation of photosynthetic pigment production, combined with the diminishing glutamate availability might reduce the intracellular pool of proline precursors (glutamate, arginine) and thereby indirectly influences diproline biosynthesis (Figure 6). Contrary, in Roseovarius sp.-treated samples, we do observe an upregulation in proline biosynthetic genes and no upregulation of LHC-related genes (see Supplementary Tables S3–S6). This could result in an increased or prolonged diproline production and release, explaining the enhancement of sexual efficiency observed by Cirri et al. (2018) and the concentration of diproline comparable to that of axenic cultures.
Figure 6. Overview of metabolic changes in S. robusta when exposed to SIP+ and Maribacter sp. exudates. In green are the upregulated processes, red the downregulated ones. Maribacter sp. exudates do not directly influence early meiotic processes. Stress induced by bacterial exudates triggers cGMP signaling cascades, an upregulation of photosynthetic pigment production and an oxidative stress response (by tocopherol and glutathione biosynthesis). Flux through the urea- and TCA cycle is reduced, diminishing intracellular arginine, fumarate, malate, and glutamate pools. Glutamate, precursor for proline synthesis, is used for porphyrin production, so the upregulated porphyrin synthesis could affect proline biosynthesis and thus also diproline production. Phe, phenylalanine; Tyr, tyrosine; Trp, tryptophan; Glu, glutamate; Pro, proline; GSH, glutathione; FA, fatty acid; PEP, phosphoenolpyruvate.
Apart from transcriptional changes in S. robusta that were specific to the exudates produced either by Maribacter sp. or Roseovarius sp., both bacterial exudates caused upregulation of metabolic processes related to oxidative stress responses, detoxification, and defense mechanisms (Supplementary Tables S10, S11).
Several genes that were upregulated in response to both Roseovarius sp. and Maribacter sp. exudates in the presence of SIP+ encode proteins that contain a flavodoxin-like fold, as a NADPH-dependent oxidoreductase (Sro481_g151580, LFC > 7) and an alcohol dehydrogenase (Sro989_g228490, LFC > 5) (Supplementary Table S10). These proteins are involved in energy metabolism, electron transfer, and in response mechanisms to reactive oxygen species (ROS)-stimulated stress (Quijano et al., 2016; Sies et al., 2017; Poirier et al., 2018).
Moreover, both bacterial exudates influenced glutathione metabolism. Glutathione is a tripeptide acting as fundamental antioxidant in many eukaryotes, including phytoplankton (Poirier et al., 2018). Glutathione S-transferases (GST) (Sro1751_g295250 and Sro945_g223090) and glutathionyl-hydroquinone reductases (GS-HQR) (Sro596_g172810 and Sro2126_g315740) were found to be especially upregulated (Supplementary Table S10). These enzymes play important roles in detoxification reactions in plants. GSTs transfer GSH to electrophilic centers of toxic, hydrophobic compounds, and the resulting conjugates are more soluble and therefore less toxic (Sheehan et al., 2001). GS-HQRs are a particular type of GSTs that reduce GS-hydroquinones and are believed to play a maintenance role for an array of metabolic pathways in photosynthetic organisms (Belchik and Xun, 2011).
Furthermore, sterol and fatty acid biosynthetic pathways were affected by the presence of both bacterial exudates. Cholesterol catabolism and the concomitant upregulation of tocopherol cyclase activity (Supplementary Table S11) indicated that S. robusta may use this molecule as a defense mechanism against oxidative stress. Tocopherols are antioxidants present in plastids of all lineages of photosynthetic eukaryotes and are involved in different stress responses in diatoms (Havaux and García-Plazaola, 2014; Lauritano et al., 2015). Fatty acid catabolism (fatty acid beta-oxidation) and ketone body synthesis were particularly influenced by Maribacter sp. exudates. Acetyl-CoA metabolism (Table 4) and fumarylacetoacetase activity (Table 4) were downregulated, leading to a decreased fumarate pool, involved in the TCA cycle. Also enoyl-CoA hydratase (Sro2125_g315680, LFC < −3.3, Supplementary Table S8), an enzyme responsible for hydrating the double bond between the second and third carbons of Acyl-CoA and involved in fatty acid catabolism to produce acetyl-CoA and energy (Bahnson et al., 2002), was downregulated. All these observations suggest that, in the presence of bacteria exudates, S. robusta metabolism shift from fatty acids catabolism to intracellular accumulation of this compounds (Shi and Tu, 2015), maybe to function as defense mechanisms. The detection of