- College of Animal Science and Technology, Northeast Agricultural University, Harbin, China
The objectives of this study were to investigate the effects of different forage-to-concentrate ratios and sampling times on the genetic diversity of carbohydrate-active enzymes (CAZymes) and the taxonomic profile of rumen microbial communities in dairy cows. Six ruminally cannulated Holstein cows were arbitrarily divided into groups fed high-forage (HF) or low-forage (LF) diets. The results showed that, for glycoside hydrolase (GH) families, there were greater differences based on dietary forage-to-concentrate ratio than sampling time. The HF treatment group at 4 h after feeding (AF4h) had the most microbial diversity. Genes that encode GHs had the highest number of CAZymes, and accounted for 57.33% and 56.48% of all CAZymes in the HF and LF treatments, respectively. The majority of GH family genes encode oligosaccharide-degrading enzymes, and GH2, GH3, and GH43 were synthesized by a variety of different genera. Notably, we found that GH3 was higher in HF than LF diet samples, and mainly produced by Prevotella, Bacteroides, and unclassified reads. Most predicted cellulase enzymes were encoded by GH5 (the BF0h group under HF treatment was highest) and GH95 (the BF0h group under LF treatment was highest), and were primarily derived from Bacteroides, Butyrivibrio, and Fibrobacter. Approximately 67.5% (GH28) and 65.5% (GH53) of the putative hemicellulases in LF and HF treatments, respectively. GH28 under LF treatment was more abundant than under HF treatment, and was mainly produced by Ruminococcus, Prevotella, and Bacteroides. This study revealed that HF-fed cows had increased microbial diversity of CAZyme producers, which encode enzymes that efficiently degrade plant cell wall polysaccharides in the cow rumen.
Introduction
Cellulose and hemicellulose in plant cell wall polysaccharides are the most abundant renewable resources in nature, and the development and use of these compounds is considered one of the most effective ways to alleviate energy problems, such as fossil fuels being a finite resource that produce pollution (Walia et al., 2017). The rumen is recognized as a natural bioreactor for highly efficient structural carbohydrates (e.g., cellulose and hemicellulose) degradation (Codron and Clauss, 2010) because it has a large number of microorganisms that can degrade cellulose. Moreover, the cellulase produced by microorganisms is usually considered safe, stable, and efficient for cellulose degradation (Bickhart and Weimer, 2017). Cellulose-degrading microorganisms in the rumen mainly include bacteria, fungi, and protozoa (Pope et al., 2012). Bacteria account for approximately 95% of all microorganisms (Mackie et al., 2000), and, unsurprisingly, they play a critical role in cellulose decomposition during rumen fermentation (Pang et al., 2017). Owing to the presence of numerous fiber-degrading microorganisms and enzymes, 60–65% of structural carbohydrates can be degraded within 48 h of fermentation by various microorganisms that provide nutrients for host ruminant growth and development (Wang and Duan, 2014).
Rumen microorganisms produce a series of enzymes known as carbohydrate-active enzymes (CAZymes) that can break down plant cell walls. There are four types of CAZymes that are distinguished based on protein sequence, gene sequence, and structural similarities: glycoside hydrolases (GHs), glycosyltransferases (GTs), polysaccharide lyases (PLs), and carbohydrate esterases (CEs); these CAZymes cooperatively contribute to dietary cellulose, hemicellulose, and pectin deconstruction (Lim et al., 2013; Kala et al., 2017). Furthermore, related non-enzymatic species are known as carbohydrate-binding modules (CBMs). Studies have shown that CBMs can increase the catalytic efficiency of enzymes by specifically binding polysaccharides and increasing enzyme concentration (Boraston et al., 2004; Jones et al., 2018).
Cellulose is the most important component in ruminant diets and is essential for rumen fermentation, and the rumen has developed into an effective and complex cellulose degradation system (Fox et al., 1992; Buchanan et al., 2010). This process has been the focus of metagenomic research aimed at identifying and capturing the diversity of enzyme activity. Many metagenomic studies have reported CAZyme diversity in different ruminants, such as Holstein–Friesian crossbred steers (Jose et al., 2017a), Angus cattle (Brulc et al., 2009), buffalo (Patel et al., 2014), and Saudi sheep (Almasaudi et al., 2017). Nine endoglucanases, 12 esterases, and one cyclodextrinase were detected from the rumen metagenomic library of dairy cows (Ferrer et al., 2010). Subsequently, several specific polysaccharide-degrading enzymes were isolated from the rumen using metagenomics techniques (Math et al., 2010; Huoqing et al., 2011). Additionally, most research has been conducted on CAZymes and digestive microbiota, but few studies have evaluated the effects of different forage-to-concentrate ratios and sampling time on the genetic diversity of CAZymes and taxonomic profile of rumen microbial communities in dairy cows. Therefore, this study was designed and carried out to explore CAZyme diversity and characteristics under different forage-to-concentrate ratios and sampling times, and identify the microbes that produce CAZymes.
Materials and Methods
This study was carried out in accordance with the regulations of Instructive Notions with Respect to Caring for Experimental Animals, Ministry of Science and Technology of China. The protocol was approved by the Ethical Committee of the College of Animal Science and Technology of Northeast Agricultural University.
Experimental Design, Animals Feeding, and Sample Collection
Six ruminally cannulated Holstein cows that averaged 3.2 ± 0.70 (mean ± SE) years of age were used in this experiment. Cows were housed in individual tie stalls. The treatments contained 70% (high-forage, HF) and 30% (low-forage, LF) dietary forage (dry matter basis), respectively. For 3 weeks before sampling, animals were fed once daily at 8:00 AM and allowed ad libitum consumption of 110% of their expected intake. The ingredient and nutritional composition of the two diets are presented in Table 1. Rumen content samples were collected before feeding (i.e., at 0 h, BF0h) and 4 h after feeding (AF4h) via a ruminal fistula. Collective representative samples of ruminal contents from each animal were extruded through four layers of cheesecloth. One part of each homogenized pellet was mixed with RNAlater (Ambion, Austin, TX, United States), which is a reagent that protects and stabilizes bacterial RNA, and the rest of each pellet was used for DNA extraction and enzyme activity determination. All samples were placed in liquid nitrogen within 5 min, and then taken to the laboratory and stored at -80°C until further testing.
Enzyme Activity Analysis
For the enzyme activity assay, frozen pellets were thawed at room temperature. After being centrifuged at 3000 g for 10 min (4°C), 10–15 mL of supernatant was taken for sonication (power, 400 W; crushed three times for 30 s each time at 30 s intervals), and the crushed liquid was subsequently tested. The assayed CMCase, β-glucosidase, xylanase, and β-xylosidase activity was measured using the 3,5-dinitrosalicylic acid method (Miller et al., 1960; Yang and Xie, 2010).
RNA Extraction, RNA Reverse Transcription, and qPCR Primer Design and Analysis
RNA extraction was performed using the liquid nitrogen grinding method and TRIzol reagent (Ambion, Carlsbad, CA, United States) following the protocols described by Kang et al. (2009) with some modifications. The RNA was reverse-transcribed into cDNA using a PrimeScriptTM 1st strand cDNA Synthesis Kit (Code No. 6110A, Takara, Dalian, China), following the kit instructions. The reverse-transcribed PCRs were conducted as follows: 37°C for 15 min, 85°C for 5 s, and 4°C for 10 min. The cDNA was stored at -80°C. The PCR primers used are listed in Table 2 and were assembled based on previous literature (Khafipour et al., 2009; Edwards et al., 2010). Primers were provided by Sangon Biotech Co., Ltd., (Shanghai, China). The Real-Time qPCR performed using Takara SYBR® Premix Ex TaqTM Synthesis Kit (Code No. RR420A, Takara, Dalian, China), following the kit instructions. Abundance of these microbes were expressed as a proportion of total estimated rumen bacterial 16S rDNA according to the equation: relative quantification = 2-(Cttarget-Cttotalbacteria), where Ct represents threshold cycle (Guo et al., 2010).
DNA Extraction, 16S rRNA Gene Amplicon Preparation, and Sequencing
Genomic DNA was extracted following the protocols described by An et al. (2005) with some modifications. DNA extraction was performed using a CTAB-based DNA extraction method. The CTAB lysis buffer contained 2% w/v CTAB (Sigma-Aldrich, Poole, United Kingdom), 100 mM Tris–HCl (pH = 8.0; Fisher Scientific, Fair Lawn, NJ, United States), 20 mM EDTA (pH = 8.0; Fisher), and 1.4 M NaCl (Fisher Scientific). The lysis buffer pH was adjusted to 5.0 prior to sterilization by autoclaving (Doyle and Dickson, 1987). The final DNA was resuspended in 100 μL TE buffer (pH = 8.0; Sigma-Aldrich) and stored at -80°C.
