- Department of Molecular, Cellular, and Biomedical Sciences, University of New Hampshire, Durham, NH, United States
Streptomyces rimosus is best known as the primary source of the tetracycline class of antibiotics, most notably oxytetracycline, which have been widely used against many gram-positive and gram-negative pathogens and protozoan parasites. However, despite the medical and agricultural importance of S. rimosus, little is known of its evolutionary history and genome dynamics. In this study, we aim to elucidate the pan-genome characteristics and phylogenetic relationships of 32 S. rimosus genomes. The S. rimosus pan-genome contains more than 22,000 orthologous gene clusters, and approximately 8.8% of these genes constitutes the core genome. A large part of the accessory genome is composed of 9,646 strain-specific genes. S. rimosus exhibits an open pan-genome (decay parameter α = 0.83) and high gene diversity between strains (genomic fluidity φ = 0.12). We also observed strain-level variation in the distribution and abundance of biosynthetic gene clusters (BGCs) and that each individual S. rimosus genome has a unique repertoire of BGCs. Lastly, we observed variation in recombination, with some strains donating or receiving DNA more often than others, strains that tend to frequently recombine with specific partners, genes that often experience recombination more than others, and variable sizes of recombined DNA sequences. We conclude that the high levels of inter-strain genomic variation in S. rimosus is partly explained by differences in recombination among strains. These results have important implications on current efforts for natural drug discovery, the ecological role of strain-level variation in microbial populations, and addressing the fundamental question of why microbes have pan-genomes.
Introduction
The gram-positive genus Streptomyces (phylum Actinobacteria) constitutes a highly diverse group that is widely distributed in nature. Streptomyces are prolific producers of bioactive specialized metabolites that have adaptive functions in nature and have found extensive utility in human medicine (Kinashi, 2011; Cruz-Morales et al., 2016; Xu et al., 2016). They are known as the major source of naturally derived antibiotics and many pharmaceutically relevant compounds (e.g., antifungals, antitumor, antihelmintics, antiprotozoans, immunosuppressants) (Kinashi, 2011). Many invertebrates such as wasps and ants also use the antibiotics produced by their Streptomyces symbionts to protect themselves against infection (Kaltenpoth et al., 2005; Seipke et al., 2012). In contrast to most bacteria, Streptomyces species are characterized by complex secondary metabolism and a fungal-like morphological differentiation that involves the formation of branching, filamentous vegetative growth and aerial hyphae bearing long chains of reproductive spores (Flärdh and Buttner, 2009); hence they were originally misclassified as fungi. The formation of aerial mycelium corresponds to the production of secondary metabolites such as antibiotics (Barka et al., 2016). Current estimate of the number of known Streptomyces species is approximately 650 (Labeda et al., 2012), making it one of the largest genera in the bacterial domain.
Whole genome sequencing of closely related, locally co-occurring microbial strains has revealed the existence of tremendous diversity within a species, arising from both allelic and gene content differences (Croucher et al., 2014; Zhu et al., 2015; Levade et al., 2017; Chang et al., 2018). Hence, using traditional taxonomic methods, it is difficult to delineate two lineages that are considered the same species yet vary substantially in gene content (Jaspers and Overmann, 2004; Segerman, 2012; Land et al., 2015). For example, fuzzy species i.e., those that that do not form clear, distinct species boundaries due to frequent gene exchange through recombination, have been reported in Neisseria meningitidis (Hanage et al., 2005). Hybrid lineages as in the case of Klebsiella pneumoniae sequence type [ST] 258 have been formed via a large chromosomal replacement event (Chen et al., 2014). Such genomic mosaicism and within-species variation can significantly impact a species’ response to selective pressures from antibiotic use, vaccination, immune responses and host environment (Brüggemann et al., 2018; Leventhal et al., 2018; Sela et al., 2018). Within-species genomic variation has also been reported to impact species divergence (Papke et al., 2007; Youngblut et al., 2015), metabolic diversity and versatility (Silby et al., 2011), and symbiotic relationships (De Maayer et al., 2014) in microbes, with medically relevant implications. For example, hyper-recombinant strains of Streptococcus pneumoniae are associated with the highest levels of drug resistance (Hanage et al., 2009). One important process that generates genomic variation in microbial species is recombination, the exchange of very similar DNA sequences between strains, and which can result to either the addition or replacement of homologous genes (Didelot and Maiden, 2010; Didelot et al., 2012). Most studies dealing with within-species genomic variation has been focused on antibiotic resistant pathogens [for example, (Grad et al., 2014; Andam et al., 2017; Grinberg et al., 2017; Lam et al., 2018)], yet rarely do we find investigations on antibiotic producers. In Streptomyces, genomic diversity between species has been widely investigated (Doroghazi and Metcalf, 2013; Kim et al., 2015; Andam et al., 2016; Huguet-Tapia et al., 2016), but the extent, origins and functional role of genomic variation among closely related strains of the same species remains poorly understood.
In this study, we focus on Streptomyces rimosus, which is best known as the primary source of the tetracycline class of antibiotics, most notably oxytetracycline (Petković et al., 2006). Tetracyclines are noted for their broad spectrum antibacterial activity and since the 1940s, have been used against a wide range of both gram-positive and gram-negative pathogens, mycoplasmas, chlamydiae, rickettsiae and protozoan parasites (Chopra and Roberts, 2001). Oxytetracycline, a well-studied polyketide natural product, is a bacteriostatic antibiotic that inhibits bacterial growth by reversibly binding to the 30S ribosomal subunit, thus inhibiting protein synthesis (Schnappinger and Hillen, 1996; Petković et al., 2006). S. rimosus is also known to produce the polyene antifungal rimocidin (Davisson et al., 1951). Although the precise mechanism of action of rimocidins is still not well understood, antifungal activity seems to be due to polyene molecules causing the sterol-containing cell membrane to become permeable (Seco et al., 2005). Despite the medical and agricultural importance of S. rimosus and the variety of antibiotics it produces, little is known of its evolutionary history and genome characteristics. Here, we explore the pan-genome characteristics and phylogenetic relationships of 32 S. rimosus genomes. We report high levels of inter-strain genomic variation, including the differential distribution and abundance of BGCs among strains. BGCs represent a collection of genes that, together are responsible for the production of a specific secondary metabolite, such as antibiotics. We also observed high frequency of recombination which may partly explain the large genomic variation among strains; however, recombination is biased, with some strains exhibiting more frequent donation or receipt of DNA than other strains. These results have important implications on current efforts for natural drug discovery, the ecological role of strain-level genomic variation in microbial populations, and addressing the fundamental question of why microbes have pan-genomes.
Materials and Methods
Dataset
A total of 32 genomes of S. rimosus available in November 2018 were downloaded from the RefSeq database of the National Center for Biotechnology Information (NCBI). Accession numbers and genomic information (genome size, % GC content, number of genes, number of protein-coding genes) are shown in Supplementary Table S1. To maintain consistency in gene annotations, the genomes were re-annotated using Prokka with default parameters (Seemann, 2014).
Pan-Genome and Phylogenetic Analysis
Core and accessory genes were identified using Roary with default settings (Page et al., 2015). Roary iteratively pre-clusters protein sequences using CD-HIT (Fu et al., 2012), a fast program for clustering and comparing, which results to a substantially reduced set of data. Sequences in this reduced dataset were compared using all-against-all BLASTP (Altschul et al., 1990) and were then clustered the second time using Markov clustering (Enright et al., 2002). Each orthologous gene family from the merged CD-HIT and MCL were aligned using MAFFT (Katoh et al., 2002). We used Phandango (Hadfield et al., 2018) to visualize the presence-absence of genes per strain. The gene sequence alignments of each identified core gene family were concatenated to give a single core alignment, and a maximum-likelihood phylogeny was then generated using the program RAxML v.8.2.11 (Stamatakis, 2006)with a general time reversible (GTR) nucleotide substitution model (Tavaré, 1986), four gamma categories for rate heterogeneity and 100 bootstrap replicates. The phylogenetic tree was visualized using the Interactive Tree of Life (iToL) (Letunic and Bork, 2016).
We used the program micropan (Snipen and Liland, 2015) implemented in R (R Core Team, 2013) to calculate the pan-genome’s decay parameter (α) (Tettelin et al., 2008) and genomic fluidity (φ) (Kislyuk et al., 2011). The decay parameter measures the number of new gene clusters observed when genomes are ordered in a random way, which provides an indication of the openness or closeness of a pan-genome (Tettelin et al., 2008). An open pan-genome indicates that the number of new genes to be observed in future genomes is large, while a closed pan-genome indicates that after a certain number of sequenced genomes are added, the number of new genes discovered reaches a plateau (Tettelin et al., 2008). The genomic fluidity is a measure of the dissimilarity of genomes based on the degree of overlap in gene content and is defined as the number of unique gene families divided by the total number of gene families (Kislyuk et al., 2011). Both metrics are used to evaluate within-species genomic variation. Genome-wide average nucleotide identity (ANI) of all orthologous genes shared between any two genomes was calculated for all possible pairs of genomes (Jain et al., 2018). ANI is a robust similarity metric that has been widely used to resolve inter- and intra-strain relatedness. The threshold value of 95% has been widely used as a cutoff for comparisons belonging to the same or different species (Jain et al., 2018).
Biosynthetic gene clusters encoding secondary metabolites were predicted and annotated using the standalone version of antiSMASH 4.1 (Blin et al., 2017). antiSMASH predicts BGCs using signature profile Hidden Markov Models (pHMMs) derived from multiple sequence alignments of experimentally characterized signature proteins or protein domains of known BGCs (Blin et al., 2017). It then aligns the identified regions at the gene cluster level to their nearest relatives from a database containing all other known gene clusters (Blin et al., 2017). BGCs that encode for oxytetracycline and rimocidin were identified by searching all the genomes for homologs of each of the genes comprising the two BGCs using BLASTP (Altschul et al., 1990) with a minimum e-value of 10-10. Individual genes in a BGC obtained from previous studies (Seco et al., 2005; Zhang et al., 2006) were used as query sequences. Presence of the BGC was ascertained if there were BLASTP hits for at least 90% of the genes within the BGC. Sequences for the individual genes of the two BGCs were obtained from the Database of BIoSynthesis cluster CUrated and InTegrated (DoBISCUIT) (Ichikawa et al., 2013) based on previous studies of the oxytetracycline and rimocidin BGCs (Seco et al., 2005; Zhang et al., 2006).
