- 1Mars Global Food Safety Center, Beijing, China
- 2Department of Food Science, College of Agriculture and Life Sciences, Cornell University, Ithaca, NY, United States
The food industry is facing a major transition regarding methods for confirmation, characterization, and subtyping of Salmonella. Whole-genome sequencing (WGS) is rapidly becoming both the method of choice and the gold standard for Salmonella subtyping; however, routine use of WGS by the food industry is often not feasible due to cost constraints or the need for rapid results. To facilitate selection of subtyping methods by the food industry, we present: (i) a comparison between classical serotyping and selected widely used molecular-based subtyping methods including pulsed-field gel electrophoresis, multilocus sequence typing, and WGS (including WGS-based serovar prediction) and (ii) a scoring system to evaluate and compare Salmonella subtyping assays. This literature-based assessment supports the superior discriminatory power of WGS for source tracking and root cause elimination in food safety incident; however, circumstances in which use of other subtyping methods may be warranted were also identified. This review provides practical guidance for the food industry and presents a starting point for further comparative evaluation of Salmonella characterization and subtyping methods.
Introduction
A number of food safety incidents and recalls caused by Salmonella contamination have been associated with ready-to-eat low-moisture products (e.g., milk powder, raw almonds, dry seasonings, and peanut butter) (Pillai and Ricke, 2002; Maciorowski et al., 2004; Park et al., 2008; GMA, 2009; Hanning et al., 2009), and other food commodities (e.g., meat products, eggs, and vegetables) (Greig and Ravel, 2009; Wu et al., 2017; Ricke et al., 2018) in recent years. These cases highlight the need to reinforce Salmonella control measures in the food industry, including rapid and accurate tracking of pathogen contamination sources with appropriate subtyping tools. Tools used in incident investigations that can differentiate Salmonella beyond the species level (defined as Salmonella subtyping) are essential to improve control of this pathogen, as Salmonella contamination can occur from diverse sources at any stage of food production (Olaimat and Holley, 2012; Barco et al., 2013; Shi et al., 2015).
Conventional serotyping (White–Kauffmann–Le minor scheme) has been used as a Salmonella subtyping method for >80 years (Salmonella Subcommittee of the Nomenclature Committee of the International Society for, Microbiology, 1934; Grimont and Weill, 2007; Guibourdenche et al., 2010; Dera-Tomaszewska, 2012; Shi et al., 2015) and has been a certified approach for public health monitoring of Salmonella infections for over 50 years (CDC, 2015). This method classifies the genus Salmonella into serovars (also known as “serotypes”) based on surface antigens including somatic (O), flagellar (H), and capsular (Vi) antigens (Brenner et al., 2000). More than 2,500 serovars of Salmonella enterica, the Salmonella species responsible for virtually all salmonellosis cases have been identified by conventional serotyping (Hadjinicolaou et al., 2009; Ferrari et al., 2017), but less than 100 serovars account for the vast majority of human infections (CDC, 2015). Due to the large variety of Salmonella serovars, a laboratory needs to maintain more than 250 different high-quality typing antisera and 350 different antigens for conventional serotyping of Salmonella (McQuiston et al., 2004; Fitzgerald et al., 2006). The turnaround time (i.e., time needed from isolate submission to a laboratory to receipt of the result) for serotyping a single isolate is usually >3 days. In some cases, it can take much longer (>12 days) as multiple antibody/agglutination reactions may be needed in a step-wise fashion to assign a final classification for complex serovars (Kim et al., 2006; Boxrud, 2010). Traditional serotyping is thus time-consuming and labor intensive requiring well-trained, experienced technicians (Boxrud, 2010; Shi et al., 2015). Unfortunately, it can also be imprecise (McQuiston et al., 2011). Moreover, the low discriminatory power of conventional serotyping may result in false-positive identification of relatedness between two unrelated isolates, as strains with the same serovar (such as the serovar Salmonella Enteritidis) may originate from multiple contamination sources. Further in-depth resolution beyond the serovar level is thus required for incident investigations (Ricke, 2017; Ricke et al., 2018). Various rapid molecular-based subtyping methods have been developed to provide faster, more discriminatory, and more accurate subtyping of Salmonella thus overcoming the limitations of traditional serotyping. Nevertheless, serovar data can still provide important historical epidemiological information, as certain serovars have specific virulence characteristics or may be associated with specific contamination sources (Ricke et al., 2018). Thus, it is important to link the subtypes identified by these molecular-based methods to Salmonella serovars.
There is no current global recommendation for the application of molecular characterization methods for Salmonella, although the food industry has applied both banding pattern-based and sequence-based subtyping methods for incident investigations. This review will provide (i) a comparison between classical serotyping and selected widely used molecular-based subtyping methods including pulsed-field gel electrophoresis (PFGE), multilocus sequence typing (MLST), and whole-genome sequencing (WGS, including WGS-based serovar prediction), and (ii) a scoring system to evaluate and compare Salmonella subtyping assays.
Banding Pattern-Based and Sequencing-Based Characterization Methods for Salmonella
There are two major types of molecular-based subtyping methods: (i) nucleotide banding pattern-based subtyping methods, representing the banding patterns generated from the restriction digestion or polymerase chain reaction (PCR) amplification of genomic or plasmid DNA (Wachsmuth et al., 1991; Hartmann and West, 1997) and (ii) sequencing-based subtyping, identifying variants at the single-nucleotide level of the selected gene markers or the entire genome of an isolate. A comparison of the resolution, turnaround time, ability of serovar prediction, cost, and feasibility of these methods is given below (Table 1).
Banding Pattern-Based Characterization Methods
Pulsed-Field Gel Electrophoresis (PFGE)
Pulsed-field gel electrophoresis was first described in 1984 and developed as a subtyping method for Salmonella in the 1990s (Threlfall and Frost, 1990; Figure 1). PFGE is currently the gold standard for PulseNet International, and has been used by public health authorities and food regulators for outbreak investigations and source tracking globally (including USCDC, USFDA, USDA, and ECDC) (Zou et al., 2010; Wattiau et al., 2011; PulseNet, 2014; CDC, 2016a). Alternative methods for Salmonella subtyping are commonly compared against PFGE (Call et al., 2008). However, PulseNet is transitioning from using PFGE and multiple locus variable number of tandem repeats analysis (MLVA) toward using WGS as the standardized genotyping method for foodborne pathogens (CDC, 2017a; Nadon et al., 2017). PulseNet International has defined standard PFGE protocols (PulseNet, 2013; CDC, 2017b) and maintains a database of Salmonella PFGE profiles with >350,000 PFGE patterns representing >500 serovars. These PFGE patterns predominantly represent isolates collected since 1996 in North America and Europe (Zou et al., 2013). PFGE has relatively high concordance with epidemiological relatedness with two decades of data accumulation (CDC, 2018a). However, the PulseNet database for PFGE patterns is not publicly available and can only be accessed by PulseNet participating laboratories.
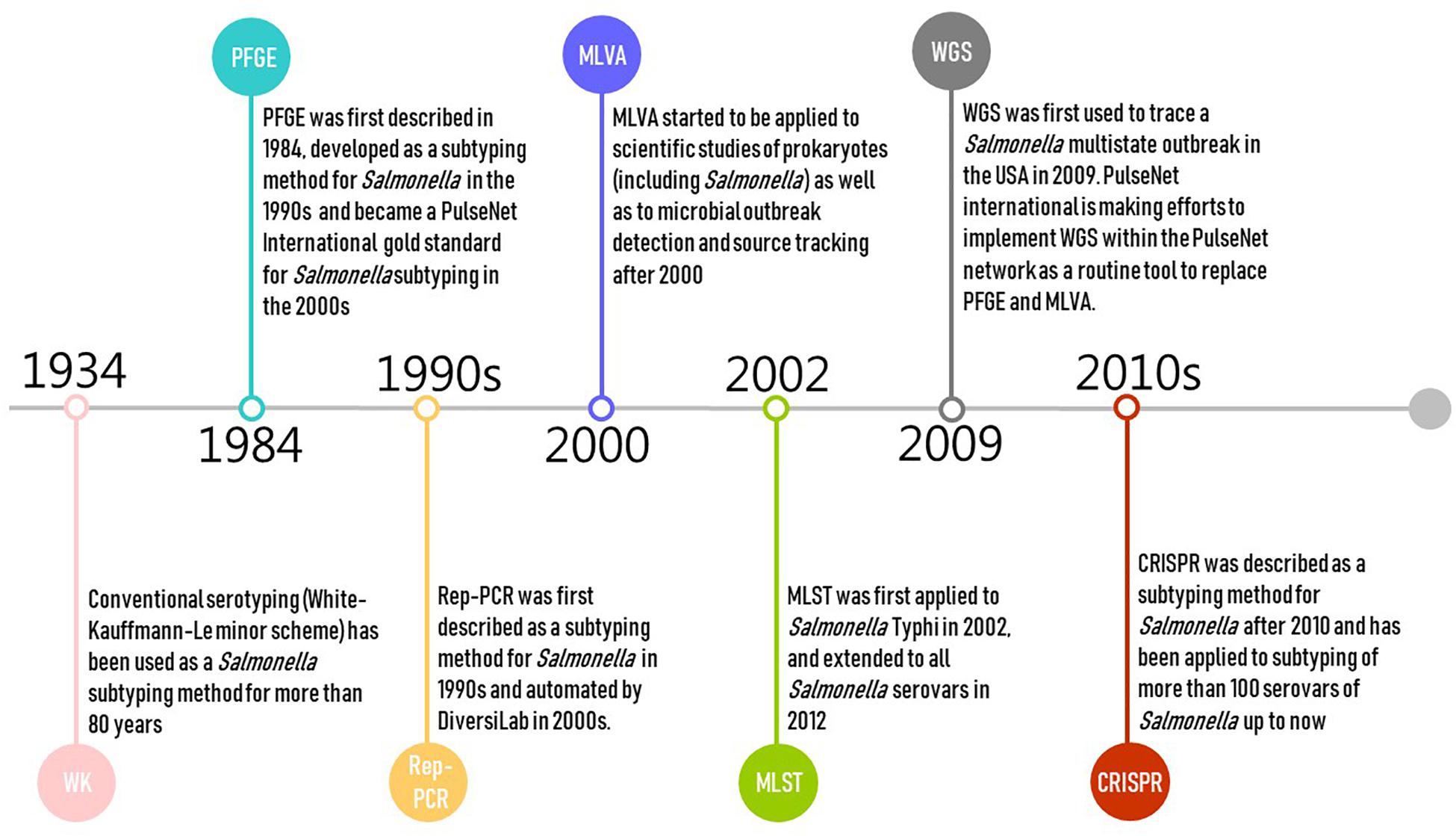
Figure 1. Timeline of the development of selected molecular subtyping and characterization methods for Salmonella (Salmonella Subcommittee of the Nomenclature Committee of the International Society for, Microbiology, 1934; Gilson et al., 1990; Threlfall and Frost, 1990; Hulton et al., 1991; Martin et al., 1992; Lindstedt et al., 2003, 2013; Healy et al., 2005; Grimont and Weill, 2007; Zou et al., 2010; Wattiau et al., 2011; PulseNet, 2014; CDC, 2016a, 2019; Nadon et al., 2017).
The PFGE approach uses restriction enzymes that recognize specific restriction sites along the genomic DNA and fragment the DNA to sizes normally ranging from 20 to 800 kb (up to 2,000 kb) (Schwartz and Cantor, 1984; Singh et al., 2006). These large fragments are separated in a flat agarose gel by constantly changing the direction of the electric current (pulsed field), which causes the DNA to separate by size, generating a specific “fingerprint pattern” for a given isolate (Foley et al., 2009). The restriction enzymes XbaI, NotI, SpeI, and SfiI have been typically used for Gram-negative bacteria including Salmonella (Barg and Goering, 1993). The primary restriction enzyme used for Salmonella PFGE is XbaI. A public health laboratory usually has access to software [e.g., BioNumerics and GelCompar (Applied Maths, Sint-Martens-Latem, Belgium); Diversity Database Fingerprinting Software (Bio-Rad Laboratories, Hercules, CA, United States)], to analyze a PFGE pattern (Nsofor, 2016) and uploads PFGE patterns to a national database. PulseNet Central’s database managers then analyze the uploaded pattern to see if a new outbreak has emerged or whether the isolate is part of an ongoing outbreak (CDC, 2018a). To make inter-laboratory comparison of DNA patterns possible, standardized protocols, molecular size standards (Salmonella Braenderup H2812, ATCC BAA-664), software, and nomenclature of PFGE patterns are required (PulseNet, 2015a). The approximate cost of the equipment and reagents required by PFGE can be accessed on the PulseNet International – PFGE site (PulseNet, 2015b).
Pulsed-field gel electrophoresis has been shown repeatedly to be more discriminatory than methods such as conventional serotyping, ribotyping, or MLST for many bacteria (Fakhr et al., 2005; Harbottle et al., 2006; Oloya et al., 2009; Soyer et al., 2010; Hauser et al., 2012). The combination of profiles generated by using additional restriction enzymes can enhance the value of this method for differentiating highly homogeneous Salmonella strains (Zheng et al., 2011); however, the cost increases as additional enzymes are used. PFGE can be used for subtyping of both Gram-positive (e.g., Listeria monocytogenes, Staphylococcus aureus) and Gram-negative (e.g., Salmonella, Escherichia coli, Shigella, Campylobacter jejuni) pathogenic bacteria. Typically, only the choice of the restriction enzyme and conditions for electrophoresis need to be optimized depending on the bacterial species investigated (PulseNet, 2015a).
Although various software platforms are available for PFGE pattern analysis, artifacts (e.g., brightly fluorescing spot) may lead to misidentification of bands. PFGE technology cannot usually be used to reliably visualize smaller fragments (e.g., <20.5 kb; Hunter et al., 2005) and has difficulty in differentiating bands differing by <5–10% in size due to the limited resolution of electrophoresis (Dijkshoorn et al., 2001; Persing et al., 2011). To address these issues, it has been recommended that users confirm PFGE pattern assignments using their experience and additional information to avoid incorrect band calling and systematic band shifts due to gel imperfections or imperfect reproducibility of electrophoretic conditions (Van Belkum et al., 2007). PFGE cannot be automated and requires high-level technical expertise and, thus, is hampered by low throughput, and may show low robustness and poor comparability of results between laboratories (Hyytia-Trees et al., 2007; Fabre et al., 2012; Kjeldsen et al., 2016).
No genetic information such as virulence potential or presence of antimicrobial resistance genes can be provided by PFGE, as the DNA fragments are separated by size rather than sequence (Ferrari et al., 2017). Observed bands of comparable size might not represent the same sequence of DNA, and a small mutation in a restriction site may result in changes in multiple bands. “Relatedness” determined by PFGE thus may not represent a true phylogenetic relationship between isolates (CDC, 2018a). Typically, multiple distinct PFGE patterns can be identified among isolates classified into the same serovar. Polyphyletic serovars, which are derived from more than one common evolutionary ancestor or ancestral group (e.g., serovars Newport, Mississippi, Saintpaul, Kentucky), show high levels of PFGE diversity (Porwollik et al., 2004; Sukhnanand et al., 2005; Alcaine et al., 2006; Harbottle et al., 2006; Sangal et al., 2010). PFGE-based prediction of these serovars is unreliable if isolates in the database are not representative of all clades of the serovar. On the other hand, PFGE may cluster epidemiologically unrelated isolates into identical PFGE types (Barco et al., 2013) and may even provide similar or identical PFGE types for isolates that represent different, but genetically very similar serovars that have a common ancestor (Barco et al., 2013; Shi et al., 2015), such as Typhimurium (antigenic formula: 1,4,[5],12:i:1,2) versus Typhimurium var. Copenhagen (antigenic formula: 1,4,12:i:1,2) (Heisig et al., 1995; Hauser et al., 2011), and Typhimurium versus 4,5,12:i:- (Guerra et al., 2000; Soyer et al., 2009; Wiedmann and Nightingale, 2009; Hoelzer et al., 2010; Ranieri et al., 2013). Furthermore, differentiation of genetically homogeneous serovars such as serovar Enteritidis challenges the usefulness of PFGE in Salmonella subtyping activities (Olson et al., 2007; Zheng et al., 2007). Approximately 45% of serovar Enteritidis isolates reported to PulseNet display the same PFGE XbaI pattern (JEGX01.0004), although many of these isolates are not epidemiologically related (Zheng et al., 2007). It is important to mention that the serovars mentioned above (i.e., Enteritidis, Typhimurium, Newport, Mississippi, Saintpaul, and Kentucky) are ranked among the most common Salmonella serovars associated with human and animal salmonellosis globally (Galanis et al., 2006; CDC, 2009).