The DNA concentration in each sample was measured using a NanoDrop ND-1000 Spectrophotometer (NanoDrop Technologies, Inc., Wilmington, DE, United States). The integrity of extracted DNA was verified by agarose (1.5%) gel electrophoresis. Subsequently, the V3–V4 region of the 16S rRNA gene was amplified using the following primers: forward 5′-ACT CCT ACG GGR SGC AGC AG-3′ and reverse 5′-GGA CTA CVV GGG TAT CTA ATC-3′ (Xie et al., 2018). The PCRs were performed using the Applied Biosystems Veriti Thermocycler (Thermo Fisher Scientific Co., Ltd., Shanghai, China) in a 20-μL reaction volume. Thermocycling parameters were as follows: initial denaturation at 95°C for 2 min; 30 cycles of further denaturation at 95°C for 15 s, annealing at 50°C for 30 s, and extension at 68°C for 1 min; and a final extension at 68°C for 7 min. All PCRs were performed in triplicate, and products were combined. PCR product integrity was verified by agarose (1.5%) gel electrophoresis, and PCR products were purified with the QIAquick Gel Extraction Kit (Qiagen, Venlo, Netherlands). The concentrations of PCR products were measured using a NanoDrop ND-1000 Spectrophotometer (NanoDrop Technologies, Inc., Wilmington, DE, United States) and subsequently pooled in equal proportions based on DNA concentration. The purified 16S rRNA gene amplicons was sequenced using the paired-end method by Illumina Hiseq 2500 system. The resulting sequences were then screened and filtered for quality and length. Sequences with short reads were extended by merging paired-end reads using FLASH v1.2.7 (Magoc and Salzberg, 2011). Any read pairs that could not be assembled and any single reads were discarded. Sequences were trimmed, quality-filtered and de-convoluted based on the 12 bp barcode sequence. Chimeras were identified and removed using UCHIME v4.2 to obtained effective tags (Edgar, 2010). Subsequently, the sequences were processed and analyzed using Quantitative Insights into Microbial Ecology (QIIME, v1.8.0) as described by Caporaso et al. (2010b). The high-quality sequences were clustered into operational taxonomic units (OTUs) defined by 97% similarity. Taxonomy assignment of representative sequences from each OTU were performed by Ribosomal Database Project classifier (Wang et al., 2007) against its reference database (Cole et al., 2014) with confidence cutoff 0.8. Then representative sequences were aligned against the SILVA bacterial database (SILVA version 128) using PyNAST (Caporaso et al., 2010a). Singletons were removed before further analysis (Bokulich et al., 2013).
Metagenome Library Preparation and Sequencing
Qualified DNA samples were first cut into smaller fractions by nebulization. Then, using T4 DNA polymerase, the Klenow fragment and T4 polynucleotide kinase convert the fragmentation-produced overhang into blunt ends. After the adenine (A) base was added to the 3′ end of the blunt-ended phosphorylated DNA fragments, the adaptor was ligated to the end of the DNA fragment. Ampure beads were used for purification and elimination the short fragments. PCR amplification were performed to enrich the adapter-ligated DNA fragments. Then, the PCR products were purified with an AxyPrep Mag PCR clean up kit (Axygen, Corning, NY, United States) following the manufacturer’s recommendations. Sample libraries were quantified and analyzed using the Agilent 2100 Bioanalyzer and the ABI StepOnePlus Real-Time PCR system. The qualified libraries were then sequenced on the Illumina HiSeqTM platform.
Metagenome Assembly and Bioinformatic Analysis
SOAPdenovo2 was used to reassemble high-quality data (Luo et al., 2012). SOAPdenovo results were further assembled with Rabbit to obtain longer contigs (You et al., 2013). For each sample, the reads were assembled in parallel with a series of different k-mer sizes. SOAP2 was used to map the reads back to each assembly result, and selected the optimal k-mer size and assembly results based on contig N50 and mapping rate (Li et al., 2009). Based on the assembly results, MetaGeneMark v2.10 (Tang and Borodovsky, 2010) using default parameters1 predicted the presence of open reading frames. Genes from different samples were combined by CD-Hit clustering (Li and Godzik, 2006) (sequence identity threshold, 95%; alignment coverage threshold, 90%).
CAZyme Annotation and Taxonomic Profiling
The CAZy gene encoding contigs from the metadata were identified and classified based on the CAZymes database (Cantarel et al., 2009)2 by the carbohydrate-active enzyme analysis toolkit (CAT) (Park et al., 2010) at an E-value of 1 × 10-5. Putative plant cell wall polysaccharide-degrading enzymes belonging to different CAZy families were identified and classified based on sequence-based annotation. The CAZyme encoding contigs were analyzed manually for different classes of CAZymes: GHs, GTs, CEs, CBMs, and PLs. Subsequently, the CAZy results obtained were analyzed manually to determine the proportions of the different CAZymes present in the rumen metagenome data.
The CAZy results of the gene were searched against the sequences in the NR database using the BLASTP algorithm with an E-value cutoff of 1 × 10-5, and the best hits were subjected to analysis with Metagenome Analyzer (MEGAN) (Huson et al., 2011), a program for taxonomic analysis, which could accurately classify DNA sequences as short as 100 bp.
Pyrosequencing Data Accession Number
The Illumina sequencing raw data for our samples have been deposited in the NCBI Sequence Read Archive (SRA) under accession number: PRJNA522848 (Metagenome) and PRJNA45088 (16S rRNA).
Statistical Analysis
Community richness and diversity, such as Chao1, and Shannon indices, which are used to illustrate significant differences among samples, were assessed by the program MOTHUR v.1.35.0 (Schloss et al., 2009). The statistical significances were tested by Kruskal–Wallis H-test adjusted with false discovery rate, using R (R Core Team, 2016) facilitated with agricolae package (Mendiburu, 2016). The statistical significance was declared at 0.01 < P-value < 0.05 “∗”, and P-value < 0.01 “∗∗”. Beta diversity was measured according to Bray–Curtis distances which were calculated by QIIME, and displayed using Principal Coordinate Analysis (PCoA). The significance of grouping in the PCoA plot was tested by analysis of similarity (ANOSIM) in QIIME with 999 permutations (R Core Team, 2016).
The percentage of GH data for each group relative to total GHs identified, enzyme activity, and real-time PCR quantification results were analyzed using the PROC MIXED procedure in SAS 9.4 (SAS Institute Inc.), which included feed, time, and feed × time as the fixed effects, and individual group as the experimental unit.
Results
Rumen Metagenome Sequence Data Statistics and Rumen Bacterial Diversity
Metagenome sequencing of the total DNA from 12 rumen samples generated approximately 9.01 gigabases of raw sequence data. The statistical elements of the assemblies were calculated and the metagenomic data analysis statistics are provided in Table 3. At the domain level, evolutionary analysis revealed that ∼95 and ∼90% of sequences were binned to bacteria, ∼0.05 and ∼0.10% to archaea, and ∼0.08 and ∼0.05% to eukaryotes in LF and HF treatments, respectively (Supplementary Table S1). There were 17 bacterial phyla identified in the rumen samples. Among these phyla, Bacteroidetes, Firmicutes, Proteobacteria, Tenericutes, and Verrucomicrobia were the dominant phyla (Supplementary Table S1), with increasing dietary forage levels, the relative abundance of Bacteroidetes and Verrucomicrobia increased. As the sampling time increased, the relative abundance of Bacteroidetes decreased, whereas that of Tenericutes and Verrucomicrobia increased.
In this study, the rumen bacterial alpha diversity was measured by Chao 1 and Shannon indices for different dietary treatments before (0 h) and after (4 h) feeding. The Chao 1 index of HF treatment was significantly (P < 0.01) higher compared with that of the LF treatment, and a similar pattern was shown by Shannon indices (Figures 1A,B). Under HF treatment, the Chao 1 index for HF4h was higher than that for HF0h. However, there was no difference in Shannon indices between the two HF groups. This result indicated that forage can increase bacterial richness and diversity. The beta diversities of bacterial communities for different diets before and after feeding were calculated and visualized by PCoA using the Bray–Curtis distance (Figure 1C). The bacterial communities were distinct between HF and LF treatments, and the samples in HF0h and HF4h groups of HF treatment clustered based on different time groups were significant distinction (Figure 1D).
