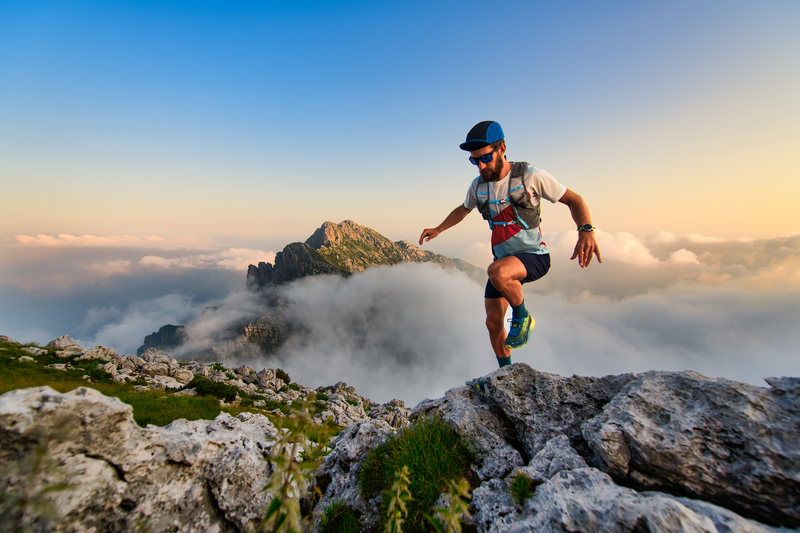
94% of researchers rate our articles as excellent or good
Learn more about the work of our research integrity team to safeguard the quality of each article we publish.
Find out more
ORIGINAL RESEARCH article
Front. Microbiol. , 26 February 2019
Sec. Antimicrobials, Resistance and Chemotherapy
Volume 10 - 2019 | https://doi.org/10.3389/fmicb.2019.00309
Whole genome sequencing (WGS) of Mycobacterium tuberculosis has been constructive in understanding its evolution, genetic diversity and the mechanisms involved in drug resistance. A large number of sequencing efforts from across the globe have revealed genetic diversity among clinical isolates and the genetic determinants for their resistance to anti-tubercular drugs. Considering the high TB burden in India, the availability of WGS studies is limited. Here we present, WGS results of 200 clinical isolates of M. tuberculosis from North India which are categorized as sensitive to first-line drugs, mono-resistant, multi-drug resistant and pre-extensively drug resistant isolates. WGS revealed that 20% of the isolates were co-infected with M. tuberculosis and non-tuberculous mycobacteria species. We identified 12,802 novel genetic variations in M. tuberculosis isolates including 343 novel SNVs in 38 genes which are known to be associated with drug resistance and are not currently used in the diagnostic kits for detection of drug resistant TB. We also identified M. tuberculosis lineage 3 to be predominant in the northern region of India. Additionally, several novel SNVs, which may potentially confer drug resistance were found to be enriched in the drug resistant isolates sampled. This study highlights the significance of employing WGS in diagnosis and for monitoring further development of MDR-TB strains.
Tuberculosis is one of the leading causes of death worldwide. The emerging drug resistance is a serious global threat and poses significant challenge to public health. According to the recent WHO report, there were an estimated 10.0 million cases of TB and 1.3 million deaths during the year 2017. India alone accounted for 24% of global MDR-TB incidence and 27% of global TB incidence among HIV-negative individuals (Global Tuberculosis Who Report [GWTR]x, 2018). There is an urgent need for improved diagnosis of TB, such as identification of markers to monitor transmission and effective treatment to deal with this deadly disease. Whole genome sequencing (WGS) studies from across the globe have revealed genetic diversity of Mycobacterium tuberculosis and have provided significant insights into its evolution and transmission (Casali et al., 2014; Walker et al., 2015; Nikolayevskyy et al., 2016). They have also revealed specific genotypes associated with drug resistance.
Several studies have shown association of the genetic variations with pathogenesis and drug resistance (Laurenzo and Mousa, 2011; Zhang et al., 2013; Casali et al., 2014). Global frontline molecular diagnostics such as line probe assays and Xpert MTB/RIF used for diagnosis of drug resistant TB, have been developed based on these genetic markers (Gagneux and Small, 2007; Thakur et al., 2015; Thirumurugan et al., 2015). However, these tests rely on a limited number of mutations. There have been several instances where phenotypic resistance could not be explained by known mutations associated with drug resistance (Rigouts et al., 2013; Banu et al., 2014; Ahmad et al., 2016). A recent study comparing the efficacy of Xpert MTB/RIF with line probe assay for detection of rifampicin mono-resistant M. tuberculosis reported the utility of country specific probes, to increase the sensitivity of Xpert MTB/RIF in India (Rufai et al., 2014). Since there is considerable genetic heterogeneity among M. tuberculosis isolates from different geographic regions, large-scale sequencing efforts are required to map genetic variations and identify the genotypes associated with drug resistance.
Whole genome sequencing studies for mapping genetic heterogeneity and identifying determinants of drug resistance among clinical isolates in India are limited (Chatterjee et al., 2017; Manson et al., 2017a). Previous studies have revealed that lineage 1 (Indo-Oceanic) and lineage 3 (East-African-Indian) are most prevalent in India and are less common in other parts of the world (Gutierrez et al., 2006; Ahmed et al., 2009). Lineage 1 is prevalent in South India whereas lineage 3 is prevalent in North India. A recent study has reported WGS data for 223 clinical isolates from South India (Manson et al., 2017a). They have observed genetic diversity among the sequenced isolates and reported potential novel genotypes that might be associated with drug resistance. The study has also observed potential mixed infections that might affect prediction of drug resistant phenotypes based on genotype data. In the current study, we performed WGS analysis of 200 culture confirmed M. tuberculosis clinical isolates from North India. We analyzed isolates from different categories such as sensitive to first line drugs, rifampicin mono-resistant, isoniazid mono-resistant, streptomycin mono-resistant, MDR and pre-extensively drug resistant (pre-XDR). We compared genetic variations observed in these isolates with data from 2,044 M. tuberculosis clinical isolates available in public domain. In addition, we also compared the genetic variations with those found in isolates prevalent in South India. We identified several novel genetic variations and novel genotypes in clinical isolates from North India that might potentially be associated with drug resistance.
Two hundred M. tuberculosis clinical isolates were collected from the Mycobacterial Repository Centre of the National JALMA Institute of Leprosy and Other Mycobacterial Diseases, Agra, India. This study was carried out in accordance with the recommendations of the guidelines of the National JALMA Institute of Leprosy and other Mycobacterial Diseases. The study was approved by the Institutional Ethics Committee of the National JALMA Institute of Leprosy and other Mycobacterial Diseases, Agra. All the subjects gave written informed consent in accordance with the Declaration of Helsinki.
Mycobacterium tuberculosis clinical isolates used in the current study were cultured and maintained at the Department of Microbiology, the National JALMA Institute of Leprosy and other Mycobacterial Diseases, Agra, India. The isolates were procured from pulmonary sites of patients diagnosed with tuberculosis. The samples were processed as per the standard N-acetyl-L-cysteine-sodium hydroxide (NALC-NaOH) and inoculated on Lowenstein-Jensen (LJ) slants for primary culture (Apers et al., 2003; Steingart et al., 2006; Peres et al., 2009). The cultures were subsequently tested using biochemical assays to rule out NTM or any other infection. The samples were sub cultured using single colony growth on Lowenstein-Jensen (LJ) for DNA isolation. DNA libraries were constructed with genomic DNA extracted using CTAB method (van Soolingen et al., 1991). DNA was fragmented in the range of 100 to 800 bases. The fragmented DNA was cleaned up using QIAquick columns (QIAGEN). The size distribution was checked by running aliquots of the samples on Agilent Bioanalyzer 7500 Nano chips. Illumina adapters were ligated to each fragment. Fragments of ∼300 bases were separated using Gel electrophoresis and sequenced at both ends using Illumina HiSeq 2500 sequencer. Sequencing depth in all the isolates was more than 100X with average 100 bp paired end read length.