Multiple Locus Variable Number of Tandem Repeats Analysis (MLVA)
Multiple locus variable number of tandem repeats analysis is a PCR-based typing method originating from forensic science where it is used for DNA “fingerprinting” samples of human origin. It has frequently been applied to scientific studies of prokaryotes as well as to microbial outbreak detection and source tracking (Lindstedt et al., 2003, 2013; Figure 1). MLVA is the second major genotyping tool (after PFGE) used in the PulseNet network (PulseNet, 2015c); prior to WGS, MLVA was one of the most popular subtyping methods used in public health surveillance and outbreak investigation of Salmonella, particularly in Europe (Torpdahl et al., 2007; Hopkins et al., 2011; Barco et al., 2013; Bauer et al., 2013; Lindstedt et al., 2013; Mughini-Gras et al., 2018). MLVA is usually performed following serotyping or PFGE for routine surveillance as a complementary technique for Salmonella subtyping (Torpdahl et al., 2007; Lienemann et al., 2015; Kjeldsen et al., 2016; CDC, 2017c; Ferrari et al., 2017), as it is challenging for PFGE to further differentiate isolates of genetically homogeneous serovars such as Salmonella Enteritidis (Kjeldsen et al., 2016). MVLA is especially used for typing Salmonella Typhimurium and Salmonella Enteritidis strains in reference or regulatory laboratories in Denmark, France, Germany, and United States [e.g., CDC, USDA – Food Safety and Inspection Service (FSIS) laboratories] (Barco et al., 2013; Bauer et al., 2013).
Multiple locus variable number of tandem repeats analysis is serovar specific, thus different Salmonella serovars usually require different MLVA schemes (Kruy et al., 2011). The first step toward uniform standardization of the MLVA profiles was collectively taken by PulseNet International and ECDC in defining the standard protocols of MLVA for Salmonella Typhimurium and Salmonella Enteritidis (ECDC, 2011, 2016b; PulseNet, 2015c). These serovars account for 26% of the culture-confirmed human Salmonella infections reported by US Laboratory-based Enteric Disease Surveillance (LEDS) and >60% of the salmonellosis cases reported by ECDC (ECDC, 2016a; Kjeldsen et al., 2016; CDC, 2018b). This uniform standardization of the MLVA profiles allowed direct comparison between laboratories irrespective of the platform used for MLVA (Larsson et al., 2009). Validated MLVA standard protocols for additional Salmonella serovars of clinical importance worldwide are largely missing, making MLVA use for serovars other than Enteritidis and Typhimurium difficult. However, with the advent of and transition into WGS, further development of MLVA may not occur.
Multiple locus variable number of tandem repeats analysis assesses the variation in the number of tandem repeated DNA sequences referred to as “variable-number tandem repeats” (VNTRs) in multiple regions of the bacterial genome to characterize bacterial isolates. The number of VNTRs in a given locus may vary between different microorganisms and even among bacterial isolates of the same species and serovar (Lindstedt et al., 2003; Torpdahl et al., 2007; Ngoi et al., 2015). The VNTR profiles vary in length between a few base pairs long to over 100 base pairs, enabling the development of techniques that utilize variation in the size of VNTR to discriminate closely related isolates (Lindstedt et al., 2003; Torpdahl et al., 2007; Fabre et al., 2012). The improved discriminatory power of MLVA varies with the serovar and phage type investigated (Torpdahl et al., 2007; Lienemann et al., 2015); e.g., in a study in Denmark, MLVA could differentiate distinct clusters within the most common phage types of Salmonella Typhimurium such as DT104, DT120, and DT12 even though these isolates displayed comparable PFGE patterns (Torpdahl et al., 2007). Public health laboratories usually have access to software (e.g., BioNumerics, GeneMapper, the free Peak Scanner) for analysis of MLVA patterns (ECDC, 2011; PulseNet, 2015c). Minimum spanning trees are frequently applied to MLVA profiles, yielding maps of predicted relationships among isolates based on single-locus and dual-locus variants (Van Belkum et al., 2007). However, web-accessible MLVA databases are not widely used for international collaboration (Guigon et al., 2008).
Multiple locus variable number of tandem repeats analysis is cheaper, faster, simpler to execute, and shows a relatively high-throughput compared with other molecular methods (Torpdahl et al., 2005, 2007; Lindstedt et al., 2007, 2013; Hopkins et al., 2011; Kruy et al., 2011). MLVA is less labor-intensive, time-consuming, and it is easier to perform than PFGE and MLST, as the protocol requires only a regular PCR step followed by capillary electrophoresis (Torpdahl et al., 2007; Lindstedt et al., 2013). Reduced handling time of pathogenic bacteria is beneficial for large-scale investigations. MLVA is also suitable for automation using a pipetting robot work station, automated sequencer, and analytical software (Barco et al., 2013; Lindstedt et al., 2013; Ferrari et al., 2017). Moreover, MLVA demonstrates good international repeatability and reproducibility for specific serovars such as Salmonella Typhimurium and Salmonella Enteritidis (Larsson et al., 2013). The data generated by MLVA can be readily analyzed and standardized for inter-laboratory comparisons (Torpdahl et al., 2007; Hopkins et al., 2011; Lindstedt et al., 2013; Wuyts et al., 2013).
A major drawback of MLVA for Salmonella subtyping is that the most effective MLVA protocols described so far are serovar-specific (Barco et al., 2013; Ngoi et al., 2015; Kjeldsen et al., 2016); hence, isolates have to be serotyped prior to selecting a specific MLVA scheme for further subtyping (Kjeldsen et al., 2016). At least 27 MLVA schemes have been developed to subtype different Salmonella serovars, whereas only Salmonella Typhimurium and Salmonella Enteritidis MLVA assays have been standardized in Europe and in the PulseNet network (PulseNet, 2015c; Kjeldsen et al., 2016). Another drawback is that rapid evolution of the target loci may decrease the reliability of results provided by MLVA regarding the relationship between strains under investigation (Hopkins et al., 2007, 2011; Lindstedt et al., 2013). This might hamper the use of MLVA, particularly in long-term epidemiological studies (Lindstedt, 2005; Li et al., 2009).
Repetitive Element PCR (Rep-PCR)
Repetitive element PCR targets the repetitive elements of genomic DNA to discriminate bacterial isolates. This method has been developed using three families of repeat sequences for subtyping Salmonella, including “enterobacterial repetitive intergenic consensus” (ERIC) sequences, “the repetitive extragenic palindromic” (REP) sequences, and the “BOX” sequences (Gilson et al., 1990; Hulton et al., 1991; Martin et al., 1992). The PCR products amplified from genome regions containing these repetitive elements are analyzed by agarose gel electrophoresis, and the banding patterns generated are used to investigate the genetic relatedness between bacterial isolates (Sabat et al., 2013). The DiversiLab system (bioMérieux, Marcy-l’Etoile, France) automated the whole process of the Rep-PCR subtyping approach after 2000 and has been used for subtyping pathogens in hospitals worldwide (Healy et al., 2005; Chenu et al., 2012; Sabat et al., 2013; Figure 1). As the low reproducibility of original Rep-PCR method may have resulted from variability in reagents and gel electrophoresis systems (Sabat et al., 2013), the application of the DiversiLab system with microfluidic capillary electrophoresis increased both the resolution and reproducibility of the Rep-PCR approach (Healy et al., 2005; Chenu et al., 2012; Sabat et al., 2013). However, the system has been discontinued, making Rep-PCR unavailable as a commercial platform.
The major advantages of this method include its relatively low cost (comparable to that of PFGE) and short turnaround time (within one day) (Sabat et al., 2013; Ngoi et al., 2015). However, the discriminatory power of Rep-PCR in subtyping Salmonella is reportedly lower than that of PFGE (Tiong et al., 2010; Thong and Ang, 2011; Elemfareji and Thong, 2013; Ngoi et al., 2015). Its relatively low reproducibility (which can at least be partially addressed by automation, such as in the DiversiLab system), and low accuracy of serovar prediction (Weigel et al., 2004; Wise et al., 2009) have limited its application in Salmonella subtyping.
Sequencing-Based Characterization Methods
Legacy Multilocus Sequence Typing (Legacy MLST)
Multilocus sequence typing is a nucleotide sequence-based approach that assesses DNA sequence variations (i.e., allelic type) of typically three, four, or seven selected well-conserved, housekeeping genes, usually using Sanger sequencing technology (Liu, 2010; Achtman et al., 2012). Schemes targeting seven genes are typically considered the “classical” MLST approach; this typing approach was originally proposed for isolates of Neisseria meningitidis (Liu, 2010). In this review, we focus on the most widely used Salmonella scheme targeting seven housekeeping genes [aroC, dnaN, hemD, hisD, thrA, sucA, and purE; hereafter denoted as legacy MLST to distinguish newer approaches (described below)] (Li et al., 2009; Yun et al., 2015). It was first introduced for Salmonella Typhi in 2002 (Kidgell et al., 2002), and extended to all Salmonella serovars in 2012 (Achtman et al., 2012; Figure 1). Legacy MLST is mainly used in research studies, assessing the population genetics and evolution of Salmonella. Public Health England (PHE) started adopting the seven-gene MLST (based on WGS data) approach as a replacement for traditional serotyping in 2015 (Ashton et al., 2016).
Historical MLST data including legacy MLST sequence types are maintained on EnteroBase (Alikhan et al., 2018). As of November 2017, the number of legacy MLST sequence types for Salmonella has reached 3,929 (Alikhan et al., 2018). Legacy MLST analysis can be conducted online by entering the sequences of amplified genes. Allelic variation at each locus is cataloged and a sequence type is assigned by comparing the allele set. The strains are characterized by their unique sequence type. With the advent of next-generation-sequencing technologies, legacy MLST data can also be extracted directly from WGS data using bioinformatics pipelines (Achtman et al., 2012; Ashton et al., 2016). The relatedness of isolates subtyped by legacy MLST can be displayed as a dendrogram or a minimum spanning tree based on the matrix of pairwise differences between their allelic profiles (Francisco et al., 2009), or as a phylogenetic tree built directly from the nucleotide alignment of the seven genes.
Legacy MLST can deliver results more rapidly than PFGE (Shi et al., 2015; Yun et al., 2015; Table 1), and the publicly available databases and online query system enable legacy MLST results to be highly reproducible and exchangeable between laboratories. However, legacy MLST shows lower discriminatory power than PFGE and MLVA, which limits its application to further discriminate isolates within a given serovar (Torpdahl et al., 2005; Alcaine et al., 2006; Foley et al., 2006; Harbottle et al., 2006; Hauser et al., 2012; Ngoi et al., 2015), and for source attribution (Barco et al., 2013). Protocols targeting sequences in genes that change more rapidly than housekeeping genes have been developed to improve the discriminatory power of legacy MLST (Ross and Heuzenroeder, 2005, 2008).
Clustered Regularly Interspaced Short Palindromic Repeat-Based Subtyping (CRISPR-Based Subtyping)
The clustered regularly interspaced short palindromic repeat (CRISPR) typing method uses the diversity of the content of CRISPR loci to distinguish bacterial strains. The application of the CRISPR system for subtyping foodborne pathogens is discussed in detail elsewhere (Shariat and Dudley, 2014; Shi et al., 2015; Barrangou and Dudley, 2016; Ferrari et al., 2017; Ricke et al., 2018). Although the CRISPR system has been applied to the subtyping of at least 100 Salmonella serovars (Shariat and Dudley, 2014; Barrangou and Dudley, 2016), this approach is not widely used by public health authorities or food regulators (Ferrari et al., 2017).
Clustered regularly interspaced short palindromic repeat loci contain variable lengths of CRISPR spacers obtained from foreign nucleic acids of plasmids or bacteriophages (Shariat and Dudley, 2014; Wright et al., 2017). These CRISPR spacers are acquired or lost during evolution of the pathogen over time in a sequential manner (Ricke et al., 2018), thus constructing a unique set of DNA sequence patterns that may provide sufficient resolution for pathogen subtyping (Fricke et al., 2011; Barrangou and Horvath, 2012; Shariat and Dudley, 2014; Wright et al., 2017). For subtyping, amplified CRISPR loci PCR products are sequenced by Sanger sequencing technology (Liu et al., 2011). The CRISPR spacer sequences are analyzed to assign each locus with an allelic type. The combination of the allelic types of analyzed CRISPR loci determine the isolate’s allelic profile (also referred to as the isolate’s sequence type) and is used to investigate the relationships between isolates (Liu et al., 2011).
The CRISPR approach has been shown to be feasible for subtyping of Salmonella (Liu et al., 2011; Fabre et al., 2012; DiMarzio et al., 2013; Shariat et al., 2013a, b, c; Almeida et al., 2017). Liu et al. (2011) developed a CRISPR–multi-virulence-locus sequence typing (MVLST) approach using virulence genes sseL and fimH with CRISPR1 and CRISPR2 loci; this approach was used to compare 171 isolates representing nine serovars (Typhimurium, Enteritidis, Newport, Heidelberg, Javiana, I 4,[5],12:i:-, Montevideo, Muenchen, Saintpaul) and was reported to be able to subtype Salmonella with resolution at the outbreak level. CRISPR–MVLST using different schemes of virulence genes has also been applied by others for subtyping Salmonella (DiMarzio et al., 2013; Shariat et al., 2013a; Almeida et al., 2017). The results from these studies suggest that CRISPR–MVLST has a higher discriminatory power than legacy MLST (Ferrari et al., 2017); however, discrimination is lower than PFGE in some cases (Almeida et al., 2015). While CRISPR typing has a relatively short turnaround time (comparable to MLST), current major drawbacks include high cost (Almeida et al., 2017; Ferrari et al., 2017), unstandardized protocol, and database, as well as limited research on the concordance between the diversity of Salmonella isolates reflected by CRISPR loci content and by the other standard subtyping methods (Shi et al., 2015).
Whole-Genome Sequencing (WGS)
Whole-genome sequencing captures DNA sequence changes across the entire genome of single microbial isolates. The data are useful to assess evolution, allowing accurate description of the genetic relatedness of isolates. The use of WGS for Salmonella subtyping in outbreak investigation and pathogen source tracking has proven effective by a rapidly increasing number of studies (den Bakker et al., 2011, 2014; Allard et al., 2012; Leekitcharoenphon et al., 2014; Deng et al., 2015; Taylor et al., 2015; Hoffmann et al., 2016; Inns et al., 2016). WGS was first used to trace a Salmonella multistate outbreak in the United States in 2009 (CDC, 2019), and has been used for pathogen subtyping by the public health surveillance systems in the United States (Allard et al., 2018), Canada (Vincent et al., 2018), the United Kingdom (Ashton et al., 2016), Denmark (Kvistholm Jensen et al., 2016), and France (Moura et al., 2016). PulseNet international is also making efforts to implement WGS within the PulseNet network as a routine tool to replace PFGE and MLVA (Nadon et al., 2017; Figure 1). Both PHE (Ashton et al., 2016) and the US FDA (2018) have started using “real-time” WGS to subtype Salmonella isolates. CDC is also using WGS in state laboratories for Salmonella outbreak investigations (CDC, 2016b). WGS will be used increasingly for contamination incident investigations in the food industry, particularly as cost continues to shrink and ease of use increases. WGS (as well as other sequencing approaches that use the same next-generation sequencing technologies used for WGS) also have a number of additional applications in the food industry, which will further drive implementation of these tools. Examples of other applications include (i) monitoring ingredient supplies, (ii) identification of microbial persistence in processing environments, and (iii) prediction of antimicrobial resistance (including in Salmonella) and other relevant phenotypes, facilitating the improvement of sanitary management, microbial hazard control, and microbiological risk assessment (Allard et al., 2018; Rantsiou et al., 2018; Ricke et al., 2018).