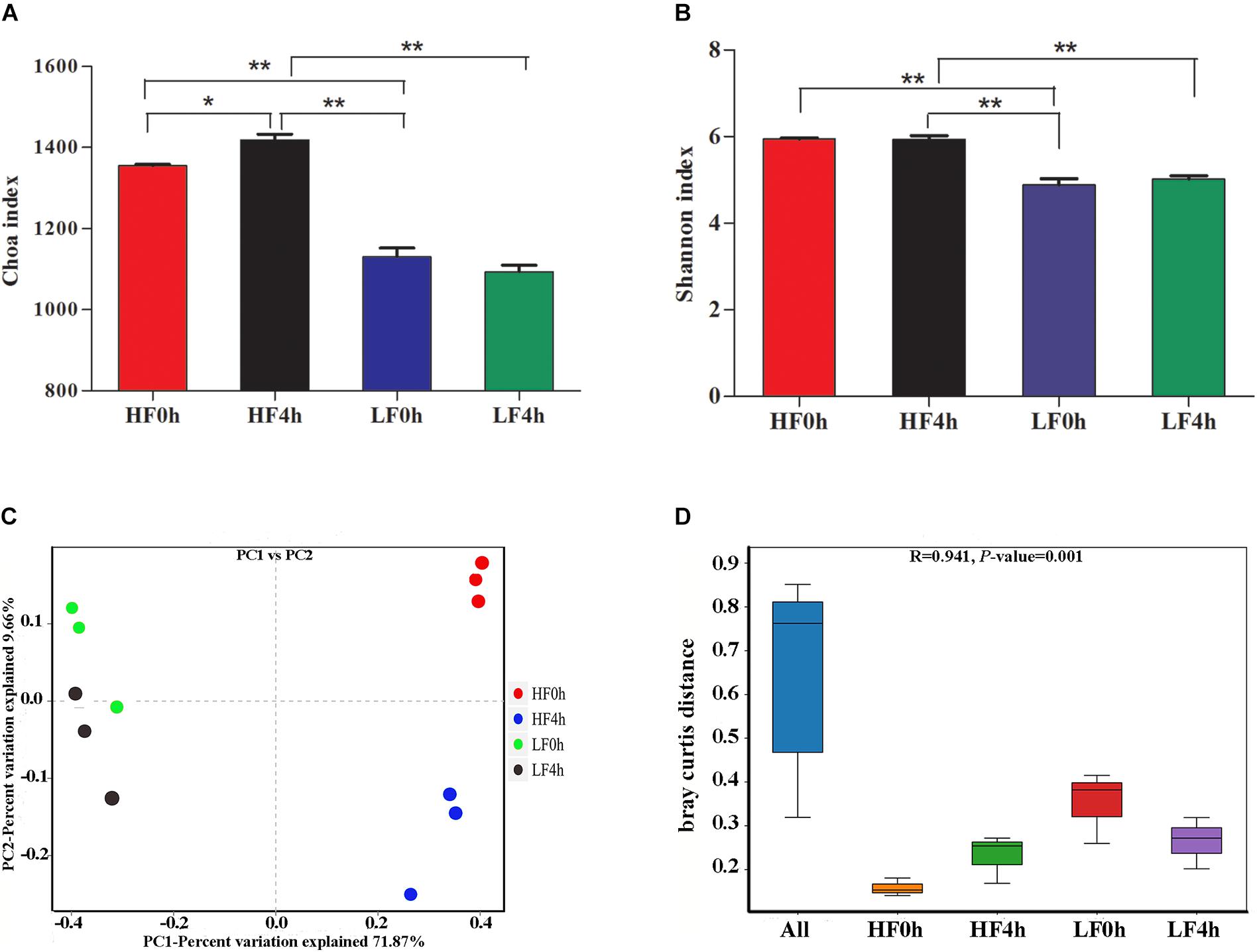
Figure 1. (A) Chao 1 indices of alpha diversity base on OUT level. (B) Shannon indices of alpha diversity base on OUT level. (C) Beta diversity: principal coordinate analysis (PCoA) of bacterial community structure based on Buray–Curtis distances for two treatments of before and after feeding. (D) ANOSIM analysis of bacterial community structure based on Buray–Curtis distances for two treatments of before and after feeding. ∗(0.01 < P < 0.05); ∗∗(P < 0.01).
Rumen Metagenome Mapping for CAZymes and Microbial Composition
A total of 43,630 putative homology-based contigs were inferred with MetaGeneMark and analyzed using the CAZymes analysis toolkit (CAT, see text footnote 2) (Cantarel et al., 2009). CAZymes were determined to belong to different classes (GHs, GTs, CBMs, CEs, and PLs) by CAT are shown in Table 4. GT and CE family abundances (25 and 15 families, respectively) were significantly affected by feed, and GT family abundance was higher under LF treatment than HF treatment (Table 4 and Supplementary Table S2); however, the CE families showed a reverse pattern. The GT families were the second-most abundant in CAZymes; of the 25 GT families identified in this study, enzymes of the GT2 and GT4 families contributed a large proportion (>65%) of the total GTs (Table 4 and Supplementary Table S2). Eight PL families were detected by CAT analysis, and a significant interaction between feed and time was observed for PLs. The abundance of GHs was not affect by diet and time (Table 5), but these families were the most abundant in the rumen metagenomes of CAZymes and included 78 different families (Supplementary Table S2), which accounted for approximately 57% (average of the four groups) of the enzymes categorized in the CAZy database (Table 5). Moreover, 28 CBM families were also detected by CAT analysis.
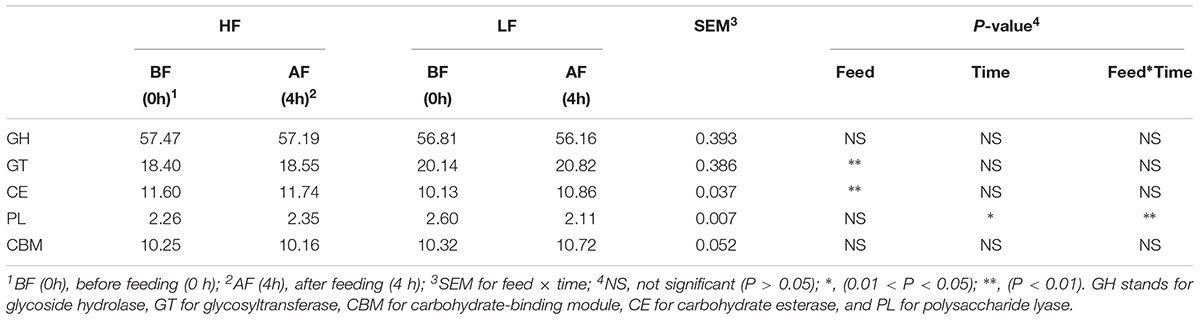
Table 4. Percentage of CAZymes contributed by GH, GT, CBM, CE, and PL (all CAZymes were collectively considered 100%).
Phylogenetic analysis of CAZyme contigs showed that Prevotella and Bacteroides primarily contributed CAZyme-encoding gene fragments of the GH, GT, CBM, CE, and PL families in the Holstein cow rumen metagenome (Figure 2). The number of enzymes that belonged to Prevotella was significantly higher in LF than HF groups; the reverse was observed for Bacteroides. Alistipes was found in all five categories, with its highest abundance in the CBM family of the HF groups, followed by the GH, GT, PL, and CE families of the HF groups (Figure 2). The number of enzymes from Ruminococcus, one of the most dominant cellulolytic bacteria of the GH families, was higher under HF treatment than LF treatment (Figure 2). Butyrivibrio and Fibrobacter were detected in the GH families, and were higher under HF treatment than LF treatment (Figure 2). Prevotella, Bacteroides, Alistipes, Clostridium, and Ruminococcus were found in all five CAZyme categories and were the primary contributors of CAZymes.
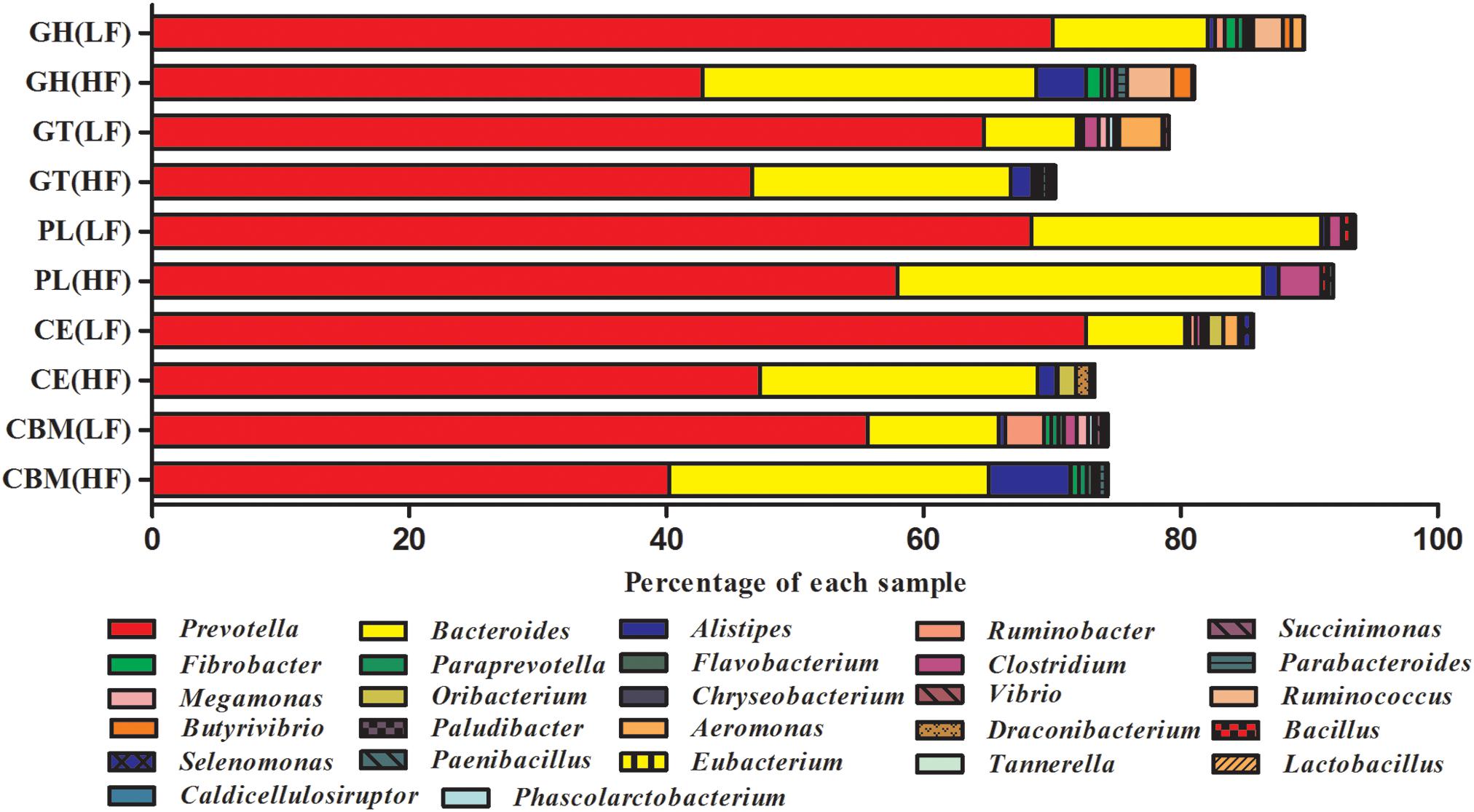
Figure 2. Percent contributions of CAZymes from the major microbial communities in cattle rumens. Each graph shows the abundance of 15 genera that are the major contributors of CAZymes to the Holstein cow rumen ecosystem. GH stands for glycoside hydrolase, GT for glycosyltransferase, CBM for carbohydrate-binding module, CE for carbohydrate esterases, and PL for polysaccharide lyase. All genera in each sample totaled 100%.
