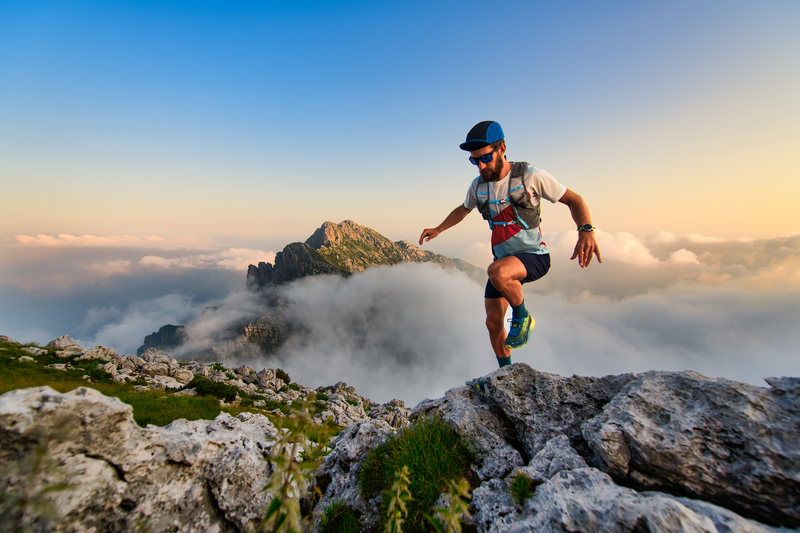
95% of researchers rate our articles as excellent or good
Learn more about the work of our research integrity team to safeguard the quality of each article we publish.
Find out more
ORIGINAL RESEARCH article
Front. Microbiol. , 22 February 2019
Sec. Microbiotechnology
Volume 10 - 2019 | https://doi.org/10.3389/fmicb.2019.00257
This article is part of the Research Topic Microbial Ecotoxicology View all 42 articles
Unraveling the relative importance of both environmental conditions and ecological processes regulating bacterioplankton communities is a central goal in microbial ecology. Marine coastal environments are among the most urbanized areas and as a consequence experience environmental pressures. The highly anthropized Toulon Bay (France) was considered as a model system to investigate shifts in bacterioplankton communities along natural and anthropogenic physicochemical gradients during a 1-month survey. In depth geochemical characterization mainly revealed strong and progressive Cd, Zn, Cu, and Pb contamination gradients between the entrance of the Bay and the north-western anthropized area. On the other hand, low-amplitude natural gradients were observed for other environmental variables. Using 16S rRNA gene sequencing, we observed strong spatial patterns in bacterioplankton taxonomic and predicted function structure along the chemical contamination gradient. Variation partitioning analysis demonstrated that multiple metallic contamination explained the largest part of the spatial biological variations observed, but DOC and salinity were also significant contributors. Network analysis revealed that biotic interactions were far more numerous than direct interactions between microbial groups and environmental variables. This suggests indirect effects of the environment, and especially trace metals, on the community through a few taxonomic groups. These spatial patterns were also partially found for predicted bacterioplankton functions, thus indicating a limited functional redundancy. All these results highlight both potential direct influences of trace metals contamination on coastal bacterioplankton and indirect forcing through biotic interactions and cascading.
Marine coastal areas are increasingly subjected to anthropogenic pressures that usually result in human-induced chemical contamination. Increasing demographic pressure and both terrestrial and marine activities threaten marine ecosystems (Halpern et al., 2008; The MerMex Group et al., 2011). Urban and industrial wastes (Levin et al., 2001; Oursel et al., 2013), biocides released by antifouling coatings (Turner, 2010) and fuel from nautical traffic (Callender, 2003) as well as historical pollution accumulated in the sediment compartment (Xu et al., 2014; Dang et al., 2015) are well-known and widespread examples. Such chemical contamination represents an increasing threat for marine coastal areas, and microorganisms inhabiting these ecosystems (Misson et al., 2016; Othman et al., 2017; Coclet et al., 2018).
Microbial communities have been shown to be a major component of marine planktonic ecosystems (Fuhrman and Azam, 1980), variable across space (Pommier et al., 2007; Ghiglione et al., 2012) and time (Fuhrman et al., 2006; Fortunato et al., 2012; Gilbert et al., 2012). Multiple environmental factors are known to drive temporal and spatial dynamics in bacterioplankton, such as salinity (Lozupone and Knight, 2007), temperature (Fuhrman et al., 2008; Gilbert et al., 2009), depth (Treusch et al., 2009), grazing, and predation (Zubkov and López-Urrutia, 2003) and resource availability (Fortunato et al., 2012; Gilbert et al., 2012; Chow et al., 2013).
Bacterioplankton communities have been largely characterized spatially or temporally in various environments, but rarely assessed over both spatial and temporal scales in a marine coastal area. These ecosystems are usually characterized by natural gradients linked to the continent-sea interface and if anthropized by a complex set of chemical contaminants, potentially affecting marine microbial communities. The links between benthic microbial communities and trace metal contamination have been extensively revealed (Gough and Stahl, 2011; Pringault et al., 2012; Quero et al., 2015; Misson et al., 2016). But how the marine bacterioplankton communities responds to trace metal exposure was scarcely investigated in coastal marine environments (Wang et al., 2015; Qian et al., 2017). Moreover, chemical perturbation on bacterioplankton communities is supposed to be complex because multiple trace metallic contaminations of the water column can result in additive, synergistic or antagonistic effects (Franklin et al., 2002; Lorenzo et al., 2002). Effects of trace metals can be dependant of hydrology, seasonal, and environmental conditions (Cloern, 2001). Furthermore, the impact of contamination on bacterioplankton may also be influenced by bacterio-phytoplankton coupling. Consequently, effects of contaminants on phytoplankton, which is sensitive to trace metal contamination (Coclet et al., 2018) might have indirect consequences for bacterioplankton.