Sequenced Salmonella genomes can be deposited and made publicly available on the National Center for Biotechnology Information site1, the European Bioinformatics Institute site2, or the DNA Data Bank of Japan site3 with data shared between all three (Kodama et al., 2012; Jagadeesan et al., 2019). NCBI provides phylogenetic tree-based clustering of all publicly available sequence data at the NCBI pathogen detection site4. These phylogenetic trees show the closest matches to any newly submitted data (Allard et al., 2018). NCBI also houses the data using GenomeTrakr Network (FDA, 2018). This was developed by the US FDA and NCBI as the first distributed network of laboratories to utilize WGS, with both genomic and geographic data, for foodborne pathogen characterization. This network includes the WGS laboratories of the CDC and USDA (Allard et al., 2016; Jackson et al., 2016). As of February 2019, there are over 184,000 genome sequences or raw sequencing data of S. enterica available on NCBI. WGS data analysis can also be performed off-line without using any public databases, an approach that may sometimes be preferred by industry.
Sequencing platforms that can be used currently for WGS include Illumina, Ion Torrent, Oxford Nanopore Technologies, and Pacific Biosciences (PacBio). Procedures to validate the complete workflow for S. enterica WGS with Illumina (MiSeq and HiSeq) and PacBio platforms from subculture of isolates to bioinformatics analysis have been reported by Portmann et al. (2018). The Illumina sequencing system is one of the most widely used sequencing platforms; it produces DNA-sequence reads with the length of 50–300 bp using sequencing-by-synthesis (SBS). This process uses fragmented DNA templates to detect single bases as they are incorporated during a DNA replication reaction on a solid surface flow cell (Illumina (2019)). For applications including comparative genomics and phylogeny, these short reads of DNA sequences can be aligned to a reference genome or de novo assembled into longer sequences called contigs (Loman and Pallen, 2015). The large amount of data generated by WGS combined with a complex data analysis process generally requires expertise in bioinformatics to deploy and run (Wyres et al., 2014; Deurenberg et al., 2017). Software with a more user-friendly interface, such as CLC Genomics Workbench5, BioNumerics, and Geneious (Biomatters, New Zealand), however, is available, including for industry users with limited bioinformatics expertise and an increasing number of user-friendly bioinformatics tools are being developed.
The rapid growth of WGS data in the publicly available databases allows industry to compare isolates with global entries of pathogen sequences used by food regulators and public health authorities (Allard et al., 2018; Rantsiou et al., 2018). Despite increasing availability of data analysis software, it is still challenging to generate consistent analytical reports due to the lack of standardized approaches to data analysis and interpretation (Clooney et al., 2016); for example, even with a standard software, choice of reference genomes can have considerable effects on the data analyses (Pightling et al., 2014). Furthermore, there are currently no clearly outlined safeguards to protect companies from regulatory action if shared WGS data show a relationship between pathogen isolates identified by a company and an outbreak isolate. Development of a mechanism for sharing data through anonymous hubs may allay concerns on confidentiality and encourage data sharing (FAO, 2016). This mechanism may also enable more effective data capture and analysis for monitoring trends and identifying related incidents.
The current cost of the entire WGS process, including DNA library preparation, sequencing, data analysis, and storage, is relatively high compared with the other molecular-based subtyping methods. The cost difference is more apparent when a small number of isolates are sequenced (as could be typical for the food industry). The cost of maintaining data analysis tools and bioinformatics personnel needs to be taken into consideration (Leekitcharoenphon et al., 2014; Ferrari et al., 2017; Nadon et al., 2017).
WGS-Analysis Procedures
Interpretation of WGS data for source tracking or outbreak investigation typically uses two approaches to represent results: (i) single-nucleotide polymorphism (SNP) or allelic differences (often presented as distance matrix tables), and (ii) phylogeny or clustering of the isolates. SNP or allelic differences show objectively the genetic distance between two isolates. Hence, if isolate A shows three SNPs or allelic differences to isolate B, and 26 SNPs or allelic differences to isolate C, then we can say that isolate A is more similar to isolate B than to isolate C. If one assumes that all three isolates evolved at the same rate, then we can say that isolates A and B are evolutionarily more closely related to each other than they are to C. However, this assumption (i.e., all isolates evolve at the same rate) may not always be true. Environmental conditions or mutations in the DNA repair system may influence the rate of genetic change accumulated in a genome; e.g., a Salmonella isolate persisting in a humid, nutritious environment such as in a chicken farm may multiply much faster than an isolate persisting in a dry food processing environment. This environmental difference will allow the “chicken farm” isolate to accumulate more mutations (per year or any other time unit) than the dry food processing environment isolate, because the “chicken farm” isolate will multiply more times during the same period than the dry food processing environment isolate. Moreover, mutations in genes involved in DNA repair may result in the so-called “mutator phenotypes” (also sometime referred to as “hypermutators”). Mutator isolates accumulate mutations at a higher rate than non-mutator isolates (Muteeb and Sen, 2010). Hence, analyzing the number of SNP or allelic differences alone may result in misinterpretation of the results if the assumption that isolates evolved at the same rate does not hold true. Phylogenetic or clustering analyses are thus better suited to an investigation, as these analyses group isolates by their similarities instead of their differences (Pightling et al., 2018). To infer the evolutionary relationship of the isolates within a data set, therefore, a phylogeny must be constructed. For more detailed and technical information on reconstructing bacterial phylogenies from WGS data, the reader is referred to two in-depth reviews on this subject (Collins and Xavier, 2017; Patané et al., 2018).
WGS Analysis Approaches for Serotyping
Genetic-based approaches have been developed for in silico determination of serovars, because the phenotypic determination of Salmonella serovars is costly, time-consuming, and labor-intensive. These in silico methods have relied on two main approaches: (i) indirect determination using genetic markers associated with particular serovars and (ii) direct determination using genes responsible for the expression of the somatic O (rfb gene cluster) and flagellar H (fljB and fliC) antigens. The latter method has the advantage of relying on the same genetic information that results in the phenotype assessed by traditional serotyping, while the former method may require validation for new described serovars. These two approaches can also be combined for more reliable serovar prediction.
With the advent of whole-genome sequencing (WGS), in silico direct serovar determination has become the most used approach, and at least two Salmonella serovar databases and programs have been routinely used for in silico serotyping of Salmonella: SeqSero (Zhang et al., 2015) and SISTR (Yoshida et al., 2016a). SeqSero uses a database of 473 alleles representing 56 fliC antigenic types and 190 alleles representing 18 fljB antigenic types in a combined H-antigen database (Zhang et al., 2015). The somatic O-antigen database associated with SeqSero consists of 46 rfb gene cluster sequences corresponding to the 46 O-antigens identified in Salmonella (Zhang et al., 2015). The rfb database was specifically designed to be used with genome assemblies (as opposed to raw sequencing reads). A third database was specifically built for determination of the somatic O-antigen using raw sequencing reads (as opposed to genome assemblies). This third database consists of the genes wzx (encoding the O-antigen flipase), wzy (encoding the O-antigen polymerase), and other targets, all of which are found within the rfb gene cluster. In total, the authors claimed that the SeqSero scheme can theoretically identify 2,389 of the 2,577 serovars that were described in the White–Kauffmann–Le minor scheme by the end of 2014 (Zhang et al., 2015). The inability to predict 188 serovars is due to the absence of the DNA sequences for the antigen-encoding genes corresponding to these serovars in the SeqSero database. Empirical data showed that the SeqSero database has an accuracy of 91.5–92.6% for serotype prediction (Zhang et al., 2015).
SISTR is a platform for in silico analysis of Salmonella draft genome assemblies. SISTR includes the Salmonella Genoserotyping Array (SGSA) tool among other resources. SGSA relies on the allelic differences found within the rfb gene cluster for determination of 18 of the 46 somatic O-antigens, and fljB and fliC for determination of 41 flagellar H antigens (Yoshida et al., 2014). SGSA targets the identification of 90% (n = 2,190) of Salmonella serovars. When serovar determination using genoserotyping is not possible or is incomplete, SISTR also has the option to use the core genome MLST (cgMLST) scheme to infer the serovar based on phylogenetic context. The accuracy of SISTR in predicting Salmonella serovars has been assessed to be close to 95% (Yoshida et al., 2016a, b; Robertson et al., 2018).
Since SISTR can use genoserotyping and the cgMLST scheme to infer the serovar, higher confidence should be attributed to assignments where both genoserotyping and cgMLST agree on the serovar designation. Moderate confidence should be attributed to serovar assignments when only cgMLST is able to identify the serovar. When neither the genoserotyping nor cgMLST can identify the serovar, SeqSero may be used and may allow for serovar prediction.
WGS Analyses for Subtype Characterization
Overview of WGS data analysis approaches
Different approaches can be used for analysis of WGS data for subtyping characterization related to source incident tracking. The most common approaches are based on (i) high-quality single-nucleotide polymorphism (hqSNP) identification and pairwise comparison of hqSNP differences, or (ii) whole-genome (wg)/cgMLST typing using pre-defined schemes (i.e., databases) containing allelic differences for either the pan (wg) or core (cg) genomes of Salmonella and subsequent pairwise comparison for assessing the number of allelic differences.
High-quality SNP analyses
High-quality SNP analyses rely on identification of SNP differences across a set of closely related isolates using raw sequence data, which are mapped to a closed or draft genome assembly (also referred to as the “reference genome”). Only SNPs that have been vertically transferred from an ancestral isolate to the current isolates are subject to the hqSNP analysis, while SNPs that were supposedly horizontally transferred are filtered out from the results. The reference can be a closely related genome outside the dataset, or a genome within the dataset. The analysis consists of two main steps: (i) mapping the raw sequence reads against the reference genome and (ii) SNP calling using stringent criteria to prevent the misidentification of sequencing errors or misaligned regions as SNPs (Davis et al., 2015; Katz et al., 2017). The choice of a closely related reference has been shown to be a key step in the analysis. Reference genomes that are not closely related to the set of isolates under investigation may result in underestimation of the number of SNPs, due to specific regions of the genome that may be present in the dataset under investigation, but that are missing in the reference genome (Pightling et al., 2014). There are at least two publicly available approaches that have been commonly used for hqSNP analysis: (i) the US FDA CFSAN (The Center for Food Safety and Applied Nutrition) SNP pipeline (Davis et al., 2015) and (ii) the US CDC-developed Lyve-SET hqSNP pipeline (Katz et al., 2017). These two pipelines rely on publicly available software to carry out the mapping and SNP calling steps and offer similar results despite some methodological differences, including different criteria for filtering out low-quality SNPs and masking regions supposedly acquired through horizontal gene transfer.
High-quality SNP analysis has been applied in several outbreak investigations in the United States, Canada, and some European countries, including a Salmonella Enteritidis outbreak in the United Kingdom that was linked to a German egg producer (Inns et al., 2015). Historical Salmonella Typhimurium isolates from humans and foods involved in five outbreaks and consisting of five distinct MLVA subtypes were re-analyzed using hqSNP analysis by Octavia et al. (2015); in this study at least 11 isolates not previously linked to the outbreaks were ruled in based on less than two SNP differences to the isolates previously linked to the outbreaks. Another retrospective study used hqSNP to analyze a collection of 55 Salmonella Enteritidis from seven epidemiologically characterized outbreaks and sporadic cases. One isolate not previously linked to any outbreak (i.e., sporadic) was identified to be part of one outbreak (“ruled in”) (Taylor et al., 2015). An investigation into a multi-state outbreak caused by Salmonella Poona was carried out in 2015 using PFGE and hqSNP analysis. Analysis by PFGE demonstrated three different patterns. However, WGS results showed that isolates with different PFGE patterns were genetically linked with less than six SNP differences (Kozyreva et al., 2016). Subtyping of Salmonella Dublin with PFGE was shown to have limited value in a recent outbreak investigation due to its low discriminatory power for this Salmonella serovar (Mohammed et al., 2016). The nine clinical isolates associated with the outbreak were indistinguishable by PFGE, but they were also indistinguishable from other unrelated Salmonella Dublin isolates. The nine isolates linked to the outbreak clustered together with one to nine SNP differences when analyzed using hqSNP, and they could be distinguished from other isolates that shared the same PFGE pattern with epidemiologically unrelated isolates showing more than 50 SNP differences when compared to the outbreak isolates (Mohammed et al., 2016). These studies show that public health agencies are increasingly relying on hqSNP analysis for outbreak investigation, including tracking the source of outbreaks. High-quality SNP analysis clearly improves subtype accuracy and outbreak investigations by not only allowing for increased discriminatory power, but also reducing instances where closely related isolates are being classified as “different.”
wgMLST
Whole-genome MLST (wgMLST) analysis relies on the comparison of individual genomes against a database containing all known alleles for all the genes representing the pan genome of a defined group of strains (i.e., serovar, subspecies, species, and genus). The pan genome is defined as all the genes present in at least one genome from a defined group. Two main approaches can be used, and these are often used in combination: (i) assembly free mapping and (ii) assembly based mapping. Raw sequencing reads are directly mapped against the database in an assembly free approach. Hence, this approach does not require de novo assembly of the genome prior to its utilization. SRST2 (Inouye et al., 2014) and BWA-MEM (Li, 2013) are the most commonly used programs to carry out this task. Because this approach deals directly with the raw sequence reads, it allows filtering low-quality reads or specific nucleotides with low quality within a good-quality read. In an assembly based approach the raw sequence reads are first used to generate a high-quality draft genome (i.e., usually not a closed genome) using a genome assembler. Later, the draft genome (i.e., assembly) is used to find matches against the database. The program most commonly used to map the draft genome against the database is BLASTN (Altschul et al., 1990), although other options also exist. Independently from the approach used (i.e., assembly free or assembly based), the result of mapping a genome against a database is a list of the alleles found in the analyzed genome. When more than one genome is analyzed, the list of alleles from each genome can be compared and the number of allele differences can be computed. Assembly free and assembly based wgMLST allele assignment should match for high confidence. Results are often shown as a distance matrix of allele differences and a dendrogram constructed from this distance matrix. The wgMLST methods allow for comparison of non-closely related isolates from different groups since all genomes are compared against the same database, which is a great advantage of this method over hqSNP (Maiden et al., 2013; Nadon et al., 2017). A disadvantage of the method is that the database must be constructed and shared across different groups, who must agree in using the same database in order to make their results comparable (Nadon et al., 2017). Construction of such databases is also time-consuming and labor-intensive, with the difficulty increasing with the diversity of the organisms included in the same database (e.g., a database for S. enterica subspecies enterica serovar Agona will require less time and labor than a database for all S. enterica).
Core genome MLST (cgMLST)
The cgMLST method is very similar to the wgMLST method. The major difference is the size and nature of the database. While the wgMLST database contains alleles for all genes in the pan genome of the defined group, the cgMLST only contains alleles for those genes that are present in all (or almost all) genomes of the defined group (i.e., the “core genome”). Hence, a cgMLST database will not capture the genetic diversity present in the accessory genes (i.e., genes that are not present in all isolates) and hence tends to be much smaller than a corresponding wgMLST database. The advantages of using the cgMLST are: (i) speed; because the cgMLST database is smaller than the wgMLST database, results can be obtained faster, and (ii) construction of the cgMLST database is generally easier than the wgMLST database, as typically less genomes are needed to identify the core genome than the pan genome of a group (den Bakker et al., 2010). While allele code schemes are used by some groups to summarize the differences observed among isolates subtyped by both cgMLST and wgMLST (Nadon et al., 2017), it generally is easier to define standard, stable, cgMLST allele codes. This allele code scheme can be easily transferred in a spreadsheet and can be interpreted similarly to what has been in use for PFGE. An allele code scheme may not, however, be fully stable and may need to be revised as new cg- or wgMLST types are identified (Nadon et al., 2017). A disadvantage of cgMLST is that it may show reduced discriminatory power over wgMLST, as shown in a comparison between the Salmonella cgMLST and wgMLST schemes defined in EnteroBase (Alikhan et al., 2018), carried out using Salmonella Enteritidis historical isolates from a UK egg-associated outbreak (Inns et al., 2015), as well as closely related non-outbreak isolates identified previously (Dallman et al., 2016). The 177 isolates from this dataset resulted in 177 unique sequence types by wgMLST (Simpson’s diversity index = 1.00) and 137 unique sequence types by cgMLST (Simpson’s diversity index = 0.98) (P < 0.05), showing the superior discriminatory power of wgMLST over cgMLST. However, both approaches grouped the isolates into identical clusters (Pearce et al., 2018).