Comparison of GH Families of Cows Fed Two Different Diets
Out of the 78 GH families identified, 39 were reported to be involved in the direct degradation of plant fiber (Table 5). Four GH families (GH5, GH9, GH88, and GH95) were mainly found to be associated with cellulolytic functions, and represented 7.96 and 8.53% (average of BF0h and AF4h) of the total GHs under HF and LF treatments, respectively. The abundances of GH5, GH9, and GH88 were significantly affected by diets, and GH5 was also affected by feed and time interaction.
Five GH families (GH8, GH10, GH26, GH28, and GH53) were detected in our study, and have important roles in hemicellulose degradation (Table 5). Time treatment did not affect GH8, GH10, GH26, GH28, and GH53, but GH8 and GH28 were significantly (P < 0.01) affected by feed. Percentage of GH10 was also significantly affected (P < 0.01) by interaction between feed and time. The pivotal series of enzymes that are responsible for hydrolysis of the main chain of galactooligosaccharides, such as galacturonases and endo-1,4-galactanase, were present in the GH28 and GH53 families. These enzymes represented approximately 66% (average of the four groups) of the endo-hemicellulases, which were more abundant under LF treatment; however, there was no difference between the LF0h and LF4h groups.
Oligosaccharide-degrading enzymes had a greater abundance of GH families than other cellulose-degrading enzymes, and represented 60.64, 55.81, 58.73, and 59.25% of the total GHs in the LF0h, LF4h, HF0h, and HF4h groups, respectively (Table 4). No noticeable differences of the GH31, GH39, and GH97 families were observed between HF and LF treatments; however, the other GH families of oligosaccharide-degrading enzymes were affected by feed (Table 5). Among them, GH38, GH42, and GH94 were also affected by time and interaction between feed and time. Among the GHs responsible for oligosaccharide degradation (oligo-GHs), GH2, GH3, GH13, GH31, and GH43 were the main GHs, which accounted for ∼70% of all oligo-GHs (Table 5). Of those GHs, GH3 was the most abundant (20.63, 22.22, 18.42, and 18.90% of total oligo-GHs in the LF0h, LF4h, HF0h, and HF4h groups, respectively). The second most abundant was the coding endoglucanase GH43, and the abundance was significantly (P < 0.01) higher under LF treatment than HF treatment.
Debranching enzymes were also identified by CAT analysis and belonged to the GH23, GH33, GH51, GH54, GH67, GH78, and GH127 families; of these, GH51, GH67, and GH78 were more abundant and have the function of α-L-arabinofuranosidases, α-glucuronidases, and α-L-rhamnosidases, respectively (Table 5). GH51 was the most abundant, but was not affected by feed, time, or the interaction between feed and time. GH78 was significantly (P < 0.01) more abundant under HF treatment than LF treatment.
Enzyme Activity of Cows Fed Two Different Diets
Endo-1,4-glucanase (the CMCase) and β-glucosidase were the main cellulases. In this study, the CMCase was affected by time, and at 0 h before feeding had significantly higher (P < 0.01) activity than at 4 h after feeding (Table 6). HF-fed cows had greater β-glucosidase activity than LF-fed cows. β-glucosidase activity at 4 h after feeding was significantly (P < 0.01) higher than that at 0 h before feeding, and β-glucosidase activity was also affected by a significant (P < 0.01) interaction between feed and time (Table 6). Xylanase and β-xylosidase are the main hemicelluloses. Xylanase activity in HF-fed cows was significantly (P < 0.01) higher than that in LF-fed cows, and at 4 h after feeding was significantly (P < 0.01) decreased compared with at 0 h before feeding. Dietary treatment did not affect β-xylosidase activity, but substantial variation was observed between the treatments. For example, β-xylosidase activity ranged from, on average, 3.49 U in HF-fed cows to 3.04 U in LF-fed cows.
Fiber-Degrading Bacteria Characterization and Predominant Fiber-Degrading Bacteria Relative Quantification
After statistical analysis, all genus-level data were converted back into CAZyme (GH) percent relative abundance are presented in Table 7. The cel-GH (GH families responsible for cellulose degradation) reads mainly originated from Bacteroides and Prevotella, and were also affected by feed. Our data showed that Fibrobacter content was the highest, and these bacteria play an important role in cellulose degradation. Butyrivibrio relative abundance was affected by time, and was higher at 4 h after feeding than at 0 h before feeding.
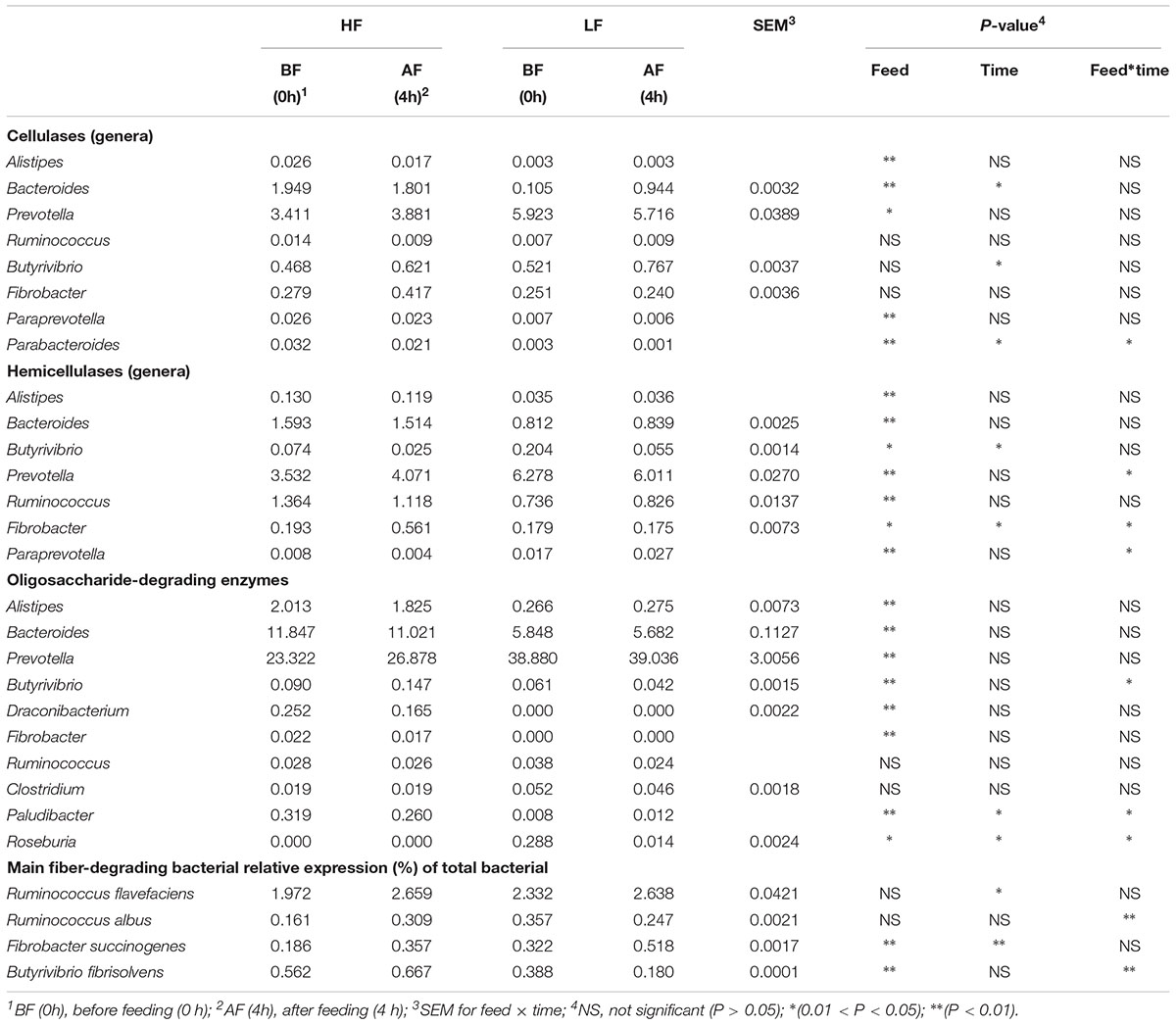
Table 7. Taxonomic affiliation of putative cellulase, hemicelluase, and oligosaccharide-degrading enzymes.