Despite several studies describing microbial taxonomic diversity in marine coastal environments, functional diversity has not been investigated intensely (Jeanbille et al., 2016). Elucidating both taxonomic and functional diversity of microbial communities is the key to understand their roles in the ecosystem (Wang et al., 2016). Recent studies have related functional diversity of microbial communities to specific habitats (Dinsdale et al., 2008), water column zone (Louca et al., 2016), seasonal changes (Ward et al., 2017) and nutrient gradients (Thompson et al., 2017; LeBrun et al., 2018). However, relationships between trace metal contamination and functional profiles related to taxonomy in seawater ecosystems are still lacking.
Trace metals contamination was studied in a number of previous studies where authors mention benthic (Pringault et al., 2012; Besaury et al., 2014; Misson et al., 2016), phytoplanktonic (Othman et al., 2017) or freshwater communities (Wang et al., 2018). This study represents the first comprehensive assessment of bacterioplankton communities living in a contaminated marine coastal ecosystem. We combined in-depth physicochemical and geochemical characterizations of Toulon Bay seawater (north-western Mediterranean Sea), flow cytometry microbial enumerations and 16S rRNA gene-based high-throughput sequencing water samples over a weekly sampling campaign (weekly during 2015, June). The objectives of the study were (i) to study both spatial and temporal patterns in bacterioplankton communities along the contaminated Toulon Bay, (ii) to reveal the main environmental factors driving bacterioplankton communities, more precisely the contribution of trace metals through direct or indirect effects; and (iii) to identify the predicted functional profile’s response of bacterioplankton communities to chemical contamination in order to assess its influence on the ecosystem functioning.
Located in the south of France, Toulon Bay is divided into two basins by an artificial seawall: the Little Bay, which is semi-enclosed, and the Large bay, which opens onto to the Mediterranean Sea (Supplementary Figure S1). The Little Bay is characterized by a high level of anthropic activities (Navy harbor, ferry transport, industries, wastewater sewage, and aquaculture). Due to its location, the Little Bay is directly impacted by pollution and is less affected than the Large Bay by offshore hydrodynamics. This confers to the Little bay a higher trace metal contamination than the Large Bay as demonstrated previously in the sediments and with punctual measurements in the water column (Tessier et al., 2011; Coclet et al., 2018).
Sampling was performed weekly from June 1st to 29th June, 2015. Two liters of seawater from the surface (1 m depth) and the bottom (1 m above sediment) of the water column were sampled at five sites across Toulon Bay (North-Western Mediterranean Sea, France), from the entrance of the bay to the north-western anthropized area (Supplementary Figure S1, Supplementary Table S1, and details in Supplementary Material). After the T1 sampling in site 41p, the French Navy performed an exercise that could have modified the water quality. In order to check that, we sampled again the water (T1b). The five sites were chosen to cover the whole range of dissolved metal contamination on the basis of trace metal distribution previously evaluated in Toulon Bay surface seawater (Coclet et al., 2018; Figure 1). Water samples were stored in a cooler and filtered through 0.2 μm polycarbonate membranes (Millipore). Filters were stored at −80°C until DNA extraction. Physico-chemical parameters including metals were weekly analyzed during the sampling period in order to characterize the environment (details in Supplementary Material). Standard oceanographic properties, including water temperature (°C), salinity, O2 (mg L−1 and %), pH, and chlorophyll a (chla) (μg L−1) were measured on sites using a multi-parameter probe (Hydrolab DS5, OTT).
Figure 1. Dynamics of dissolved trace metals concentrations in surface seawater at the different sampling stations. Symbols and errors bars represent average and standard deviation, respectively.
Subsamples of 10 mL of seawater were sampled at each site, depth, and date, filtered through a 90 μm nylon mesh, fixed with 0.25% (final concentration) glutaraldehyde on field and stored at −80°C until flow cytometry analysis. Autotrophic prokaryotes (Synechococcus-like), eukaryotic phytoplankton (picoeukaryotes and nanoeukaryotes) populations were characterized and enumerated using a BD AccuriTM C6 (BD Biosciences) flow cytometer, as previously described (Coclet et al., 2018). Heterotrophic prokaryotes were enumerated after staining with SYBR Green as previously described (Cabrol et al., 2017).
DNA was extracted from the Millipore filters by a combination of enzymatic cell lysis (Ghiglione et al., 2009) and AllPrep DNA/RNA Mini Kit (QIAGEN) according to the manufacturer’s instructions. The protocol for the DNA extraction and the library preparation is fully described in Supplementary Material. We assessed bacterial community composition (BCC) by targeting the V4–V5 region of the 16S rRNA gene (Parada et al., 2016) and using Illumina Miseq 2 × 250 pb paired-end sequencing (Genoscreen, France).
Sequences were then demultiplexed and assigned to corresponding samples using CASAVA (Illumina). Forward and reverse reads were merged using PEAR 0.9.8 with default options (Zhang et al., 2014). Raw sequences were analyzed using MacQiime v.1.9.1 software (Caporaso et al., 2010). Briefly, barcode, primer, shorter sequences (<100 bp in length), and sequences with ambiguous base calls or homopolymer runs exceeding 10 bp were removed. The remaining sequences were assigned to operational taxonomic units (OTUs) and clustered at a 97% threshold using Uclust algorithm (Edgar, 2010), both closed and open reference OTU picking, based on the SILVA (release 128) database (Pruesse et al., 2007; Quast et al., 2013). Low abundance OTU (<0.005%) were filtered as recommended by Bokulich et al. (2013). Sequences classified as mitochondria or chloroplast were removed from the OTU table, corresponding to 3393 OTUs. A total of 349,009 reads were finally obtained representing 31,132 OTUs. OTU table was normalized by random subsampling to the smallest number of sequences (i.e., 4044). Samples with lower number of sequences were discarded (n = 16), 36 samples were remaining for following analyses (Supplementary Table S1). The 16S rRNA gene sequences have been deposited in the NCBI Sequence Read Archive (SRA) database under BioProject ID PRJNA5142221.