upregulated acyl-CoA metabolic pathways in presence of Maribacter sp. exudates (SIP + M vs. SIP, Supplementary Table S11), different from the downregulated acyl-CoA pathways mentioned above, supports this hypothesis. Interestingly, a putative 12-oxophytodienoate reductase (OPR) (Sro250_g098890) was strongly upregulated in induced cultures when both bacterial exudates were present (LFC > 6) (Supplementary Table S10). OPRs are flavoprotein enzymes that regulate jasmonic acid biosynthesis from the fatty acid linolenic acid, a crucial mediator of chemical defense mechanisms and plant–microbe interactions in plants (Erb, 2018; Koo, 2018). More generally, OPRs function in α-linolenic acid metabolism and oxylipin biosynthesis (Weber, 2002), well-studied oxygenated fatty acid derivates known for their function as defense molecules in algae (Wasternack, 2007) and especially in diatoms (Pohnert, 2002). A targeted lipidomics analysis for fatty acids and oxylipins was performed to check if indeed the production of these compounds was increased in the presence of bacterial exudates. Arachidonic acid, a fundamental polyunsaturated fatty acid involved in cell signaling (Piomelli, 1993) and inflammation (Calder, 2011) and also synthesized by diatoms (Dunstan et al., 1993), was the only detectable oxylipin in our metabolomics analysis. This is possibly because it is one of the most abundant and important precursor for a range of oxylipins (Pohnert and Boland, 2002; Rettner et al., 2018). The concentration of released arachidonic acid was significantly higher in both SIP + M and SIP + R when compared to induced axenic conditions (SIP) and also in the presence of Roseovarius exudates without SIP+ (R) compared to the axenic control (C) (Figure 5B). We further investigated oxylipins that were also measured by Rettner et al. (2018), but could find no upregulation in any treatment. Oxylipins were so far predominantly detected from lysed or damaged diatom cells (Pohnert and Boland, 2002), but recently it was suggested that these compounds could have a role in diatom resistance against algicidal bacteria (Meyer et al., 2018) and our study expands this concept even further.
The medium of S. robusta cultures in different treatments was used for metabolomic analysis to gain insights into chemical responses of the induced S. robusta cells exposed to bacterial exudates. A principal component analysis (PCA) of both bacterial exudates treatments and an axenic control in presence of SIP+ (SIP + M, SIP + R, SIP) shows that the exometabolome of S. robusta changes under the influence of bacterial exudates, but the separation of the groups is small (Supplementary Figure S3). We therefore decided to analyze the Roseovarius and Maribacter datasets separately to highlight potential differences between the two bacterial treatments.
SIP + M and SIP + R samples clearly clustered separately from S. robusta axenic samples (SIP) and from bacterial exudates alone (Figures 7A,C), confirming that both bacterial exudates influenced the S. robusta exometabolome. To check if these differences were due to presence of molecules from bacterial exudates or in fact caused by S. robusta exometabolites, features found in exudates of the bacteria were removed from the feature list of SIP + M and SIP + R. The PCA plots show a clear separation of Maribacter sp. exudates-treated induced cultures (SIP + M) from the induced axenic controls (SIP) (Figure 7B), while cultures treated with Roseovarius sp. exudates (SIP + R) are largely overlapping with induced axenic cultures (Figure 7D). When we compared the metabolome of non-induced cultures in presence of bacterial exudates (R and M) to the axenic non-induced controls (C), both Roseovarius- (R) and Maribacter exudates-treated cultures (M) are overlapping with the controls (Supplementary Figure S4). These results corroborate the outcome of our physiological and transcriptomic analysis, with Maribacter sp. having a stronger effect on the sexual reproduction and the metabolism of sexually induced MT– S. robusta cells.
Figure 7. PCA scores plot of exometabolome samples of SIP+-induced cultures and bacteria exudates. (A) PCA of SIP axenic control, induced cultures + Maribacter sp. exudates and Maribacter sp. exudates alone. (B) PCA of SIP axenic control and induced cultures + Maribacter sp. exudates with subtraction of features from Maribacter sp. exudates alone. (C) PCA of SIP axenic control, induced cultures + Roseovarius sp. exudates and Roseovarius sp. exudates. (D) PCA of SIP axenic control and induced cultures + Roseovarius sp. exudates with subtraction of features from Roseovarius sp. exudates.