Recombination Detection
We used three approaches to detect recombination in the population. First, the pairwise homoplasy index or PHI (Φw) test was used to determine the statistical likelihood of recombination being present in our dataset (Bruen et al., 2006). This statistic measures the genealogical correlation or similarity of adjacent sites. Under the null hypothesis of no recombination, the genealogical correlation of adjacent sites is invariant to permutations of the sites as all sites have the same history (Bruen et al., 2006). Significance of the observed Φw was obtained using a permutation test. We then visualized potential recombination events using Splitstree v.4.14.4, which integrates reticulations due to recombinations in phylogenetic relationships rather than forcing the data to be represented in a bifurcating tree (Huson and Bryant, 2006). Next, we ran fastGEAR (Mostowy et al., 2017) with default parameters to detect genome-wide mosaicism. Using the individual sequence alignments of all core and shared accessory genes, sequence clusters were first identified using BAPS (Cheng et al., 2013) implemented in fastGEAR. fastGEAR infers the population structure of individual alignments using a Hidden Markov Model to identify lineages in an alignment. Lineages are defined as groups which are genetically distinct in at least 50% of the alignment. Within each lineage, recombinations are identified by comparing every nucleotide site in the target sequence to all remaining lineages and asks whether it is more similar to something else compared to other strains in the same lineage. In other words, fastGEAR infers recombination by searching for similar nucleotide segments between diverse sequence clusters. To test the significance of the inferred recombinations and identify false-positive recombinations, fastGEAR uses a diversity test, wherein the diversity of the fragment in question is different compared to its background. To predict the origin of the recently recombined regions, the sequences on which the recombination event was predicted to have occurred were first extracted from the genome data. The recombined regions were then used as query sequences in BLASTN (Altschul et al., 1990) searches against all possible genomes from the identified donor lineage as well as from the non-redundant (nr) nucleotide database in NCBI. The top BLAST hit with the highest bit score was considered as the potential donor, provided that the hit covered at least 50% of the recombination fragment length and had a minimum of 99% nucleotide identity.
Results
Pan-Genome Characteristics of S. rimosus
We used a total of 32 S. rimosus genomes downloaded from the RefSeq database of NCBI (Supplementary Table S1). Genome sizes range from 8.14 to 10.02 Mb (mean = 9.20 Mb), while the number of predicted genes per genome ranges from 7,071 to 8,666 (mean = 8,020). The % G+C content also varies among genomes, ranging from 71.7 to 72.1%. We used Roary (Page et al., 2015) to calculate the S. rimosus pan-genome, defined as the totality of genes present in a group of genomes (Page et al., 2015). Roary classifies orthologous gene families into core genes and accessory genes. Core genes are present in 99% ≤ strains ≤ 100% (Supplementary Tables S2, S3). To take sequencing and assembly errors into account, Roary also calculates the number of soft core genes which are present in 95% ≤ strains < 99%. Accessory genes comprise the shell genes which are present in 15% ≤ strains < 95% and cloud genes which are present in < 15% of strains (Figure 1A). We found a considerably small core genome (1,945 genes) comprising 8.8% of the pan-genome (22,114 genes). Broadening our definition of the core genome to incorporate the soft core genes still only represented approximately 17% of the total pan-genome. The core genome comprises 22.44–27.51% of each individual genome. It is also notable that the vast majority of accessory genes (9,646, representing 44% of the pan-genome) are unique to a single strain. In microbes, large accessory genomes and high number of strain-specific genes are often associated with horizontal gene transfer (HGT) (Pohl et al., 2014; Vos et al., 2015; Zhu et al., 2016).
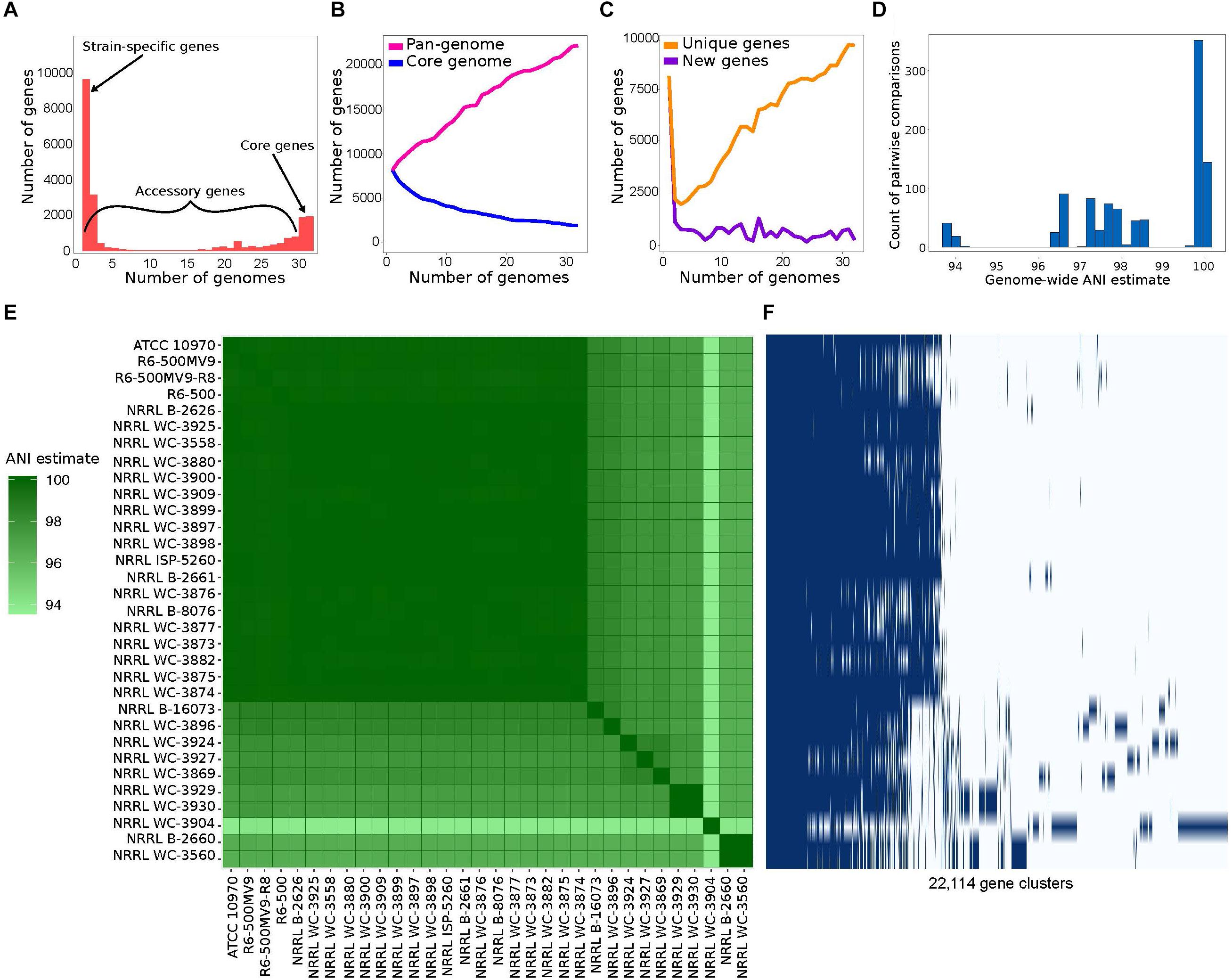
Figure 1. Pan-genome analysis of 32 Streptomyces rimosus strains. (A) The number of unique genes that are shared by any given number of genomes or unique to a single genome. Numerical values for each gene category are shown in Supplementary Table S2. (B) The size of the core genome, i.e., genes that are present in at least 31 of the 32 strains (blue line) and pan-genome, i.e., the totality of unique genes present in the population (pink line) in relation to numbers of genomes compared. The list of core genes is listed in Supplementary Table S3. (C) The number of unique genes, i.e., genes unique to individual strains (yellow line) and new genes, i.e., genes not found in the previously compared genomes (purple line) in relation to numbers of genomes compared. (D) Distribution of pairwise average nucleotide identity (ANI) values. ANI calculates the average nucleotide identity of all orthologous genes shared between any two genomes. The 95% ANI cutoff is a frequently used standard for species demarcation. (E) Pairwise whole genome ANI comparison. Percentage values are shown in Supplementary Table S4. (F) Gene presence-absence matrix showing the distribution of genes present in a genome. Each row corresponds to a strain in panel E. Each column represents an orthologous gene family. Dark blue blocks represent the presence of a gene, while light blue blocks represent the absence of a gene.
The size of the pan-genome and its increase/decrease in size upon addition of new strains can be used to predict the future rate of discovery of novel genes in a species (Medini et al., 2005; Tettelin et al., 2008). We used the program micropan to estimate the openness of the S. rimosus pan-genome by using the Heap’s power law function (Tettelin et al., 2008) for all possible permutations of all S. rimosus genomes. We calculated the decay parameter α, wherein an α > 1.0 indicates that the size of the pan-genome approaches a constsant as more genomes are sampled (i.e., the pan-genome is closed), while α < 1.0 indicates that the size of the pan-genome is increasing and unbounded by the number of genomes considered (i.e., the size of the pan-genome follows Heaps’ law and the pan-genome is open) (Medini et al., 2005; Tettelin et al., 2008). We obtained an α = 0.83 using 100 permutations in S. rimosus and suggests an open pan-genome; hence, we are likely to find new genes as more genomes are sequenced in the future. The openness of a pan-genome reflects the diversity of the gene pool within bacterial species, and is often associated with bacterial species that inhabit multiple environments or have different mechanisms and opportunities for gene exchange (Rouli et al., 2015; Brito et al., 2018). We find that the pan-genome of S. rimosus increases with the addition of new genomes, while the core genome decreases and begins to plateau at approximately 20 genomes (Figure 1B). The number of new, previously unseen, genes found as each genome is added to the plot averages 450 (Figure 1C). Finally, we also show the number of unique genes overall that have been observed exactly once continues to increase as each genome is added (Figure 1C).