Comparison of hqSNP-based analysis and genomic MLST analysis
Theoretically, hqSNP analysis is the most discriminatory approach for molecular subtyping, as it investigates all possible SNPs between each pair of isolates in the dataset. The second most discriminatory approach is wgMLST, which is designed to investigate virtually all genes in the genomes; intergenic regions and genes not present in the wgMLST scheme will not be investigated and polymorphisms present in these regions will be missed. The cgMLST approach is the least discriminatory of the three as it relies on only a subset of the genes present in the wgMLST scheme. Hence, similarly to the wgMLST approach, polymorphisms present in intergenic regions and in genes not included in the cgMLST scheme will not be assessed (Chen et al., 2017). Both wgMLST and cgMLST are reference-independent which makes the results more reproducible and transferable than hqSNP analysis (Nadon et al., 2017). In order to reproduce the results obtained from hqSNP analysis, one needs to use the same reference and parameters that were used in the original analysis (Nadon et al., 2017). This is not an issue with wgMLST or cgMLST analysis as long as analyses use the same scheme containing the same genes and alleles to allow for comparisons. Transference and communication of the results also seem to be more complicated for hqSNP analysis than for cgMLST or wgMLST (Nadon et al., 2017). This is because hqSNP analysis, as compared to cgMLST or wgMLST analyses, requires more parameter settings, which must be communicated for better interpretation. wgMLST and cgMLST analyses are also typically integrated into commercially available software, while the hqSNP pipelines are available as free open software or integrated into commercial software. Free-of-charge hqSNP pipelines require UNIX-based systems and are run through the command line, which may require specialized expertise (Nadon et al., 2017). Commercially available software, which can run cgMLST and wgMLST (e.g., BioNumerics) tends to be more user-friendly. BioNumerics uses a graphical user interface and can be installed in Microsoft Windows computers. The hqSNP analysis can easily be kept private as the analysis can be run within a closed dataset of genomes. The cgMLST and wgMLST can also be kept private; however, it may require some additional infrastructure (i.e., a private cloud) to be built around the commercial software.
Comparison of Molecular Methods for Predicting the Serovar of Salmonella
A comparison of different molecular methods for predicting the serovar of Salmonella is shown Table 2. Acceptable correlation between PFGE patterns and serovars has been described by several researchers (Weigel et al., 2004; Nde et al., 2006; Gaul et al., 2007; Kerouanton et al., 2007; Zou et al., 2010; Shi et al., 2015; Bopp et al., 2016). Shi et al. (2015) summarized the serovar-prediction accuracy of different molecular serotyping methods with studies from 1993 to 2013. The proportion of isolates that may not be accurately serotyped with PFGE is generally comparable to the proportion that is not typeable, or that requires extensive additional labor and reagents using conventional serotyping (Bopp et al., 2016). Examples of serovars incorrectly predicted by PFGE are summarized below (Table 3). Overall, with PFGE patterns for approx. 500 Salmonella serovars in the PFGE pattern database (Ranieri et al., 2013; Shi et al., 2015) and the reported good correlation between PFGE patterns and serovars, PFGE-based serovar prediction should be possible for a large proportion of these serovars, but will not be possible for a large number of less common serovars not represented in the database.
Multiple locus variable number of tandem repeats analysis is not widely used for serovar prediction even though efforts have been made to develop MLVA subtyping schemes to subtype multiple serovars of Salmonella with one protocol (Van Cuyck et al., 2011; Kjeldsen et al., 2016). A universal MLVA scheme for most frequently isolated Salmonella serovars (accounting for 80% of the clinical isolates from humans in Europe) has been developed by Kjeldsen et al. (2016). In another study, an MLVA scheme identified 31 serovars (Van Cuyck et al., 2011). Nevertheless, further development of multiple-serovar MLVA schemes and robust MLVA profile databases is unlikely to occur given the benefits offered by WGS.
The serovar-prediction accuracy of Rep-PCR has been reported to range between 0 and 100%, indicating some limitations of this method (Shi et al., 2015). Ranieri et al. (2013) showed that Rep-PCR accurately predicted the serovar of 30 out of 46 isolates representing the top 40 Salmonella serovars isolated from human and non-human sources, with an accuracy of 65%. This accuracy was relatively lower than that obtained with PFGE or MLST, when the same set of isolates were evaluated.
Ashton et al. (2016) compared the serovars predicted by using legacy MLST sequences extracted from WGS data to the results generated by conventional serotyping, for 7,338 isolates representing 263 serovars of Salmonella enterica subspecies I. The 10 most common serovars in this S. enterica subspecies I dataset were serovars Enteritidis, Typhimurium, Infantis, Typhi, Newport, Virchow, Kentucky, Stanley, Paratyphi A, and Java. They found that the serovar prediction accuracy of legacy MLST was 96%.
The overall serovar-prediction accuracy for the CRISPR subtyping approach has been reported to range from 78 to 90% (Liu et al., 2011; Fabre et al., 2012; Shi et al., 2015). More studies are needed to further assess serovar-prediction accuracy using CRISPR.
Given the range of serovars represented in the SeqSero and SISTR databases, WGS can be used to theoretically predict 2,389 and 2,190 of the 2,577 serovars described in the White–Kauffmann–Le minor when using the serovar prediction programs SeqSero (Zhang et al., 2015) and SISTR (Yoshida et al., 2016a), respectively. Using empirical data, the accuracy of serotype prediction with SeqSero and SISTR has been reported to be approx. 92 and 95%, respectively (Zhang et al., 2015; Yoshida et al., 2016a, b; Robertson et al., 2018). By comparison, traditional Salmonella serotyping had an accuracy of 73% when 33–36 independent laboratories performed serotyping of the same eight Salmonella strains representing seven different serovars (Petersen et al., 2002), suggesting that WGS-based methods may be more reliable than traditional serotyping to assign Salmonella isolates to serovars. Nevertheless, further experimental studies are needed to continue to quantify the ability of WGS-based methods to identify Salmonella serovars.
Comparison of Molecular Methods for Subtype Differentiation of Salmonella
Molecular methods are used for subtyping Salmonella isolates that belong to the same serovar, as well as being used for serovar prediction. This section briefly provides some examples of comparative studies of subtyping methods. In one study, PFGE was compared to MLVA to subtype 163 non-typhoidal Salmonella isolates representing 15 serovars; MLVA differentiated the isolates into 79 MLVA subtypes while PFGE differentiated the same isolates into 87 subtypes. The Nei’s diversity index for MLVA was 0.979 compared to 0.999 for PFGE (Kjeldsen et al., 2016). However, for specific serovars (e.g., Salmonella Enteritidis) MLVA has been reported to provide improved discriminatory power over PFGE (Boxrud et al., 2007; Beranek et al., 2009; De Cesare et al., 2015). MLST has the advantage of being highly reproducible and easily transferable among laboratories. However, in a study of 110 Salmonella isolates from 25 serovars (Torpdahl et al., 2005), MLST resulted in 43 sequence types, while PFGE was able to differentiate the isolates into 73 PFGE subtypes. The downside of PFGE in this study was the inability to type 11 of the 110 (10%) isolates. In a study comparing different molecular methods to differentiate 52 Salmonella Enteritidis isolates, PFGE resulted in eight subtypes, while MLVA resulted in 18 subtypes and WGS resulted in 34 subtypes. The discriminatory power of PFGE, MLVA, and WGS was 0.81, 0.92, and 0.97 (Simpson’s index of diversity), respectively (Deng et al., 2015). In another study, PFGE and WGS were used to differentiate 55 Salmonella Enteritidis isolates; PFGE resulted in 10 subtypes; however, WGS was able to further differentiate the isolates into 45 unique subtypes (Taylor et al., 2015), showing the greater discriminatory power of WGS over PFGE. In a study of isolates from a Salmonella Poona outbreak (Kozyreva et al., 2016), 4 PFGE subtypes and 7 WGS subtypes were observed among the 16 isolates; in silico MLST using the WGS data resulted in one MLST sequence type. Phylogenetic analysis using WGS data showed that the distinct PFGE types did not necessarily correlate with increased genetic distance between isolates. Isolates that differed by 0 SNPs showed distinct PFGE subtypes, suggesting that PFGE results would be misleading for these isolates (Kozyreva et al., 2016). While the relative discriminatory power of different subtyping methods depends on the strains and serovars tested, WGS methods were consistently found to be most discriminatory, followed by PFGE. While some MLVA schemes provide enhanced discriminatory power over PFGE for some serovars, for other serovars PFGE may be more discriminatory than MLVA.
Criteria to Evaluate and Validate Different Salmonella Characterization Methods
Molecular-based Salmonella characterization methods including WGS are evolving very fast. Many of the characterization methods and technologies, as well as data analysis pipelines, are operated as research tools, and are under continuous development. Evaluation of these tools for Salmonella investigation, especially for those serovars/strains highly relevant to food products and processing environments, is pre-requisite for the implementation of these methods. Methods that can be used by the food industry must be thoroughly validated before implementation to ensure reliability and consistency of the method when it is used across different laboratories. Validation should cover the end-to-end workflow for source tracking from isolate subculture to bioinformatic analysis, articulating the key quality requirements and criteria (Ferrari et al., 2017; Nadon et al., 2017; Portmann et al., 2018). Proposed criteria for evaluation of Salmonella characterization methods for potential routine use in the food industry are shown below (Table 4).
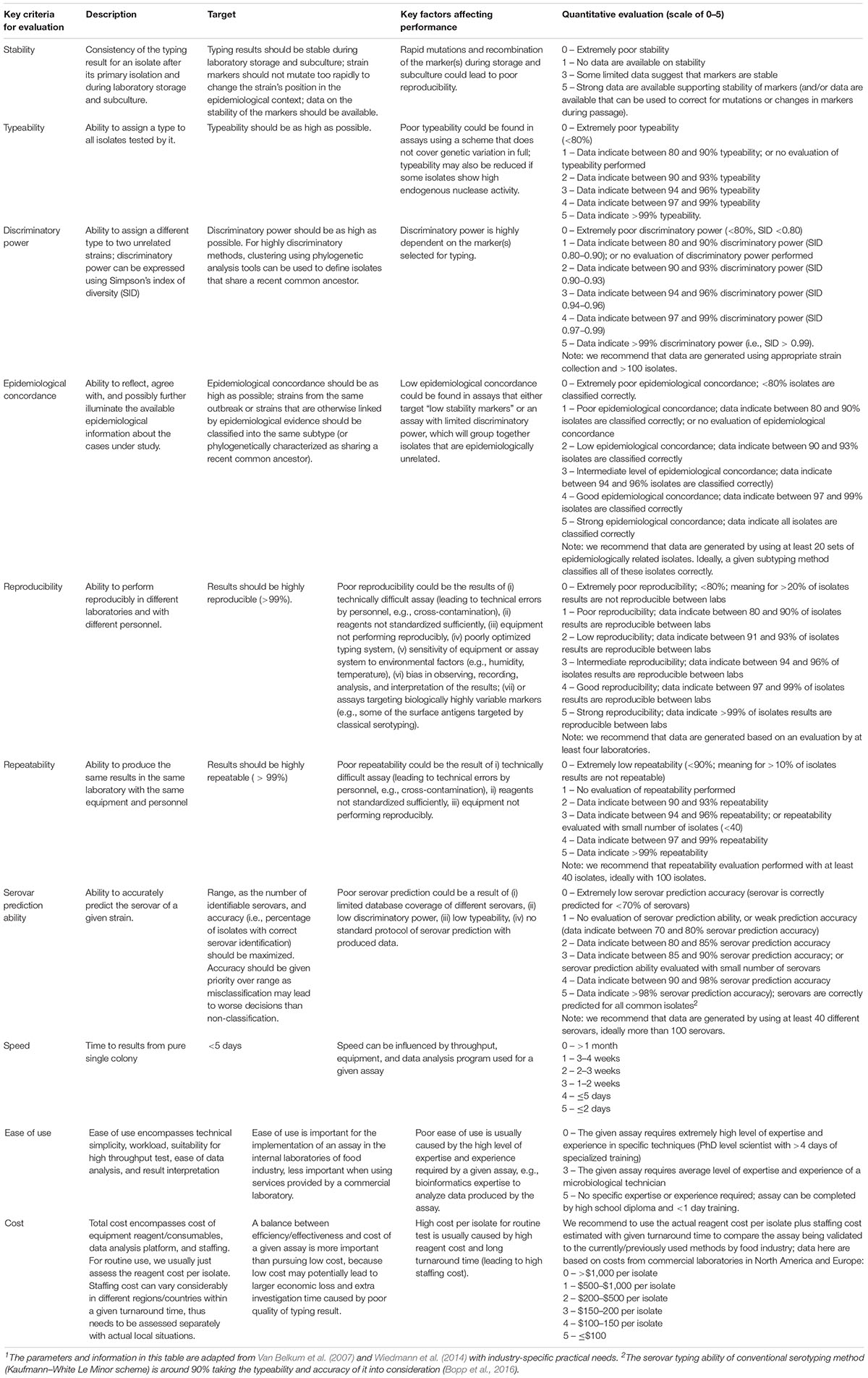
Table 4. Proposed evaluation criteria for Salmonella characterization methods that may be used routinely in the food industry1.
Implementation of Molecular-Based Salmonella Subtyping Methods by the Food Industry
We consider that WGS is the most suitable method to characterize Salmonella for incident investigation at production facilities in the food industry. This opinion is based on comparison of the resolution, turnaround time, ability of serovar prediction, cost, and feasibility of the available methods. Bioinformatics is a key capability required for WGS. The food industry may choose to invest in in-house capability that can interface with outside resources (e.g., academic partners, industry partners, government agencies), however, there are also opportunities to outsource data analyses to commercial or academic partner labs. Both the CFSAN pipeline and the Lyve-SET pipeline have been widely tested and seem to provide comparable and reliable results for hqSNP analysis. Implementation of wgMLST and cgMLST within BioNumerics has been successfully completed for L. monocytogenes in the United States. A cgMLST scheme is publicly available from EnteroBase (EnteroBase URL: https://enterobase.warwick.ac.uk/) and it is likely to be implemented within BioNumerics in the future. Other data analysis methods such as genome distance analysis (Pinho et al., 2009; Auch et al., 2010) can also become possible future approaches that allow for the food industry to develop data analysis capabilities for contamination source tracking.
The turnaround time of in-house WGS subtyping can be comparable to many conventional subtyping methods including conventional serotyping and PFGE (Table 1). WGS, however, provides much more information about an isolate with one single experimental procedure, enabling full characterization of the pathogen (including in silico serovar prediction and antimicrobial resistance gene identification) and more accurate clustering/discrimination of the isolates investigated. This is faster than using multiple conventional subtyping approaches in a stepwise approach to get equal information. The cost of WGS is also comparable to that of the conventional subtyping tools, considering the high quality and volume of information provided by WGS within one experimental procedure. In silico serotyping should be performed instead of traditional serotyping for determination of serovars once WGS is implemented as the subtyping method for Salmonella. This approach will greatly reduce the costs and time associated with serotyping.
Legacy MLST targeting variants of seven housekeeping genes of Salmonella can be used in combination with WGS. While legacy MLST classification can be obtained using Sanger sequencing technology (also known as first-generation sequencing technology) within 1 week, it can also be obtained by using the sequence information extracted from WGS data. Although legacy MLST has relatively lower discriminatory power compared with PFGE and MLVA, it is faster than PFGE when using an in-house Sanger sequencer such as Applied Biosystems Genetic Analysis Systems (Thermo Fisher Scientific). It is also more universal to all Salmonella serovars than MLVA which usually requires a specific scheme for each serovar. In addition, the serovar prediction ability of legacy MLST has been demonstrated to be comparable to that of PFGE (Tables 1, 3).
PFGE is currently still the “gold standard” and most widely used Salmonella DNA fingerprinting method used by public health authorities and food regulators to characterize and track this pathogen in outbreaks, although it is being replaced by WGS. PFGE remains a valuable tool for foodborne pathogen characterization by the food industry, while a transition to WGS occurs. PFGE has been repeatedly shown to be more discriminatory than methods such as conventional serotyping, automated ribotyping, or MLST for many bacteria including Salmonella. In addition to these methods, single-plex or multiplex PCR assays that can detect and identify specific Salmonella serotypes have been described (Kim et al., 2006; Akiba et al., 2011; Zhu et al., 2015; Xiong et al., 2018; Xu L. et al., 2018; Xu Y. et al., 2018); these tools provide an alternative approach for detection and identification of specific Salmonella serovars.