We therefore used a comparative metabolomics approach to investigate the exudates of Maribacter sp. and the exometabolome of induced (SIP+-treated) S. robusta when exposed to Maribacter sp. exudates (SIP + M) to search for putative signaling molecules. We performed a one-way ANOVA (FDR cutoff = 0.05, Fisher LSD post hoc analysis) to select for significant features and chose the top 25 among them (ranked by adjusted p-value). Although most of the molecules were identified as unknown, retention times allowed a classification based on their polarity (Figure 8A). Most of the upregulated compounds in SIP + M treatment ranged from mid-polar to non-polar, eluting between 4.5 and 9 min (from 45% of acetonitrile to 100% of acetonitrile solvent composition), while many of the upregulated molecules in SIP medium were non-polar, eluting after 9 min. When we included molecules from bacterial exudates in the analysis (Figure 8B), several compounds released in the medium by Maribacter sp. showed a high chromatographic peak intensity that was significantly decreased in SIP + M treatment, suggesting the potential involvement of a signaling mechanism in which the bacterial compound could be degraded by the diatom. In particular, two compounds, eluting at 2.95 min (MW = 165.06493 Da, putative chemical molecular formula C6H7N5O) and at 8.45 min (MW = 224.08345 Da, putative molecular formula C15H12O2) had high peak intensities in Maribacter sp. exudates (peak intensity = 106), while their intensities were, respectively, three and one order of magnitude lower in SIP + M treatments. Moreover, these compounds were not present in high amounts in Roseovarius sp. exudates (Supplementary Figure S5). After obtaining a fragmentation tree from our MS/MS data and comparing it to public and in-house libraries, we got putative structure for the two compounds: the first one was annotated as a presumed methylguanine, a methyl derivative of the nucleobase guanine, while the second one was annotated as a small weight flavanone (Figure 6B). Methylguanines are naturally occurring modified purines from tRNA in humans (De Bont and van Larebeke, 2004) but they are not known to be produced by bacteria as exometabolites. Flavanones are a type of flavonoids that often occur in plants and have several functions, from antioxidants to antimicrobial (Cushnie and Lamb, 2005), and were also found in a Pseudovibrio sp. (Crowley et al., 2014). However, flavanone production by other marine bacteria as well as a function in inter-kingdom crosstalk has not yet been described. Further metabolomics experiments using larger volumes of bacterial exudates and S. robusta are needed to better elucidate the nature of these compounds. Moreover, fractionation-guided bioassays may explain their biological function.
Figure 8. Heatmaps of up- and downregulated S. robusta exometabolites: (A) in presence or absence of Maribacter exudates after subtraction of Maribacter sp. features and (B) in presence or absence of Maribacter exudates and up- and downregulated exometabolites from Maribacter sp. For two way comparisons, significance was evaluated with a t-test (α = 0.05), hierarchical clustering is based on Euclidean distances and using Ward’s method. For multiple comparisons, significance was evaluated with a one-way ANOVA (adjusted p-value after Fisher LSD post hoc test = 0.05), hierarchical clustering was based on Euclidean distances and using Ward’s method. Red is for upregulated metabolites and blue is for downregulated metabolites.
Bacteria associated to S. robusta are able to modulate diproline concentrations in the medium and two of them (Roseovarius sp. and Maribacter sp.) have an opposite effect on the sexual efficiency of S. robusta, with Maribacter sp. reducing mating efficiency and Roseovarius sp. slightly improving it (Cirri et al., 2018). This effect is observed also when S. robusta cultures are treated with exudates from this two bacteria (Cirri et al., 2018). Following these findings, we now provide the first insights into the bacterial exudates effect on sexual reproduction of S. robusta on a molecular level using a combination of physiological, metabolomic, and transcriptomic approaches. With the integration of different data types, we were able to conclude that both bacterial exudates do not directly interfere with cell cycle arrest and expression of genes related to sexual reproduction of S. robusta. Rather, Roseovarius sp. exudates cause an increase of proline biosynthetic activity, whereas Maribacter sp. exudates influence amino acid and LHC biosynthetic processes. We hypothesize that these two distinct responses lead to opposite effects on production of the attraction pheromone diproline released by S. robusta. Moreover, both bacterial exudates are triggering an oxidative stress response in the diatom, which is involving fatty acid metabolism and oxylipin production. It is important to highlight that in addition to the annotated DE genes discussed here, several highly up- and downregulated genes in all treatments were lacking a functional annotation. Better annotations will provide future studies with more knowledge to unravel the influence of bacteria on diatom sexuality and metabolic regulation. These results will pave the way to a better understanding of diatoms life cycle regulation in natural environments and more generally of the importance of inter-kingdom signaling for diatom reproduction and survival.
The datasets generated for this study can be found in the Gene Expression Omnibus, https://www.ncbi.nlm.nih.gov/geo/query/acc.cgi?acc=GSE131727.
EC, SDD, GB, and MW performed the experiments and analyzed the data. EC and SDD analyzed the transcriptomics data. EC analyzed the metabolomics data. GB analyzed the flow cytometry data. CO-C and KV performed the gene model prediction. MW analyzed the oxylipins concentration. EC, SDD, WV, and GP conceived the experiments and the experimental setup. EC, SDD, WV, and GP wrote the manuscript. All authors reviewed the manuscript and the results.
This work was supported by the European Union’s Horizon 2020 research and innovation programme under the Marie Sklodowska-Curie grant agreement No. 642575. SDD was supported by the Fund for Scientific Research – Flanders (FWO–Flanders, Belgium), grant No. G0D6114N and the research council of Ghent University (BOF/GOA No. 01G01715). GB was supported by a Research Foundation Flanders (FWO) Aspirant grant (No. 3F001916).
The authors declare that the research was conducted in the absence of any commercial or financial relationships that could be construed as a potential conflict of interest.
The authors would like to thank Koen Van den Berge for the support in transcriptomics statistical analysis, Katerina Pargana for transcriptomics analysis, and Remington X. Poulin for proofreading.