Drug susceptibility testing of M. tuberculosis isolates was performed using minimal inhibitory concentration (MIC) method on LJ slants. Drug concentrations used for testing were- 64 μg/ml for Streptomycin sulfate (STR), 1 μg/ml for Isoniazid (INH), 64 μg/ml for Rifampicin (RMP), 6 μg/ml for Ethambutol (EMB), 64 μg/ml for Kanamycin (KAN), and 6 μg/ml for Ofloxacin (OFX) (Canetti et al., 1969; Singh et al., 2009; Purwar et al., 2011). All drugs were procured from Sigma Aldrich. Inoculated LJ slants were examined for growth after 28 days of incubation and the colony count was recorded. The strain was considered resistant if the colony count on the slant was 20 or more (Canetti et al., 1969). Isolates were classified into the following categories during data analysis on the basis of drug resistance profiles: Sensitive to first line drugs (n = 50), MDR (n = 91) Rifampicin mono-resistant (n = 12), Isoniazid mono-resistant (n = 31), Streptomycin mono-resistant (n = 6), Pre-XDR (n = 10).
The quality of the raw reads was checked using FastQC version-0.11.5 toolkit followed by trimming of adapters and low-quality bases with a Phred quality score of less than 20. Reads were then mapped on to H37Rv reference genome (NC_000962.3) using Burrows-Wheeler Alignment Tool (BWA) (version-0.7.15) (Li and Durbin, 2009). The alignment files were subjected to local realignment and de-duplication using the Genome Analysis Toolkit (GATK) (version-3.6) and Picard. Variants SNVs and Insertion/Deletions (In/Dels) were called from each alignment file using GATK and Pindel (version 0.2.5b8) (Ye et al., 2009). Variant filtering was carried out by removing variants with <5 reads. Variants with low base quality of <20 were discarded. The variants were annotated using the scripts1. Variants selected had a minimum read depth of 5 reads and a mapping quality score of above 20. All variants identified in this study were manually inspected using Integrative Genomics Viewer (IGV) (version-2.3.86) (Ye et al., 2009).
Metagenomic analysis was performed using Kraken which, is a program for assigning taxonomic labels to short DNA sequences obtained through metagenomic studies (Wood and Salzberg, 2014). This analysis was carried out for 39 isolates where we observed poor sequence alignment with M. tuberculosis H37Rv genome.
Sequence variants from GMTV and NIRT datasets were used along with our dataset to carry out principal component analysis (PCA) (Chernyaeva et al., 2014; Manson et al., 2017b). The Genome-wide Mycobacterium tuberculosis Variation (GMTV) database is a cumulative catalog of all genetic variations observed in M. tuberculosis strains from all over world and the NIRT data comprises of genome information on 220 M. tuberculosis isolates sequenced recently from South India (Chernyaeva et al., 2014; Manson et al., 2017b). A total of 87,613 SNVs from 2,201 isolates was used to generate the matrix of SNVs. PCA was performed using ggfortify package in R version-3.2.0.
Mycobacterium tuberculosis complex lineages/sublineages and in silico spoligotyping were determined using KvarQ (version-0.12.2) (Steiner et al., 2014). SNVs identified in each isolate were used to construct the phylogenetic tree. Individual consensus sequence fasta files for isolates with SNVs identified in each of the isolates were generated using vcf-consensus module (Applies VCF variants to a fasta file to create consensus sequence) from VCFtools (Version 0.1.16) (Danecek et al., 2011). Multiple sequence alignment was carried out using MAFFT tool for the consensus sequences fasta files for all the isolates (Version 7.407) (Nakamura et al., 2018). The output file generated after multiple sequence alignment using MAFFT was further used to constructing the phylogenetic tree. The maximum-likelihood method was implemented in IQ-TREE for constructing the phylogenetic tree (Nguyen et al., 2015). The output was visualized with Dendroscope (version-3.5.8) (Huson and Scornavacca, 2012).
We compiled list of genes associated with drug resistance from literature and databases and a list of non-synonymous SNVs observed in these genes among drug-resistant isolates (Supplementary Table S5).
Different drug resistant categories (Sensitive to first line drugs, MDR, Rifampicin mono-resistant, Isoniazid mono-resistant, Streptomycin mono-resistant, Pre-XDR) were used to identify the SNVs that were observed in these genes among drug-resistant isolates. The proportion of drug-resistant isolates (FR) in which a given gene was mutated minus the proportion of drug-sensitive isolates (FS) in which the same gene was mutated (i.e., FR – FS) was calculated, The mean value and standard deviation of this dataset was used to obtain the quantile P-value (P < 0.05) of the corresponding normal distribution for each gene (Zhang et al., 2013). The resulting non-synonymous SNVs were designated as relatively high-frequency non-synonymous SNVs in the drug-resistant isolates. A two-tailed t-test was used to compare and characterize the significant mutations. Bonferroni-corrected P-values were calculated using the hyper geometric function (entries with P-value ≤ 0.05).
The matrix of mutations associated with drug resistance for isolates from our dataset and isolates from NIRT study was generated to identify co-occurring and compensatory mutations (Manson et al., 2017b).
We carried out WGS of 200 culture confirmed M. tuberculosis clinical isolates from North India spanning a broad range of drug resistance profiles (50 sensitive to four first line drugs, 12 rifampicin mono-resistant, 31 isoniazid mono-resistant, 6 streptomycin mono-resistant, 91 MDR and 10 Pre-XDR) (Supplementary Table S1). The isolates were collected over a period of 4 years (2010–2014) at the National JALMA Institute of Leprosy and other Mycobacterial Diseases, Agra, India. We compared genetic diversity between clinical isolates from North India and South India. In addition, we determined novel genetic variations observed in isolates from India when compared with publicly available WGS data of isolates from rest of the world.
The M. tuberculosis genome is 4.4 million base pairs long and encodes for approximately 4,000 genes (Cole et al., 1998). All the 200 culture confirmed isolates sequenced in this study were aligned against M. tuberculosis H37Rv reference genome (NC_000962.3). We observed poor sequence alignment for 39 isolates (Supplementary Table S1). As co-infections are generally suspected in TB, we performed alignment of these 39 isolates with multiple non-tuberculous mycobacterium species. Our analysis indicated co-infection (Figure 1).
Figure 1. Isolates identified as co-infections. The length of bars indicates proportion of reads that were aligned to corresponding mycobacterial species. (WHO definitions for case history. (i) New case- Patient has never been treated for TB. (ii) Retreatment case- Patient on retreatment regimen with first-line drugs. (iii) Second-line treatment- Patient has been started directly on second-line treatment for MDR-TB or RR-TB, without being started on a first line drugs. (iv) Relapse case- Patient has been previously treated for TB and are now diagnosed with a recurrent episode of TB. (v) Not known- Case history unavailable).