The results of any subtyping approach can be used to assess the relationship of isolates in an investigation. Nevertheless, the epidemiological context is indispensable in final decision making in incident investigation and to determine further actions for food safety management improvement. High-resolution WGS subtyping results should not be interpreted in the absence of epidemiological information.
The raw sequence data generated by molecular-based subtyping methods, especially WGS, require both physical and virtual space for storage. It is desirable to retain the original sequence reads (usually files with >200 MB for each Salmonella isolate) for potential future analysis using alternative data analysis methods or for a retrospective investigation. Commercial clouds can provide a storage solution, provided that special attention is paid to data security. A robust Internet connection and high band-width is needed to transfer WGS data if data storage is outsourced. Subtyping analysis needs to be supported by complete metadata providing the relevant epidemiological context to identify the root cause of the contamination. Thus, the capability for metadata collection, organization, and storage is needed together with building the capability for WGS. The metadata should include information such as the geographic and temporal background of the isolates, the sample type, and sample source (e.g., raw ingredients, finished products, environment), etc. The Consortium for Sequencing the Food Supply Chain, founded by IBM and Mars Incorporated, represents industrial groups putting effort into collecting genomic information on pathogenic bacteria across the food supply chain6. This consortium represents one part of the broader goal to increase knowledge of foodborne pathogens at the genomic level.
Conclusion
The application of DNA-based methods for characterization of pathogens such as Salmonella has become common practice. Our literature-based assessment supports the superior discriminatory power of WGS and its advantages compared with other methods for Salmonella subtyping and source tracking for the food industry. We also identified circumstances under which use of other subtyping methods may be warranted. Implementation of molecular-based Salmonella characterization methods, including WGS, provides improvement of source tracking and root cause elimination; however, these methods require investment in bioinformatics capability. Routine use of WGS or complete replacement of current subtyping methods by WGS will require attention to key issues including standardization, robustness, and validation of the analytical methodology. High resolution WGS subtyping of Salmonella promises to vastly improve the ability of the food industry to track and control Salmonella and is poised to become standard methodology in food safety for characterization of foodborne pathogens by public health authorities and food regulators. Nevertheless, standardization of WGS operation and data analysis, in particularly source tracking analysis, is required at a global level. A common agreement of understanding and the application of WGS between the food industry, public health, and food safety regulators are expected to guide the implementation of WGS in food safety management.
Author Contributions
ST and MW conceived and designed the work. ST, RO, and HL collected the data, conducted data analysis, and interpreted it. ST, RO, HL, and MW drafted the article. MW, AS, RB, CG, and GZ critically revised the article.
Funding
The authors declare that this study received funding from Mars Global Food Safety Center. The funder was not involved in the study design, collection, analysis, interpretation of data, the writing of this article or the decision to submit it for publication.
Conflict of Interest Statement
ST, HL, CG, GZ, RB, and AS were employed by the Mars Global Food Safety Center. MW serves as a compensated scientific advisor for BioMérieux, Mérieux NutriSciences, Mars, and Neogen and has served as a paid speaker for 3M and IBM.
The remaining author declares that the research was conducted in the absence of any commercial or financial relationships that could be construed as a potential conflict of interest.
Acknowledgments
We thank Peter Markwell, Dr. Bala Ganesan, and Dr. Kristel Hauben for comments that greatly improved the manuscript.
Footnotes
- ^ https://www.ncbi.nlm.nih.gov/sra
- ^ https://www.ebi.ac.uk/ena/
- ^ https://www.ddbj.nig.ac.jp/index-e.html
- ^ https://www.ncbi.nlm.nih.gov/pathogens/
- ^ https://www.qiagenbioinformatics.com
- ^ https://researcher.watson.ibm.com/researcher/view_group.php?id=9635
References
Achtman, M., Wain, J., Weill, F. X., Nair, S., Zhou, Z., and Sangal, V. (2012). Multilocus sequence typing as a replacement for serotyping in Salmonella enterica. PLoS Pathog. 8:e1002776. doi: 10.1371/journal.ppat.1002776
Akiba, M., Kusumoto, M., and Iwata, T. (2011). Rapid identification of Salmonella enterica serovars, Typhimurium, Choleraesuis, Infantis, Hadar, Enteritidis, Dublin and Gallinarum, by multiplex PCR. J. Microbiol. Methods 85, 9–15. doi: 10.1016/j.mimet.2011.02.002
Alcaine, S. D., Soyer, Y., Warnick, L. D., Su, W. L., Sukhnanand, S., Richards, J., et al. (2006). Multilocus sequence typing supports the hypothesis that cow- and human-associated Salmonella isolates represent distinct and overlapping populations. Appl. Environ. Microbiol. 72, 7575–7585. doi: 10.1128/aem.01174-06
Alikhan, N. F., Zhou, Z., Sergeant, M. J., and Achtman, M. (2018). A genomic overview of the population structure of Salmonella. PLoS Genet. 14:e1007261. doi: 10.1371/journal.pgen.1007261
Allard, M. W., Bell, R., Ferreira, C. M., Gonzalez-Escalona, N., Hoffmann, M., Muruvanda, T., et al. (2018). Genomics of foodborne pathogens for microbial food safety. Curr. Opin. Biotechnol. 49, 224–229. doi: 10.1016/j.copbio.2017.11.002
Allard, M. W., Luo, Y., Strain, E., Li, C., Keys, C. E., Son, I., et al. (2012). High resolution clustering of Salmonella enterica serovar Montevideo strains using a next-generation sequencing approach. BMC Genomics 13:32. doi: 10.1186/1471-2164-13-32
Allard, M. W., Strain, E., Melka, D., Bunning, K., Musser, S. M., Brown, E. W., et al. (2016). Practical value of food pathogen traceability through building a whole-genome sequencing network and database. J. Clin. Microbiol. 54, 1975–1983. doi: 10.1128/JCM.00081-16
Almeida, F., Medeiros, M. I., Rodrigues, P., Dos, D., and Falcao, J. P. (2015). Genotypic diversity, pathogenic potential and the resistance profile of Salmonella typhimurium strains isolated from humans and food from 1983 to 2013 in Brazil. J. Med. Microbiol. 64, 1395–1407. doi: 10.1099/jmm.0.000158
Almeida, F., Seribelli, A. A., da Silva, P., Medeiros, M. I. C., Dos Prazeres Rodrigues, D., Moreira, C. G., et al. (2017). Multilocus sequence typing of Salmonella typhimurium reveals the presence of the highly invasive ST313 in Brazil. Infect. Genet. Evol. 51, 41–44. doi: 10.1016/j.meegid.2017.03.009
Altschul, S. F., Gish, W., Miller, W., Myers, E. W., and Lipman, D. J. (1990). Basic local alignment search tool. J. Mol. Biol. 215, 403–410. doi: 10.1006/jmbi.1990.9999
Amirkhanian, V., Lui, M., Guttman, A., and Szantai, E. (2006). Cost Benefit Analysis of a Multicapillary Electrophoresis System American Laboratory. Available at: https://www.americanlaboratory.com/913-Technical-Articles/35725-Cost-Benefit-Analysis-of-a-Multicapillary-Electrophoresis-System/ (accessed June 1, 2006).
Ashton, P. M., Nair, S., Peters, T. M., Bale, J. A., Powell, D. G., and Painset, A. (2016). Identification of Salmonella for public health surveillance using whole genome sequencing. PeerJ 4:e1752. doi: 10.7717/peerj.1752
Auch, A. F., Klenk, H., and Göker, M. (2010). Standard operating procedure for calculating genome-to-genome distances based on high-scoring segment pairs. Stand. Genomic Sci. 2, 142–148. doi: 10.4056/sigs.541628
Barco, L., Barrucci, F., Olsen, J. E., and Ricci, A. (2013). Salmonella source attribution based on microbial subtyping. Int. J. Food Microbiol. 163, 193–203. doi: 10.1016/j.ijfoodmicro.2013.03.005
Barg, N. L., and Goering, R. V. (1993). Molecular epidemiology of nosocomial infection: analysis of chromosomal restriction fragment patterns by pulsed-field gel electrophoresis. Infect. Control Hosp. Epidemiol. 14, 595–600. doi: 10.1086/646645
Barrangou, R., and Dudley, E. G. (2016). CRISPR-based typing and next-generation tracking technologies. Annu. Rev. Food Sci. Technol. 7, 395–411. doi: 10.1146/annurev-food-022814-015729
Barrangou, R., and Horvath, P. (2012). CRISPR: new horizons in phage resistance and strain identification. Annu. Rev. Food Sci. Technol. 3, 143–162. doi: 10.1146/annurev-food-022811-101134
Bauer, N., Evans, P., Leopold, B., Levine, J., and White, P. (2013). USDA-FSIS Subtyping Work Group: Current and Future Development and Use of Molecular Subtyping by USDA-FSI. Available at: https://www.fsis.usda.gov/wps/wcm/ connect/6c7f71fd-2c0c-4ff0-b2bc-4977c7947516/Molecular-Subtyping-White- Paper.pdf?MOD=AJPERES (accessed October 27, 2018).
Ben-Darif, E., De Pinna, E., Threlfall, E. J., Bolton, F. J., Upton, M., Fox, A. J., et al. (2010). Comparison of a semi-automated rep-PCR system and multilocus sequence typing for differentiation of Salmonella enterica isolates. J. Microbiol. Methods 81, 11–16. doi: 10.1016/j.mimet.2010.01.013
Beranek, A., Mikula, C., Rabold, P., Arnhold, D., Berghold, C., Lederer, I., et al. (2009). Multiple-locus variable-number tandem repeat analysis for subtyping of Salmonella enterica subsp. enterica serovar Enteritidis. Int. J. Med. Microbiol. 299, 43–51. doi: 10.1016/j.ijmm.2008.06.002
Bopp, D. J., Baker, D. J., Thompson, L., Saylors, A., Root, T. P., Armstrong, L., et al. (2016). Implementation of Salmonella serotype determination using pulsed-field gel electrophoresis in a state public health laboratory. Diagn. Microbiol. Infect. Dis. 85, 416–418. doi: 10.1016/j.diagmicrobio.2016.04.023
Boxrud, D. (2010). Advances in subtyping methods of foodborne disease pathogens. Curr. Opin. Biotechnol. 21, 137–141. doi: 10.1016/j.copbio.2010.02.011
Boxrud, D., Pederson-Gulrud, K., Wotton, J., Medus, C., Lyszkowicz, E., Besser, J., et al. (2007). Comparison of multiple-locus variable-number tandem repeat analysis, pulsed-field gel electrophoresis, and phage typing for subtype analysis of Salmonella enterica serotype Enteritidis. J. Clin. Microbiol. 45, 536–543. doi: 10.1128/jcm.01595-06
Brenner, F. W., Villar, R. G., Angulo, F. J., Tauxe, R., and Swaminathan, B. (2000). Salmonella nomenclature - Guest commentary. J. Clin. Microbiol. 38, 2465–2467.
Call, D. R., Orfe, L., Davis, M. A., Lafrentz, S., and Kang, M. S. (2008). Impact of compounding error on strategies for subtyping pathogenic bacteria. Foodborne Pathog. Dis. 5, 505–516. doi: 10.1089/fpd.2008.0097
CDC (2009). U Centers, S., for Disease Control and Prevention: Salmonella Surveillance: Annual Summary. Available at: https://www.cdc.gov/ncezid/dfwed/pdfs/salmonellaannualsummarytables2009.pdf (accessed October 27, 2018).
CDC (2015). Serotypes and the Importance of Serotyping Salmonella. Available at: https://www.cdc.gov/salmonella/reportspubs/salmonella-atlas/serotyping-importance.html (accessed February 8, 2019).
CDC (2016a). Frequently Asked Questions. Available at: https://www.cdc.gov/pulsenet/about/faq.html (accessed February 16, 2016).
CDC (2016b). Whole Genome Sequencing (WGS). Available at: https://www.cdc.gov/pulsenet/pathogens/wgs.html (accessed February 11, 2016).
CDC (2017a). PulseNet International - On the Path to Implementing Whole Genome Sequencing for Foodborne Disease Surveillance. Available at: https://www.cdc.gov/pulsenet/participants/international/wgs-vision.html (accessed June 9, 2017).
CDC (2017b). Standard Operating Procedure for PulseNet PFGE of Escherichia coli O157:H7, Escherichia coli non-O157 (STEC), Salmonella serotypes, Shigella sonnei and Shigella flexneri. Available at: https://www.cdc.gov/pulsenet/pdf/ecoli-shigella-salmonella-pfge-protocol-508c.pdf (accessed October 27, 2018).
CDC (2017c). US Centers for Disease Control and Prevention (CDC): PulseNet Methods - Multiple Locus Variable-Number Tandem Repeat Analysis (MLVA). Available at: https://www.cdc.gov/pulsenet/pathogens/mlva.html (accessed October 27, 2018).
CDC (2018a). Pulsed-Field Gel Electrophoresis (PFGE). Available at: https://www.cdc.gov/pulsenet/pathogens/pfge.html (accessed December 30, 2018).
CDC (2018b). US Centers for Disease Control and Prevention (CDC): National Enteric Disease Surveillance - Salmonella Annual Report, 2016. Available at: https://www.cdc.gov/nationalsurveillance/salmonella-surveillance.html (accessed December 30, 2018).
CDC (2019). Outbreak of Salmonella Infections Linked to Pet Hedgehogs. Available at: https://www.cdc.gov/salmonella/typhimurium-01-19/index.html (accessed May 30, 2019).
Chen, Y., Luo, Y., Carleton, H., Timme, R., Melka, D., and Muruvanda, T. (2017). Whole genome and core genome multilocus sequence typing and single nucleotide polymorphism analyses of Listeria monocytogenes isolates associated with an outbreak linked to cheese, United States, 2013. Appl. Environ. Microbiol. 83:e00633-17. doi: 10.1128/AEM.00633-17
Chenu, J. W., Cox, J. M., and Pavic, A. (2012). Classification of Salmonella enterica serotypes from Australian poultry using repetitive sequence-based PCR. J. Appl. Microbiol. 112, 185–196. doi: 10.1111/j.1365-2672.2011.05172.x
Clooney, A. G., Fouhy, F., Sleator, R. D., O’Driscoll, A., Stanton, C., Cotter, P. D., et al. (2016). Comparing apples and oranges?: next generation sequencing and its impact on microbiome analysis. PLoS One 11:e0148028. doi: 10.1371/journal.pone.0148028
Collins, C., and Xavier, D. (2017). “Reconstructing the ancestral relationships between bacterial pathogen genomes,” in Bacterial Pathogenesis: Methods and Protocols, eds P. Nordenfelt and M. Collin (New York, NY: Springer), 109–137. doi: 10.1007/978-1-4939-6673-8_8
Dallman, T., Inns, T., Jombart, T., Ashton, P., Loman, N., and Chatt, C. (2016). Phylogenetic structure of European Salmonella Enteritidis outbreak correlates with national and international egg distribution network. Microb. Genom. 2:e000070. doi: 10.1099/mgen.0.000070
Davis, S., Pettengill, J. B., Luo, Y., Payne, J., Shpuntoff, A., Rand, H., et al. (2015). CFSAN SNP Pipeline: an automated method for constructing SNP matrices from next-generation sequence data. PeerJ Comput. Sci. 1:e20. doi: 10.7717/peerj-cs.20
De Cesare, A., Krishnamani, K., Parisi, A., Ricci, A., Luzzi, I., Barco, L., et al. (2015). Comparison between Salmonella enterica serotype Enteritidis genotyping methods and phage type. J. Clin. Microbiol. 53, 3021–3031. doi: 10.1128/JCM.01122-15
den Bakker, H. C., Allard, M. W., Bopp, D., Brown, E. W., Fontana, J., Iqbal, Z., et al. (2014). Rapid whole-genome sequencing for surveillance of Salmonella enterica serovar Enteritidis. Emerg. Infect. Dis. 20, 1306–1314. doi: 10.3201/eid2008.131399
den Bakker, H. C., Cummings, C. A., Ferreira, V., Vatta, P., Orsi, R. H., Degoricija, L., et al. (2010). Comparative genomics of the bacterial genus Listeria: genome evolution is characterized by limited gene acquisition and limited gene loss. BMC Genomics 11:688. doi: 10.1186/1471-2164-11-688
den Bakker, H. C., Switt, A. I., Cummings, C. A., Hoelzer, K., Degoricija, L., Rodriguez-rivera, L. D., et al. (2011). A whole-genome single nucleotide polymorphism-based approach to trace and identify outbreaks linked to a common Salmonella enterica subsp. enterica serovar Montevideo pulsed-field gel electrophoresis type. Appl. Environ. Microbiol. 77, 8648–8655. doi: 10.1128/AEM.06538-11
Deng, X., Shariat, N., Driebe, E. M., Roe, C. C., Tolar, B., Trees, E., et al. (2015). Comparative analysis of subtyping methods against a whole-genome-sequencing standard for Salmonella enterica serotype enteritidis. J. Clin. Microbiol. 53, 212–218. doi: 10.1128/JCM.02332-14
Dera-Tomaszewska, B. (2012). Salmonella serovars isolated for the first time in Poland, 1995-2007. Int. J. Occup. Med. Environ. Health 25, 294–303. doi: 10.2478/S13382-012-0038-2
Deurenberg, R. H., Bathoorn, E., Chlebowicz, M. A., Couto, N., Ferdous, M., and García-Cobos, S. (2017). Application of next generation sequencing in clinical microbiology and infection prevention. J. Biotechnol. 243, 16–24. doi: 10.1016/j.jbiotec.2016.12.022
Dijkshoorn, L., Towner, K. J., and Struelens, M. (2001). New Approaches for the Generation and Analysis of Microbial Typing Data. Amsterdam: Elsevier.