The majority of the hemi-GH (GH families responsible for hemicellulose degradation) reads in samples originated from Bacteroides, Prevotella, and Ruminococcus (Table 7), which were significantly affected by feed, and Bacteroides and Prevotella abundances were higher under LF treatment than under HF treatment. Under HF treatment, Fibrobacter relative abundance was higher than that under LF treatment, and after feeding was higher compared with before feeding; there was a significant (P < 0.05) interaction between feed and time related to Fibrobacter relative abundance.
The oligo-GHs (GHs responsible for oligosaccharide degradation) contained the most bacterial genera and the greatest relative abundance compared with cel-GHs and hemi-GHs (Table 7). The bacterial genera were primarily Bacteroides and Prevotella, and the relative abundances were significantly (P < 0.01) higher compared with that of cel-GHs and hemi-GHs. Bacteroides and Prevotella were the main contributors to oligosaccharide degradation, followed by Alistipes, Draconibacterium, Paludibacter, and Clostridium. Based on our data, the relative abundances of Butyrivibrio, Fibrobacter, and Ruminococcus, which were the major producers of cel-GH, appeared to contribute little to oligo-GH hydrolysis.
Abundance of the predominant fiber-degrading bacteria was measured by Real-Time qPCR quantification in two treatments, both before or after feeding. Ruminococcus flavefaciens and R. albus bacteria were derived from Ruminococcus. Based on cDNA level, the R. flavefaciens activity at 4 h after feeding was significantly higher compared with that at 0 h before feeding. Fibrobacter succinogenes activity was significantly affected by feed and time. Butyrivibrio fibrisolvens activity was significantly (P < 0.01) affected by feed, and a significant (P < 0.01) interaction between feed and time was observed for R. albus and B. fibrisolvens activity.
Discussion
The rumen is a complex ecosystem that harbors a wide variety of microorganisms. Bacteroidetes, Firmicutes, and Proteobacteria were the most predominant bacteria in the rumen, which was recognized by most previous studies (Dai et al., 2015; Wang et al., 2017; Zhang et al., 2017). Martinezgarcia et al. (2012) showed that Verrucomicrobia could hydrolyze diverse polysaccharides. In the present study, Verrucomicrobia was significantly higher in the HF group than in the LF group, which might indicate that Verrucomicrobia plays an important role in the degradation of plant cell wall polysaccharides.
Linking phylogeny to function is a recurrent question in microbiology of rumen. A growing number of rumen metagenomic studies have shown physical and chemical properties of functional genes in rumen samples, which provided a way to evaluate the relationship of phylogeny to function (Hess et al., 2011; Singh et al., 2015). Therefore, analysis of CAZyme-regulating gene abundance and categories in cow rumens could help characterize fiber degradation. Metagenomic analysis of microbial consortia enriched in the rumen showed that GH families were most abundant.
Rumen Metagenome Mapping for CAZymes
The GHs comprise a large group of enzymes involved in the metabolism of polysaccharides such as starch, cellulose, xylan, and chitin (Stewart et al., 2018). Because of the most abundance and wide distribution of GH-encoding genes across genomes, this enzyme class is the best characterized of the CAZymes (Berlemont and Martiny, 2015). GTs were the second most abundant CAZy family in the Holstein cow rumen, and were reported to catalyze the activated oligosaccharides or glycosidic bonds to different receptors (e.g., proteins, nucleic acids, oligosaccharides, lipids, and small molecules) (Lairson et al., 2008). The results of this study showed that the GTs family abundance was higher under LF treatment and was significantly affected by feed. 25 GT families were identified in the rumen metagenome, and GT2 and GT4 family enzymes were a large proportion (>65%) of the total GTs (Supplementary Table S2); these results were similar to those for Indian crossbred cattle (Jose et al., 2017b). The results showed that the CE families was higher in HF treatment than that in LF treatment. The CE1 family made up 36.30 and 30.60% of all CE families in the rumen of HF- and LF-fed cows, respectively. These results indicate that the greater abundance of CE families under HF treatment was caused by the presence of the CE1 family. Feruloyl esterase, which is encoded by the CE1 family, is essential for plant fiber degradation and is the predominant member of the CE family (Biely, 2012). PLs, which cleave glycosidic bonds in acidic polysaccharides (Stewart et al., 2018), were the least abundant CAZyme classes in the cow rumen metagenome, and this result was consistent with that of Jose et al. (2017a).
GH Family Composition and Diversity
The GH families include enzymes that hydrolyze glycosidic bonds by various glucosides or oligosaccharides, and was the most abundant family among those included in the CAZy databases (Solden et al., 2018). Among the 156 GH families in the CAZy database3, 78 families were presence in the Holstein cow rumen metagenome in this study, which indicates that the Holstein cow rumen might undergo an intricate process to break down plant cell wall polysaccharides. Our results revealed that genes that encoded cellulases (cel-GH) mainly belonged to the GH5, GH9, GH88, and GH95 families, which was also determined by previous studies (Wang et al., 2013; Patel et al., 2014; Solden et al., 2018). Among cel-GHs, the GH5 family was the most abundant, and GH5 is well known with activities of β-1,4-endoglucanase and β-1,4-endomannanase, and GH9 also have endoglucanase activity (Maharjan et al., 2018). Naas et al. (2014) predicted that the GH5 and GH9 families could break down cellulose into cellobiose and act on β-(1,4)-linked glucose units in amorphous cellulose and β-glucan. In this study, endoglucanase (CMCase) activity was higher under LF treatment than HF treatment (Table 6), and was similar to GH5 and GH9 relative abundances.
There are five GH families that are degrading hemicellulose and belong to hemicellulase, referred to as hemi-GHs. Among hemi-GH, GH28 family was the most abundant, followed by GH53, and the relative abundant of both under LF treatment was higher than under HF treatment. Polygalacturonase were dominated belong to GH28 and acting α-1,4-glycosidic bond, which plays an important role in pectin digestion (Zhao et al., 2014). GH8 representing the endoxylanses has been found to be the most critical hydrolase and in xylan hydrolase system and it hydrolyzes xylan into oligosaccharides and xylodisaccharides. Then β-xylosidase hydrolyzes oligosaccharides and xylodisaccharides into xylose. In present study, the content of xylose in HF treatment was higher than in LF treatment (Table 6, β-xylosidase). Our results indicate that xylose has feedback inhibition on endoxylanase. Putative arabinogalactan end-1,4-β-galactosidase gene were found belong to GH53, and degrading the hemicellulose side chains and pectin. The large number of CAZy genes found is indicative of the potential of various rumen bacteria to utilize carbohydrates as their main substrates (Supplementary Table S3).
Patel et al. (2014) reported that oligosaccharide-degrading enzymes in buffalo were more abundant by approximately 64%, with GH43 being the most abundant family, followed by GH3. The GH3 family encodes β-glucosidase, xylan, β-1,4-xylosidase, and glucosylceramidase. β-glucosidase play an central role both in the production of glucose and, crucially, in the alleviation of the product inhibition of cellobiose during the cellulose degradation process itself (Agirre et al., 2016). In this study, among the four groups, β-glucosidase was the highest in the LF 4h group, this indicates that the LF 4h group has more glucose, then, glucose is uptaken by rumen microbes and rapidly degraded into volatile fatty acids by enzymes, which provides energy for the host and have lowest pH (Kuruti et al., 2017). The function of GH3 indicates that it is a rate-limiting enzyme in the rumen cellulose degradation process.
Debranching enzymes, such as β-xylosidase, α-larabinofuranosidase, and arabinanase, are crucial components of hemicellulolytic enzyme that promote endo-enzymes acting on their substrate (Comtetmarre et al., 2017). GH51 (α-l-arabinofuranosidases), GH67 (α-glucuronidases), and GH78 (α-L-rhamnosidase) were the main debranching enzymes (Patel et al., 2014; Dai et al., 2015; Jose et al., 2017a). In our study, GH51 (α-L-arabinofuranosidases/endoglucanase) was the most abundant debranching enzyme, which indicated that was the main debranching enzyme.