All plots and statistical analysis were done in R RStudio (R Core Team, 2015). Alpha diversity calculations and error estimates were averaged, using QIIME script core_diversity_analyses.py (Caporaso et al., 2010), including estimates of rarefaction, Chao1, equitability, Shannon, and Simpson’s diversity. In order to compare the sites based on their chemical composition, taxonomic and functional community profiles, seawater samples were sorted in a non-metric multidimensional scaling (NMDS). Pairwise dissimilarities were calculated using Bray–Curtis metrics. Each resulting dissimilarity matrix was used to visualize sample differences via NMDS ordination using the “vegan” package in R (Oksanen et al., 2016). To test the null hypothesis that there were no significant difference between the groups discriminated according to sampling stations, sampling dates, and depth, similarities were analyzed by global and pairwise PERMANOVA tests, using “vegan” and “RVAideMemoire” packages, respectively.
To identify taxa that discriminated the sampling sites, the linear discriminant analysis (LDA) effect size method (LEfSe) (Segata et al., 2011) was used. This was performed with the LEfSe online tool in the Galaxy framework, using all default setting for data formatting and LDA effect size (Goecks et al., 2010). A similarity percentage (SIMPER) was run with a 90% cutoff and used to rank the percent contribution of individual biomarker to the dissimilarity between site differences (Clarke, 1993).
To investigate the relationships between BCC and measured environmental variables, Spearman rank correlation analysis, and redundancy analysis (RDA) were performed using the “vegan” package in R (Oksanen et al., 2016) (details in Supplementary Material). Briefly, for variable reduction and in order to create an efficient model from the most significant explanatory variables, vegan’s ordistep function were applied and among the 31 explanatory variables, 20 significant variables (P < 0.05) were kept for the following analyses The RDA model was tested by performing partial RDA, and variation partitioning to test the significance of the contribution of both groups of variables and each individual variable.
Interactions among the OTUs and between these OTUs and the 31 environmental variables were evaluated using CoNeT (Co-occurrence Network) plugin 1.1.1.beta (Faust et al., 2012), a plugin in a Cytoscape software 3.4.0. A similarity matrix was built with different metrics (Spearman correlation and Kullback–Leibler distance and a mutual information score) from OTUs. This initial network was re-defined by randomization. A permutation matrix representing a null distribution was obtained by resampling OTUs as described in Faust et al. (2012). In a permutation step, edge specific p-values were computed; however, for the final network, p-values of an edge were merged into one p-value according to Brown’s method. In the final step, the Benjamini–Hochberg multiple testing correction was applied (P < 0.05). Network characterization was evaluated using betweenness centrality (BC) and closeness centrality (CC) (details in Supplementary Material). Highly connected clusters were identified using the MCODE plugin version 1.4.0.beta2 (Smoot et al., 2011). Network characterization were evaluated using different topological indexes generated by Network Analyzer plugin.
Functional profiles were predicted from obtained 16S rRNA gene data using Tax4Fun (Aßhauer et al., 2015) based on KEGG category.
An increase in the trace metal concentrations was rationally observed from a reference site open on the sea with low metal concentrations (41p) to enclosed sites in the most anthropized area (MIS and 6ext) with high level of contaminations (Figure 1). The highest trace metal concentrations found in surface seawater were Zn, Cu, Pb, and Cd, while other metals/metalloids (As, Ba, Be, Cs, Cr, Mn, Sb, Sn, Ti, U, and V) were kept to concentration levels close to the geochemical background (Figure 1 and Supplementary Table S2). At the surface of seawater, Cd, Cu, Zn, and Pb concentrations were 6, 33, 35, and 48 times higher in 6ext water than in 41p water, respectively. These enrichment factors were quite similar (8, 25, 43, and 73 times) at the bottom of the seawater (Supplementary Figure S2). For both surface and bottom seawater, the concentrations of trace metals/metalloids were relatively stable during the sampling period (Figure 1, Supplementary Figure S2, and Supplementary Table S2). The most contaminated sites MIS and 6ext were significantly discriminated from the reference site 41p (PERMANOVA, P < 0.01) (Supplementary Figure S3A) and from sampling date (Supplementary Figure S3B) but not with depth (Supplementary Figure S3C).
Seawater temperature increased gradually during the studied period at all five sites, from 18.8°C–21.2°C in T0 to 22.0°C–24.3°C in TF (Supplementary Figure S4). Additionally, temperature was lower (1.9 ± 0.04°C) in bottom than in surface seawater. Surface salinity levels demonstrated low variations (38.4 ± 0.11). A very punctual drop was recorded in all sites at T2, down to 36.7 in MIS (Supplementary Figure S4). Chlorophyll a in surface seawater were lower in 41p (0.16 to 0.31 mg L−1) than in other sites (0.59 to 2.08 mg L−1). Additionally, chlorophyll a concentrations peaked in all sites at the middle of the survey. Except for 41p, chlorophyll a concentrations were higher at the bottom seawater than at the surface (Supplementary Figure S4). On average, in surface seawater, higher concentrations of DOC were found in 6ext (1.4 ± 0.07 mg L−1) compared to 41p (1.2 ± 0.06 mg L−1) (Supplementary Figure S4). Similarly, higher concentrations of TN were found in MIS (0.15 ± 0.02 mg L−1) compared to 41p. (0.08 ± 0.02 mg L−1) (Supplementary Figure S4). No significant difference was found in dissolved oxygen concentrations (mg L−1, %), and pH between both all five sites and all sampling date (Supplementary Table S2). NO3- and PO43- concentrations of were below the detection limit (0.02 and 0.020 μM, respectively) in a majority of samples. As a consequence, they are not presented and will not be considered further.
Heterotrophic bacterioplankton abundances estimated using flow cytometry are shown in Supplementary Figure S5. We observed an increase in the bacterioplankton abundance from the reference site 41p (3.8 × 105 to 4.2 × 105 cell mL−1) to the most enclosed site 6ext (8.0 × 105 to 1.4 × 106 cell mL−1) in both surface and bottom seawater. Additionally, heterotrophic bacterioplankton abundance was stable in 41p during the experiment whatever the depth, while strong dynamic was observed in both surface and bottom 6ext seawater, with highest abundances in T2 and T3, respectively.
Over the sampling, observed richness, and Chao1 index (Supplementary Figure S6) were significantly higher in the uncontaminated site 41p and declined to less than half compared to 6ext (ANOVA, P < 0.01). In contrast, Shannon, Simpson, and evenness indexes did not significantly vary between sampling sites (Supplementary Figure S6).