To estimate the degree of overlap with respect to gene cluster content between any two genomes, we also calculated the genomic fluidity (φ), which provides an overview of gene-level similarity between genomes and is defined as the number of unique gene families divided by the total number of gene families (Kislyuk et al., 2011). Fluidity values range from 0 to 1, with 0.0 to indicate that the two genomes contain identical gene clusters, while 1.0 if the two genomes are non-overlapping (Kislyuk et al., 2011). Hence, a fluidity value of 0.2 for example implies that 20% of the genes are unique to their host genome and the remaining 80% are shared between genomes (Halachev et al., 2011). We obtained a genomic fluidity value of 0.12, which suggests that S. rimosus has a high degree of genomic diversity and is within the range found in other bacterial species (Halachev et al., 2011; Kislyuk et al., 2011).
To determine the degree of genomic relatedness and hence clarify whether these 32 genomes belong to the same species, we calculated the pairwise ANI for all possible pairs of genomes. ANI calculates the average nucleotide identity of all orthologous genes shared between any two genomes and organisms belonging to the same species typically exhibit ≥95% ANI (Jain et al., 2018). The distribution of pairwise ANI values reveal that the S. rimosus genomes are within the 95% cutoff and should therefore considered the same species (Figures 1D,E and Supplementary Table S4). Strain NRRL WC-3904 exhibits a slightly lower ANI value of 94% when compared to the rest of the genomes in the dataset. To further visualize the distribution of genes among the strains, we generated a pan-genome matrix using Roary and Phandango (Figure 1F). We find that NRRL WC-3904 exhibits a highly divergent accessory genome profile compared to the remaining 31 genomes, which may explain its slightly lower ANI values.
Strain-Level Variation in the Distribution and Abundance of BGCs
Streptomyces are renowned for their ability to produce structurally diverse natural products (called secondary metabolites), many of which are widely used in medicine, agriculture and bioenergy processes. Secondary metabolites differ from primary metabolites in that they are not involved in essential metabolic activities required for normal growth and reproduction of the organism, but may contribute significantly to an individual’s fitness and ecological adaptation (Zotchev, 2014). Mining bacterial genomes has shown that their potential for producing secondary metabolites and other bioactive compounds is much higher than what is observed in the laboratory (Doroghazi and Metcalf, 2013), and hence has important implications in discovering novel bioactive compounds.
Biosynthesis of secondary metabolites is typically governed by 10–30 genes organized as clusters in the genome, allowing the coordinated expression of the genes involved in their biosynthesis, resistance and efflux (Zotchev, 2014). We used antiSMASH 4.1 (Blin et al., 2017) to identify BGCs present in each S. rimosus genome. Each genome harbors 35–71 BGCs, with more than half of the BGCs predicted to produce polyketide (PKS) and non-ribosomal peptide synthetase (NRPS), or hybrids of the two (Figure 2A). This range in BGC content in S. rimosus is consistent with results from previous BGC surveys in other Streptomyces species (Seipke et al., 2011; Seipke et al., 2015; Choudoir et al., 2018; Vicente et al., 2018) and the widely studied actinobacterium Salinispora (Udwary et al., 2007; Letzel et al., 2017), although many BGCs often remain “silent” under standard laboratory conditions (Bentley et al., 2002; Ikeda et al., 2003; Ohnishi et al., 2008). Hybrid BGCs contain genes that code for more than one type of scaffold-synthesizing enzymes (Cimermancic et al., 2014; Zotchev, 2014). Many of the NRPS or PKS hybrids are found in one or few genomes: lantipeptide-t1pks-nrps hybrid in two genomes, melanin-t1pks hybrid in two genomes, phosphonate in one genome, t1pks-lassopeptide-nrps hybrid in two genomes, terpene-t2pks-t1pks-lassopeptide hybrid in two genomes, and terpene-t2pks-t1pks-lassopeptide hybrid in one genome. Aside from NRPS and PKS, other commonly shared BGCs are bacteriocin, butyrolactone, ectoine, lantipeptide, lasso peptide, melanin, nucleoside, siderophore, and terpene. Other BGCs are also differentially distributed among the 32 genomes: indoles in five genomes, ladderane in two genomes, phosphonate in one genome, and thiopeptide in one genome. Interestingly, Type II PKS and its hybrids were detected in 29 strains. Type II PKS synthesize tetracyclines and other aromatic polyketides such as anthracyclines, angucyclines, and pentangular polyphenols, which are also widely used as antibiotics or chemotherapeutics (Hertweck et al., 2007; Kim and Yi, 2012). Overall, we find that each individual S. rimosus genome harbors a unique combination of BGCs, further highlighting the extent of inter-strain genomic variation in S. rimosus. We note, however, that the reported numbers have likely been overestimated due to the low quality of some of the genome assemblies, which can affect the accurate BGC prediction in antiSMASH.
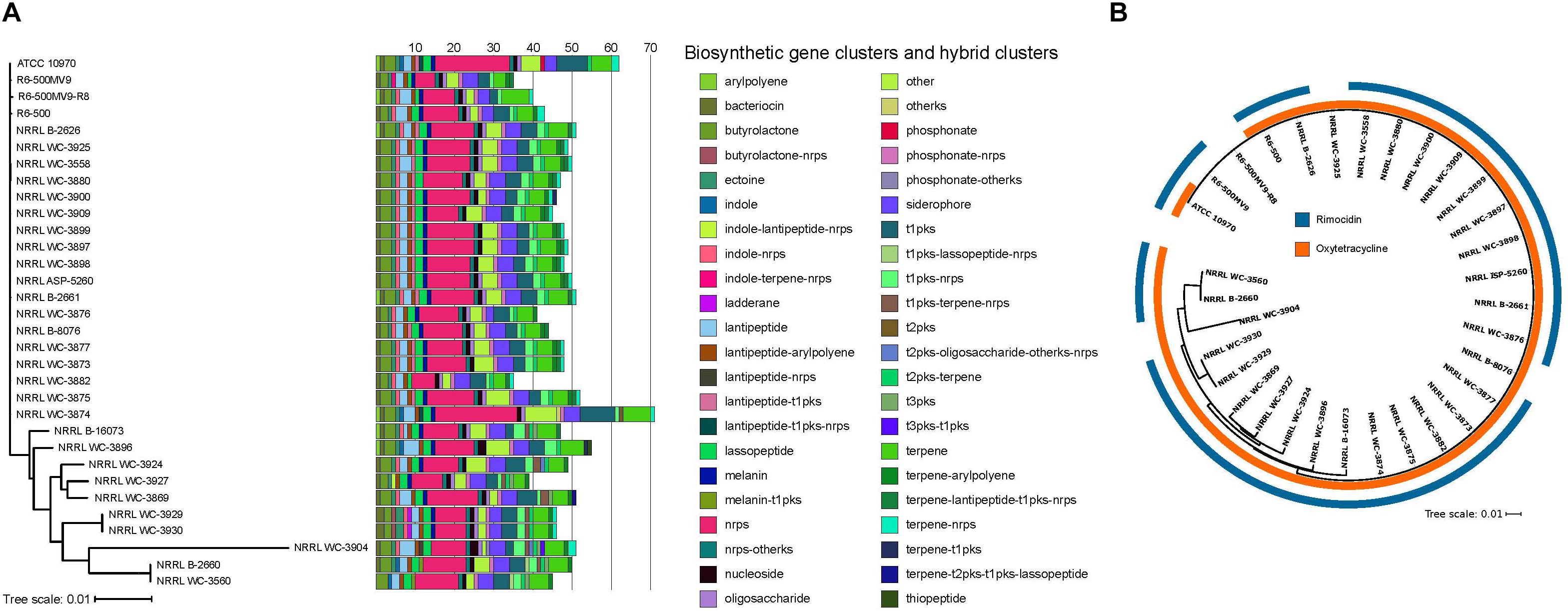
Figure 2. Distribution of BGCs per genome. (A) BGCs and hybrid clusters were identified using antiSMASH. The maximum likelihood phylogenetic tree was reconstructed using concatenated alignments of 1,945 core genes. Scale bar of phylogenetic tree represents nucleotide substitutions per site. Acronyms: nrps, non-ribosomal peptide synthase; t1pks, type 1 polyketide synthase; t2pks, type II polyketide synthase; t3pks, type III polyketide synthase; ks, ketosynthase. (B) Phylogenetic distribution of the oxytetracycline and rimocidin BGCs. Colored rings outside the tree show the presence/absence of BGCs known to encode for oxytetracycline and rimocidin. The two BGCs were identified by searching all the genomes for homologs of each of the genes comprising the BGCs using BLASTP (Altschul et al., 1990) with a minimum e-value of 10-10. Individual genes in a BGC obtained from previous studies (Seco et al., 2004; Zhang et al., 2006) were used as query sequences. Presence of the BGC was inferred if there were significant BLASTP hits for at least 90% of the individual genes within the BGC.
Streptomyces rimosus is particularly well known for its production of the antibiotics oxytetracycline and rimocidin (Petković et al., 2006). To determine the presence of BGCs that encode for these two antibiotics, we used BLASTP to search the 32 genomes for the individual genes of each BGC (Figure 2B and Supplementary Tables S5, S6). We found that, except for a single genome (R6-500MV9-R8), all S. rimosus genomes carry one or both BGCs. A total of 30 genomes had nearly 100% matches for each of the 21 genes found in the oxytetracycline BGC, while two showed a match for only a single gene. On the other hand, the rimocidin BGC was detected in 28 genomes (Supplementary Tables S7, S8).