The reads from 20% (n = 39) of the clinical isolates in our study aligned to genomes of multiple mycobacterial species, of which 9 were found to be NTMs and the other 30 were co-infection cases with M. tuberculosis. We observed M. mageritense to be prevalent in 46% (14/30) of the cases followed by M. intracellulare (7/30) and M. abscessus (4/30). M. mageritense is a recently described, non-pigmented infectious mycobacterium with closest similarity to the M. fortuitum third biovariant complex (Domenech et al., 1997). These clinical isolates were earlier reported to contain only M. tuberculosis by conventional culture-based methods. Of the 30 co-infection with M. tuberculosis cases 36% (11/30) were tuberculosis relapse cases and 53% (16/30) were resistant to first line anti-tubercular drugs (MDR) as per phenotypic DST (Figure 1). Our findings are in line with previous reports that have observed high prevalence of co-infections in cases with multidrug resistance and which results in adverse treatment outcomes (Gopinath and Singh, 2009; Fusco da Costa et al., 2013).
We called SNVs, insertions and deletions from the remaining 161 M. tuberculosis clinical isolates. A total of 18,970 non-synonymous SNVs (Supplementary Table S2), 3,052 insertions (Supplementary Table S3) and 2,739 deletions (Supplementary Table S4) were identified when compared with H37Rv genome. We compared the SNVs identified in our dataset with (i) those reported in genome-wide M. tuberculosis variation database (GMTV) (Chernyaeva et al., 2014), which has data from 2,044 M. tuberculosis isolates around the globe and (ii) 223 isolates from South India (Manson et al., 2017b). There were 12,802 SNVs that were unique to our dataset when compared to these publicly available datasets (Figure 2A). We carried out PCA to determine genetic diversity of isolates based on sequence variations. We used sequence variations cataloged from global data in GMTV, our dataset from North India and dataset from South India. PCA revealed distinct clustering of isolates from GMTV, North India and South India indicating genetic diversity of isolates from different regions (Figure 2B).
Figure 2. Genetic variations in Mycobacterium tuberculosis isolates. (A) Circos plot depicting novel SNVs and INDELS. (B) Principal Component Analysis (PCA) of non-synonymous SNVs from GMTV, isolates from South India and isolates from the current study (North India). Blue, green, and pink dots represent isolates from North India, South India and GMTV database, respectively.
We carried out phylogenetic analysis of 161 confirmed cases of M. tuberculosis isolates to determine lineage distribution. Phylogenetic analysis revealed that the isolates in our study belonged to seven different lineages with East-African-Indian (Lineage 3, n = 114) being the most common. Other lineages include Indo-Oceanic (Lineage 1, n = 23), East-Asian (Beijing) (Lineage 2.2, n = 10), Euro-American (Lineage 4, n = 6), Euro-American (X-type) (Lineage 4.1.1, n = 1) and Euro-American (H37Rv-like) (Lineage 4.9, n = 7) (Table 1). To examine Indian phylogeographical patterns, phylogenetic clustering of isolates from our study (North India) and isolates from South India was performed (Manson et al., 2017b). While East-African-Indian (lineage 3) was found prevalent in North India (70%, 114/161), Indo-Oceanic lineage was most prevalent in South (67.7%, 151/223) (Figure 3A). This observation is in agreement with previous findings (Gutierrez et al., 2006; Ahmed et al., 2009). East-African-Indian and Indo-Oceanic lineages are most prevalent in Indian subcontinent compared to elsewhere (Figure 3B).
Figure 3. Distribution of isolates. (A) Phylogenetic clustering of M. tuberculosis isolates from North and South India. (B) Geographical distribution of different M. tuberculosis lineages across the globe.
All isolates sequenced in this study were tested for phenotypic resistance for four first line drugs (rifampicin, isoniazid, ethambutol, and streptomycin). In addition, 10 of them were also tested for two second-line drugs kanamycin and ofloxacin (Supplementary Table S1). We compared our SNV data with known drug resistance causing SNVs. A total of 160 previously reported SNVs in 25 genes that are known to confer resistance to first- and second-line drugs were identified in our dataset (Supplementary Table S5) (Figure 4A). Of the 160 known SNVs, 35% (56/161) of the SNVs were exclusive to MDR-TB clinical isolates. In addition, 343 novel SNVs were identified in 38 genes, SNVs in which have been previously reported to confer drug resistance (Supplementary Table S6) (Figure 4B). We also observed 1,553 non-synonymous SNVs corresponding to 974 genes that were significantly enriched in drug resistant isolates compared to sensitive isolates (P-value ≤ 0.05) (Supplementary Table S7). Thus, we reasoned that drug resistant isolates should potentially be enriched with SNVs conferring resistance first and second-line drugs.
Figure 4. Frequency of SNVs that confer drug resistance. (A) Frequency of SNVs known to confer resistance against first and second-line anti-tubercular drugs in clinical isolates from North India. (B) Frequency of novel SNVs in genes that confer drug resistance.
Mutations in 81-bp rifampicin resistance-determining region (RRDR) of rpoB gene, also known as hot spot region, have been accurate predictors of rifampicin resistance (Telenti et al., 1993). We observed 21 known SNVs within this region in our dataset. The most common rifampicin resistance genotype, encoding a (S450L) substitution was identified in 28% (45/161) of clinical isolates (Mandin et al., 2007). We found the katG (S315T) SNV that confers resistance to isoniazid in 45% (72/161) of the isolates. Of the 72 isolates that carried this katG (S315T) SNV, 47 were MDR as per first line DST. On the other hand, SNV (S450L) in rpoB gene, which confers resistance to rifampicin, was found only in 28% (45/161) of the isolates.
We evaluated the prevalence of fluoroquinolone resistance among isolates sequenced in our study by looking at gyrA and gyrB gene mutation frequencies. We identified 26 SNVs in gyrA gene of which 10 are known to confer drug resistance to fluoroquinolones. Of the 10 known SNVs in gyrA gene, 8 were found to affect quinolone resistance-determining regions (QRDR) region. Additionally, the known SNV (S95T) in gyrA gene was found in 158 isolates. The gyrB gene was found to harbor 19 SNVs outside the QRDR region. Eight of these SNVs were found in 20% (33/161) isolates that are known to confer drug resistance. The SNV in (D94N) in gyrA gene which is known to confer fluoroquinolone resistance was found to be more prevalent in pre-XDR isolates. This SNV was identified in six isolates of which four were pre-XDR. The isolates sampled in the dataset from South India also display a similar frequency of SNV in gyrA gene thus, indicating the high prevalence of fluoroquinolone resistance in India (Figure 5A and Table 2).
Figure 5. Single nucleotide variations in genes that confer resistance to fluoroquinolone and bedaquiline. (A) SNVs in quinolone resistance-determining regions (QRDRs) of gyrA and gyrB genes. SNVs marked in blue are reported in isolates obtained from South India and SNVs marked in red are the ones identified in our study. (B) SNVs in Rv0678 gene that potentially confer bedaquiline resistance. SNVs marked in blue are reported earlier and SNVs marked in red are novel.
We identified a novel non-synonymous SNV (G162E) in Rv0678 gene in three MDR and one isoniazid mono-resistant clinical isolate in our dataset (Figure 5B). In addition, we also observed SNV (N148H) in three isolates from South India. The Rv0678 gene is known to be associated with bedaquiline resistance. Keeping in mind that bedaquiline is a fairly new anti-TB drug; the identification of SNVs in this gene suggests the dynamic and rapid evolution of M. tuberculosis clinical strains.