NMDS results showed a significant segregation of bacterioplankton communities between both sampling sites (PERMANOVA; P = 0.001) and dates (PERMANOVA; P = 0.024) but not with depths (PERMANOVA; P = 0.983) (Figure 2A and Supplementary Table S3). More precisely, pairwise PERMANOVA tests (Supplementary Table S3) confirmed significant differences between the reference 41p site and all other sites (PERMANOVA; P < 0.05) and significant differences between 6ext and Pt12–Pt15 (PERMANOVA; P < 0.05).
Figure 2. Spatial variations of bacterioplankton community structure. (A) Non-metric dimensional scaling (nMDS) ordination based on Bray–Curtis dissimilarity for 16S rRNA gene libraries between the different sampling sites. Each symbol corresponds to a distinct site, time, and depth point. (B) Bar plot showing the average family-level contribution for each site. Families that on average comprised less than 1% of the libraries are grouped as “Others.” Colors indicate different classes within phyla.
The most abundant phyla ( > 1% of all sequences across all samples) were Proteobacteria (53 ± 1.1%), followed by Bacteroidetes (28 ± 1.1%), Cyanobacteria (9.1 ± 0.7), Actinobacteria (6.1 ± 0.6), and Verrucomicrobia (3.0 ± 0.3%). At the family level (Figure 2B), Flavobacteriacea comprised the majority (22 ± 1.1%) of the total sequences, followed by Alphaproteobacteria groups SAR11 Surface 1 clade (18 ± 1.1%), Rhodobacteraceae (13 ± 0.65%) and SAR116 clade (6.1 ± 0.49%). Cyanobacteria, Actinobacteria, and Verrucomicrobia phyla were dominated by Synechococcus Family I (9.1 ± 0.72%), Microbacteriaceae (4.9 ± 0.71%), and Puniceicoccaceae (1.7 ± 0.19%). Archaea represented less than 0.1% of the total sequences, thus they were considered as “Others” in this study and variations of this group have not been taken into consideration.
Clear shifts in the relative abundance of individual bacterioplankton OTUs were observed between sampling sites. Using LEfSe analysis, we determined 43 differentially abundant taxa between four sites. 41p and 6ext, the two most geochemically contrasted sites, exhibited the highest number of taxonomic indicators (25 and 6, respectively) (Figure 3). For 41p site, the most significantly enriched bacterioplankton sequences were Acidimicrobiia Candidatus Actinomarina, members of the family SAR406, 7 Alphaproteobacteria OTUs and Gammaproteobacteria Thiothrix. Actinobacteria Candidatus Aquilina and both Flavobacteriia Formosa and Betaproteobacteria Hydrogenophilaceae were found to be overrepresented in MIS and 6ext, respectively. SIMPER analysis revealed that, on average, 13 biomarkers were identified as major contributors to differences between sites detected in the NMDS. The higher number of biomarkers identified as major contributors was found between 41p and the other sampling sites (between 17 and 32 biomarkers) (Supplementary Table S4). Overall, each biomarker contributed to at least 1% of the dissimilarity between sites. Candidatus Aquilina, PS1 clade, NS7 marine group, Planktomarina and Tenacibaculum, notably, drove together more to 32% of disparity between cluster 41p and the other clusters (Pt15, 6ext, and MIS).
Figure 3. Taxonomic tree generated using LefSe analysis (P < 0.05, LDA effect size > 2) highlighting the biomarkers that statistically differentiate sampling sites. No biomarker was evidenced for the sampling site Pt12. The roots of cladogram stand for the domain (i.e., Bacteria and Archaea), and concentric circles represent the following taxonomic levels until the tips standing for genera.
Among the 31 environmental variables available for multivariate analysis, 20 parameters were selected by pairwise correlations. The first and the second axes of RDA captured 47.1% and 6.4% of BCC variance, respectively (Figure 4A).
Figure 4. Contributions of environmental variables to spatial differentiation in bacterioplankton community structure. (A) Redundancy analysis (RDA) ordination diagram of the first two axes for bacterioplankton community composition. The percentage of the spatial variation in community structure explained by each axis is indicated in parentheses after the axis label. The constrained sets of environmental variables analyzed are indicated as vectors. NanoEuk: Nanoeukaryote abundance; PicoEuk: Picoeukaryotes abundance. (B) Venn diagram showing the variation partitioning (%) of the spatial variations in bacterioplankton community structure among four environmental datasets (Contaminants, Terrigenous elements, Marine tracers, and Nutrient and Biotic).
Variation partitioning provided more details about the relative contributions of nutrients, contaminants, terrigenous, and “others” factors in the observed spatial changes in BCC (Figure 4B). The combination of the four sets of explanatory variables explained 41% of BCC variation across sites. Among the set of explanatory variables, contaminants were the main contributors to variations in BCC (30%). Contaminants also appeared to have significant influence on BCC through their interaction with the other sets of variables. The contribution of terrigenous (19.6%), nutrient and biotic (15.0%) and “marine tracers” (21.6%) variables were also significant but to a lower extent than the contribution of contaminants variables. The highest individual contributions were attributed to Mn (1.2%), DOC (1.2%), salinity (1.1%), Zn (1.1%), and Cd (1.0%) (Supplementary Table S5). Thus, among the five explanatory variables which contributed the most to the biological variation, three variables were contaminants (Mn, Cu, and Cd).
Network analysis resulted in a global network representing 158 OTUs and 21 environmental variables significantly connected. Among 1664 edges, 92% were inter taxa edges, and 8% represented taxon-environment interactions. Most of the connections (67%) corresponded to negative interactions. To visualize the results, eight subnetworks were extracted from the global network (Figure 5 and Supplementary Figure S7).
Figure 5. Co-occurrence subnetworks of bacterial taxa and environmental variables derived from the OTU table and showing only statistically significant correlations. Node size correspond to the relative abundance of the OTU. Nodes colors correspond to main bacterioplankton classes. Green and red lines represent positive and negative correlations, respectively.