Frequent but Biased Recombination Between Strains
In Streptomyces, recombination is known to have greatly contributed to shaping its evolution and diversity, with some taxonomically recognized species exhibiting significant genetic mosaicism (Doroghazi and Buckley, 2010; Andam et al., 2016; Cheng et al., 2016). To infer the phylogenetic relationships of the 32 S. rimosus genomes, the 1,945 core genes were aligned and concatenated, giving a total length of 2,017,766 bp. The core genome phylogeny reveals four clusters (Figure 3). Under the null hypothesis of no recombination, we calculated the PHI statistic (Bruen et al., 2006) and detected evidence for significant recombination in the core genome (p-value = 0.0). Recombination in S. rimosus core genome can be visualized using Neighbor Net implemented in SplitsTree4, which shows the reticulations in their phylogenetic relationships (Huson, 1998; Figure 3A). To further characterize the extent of genome-wide recombination in S. rimosus, we ran fastGEAR (Mostowy et al., 2017) on individual sequence alignments of core and shared accessory genes. Each predicted recombination fragment was then used as a query in BLASTN (Altschul et al., 1990) against the predicted lineage of donors to identify the most likely donor-recipient linkages. We found that recombination is frequent, with a total of 2,148 genes that had experienced recombination. However, when we mapped the donor-recipient recombination partners, we found that although recombination is frequent, it does not impact all genomes similarly (Figure 3B). A total of 12 genomes were not identified to be either a donor or recipient of recombined DNA. Of those genomes wherein recombination was detected, there were genomes that appear to accept more recombined DNA than others. We calculated the number of recombination events for any genome pair that is at least one standard deviation above the group’s average of 36 recombination events. We identified five genomes (NRRL WC-3869, NRRL WC-3927, NRRL WC-3924, NRRL WC-3896, NRRL B-16073) that have received more recombined DNA than others. We observed that although recombination is frequent, it does not impact all 32 genomes similarly. We find that recombination is biased, with some strains receiving more recombined DNA more often (NRRL WC-3896 and B-16073), while others exhibit preferences to specific exchange partners (Figure 3B).
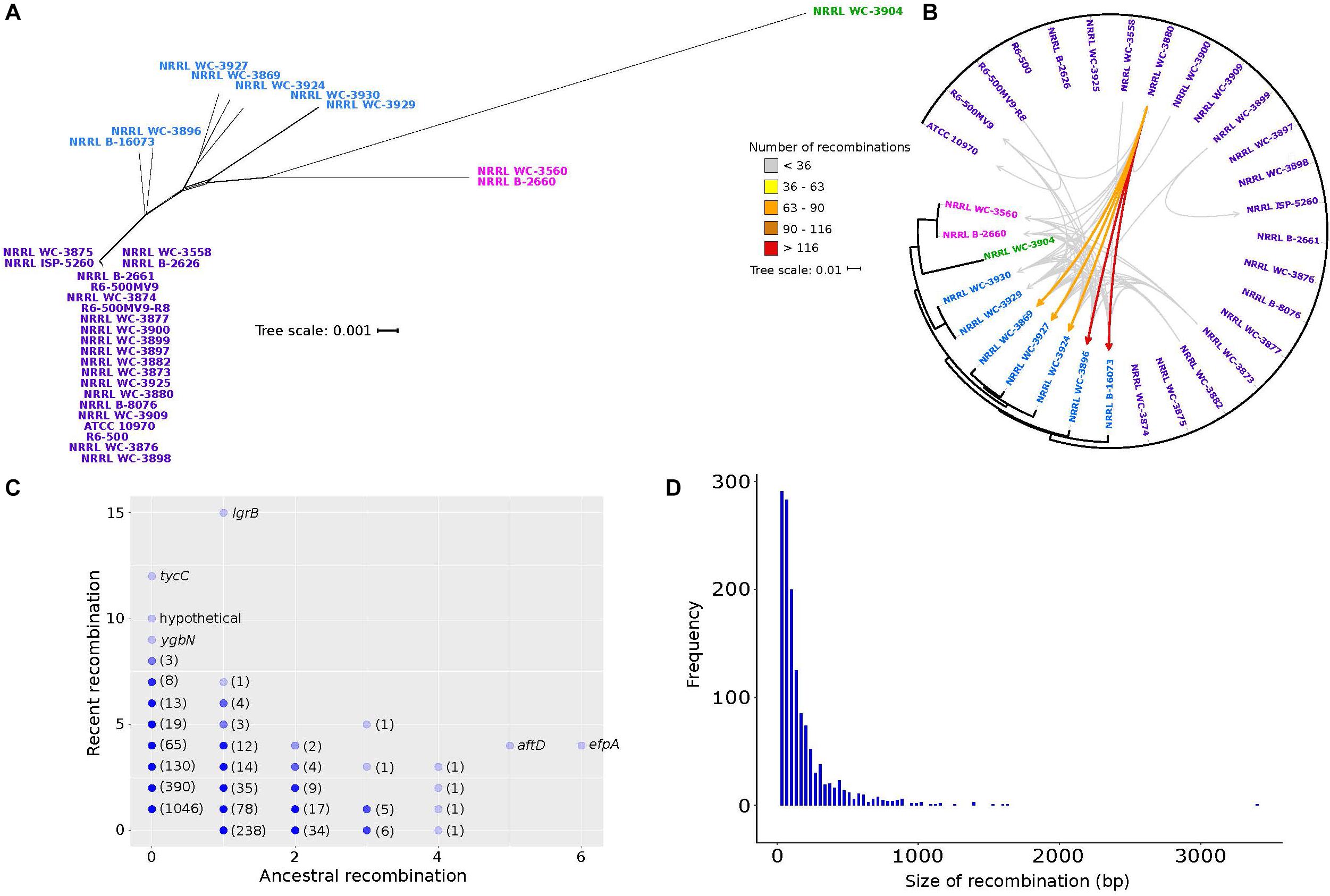
Figure 3. Genetic relationships among S. rimosus strains are influenced by homologous recombination. (A) A phylogenetic network of the S. rimosus core genome generated using SplitsTree. The strain names were colored according to clustering results using BAPS. (B) Donor-recipient linkages of major recombination events (i.e., highways of recombination) identified using fastGEAR and BLASTN. Scale bar represents nucleotide substitutions per site. Each arrow represents a certain number of recombination events between a pair of genomes, with different colors representing the range of numbers. (C) Genes that have undergone recent or ancestral recombination. Horizontal axis shows the estimated number of ancestral recombinations and vertical axis shows the estimated number of recent recombinations. Names of some of the genes are shown. Numbers in parenthesis indicate the number of genes represented by overlapping dots found on the same position. (D) Frequency histogram of the size of recombination events of all genes in the pan-genome.
The strength of fastGEAR is its ability to identify both recent (affecting a few strains) and ancestral (affecting entire lineages) recombinations (Mostowy et al., 2017). Of the recent recombination events identified, we observed a total of 91 unique donor-recipient pairs and five of these pairs contributed 49% or more of the total recombination events (Figure 3B). A total of 30 recent recombination events originate from donors outside of the S. rimosus dataset. Of these, half came from other Streptomyces species and two from other genera in Actinobacteria (Micromonospora and Rhodococcus). The taxonomic origins of the remaining recombination events could not be precisely determined due to the short length of the recombined sequences. Finally, we found that, of the 22,114 genes that comprise the pan-genome, a total of 2,149 genes have had a history of recombination (Figure 3C). Of these, 1,147 genes were involved in recent recombination and 386 genes in ancestral recombination. The most frequently recombined genes include those associated with antibiotic biosynthesis (lgrB, tycC), transmembrane transport (ygbN, efpA) and transferase (aftD) (Figure 3C and Supplementary Table S9).
The lengths of the recombined regions have an approximately exponential distribution, with majority of recombination events being small (<500 bp) and large events occurring relatively infrequently (Figure 3D). The median length of recombined fragments is 230 bp and the largest recombination event is 11,934 bp in strain NRRL B-16073. Our finding of a heterogeneous model of recombination is consistent with those reported in other bacterial species, such as the pathogens Streptococcus pneumoniae (Chewapreecha et al., 2014) and Legionella pneumophila (David et al., 2017), and our results demonstrate that it also holds true for non-pathogenic species. The observed heterogeneity in recombination sizes has been previously described and classified into micro-recombinations (i.e., short, frequent sequence replacements) and macro-recombinations (i.e., rarer, multi-fragment, saltational sequence replacements) (Mostowy et al., 2014), and our results are consistent with these. Overall, our analysis of recombination in S. rimosus reveals inter-strain variation in terms of the frequency of DNA donation or receipt, genes that experience the most frequent recombination and the size of recombination events.
Discussion
The tremendous diversity and ability of Streptomyces to inhabit numerous ecological niches and produce diverse clinically useful compounds have been attributed to their large pan-genomes (Zhou et al., 2012; Kim et al., 2015). In a recent study of 122 Streptomyces genomes comprising multiple species, a mere 2.63% (n = 1,048 genes present in ≥95% of all genomes) of the 39,893 gene families present constitutes the core genome while the remaining genes are classified as accessory genes (McDonald and Currie, 2017). At the species level, our results on 32 S. rimosus genomes reveal similar patterns of having a small fraction of core genes (n = 1,945 genes) which make up 8.8% of a much larger pan-genome (22,114 genes). When we include the soft core genes (genes present in at least 95% of the strains) numbering 1,874 genes, the core genome still represents only 17% of the pan-genome. While sequencing errors and the draft nature of the genomes used here may partly explain the low number of core genes in S. rimosus, the observation of a small core genome in microbial species is not uncommon and has been reported in other species (McInerney et al., 2017), including Actinobacteria. For example, the core genome of 28 Bifidobacterium longum subsp. longum strains consists of 1,160 genes from a pan-genome of 4,169 genes (Chaplin et al., 2015). In 18 strains of Corynebacterium pseudotuberculosis, the core genome consists of 1,355 genes and a pan-genome of 3,183 genes (Baraúna et al., 2017). In an analysis of 2,085 Escherichia coli genomes, the largest pan-genome analysis to date, a total of 3,188 genes comprises the core genome and is a remarkably small number compared to the stunning 90,000 genes that comprise the E. coli pan-genome, with a third of these genes occurring in only one genome (Land et al., 2015). The open pan-genome of S. rimosus means that the sequencing of new genomes will possibly add new genes not described in this current pan-genome study. Lastly, while it is difficult to speculate on the causes of why one strain (NRRL WC-3904) has an ANI of 94% compared to the other genomes (slightly below the 95% cutoff for species delineation), previous ANI-based studies have found similar results and may reflect the edge of a genetic discontinuum between species (Caro-Quintero et al., 2009; Jain et al., 2018). However, using the 83% ANI cutoff to delineate different species (Jain et al., 2018), WC-3904 cannot be classified as a separate species.