Microbial Community Analysis of Putative CAZyme Contigs and Cellulase Degradation
In this report, we present a metagenomic analysis of the fiber-degrading microbiomes of cows fed two different diets. Previous studies showed that rumen microbiota play vital roles in plant fiber degradation (Kittelmann and Janssen, 2011; Li et al., 2012). At the genus level, the comparative abundances of the 15 most abundant genera among GHs, GTs, CEs, PLs, and CBMs are shown in Figure 2 and indicate that Prevotella and Bacteroides contribute a significant proportion of CAZymes under LF treatment, which is consistent with the findings of Stewart et al. (2018). Prevotella and Bacteroides both belong to the phylum Bacteroidetes, and Solden et al. (2018) reported that Bacteroidetes play an important role in rumen carbon degradation, and the abundance of Bacteroides increased with the increase of fiber in diets (Pitta et al., 2014). The results of this study showed that the Prevotella abundance was highest in GHs, GTs, CEs, PLs, and CBMs, and significantly higher in LF treatment than HF treatment, which was consistent with the findings of previous studies (Bekele et al., 2010), and indicated that they might be the essential microorganisms and maintained normal digestive function of the rumen. Alistipes in HF groups was more abundant in CAZyme class GHs and CBMs than in LF groups. He et al. (2015) showed that a reduction in the amount of Alistipes and Bacteroides is known to be associated with low-carbohydrate diets.
In the rumen, Bacteroides, Butyrivibrio, Ruminococcus, and Fibrobacter were the dominant fibrolytic microorganisms. The enzymes produced by these microbial communities are reported to have the potential to digest plant polymers, such as cellulose, hemicellulose, and oligosaccharide-degrading enzymes (Whitman, 2015; Díaz et al., 2017). In present study, the results of Bacteroides relative abundances further confirmed that Bacteroides was the main producer of oligosaccharide-degrading enzymes. Meanwhile, Ruminococcus relative abundance in hemicellulases was higher under HF treatment than LF treatment, which indicates that Ruminococcus may play an important role in hemicellulose degradation. Prevotella abundance was relatively highest in oligosaccharide-degrading enzymes (Table 7). This could be explained by the fact that Prevotella can degrade and utilize starch and plant cell wall polysaccharides, such as xylan and pectin, but cannot degrade cellulose (Zened et al., 2013). Alistipes was higher in oligosaccharide-degrading enzymes and significantly affected by feed. The results of this study indicated that Alistipes are associated with carbohydrate, especially oligosaccharide metabolism, as previously reported (Wang et al., 2017). We also found that Draconibacterium and Paludibacter were associated with oligosaccharide-degrading enzymes, and with high relative abundance (Supplementary Table S3). Paludibacter is involved in oligosaccharide degradation in plants (Ghanbari et al., 2016). Draconibacterium, which belongs to phylum Bacteroidetes, encodes a variety of enzymes and proteins required for glycolysis, the Krebs cycle, the pentose phosphate pathway, and oxidative phosphorylation, which reflects the integrity of the Draconibacterium metabolic pathway (Li et al., 2016), which could explain the Draconibacterium was the main producer of oligosaccharide-degrading enzymes. Furfure work should investigate the glycan-degrading abilities of these different bacteria to determine if the bacteria evolved to specialize on different diets.
Conclusion
In conclusion, this study investigated individual changes in CAZymes, and microbial composition variation in response to the change of diet and time. We revealed that dietary treatment has significant effects on the CAZymes in cattle rumens. The dominant phyla and genera composition of the CAZymes varied among the four groups, the Bacteroides, Fibrobacter, and Ruminococcus largely increased as forage increased, and were identified as the key contributors of CAZymes, which indicated that the disparity amongst these two factors should be taken into account when exploring the CAZymes and related microbial composition. Therefore, this study can enhance our understanding of a biomass conversion system and demonstrates that numerous enzymes are involved in cellulose degradation in the cow rumen, which contributes to the improvement of forage utilization in ruminant nutrition.
Ethics Statement
All animal studies were conducted according to the animal care and use guidelines of the Animal Care and Use Committee of Animal Science and Technology College, Northeast Agricultural University.
Author Contributions
LW and YZ designed the research. LW conducted the research. GZ and HoX analyzed the data. LW and HaX wrote the manuscript. All authors approved the final manuscript.
Funding
This research was supported by the Agricultural Research System of China (Grant No. CARS-36) and the National Natural Science Foundation of China (Grant No. 31702135).
Conflict of Interest Statement
The authors declare that the research was conducted in the absence of any commercial or financial relationships that could be construed as a potential conflict of interest.
Acknowledgments
We thank Yonggen Zhang and Huangshu Xin at the Research and Development Centre for their technical assistance.
Supplementary Material
The Supplementary Material for this article can be found online at: https://www.frontiersin.org/articles/10.3389/fmicb.2019.00649/full#supplementary-material
Footnotes
- ^http://exon.gatech.edu/GeneMark/
- ^http://www.cazy.org
- ^http://www.cazy.org/Glycoside-Hydrolases.html
References
Agirre, J., Ariza, A., Offen, W. A., Turkenburg, J. P., Roberts, S. M., Mcnicholas, S., et al. (2016). Three-dimensional structures of two heavily N-glycosylated Aspergillus sp. family GH3 β- d -glucosidases. Acta Cryst. 72, 254–265. doi: 10.1107/S2059798315024237
Almasaudi, S., El Kaoutari, A., Drula, E., Almehdar, H., Redwan, E. M., Lombard, V., et al. (2017). A metagenomics investigation of carbohydrate-active enzymes along the gastrointestinal tract of Saudi sheep. Front. Microbiol. 8:666. doi: 10.3389/fmicb.2017.00666
An, D., Dong, X., and Dong, Z. (2005). Prokaryote diversity in the rumen of yak (Bos grunniens) and Jinnan cattle (Bos taurus) estimated by 16S rDNA homology analyses. Anaerobe 11, 207–215. doi: 10.1016/j.anaerobe.2005.02.001
Bekele, A. Z., Satoshi, K., and Yasuo, K. (2010). Genetic diversity and diet specificity of ruminal Prevotella revealed by 16S rRNA gene-based analysis. FEMS Microbiol. Lett. 305, 49–57. doi: 10.1111/j.1574-6968.2010.01911.x
Berlemont, R., and Martiny, A. (2015). Genomic potential for polysaccharide deconstruction in bacteria. Appl. Environ. Microbiol. 81, 1513–1519. doi: 10.1128/AEM.03718-14
Bickhart, D. M., and Weimer, P. J. (2017). Symposium review: host-rumen microbe interactions may be leveraged to improve the productivity of dairy cows. J. Dairy Sci. 101, 7680–7689. doi: 10.3168/jds.2017-13328
Biely, P. (2012). Microbial carbohydrate esterases deacetylating plant polysaccharides. Biotechnol. Adv. 30, 1575–1588. doi: 10.1016/j.biotechadv.2012.04.010
Bokulich, N. A., Subramanian, S., Faith, J. J., Gevers, D., Gordon, J. I., Knight, R., et al. (2013). Quality-filtering vastly improves diversity estimates from Illumina amplicon sequencing. Nat. Methods 10, 57–59. doi: 10.1038/nmeth.2276
Boraston, A. B., Bolam, D. N., Gilbert, H. J., and Davies, G. J. (2004). Carbohydrate-binding modules: fine-tuning polysaccharide recognition. Biochem. J. 382, 769–781. doi: 10.1042/BJ20040892
Brulc, J. M., Antonopoulos, D. A., Miller, M. E. B., Wilson, M. K., Yannarell, A. C., Dinsdale, E. A., et al. (2009). Gene-centric metagenomics of the fiber-adherent bovine rumen microbiome reveals forage specific glycoside hydrolases. Proc. Natl. Acad. Sci. U.S.A. 106, 1948–1953. doi: 10.1073/pnas.0806191105
Buchanan, G., Herdt, R. W., and Tweeten, L. G. (2010). Agricultural productivity strategies for the future: addressing U.S. and global challenges. CAST 52, 221–230.