The Supplementary Material for this article can be found online at: https://www.frontiersin.org/articles/10.3389/fmicb.2019.01790/full#supplementary-material
Ajimura, M., Leem, S. H., and Ogawa, H. (1993). Identification of new genes required for meiotic recombination in Saccharomyces cerevisiae. Genetics 133, 51–66.
Alexa, A., and Rahnenführer, J. (2009). Gene set enrichment analysis with topGO. Bioconductor Improv. 27.
Aline, R. F., Reeves, C. D., Russo, A. F., and Volcani, B. E. (1984). Role of silicon in diatom metabolism: cyclic nucleotide levels, nucleotide cyclase, and phosphodiesterase activities during synchronized growth of Cylindrotheca fusiformis. Plant Physiol. 76, 674–679. doi: 10.1104/pp.76.3.674
Amin, S. A., Hmelo, L. R., van Tol, H. M., Durham, B. P., Carlson, L. T., Heal, K. R., et al. (2015). Interaction and signalling between a cosmopolitan phytoplankton and associated bacteria. Nature 522, 98–101. doi: 10.1038/nature14488
Amin, S. A., Parker, M. S., and Armbrust, E. V. (2012). Interactions between diatoms and bacteria. Microbiol. Mol. Biol. R. 76, 667–684. doi: 10.1128/MMBR.00007-12
Azam, F., and Malfatti, F. (2007). Microbial structuring of marine ecosystems. Nat. Rev. Microbiol. 5, 782–791. doi: 10.1038/nrmicro1747
Bahnson, B. J., Anderson, V. E., and Petsko, G. A. (2002). Structural mechanism of enoyl-coa hydratase:? three atoms from a single water are added in either an e1cb stepwise or concerted fashion. Biochemistry 41, 2621–2629. doi: 10.1021/bi015844p
Bairoch, A., and Apweiler, R. (2000). The SWISS-PROT protein sequence database and its supplement TrEMBL in 2000. Nucleic Acids Res. 28, 45–48. doi: 10.1093/nar/28.1.45
Basu, S., Patil, S., Mapleson, D., Russo, M. T., Vitale, L., Fevola, C., et al. (2017). Finding a partner in the ocean: molecular and evolutionary bases of the response to sexual cues in a planktonic diatom. New Phytol. 215, 140–156. doi: 10.1111/nph.14557
Belchik, S. M., and Xun, L. (2011). S-glutathionyl-(chloro)hydroquinone reductases: a new class of glutathione transferases functioning as oxidoreductases. Drug Met. Rev. 43, 307–316. doi: 10.3109/03602532.2011.552909
Bell, W., and Mitchell, R. (1972). Chemotactic and growth responses of marine bacteria to algal extracellular products. Biol. Bull. 143, 265–277. doi: 10.2307/1540052
Blanton, H. L., Radford, S. J., McMahan, S., Kearney, H. M., Ibrahim, J. G., and Sekelsky, J. (2005). REC, Drosophila MCM8, drives formation of meiotic crossovers. PLoS Genet. 1:e40. doi: 10.1371/journal.pgen.0010040
Böcker, S., Letzel, M. C., Lipták, Z., and Pervukhin, A. (2009). SIRIUS: decomposing isotope patterns for metabolite identification. Bioinformatics 25, 218–224. doi: 10.1093/bioinformatics/btn603
Bromke, M. A. (2013). Amino acid biosynthesis pathways in diatoms. Metabolites 3, 294–311. doi: 10.3390/metabo3020294
Buchfink, B., Xie, C., and Huson, D. H. (2015). Fast and sensitive protein alignment using diamond. Nat. Met. 12, 59–60. doi: 10.1038/nmeth.3176
Calder, P. C. (2011). Fatty acids and inflammation: the cutting edge between food and pharma. Eur. J. Pharmacol. 668(Suppl. 1), 50–58. doi: 10.1016/j.ejphar.2011.05.085
Chong, J., and Xia, J. (2018). MetaboAnalystR: an R package for flexible and reproducible analysis of metabolomics data. Bioinformatics 34, 4313–4314. doi: 10.1093/bioinformatics/bty528
Cirri, E., Vyverman, W., and Pohnert, G. (2018). Biofilm interactions—bacteria modulate sexual reproduction success of the diatom Seminavis robusta. FEMS Microbiol. Ecol. 94:fiy161. doi: 10.1093/femsec/fiy161
Crowley, S., O’Gara, F., O’Sullivan, O., Cotter, P., and Dobson, A. (2014). Marine pseudovibrio sp. as a novel source of antimicrobials. Mar. Drugs 12, 5916–5929. doi: 10.3390/md12125916
Cushnie, T. T., and Lamb, A. J. (2005). Antimicrobial activity of flavonoids. Int. J. Antimicrob. Agents 26, 343–356. doi: 10.1016/j.ijantimicag.2005.09.002
De Bont, R., and van Larebeke, N. (2004). Endogenous DNA damage in humans: a review of quantitative data. Mutagen 19, 169–185. doi: 10.1093/mutage/geh025
Dong, H. P., Dong, Y. L., Cui, L., Balamurugan, S., Gao, J., Lu, S. H., et al. (2016). High light stress triggers distinct proteomic responses in the marine diatom Thalassiosira pseudonana. BMC Genet. 17:994. doi: 10.1186/s12864-016-3335-5