Compared to other Actinobacteria species and other bacterial phyla, Streptomyces also harbors the highest numbers of secondary metabolite BGCs from a large variety of classes and often with little overlap between strains (Doroghazi and Metcalf, 2013). Here, each S. rimosus genome harbors a unique repertoire of BGCs ranging from 35 to 71 BGCs per genome, including many NRPS, PKS and hybrid clusters. These results highlight the importance of sampling multiple strains of the same species in improving efforts for natural drug discovery. Antibiotics with new inhibitory mechanisms or cellular targets are urgently needed as resistance to our existing arsenal of drugs is growing and multidrug resistance becomes widespread. While emergence of resistance to and decreased effectiveness of existing tetracyclines as front-line antibiotics have grown over the years (Chopra and Roberts, 2001), our genomic analyses suggest that the potential of S. rimosus as producers of novel antibiotics has not been fully explored and many natural products are yet to be discovered from this species.
Only recently with whole genome sequencing do we come to recognize the extent in which, within each bacterial species, different strains may vary in the set of genes they encode (Konstantinidis et al., 2006; Seipke, 2015; Leonard et al., 2016; Truong et al., 2017). Recently, a polyphasic analyses was conducted on ten strains closely related to Streptomyces cyaneofuscatus, with all strains having identical 16S rRNA sequences (Antony-Babu et al., 2017). Authors reported significant differences in morphological, phenotypical and metabolic characteristics, and could in fact be distinguished as five different species (Antony-Babu et al., 2017). Such variation is not uncommon and has been reported to influence functions relevant to the structure and dynamics of the entire microbial community, adaptation to changes in the environment, and interactions with the eukaryotic host (Greenblum et al., 2015). However, the large pan-genome size of a microbial species remains intriguing. Efforts to elucidate the factors that shape and maintain the existence of a multitude of genes in a few strains have recently demonstrated the contributions of selection, drift, recombination, migration, and effective population size (Andreani et al., 2017; McInerney et al., 2017; Vos and Eyre-Walker, 2017; Bobay and Ochman, 2018). While the relative contributions of these processes across multiple microbial species remain unclear, it is likely that one or few of these processes may explain the large pan-genome size of S. rimosus.
Equally intriguing is our observation of heterogeneity in the frequency and characteristics of recombination. We observed that some strains donate or receive DNA more often than others, while some strains tend to frequently recombine with specific partners. Such a pair of strains or lineages exchanging DNA more often between them than with others is said to be linked by a highway of gene sharing (Beiko et al., 2005; Bansal et al., 2013). A highway of recombination between a pair of genomes, wherein they exchange DNA more often between them than with others, are likely to represent specific lineages that function as hubs of gene flow, facilitating the rapid spread of genes (for example, those associated with antibiotic resistance, metabolic genes, niche-specific genes) (Chewapreecha et al., 2014). These highways have been previously identified at higher taxonomic groups (domains, phyla, families) (Beiko et al., 2005; Zhaxybayeva et al., 2009; Bansal et al., 2013), but have only recently been reported at the sub-species level (Chewapreecha et al., 2014). However, the drivers of heterogeneity in the frequency and characteristics of recombination among members of the same species is poorly understood. Biases in recombination partners and other forms of genetic exchange have been reported to arise from phylogenetic relatedness (including compatible mismatch repair systems), geographical or physical proximity, shared ecological niches, or common set of mobile elements (Beiko et al., 2005; Andam and Gogarten, 2011; Smillie et al., 2011; Skippington and Ragan, 2012). However, it is unclear whether this variation in recombination is adaptive or not at the population level, to what extent strains that less often recombine benefit from the population, and how the population evolves with a mix of strains that vary in recombination frequencies and partners. In the future, a possible approach to further understand the variation in the recombination process in microbial genomes is to integrate evolutionary game theory with genome sequencing of closely related bacterial strains, composed of recombining (“cooperators”) and non-recombining (“cheaters”) that can be modeled over hundreds of generations (Van Dyken et al., 2013; Rauch et al., 2017; Zomorrodi and Segrè, 2017).
The principal caveat in this analysis is that that the quality of the S. rimosus genomes we examined are of varying quality, with some genomes having several hundred contigs. The draft nature of the genomes can have a significant impact on the antiSMASH output, particularly so in the identification of hybrid BGCs. There are two reasons for this. First, antiSMASH is conservative in terms of predicting the borders of BGCs and second, most strains harbor BGC islands on the arms of linear chromosomes [as in Streptomyces (Kinashi, 2011)], which antiSMASH can misidentify as hybrid BGCs. Another important limitation is that NCBI did not have information about the specific ecological and/or geographical origins of these strains (Supplementary Table S1). Moreover, only 32 genomes were considered. Because the size of the core and accessory genomes is a function of the number and characteristics of the dataset, improved sequencing quality as well as the sequencing of additional genomes is likely to alter some of our results. In our study, we found evidence of an open S. rimosus pan-genome (i.e., the number of new genes discovered increases with the number of additionally sequenced strains) even with the use of draft genomes. Hence, we may expect to find a larger core genome and additional accessory genes if these 32 strains are re-sequenced and complete genomes are generated. We also expect to find additional new and unique S. rimosus genes from strains inhabiting diverse environments. While they are most prevalent in soil and decaying vegetation, many Streptomyces species have also been identified in extreme environments and the gut of insects (Barka et al., 2016; van der Meij et al., 2017). In these places, we are likely to find niche-specific genes (Croucher et al., 2014; Gupta et al., 2015; Zhu et al., 2016), further expanding the size of the accessory genomes of Streptomyces species. Much of the work on Streptomyces isolation have only concentrated on soil environments, but future work should increase sampling efforts of S. rimosus in previously unexplored niches.
Conclusion
In this study, we focus on elucidating the pan-genome characteristics and phylogenetic relationships of 32 S. rimosus genomes, which is best known as the primary source of the tetracyclines used against many species of pathogens and parasites. There are two major conclusions from this study. First, S. rimosus exhibits tremendous inter-strain genomic and biosynthetic variation, which suggests that their potential as an antibiotic producer remains to be fully explored. Second, we observed high levels of recombination between strains; however, recombination is not a homogenous process in this species. Our findings contribute to addressing the puzzle of why microbes have pan-genomes (Andreani et al., 2017; McInerney et al., 2017; Vos and Eyre-Walker, 2017; Bobay and Ochman, 2018) and the contributions of biased gene exchange to maintaining gene content variability within a species (Beiko et al., 2005; Andam and Gogarten, 2011; Bansal et al., 2013; Chewapreecha et al., 2014).
Data Availability
Publicly available datasets were analyzed in this study. This data can be found here: https://www.ncbi.nlm.nih.gov/genome/?term=streptomyces%20rimosus.
Author Contributions
CA and CP designed the work and wrote the manuscript. CP performed all bioinformatics analyses. CA guided the work. Both authors read and approved the final manuscript.
Funding
The study was supported by the New Hampshire Agricultural Experiment Station (NHAES) grant number NH00653 to CA. CP is funded by the New Hampshire NASA Space Grant Graduate Student Fellowship from the New Hampshire Space Grant Consortium.
Conflict of Interest Statement
The authors declare that the research was conducted in the absence of any commercial or financial relationships that could be construed as a potential conflict of interest.
Acknowledgments
The authors thank the University of New Hampshire Resource Computing Center and Anthony Westbrook for providing technical and bioinformatics assistance.
Supplementary Material
The Supplementary Material for this article can be found online at: https://www.frontiersin.org/articles/10.3389/fmicb.2019.00552/full#supplementary-material
TABLE S1 | Accession numbers and genome characteristics of S. rimosus genomes used in this study.
TABLE S2 | Number of genes in each gene category of the pan-genome.
TABLE S3 | List of core genes inferred by Roary.
TABLE S4 | ANI values (%) for each pairwise genome comparison in Figure 1E.
TABLE S5 | List of genes in the oxytetracycline BGC used in Figure 2B. Sequences were obtained from DoBISCUIT (Database of BIoSynthesis cluster CUrated and InTegrated) (Ichikawa et al., 2013).
TABLE S6 | List of genes in the rimocidin BGC used in Figure 2B. Sequences were obtained from DoBISCUIT (Database of BIoSynthesis cluster CUrated and InTegrated) (Ichikawa et al., 2013).
TABLE S7 | BLAST results for individual genes in the oxytetracycline BGCs. Numbers represent the percentage sequence similarity to the query sequence.
TABLE S8 | BLAST results for individual genes in the rimocidin BGC. Numbers represent the percentage sequence similarity to the query sequence.
TABLE S9 | List of recombined genes inferred by fastGEAR.