Globally, by plotting BC vs. CC, 12 OTUs with the highest values for both parameters (arbitrarily determined as BC > 0.02 and CC > 0.5) were selected, representing putative keystone species within Toulon Bay (Supplementary Table S6). Both of these indices indicate that SAR11 Surface 1 clade and Rhodobacteraceae (Alphaproteobacteria), Synechococcus (Cyanobacteria), Candidatus Aquiluna (Actinobacteria), and Balneola (Bacteroidetes) were central in this network. Most of Alphaproteobacteria, including SAR11, SAR116, and Rhodobacteraceae, and Cyanobacteria Synechococcus were positively correlated with each other, but negatively correlated with Bacteroidetes (NS11-12 and NS5 marine group, Cryomorphaceae and Balneola), and Actinobacteria (Candidatus Aquilina).
In the subnetworks, there were 19 correlations with contaminants (8 with Pb, 6 with Cu, 3 with Cd, and 2 with Zn) and two correlations with chlorophyll a. Several taxa that were identified as being key drivers of community dissimilarity using LEfSe analysis were highly correlated to contaminants. In particular, the Alphaproteobacteria Rhodobacteraceae and SAR11, the Bacteroidetes Balneola and the Cyanobacteria Synechococcus showed negative correlations with at least one of the contaminants (Cd, Cu, Pb, or Zn). Conversely, Actinobacteria Candidatus aquilina and Bacteroidetes NS11-12 marine group were positively correlated with Pb and Cu, respectively. Gammaproteobacteria Haliaceae was positively correlated with Cd.
The functional profiles of bacterioplankton communities was predicted using Tax4Fun (Aßhauer et al., 2015). Predicted functions were classified as KEGG orthologs (KOs) resulting in the identification of 3619 KOs across all samples, using on average, 60 ± 12% of total OTUs (Supplementary Table S7). Clustering showed that the differentiation of the sampling sites based on microbial predicted function profiles followed the chemical gradient described earlier, from the reference site toward the most enclosed and contaminated 6ext site (Figure 6). Compared to the uncontaminated site (41p), used here as a reference, some KEGG pathways seemed overrepresented in contaminated sites: Carbohydrate metabolism, Xenobiotics biodegradation and metabolism, Metabolism of terpenoids, and polyketides. Other KEGG pathways were found to be underrepresented as “Cell growth and death,” “Nucleotide metabolism,” “Energy metabolism,” and “Metabolism of cofactors and vitamins.”
Figure 6. Heatmap based on the main KEGG pathways predicted at the different sampling sites. Frequencies of predicted KEGG categories were normalized with reference to the uncontaminated site 41p. Enrichments correspond to the positive values and red colors, depletions correspond to negative values and blue colors.
Bacterioplankton community composition and associated functions in highly metal contaminated ecosystems were scarcely studied (Nayar et al., 2004; Boyd et al., 2005; Pringault et al., 2016), even less using NGS techniques (Wang et al., 2015; Qian et al., 2017). This study was performed in a multi contaminated coastal area, Toulon Bay, which presents far wider contamination gradients than natural environmental gradients. By combining seawater physicochemical and geochemical analyses with patterns of bacterioplankton diversity and predicted functions, we provide an innovative insight into bacterioplankton ecology allowing to better understand how trace metals could shape communities.
Marine coastal areas are seen as valuable sentinel ecosystems, providing signals that reflect anthropogenic chemical pollution (The MerMex Group et al., 2011). Concentrations of trace metals in the Toulon Bay were found to be at least comparable or higher to those reported in aquatic ecosystems receiving high contamination loads (Boyd et al., 2005; Pringault et al., 2012). The high enrichment factors (6- to 234-fold) compared to the geochemical background of the Mediterranean Sea (Morley et al., 1997) indicated significant anthropogenic inputs that could be attributed to numerous recent (e.g., large boat traffic, harbor activities, antifouling coatings) (Turner, 2010) and historical events (2nd World War) (Tessier et al., 2011). Thus, we hypothesized a potential effect of trace metals on the bacterioplankton communities.
As previously reported in coastal waters (Nayar et al., 2004; Caroppo et al., 2006; Pringault et al., 2016), bacterioplankton was more abundant in the most contaminated zones (MIS and 6ext) than in the uncontaminated site 41p. Bacteria have been reported to have a wide range of metal detoxification or resistance mechanisms by sequestering, excluding or precipitating metals (Nies, 1999). The higher abundance in contaminated sites could therefore suggest that the community is well adapted. Moreover, bioavailability of trace metals could also contribute to explain the absence of bacterioplankton abundance decrease in the most contaminated sites in term of abundance. Trace metals bioavailability is strongly controlled by the presence of dissolved organic ligand, especially in marine waters (Louis et al., 2009; Bruland et al., 2013). We considered here DOC concentrations and the dissolved fraction of trace metals, which is closer to the bioavailable fraction than the total concentration (Paquin et al., 2002; Cindrić et al., 2017) and consequently allow a more reliable assessment of their roles. Nevertheless, we neither evaluated the speciation of the main trace metals nor DOC composition in this study. More work will be thus needed to state about the respective contributions of bioavailability modulation and community adaptation in the bacterial abundance increase in Toulon Bay.
While bacterioplankton diversity did not show any significant trend along the contamination gradient, the community richness (Chao1 index) was shown to decrease progressively from the uncontaminated site (41p) toward the most contaminated sites (MIS and 6ext). A similar pattern was observed in South-Eastern Australia in metal- and PAH-contaminated sediments (Sun et al., 2012) as well as in contaminated sediments in the Northern Adriatic Sea (Korlević et al., 2015). This may be interpreted in light of classis disturbance theory that predict that in highly disturbed ecosystems, richness would decrease and a few species would dominate (Odum, 1985; Allison and Martiny, 2008). As a matter of fact, sensitive species are expected to be lost whereas tolerant species benefit from emptying ecological niches (Atlas et al., 2015). Thus, the multi-contamination appeared to have disruptive effect on the alpha diversity of the bacterioplankton communities.