Dührkop, K., Shen, H., Meusel, M., Rousu, J., and Bocker, S. (2015). Searching molecular structure databases with tandem mass spectra using CSI:fingerid. Proc. Natl. Acad. Sci. U.S.A. 112, 12580–12585. doi: 10.1073/pnas.1509788112
Dunn, W. B., Broadhurst, D., Begley, P., Zelena, E., Francis-McIntyre, S., Anderson, N., et al. (2011). Procedures for large-scale metabolic profiling of serum and plasma using gas chromatography and liquid chromatography coupled to mass spectrometry. Nat. Protoc. 6, 1060–1083. doi: 10.1038/nprot.2011.335
Dunstan, G. A., Volkman, J. K., Barrett, S. M., Leroi, J. M., and Jeffrey, S. W. (1993). Essential polyunsaturated fatty acids from 14 species of diatom (Bacillariophyceae). Phytochemistry 35, 155–161. doi: 10.1016/s0031-9422(00)90525-9
Durham, B. P., Dearth, S. P., Sharma, S., Amin, S. A., Smith, C. B., Campagna, S. R., et al. (2017). Recognition cascade and metabolite transfer in a marine bacteria-phytoplankton model system. Env. Mircobiol. 19, 3500–3513. doi: 10.1111/1462-2920.13834
Erb, M. (2018). Plant defenses against herbivory: closing the fitness gap. Trends Plant Sci. 23, 187–194. doi: 10.1016/j.tplants.2017.11.005
Field, C. B., Behrenfeld, M. J., Randerson, J. T., and Falkowski, P. (1998). Primary production of the biosphere: integrating terrestrial and oceanic components. Science 281, 237–240. doi: 10.1126/science.281.5374.237
Frenkel, J., Vyverman, W., and Pohnert, G. (2014a). Pheromone signaling during sexual reproduction in algae. Plant J. 79, 632–644. doi: 10.1111/tpj.12496
Frenkel, J., Wess, C., Vyverman, W., and Pohnert, G. (2014b). Chiral separation of a diketopiperazine pheromone from marine diatoms using supercritical fluid chromatography. J. Chromatogr. B 951, 58–61. doi: 10.1016/j.jchromb.2013.12.040
Gillard, J., Frenkel, J., Devos, V., Sabbe, K., Paul, C., Rempt, M., et al. (2013). Metabolomics enables the structure elucidation of a diatom sex pheromone. Angew. Chem. Int. Ed. Engl. 52, 854–857. doi: 10.1002/anie.201208175
Guillard, R. R. L. (1975). “Culture of phytoplankton for feeding marine invertebrates,” in Culture of Marine Invertebrate Animals: Proceedings — 1st Conference on Culture of Marine Invertebrate Animals Greenport, eds W. L. Smithcpesnm and M. H. Chanleycpesnm, (Boston, MA: Springer), 29–60. doi: 10.1007/978-1-4615-8714-9_3
Havaux, M., and García-Plazaola, J. I. (2014). “Beyond non-photochemical fluorescence quenching: the overlapping antioxidant functions of zeaxanthin and tocopherols,” in Non-Photochemical Quenching and Energy Dissipation in Plants, Algae and Cyanobacteria, eds B Demmig-Adams, G. Garab, and W. Govindjeecpesnm, (Dordrecht: Springer), 583–603. doi: 10.1007/978-94-017-9032-1_26
Helliwell, K. E., Scaife, M. A., Sasso, S., Araujo, A. P. U., Purton, S., and Smith, A. G. (2014). Unravelling vitamin B12-responsive gene regulation in algae. Plant Physiol. 165, 388–397. doi: 10.1104/pp.113.234369
Hennon, G. M. M., Ashworth, J., Groussman, R. D., Berthiaume, C., Morales, R. L., Baliga, N. S., et al. (2015). Diatom acclimation to elevated CO2 via cAMP signalling and coordinated gene expression. Nat. Clim. Change 5, 761–765. doi: 10.1038/nclimate2683
Hoff, K. J., Lange, S., Lomsadze, A., Borodovsky, M., and Stanke, M. (2016). Braker1: unsupervised RNA-Seq-Based genome annotation with GeneMark-ET and augustus. Bioinformatics 32, 767–769. doi: 10.1093/bioinformatics/btv661
Horton, P., and Ruban, A. (2004). Molecular design of the photosystem II light-harvesting antenna: photosynthesis and photoprotection. J. Exp. Bot. 56, 365–373. doi: 10.1093/jxb/eri023
Hothorn, T., Bretz, F., and Westfall, P. (2008). Simultaneous inference in general parametric models. Biomol. J. 50, 346–363. doi: 10.1002/bimj.200810425
Hu, C. A., Delauney, A. J., and Verma, D. P. (1992). A bifunctional enzyme (delta 1-pyrroline-5-carboxylate synthetase) catalyzes the first two steps in proline biosynthesis in plants. Proc. Natl. Acad. Sci. U.S.A. 89, 9354–9358. doi: 10.1073/pnas.89.19.9354
Huerta-Cepas, J., Forslund, K., Coelho, L. P., Szklarczyk, D., Jensen, L. J., von Mering, C., et al. (2017). Fast genome-wide functional annotation through orthology assignment by eggNOG-mapper. Mol. Biol. Evol. 34, 2115–2122. doi: 10.1093/molbev/msx148