DiMarzio, M., Shariat, N., Kariyawasam, S., Barrangou, R., and Dudley, E. G. (2013). Antibiotic resistance in Salmonella enterica serovar typhimurium associates with CRISPR sequence type. Antimicrob. Agents Chemother. 57, 4282–4289. doi: 10.1128/AAC.00913-13
ECDC (2011). European Centre for Disease Prevention and Control (ECDC) Technical Document: Laboratory Standard Operating Procedure for MLVA of Salmonella enterica Serotype Typhimurium. Available at: https://ecdc.europa. eu/sites/portal/files/media/en/publications/Publications/1109_SOP_Salmonella _Typhimurium_MLVA.pdf (accessed October 27, 2018).
ECDC (2015). European Centre for Disease Prevention and Control (ECDC): Expert Opinion on the Introduction of Next-Generation Typing Methods for Food-and Waterborne Diseases in the EU and EEA. Stockholm: ECDC.
ECDC (2016a). European Centre for Disease Prevention and Control (ECDC): Salmonellosis - Annual Epidemiological Report 2016 [2014 Data]. Available at: https://ecdc.europa.eu/en/publications-data/salmonellosis-annual-epidemiological-report-2016-2014-data (accessed January 31, 2016).
ECDC (2016b). European Centre for Disease Prevention and Control (ECDC) Technical Document: Laboratory Standard Operating Procedure for Multiple-Locus Variable-Number Tandem Repeat Analysis of Salmonella enterica Serotype Enteritidis. Available at: https://ecdc.europa.eu/sites/portal/files/media/en/publications/Publications/Salmonella-Enteritidis-Laboratory-standard-operating-procedure.pdf (accessed October 27, 2018).
Elemfareji, O. I., and Thong, K. L. (2013). Comparative virulotyping of Salmonella typhi and Salmonella enteritidis. Indian J. Microbiol. 53, 410–417. doi: 10.1007/s12088-013-0407-y
Fabre, L., Zhang, J., Guigon, G., Le Hello, S., Guibert, V., and Accou-Demartin, M. (2012). CRISPR typing and subtyping for improved laboratory surveillance of Salmonella infections. PLoS One 7:e36995. doi: 10.1371/journal.pone.0036995
Fakhr, M. K., Nolan, L. K., and Logue, C. M. (2005). Multilocus sequence typing lacks the discriminatory ability of pulsed-field gel electrophoresis for typing Salmonella enterica serovar typhimurium. J. Clin. Microbiol. 43, 2215–2219. doi: 10.1128/JCM.43.5.2215-2219.2005
FAO (2016). Food and Agriculture Organization of the United Nations: Applications of Whole Genome Sequencing in Food Safety Management. Rome: FAO.
FDA (2018). GenomeTrakr Network. Available at: https://www.fda.gov/Food/FoodScienceResearch/WholeGenomeSequencingProgramWGS/ucm363134.htm (accessed March 21, 2019).
Ferrari, R. G., Panzenhagen, P. H. N., and Conte, C. A. Jr. (2017). Phenotypic and genotypic eligible methods for Salmonella typhimurium source tracking. Front. Microbiol. 8:2587. doi: 10.3389/fmicb.2017.02587
Fitzgerald, C., Gheesling, L., Collins, M., and Fields, P. I. (2006). Sequence analysis of the rfb loci, encoding proteins involved in the biosynthesis of the Salmonella enterica O17 and O18 antigens: serogroup-specific identification by PCR. Appl. Environ. Microbiol. 72, 7949–7953. doi: 10.1128/aem.01046-06
Foley, S. L., Lynne, A. M., and Nayak, R. (2009). Molecular typing methodologies for microbial source tracking and epidemiological investigations of Gram-negative bacterial foodborne pathogens. Infect. Genet. Evol. 9, 430–440. doi: 10.1016/j.meegid.2009.03.004
Foley, S. L., White, D. G., McDermott, P. F., Walker, R. D., Rhodes, B., Fedorka-Cray, P. J., et al. (2006). Comparison of subtyping methods for differentiating Salmonella enterica serovar typhimurium isolates obtained from food animal sources. J. Clin. Microbiol. 44, 3569–3577. doi: 10.1128/jcm.00745-06
Francisco, A. P., Bugalho, M., Ramirez, M., and Carrico, J. A. (2009). Global optimal eBURST analysis of multilocus typing data using a graphic matroid approach. BMC Bioinformatics 10:152. doi: 10.1186/1471-2105-10-152
Fricke, W. F., Mammel, M. K., McDermott, P. F., Tartera, C., White, D. G., Leclerc, J. E., et al. (2011). Comparative genomics of 28 Salmonella enterica isolates: evidence for CRISPR-mediated adaptive sublineage evolution. J. Bacteriol. 193, 3556–3568. doi: 10.1128/JB.00297-11
Galanis, E., Lo Fo Wong, D. M., Patrick, M. E., Binsztein, N., Cieslik, A., and Chalermchikit, T. (2006). Web-based surveillance and global Salmonella distribution, 2000-2002. Emerg. Infect. Dis. 12, 381–388. doi: 10.3201/eid1205.050854
Gaul, S. B., Wedel, S., Erdman, M. M., Harris, D. L., Harris, I. T., Ferris, K. E., et al. (2007). Use of pulsed-field gel electrophoresis of conserved XbaI fragments for identification of swine Salmonella serotypes. J. Clin. Microbiol. 45, 472–476. doi: 10.1128/jcm.00962-06
Gilson, E., Bachellier, S., Perrin, S., Perrin, D., Grimont, P. A., Grimont, F., et al. (1990). Palindromic unit highly repetitive DNA sequences exhibit species specificity within Enterobacteriacea. Res. Microbiol. 141, 1103–1116. doi: 10.1016/0923-2508(90)90084-4
GMA (2009). The Association of Food, Beverage and Consumer Products Companies (GMA): Control of Salmonella in Low-Moisture Foods. Available at: https://www.gmaonline.org/downloads/technical-guidance-and-tools/SalmonellaControlGuidance.pdf (accessed March 16, 2009).
Greig, J. D., and Ravel, A. (2009). Analysis of foodborne outbreak data reported internationally for source attribution. Int. J. Food Microbiol. 130, 77–87. doi: 10.1016/j.ijfoodmicro.2008.12.031
Grimont, P., and Weill, F. (2007). Antigenic Formulae of the Salmonella Serovars, 9th Edn. Paris: WHO Collaborating Centre for Reference and Research on Salmonella.
Guerra, B., Laconcha, I., Soto, S. M., Gonzalez-Hevia, M. A., and Mendoza, M. C. (2000). Molecular characterisation of emergent multiresistant Salmonella enterica serotype [4,5,12:i:-] organisms causing human salmonellosis. FEMS Microbiol. Lett. 190, 341–347. doi: 10.1111/j.1574-6968.2000.tb09309.x
Guibourdenche, M., Roggentin, P., Mikoleit, M., Fields, P. I., Bockemuhl, J., Grimont, P. A., et al. (2010). Supplement 2003-2007 (No. 47) to the White-Kauffmann-Le Minor scheme. Res. Microbiol. 161, 26–29. doi: 10.1016/j.resmic.2009.10.002
Guigon, G., Cheval, J., Cahuzac, R., and Brisse, S. (2008). MLVA-NET–a standardised web database for bacterial genotyping and surveillance. Euro Surveill. 13:18863. doi: 10.2807/ese.13.19.18863-en
Hadjinicolaou, A. V., Demetriou, V. L., Emmanuel, M. A., Kakoyiannis, C. K., and Kostrikis, L. G. (2009). Molecular beacon-based real-time PCR detection of primary isolates of Salmonella typhimurium and Salmonella enteritidis in environmental and clinical samples. BMC Microbiol. 9:97. doi: 10.1186/1471-2180-9-97
Hanning, I. B., Nutt, J. D., and Ricke, S. C. (2009). Salmonellosis outbreaks in the United States due to fresh produce: sources and potential intervention measures. Foodborne Pathog. Dis. 6, 635–648. doi: 10.1089/fpd.2008.0232
Harbottle, H., White, D. G., McDermott, P. F., Walker, R. D., and Zhao, S. (2006). Comparison of multilocus sequence typing, pulsed-field gel electrophoresis, and antimicrobial susceptibility typing for characterization of Salmonella enterica serotype Newport isolates. J. Clin. Microbiol. 44, 2449–2457. doi: 10.1128/jcm.00019-06
Hartmann, F. A., and West, S. E. (1997). Utilization of both phenotypic and molecular analyses to investigate an outbreak of multidrug-resistant Salmonella anatum in horses. Can. J. Vet. Res. 61, 173–181.
Hauser, E., Tietze, E., Helmuth, R., Junker, E., Prager, R., Schroeter, A., et al. (2012). Clonal dissemination of Salmonella enterica serovar infantis in Germany. Foodborne Pathog. Dis. 9, 352–360. doi: 10.1089/fpd.2011.1038
Hauser, E., Tietze, E., Helmuth, R., and Malorny, B. (2011). Different mutations in the oafA gene lead to loss of O5-antigen expression in Salmonella enterica serovar typhimurium. J. Appl. Microbiol. 110, 248–253. doi: 10.1111/j.1365-2672.2010.04877.x
Healy, M., Huong, J., Bittner, T., Lising, M., Frye, S., Raza, S., et al. (2005). Microbial DNA typing by automated repetitive-sequence-based PCR. J. Clin. Microbiol. 43, 199–207. doi: 10.1128/JCM.43.1.199-207.2005
Heisig, P., Kratz, B., Halle, E., Graser, Y., Altwegg, M., Rabsch, W., et al. (1995). Identification of DNA gyrase A mutations in ciprofloxacin-resistant isolates of Salmonella typhimurium from men and cattle in Germany. Microb. Drug Resist. 1, 211–218. doi: 10.1089/mdr.1995.1.211
Hoelzer, K., Soyer, Y., Rodriguez-Rivera, L. D., Cummings, K. J., McDonough, P. L., and Schoonmaker-Bopp, D. J. (2010). The prevalence of multidrug resistance is higher among bovine than human Salmonella enterica serotype Newport, Typhimurium, and 4,5,12:i:- isolates in the United States but differs by serotype and geographic region. Appl. Environ. Microbiol. 76, 5947–5959. doi: 10.1128/AEM.00377-10
Hoffmann, M., Luo, Y., Monday, S. R., Gonzalez-Escalona, N., Ottesen, A. R., and Muruvanda, T. (2016). Tracing origins of the Salmonella bareilly strain causing a food-borne outbreak in the united states. J. Infect. Dis. 213, 502–508. doi: 10.1093/infdis/jiv297
Hopkins, K. L., Maguire, C., Best, E., Liebana, E., and Threlfall, E. J. (2007). Stability of multiple-locus variable-number tandem repeats in Salmonella enterica serovar typhimurium. J. Clin. Microbiol. 45, 3058–3061. doi: 10.1128/jcm.00715-07
Hopkins, K. L., Peters, T. M., de Pinna, E., and Wain, J. (2011). Standardisation of multilocus variable-number tandem-repeat analysis (MLVA) for subtyping of Salmonella enterica serovar Enteritidis. Euro Surveill. 16:19942. doi: 10.2807/ese.16.32.19942-en
Hulton, C. S. J., Higgins, C. F., and Sharp, P. M. (1991). ERIC sequences: a novel family of repetitive elements in the genomes of Escherichia coli, Salmonella typhimurium and other enterobacteria. Mol. Microbiol. 5, 825–834. doi: 10.1111/j.1365-2958.1991.tb00755.x
Hunter, S. B., Vauterin, P., Lambert-Fair, M. A., Van Duyne, M. S., Kubota, K., Graves, L., et al. (2005). Establishment of a universal size standard strain for use with the pulsenet standardized pulsed-field gel electrophoresis protocols: converting the national databases to the new size standard. J. Clin. Microbiol. 43, 1045. doi: 10.1128/JCM.43.3.1045-1050.2005
Hyytia-Trees, E. K., Cooper, K., Ribot, E. M., and Gerner-Smidt, P. (2007). Recent developments and future prospects in subtyping of foodborne bacterial pathogens. Future Microbiol. 2, 175–185. doi: 10.2217/17460913.2.2.175
Illumina (2019). Illumina - Introduction to SBS Technology. Available at: https://www.illumina.com/science/technology/next-generation-sequencing/sequencing-technology.html (accessed October 27, 2018).