Gillan et al. (2005) demonstrated that alpha-diversity alone is a poor indicator of ecosystem stress in chronically polluted systems, as the proliferation of new tolerant species can lead to a recovery of the diversity. As in many coastal sites, microbial assemblages in our study were dominated by a few families Flavobacteriaceae, SAR11 Surface 1 clade, Rhodobacteraceae, SAR116 clade, Synechococcus Family I, and Microbacteriaceae. This is a core of generalist families in marine seawater and its members have been found abundant in numerous other marine coastal studies (Chow et al., 2013; Cram et al., 2015). Despite overall similarities, BCC was strongly affect by trace metal contamination, the five sampling sites clearly exhibited distinct microbial community’s structures, along low-to-high contamination continuum. This observation is also in agreement with the disturbance hypothesis, as discussed for alpha diversity, and tends to complement previous observations of the structural role of contaminants for bacterioplankton in other coastal areas (Pringault et al., 2016; Marisol et al., 2018; Wang et al., 2018).
The uncontaminated site (41p) was characterized by higher relative abundances of OM1 clade (Acidimicrobiia), SAR11, Rickettsiales, Rhizobiales, and Rhodobacteraceae (Alphaproteobacteria), Thiothrichaceae (Gammaproteobacteria), and Synechococcus (Cyanobacteria). Correspondingly, a number of previous works reported that Synechococcus were sensitive to high trace metal levels (Cassier-Chauvat and Chauvat, 2014; Coclet et al., 2018). Additionally, SAR11, Rickettsiales, Rhizobiales, and Synechococcus are well known to be characteristic of marine zones away from anthropogenic influence (Gilbert et al., 2009, 2012; Fuhrman et al., 2015).
The most contaminated sites (MIS and 6ext) were enriched in Candidatus Aquiluna (Actinobacteria), Formosa and Tenacibaculum (Flavobacteriia) as well as one from Sphingobacteriales NS11-12 marine group (Bacteroidetes). Networks analysis showed also that Planktomarina (Alphaproteobacteria), Hydrogenophilaceae (Betaproteobacteria) and Halieaceae (Gammaproteobacteria) were positively associated with contaminants, in agreement with the distribution of these groups along the contamination gradient. Previous studies in freshwater or marine sediment reported that Hydrogenophilaceae and Candidatus Aquiluna (Microbacteriaceae) were positively correlated to high trace contaminant levels (Acosta-González et al., 2013; Garris et al., 2018). The increase of Actinobacteria and Betaproteobacteria in the most contaminated site was not unexpected, as they are widely recognized to play a key role in oil degradation (Acosta-González et al., 2013) and heavy metals transformation, especially, in the case of nutrient enrichments (Garris et al., 2018). Microbacteriaceae (Actinobacteria) were widely reported as members of highly copper-polluted sediment (Besaury et al., 2014) or Zn-polluted freshwater (Ni et al., 2016). Albarracín et al. (2010) have shown that Actinobacteria members isolated from copper-contaminated sediment are able to significantly diminish the bioavailable copper throughout bioaccumulation. Members of the Bacteroidetes members, especially Flavobacteria families are also known to represent one of the most abundant groups of bacteria in coastal areas, especially in contaminated sites due to their ability to tolerate toxic effects of certain metals (Sun et al., 2013: Korlević et al., 2015). The Bacteroidetes NS11-12 marine group have been detected mainly in marine habitats (Meziti et al., 2015), unfortunately without clear ecological implications. At a finer scale, for most of clusters, members of both Alphaproteobacteria (mainly SAR11) and Bacteroidetes (mainly Flavobacteriia) groups describe above, exhibit the highest number of edges with high level of connections within each group in the network analysis. These important findings display that Alphaproteobacteria would highly influence the functioning of low contaminated site while Bacteroidetes would highly influence the functioning of contaminated zones.
Most of studies on bacterioplankton communities have focused on community diversity and composition responses to perturbation (Jeffries et al., 2016). The effect of chemical contaminations on bacterial community functionalities have only been investigated in freshwater ecosystems (Wang et al., 2018; Sjöstedt et al., 2018). In a highly polluted area such as Toulon Bay, the functional stability of microbial communities could be challenged. Major predicted bacterial functions including those necessary to basic metabolisms were conserved across the chemical gradient. Functional recovery occurred because of species replacement or change in relative abundance of taxa, i.e., functionally redundant rare species could become abundant in response to the contaminations. This is in agreement with the replacement scenario (Comte and Del Giorgio, 2011), and previous findings showing that the rare biosphere is an important reservoir for recruitment of bacterial taxa during environmental change (Sjöstedt et al., 2012; Comte et al., 2014). However, both a decrease in some critical functions (cell growth and death or energy metabolism) and an increase in functions associated to multiple stress resistance (e.g., xenobiotic biodegradation and metabolism, signaling molecules and interactions, secondary metabolites metabolism) remarkably exhibited that bacterial communities in the most polluted sites developed specific functions to face their environment. As the sites are not physically separated, there was no discontinuity in both communities and functions but a clear gradient of the relative components throughout the metal gradient. Metal contaminated environments were shown to exert a high selective pressure toward the transfer of several genes, developing thus resistance against high metal concentrations (Azarbad et al., 2016). Only two field studies on long-term metal polluted areas have shown shifts in microbial community structure and functions or increased occurrence of resistance genes that have made microbial communities resistant to toxic metals concentrations (Hemme et al., 2010; Kang et al., 2013). However, both taxonomic affiliation of metal-specific metabolic traits and the currently available KEGG database are insufficient to validate the hypothesis that heavy metal stress is a key environmental factor shaping the function of microbial communities.
Although trace metal gradient seemed to be the main driver of the bacterioplankton community features, other local selective pressures could explain a significant proportion of their variance. Indeed, bacterioplankton communities in coastal areas greatly vary in space because of sharp gradients in salinity, nutrients, depth, among other properties (Fuhrman et al., 2006; Gilbert et al., 2009; Fortunato et al., 2012).