We investigated the occurrence of compensatory mutations in rpoC gene in the isolates carrying rifampicin resistance mutations in rpoB gene. Of the 51 isolates carrying SNVs in rpoB gene, 20% (10/51, P-value ≤ 0.05) were found to carry a SNV in rpoC gene (Figure 6). We observed (S450L) SNV in rpoB gene among 3.4% of isolates from South India compared to 25% among isolates from North India. However, the frequency of compensatory mutation (A172V) in rpoC gene in the same isolates was overwhelmingly high in isolates from South India (74%) than in North India (12.5%) (Supplementary Figure S1). In addition to identification of mutations in rpo genes, we also identified four major clusters of co-occurring mutations highly prevalent in specific lineages. The SNV (D229G) in accD6 gene (Rv2247) was exclusively found to be co-occurring with katG (R463L) in all East-Asian (Beijing) clade MDR and Pre-XDR isolates. Similarly, the gid (E92D) SNV was found to co-occur with rpsL (K43R) and was prevalent in East-Asian (Beijing) clade with MDR and pre-XDR drug resistance phenotype. We also observed a cluster of SNVs associated with ethambutol resistance, which were overrepresented with higher prevalence in East-African-Indian MDR clinical isolates which include – 2 novel SNVs embB (G406A) and embB (M306I) and one known SNV embB (M306V) (P-value ≤ 0.05).
Figure 6. Number of SNVs in rpo A, B, and C genes in different lineages in isolates from North and South India. (A) Colored bands represent number of SNVs in rpo A, B, and C genes in isolates from North India across different lineages. (B) Colored bands represent number of SNVs in rpo A, B, and C genes in isolates from South India across different lineages.
In this study, we carried out WGS of 200 culture confirmed Mycobacterium tuberculosis clinical isolates from a tertiary care centre in North India. WGS revealed 39 cases of M. tuberculosis co-infection with various species of NTM. Surprisingly, we observed M. mageritense to be the most prevalent NTM co-infection with M. tuberculosis unlike previous studies that have reported M. avium complex (MAC) and M. abscessus. NTM infections in high endemic countries such as India either go unrecognized or are misdiagnosed as pulmonary TB due to their indistinguishable clinical presentations (Gopinath and Singh, 2010). Several reports of concomitant identification of multiple infections with M. tuberculosis, M. avium and others have been published (Kox et al., 1995; Montessori et al., 1996; Fusco da Costa et al., 2013). Co-infections may lead to misidentification of strains and result in underestimation of disease transmission (Theisen et al., 1995; Niemann et al., 2000; van Rie et al., 2005). They also lead to erroneous drug-susceptibility profiles (DST) resulting in poor treatment outcomes. Using multiplex PCR assay, Gopinath and Singh (2009) have reported NTM infection in more than 17% of confirmed pulmonary TB cases suggesting a higher proportion of co-infections with M. tuberculosis cases missed during routine TB diagnosis. In addition, studies have also shown that majority of cases with co-infections were found to be drug resistant (Fusco da Costa et al., 2013).
Among several species of NTMs infecting humans, M. avium complex (MAC) and M. abscessus are reported to be more common (Gopinath and Singh, 2009; Nishiuchi et al., 2017). Most NTMs have been found to be either resistant or partially resistant to standard anti-tubercular drugs and require distinct treatment strategies (Gopinath and Singh, 2010). For example, M. mageritense is susceptible to ciprofloxacin and sulfamethoxazole and resistant to clarithromycin (Wallace et al., 2002). Further investigations are needed to evaluate if combined treatment would result in better outcomes among co-infected patients. Accurate diagnosis of these infections thus becomes critical for effective treatment. This highlights the significance of involving WGS approaches for diagnosis and treatment of tuberculosis.
Our study identified several novel genetic variations that were not previously reported in M. tuberculosis. Among these were several novel genetic variations in genes that are known to confer drug resistance. However, the implication of these novel SNVs on drug susceptibility is yet to be characterized. We also observed significant differences in frequency of these SNVs across different lineages. In a recent analysis of M. tuberculosis genomes from different countries, Manson et al. (2017b) have reported that majority of MDR-TB and XDR-TB strains evolved resistance to isoniazid before resistance to rifampicin. This is attributed to introduction of isoniazid for clinical use approximately 20 years before rifampicin resulting in selection of M. tuberculosis populations carrying mutations conferring isoniazid resistance. We observed similar trend from genotypic data on katG and rpoB in our dataset. Mutations in rpoB gene detected by Xpert MTB/RIF are those which occur later during infection. As other high-frequency mutations precede emergence of rpoB mutations, probes that monitor these high frequency mutations might be valuable to identify patients with higher risk of acquiring MDR-TB. The 343 novel SNVs identified in this study can further have important implications for designing rapid methods for detecting drug resistant strains. These findings signify that molecular assay kit diagnosis that is based on the rpoB and katG genes should be improved by incorporating SNVs associated with other drugs and considering geographic specificity.
TB strains that are resistant to any fluoroquinolone in addition to first line drugs and one of the three injectable second-line drugs, amikacin, kanamycin, or capreomycin are classified as extremely drug resistant (XDR) (Von Groll et al., 2009). Fluoroquinolones are a class of antibiotics, which inhibit DNA gyrases and thus prevent bacterial DNA synthesis. They play an important role in MDR-TB treatment (Moadebi et al., 2007). In addition to TB, fluoroquinolones have been extensively used for treatment of several bacterial infections including S. pneumoniae (Low, 2009). Increasing fluoroquinolone prescriptions and the expanded use of these broad-spectrum agents for many infections has resulted in emergence of fluoroquinolone resistance. Global surveillance studies demonstrate that fluoroquinolone resistance rates increased in the past years in almost all bacterial species (Dalhoff, 2012). This may be due to easy availability of these drugs without prescription in many places and indiscriminate use of them for undiagnosed respiratory tract infections (Dalhoff, 2012). Frequent cases of fluoroquinolone resistance in TB patients are impeding the use of this class of drug for TB treatment (Devasia et al., 2009). However, resistance to fluoroquinolones is not routinely evaluated in tuberculosis. Resistance to fluoroquinolones in M. tuberculosis is primarily due SNVs in gyrA or gyrB genes affecting the quinolone resistance-determining regions (QRDRs) that interacts with the drugs (Devasia et al., 2012; Maruri et al., 2012; Li et al., 2014). This data strongly suggests that more studies should be carried out from India which would contribute to the development, refinement, and improvement of rapid molecular diagnostic tests for determining fluoroquinolone resistance. This will also help in identification of MDR and pre-XDR TB in more timely and effective manner.