References
Altschul, S. F., Gish, W., Miller, W., Myers, E. W., and Lipman, D. J. (1990). Basic local alignment search tool. J. Mol. Biol. 215, 403–410. doi: 10.1016/S0022-2836(05)80360-2
Andam, C. P., Choudoir, M. J., Vinh Nguyen, A., Sol Park, H., and Buckley, D. H. (2016). Contributions of ancestral inter-species recombination to the genetic diversity of extant Streptomyces lineages. ISME J. 10, 1731–1741. doi: 10.1038/ismej.2015.230
Andam, C. P., and Gogarten, J. P. (2011). Biased gene transfer in microbial evolution. Nat. Rev. Microbiol. 9, 543–555. doi: 10.1038/nrmicro2593
Andam, C. P., Mitchell, P. K., Callendrello, A., Chang, Q., Corander, J., Chaguza, C., et al. (2017). Genomic epidemiology of penicillin-nonsusceptible pneumococci with nonvaccine serotypes causing invasive disease in the United States. J. Clin. Microbiol. 55, 1104–1115. doi: 10.1128/JCM.02453-16
Andreani, N. A., Hesse, E., and Vos, M. (2017). Prokaryote genome fluidity is dependent on effective population size. ISME J. 11, 1719–1721. doi: 10.1038/ismej.2017.36
Antony-Babu, S., Stien, D., Eparvier, V., Parrot, D., Tomasi, S., and Suzuki, M. T. (2017). Multiple Streptomyces species with distinct secondary metabolomes have identical 16S rRNA gene sequences. Sci. Rep. 7:11089. doi: 10.1038/s41598-017-11363-1
Bansal, M. S., Banay, G., Harlow, T. J., Gogarten, J. P., and Shamir, R. (2013). Systematic inference of highways of horizontal gene transfer in prokaryotes. Bioinformatics 29, 571–579. doi: 10.1093/bioinformatics/btt021
Baraúna, R. A., Ramos, R. T. J., Veras, A. A. O., Pinheiro, K. C., Benevides, L. J., Viana, M. V. C., et al. (2017). Assessing the genotypic differences between trains of Corynebacterium pseudotuberculosis biovar equi through comparative genomics. PLoS One 12:e0170676. doi: 10.1371/journal.pone.0170676
Barka, E. A., Vatsa, P., Sanchez, L., Gaveau-Vaillant, N., Jacquard, C., Klenk, H.-P., et al. (2016). Taxonomy, physiology, and natural products of Actinobacteria. Microbiol. Mol. Biol. Rev. 80, 1–43. doi: 10.1128/MMBR.00019-15
Beiko, R. G., Harlow, T. J., and Ragan, M. A. (2005). Highways of gene sharing in prokaryotes. Proc. Natl. Acad. Sci. U.S.A. 102, 14332–14337. doi: 10.1073/pnas.0504068102
Bentley, S. D., Chater, K. F., Cerdeño-Tárraga, A.-M., Challis, G. L., Thomson, N. R., James, K. D., et al. (2002). Complete genome sequence of the model actinomycete Streptomyces coelicolor A3(2). Nature 417, 141–147. doi: 10.1038/417141a
Blin, K., Wolf, T., Chevrette, M. G., Lu, X., Schwalen, C. J., Kautsar, S. A., et al. (2017). antiSMASH 4.0-improvements in chemistry prediction and gene cluster boundary identification. Nucleic Acids Res. 45, W36–W41. doi: 10.1093/nar/gkx319
Bobay, L.-M., and Ochman, H. (2018). Factors driving effective population size and pan-genome evolution in bacteria. BMC Evol. Biol. 18:153. doi: 10.1186/s12862-018-1272-4
Brito, P. H., Chevreux, B., Serra, C. R., Schyns, G., Henriques, A. O., and Pereira-Leal, J. B. (2018). Genetic competence drives genome diversity in Bacillus subtilis. Genome Biol. Evol. 10, 108–124. doi: 10.1093/gbe/evx270
Bruen, T. C., Philippe, H., and Bryant, D. (2006). A simple and robust statistical test for detecting the presence of recombination. Genetics 172, 2665–2681. doi: 10.1534/genetics.105.048975
Brüggemann, H., Jensen, A., Nazipi, S., Aslan, H., Meyer, R. L., Poehlein, A., et al. (2018). Pan-genome analysis of the genus Finegoldia identifies two distinct clades, strain-specific heterogeneity, and putative virulence factors. Sci. Rep. 8:266. doi: 10.1038/s41598-017-18661-8
Caro-Quintero, A., Rodriguez-Castaño, G. P., and Konstantinidis, K. T. (2009). Genomic insights into the convergence and pathogenicity factors of Campylobacter jejuni and Campylobacter coli species. J. Bacteriol. 191, 5824–5831. doi: 10.1128/JB.00519-09
Chang, Q., Abuelaish, I., Biber, A., Jaber, H., Callendrello, A., Andam, C. P., et al. (2018). Genomic epidemiology of meticillin-resistant Staphylococcus aureus ST22 widespread in communities of the Gaza Strip, 2009. Euro Surveill. 23:1700592. doi: 10.2807/1560-7917.ES.2018.23.34.1700592
Chaplin, A. V., Efimov, B. A., Smeianov, V. V., Kafarskaia, L. I., Pikina, A. P., and Shkoporov, A. N. (2015). Intraspecies genomic diversity and long-term persistence of Bifidobacterium longum. PLoS One 10:e0135658. doi: 10.1371/journal.pone.0135658
Chen, L., Mathema, B., Pitout, J. D. D., DeLeo, F. R., and Kreiswirth, B. N. (2014). Epidemic Klebsiella pneumoniae ST258 is a hybrid strain. mBio 5:e1355-14. doi: 10.1128/mBio.01355-14
Cheng, K., Rong, X., and Huang, Y. (2016). Widespread interspecies homologous recombination reveals reticulate evolution within the genus Streptomyces. Mol. Phylogenet. Evol. 102, 246–254. doi: 10.1016/j.ympev.2016.06.004
Cheng, L., Connor, T. R., Sirén, J., Aanensen, D. M., and Corander, J. (2013). Hierarchical and spatially explicit clustering of DNA sequences with BAPS software. Mol. Biol. Evol. 30, 1224–1228. doi: 10.1093/molbev/mst028
Chewapreecha, C., Harris, S. R., Croucher, N. J., Turner, C., Marttinen, P., Cheng, L., et al. (2014). Dense genomic sampling identifies highways of pneumococcal recombination. Nat. Genet. 46, 305–309. doi: 10.1038/ng.2895
Chopra, I., and Roberts, M. (2001). Tetracycline antibiotics: mode of action, applications, molecular biology, and epidemiology of bacterial resistance. Microbiol. Mol. Biol. Rev. 65, 232–260. doi: 10.1128/MMBR.65.2.232-260.2001
Choudoir, M. J., Pepe-Ranney, C., and Buckley, D. H. (2018). Diversification of secondary metabolite biosynthetic gene clusters coincides with lineage divergence in Streptomyces. Antibiot. Basel Switz. 7:E12. doi: 10.3390/antibiotics7010012
Cimermancic, P., Medema, M. H., Claesen, J., Kurita, K., Wieland Brown, L. C., Mavrommatis, K., et al. (2014). Insights into secondary metabolism from a global analysis of prokaryotic biosynthetic gene clusters. Cell 158, 412–421. doi: 10.1016/j.cell.2014.06.034
Croucher, N. J., Coupland, P. G., Stevenson, A. E., Callendrello, A., Bentley, S. D., and Hanage, W. P. (2014). Diversification of bacterial genome content through distinct mechanisms over different timescales. Nat. Commun. 5:5471. doi: 10.1038/ncomms6471
Cruz-Morales, P., Kopp, J. F., Martínez-Guerrero, C., Yáñez-Guerra, L. A., Selem-Mojica, N., Ramos-Aboites, H., et al. (2016). Phylogenomic analysis of natural products biosynthetic gene clusters allows discovery of arseno-organic metabolites in model Streptomycetes. Genome Biol. Evol. 8, 1906–1916. doi: 10.1093/gbe/evw125
David, S., Sánchez-Busó, L., Harris, S. R., Marttinen, P., Rusniok, C., Buchrieser, C., et al. (2017). Dynamics and impact of homologous recombination on the evolution of Legionella pneumophila. PLoS Genet. 13:e1006855. doi: 10.1371/journal.pgen.1006855
Davisson, J. W., Tanner, F. W., Finlay, A. C., and Solomons, I. A. (1951). Rimocidin, a new antibiotic. Antibiot. Chemother. 1, 289–290.