A very similar trend could have been observed if DOM quantity or quality varied and became more labile in the most contaminated sites. Indeed, an increase in DOM lability often leads to the development of a copiotrophic response of the bacterial community, selecting a limited number of bacterial taxa, and temporarily reducing microbial diversity (Nelson and Wear, 2014; Pedler et al., 2014). The variation partitioning performed in our study is in agreement with this hypothesis, since DOC appeared as one of the most influent drivers of community structure variations. Thus, organic matter spatio-temporal dynamics could indeed be an important driver of microbial communities in Toulon Bay. However, according to the literature, only a part of the bacterial taxa significantly enriched in the most contaminated sites of Toulon Bay is known as copiotroph. Indeed, while OM60 (NOR5) clade (Halieaceae), Candidatus Aquiluna (Microbacteriaceae), and Planktomarina (Rhodobacterales) are known to benefit from labile DOM (Yan et al., 2009; Spring et al., 2015), Alphaproteobacteria members (SAR11 and AEGEAN-169 marine group) and Bacteroidetes (Balneola and Aquibacter) are rather known as oligotrophic groups (Cram et al., 2015; Sipler et al., 2017). Copiotrophs are expected to outcompete oligotrophs, so the fact that presence of groups identified as copiotrophs suggest that each has capitalized on reduced competition following trace metal contamination. Taken together, all these arguments point out the major and predominant influence of the multi-contamination gradient on microbial diversity and represent a significant demonstration of the potential influence of human activities on marine microbial life. Since we only considered inorganic contaminants, we cannot rule out the potential influence of organic contaminants such as PAH, PCBS, or organometals which were already measured in the sediment of Toulon Bay (Misson et al., 2016).
Organic contaminants as polyaromatic hydrocarbons could explained more precisely the distribution of OTUs (Qian et al., 2017). This hypothesis could be confirmed by the strong enrichment in xenobiotics biodegradation and metabolism, carbohydrate metabolism and metabolism of terpenoids and polyketides in the highest contaminated sites. Naphthalene degradation, benzoate, and aminobenzoate biodegradation were found to be higher in the most contaminated zones and is consistent with the finding of previous studies (Wang et al., 2018).
Finally, another striking result is that the multi-contamination gradient in the water column of Toulon Bay represented a significant perturbation, affecting microbial diversity all along our survey. This observation differs from what was observed previously in surface sediment of Toulon Bay (Misson et al., 2016), suggesting that the contemporary contamination in the water affects more strongly microbial communities that the large historical contamination trapped in the sediment. Such difference could be linked to the probably higher dispersal rate in the water column than in the sediment, i.e., 3.4 days for a total renewal of the water of Toulon Bay (Dufresne et al., 2014). Thus, this higher dispersal rate could tend to reduce the adaptive abilities of bacterioplankton, leading to a higher sensitivity to contamination when compared to the longer-term stability of the sediment.
The network indices (BC and CC) and large numbers of nodes (179) and edges (1664) between taxa in our network analysis suggest that SAR11, Rhodobacteraceae, Synechococcus, and Microbacteriaceae play a key role in Toulon Bay seawater. Despite some connections between OTUs and trace metals, there were far more numerous connections among OTUs. This suggests that trace metals significantly influence the dynamics of only a few microbial groups, and could rather influence indirectly, via biotic interactions, the whole community in Toulon Bay seawater. Community structure is known to be influenced by interaction among species (Lima-Mendez et al., 2015). Some of these interactions could be cooperative interspecific interactions (Eiler et al., 2012; Chow et al., 2013) or competitive interactions (i.e., competition, niche partitioning, grazing, or parasitism) (Fuhrman et al., 2008; Berdjeb et al., 2018) in a multispecies community.
Keystone OTUs play important roles in the regulation of network interactions (Wright et al., 2012) and their loss may increase a community’s vulnerability to perturbation. Thus, the strong decline of Alphaproteobacterial and Cyanobacterial keystone OTUs, especially, along the trace metal contamination gradient could have indirect effect on the BCC by modifying biotic interactions and favor opportunist or tolerant taxa (Lawes et al., 2016). This is in agreement with community turnover and the development of copiotrophic groups highlighted above.
Additionally, in network analysis, positive correlation between on the one hand pico- and nanophytoplankton abundances, as well as chlorophyll a, and on the other hand trace metals concentrations describe potential conditions that may favor specific groups of bacterioplankton. Indeed, Fuhrman et al. (2015) explained that weekly timescale is appropriate for studying bacterial dynamics associated with phytoplankton, which have a direct influence on the composition of bacterial communities via cross-feeding interactions or the effect of toxins, as well as indirect influence (e.g., oxygen depletion). Phytoplankton succession may influence the dynamics of the bacterioplankton community, as differences in the phytoplankton structure lead to differences in quantity and quality of exuded organic matter that can be used by the bacterial community for growth (Marisol et al., 2018). Consequently, effects of pollutants on phytoplankton or bacterioplankton might have indirect consequences for the counterpart, depending on the possible interactions between both compartments.
In summary, Toulon Bay offered a remarkable study area allowing to address trace metal multi-contamination impacts on prokaryotic community shaping throughout contrasted but connected sites. Where trace metal contamination increased, bacterioplankton abundance was higher together with a reduction of only community richness. Seawater contamination induced significant bacterioplankton communities shifts, with Alphaproteobacteria (SAR11, Rickettsiales, Rhizobiales, and Rhodobacteraceae) and Synechococcus dominance where trace metal concentrations were the lowest, whereas Flavobacteria, Betaproteobacteria (Hydrogenophilaceae) and Gammaproteobacteria (Halieaceae), especially including copiotrophic organisms, dominated in highly contaminated sites. In addition, this study showed that human-induced disturbances can have an effect on predicted functional potential of marine coastal bacterioplankton, and that the response can differ according to the type of functions. However, observed functional redundancy lead to think that the effect of trace metals could be complex, highly context-dependent and depending on biotic interactions. While our results provide a better understanding of anthropogenic influence on coastal ecosystems and the response of microbial communities, future studies are needed to elucidate the contribution of DOC, phytoplankton-bacteria specific interactions and organic pollutants on bacterioplankton communities in Toulon Bay.