Bedaquiline is a fairly recent anti-tuberculosis drug. It has shown high potency against multi and extremely drug resistant cases. As of 2017, approximately 12,194 patients have been treated with bedaquiline (Tiberi et al., 2018). It was recently granted accelerated approval by FDA as part of combination therapy to treat adults with MDR-TB when an effective treatment regimen cannot otherwise be provided (Zumla et al., 2014). It targets ATP synthase activity in M. tuberculosis (Andries et al., 2005). Unfortunately, the rate at which mycobacteria are acquiring resistance to this newly introduced drug across the globe is alarming (Andries et al., 2014; Nguyen et al., 2017). A recent study showed that there is an alarming rate of increase in bedaquiline resistance in both patients with or without previous TB-treatment exposure (Veziris et al., 2017). It has been previously described that mutations in atpE gene (ATP synthase subunit c) is associated with bedaquiline resistance (Andries et al., 2014). However, target-based assays showed that approximately 30% of the isolates which are resistant to bedaquiline carry mutations in ATP synthase genes suggesting an alternate mechanism of resistance that does not involve drug target (Huitric et al., 2010; Ismail et al., 2018). Andries colleagues have shown that mutations in Rv0678 gene, which is not a target of bedaquiline, may account for non-target based resistance to bedaquiline and cross-resistance between clofazimine and bedaquiline. They demonstrated that Rv0678, which is also known as “mycobacterial membrane protein repressor” (MmpR5), is a negative regulator of MmpS5 and MmpL5. Mutations in this gene leads to an increased expression of this efflux pump. Several non-atpE mutants resistant to bedaquiline have been known to carry mutation in Rv0678, including missense mutations (G281A), single nucleotide insertions (Ins A 38–39), and IS6110 insertion sequences (IS6110 nt 272) (Milano et al., 2009; Ioerger et al., 2013). Considering mutations that potentially confer resistance to bedaquiline already exist in some of the isolates, it is important to monitor the efficacy of bedaquiline and its resistance pattern.
Recent studies have suggested that the fitness cost of antimicrobial resistance can be mitigated by compensatory mutations (Andersson and Hughes, 2010; Borrell and Gagneux, 2011). For example, M. tuberculosis carrying mutation rpoB (S450L) that acquires a compensatory mutation in rpoC restores its fitness and allows bacteria to thrive without any notable reduction in growth (Zhang et al., 2013). Compensatory mutations associated with rpoB gene harboring mutations in the 81-bp rifampicin resistance-determining region have been well-studied. Mutations in some genes also tend to co-occur in drug resistant bacteria. We observed co-occurring SNVs in gid (E92D) and rpsL (K43R) genes among the East Asian (Beijing) clade isolates sequenced in our study. gid (E92D) is a lineage specific polymorphism associated with Beijing clade (Spies et al., 2011) whereas rpsL (K43R) is the most common rpsL mutation significantly associated with Beijing strains compared to non-Beijing strains (Sun et al., 2010). In addition, gidB is reported to be a highly polymorphic gene suggesting it as a proposed target of selective pressure (Wong et al., 2011). These co-occurring and compensatory mutations can further be validated on large number of isolates which can provide information on drug resistance and further can be used as a novel approach for optimizing current treatment regimens.
As novel SNVs may have direct implications on drug resistance; country specific probes would be needed for rapid and effective diagnosis and treatment of drug resistant TB. Identification of large number of genetic variations in M. tuberculosis isolates sequenced in our study also suggests that such large-scale efforts are needed for comprehensive characterization of genetic diversity of M. tuberculosis. This is particularly true for isolates from Indian subcontinent as they are relatively underrepresented in global datasets.
The raw WGS data has been deposited in the NCBI Sequence Read Archive (SRA) (http://www.ncbi.nlm.nih.gov/sra) and can be accessed through the accession PRJNA379070.
TP, ST, and HG designed the study and managed the study. JA, RV, PP, JY, and RS collected isolates. RV, JA, PP, JY, RS, and FN cultured the isolates. PP, PU, RS, and JY performed drug susceptibility testing. RV, JA, and FN prepared DNA samples. JA performed whole genome sequencing analysis. RV and JA interpreted the data. JA, RV, and OC analyzed the data. RV, JA, OC, HG, and TP wrote the manuscript. OC, JA, and RV illustrated figures. TP, HG, ST, DC, AP, RR, SB, MS, and UG reviewed the manuscript. HG and TP directed the work. TP, HG, ST, AP, and DC designed and provided overall direction for the project.
This work was supported by internal funding from Yenepoya (Deemed to be University) and the Olav Thon Foundation grant on “Discovering new therapeutic targets and drugs to combat AMR tuberculosis.” JA is a recipient of Senior Research Fellowship from Council of Scientific & Industrial Research (CSIR) Government of India. RV is a recipient of Senior Research Fellowship from University Grants Commission (UGC), Government of India. OC is a recipient of INSPIRE Fellowship from the Department of Science and Technology (DST), Government of India.
The authors declare that the research was conducted in the absence of any commercial or financial relationships that could be construed as a potential conflict of interest.
The reviewer AK declared a shared affiliation, with no collaboration, with one of the authors, AP, to the handling Editor at the time of review.
We thank the Infosys Foundation and Department of Biotechnology (DBT), Government of India for research support to Institute of Bioinformatics.
The Supplementary Material for this article can be found online at: https://www.frontiersin.org/articles/10.3389/fmicb.2019.00309/full#supplementary-material
FIGURE S1 | Co-occurring mutations in M. tuberculosis clinical isolates. The mutations marked in red are the co-occurring mutations. (A) The highlighted clusters show the prevalence of these mutations in different lineages of M. tuberculosis in isolates from North India. (B) The highlighted clusters show the prevalence of these mutations in different lineages of M. tuberculosis in isolates from South India.
TABLE S1 | Patient details, characterization of isolates as per WGS analysis and drug susceptibility testing status of M. tuberculosis clinical isolates.
TABLE S2 | List of SNVs identified in the study.
TABLE S3 | (A) List of insertions identified in our study in the CDS region. (B) List of insertions identified in our study in the intergenic region.
TABLE S4 | (A) List of deletions identified in our study in the CDS region. (B) List of deletions identified in our study in the intergenic region.
TABLE S5 | List of SNVs in genes known to confer drug resistance and their relative frequency.
TABLE S6 | List of novel SNVs associated with first- and second-line drug resistance identified in the study.
TABLE S7 | List of SNVs that are identified in a higher proportion of drug resistant isolates than drug-sensitive isolates.
MDR, multi-drug resistant; MTB, Mycobacterium tuberculosis; MTBC, Mycobacterium tuberculosis complex; NCBI, National Centre for Biotechnology Information; NTM, non-tuberculous mycobacteria; SNVs, single nucleotide variations; TB, tuberculosis; XDR, extensively-drug resistant.
Ahmad, S., Mokaddas, E., Al-Mutairi, N., Eldeen, H. S., and Mohammadi, S. (2016). Discordance across phenotypic and molecular methods for drug susceptibility testing of drug-resistant mycobacterium tuberculosis isolates in a low tb incidence country. PLoS One 11:e0153563. doi: 10.1371/journal.pone.0153563
Ahmed, N., Ehtesham, N. Z., and Hasnain, S. E. (2009). Ancestral Mycobacterium tuberculosis genotypes in India: implications for TB control programmes. Infect. Genet. Evol. 9, 142–146. doi: 10.1016/j.meegid.2008.10.001
Andersson, D. I., and Hughes, D. (2010). Antibiotic resistance and its cost: is it possible to reverse resistance? Nat. Rev. Microbiol. 8, 260–271. doi: 10.1038/nrmicro2319
Andries, K., Verhasselt, P., Guillemont, J., Gohlmann, H. W., Neefs, J. M., Winkler, H., et al. (2005). A diarylquinoline drug active on the ATP synthase of Mycobacterium tuberculosis. Science 307, 223–227. doi: 10.1126/science.1106753
Andries, K., Villellas, C., Coeck, N., Thys, K., Gevers, T., Vranckx, L., et al. (2014). Acquired resistance of Mycobacterium tuberculosis to bedaquiline. PLoS One 9:e102135. doi: 10.1371/journal.pone.0102135
Apers, L., Mutsvangwa, J., Magwenzi, J., Chigara, N., Butterworth, A., Mason, P., et al. (2003). A comparison of direct microscopy, the concentration method and the mycobacteria growth indicator tube for the examination of sputum for acid-fast bacilli. Int. J. Tuberc. Lung Dis. 7, 376–381.