De Maayer, P., Chan, W. Y., Rubagotti, E., Venter, S. N., Toth, I. K., Birch, P. R. J., et al. (2014). Analysis of the Pantoea ananatis pan-genome reveals factors underlying its ability to colonize and interact with plant, insect and vertebrate hosts. BMC Genomics 15:404. doi: 10.1186/1471-2164-15-404
Didelot, X., and Maiden, M. C. J. (2010). Impact of recombination on bacterial evolution. Trends Microbiol. 18, 315–322. doi: 10.1016/j.tim.2010.04.002
Didelot, X., Méric, G., Falush, D., and Darling, A. E. (2012). Impact of homologous and non-homologous recombination in the genomic evolution of Escherichia coli. BMC Genomics 13:256. doi: 10.1186/1471-2164-13-256
Doroghazi, J. R., and Buckley, D. H. (2010). Widespread homologous recombination within and between Streptomyces species. ISME J. 4, 1136–1143. doi: 10.1038/ismej.2010.45
Doroghazi, J. R., and Metcalf, W. W. (2013). Comparative genomics of actinomycetes with a focus on natural product biosynthetic genes. BMC Genomics 14:611. doi: 10.1186/1471-2164-14-611
Enright, A. J., Van Dongen, S., and Ouzounis, C. A. (2002). An efficient algorithm for large-scale detection of protein families. Nucleic Acids Res. 30, 1575–1584. doi: 10.1093/nar/30.7.1575
Flärdh, K., and Buttner, M. J. (2009). Streptomyces morphogenetics: dissecting differentiation in a filamentous bacterium. Nat. Rev. Microbiol. 7, 36–49. doi: 10.1038/nrmicro1968
Fu, L., Niu, B., Zhu, Z., Wu, S., and Li, W. (2012). CD-HIT: accelerated for clustering the next-generation sequencing data. Bioinformatics 28, 3150–3152. doi: 10.1093/bioinformatics/bts565
Grad, Y. H., Kirkcaldy, R. D., Trees, D., Dordel, J., Harris, S. R., Goldstein, E., et al. (2014). Genomic epidemiology of Neisseria gonorrhoeae with reduced susceptibility to cefixime in the USA: a retrospective observational study. Lancet Infect. Dis. 14, 220–226. doi: 10.1016/S1473-3099(13)70693-5
Greenblum, S., Carr, R., and Borenstein, E. (2015). Extensive strain-level copy-number variation across human gut microbiome species. Cell 160, 583–594. doi: 10.1016/j.cell.2014.12.038
Grinberg, A., Biggs, P. J., Zhang, J., Ritchie, S., Oneroa, Z., O’Neill, C., et al. (2017). Genomic epidemiology of methicillin-susceptible Staphylococcus aureus across colonisation and skin and soft tissue infection. J. Infect. 75, 326–335. doi: 10.1016/j.jinf.2017.07.010
Gupta, V. K., Chaudhari, N. M., Iskepalli, S., and Dutta, C. (2015). Divergences in gene repertoire among the reference Prevotella genomes derived from distinct body sites of human. BMC Genomics 16:153. doi: 10.1186/s12864-015-1350-6
Hadfield, J., Croucher, N. J., Goater, R. J., Abudahab, K., Aanensen, D. M., and Harris, S. R. (2018). Phandango: an interactive viewer for bacterial population genomics. Bioinformatics 34, 292–293. doi: 10.1093/bioinformatics/btx610
Halachev, M. R., Loman, N. J., and Pallen, M. J. (2011). Calculating orthologs in bacteria and Archaea: a divide and conquer approach. PLoS One 6:e28388. doi: 10.1371/journal.pone.0028388
Hanage, W. P., Fraser, C., and Spratt, B. G. (2005). Fuzzy species among recombinogenic bacteria. BMC Biol. 3:6. doi: 10.1186/1741-7007-3-6
Hanage, W. P., Fraser, C., Tang, J., Connor, T. R., and Corander, J. (2009). Hyper-recombination, diversity, and antibiotic resistance in pneumococcus. Science 324, 1454–1457. doi: 10.1126/science.1171908
Hertweck, C., Luzhetskyy, A., Rebets, Y., and Bechthold, A. (2007). Type II polyketide synthases: gaining a deeper insight into enzymatic teamwork. Nat. Prod. Rep. 24, 162–190. doi: 10.1039/b507395m
Huguet-Tapia, J. C., Lefebure, T., Badger, J. H., Guan, D., Pettis, G. S., Stanhope, M. J., et al. (2016). Genome content and phylogenomics reveal both ancestral and lateral evolutionary pathways in plant-pathogenic Streptomyces species. Appl. Environ. Microbiol. 82, 2146–2155. doi: 10.1128/AEM.03504-15
Huson, D. H. (1998). SplitsTree: analyzing and visualizing evolutionary data. Bioinformatics 14, 68–73. doi: 10.1093/bioinformatics/14.1.68
Huson, D. H., and Bryant, D. (2006). Application of phylogenetic networks in evolutionary studies. Mol. Biol. Evol. 23, 254–267. doi: 10.1093/molbev/msj030
Ichikawa, N., Sasagawa, M., Yamamoto, M., Komaki, H., Yoshida, Y., Yamazaki, S., et al. (2013). DoBISCUIT: a database of secondary metabolite biosynthetic gene clusters. Nucleic Acids Res. 41, D408–D414. doi: 10.1093/nar/gks1177
Ikeda, H., Ishikawa, J., Hanamoto, A., Shinose, M., Kikuchi, H., Shiba, T., et al. (2003). Complete genome sequence and comparative analysis of the industrial microorganism Streptomyces avermitilis. Nat. Biotechnol. 21, 526–531. doi: 10.1038/nbt820
Jain, C., Rodriguez-R, L. M., Phillippy, A. M., Konstantinidis, K. T., and Aluru, S. (2018). High throughput ANI analysis of 90K prokaryotic genomes reveals clear species boundaries. Nat. Commun. 9:5114. doi: 10.1038/s41467-018-07641-9
Jaspers, E., and Overmann, J. (2004). Ecological significance of microdiversity: identical 16S rRNA gene sequences can be found in bacteria with highly divergent genomes and ecophysiologies. Appl. Environ. Microbiol. 70, 4831–4839. doi: 10.1128/AEM.70.8.4831-4839.2004
Kaltenpoth, M., Göttler, W., Herzner, G., and Strohm, E. (2005). Symbiotic bacteria protect wasp larvae from fungal infestation. Curr. Biol. 15, 475–479. doi: 10.1016/j.cub.2004.12.084
Katoh, K., Misawa, K., Kuma, K., and Miyata, T. (2002). MAFFT: a novel method for rapid multiple sequence alignment based on fast Fourier transform. Nucleic Acids Res. 30, 3059–3066. doi: 10.1093/nar/gkf436
Kim, J., and Yi, G.-S. (2012). PKMiner: a database for exploring type II polyketide synthases. BMC Microbiol. 12:169. doi: 10.1186/1471-2180-12-169
Kim, J.-N., Kim, Y., Jeong, Y., Roe, J.-H., Kim, B.-G., and Cho, B.-K. (2015). Comparative genomics reveals the core and accessory genomes of Streptomyces species. J. Microbiol. Biotechnol. 25, 1599–1605. doi: 10.4014/jmb.1504.04008
Kinashi, H. (2011). Giant linear plasmids in Streptomyces: a treasure trove of antibiotic biosynthetic clusters. J. Antibiot. 64, 19–25. doi: 10.1038/ja.2010.146
Kislyuk, A. O., Haegeman, B., Bergman, N. H., and Weitz, J. S. (2011). Genomic fluidity: an integrative view of gene diversity within microbial populations. BMC Genomics 12:32. doi: 10.1186/1471-2164-12-32
Konstantinidis, K. T., Ramette, A., and Tiedje, J. M. (2006). The bacterial species definition in the genomic era. Philos. Trans. R. Soc. Lond. B Biol. Sci. 361, 1929–1940. doi: 10.1098/rstb.2006.1920
Labeda, D. P., Goodfellow, M., Brown, R., Ward, A. C., Lanoot, B., Vanncanneyt, M., et al. (2012). Phylogenetic study of the species within the family Streptomycetaceae. Antonie Van Leeuwenhoek 101, 73–104. doi: 10.1007/s10482-011-9656-0
Lam, M. M. C., Wyres, K. L., Duchêne, S., Wick, R. R., Judd, L. M., Gan, Y.-H., et al. (2018). Population genomics of hypervirulent Klebsiella pneumoniae clonal-group 23 reveals early emergence and rapid global dissemination. Nat. Commun. 9:2703. doi: 10.1038/s41467-018-05114-7
Land, M., Hauser, L., Jun, S.-R., Nookaew, I., Leuze, M. R., Ahn, T.-H., et al. (2015). Insights from 20 years of bacterial genome sequencing. Funct. Integr. Genomics 15, 141–161. doi: 10.1007/s10142-015-0433-4
Leonard, S. R., Mammel, M. K., Lacher, D. W., and Elkins, C. A. (2016). Strain-level discrimination of shiga toxin-producing escherichia coli in spinach using metagenomic sequencing. PLoS One 11:e0167870. doi: 10.1371/journal.pone.0167870
Letunic, I., and Bork, P. (2016). Interactive tree of life (iTOL) v3: an online tool for the display and annotation of phylogenetic and other trees. Nucleic Acids Res. 44, W242–W245. doi: 10.1093/nar/gkw290
Letzel, A.-C., Li, J., Amos, G. C. A., Millán-Aguiñaga, N., Ginigini, J., Abdelmohsen, U. R., et al. (2017). Genomic insights into specialized metabolism in the marine actinomycete Salinispora. Environ. Microbiol. 19, 3660–3673. doi: 10.1111/1462-2920.13867
Levade, I., Terrat, Y., Leducq, J.-B., Weil, A. A., Mayo-Smith, L. M., Chowdhury, F., et al. (2017). Vibrio cholerae genomic diversity within and between patients. Microb. Genomics 3:e000142. doi: 10.1099/mgen.0.000142
Leventhal, G. E., Boix, C., Kuechler, U., Enke, T. N., Sliwerska, E., Holliger, C., et al. (2018). Strain-level diversity drives alternative community types in millimetre-scale granular biofilms. Nat. Microbiol. 3, 1295–1303. doi: 10.1038/s41564-018-0242-3
McDonald, B. R., and Currie, C. R. (2017). Lateral gene transfer dynamics in the ancient bacterial genus Streptomyces. mBio 8:e00644-17. doi: 10.1128/mBio.00644-17
McInerney, J. O., McNally, A., and O’Connell, M. J. (2017). Why prokaryotes have pangenomes. Nat. Microbiol. 2:17040. doi: 10.1038/nmicrobiol.2017.40
Medini, D., Donati, C., Tettelin, H., Masignani, V., and Rappuoli, R. (2005). The microbial pan-genome. Curr. Opin. Genet. Dev. 15, 589–594. doi: 10.1016/j.gde.2005.09.006
Mostowy, R., Croucher, N. J., Andam, C. P., Corander, J., Hanage, W. P., and Marttinen, P. (2017). Efficient inference of recent and ancestral recombination within bacterial populations. Mol. Biol. Evol. 34, 1167–1182. doi: 10.1093/molbev/msx066
Mostowy, R., Croucher, N. J., Hanage, W. P., Harris, S. R., Bentley, S., and Fraser, C. (2014). Heterogeneity in the frequency and characteristics of homologous recombination in pneumococcal evolution. PLoS Genet. 10:e1004300. doi: 10.1371/journal.pgen.1004300
Ohnishi, Y., Ishikawa, J., Hara, H., Suzuki, H., Ikenoya, M., Ikeda, H., et al. (2008). Genome sequence of the streptomycin-producing microorganism Streptomyces griseus IFO 13350. J. Bacteriol. 190, 4050–4060. doi: 10.1128/JB.00204-08
Page, A. J., Cummins, C. A., Hunt, M., Wong, V. K., Reuter, S., Holden, M. T. G., et al. (2015). Roary: rapid large-scale prokaryote pan genome analysis. Bioinformatics 31, 3691–3693. doi: 10.1093/bioinformatics/btv421
Papke, R. T., Zhaxybayeva, O., Feil, E. J., Sommerfeld, K., Muise, D., and Doolittle, W. F. (2007). Searching for species in haloarchaea. Proc. Natl. Acad. Sci. U.S.A. 104, 14092–14097. doi: 10.1073/pnas.0706358104
Petković, H., Cullum, J., Hranueli, D., Hunter, I. S., Perić-Concha, N., Pigac, J., et al. (2006). Genetics of Streptomyces rimosus, the oxytetracycline producer. Microbiol. Mol. Biol. Rev. 70, 704–728. doi: 10.1128/MMBR.00004-06
Pohl, S., Klockgether, J., Eckweiler, D., Khaledi, A., Schniederjans, M., Chouvarine, P., et al. (2014). The extensive set of accessory Pseudomonas aeruginosa genomic components. FEMS Microbiol. Lett. 356, 235–241. doi: 10.1111/1574-6968.12445
R Core Team (2013). R: A Language and Environment for Statistical Computing. Vienna: R Foundation for Statistical Computing.