Huerta-Cepas, J., Szklarczyk, D., Forslund, K., Cook, H., Heller, D., Walter, M. C., et al. (2016). Eggnog 4.5: a hierarchical orthology framework with improved functional annotations for eukaryotic, prokaryotic and viral sequences. Nucleic Acids Res. 44, D286–D293. doi: 10.1093/nar/gkv1248
Isner, J. C., and Maathuis, F. J. M. (2018). cGMP signalling in plants: from enigma to main stream. Funct. Plant Biol. 45, 93–101. doi: 10.1071/FP16337
Jones, P., Binns, D., Chang, H. Y., Fraser, M., Li, W., McAnulla, C., et al. (2014). InterProScan 5: genome-scale protein function classification. Bioinformatics 30, 1236–1240. doi: 10.1093/bioinformatics/btu031
Kolas, N. K., and Cohen, P. E. (2004). Novel and diverse functions of the DNA mismatch repair family in mammalian meiosis and recombination. Cytogenet. Genome Res. 107, 216–231. doi: 10.1159/000080600
Koo, A. J. (2018). Metabolism of the plant hormone jasmonate: a sentinel for tissue damage and master regulator of stress response. Phytochem. Rev. 17, 51–80. doi: 10.1007/s11101-017-9510-8
Kuczynska, P., Jemiola-Rzeminska, M., and Strzalka, K. (2015). Photosynthetic pigments in diatoms. Mar. Drugs 13, 5847. doi: 10.3390/md13095847
Kumar, S., Stecher, G., and Tamura, K. (2016). MEGA7: molecular evolutionary genetics analysis version 7.0 for bigger datasets. Mol. Biol. Evol. 33, 1870–1874. doi: 10.1093/molbev/msw054
Lauritano, C., Orefice, I., Procaccini, G., Romano, G., and Ianora, A. (2015). Key genes as stress indicators in the ubiquitous diatom Skeletonema marinoi. BMC Genom. 16:411. doi: 10.1186/s12864-015-1574-5
Lun, A. T., Chen, Y., and Smyth, G. K. (2016). It’s DE-licious: a recipe for differential expression analyses of rna-seq experiments using quasi-likelihood methods in edgeR. Method Mol. Biol. 1418, 391–416. doi: 10.1007/978-1-4939-3578-9_19
Malviya, S., Scalco, E., Audic, S., Vincent, F., Veluchamy, A., Poulain, J., et al. (2016). Insights into global diatom distribution and diversity in the world’s ocean. Proc. Natl. Acad. Sci. U.S.A. Epub 2016 Feb 29.
Meyer, N., Bigalke, A., Kaulfuss, A., and Pohnert, G. (2017). Strategies and ecological roles of algicidal bacteria. FEMS Microbiol. Rev. 41, 880–899. doi: 10.1093/femsre/fux029
Meyer, N., Rettner, J., Werner, M., Werz, O., and Pohnert, G. (2018). Algal oxylipins mediate the resistance of diatoms against algicidal bacteria. Mar. Drugs 16:486. doi: 10.3390/md16120486
Moeys, S., Frenkel, J., Lembke, C., Gillard, J. T. F., Devos, V., Van den Berge, K., et al. (2016). A sex-inducing pheromone triggers cell cycle arrest and mate attraction in the diatom Seminavis robusta. Sci. Rep. 6:19252. doi: 10.1038/srep19252
Oliveros, J. (2007). Venny. An Interactive Tool for Comparing Lists with Venn Diagrams. Available at: http://bioinfogp.cnb.csic.es/tools/venny/index.html
Patro, R., Duggal, G., Love, M. I., Irizarry, R. A., and Kingsford, C. (2017). Salmon provides fast and bias-aware quantification of transcript expression. Nat. Method 14:417. doi: 10.1038/nmeth.4197
Piomelli, D. (1993). Arachidonic acid in cell signaling. Curr. Opin. Cell Biol. 5, 274–280. doi: 10.1016/0955-0674(93)90116-8
Pohnert, G. (2002). Phospholipase A2 activity triggers the wound-activated chemical defense in the diatom Thalassiosira rotula. Plant Physiol. 129, 103–111. doi: 10.1104/pp.010974
Pohnert, G., and Boland, W. (2002). The oxylipin chemistry of attraction and defense in brown algae and diatoms. Nat. Prod. Rep. 19, 108–122. doi: 10.1104/pp.010974
Poirier, I., Pallud, M., Kuhn, L., Hammann, P., Demortiere, A., Jamali, A., et al. (2018). Toxicological effects of CdSe nanocrystals on the marine diatom Phaeodactylum tricornutum: the first mass spectrometry-based proteomic approach. Ecotox. Environ. Safe. 152, 78–90. doi: 10.1016/j.ecoenv.2018.01.043
Pokrzywinski, K. L., Tilney, C. L., Warner, M. E., and Coyne, K. J. (2017). Cell cycle arrest and biochemical changes accompanying cell death in harmful dinoflagellates following exposure to bacterial algicide IRI-160AA. Sci. Rep. 7:45102. doi: 10.1038/srep45102
Quijano, C., Trujillo, M., Castro, L., and Trostchansky, A. (2016). Interplay between oxidant species and energy metabolism. Redox Biol. 8, 28–42. doi: 10.1016/j.redox.2015.11.010
R Development Core Team (2008). R: A language and Environment for Statistical Computing. Vienna: R Foundation for Statistical Computing.