Banu, S., Rahman, S. M., Khan, M. S., Ferdous, S. S., Ahmed, S., Gratz, J., et al. (2014). Discordance across several methods for drug susceptibility testing of drug-resistant Mycobacterium tuberculosis isolates in a single laboratory. J. Clin. Microbiol. 52, 156–163. doi: 10.1128/JCM.02378-13
Borrell, S., and Gagneux, S. (2011). Strain diversity, epistasis and the evolution of drug resistance in Mycobacterium tuberculosis. Clin. Microbiol. Infect. 17, 815–820. doi: 10.1111/j.1469-0691.2011.03556.x
Canetti, G., Fox, W., Khomenko, A., Mahler, H. T., Menon, N. K., Mitchison, D. A., et al. (1969). Advances in techniques of testing mycobacterial drug sensitivity, and the use of sensitivity tests in tuberculosis control programmes. Bull. World Health Organ. 41, 21–43.
Casali, N., Nikolayevskyy, V., Balabanova, Y., Harris, S. R., Ignatyeva, O., Kontsevaya, I., et al. (2014). Evolution and transmission of drug-resistant tuberculosis in a Russian population. Nat. Genet. 46, 279–286. doi: 10.1038/ng.2878
Chatterjee, A., Nilgiriwala, K., Saranath, D., Rodrigues, C., and Mistry, N. (2017). Whole genome sequencing of clinical strains of Mycobacterium tuberculosis from Mumbai, India: a potential tool for determining drug-resistance and strain lineage. Tuberculosis 107, 63–72. doi: 10.1016/j.tube.2017.08.002
Chernyaeva, E. N., Shulgina, M. V., Rotkevich, M. S., Dobrynin, P. V., Simonov, S. A., Shitikov, E. A., et al. (2014). Genome-wide Mycobacterium tuberculosis variation (GMTV) database: a new tool for integrating sequence variations and epidemiology. BMC Genomics 15:308. doi: 10.1186/1471-2164-15-308
Cole, S. T., Brosch, R., Parkhill, J., Garnier, T., Churcher, C., Harris, D., et al. (1998). Deciphering the biology of Mycobacterium tuberculosis from the complete genome sequence. Nature 393, 537–544. doi: 10.1038/31159
Dalhoff, A. (2012). Global fluoroquinolone resistance epidemiology and implictions for clinical use. Interdiscip. Perspect. Infect. Dis. 2012:976273. doi: 10.1155/2012/976273
Danecek, P., Auton, A., Abecasis, G., Albers, C. A., Banks, E., Depristo, M. A., et al. (2011). The variant call format and VCFtools. Bioinformatics 27, 2156–2158. doi: 10.1093/bioinformatics/btr330
Devasia, R., Blackman, A., Eden, S., Li, H., Maruri, F., Shintani, A., et al. (2012). High proportion of fluoroquinolone-resistant Mycobacterium tuberculosis isolates with novel gyrase polymorphisms and a gyrA region associated with fluoroquinolone susceptibility. J. Clin. Microbiol. 50, 1390–1396. doi: 10.1128/JCM.05286-11
Devasia, R. A., Blackman, A., Gebretsadik, T., Griffin, M., Shintani, A., May, C., et al. (2009). Fluoroquinolone resistance in Mycobacterium tuberculosis: the effect of duration and timing of fluoroquinolone exposure. Am. J. Respir. Crit. Care Med. 180, 365–370. doi: 10.1164/rccm.200901-0146OC
Domenech, P., Jimenez, M. S., Menendez, M. C., Bull, T. J., Samper, S., Manrique, A., et al. (1997). Mycobacterium mageritense sp. nov. Int. J. Syst. Bacteriol. 47, 535–540. doi: 10.1099/00207713-47-2-535
Fusco da Costa, A. R., Falkinham, J. O. III, Lopes, M. L., Barretto, A. R., Felicio, J. S., Sales, L. H., et al. (2013). Occurrence of nontuberculous mycobacterial pulmonary infection in an endemic area of tuberculosis. PLoS Negl. Trop. Dis. 7:e2340. doi: 10.1371/journal.pntd.0002340
Gagneux, S., and Small, P. M. (2007). Global phylogeography of Mycobacterium tuberculosis and implications for tuberculosis product development. Lancet Infect. Dis. 7, 328–337. doi: 10.1016/S1473-3099(07)70108-1
Global Tuberculosis Who Report. (2018). WHO. Global Tuberculosis Report 2018. Geneva: World Health Organization.
Gopinath, K., and Singh, S. (2009). Multiplex PCR assay for simultaneous detection and differentiation of Mycobacterium tuberculosis, Mycobacterium avium complexes and other Mycobacterial species directly from clinical specimens. J. Appl. Microbiol. 107, 425–435. doi: 10.1111/j.1365-2672.2009.04218.x
Gopinath, K., and Singh, S. (2010). Non-tuberculous mycobacteria in TB-endemic countries: are we neglecting the danger? PLoS Negl. Trop. Dis. 4:e615. doi: 10.1371/journal.pntd.0000615
Gutierrez, M. C., Ahmed, N., Willery, E., Narayanan, S., Hasnain, S. E., Chauhan, D. S., et al. (2006). Predominance of ancestral lineages of Mycobacterium tuberculosis in India. Emerg. Infect. Dis. 12, 1367–1374. doi: 10.3201/eid1209.050017
Huitric, E., Verhasselt, P., Koul, A., Andries, K., Hoffner, S., and Andersson, D. I. (2010). Rates and mechanisms of resistance development in Mycobacterium tuberculosis to a novel diarylquinoline ATP synthase inhibitor. Antimicrob. Agents Chemother. 54, 1022–1028. doi: 10.1128/AAC.01611-09
Huson, D. H., and Scornavacca, C. (2012). Dendroscope 3: an interactive tool for rooted phylogenetic trees and networks. Syst. Biol. 61, 1061–1067. doi: 10.1093/sysbio/sys062
Ioerger, T. R., O’malley, T., Liao, R., Guinn, K. M., Hickey, M. J., Mohaideen, N., et al. (2013). Identification of new drug targets and resistance mechanisms in Mycobacterium tuberculosis. PLoS One 8:e75245. doi: 10.1371/journal.pone.0075245
Ismail, N. A., Omar, S. V., Joseph, L., Govender, N., Blows, L., Ismail, F., et al. (2018). Defining bedaquiline susceptibility, resistance, cross-resistance and associated genetic determinants: a retrospective cohort study. EBioMedicine 28, 136–142. doi: 10.1016/j.ebiom.2018.01.005
Kox, L. F., Van Leeuwen, J., Knijper, S., Jansen, H. M., and Kolk, A. H. (1995). PCR assay based on DNA coding for 16S rRNA for detection and identification of mycobacteria in clinical samples. J. Clin. Microbiol. 33, 3225–3233.