Cantarel, B. L., Coutinho, P. M., Rancurel, C., Bernard, T., Lombard, V., and Henrissat, B. (2009). The carbohydrate-active enzymes database (CAZy): an expert resource for Glycogenomics. Nucleic Acids Res. 37, 233–238. doi: 10.1093/nar/gkn663
Caporaso, J. G., Bittinger, K., Bushman, F. D., Desantis, T. Z., Andersen, G. L., and Knight, R. (2010a). PyNAST: a flexible tool for aligning sequences to a template alignment. Bioinformatics 26, 266–267. doi: 10.1093/bioinformatics/btp636
Caporaso, J. G., Kuczynski, J., Stombaugh, J., Bittinger, K., Bushman, F. D., Costello, E. K., et al. (2010b). QIIME allows analysis of high-throughput community sequencing data. Nat. Methods 7, 335–336. doi: 10.1038/nmeth.f.303
Codron, D., and Clauss, M. (2010). Rumen physiology constrains diet niche: linking digestive physiology and food selection across wild ruminant species. Can. J. Zool. 88, 1129–1138. doi: 10.1139/Z10-077
Cole, J. R., Wang, Q., Fish, J. A., Chai, B., Mcgarrell, D. M., Sun, Y., et al. (2014). Ribosomal database project: data and tools for high throughput rRNA analysis. Nucleic Acids Res. 42, D633–D642. doi: 10.1093/nar/gkt1244
Comtetmarre, S., Parisot, N., Lepercq, P., Chaucheyrasdurand, F., Mosoni, P., Peyretaillade, E., et al. (2017). Metatranscriptomics reveals the active bacterial and eukaryotic fibrolytic communities in the rumen of dairy cow fed a mixed diet. Front. Microbiol. 8:67. doi: 10.3389/fmicb.2017.00067
Dai, X., Tian, Y., Li, J., Luo, Y., Liu, D., Zheng, H., et al. (2015). Metatranscriptomic analyses of plant cell wall polysaccharide degradation by microorganisms in the cow rumen. Appl. Environ. Microbiol. 81, 1375–1386. doi: 10.1128/AEM.03682-14
Díaz, J. C., Cabral, C., Redondo, L. M., Pin, N. V., Colombatto, D., Farber, M. D., et al. (2017). Impact of chestnut and quebracho tannins on rumen microbiota of bovines. Biomed. Res. Int. 3, 1–11. doi: 10.1155/2017/9610810
Doyle, J. J., and Dickson, E. E. (1987). Preservation of plant samples for DNA restriction endonuclease analysis. Taxon 36, 715–722. doi: 10.3390/toxins9110358
Edgar, R. C. (2010). Search and clustering orders of magnitude faster than blast. Bioinformatics 26:2460. doi: 10.1093/bioinformatics/btq461
Edwards, J. E., Kingston-Smith, A. H., Jimenez, H. R., Huws, S. A., Skøt, K. P., Griffith, G. W., et al. (2010). Dynamics of initial colonization of nonconserved perennial ryegrass by anaerobic fungi in the bovine rumen. FEMS Microbiol. Ecol. 66, 537–545. doi: 10.1111/j.1574-6941.2008.00563.x
Ferrer, M., Golyshina, O. V., Chernikova, T. N., Khachane, A. N., Reyes-Duarte, D., Santos, V. A., et al. (2010). Novel hydrolase diversity retrieved from a metagenome library of bovine rumen microflora. Environ. Microbiol. 7, 1996–2010. doi: 10.1111/j.1462-2920.2005.00920.x
Fox, D. G., Sniffen, C. J., O’Connor, J. D., Russell, J. B., and Van Soest, P. J. (1992). A net carbohydrate and protein system for evaluating cattle diets: III. cattle requirements and diet adequacy. J. Anim. Sci. 70, 3578–3596. doi: 10.2527/1992.70113578x
Ghanbari, M., Shahraki, H., Kneifel, W., and Domig, K. J. (2016). A first insight into the intestinal microbiota of snow trout ( schizothorax zarudnyi). Symbiosis 72, 183–193. doi: 10.1007/s13199-016-0455-2
Guo, J. X., Lu, Y., Zhu, W. Y., Denman, S. E., and Mcsweeney, C. S. (2010). Effect of tea saponin on methanogenesis, microbial community structure and expression of mcrA gene, in cultures of rumen micro-organisms. Lett. Appl. Microbiol. 47, 421–426. doi: 10.1111/j.1472-765X.2008.02459.x
He, B., Nohara, K., Ajami, N. J., Michalek, R. D., Tian, X., Wong, M., et al. (2015). Transmissible microbial and metabolomic remodeling by soluble dietary fiber improves metabolic homeostasis. Sci. Rep. 5:10604. doi: 10.1038/srep10604
Hess, M., Sczyrba, A., Egan, R., Kim, T.-W., Chokhawala, H., Schroth, G., et al. (2011). Metagenomic discovery of biomass-degrading genes and genomes from cow rumen. Science 331, 463–467. doi: 10.1126/science.1200387
Huoqing, H., Rui, Z., Dawei, F., Jianjie, L., Zhongyuan, L., Huiying, L., et al. (2011). Diversity, abundance and characterization of ruminal cysteine phytases suggest their important role in phytate degradation. Environ. Microbiol. 13, 747–757. doi: 10.1111/j.1462-2920.2010.02379.x
Huson, D., Mitra, S., Ruscheweyh, H.-J., Weber, N., and Schuster, S. C. (2011). Integrative analysis of environmental sequences using MEGAN4. Genome Res. 21, 1552–1560. doi: 10.1101/gr.120618.111
Jones, D. R., Thomas, D., Alger, N., Ghavidel, A., Inglis, G. D., and Abbott, D. W. (2018). SACCHARIS: an automated pipeline to streamline discovery of carbohydrate active enzyme activities within polyspecific families and de novo sequence datasets. Biotechnol. Biofuels 11:27. doi: 10.1186/s13068-018-1027-x
Jose, V. L., Appoothy, T., More, R. P., and Arun, A. S. (2017a). Metagenomic insights into the rumen microbial fibrolytic enzymes in Indian crossbred cattle fed finger millet straw. Amb. Express 7:13. doi: 10.1186/s13568-016-0310-0
Jose, V. L., More, R. P., Appoothy, T., and Arun, A. S. (2017b). In depth analysis of rumen microbial and carbohydrate-active enzymes profile in Indian crossbred cattle. Syst. Appl. Microbiol. 40, 160–170. doi: 10.1016/j.syapm.2017.02.003
Kala, A., Kamra, D. N., Kumar, A., Agarwal, N., Chaudhary, L. C., and Joshi, C. G. (2017). Impact of levels of total digestible nutrients on microbiome, enzyme profile and degradation of feeds in buffalo rumen. PLoS One 12:e0172051. doi: 10.1371/journal.pone.0172051
Kang, S., Denman, S. E., Morrison, M., Yu, Z., and McSweeney, C. S. (2009). An efficient RNA extraction method for estimating gut microbial diversity by polymerase chain reaction. Curr. Microbiol. 58, 464–471. doi: 10.1007/s00284-008-9345-z
Khafipour, E., Li, S., Plaizier, J. C., and Krause, D. O. (2009). Rumen microbiome composition determined using two nutritional models of subacute ruminal acidosis. Appl. Environ. Microbiol. 75, 7115–7124. doi: 10.1128/AEM.00739-09
Kittelmann, S., and Janssen, P. H. (2011). Characterization of rumen ciliate community composition in domestic sheep, deer, and cattle, feeding on varying diets, by means of PCR-DGGE and clone libraries. FEMS Microbiol. Ecol. 75, 468–481. doi: 10.1111/j.1574-6941.2010.01022.x
Kuruti, K., Nakkasunchi, S., Begum, S., Juntupally, S., Arelli, V., and Anupoju, G. R. (2017). Rapid generation of volatile fatty acids (vfa) through anaerobic acidification of livestock organic waste at low hydraulic residence time (HRT). Bioresour. Technol. 238, 188–193. doi: 10.1016/j.biortech.2017.04.005
Lairson, L. L., Henrissat, B., Davies, G. J., and Withers, S. G. (2008). Glycosyltransferases: structures, functions, and mechanisms. Annu. Rev. Biochem. 77, 521–555. doi: 10.1146/annurev.biochem.76.061005.092322
Li, R., Yu, C., Li, Y., Lam, T. W., Yiu, S. M., Kristiansen, K., et al. (2009). SOAP2: an improved ultrafast tool for short read alignment. Bioinformatics 25, 1966–1967. doi: 10.1093/bioinformatics/btp336
Li, R. W., Connor, E. E., Li, C., Baldwin Vi, R. L., and Sparks, M. E. (2012). Characterization of the rumen microbiota of pre-ruminant calves using metagenomic tools. Environ. Microbiol. 14, 129–139. doi: 10.1111/j.1462-2920.2011.02543.x
Li, W., and Godzik, A. (2006). Cd-hit: a fast program for clustering and comparing large sets of protein or nucleotide sequences. Bioinformatics 22:1658. doi: 10.1093/bioinformatics/btl158
Li, X., Song, L., Wang, G., Ren, L., Yu, D., Chen, G., et al. (2016). Complete genome sequence of a deeply branched marine Bacteroidia bacterium Draconibacterium orientale type strain FH5T. Mar. Genom 26, 13–16. doi: 10.1016/j.margen.2016.01.002
Lim, S., Seo, J., Choi, H., Yoon, D., Nam, J., Kim, H., et al. (2013). Metagenome analysis of protein domain collocation within cellulase genes of goat rumen microbes. Asian Austral. J. Anim. 26, 1144–1151. doi: 10.5713/ajas.2013.13219
Luo, R., Liu, B., Xie, Y., Li, Z., Huang, W., Yuan, J., et al. (2012). SOAPdenovo2: an empirically improved memory-efficient short-readde novoassembler. Gigascience 1:18. doi: 10.1186/2047-217X-1-18
Mackie, R. I., Aminov, R. I., White, B. A., Mcsweeney, C. S., and Cronje, P. B. (2000). “Molecular ecology and diversity in gut microbial ecosystems,” in Ruminant Physiology: Digestion, Metabolism, Growth and Reproduction, ed. P. B. Cronje (Wallingford: CABI), 61–77. doi: 10.1079/9780851994635.0061
Magoc, T., and Salzberg, S. L. (2011). FLASH: fast length adjustment of short reads to improve genome assemblies. Bioinformatics 27, 2957–2963. doi: 10.1093/bioinformatics/btr507
Maharjan, A., Alkotaini, B., and Kim, B. S. (2018). Fusion of carbohydrate binding modules to bifunctional cellulase to enhance binding affinity and cellulolytic activity. Biotechnol. Bioproc. Eng. 23, 79–85. doi: 10.1007/s12257-018-0011-4
Martinezgarcia, M., Brazel, D. M., Swan, B. K., Arnosti, C., Chain, P. S., Reitenga, K. G., et al. (2012). Capturing single cell genomes of active polysaccharide degraders: an unexpected contribution of Verrucomicrobia. PLoS One 7:e35314. doi: 10.1371/journal.pone.0035314
Math, R. K., Islam, S. M. A., Cho, K. M., Hong, S. J., Kim, J. M., Yun, M. G., et al. (2010). Isolation of a novel gene encoding a 3,5,6-trichloro-2-pyridinol degrading enzyme from a cow rumen metagenomic library. Biodegradation 21, 565–573. doi: 10.1007/s10532-009-9324-5
Mendiburu, F. (2016). Agricolae: Statistical Procedures for Agricultural Research. Available at: https://cran.r-project.org/web/packages/agricolae/index.html.