Ramanan, R., Kim, B. H., Cho, D. H., Oh, H. M., and Kim, H. S. (2016). Algae–bacteria interactions: evolution, ecology and emerging applications. Biotech. Adv. 34, 14–29. doi: 10.1016/j.biotechadv.2015.12.003
Rettner, J., Werner, M., Meyer, N., Werz, O., and Pohnert, G. (2018). Survey of the C20 and C22 oxylipin family in marine diatoms. Tetrahedron Lett. 59, 828–831. doi: 10.1016/j.tetlet.2018.01.057
Riemann, L., Steward, G. F., and Azam, F. (2000). Dynamics of bacterial community composition and activity during a mesocosm diatom bloom. Appl. Environ. Microb. 66, 578–587. doi: 10.1128/aem.66.2.578-587.2000
Ritchie, M. E., Phipson, B., Wu, D., Hu, Y., Law, C. W., Shi, W., et al. (2015). limma powers differential expression analyses for RNA-sequencing and microarray studies. Nucleic Acid Res. 43:e47. doi: 10.1093/nar/gkv007
Robinson, M. D., McCarthy, D. J., and Smyth, G. K. (2010). edgeR: a Bioconductor package for differential expression analysis of digital gene expression data. Bioinformatics 26, 139–140. doi: 10.1093/bioinformatics/btp616
Robinson, M. D., and Oshlack, A. (2010). A scaling normalization method for differential expression analysis of RNA-seq data. Genome Biol. 11:R25. doi: 10.1186/gb-2010-11-3-r25
Santucci, A., Bernardini, G., Braconi, D., Petricci, E., and Manetti, F. (2017). 4-hydroxyphenylpyruvate dioxygenase and its inhibition in plants and animals: small molecules as herbicides and agents for the treatment of human inherited diseases. J. Med. Chem. 60, 4101–4125. doi: 10.1021/acs.jmedchem.6b01395
Schellenberger Costa, B., Jungandreas, A., Jakob, T., Weisheit, W., Mittag, M., and Wilhelm, C. (2012). Blue light is essential for high light acclimation and photoprotection in the diatom Phaeodactylum tricornutum. J. Exp. Bot. 64, 483–493. doi: 10.1093/jxb/ers340
Seymour, J. R., Amin, S. A., Raina, J. B., and Stocker, R. (2017). Zooming in on the phycosphere: the ecological interface for phytoplankton-bacteria relationships. Nat. Microb. 2:17065. doi: 10.1038/nmicrobiol.2017.65
Sheehan, D., Meade, G., Foley, V. M., and Dowd, C. A. (2001). Structure, function and evolution of glutathione transferases: implications for classification of non-mammalian members of an ancient enzyme superfamily. Biochem. J. 360, 1–16. doi: 10.1042/bj3600001
Shi, L., and Tu, B. P. (2015). Acetyl-CoA and the regulation of metabolism: mechanisms and consequences. Curr. Opin. Cell Biol. 33, 125–131. doi: 10.1016/j.ceb.2015.02.003
Sies, H., Berndt, C., and Jones, D. P. (2017). Oxidative stress. Annu. Rev. Biochem. 86, 715–748. doi: 10.1146/annurev-biochem-061516-045037
Smith, D. J., and Underwood, G. J. (1998). Exopolymer production by intertidal epipelic diatoms. Limnol. Oceanogr. 43, 1578–1591. doi: 10.4319/lo.1998.43.7.1578
Smith, S. R., Gle, C., Abbriano, R. M., Traller, J. C., Davis, A., Trentacoste, E., et al. (2016). Transcript level coordination of carbon pathways during silicon starvation-induced lipid accumulation in the diatom Thalassiosira pseudonana. New Phytol. 210, 890–904. doi: 10.1111/nph.13843
Soneson, C., Love, M. I., and Robinson, M. D. (2015). Differential analyses for RNA-seq: transcript-level estimates improve gene-level inferences. F1000 Res. 2015:4. doi: 10.12688/f1000research.7563.2
Stock, F., Syrpas, M., Graff van Creveld, S., Backx, S., Blommaert, L., Dow, L., et al. (2019). N-acyl homoserine lactone derived tetramic acids impair photosynthesis in Phaeodactylum tricornutum. ACS Chem. Biol. 14, 198–203. doi: 10.1021/acschembio.8b01101
Supek, F., Bošnjak, M., Škunca, N., and Šmuc, T. (2011). REVIGO summarizes and visualizes long lists of gene ontology terms. PLoS One 6:e21800. doi: 10.1371/journal.pone.0021800