Laurenzo, D., and Mousa, S. A. (2011). Mechanisms of drug resistance in Mycobacterium tuberculosis and current status of rapid molecular diagnostic testing. Acta Trop. 119, 5–10. doi: 10.1016/j.actatropica.2011.04.008
Li, H., and Durbin, R. (2009). Fast and accurate short read alignment with Burrows-Wheeler transform. Bioinformatics 25, 1754–1760. doi: 10.1093/bioinformatics/btp324
Li, J., Gao, X., Luo, T., Wu, J., Sun, G., Liu, Q., et al. (2014). Association of gyrA/B mutations and resistance levels to fluoroquinolones in clinical isolates of Mycobacterium tuberculosis. Emerg. Microbes Infect. 3:e19. doi: 10.1038/emi.2014.21
Low, D. E. (2009). Fluoroquinolones for treatment of community-acquired pneumonia and tuberculosis: putting the risk of resistance into perspective. Clin. Infect. Dis. 48, 1361–1363. doi: 10.1086/598197
Mandin, P., Repoila, F., Vergassola, M., Geissmann, T., and Cossart, P. (2007). Identification of new noncoding RNAs in Listeria monocytogenes and prediction of mRNA targets. Nucleic Acids Res. 35, 962–974. doi: 10.1093/nar/gkl1096
Manson, A. L., Abeel, T., Galagan, J. E., Sundaramurthi, J. C., Salazar, A., Gehrmann, T., et al. (2017a). Mycobacterium tuberculosis whole genome sequences from southern india suggest novel resistance mechanisms and the need for region-specific diagnostics. Clin. Infect. Dis. 64, 1494–1501. doi: 10.1093/cid/cix169
Manson, A. L., Cohen, K. A., Abeel, T., Desjardins, C. A., Armstrong, D. T., Barry, C. E., et al. (2017b). Genomic analysis of globally diverse Mycobacterium tuberculosis strains provides insights into the emergence and spread of multidrug resistance. Nat. Genet. 49, 395–402. doi: 10.1038/ng.3767
Maruri, F., Sterling, T. R., Kaiga, A. W., Blackman, A., Van Der Heijden, Y. F., Mayer, C., et al. (2012). A systematic review of gyrase mutations associated with fluoroquinolone-resistant Mycobacterium tuberculosis and a proposed gyrase numbering system. J. Antimicrob. Chemother. 67, 819–831. doi: 10.1093/jac/dkr566
Milano, A., Pasca, M. R., Provvedi, R., Lucarelli, A. P., Manina, G., Ribeiro, A. L., et al. (2009). Azole resistance in Mycobacterium tuberculosis is mediated by the MmpS5-MmpL5 efflux system. Tuberculosis 89, 84–90. doi: 10.1016/j.tube.2008.08.003
Moadebi, S., Harder, C. K., Fitzgerald, M. J., Elwood, K. R., and Marra, F. (2007). Fluoroquinolones for the treatment of pulmonary tuberculosis. Drugs 67, 2077–2099. doi: 10.2165/00003495-200767140-00007
Montessori, V., Phillips, P., Montaner, J., Haley, L., Craib, K., Bessuille, E., et al. (1996). Species distribution in human immunodeficiency virus-related mycobacterial infections: implications for selection of initial treatment. Clin. Infect. Dis. 22, 989–992. doi: 10.1093/clinids/22.6.989
Nakamura, T., Yamada, K. D., Tomii, K., and Katoh, K. (2018). Parallelization of MAFFT for large-scale multiple sequence alignments. Bioinformatics 34, 2490–2492. doi: 10.1093/bioinformatics/bty121
Nguyen, L. T., Schmidt, H. A., Von Haeseler, A., and Minh, B. Q. (2015). IQ-TREE: a fast and effective stochastic algorithm for estimating maximum-likelihood phylogenies. Mol. Biol. Evol. 32, 268–274. doi: 10.1093/molbev/msu300
Nguyen, T. V. A., Anthony, R. M., Banuls, A. L., Vu, D. H., and Alffenaar, J. C. (2017). Bedaquiline resistance: its emergence, mechanism and prevention. Clin. Infect. Dis. 66, 1625–1630. doi: 10.1093/cid/cix992
Niemann, S., Richter, E., Rusch-Gerdes, S., Schlaak, M., and Greinert, U. (2000). Double infection with a resistant and a multidrug-resistant strain of Mycobacterium tuberculosis. Emerg. Infect. Dis. 6, 548–551. doi: 10.3201/eid0605.000518
Nikolayevskyy, V., Kranzer, K., Niemann, S., and Drobniewski, F. (2016). Whole genome sequencing of Mycobacterium tuberculosis for detection of recent transmission and tracing outbreaks: a systematic review. Tuberculosis 98, 77–85. doi: 10.1016/j.tube.2016.02.009
Nishiuchi, Y., Iwamoto, T., and Maruyama, F. (2017). Infection sources of a common non-tuberculous mycobacterial pathogen, mycobacterium avium complex. Front. Med. 4:27. doi: 10.3389/fmed.2017.00027
Peres, R. L., Maciel, E. L., Morais, C. G., Ribeiro, F. C., Vinhas, S. A., Pinheiro, C., et al. (2009). Comparison of two concentrations of NALC-NaOH for decontamination of sputum for mycobacterial culture. Int. J. Tuberc. Lung Dis. 13, 1572–1575.
Purwar, S., Chaudhari, S., Katoch, V. M., Sampath, A., Sharma, P., Upadhyay, P., et al. (2011). Determination of drug susceptibility patterns and genotypes of Mycobacterium tuberculosis isolates from Kanpur district, North India. Infect. Genet. Evol. 11, 469–475. doi: 10.1016/j.meegid.2010.12.010
Rigouts, L., Gumusboga, M., De Rijk, W. B., Nduwamahoro, E., Uwizeye, C., De Jong, B., et al. (2013). Rifampin resistance missed in automated liquid culture system for Mycobacterium tuberculosis isolates with specific rpoB mutations. J. Clin. Microbiol. 51, 2641–2645. doi: 10.1128/JCM.02741-12
Rufai, S. B., Kumar, P., Singh, A., Prajapati, S., Balooni, V., and Singh, S. (2014). Comparison of Xpert MTB/RIF with line probe assay for detection of rifampin-monoresistant Mycobacterium tuberculosis. J. Clin. Microbiol. 52, 1846–1852. doi: 10.1128/JCM.03005-13
Singh, M., Chauhan, D. S., Gupta, P., Das, R., Srivastava, R. K., Upadhyay, P., et al. (2009). In vitro effect of fluoroquinolones against Mycobacterium tuberculosis isolates from Agra & Kanpur region of north India. Indian J. Med. Res. 129, 542–547.