Miller, G. L., Blum, R., Glennon, W. E., and Burton, A. L. (1960). Measurement of carboxymethylcellulase activity. AnBio 1, 127–132. doi: 10.1016/0003-2697(60)90004-X
Naas, A. E., Mackenzie, A. K., Mravec, J., Schückel, J., Willats, W. G. T., Eijsink, V. G. H., et al. (2014). Do rumen Bacteroidetes utilize an alternative mechanism for cellulose degradation? MBio 5, e01401–e01414. doi: 10.1128/mBio.01401-14
Pang, J., Liu, Z. Y., Hao, M., Zhang, Y. F., and Qi, Q. S. (2017). An isolated cellulolytic Escherichia coli from bovine rumen produces ethanol and hydrogen from corn straw. Biotechnol. Biofuels 10:165. doi: 10.1186/s13068-017-0852-7
Park, B. H., Karpinets, T. V., Syed, M. H., Leuze, M. R., and Uberbacher, E. C. (2010). CAZymes analysis toolkit (CAT): web service for searching and analyzing carbohydrate-active enzymes in a newly sequenced organism using CAZy database. Glycobiology 20, 1574–1584. doi: 10.1093/glycob/cwq106
Patel, D. D., Patel, A. K., Parmar, N. R., Shah, T. M., Patel, J. B., Pandya, P. R., et al. (2014). Microbial and Carbohydrate Active Enzyme profile of buffalo rumen metagenome and their alteration in response to variation in the diet. Gene 545, 88, 94. doi: 10.1016/j.gene.2014.05.003
Pitta, D. W., Pinchak, W. E., Dowd, S., Dorton, K., Yoon, I., Min, B. R., et al. (2014). Longitudinal shifts in bacterial diversity and fermentation pattern in the rumen of steers grazing wheat pasture. Anaerobe 30, 11–17. doi: 10.1016/j.anaerobe.2014.07.008
Pope, P. B., Mackenzie, A. K., Gregor, I., Smith, W., Sundset, M. A., Mchardy, A. C., et al. (2012). Metagenomics of the svalbard reindeer rumen microbiome reveals abundance of polysaccharide utilization Loci. PLoS One 7:e38571. doi: 10.1371/journal.pone.0038571
R Core Team (2016). R: A Language and Environment for Statistical Computing. Vienna: R Foundation for Statistical Computing.
Schloss, P. D., Westcott, S. L., Ryabin, T., Hall, J. R., Hartmann, M., Hollister, E. B., et al. (2009). Introducing mothur: open-source, platformindependent, community-supported software for describing and comparing microbial communities. Appl. Environ. Microbiol. 75, 7537–7541. doi: 10.1128/AEM.01541-09
Singh, K. M., Bagath, M., Chikara, S. K., Joshi, C. G., and Kothari, R. K. (2015). Metagenomic Approaches in Understanding the Rumen Function and Establishing the Rumen Microbial Diversity. New Delhi: Springer. doi: 10.1007/978-81-322-2265-1_14
Solden, L. M., Naas, A. E., Roux, S., Daly, R. A., Collins, W. B., Nicora, C. D., et al. (2018). Interspecies cross-feeding orchestrates carbon degradation in the rumen ecosystem. Nat. Microbiol. 3, 1274–1284. doi: 10.1038/s41564-018-0225-4
Stewart, R. D., Auffret, M. D., Warr, A., Wiser, A. H., Press, M. O., Langford, K. W., et al. (2018). Assembly of 913 microbial genomes from metagenomic sequencing of the cow rumen. Nat. Commun. 9:870. doi: 10.1038/s41467-018-03317-6
Tang, S., and Borodovsky, M. (2010). Ab initio gene identification in metagenomic sequences. Nucleic Acids Res. 38:e132. doi: 10.1093/nar/gkq275
Walia, A., Guleria, S., Mehta, P., Chauhan, A., and Parkash, J. (2017). Microbial xylanases and their industrial application in pulp and paper biobleaching: a review. Biotech 7:11. doi: 10.1007/s13205-016-0584-6
Wang, G. R., and Duan, Y. L. (2014). Studies on lignocellulose degradation by rumen microorganism. Adv. Mater. Res. 853, 253–259. doi: 10.4028/www.scientific.net/AMR.853.253
Wang, L., Hatem, A., Catalyurek, U. V., Morrison, M., and Yu, Z. (2013). Metagenomic insights into the carbohydrate-active enzymes carried by the microorganisms adhering to solid digesta in the rumen of cows. PLoS One 8:e78507. doi: 10.1371/journal.pone.0078507
Wang, L., Hu, L., Yan, S., Jiang, T., Fang, S., Wang, G., et al. (2017). Effects of different oligosaccharides at various dosages on the composition of gut microbiota and short-chain fatty acids in mice with constipation. Food Funct. 8, 1966–1978. doi: 10.1039/c7fo00031f
Wang, Q., Garrity, G. M., Tiedje, J. M., and Cole, J. R. (2007). Naive Bayesian classifier for rapid assignment of rRNA sequences into the new bacterial taxonomy. Appl. Environ. Microbiol 73, 5261–5267. doi: 10.1128/AEM.00062-07
Whitman, W. B. (2015). Bergey’s Manual of Systematics of Archaea and Bacteria. Hoboken, NY: Wiley. doi: 10.1002/9781118960608Online
Xie, X., Yang, C., Guan, L. L., Wang, J., Xue, M., and Liu, J. (2018). Persistence of cellulolytic bacteria fibrobacterand treponema after short-term corn stover-based dietary intervention reveals the potential to improve rumen fibrolytic function. Front. Microbiol. 9:1363. doi: 10.3389/fmicb.2018.01363
Yang, H. J., and Xie, C. Y. (2010). Assessment of fibrolytic activities of 18 commercial enzyme products and their abilities to degrade the cell wall fraction of corn stalks in in vitro enzymatic and ruminal batch cultures. Anim. Feed Sci. Technol. 159, 110–121. doi: 10.1016/j.anifeedsci.2010.06.006
You, M., Yue, Z., He, W., Yang, X., Yang, G., Xie, M., et al. (2013). A heterozygous moth genome provides insights into herbivory and detoxification. Nat. Genet. 45, 220. doi: 10.1038/ng.2524
Zened, A., Combes, S., Cauquil, L., Jerome, M., Klopp, C., and Bouchez, O. (2013). Microbial ecology of the rumen evaluated by 454 gs flx pyrosequencing is affected by starch and oil supplementation of diets. FEMS Microbiol. Ecol. 83, 504–514. doi: 10.1111/1574-6941.12011
Zhang, J., Shi, H., Wang, Y., Li, S., Cao, Z., Ji, S., et al. (2017). Effect of dietary forage to concentrate ratios on dynamic profile changes and interactions of ruminal microbiota and metabolites in holstein heifers. Front. Microbiol. 8:2206. doi: 10.3389/fmicb.2017.02206
Keywords: holstein cow rumen, metagenomics, microbiome, CAZymes, taxonomic diversity
Citation: Wang L, Zhang G, Xu H, Xin H and Zhang Y (2019) Metagenomic Analyses of Microbial and Carbohydrate-Active Enzymes in the Rumen of Holstein Cows Fed Different Forage-to-Concentrate Ratios. Front. Microbiol. 10:649. doi: 10.3389/fmicb.2019.00649
Received: 21 December 2018; Accepted: 14 March 2019;
Published: 29 March 2019.
Edited by:
Alejandro Belanche, Estación Experimental del Zaidín (EEZ), SpainReviewed by:
Toby James Wilkinson, The University of Edinburgh, United KingdomLinda Boniface Oyama, Queen’s University Belfast, United Kingdom
K. M. Singh, Ashok & Rita Patel Institute of Integrated Study & Research in Biotechnology and Allied Sciences, India
Copyright © 2019 Wang, Zhang, Xu, Xin and Zhang. This is an open-access article distributed under the terms of the Creative Commons Attribution License (CC BY). The use, distribution or reproduction in other forums is permitted, provided the original author(s) and the copyright owner(s) are credited and that the original publication in this journal is cited, in accordance with accepted academic practice. No use, distribution or reproduction is permitted which does not comply with these terms.
*Correspondence: Hangshu Xin, eGluaGFuZ3NodUAxNjMuY29t Yonggen Zhang, emhhbmd5b25nZ2VuQHNpbmEuY29t