Inns, T., Ashton, P., Herrera-Leon, S., Lighthill, J., Foulkes, S., and Jombart, T. (2016). Prospective use of whole genome sequencing (WGS) detected a multi-country outbreak of Salmonella enteritidis. Epidemiol. Infect. 145, 289–298. doi: 10.1017/S0950268816001941
Inns, T., Lane, C., Peters, T., Dallman, T., Chatt, C., and McFarland, N. (2015). A multi-country Salmonella enteritidis phage type 14b outbreak associated with eggs from a German producer: ‘near real-time’ application of whole genome sequencing and food chain investigations, United Kingdom, May to September 2014. Euro Surveill. 20:21098. doi: 10.2807/1560-7917.ES2015.20.16.21098
Inouye, M., Dashnow, H., Raven, L. A., Schultz, M. B., Pope, B. J., Tomita, T., et al. (2014). SRST2: rapid genomic surveillance for public health and hospital microbiology labs. Genome Med. 6:90. doi: 10.1186/s13073-014-0090-6
Jackson, B. R., Tarr, C., Strain, E., Jackson, K. A., Conrad, A., and Carleton, H. (2016). Implementation of nationwide real-time whole-genome sequencing to enhance listeriosis outbreak detection and investigation. Clin. Infect. Dis. 63, 380–386. doi: 10.1093/cid/ciw242
Jagadeesan, B., Gerner-Smidt, P., Allard, M. W., Leuillet, S., Winkler, A., and Xiao, Y. (2019). The use of next generation sequencing for improving food safety: translation into practice. Food Microbiol. 79, 96–115. doi: 10.1016/j.fm.2018.11.005
Katz, L. S., Griswold, T., Williams-Newkirk, A. J., Wagner, D., Petkau, A., Sieffert, C., et al. (2017). A comparative analysis of the Lyve-SET phylogenomics pipeline for genomic epidemiology of foodborne pathogens. Front. Microbiol. 8:375. doi: 10.3389/fmicb.2017.00375
Kerouanton, A., Marault, M., Lailler, R., Weill, F. X., Feurer, C., Espie, E., et al. (2007). Pulsed-field gel electrophoresis subtyping database for foodborne Salmonella enterica serotype discrimination. Foodborne Pathog. Dis. 4, 293–303. doi: 10.1089/fpd.2007.0090
Kidgell, C., Reichard, U., Wain, J., Linz, B., Torpdahl, M., Dougan, G., et al. (2002). Salmonella typhi, the causative agent of typhoid fever, is approximately 50,000 years old. Infect. Genet. Evol. 2, 39–45. doi: 10.1016/s1567-1348(02)00089-8
Kim, S., Frye, J. G., Hu, J., Fedorka-Cray, P. J., Gautom, R., Boyle, D. S., et al. (2006). Multiplex PCR-based method for identification of common clinical serotypes of Salmonella enterica subsp. enterica. J. Clin. Microbiol. 44, 3608–3615. doi: 10.1128/jcm.00701-06
Kjeldsen, M. K., Torpdahl, M., Pedersen, K., and Nielsen, E. M. (2016). Development and comparison of a generic multiple-locus variable-number tandem repeat analysis with PFGE for typing of Salmonella enterica subsp. enterica. J. Appl. Microbiol. 119, 1707–1717. doi: 10.1111/jam.12965
Kodama, Y., Shumway, M., and Leinonen, R. (2012). The Sequence Read Archive: explosive growth of sequencing data. Nucleic Acids Res. 40, 54–56. doi: 10.1093/nar/gkr854
Kozyreva, V. K., Crandall, J., Sabol, A., Poe, A., Zhang, P., Concepcion-Acevedo, J., et al. (2016). Laboratory investigation of Salmonella enterica serovar Poona outbreak in California: comparison of pulsed-field gel electrophoresis (PFGE) and whole genome sequencing (WGS) results. PLoS Curr. 8:ecurrents.outbreaks.1bb3e36e74bd5779bc43ac3a8dae52e6. doi: 10.1371/currents.outbreaks.1bb3e36e74bd5779bc43ac3a8dae52e6
Kruy, S. L., van Cuyck, H., and Koeck, J. L. (2011). Multilocus variable number tandem repeat analysis for Salmonella enterica subspecies. Eur. J. Clin. Microbiol. Infect. Dis. 30, 465–473. doi: 10.1007/s10096-010-1110-0
Kvistholm Jensen, A., Nielsen, E. M., Bjorkman, J. T., Jensen, T., Muller, L., Persson, S., et al. (2016). Whole-genome sequencing used to investigate a nationwide outbreak of listeriosis caused by ready-to-eat delicatessen meat, Denmark, 2014. Clin. Infect. Dis. 63, 64–70. doi: 10.1093/cid/ciw192
Larsson, J. T., and Torpdahl, M., Mlva working group Moller, N. E. (2013). Proof-of-concept study for successful inter-laboratory comparison of MLVA results. Euro Surveill. 18:20566. doi: 10.2807/1560-7917.ES2013.18.35.20566
Larsson, J. T., Torpdahl, M., Petersen, R. F., Sorensen, G., Lindstedt, B. A., Nielsen, E. M., et al. (2009). Development of a new nomenclature for Salmonella typhimurium multilocus variable number of tandem repeats analysis (MLVA). Euro Surveill. 14:19174. doi: 10.2807/ese.14.15.19174-en
Leekitcharoenphon, P., Nielsen, E. M., Kaas, R. S., Lund, O., and Aarestrup, F. M. (2014). Evaluation of whole genome sequencing for outbreak detection of Salmonella enterica. PLoS One 9:e87991. doi: 10.1371/journal.pone.0087991
Li, H. (2013). Aligning Sequence Reads, Clone Sequences and Assembly Contigs with BWA-MEM. Available at: http://arxiv.org/abs/1303.3997 (accessed March 16, 2013).
Li, W., Raoult, D., and Fournier, P. E. (2009). Bacterial strain typing in the genomic era. FEMS Microbiol. Rev. 33, 892–916. doi: 10.1111/j.1574-6976.2009.00182.x
Lienemann, T., Kyyhkynen, A., Halkilahti, J., Haukka, K., and Siitonen, A. (2015). Characterization of Salmonella typhimurium isolates from domestically acquired infections in Finland by phage typing, antimicrobial susceptibility testing, PFGE and MLVA. BMC Microbiol. 15:131. doi: 10.1186/s12866-015-0467-8
Lindstedt, B. A. (2005). Multiple-locus variable number tandem repeats analysis for genetic fingerprinting of pathogenic bacteria. Electrophoresis 26, 2567–2582. doi: 10.1002/elps.200500096
Lindstedt, B. A., Heir, E., Gjernes, E., and Kapperud, G. (2003). DNA fingerprinting of Salmonella enterica subsp. enterica serovar typhimurium with emphasis on phage type DT104 based on variable number of tandem repeat loci. J. Clin. Microbiol. 41, 1469–1479. doi: 10.1128/JCM.41.4.1469-1479.203
Lindstedt, B. A., Torpdahl, M., Nielsen, E. M., Vardund, T., Aas, L., Kapperud, G., et al. (2007). Harmonization of the multiple-locus variable-number tandem repeat analysis method between Denmark and Norway for typing Salmonella typhimurium isolates and closer examination of the VNTR loci. J. Appl. Microbiol. 102, 728–735. doi: 10.1111/j.1365-2672.2006.03134.x
Lindstedt, B. A., Torpdahl, M., Vergnaud, G., Le Hello, S., Weill, F. X., Tietze, E., et al. (2013). Use of multilocus variable-number tandem repeat analysis (MLVA) in eight European countries, 2012. Euro Surveill. 18:20385. doi: 10.2807/ese.18.04.20385-en
Liu, F. (2010). Virulence Gene and CRISPR Multilocus Sequence Typing Scheme for Subtyping the Major Serovars of Salmonella enterica Subspecies enterica. Available at: https://etda.libraries.psu.edu/files/final_submissions/1960 (accessed October 27, 2018).
Liu, F., Barrangou, R., Gernersmidt, P., Ribot, E. M., Knabel, S. J., Dudley, E. G., et al. (2011). Novel virulence gene and clustered regularly interspaced short palindromic repeat (CRISPR) multilocus sequence typing scheme for subtyping of the major serovars of Salmonella enterica subsp. enterica. Appl. Environ. Microbiol. 77, 1946–1956. doi: 10.1128/AEM.02625-10
Loman, N. J., and Pallen, M. J. (2015). Twenty years of bacterial genome sequencing. Nat. Rev. Microbiol. 13, 787–794. doi: 10.1038/nrmicro3565
Maciorowski, K. G., Jones, F. T., Pillaiand, S. D., and Ricke, S. C. (2004). Incidence, sources, and control of food-borne Salmonella spp. in poultry feeds. Worlds Poult. Sci. J. 60, 446–457. doi: 10.1079/WPS200428
Maiden, M. C., Jansen van Rensburg, M. J., Bray, J. E., Earle, S. G., Ford, S. A., Jolley, K. A., et al. (2013). MLST revisited: the gene-by-gene approach to bacterial genomics. Nat. Rev. Microbiol. 11, 728–736. doi: 10.1038/nrmicro3093
Martin, B., Humbert, O., Camara, M., Guenzi, E., Walker, J., Mitchell, T., et al. (1992). A highly conserved repeated DNA element located in the chromosome of Streptococcus pneumoniae. Nucleic Acids Res. 20, 3479–3483. doi: 10.1093/nar/20.13.3479
McQuiston, J. R., Parrenas, R., Ortiz-Rivera, M., Gheesling, L., Brenner, F., Fields, P. I., et al. (2004). Sequencing and comparative analysis of flagellin genes fliC, fljB, and flpA from Salmonella. J. Clin. Microbiol. 42, 1923–1932. doi: 10.1128/JCM.42.5.1923-1932.2004
McQuiston, J. R., Waters, R. J., Dinsmore, B. A., Mikoleit, M. L., and Fields, P. I. (2011). Molecular determination of H antigens of Salmonella by use of a microsphere-based liquid array. J. Clin. Microbiol. 49, 565–573. doi: 10.1128/JCM.01323-10
Mohammed, M., Delappe, N., O’Connor, J., McKeown, P., Garvey, P., Cormican, M., et al. (2016). Whole genome sequencing provides an unambiguous link between Salmonella Dublin outbreak strain and a historical isolate. Epidemiol. Infect. 144, 576–581. doi: 10.1017/S0950268815001636
Moura, A., Criscuolo, A., Pouseele, H., Maury, M. M., Leclercq, A., Tarr, C., et al. (2016). Whole genome-based population biology and epidemiological surveillance of Listeria monocytogenes. Nat. Microbiol. 2:16185. doi: 10.1038/nmicrobiol.2016.185
Mughini-Gras, L., Franz, E., and van Pelt, W. (2018). New paradigms for Salmonella source attribution based on microbial subtyping. Food Microbiol. 71, 60–67. doi: 10.1016/j.fm.2017.03.002
Muteeb, G., and Sen, R. (2010). “Random mutagenesis using a mutator strain,” in In Vitro Mutagenesis Protocols, 3rd Edn, ed. J. Braman (Totowa, NJ: Humana Press), 411–419. doi: 10.1007/978-1-60761-652-8_29
Nadon, C., Van Walle, I., Gerner-Smidt, P., Campos, J., Chinen, I., Concepcion-Acevedo, J., et al. (2017). PulseNet International: vision for the implementation of whole genome sequencing (WGS) for global food-borne disease surveillance. Euro Surveill. 22:30544. doi: 10.2807/1560-7917.ES.2017.22.23.30544
Nde, C. W., Sherwood, J. S., Doetkott, C., and Logue, C. M. (2006). Prevalence and molecular profiles of Salmonella collected at a commercial turkey processing plant. J. Food Prot. 69, 1794–1801. doi: 10.4315/0362-028X-69.8.1794
Ngoi, S. T., Teh, C. S., Chai, L. C., and Thong, K. L. (2015). Overview of molecular typing tools for the characterization of Salmonella enterica in Malaysia. Biomed. Environ. Sci. 28, 751–764. doi: 10.3967/bes2015.105
Nsofor, C. (2016). Pulsed-field gel electrophoresis (PFGE): principles and applications in molecular epidemiology: a review. Int. J. Curr. Res. Med. Sci. 2, 38–51. doi: 10.1111/j.1863-2378.2009.01259.x
Octavia, S., Wang, Q., Tanaka, M. M., Kaur, S., Sintchenko, V., Lan, R., et al. (2015). Delineating community outbreaks of Salmonella enterica serovar Typhimurium by use of whole-genome sequencing: insights into genomic variability within an outbreak. J. Clin. Microbiol. 53, 1063–1071. doi: 10.1128/JCM.03235-14
Olaimat, A. N., and Holley, R. A. (2012). Factors influencing the microbial safety of fresh produce: a review. Food Microbiol. 32, 1–19. doi: 10.1016/j.fm.2012.04.016
Oloya, J., Doetkott, D., and Khaitsa, M. L. (2009). Antimicrobial drug resistance and molecular characterization of Salmonella isolated from domestic animals, humans, and meat products. Foodborne Pathog. Dis. 6, 273–284. doi: 10.1089/fpd.2008.0134
Olson, A. B., Andrysiak, A. K., Tracz, D. M., Guard-Bouldin, J., Demczuk, W., Ng, L. K., et al. (2007). Limited genetic diversity in Salmonella enterica serovar Enteritidis PT13. BMC Microbiol. 7:87. doi: 10.1186/1471-2180-7-87
Park, S. Y., Woodward, C. L., Kubena, L. F., Nisbet, D. J., Birkhold, S. G., Ricke, S. C., et al. (2008). Environmental dissemination of foodborne Salmonella in preharvest poultry production: reservoirs, critical factors, and research strategies. Crit. Rev. Environ. Sci. Technol. 38, 73–111. doi: 10.1080/10643380701598227
Patané, J. S. L., Martins, J., and Setubal, J. C. (2018). “Phylogenomics,” in Comparative Genomics: Methods and Protocols, eds J. C. Setubal, J. Stoye, and P. F. Stadler (New York, NY: Springer).
Pearce, M. E., Alikhan, N. F., Dallman, T. J., Zhou, Z., Grant, K., Maiden, M. C. J., et al. (2018). Comparative analysis of core genome MLST and SNP typing within a European Salmonella serovar Enteritidis outbreak. Int. J. Food. Microbiol. 274, 1–11. doi: 10.1016/j.ijfoodmicro.2018.02.023
Persing, D. H., Tenover, F. C., Versalovic, J., Tang, Y. W., Unger, E. R., and Relman, D. A. (2011). Molecular Microbiology: Diagnostic Principles and Practice. Washington, DC: ASM Press.
Petersen, A., Aarestrup, F. M., Angulo, F. J., Wong, S., Stöhr, K., Wegener, H. C., et al. (2002). WHO global salm-surv external quality assurance system (EQAS): an important step toward improving the quality of Salmonella serotyping and antimicrobial susceptibility testing worldwide. Microb. Drug Resist. 8, 345–353. doi: 10.1089/10766290260469615
Pightling, A. W., Petronella, N., and Pagotto, F. (2014). Choice of reference sequence and assembler for alignment of Listeria monocytogenes short-read sequence data greatly influences rates of error in SNP analyses. PLoS One 9:e104579. doi: 10.1371/journal.pone.0104579
Pightling, A. W., Pettengill, J. B., Luo, Y., Baugher, J. D., Rand, H., Strain, E., et al. (2018). Interpreting whole-genome sequence analyses of foodborne bacteria for regulatory applications and outbreak investigations. Front. Microbiol. 9:1482. doi: 10.3389/fmicb.2018.01482
Pillai, S. D., and Ricke, S. C. (2002). Bioaerosols from municipal and animal wastes: background and contemporary issues. Can. J. Microbiol. 48, 681–696. doi: 10.1139/w02-070
Pinho, A. J., Bastos, C. A. C., Ferreira, P. J. S. G., Garcia, S. P., and Afreixo, V. (2009). Genome analysis with inter-nucleotide distances. Bioinformatics 25, 3064–3070. doi: 10.1093/bioinformatics/btp546
Portmann, A. C., Fournier, C., Gimonet, J., Ngom-Bru, C., Barretto, C., and Baert, L. (2018). A validation approach of an end-to-end whole genome sequencing workflow for source tracking of Listeria monocytogenes and Salmonella enterica. Front. Microbiol. 9:446. doi: 10.3389/fmicb.2018.00446
Porwollik, S., Boyd, E. F., Choy, C., Cheng, P., Florea, L., Proctor, E., et al. (2004). Characterization of Salmonella enterica subspecies I genovars by use of microarrays. J. Bacteriol. 186, 5883–5898. doi: 10.1128/JB.186.17.5883-5898.2004
PulseNet (2013). Standard Operating Procedure for PulseNet PFGE of Escherichia coli O157:H7, Escherichia coli non-O157 (STEC), Salmonella Serotypes, Shigella sonnei and Shigella flexneri. Available at: http://www.pulsenetinternational.org/assets/PulseNet/uploads/pfge/PNL05_Ec-Sal-ShigPFGEprotocol.pdf (accessed March 24, 2013).
PulseNet (2014). PulseNet Europe. Available at: http://www.pulsenetinternational.org/networks/europe/ (accessed November 4, 2014).
PulseNet (2015a). PulseNet Explained. Available at: http://www.pulsenetinternational.org/international/pulsenetexplained/ (accessed May 13, 2015).
PulseNet (2015b). PulseNet Protocols A - Pulse Field Gel Electrophoresis (PFGE). Available at: http://www.pulsenetinternational.org/protocols/pfge/ (accessed October 22, 2015).
PulseNet (2015c). PulseNet Protocols B - Molecular Typing for MLVA. Available at: http://www.pulsenetinternational.org/protocols/mlva/ (accessed May 13, 2015).