Spies, F. S., Ribeiro, A. W., Ramos, D. F., Ribeiro, M. O., Martin, A., Palomino, J. C., et al. (2011). Streptomycin resistance and lineage-specific polymorphisms in Mycobacterium tuberculosis gidB gene. J. Clin. Microbiol. 49, 2625–2630. doi: 10.1128/JCM.00168-11
Steiner, A., Stucki, D., Coscolla, M., Borrell, S., and Gagneux, S. (2014). KvarQ: targeted and direct variant calling from fastq reads of bacterial genomes. BMC Genomics 15:881. doi: 10.1186/1471-2164-15-881
Steingart, K. R., Ng, V., Henry, M., Hopewell, P. C., Ramsay, A., Cunningham, J., et al. (2006). Sputum processing methods to improve the sensitivity of smear microscopy for tuberculosis: a systematic review. Lancet Infect. Dis. 6, 664–674. doi: 10.1016/S1473-3099(06)70602-8
Sun, Y. J., Luo, J. T., Wong, S. Y., and Lee, A. S. (2010). Analysis of rpsL and rrs mutations in Beijing and non-Beijing streptomycin-resistant Mycobacterium tuberculosis isolates from Singapore. Clin. Microbiol. Infect. 16, 287–289. doi: 10.1111/j.1469-0691.2009.02800.x
Telenti, A., Imboden, P., Marchesi, F., Lowrie, D., Cole, S., Colston, M. J., et al. (1993). Detection of rifampicin-resistance mutations in Mycobacterium tuberculosis. Lancet 341, 647–650. doi: 10.1016/0140-6736(93)90417-F
Thakur, C., Kumar, V., and Gupta, A. K. (2015). Detecting mutation pattern of drug-resistant Mycobacterium tuberculosis isolates in Himachal Pradesh using GenoType((R)) MTBDRplus assay. Indian J. Med. Microbiol. 33, 547–553. doi: 10.4103/0255-0857.167336
Theisen, A., Reichel, C., Rusch-Gerdes, S., Haas, W. H., Rockstroh, J. K., Spengler, U., et al. (1995). Mixed-strain infection with a drug-sensitive and multidrug-resistant strain of Mycobacterium tuberculosis. Lancet 345:1512. doi: 10.1016/S0140-6736(95)91073-5
Thirumurugan, R., Kathirvel, M., Vallayyachari, K., Surendar, K., Samrot, A. V., and Muthaiah, M. (2015). Molecular analysis of rpoB gene mutations in rifampicin resistant Mycobacterium tuberculosis isolates by multiple allele specific polymerase chain reaction in Puducherry, South India. J. Infect. Public Health 8, 619–625. doi: 10.1016/j.jiph.2015.05.003
Tiberi, S., Du Plessis, N., Walzl, G., Vjecha, M. J., Rao, M., Ntoumi, F., et al. (2018). Tuberculosis: progress and advances in development of new drugs, treatment regimens, and host-directed therapies. Lancet Infect. Dis. 18, e183–e198. doi: 10.1016/S1473-3099(18)30110-5
van Rie, A., Victor, T. C., Richardson, M., Johnson, R., Van Der Spuy, G. D., Murray, E. J., et al. (2005). Reinfection and mixed infection cause changing Mycobacterium tuberculosis drug-resistance patterns. Am. J. Respir. Crit. Care Med. 172, 636–642. doi: 10.1164/rccm.200503-449OC
van Soolingen, D., Hermans, P. W., De Haas, P. E., Soll, D. R., and Van Embden, J. D. (1991). Occurrence and stability of insertion sequences in Mycobacterium tuberculosis complex strains: evaluation of an insertion sequence-dependent DNA polymorphism as a tool in the epidemiology of tuberculosis. J. Clin. Microbiol. 29, 2578–2586.
Veziris, N., Bernard, C., Guglielmetti, L., Le Du, D., Marigot-Outtandy, D., Jaspard, M., et al. (2017). Rapid emergence of Mycobacterium tuberculosis bedaquiline resistance: lessons to avoid repeating past errors. Eur. Respir. J. 49:1601719. doi: 10.1183/13993003.01719-2016
Von Groll, A., Martin, A., Jureen, P., Hoffner, S., Vandamme, P., Portaels, F., et al. (2009). Fluoroquinolone resistance in Mycobacterium tuberculosis and mutations in gyrA and gyrB. Antimicrob. Agents Chemother. 53, 4498–4500. doi: 10.1128/AAC.00287-09
Walker, T. M., Kohl, T. A., Omar, S. V., Hedge, J., Del Ojo Elias, C., Bradley, P., et al. (2015). Whole-genome sequencing for prediction of Mycobacterium tuberculosis drug susceptibility and resistance: a retrospective cohort study. Lancet Infect. Dis. 15, 1193–1202. doi: 10.1016/S1473-3099(15)00062-6
Wallace, R. J. Jr., Brown-Elliott, B. A., Hall, L., Roberts, G., Wilson, R., WMann, L. B., et al. (2002). Clinical and laboratory features of Mycobacterium mageritense. J. Clin. Microbiol. 40, 2930–2935. doi: 10.1128/JCM.40.8.2930-2935.2002
Wong, S. Y., Lee, J. S., Kwak, H. K., Via, L. E., Boshoff, H. I., Barry, C. E. III, et al. (2011). Mutations in gidB confer low-level streptomycin resistance in Mycobacterium tuberculosis. Antimicrob. Agents Chemother. 55, 2515–2522. doi: 10.1128/AAC.01814-10
Wood, D. E., and Salzberg, S. L. (2014). Kraken: ultrafast metagenomic sequence classification using exact alignments. Genome Biol. 15:R46. doi: 10.1186/gb-2014-15-3-r46
Ye, K., Schulz, M. H., Long, Q., Apweiler, R., and Ning, Z. (2009). Pindel: a pattern growth approach to detect break points of large deletions and medium sized insertions from paired-end short reads. Bioinformatics 25, 2865–2871. doi: 10.1093/bioinformatics/btp394
Zhang, H., Li, D., Zhao, L., Fleming, J., Lin, N., Wang, T., et al. (2013). Genome sequencing of 161 Mycobacterium tuberculosis isolates from China identifies genes and intergenic regions associated with drug resistance. Nat. Genet. 45, 1255–1260. doi: 10.1038/ng.2735
Keywords: fluoroquinolones, metagenomics, molecular genotyping, mycobacterial genetic heterogeneity, next generation sequencing
Citation: Advani J, Verma R, Chatterjee O, Pachouri PK, Upadhyay P, Singh R, Yadav J, Naaz F, Ravikumar R, Buggi S, Suar M, Gupta UD, Pandey A, Chauhan DS, Tripathy SP, Gowda H and Prasad TSK (2019) Whole Genome Sequencing of Mycobacterium tuberculosis Clinical Isolates From India Reveals Genetic Heterogeneity and Region-Specific Variations That Might Affect Drug Susceptibility. Front. Microbiol. 10:309. doi: 10.3389/fmicb.2019.00309
Received: 01 November 2018; Accepted: 05 February 2019;
Published: 26 February 2019.
Edited by:
Farhat Afrin, Taibah University, Saudi ArabiaReviewed by:
Jason Sahl, Northern Arizona University, United StatesCopyright © 2019 Advani, Verma, Chatterjee, Pachouri, Upadhyay, Singh, Yadav, Naaz, Ravikumar, Buggi, Suar, Gupta, Pandey, Chauhan, Tripathy, Gowda and Prasad. This is an open-access article distributed under the terms of the Creative Commons Attribution License (CC BY). The use, distribution or reproduction in other forums is permitted, provided the original author(s) and the copyright owner(s) are credited and that the original publication in this journal is cited, in accordance with accepted academic practice. No use, distribution or reproduction is permitted which does not comply with these terms.
*Correspondence: Srikanth Prasad Tripathy, c3Jpa2FudGh0cmlwYXRoeUBnbWFpbC5jb20= Harsha Gowda, aGFyc2hhQGliaW9pbmZvcm1hdGljcy5vcmc= T. S. Keshava Prasad, a2VzaGF2QHllbmVwb3lhLmVkdS5pbg==
†These authors have contributed equally to this work.
‡Present address: Shashidhar Buggi, National Institute of Mental Health and Neurosciences, Bengaluru, India Srikanth Prasad Tripathy, National Institute for Research in Tuberculosis, Chennai, India
Disclaimer: All claims expressed in this article are solely those of the authors and do not necessarily represent those of their affiliated organizations, or those of the publisher, the editors and the reviewers. Any product that may be evaluated in this article or claim that may be made by its manufacturer is not guaranteed or endorsed by the publisher.
Research integrity at Frontiers
Learn more about the work of our research integrity team to safeguard the quality of each article we publish.