Tian, C. F., Garnerone, A. M., Mathieu-Demaziere, C., Masson-Boivin, C., and Batut, J. (2012). Plant-activated bacterial receptor adenylate cyclases modulate epidermal infection in the Sinorhizobium meliloti-medicago symbiosis. Proc. Natl. Acad. Sci. U.S.A. 109, 6751–6756. doi: 10.1073/pnas.1120260109
Trezzini, G. F., Horrichs, A., and Somssich, I. E. (1993). Isolation of putative defense-related genes from Arabidopsis thaliana and expression in fungal elicitor-treated cells. Plant Mol. Biol. 21, 385–389. doi: 10.1007/BF00019954
Tzin, V., and Galili, G. (2010). The biosynthetic pathways for shikimate and aromatic amino acids in Arabidopsis Thaliana. Arabidopsis book 8:e0132. doi: 10.1199/tab.0132
van Tol, H. M., Amin, S. A., and Armbrust, E. V. (2017). Ubiquitous marine bacterium inhibits diatom cell division. ISME J. 11, 31–42. doi: 10.1038/ismej.2016.112
Vandepoele, K., Van Bel, M., Richard, G., Van Landeghem, S., Verhelst, B., Moreau, H., et al. (2013). pico-PLAZA, a genome database of microbial photosynthetic eukaryotes. Environ. Microb. 15, 2147–2153. doi: 10.1111/1462-2920.12174
Wang, R., and Seyedsayamdost, M. R. (2017). Roseochelin B, an algaecidal natural product synthesized by the roseobacter Phaeobacter inhibens in response to algal sinapic acid. Org. Lett. 19, 5138–5141. doi: 10.1021/acs.orglett.7b02424
Wasternack, C. (2007). Jasmonates: an update on biosynthesis, signal transduction and action in plant stress response, growth and development. Ann. Bot. 100, 681–697. doi: 10.1093/aob/mcm079
Weber, H. (2002). Fatty acid-derived signals in plants. Trend Plant Sci. 7, 217–224. doi: 10.1146/annurev-phyto-080508-081820
Winger, J. A., Derbyshire, E. R., Lamers, M. H., Marletta, M. A., and Kuriyan, J. (2008). The crystal structure of the catalytic domain of a eukaryotic guanylate cyclase. BMC Struct. Biol. 8:42. doi: 10.1186/1472-6807-8-42
Keywords: pheromones, diatoms, bacterial exudates, cross-kingdom interactions, metabolomics, transcriptomics
Citation: Cirri E, De Decker S, Bilcke G, Werner M, Osuna-Cruz CM, De Veylder L, Vandepoele K, Werz O, Vyverman W and Pohnert G (2019) Associated Bacteria Affect Sexual Reproduction by Altering Gene Expression and Metabolic Processes in a Biofilm Inhabiting Diatom. Front. Microbiol. 10:1790. doi: 10.3389/fmicb.2019.01790
Received: 02 May 2019; Accepted: 19 July 2019;
Published: 02 August 2019.
Edited by:
Ondrej Prasil, Institute of Microbiology (ASCR), CzechiaReviewed by:
Kimberly H. Halsey, Oregon State University, United StatesCopyright © 2019 Cirri, De Decker, Bilcke, Werner, Osuna-Cruz, De Veylder, Vandepoele, Werz, Vyverman and Pohnert. This is an open-access article distributed under the terms of the Creative Commons Attribution License (CC BY). The use, distribution or reproduction in other forums is permitted, provided the original author(s) and the copyright owner(s) are credited and that the original publication in this journal is cited, in accordance with accepted academic practice. No use, distribution or reproduction is permitted which does not comply with these terms.
*Correspondence: Wim Vyverman, V2ltLlZ5dmVybWFuQFVHZW50LmJl; Georg Pohnert, Z2VvcmcucG9obmVydEB1bmktamVuYS5kZQ==
†These authors have contributed equally to this work
Disclaimer: All claims expressed in this article are solely those of the authors and do not necessarily represent those of their affiliated organizations, or those of the publisher, the editors and the reviewers. Any product that may be evaluated in this article or claim that may be made by its manufacturer is not guaranteed or endorsed by the publisher.
Research integrity at Frontiers
Learn more about the work of our research integrity team to safeguard the quality of each article we publish.