Rauch, J., Kondev, J., and Sanchez, A. (2017). Cooperators trade off ecological resilience and evolutionary stability in public goods games. J. R. Soc. Interface 14:20160967. doi: 10.1098/rsif.2016.0967
Rouli, L., Merhej, V., Fournier, P.-E., and Raoult, D. (2015). The bacterial pangenome as a new tool for analysing pathogenic bacteria. New Microbes New Infect. 7, 72–85. doi: 10.1016/j.nmni.2015.06.005
Schnappinger, D., and Hillen, W. (1996). Tetracyclines: antibiotic action, uptake, and resistance mechanisms. Arch. Microbiol. 165, 359–369. doi: 10.1007/s002030050339
Seco, E. M., Cuesta, T., Fotso, S., Laatsch, H., and Malpartida, F. (2005). Two polyene amides produced by genetically modified Streptomyces diastaticus var. 108. Chem. Biol. 12, 535–543. doi: 10.1016/j.chembiol.2005.02.015
Seco, E. M., Pérez-Zúñiga, F. J., Rolón, M. S., and Malpartida, F. (2004). Starter unit choice determines the production of two tetraene macrolides, rimocidin and CE-108, in Streptomyces diastaticus var. 108. Chem. Biol. 11, 357–366. doi: 10.1016/j.chembiol.2004.02.017
Seemann, T. (2014). Prokka: rapid prokaryotic genome annotation. Bioinformatics 30, 2068–2069. doi: 10.1093/bioinformatics/btu153
Segerman, B. (2012). The genetic integrity of bacterial species: the core genome and the accessory genome, two different stories. Front. Cell. Infect. Microbiol. 2:116. doi: 10.3389/fcimb.2012.00116
Seipke, R. F. (2015). Strain-level diversity of secondary metabolism in Streptomyces albus. PLoS One 10:e0116457. doi: 10.1371/journal.pone.0116457
Seipke, R. F., Barke, J., Brearley, C., Hill, L., Yu, D. W., Goss, R. J. M., et al. (2011). A single Streptomyces symbiont makes multiple antifungals to support the fungus farming ant Acromyrmex octospinosus. PLoS One 6:e22028. doi: 10.1371/journal.pone.0022028
Seipke, R. F., Kaltenpoth, M., and Hutchings, M. I. (2012). Streptomyces as symbionts: an emerging and widespread theme? FEMS Microbiol. Rev. 36, 862–876. doi: 10.1111/j.1574-6976.2011.00313.x
Sela, U., Euler, C. W., Correa da Rosa, J., and Fischetti, V. A. (2018). Strains of bacterial species induce a greatly varied acute adaptive immune response: the contribution of the accessory genome. PLoS Pathog. 14:e1006726. doi: 10.1371/journal.ppat.1006726
Silby, M. W., Winstanley, C., Godfrey, S. A. C., Levy, S. B., and Jackson, R. W. (2011). Pseudomonas genomes: diverse and adaptable. FEMS Microbiol. Rev. 35, 652–680. doi: 10.1111/j.1574-6976.2011.00269.x
Skippington, E., and Ragan, M. A. (2012). Phylogeny rather than ecology or lifestyle biases the construction of Escherichia coli-Shigella genetic exchange communities. Open Biol. 2, 120112. doi: 10.1098/rsob.120112
Smillie, C. S., Smith, M. B., Friedman, J., Cordero, O. X., David, L. A., and Alm, E. J. (2011). Ecology drives a global network of gene exchange connecting the human microbiome. Nature 480, 241–244. doi: 10.1038/nature10571
Snipen, L., and Liland, K. H. (2015). micropan: an R-package for microbial pan-genomics. BMC Bioinformatics 16:79. doi: 10.1186/s12859-015-0517-0
Stamatakis, A. (2006). RAxML-VI-HPC: maximum likelihood-based phylogenetic analyses with thousands of taxa and mixed models. Bioinformatics 22, 2688–2690. doi: 10.1093/bioinformatics/btl446
Tavaré, S. (1986). Some Probabilistic and Statistical Problems in the Analysis of DNA Sequences. Available at: http://www.amazon.ca/exec/obidos/redirect?tag=citeulike09-20&path=ASIN/0821811673 [accessed June 13, 2013].
Tettelin, H., Riley, D., Cattuto, C., and Medini, D. (2008). Comparative genomics: the bacterial pan-genome. Curr. Opin. Microbiol. 11, 472–477. doi: 10.1016/j.mib.2008.09.006
Truong, D. T., Tett, A., Pasolli, E., Huttenhower, C., and Segata, N. (2017). Microbial strain-level population structure and genetic diversity from metagenomes. Genome Res. 27, 626–638. doi: 10.1101/gr.216242.116
Udwary, D. W., Zeigler, L., Asolkar, R. N., Singan, V., Lapidus, A., Fenical, W., et al. (2007). Genome sequencing reveals complex secondary metabolome in the marine actinomycete Salinispora tropica. Proc. Natl. Acad. Sci. U.S.A. 104, 10376–10381. doi: 10.1073/pnas.0700962104
van der Meij, A., Worsley, S. F., Hutchings, M. I., and van Wezel, G. P. (2017). Chemical ecology of antibiotic production by actinomycetes. FEMS Microbiol. Rev. 41, 392–416. doi: 10.1093/femsre/fux005
Van Dyken, J. D., Müller, M. J. I., Mack, K. M. L., and Desai, M. M. (2013). Spatial population expansion promotes the evolution of cooperation in an experimental Prisoner’s Dilemma. Curr. Biol. 23, 919–923. doi: 10.1016/j.cub.2013.04.026
Vicente, C. M., Thibessard, A., Lorenzi, J.-N., Benhadj, M., Hôtel, L., Gacemi-Kirane, D., et al. (2018). Comparative genomics among closely related streptomyces strains revealed specialized metabolite biosynthetic gene cluster diversity. Antibiot. Basel Switz. 7:86. doi: 10.3390/antibiotics7040086
Vos, M., and Eyre-Walker, A. (2017). Are pangenomes adaptive or not? Nat. Microbiol. 2:1576. doi: 10.1038/s41564-017-0067-5
Vos, M., Hesselman, M. C., Te Beek, T. A., van Passel, M. W. J., and Eyre-Walker, A. (2015). Rates of lateral gene transfer in prokaryotes: high but why? Trends Microbiol. 23, 598–605. doi: 10.1016/j.tim.2015.07.006
Xu, M., Wang, Y., Zhao, Z., Gao, G., Huang, S.-X., Kang, Q., et al. (2016). Functional genome mining for metabolites encoded by large gene clusters through heterologous expression of a whole-genome bacterial artificial chromosome library in Streptomyces spp. Appl. Environ. Microbiol. 82, 5795–5805. doi: 10.1128/AEM.01383-16
Youngblut, N. D., Wirth, J. S., Henriksen, J. R., Smith, M., Simon, H., Metcalf, W. W., et al. (2015). Genomic and phenotypic differentiation among Methanosarcina mazei populations from Columbia River sediment. ISME J. 9, 2191–2205. doi: 10.1038/ismej.2015.31
Zhang, W., Ames, B. D., Tsai, S.-C., and Tang, Y. (2006). Engineered biosynthesis of a novel amidated polyketide, using the malonamyl-specific initiation module from the oxytetracycline polyketide synthase. Appl. Environ. Microbiol. 72, 2573–2580. doi: 10.1128/AEM.72.4.2573-2580.2006
Zhaxybayeva, O., Doolittle, W. F., Papke, R. T., and Gogarten, J. P. (2009). Intertwined evolutionary histories of marine Synechococcus and Prochlorococcus marinus. Genome Biol. Evol. 1, 325–339. doi: 10.1093/gbe/evp032
Zhou, Z., Gu, J., Li, Y.-Q., and Wang, Y. (2012). Genome plasticity and systems evolution in Streptomyces. BMC Bioinformatics 13(Suppl. 10):S8. doi: 10.1186/1471-2105-13-S10-S8
Zhu, A., Sunagawa, S., Mende, D. R., and Bork, P. (2015). Inter-individual differences in the gene content of human gut bacterial species. Genome Biol. 16:82. doi: 10.1186/s13059-015-0646-9
Zhu, B., Ibrahim, M., Cui, Z., Xie, G., Jin, G., Kube, M., et al. (2016). Multi-omics analysis of niche specificity provides new insights into ecological adaptation in bacteria. ISME J. 10, 2072–2075. doi: 10.1038/ismej.2015.251
Zomorrodi, A. R., and Segrè, D. (2017). Genome-driven evolutionary game theory helps understand the rise of metabolic interdependencies in microbial communities. Nat. Commun. 8:1563. doi: 10.1038/s41467-017-01407-5
Keywords: Streptomyces, tetracycline, pan-genome, core genome, accessory genome, recombination, biosynthetic gene cluster
Citation: Park CJ and Andam CP (2019) Within-Species Genomic Variation and Variable Patterns of Recombination in the Tetracycline Producer Streptomyces rimosus. Front. Microbiol. 10:552. doi: 10.3389/fmicb.2019.00552
Received: 04 January 2019; Accepted: 04 March 2019;
Published: 21 March 2019.
Edited by:
Iain Sutcliffe, Northumbria University, United KingdomReviewed by:
Ryan Seipke, University of Leeds, United KingdomHrvoje Petkovic Petkovic, University of Ljubljana, Slovenia
Copyright © 2019 Park and Andam. This is an open-access article distributed under the terms of the Creative Commons Attribution License (CC BY). The use, distribution or reproduction in other forums is permitted, provided the original author(s) and the copyright owner(s) are credited and that the original publication in this journal is cited, in accordance with accepted academic practice. No use, distribution or reproduction is permitted which does not comply with these terms.
*Correspondence: Cheryl P. Andam, Q2hlcnlsLkFuZGFtQHVuaC5lZHU=