Ranieri, M. L., Shi, C., Switt, M. A. I., den Bakker, H. C., and Wiedmann, M. (2013). Comparison of typing methods with a new procedure based on sequence characterization for Salmonella serovar prediction. J. Clin. Microbiol. 51, 1786–1797. doi: 10.1128/JCM.03201-12
Rantsiou, K., Kathariou, S., Winkler, A., Skandamis, P., Saint-Cyr, M. J., Rouzeau-Szynalski, K., et al. (2018). Next generation microbiological risk assessment: opportunities of whole genome sequencing (WGS) for foodborne pathogen surveillance, source tracking and risk assessment. Int. J. Food Microbiol. 287, 3–9. doi: 10.1016/j.ijfoodmicro.2017.11.007
Ricke, S. C. (2017). Insights and challenges of Salmonella infection of laying hens. Curr. Opin. Food Sci. 18, 43–49. doi: 10.1016/j.cofs.2017.10.012
Ricke, S. C., Kim, S. A., Shi, Z., and Park, S. H. (2018). Molecular-based identification and detection of Salmonella in food production systems: current perspectives. J. Appl. Microbiol. 125, 313–327. doi: 10.1111/jam.13888
Robertson, J., Yoshida, C., Kruczkiewicz, P., Nadon, C., Nichani, A., Taboada, E. N., et al. (2018). Comprehensive assessment of the quality of Salmonella whole genome sequence data available in public sequence databases using the Salmonella in silico typing resource (SISTR). Microb. Genom. 4:e000151. doi: 10.1099/mgen.0.000151
Ross, I. L., and Heuzenroeder, M. W. (2005). Discrimination within phenotypically closely related definitive types of Salmonella enterica serovar typhimurium by the multiple amplification of phage locus typing technique. J. Clin. Microbiol. 43, 1604–1611. doi: 10.1128/JCM.43.4.1604-1611.2005
Ross, I. L., and Heuzenroeder, M. W. (2008). A comparison of three molecular typing methods for the discrimination of Salmonella enterica serovar Infantis. FEMS Immunol. Med. Microbiol. 53, 375–384. doi: 10.1111/j.1574-695X.2008.00435.x
Sabat, A. J., Budimir, A., Nashev, D., Sá-Leão, R., van Dijl, J. M., Laurent, F., et al. (2013). Overview of molecular typing methods for outbreak detection and epidemiological surveillance. Euro Surveill. 18:20380. doi: 10.2807/ese.18.04.20380-en
Salmonella Subcommittee of the Nomenclature Committee of the International Society for, Microbiology (1934). The genus Salmonella Lignières, 1900. J. Hyg. 34, 333–350. doi: 10.1017/s0022172400034677
Sangal, V., Harbottle, H., Mazzoni, C. J., Helmuth, R., Guerra, B., Didelot, X., et al. (2010). Evolution and population structure of Salmonella enterica serovar Newport. J. Bacteriol. 192, 6465–6476. doi: 10.1128/JB.00969-10
Schouls, L. M., Spalburg, E. C., van Luit, M., Huijsdens, X. W., Pluister, G. N., van Santen-Verheuvel, M. J., et al. (2009). Multiple-locus variable number tandem repeat analysis of Staphylococcus aureus: comparison with pulsed-field gel electrophoresis and spa-typing. PLoS One 4:e5082. doi: 10.1371/journal.pone.0005082
Schwartz, D. C., and Cantor, C. R. (1984). Separation of yeast chromosome-sized DNAs by pulsed field gradient gel electrophoresis. Cell 37, 67–75. doi: 10.1016/0092-8674(84)90301-5
Shariat, N., DiMarzio, M. J., Yin, S., Dettinger, L., Sandt, C. H., Lute, J. R., et al. (2013a). The combination of CRISPR-MVLST and PFGE provides increased discriminatory power for differentiating human clinical isolates of Salmonella enterica subsp. enterica serovar Enteritidis. Food Microbiol. 34, 164–173. doi: 10.1016/j.fm.2012.11.012
Shariat, N., Sandt, C. H., DiMarzio, M. J., Barrangou, R., and Dudley, E. G. (2013b). CRISPR-MVLST subtyping of Salmonella enterica subsp. enterica serovars Typhimurium and Heidelberg and application in identifying outbreak isolates. BMC Microbiol. 13:254. doi: 10.1186/1471-2180-13-254
Shariat, N., Sandt, C. H., Kirchner, M. K., Trees, E., Barrangou, R., and Dudley, E. G. (2013c). Subtyping of Salmonella enterica serovar Newport outbreak isolates by CRISPR-MVLST and determination of the relationship between CRISPR-MVLST and PFGE results. J. Clin. Microbiol. 51, 2328–2336. doi: 10.1128/JCM.00608-13
Shariat, N., and Dudley, E. G. (2014). CRISPRs: molecular signatures used for pathogen subtyping. Appl. Environ. Microbiol. 80, 430–439. doi: 10.1128/AEM.02790-13
Shi, C., Singh, P., Ranieri, M. L., Wiedmann, M., and Moreno Switt, A. I. (2015). Molecular methods for serovar determination of Salmonella. Crit. Rev. Microbiol. 41, 309–325. doi: 10.3109/1040841X.2013.837862
Singh, A., Goering, R. V., Simjee, S., Foley, S. L., and Zervos, M. J. (2006). Application of molecular techniques to the study of hospital infection. Clin. Microbiol. Rev. 19, 512–530.
Soyer, Y., Alcaine, S. D., Schoonmaker-Bopp, D. J., Root, T. P., Warnick, L. D., McDonough, P. L., et al. (2010). Pulsed-field gel electrophoresis diversity of human and bovine clinical Salmonella isolates. Foodborne Pathog. Dis. 7, 707–717. doi: 10.1089/fpd.2009.0424
Soyer, Y., Moreno Switt, A., Davis, M. A., Maurer, J., McDonough, P. L., Schoonmaker-Bopp, D. J., et al. (2009). Salmonella enterica serotype 4,5,12:i:-, an emerging Salmonella serotype that represents multiple distinct clones. J. Clin. Microbiol. 47, 3546–3556. doi: 10.1128/JCM.00546-09
Sukhnanand, S., Alcaine, S., Warnick, L. D., Su, W. L., Hof, J., Craver, M. P., et al. (2005). DNA sequence-based subtyping and evolutionary analysis of selected Salmonella enterica serotypes. J. Clin. Microbiol. 43, 3688–3698. doi: 10.1128/JCM.43.8.3688-3698.2005
Taylor, A. J., Lappi, V., Wolfgang, W. J., Lapierre, P., Palumbo, M. J., and Medus, C. (2015). Characterization of foodborne outbreaks of Salmonella enterica serovar enteritidis with whole-genome sequencing single nucleotide polymorphism-based analysis for surveillance and outbreak detection. J. Clin. Microbiol. 53, 3334–3340. doi: 10.1128/JCM.01280-15
Thong, K. L., and Ang, C. P. (2011). Genotypic and phenotypic differentiation of Salmonella enterica serovar Paratyphi B in Malaysia. Southeast Asian J. Trop. Med. Public Health 42, 1178–1189.
Threlfall, E. J., and Frost, J. A. (1990). The identification, typing and fingerprinting of Salmonella: laboratory aspects and epidemiological applications. J. Appl. Bacteriol. 68, 5–16. doi: 10.1111/j.1365-2672.1990.tb02542.x
Tiong, V., Thong, K. L., Yusof, M. Y., Hanifah, Y. A., Sam, J. I., and Hassan, H. (2010). Macrorestriction analysis and antimicrobial susceptibility profiling of Salmonella enterica at a University Teaching Hospital, Kuala Lumpur. Jpn. J. Infect. Dis. 63, 317–322.
Top, J., Banga, N. M., Hayes, R., Willems, R. J., Bonten, M. J., and Hayden, M. K. (2008). Comparison of multiple-locus variable-number tandem repeat analysis and pulsed-field gel electrophoresis in a setting of polyclonal endemicity of vancomycin-resistant Enterococcus faecium. Clin. Microbiol. Infect. 14, 363–369. doi: 10.1111/j.1469-0691.2007.01945.x
Torpdahl, M., Skov, M. N., Sandvang, D., and Baggesen, D. L. (2005). Genotypic characterization of Salmonella by multilocus sequence typing, pulsed-field gel electrophoresis and amplified fragment length polymorphism. J. Microbiol. Methods 63, 173–184. doi: 10.1016/j.mimet.2005.03.006
Torpdahl, M., Sorensen, G., Lindstedt, B. A., and Nielsen, E. M. (2007). Tandem repeat analysis for surveillance of human Salmonella typhimurium infections. Emerg. Infect. Dis. 13, 388–395. doi: 10.3201/eid1303.060460
Van Belkum, A., Tassios, P. T., Dijkshoorn, L., Haeggman, S., Cookson, B., Fry, N. K., et al. (2007). Guidelines for the validation and application of typing methods for use in bacterial epidemiology. Clin. Microbiol. Infect. 13, 1–46. doi: 10.1111/j.1469-0691.2007.01786.x
Van Cuyck, H., Farbos-Granger, A., Leroy, P., Yith, V., Guillard, B., Sarthou, J. L., et al. (2011). MLVA polymorphism of Salmonella enterica subspecies isolated from humans, animals, and food in Cambodia. BMC Res. Notes 4:306. doi: 10.1186/1756-0500-4-306
Vincent, C., Usongo, V., Berry, C., Tremblay, D. M., Moineau, S., Yousfi, K., et al. (2018). Comparison of advanced whole genome sequence-based methods to distinguish strains of Salmonella enterica serovar Heidelberg involved in foodborne outbreaks in Quebec. Food Microbiol. 73, 99–110. doi: 10.1016/j.fm.2018.01.004
Wachsmuth, I. K., Kiehlbauch, J. A., Bopp, C. A., Cameron, D. N., Strockbine, N. A., Wells, J. G., et al. (1991). The use of plasmid profiles and nucleic acid probes in epidemiologic investigations of foodborne, diarrheal diseases. Int. J. Food Microbiol. 12, 77–89. doi: 10.1016/0168-1605(91)90049-U
Wattiau, P., Boland, C., and Bertrand, S. (2011). Methodologies for Salmonella enterica subsp. enterica subtyping: gold standards and alternatives. Appl. Environ. Microbiol. 77, 7877–7885. doi: 10.1128/AEM.05527-11
Weigel, R. M., Qiao, B., Teferedegne, B., Suh, D. K., Barber, D. A., and Isaacson, R. E. (2004). Comparison of pulsed field gel electrophoresis and repetitive sequence polymerase chain reaction as genotyping methods for detection of genetic diversity and inferring transmission of Salmonella. Vet. Microbiol. 100, 205–217. doi: 10.1016/j.vetmic.2004.02.009
Wiedmann, M., and Nightingale, K. (2009). DNA-based subtyping methods facilitate identification of foodborne pathogens. Food Technol. 63, 44–49.
Wiedmann, M., Wang, S., Post, L., and Nightingale, K. (2014). Assessment criteria and approaches for rapid detection methods to be used in the food industry. J. Food Prot. 77, 670–690. doi: 10.4315/0362-028X.JFP-13-138
Wise, M. G., Siragusa, G. R., Plumblee, J., Healy, M., Cray, P. J., and Seal, B. S. (2009). Predicting Salmonella enterica serotypes by repetitive sequence-based PCR. J. Microbiol. Methods 76, 18–24. doi: 10.1016/j.mimet.2008.09.006
Wright, A. V., Liu, J. J., Knott, G. J., Doxzen, K. W., Nogales, E., and Doudna, J. A. (2017). Structures of the CRISPR genome integration complex. Science 357, 1113–1118. doi: 10.1126/science.aao0679
Wu, S., Ricke, S. C., Schneider, K. R., and Ahn, S. (2017). Food safety hazards associated with ready-to-bake cookie dough and its ingredients. Food Control 73, 986–993. doi: 10.1016/j.foodcont.2016.10.010
Wuyts, V., Mattheus, W., De Laminne de Bex, G., Wildemauwe, C., Roosens, N. H., Marchal, K., et al. (2013). MLVA as a tool for public health surveillance of human Salmonella typhimurium: prospective study in Belgium and evaluation of MLVA loci stability. PLoS One 8:e84055. doi: 10.1371/journal.pone.0084055
Wyres, K., Conway, T., Garg, S., Queiroz, C., Reumann, M., and Holt, K. (2014). WGS analysis and interpretation in clinical and public health microbiology laboratories: what are the requirements and how do existing tools compare? Pathogens 3, 437–458. doi: 10.3390/pathogens3020437
Xiong, D., Song, L., Pan, Z., and Jiao, X. (2018). Identification and discrimination of Salmonella enterica serovar gallinarum biovars pullorum and gallinarum based on a one-step multiplex PCR assay. Front. Microbiol. 9:1718. doi: 10.3389/fmicb.2018.01718
Xu, L., Liu, Z., Li, Y., Yin, C., Hu, Y., Xie, X., et al. (2018). A rapid method to identify Salmonella enterica serovar Gallinarum biovar Pullorum using a specific target gene ipaJ. Avian Pathol. 47, 238–244. doi: 10.1080/03079457.2017.1412084
Xu, Y., Hu, Y., Guo, Y., Zhou, Z., Xiong, D., Meng, C., et al. (2018). A new PCR assay based on the new gene-SPUL_2693 for rapid detection of Salmonella enterica subsp. enterica serovar Gallinarum biovars Gallinarum and Pullorum. Poult. Sci. 97, 4000–4007. doi: 10.3382/ps/pey254
Yoshida, C., Ahmad, A., Gurnik, S., Blimkie, T., Murphy, S. A., and Kropinski, A. M. (2016a). Evaluation of molecular methods for identification of Salmonella serovars. J. Clin. Microbiol. 54, 1992–1998. doi: 10.1128/JCM.00262-16
Yoshida, C., Kruczkiewicz, P., Laing, C. R., Lingohr, E. J., Gannon, V. P., and Nash, J. H. (2016b). The Salmonella in silico typing resource (SISTR): an open web-accessible tool for rapidly typing and subtyping draft Salmonella genome assemblies. PLoS One 11:e0147101. doi: 10.1371/journal.pone.0147101
Yoshida, C., Lingohr, E. J., Trognitz, F., MacLaren, N., Rosano, A., Murphy, S. A., et al. (2014). Multi-laboratory evaluation of the rapid genoserotyping array (SGSA) for the identification of Salmonella serovars. Diagn. Microbiol. Infect. Dis. 80, 185–190. doi: 10.1016/j.diagmicrobio.2014.08.006
Yun, Y. S., Chae, S. J., Na, H. Y., Chung, G. T., Yoo, C. K., and Lee, D. Y. (2015). Modified method of multilocus sequence typing (MLST) for serotyping in Salmonella species. J. Bacteriol. Virol. 45, 314–318. doi: 10.4167/jbv.2015.45.4.314
Zhang, S., Yin, Y., Jones, M. B., Zhang, Z., Kaiser, D. B. L., Dinsmore, B. A., et al. (2015). Salmonella serotype determination utilizing high-throughput genome sequencing data. J. Clin. Microbiol. 53, 1685–1692. doi: 10.1128/JCM.00323-15
Zheng, J., Keys, C. E., Zhao, S., Ahmed, R., Meng, J., and Brown, E. W. (2011). Simultaneous analysis of multiple enzymes increases accuracy of pulsed-field gel electrophoresis in assigning genetic relationships among homogeneous Salmonella strains. J. Clin. Microbiol. 49, 85–94. doi: 10.1128/JCM.00120-10
Zheng, J., Keys, C. E., Zhao, S., Meng, J., and Brown, E. W. (2007). Enhanced subtyping scheme for Salmonella enteritidis. Emerg. Infect. Dis. 13, 1932–1935. doi: 10.3201/eid1312.070185
Zhu, C., Yue, M., Rankin, S., Weill, F. X., Frey, J., and Dieter Schifferli, M. (2015). One-step identification of five prominent chicken Salmonella serovars and biotypes. J. Clin. Microbiol. 53, 3881–3883. doi: 10.1128/JCM.01976-15
Zou, W., Lin, W. J., Foley, S. L., Chen, C. H., Nayak, R., Chen, J. J., et al. (2010). Evaluation of pulsed-field gel electrophoresis profiles for identification of Salmonella serotypes. J. Clin. Microbiol. 48, 3122–3126. doi: 10.1128/JCM.00645-10
Keywords: Salmonella, subtyping, serotyping, WGS, PFGE, MLST, food industry
Citation: Tang S, Orsi RH, Luo H, Ge C, Zhang G, Baker RC, Stevenson A and Wiedmann M (2019) Assessment and Comparison of Molecular Subtyping and Characterization Methods for Salmonella. Front. Microbiol. 10:1591. doi: 10.3389/fmicb.2019.01591
Received: 20 March 2019; Accepted: 26 June 2019;
Published: 12 July 2019.
Edited by:
Learn-Han Lee, Monash University Malaysia, MalaysiaReviewed by:
Min Yue, Zhejiang University, ChinaDapeng Wang, Shanghai Jiao Tong University, China
Soohyoun Ahn, University of Florida, United States
Copyright © 2019 Tang, Orsi, Luo, Ge, Zhang, Baker, Stevenson and Wiedmann. This is an open-access article distributed under the terms of the Creative Commons Attribution License (CC BY). The use, distribution or reproduction in other forums is permitted, provided the original author(s) and the copyright owner(s) are credited and that the original publication in this journal is cited, in accordance with accepted academic practice. No use, distribution or reproduction is permitted which does not comply with these terms.
*Correspondence: Silin Tang, silin.tang@effem.com