Human-induced chemical contamination gradients challenge our understanding of microbial communities’ ecology, especially in the very dynamic marine coastal environment. Through the spatio-temporal investigation of microbial diversity dynamics in a model ecosystem, this work highlights contributions of anthropogenic trace metal contamination to marine bacterioplankton ecology. Identify the key aspects of originality and significance that place the work within the top 10% of current research in environmental microbiology.
CG, J-FB, and BM proposed and designed the study. CC, CG, GD, CLP, J-UM, J-FB, and BM organized and performed field sampling. CC, CG, GD, DO, SD, CLP, and BM analyzed the samples. CC, CG, J-FB, and BM interpreted the results. All authors participated in manuscript redaction, thus approving the publication of the content.
This study was supported by PREVENT research program [funded by Toulon University, Toulon-Provence-Méditerranée (TPM) and the Conseil Départemental du Var]. CC was funded by the Provence-Alpes-Côte d’Azur region and supported by TPM.
The authors declare that the research was conducted in the absence of any commercial or financial relationships that could be construed as a potential conflict of interest.
The authors wish to thank the French Navy and the LASEM for diving and sampling assistance, Dr. G. Culioli (MAPIEM, Toulon, France) and L. Favre (MAPIEM, Toulon, France) for sampling and sample treatment assistance.
The Supplementary Material for this article can be found online at: https://www.frontiersin.org/articles/10.3389/fmicb.2019.00257/full#supplementary-material
Acosta-González, A., Rosselló-Móra, R., and Marqués, S. (2013). Characterization of the anaerobic microbial community in oil-polluted subtidal sediments: aromatic biodegradation potential after the Prestige oil spill. Environ. Microbiol. 15, 77–92. doi: 10.1111/j.1462-2920.2012.02782.x
Albarracín, V. H., Alonso-Vega, P., Trujillo, M. E., Amoroso, M. J., and Abate, C. M. (2010). Amycolatopsis tucumanensis sp. nov., a copper-resistant actinobacterium isolated from polluted sediments. Int. J. Syst. Evol. Microbiol. 60, 397–401. doi: 10.1099/ijs.0.010587-0
Allison, S. D., and Martiny, J. B. (2008). Resistance, resilience, and redundancy in microbial communities. Proc. Natl. Acad. Sci. U.S.A. 105(Suppl. 1), 11512–11519. doi: 10.1073/pnas.0801925105
Atlas, R. M., Stoeckel, D. M., Faith, S. A., Minard-Smith, A., Thorn, J. R., and Benotti, M. J. (2015). Oil biodegradation and oil-degrading microbial populations in marsh sediments impacted by oil from the deepwater horizon well blowout. Environ. Sci. Technol. 49, 8356–8366. doi: 10.1021/acs.est.5b00413
Azarbad, H., van Gestel, C. A., Niklińska, M., Laskowski, R., Röling, W. F., and van Straalen, N. M. (2016). Resilience of soil microbial communities to metals and additional stressors: DNA-based approaches for assessing “stress-on-stress” responses. Int. J. Mol. Sci. 17:933. doi: 10.3390/ijms17060933
Aßhauer, K. P., Wemheuer, B., Daniel, R., and Meinicke, P. (2015). Tax4Fun: predicting functional profiles from metagenomic 16S rRNA data. Bioinformatics 31, 2882–2884. doi: 10.1093/bioinformatics/btv287
Berdjeb, L., Parada, A., Needham, D. M., and Fuhrman, J. A. (2018). Short-term dynamics and interactions of marine protist communities during the spring–summer transition. ISME J. 12, 1907–1917. doi: 10.1038/s41396-018-0097-x
Besaury, L., Ghiglione, J. F., and Quillet, L. (2014). Abundance, activity, and diversity of archaeal and bacterial communities in both uncontaminated and highly copper-contaminated marine sediments. Mar. Biotechnol. 16, 230–242. doi: 10.1007/s10126-013-9542-z
Bokulich, N. A., Subramanian, S., Faith, J. J., Gevers, D., Gordon, J. I., and Knight, R. (2013). Quality-filtering vastly improves diversity estimates from Illumina amplicon sequencing. Nat. Methods 10, 57–59. doi: 10.1038/nmeth.2276
Boyd, T. J., Wolgast, D. M., Rivera-Duarte, I., Holm-Hansen, O., Hewes, C. D., Zirino, A., et al. (2005). Effects of dissolved and complexed copper on heterotrophic bacterial production in San Diego Bay. Microb. Ecol. 49, 353–366. doi: 10.1007/s00248-003-1065-0
Bruland, K. W., Middag, R., and Lohan, M. C. (2013). “Controls of trace metals in seawater,” in Treatise on Geochemistry, 2nd Edn, eds M. J. Mottl and H. Elderfield (Philadelphia, PA: Saunders), 19–51.
Cabrol, L., Quéméneur, M., and Misson, B. (2017). Inhibitory effects of sodium azide on microbial growth in experimental resuspension of marine sediment. J. Microbiol. Methods 133, 62–65. doi: 10.1016/j.mimet.2016.12.021
Callender, E. (2003). Heavy metals in the environment-historical trends. Treat. Geochem. 9:612. doi: 10.1016/B0-08-043751-6/09161-1
Caporaso, J. G., Kuczynski, J., Stombaugh, J., Bittinger, K., Bushman, F. D., and Costello, E. K. (2010). QIIME allows analysis of high-throughput community sequencing data. Nat. Methods 7, 335–336. doi: 10.1038/nmeth.f.303
Caroppo, C., Stabili, L., Aresta, M., Corinaldesi, C., and Danovaro, R. (2006). Impact of heavy metals and PCBs on marine picoplankton. Environ. Toxicol. 21, 541–551. doi: 10.1002/tox.20215
Cassier-Chauvat, C., and Chauvat, F. (2014). Responses to oxidative and heavy metal stresses in cyanobacteria: recent advances. Int. J. Mol. Sci. 16, 871–886. doi: 10.3390/ijms16010871
Chow, C. E. T., Sachdeva, R., Cram, J. A., Steele, J. A., Needham, D. M., and Patel, A. (2013). Temporal variability and coherence of euphotic zone bacterial communities over a decade in the Southern California Bight. ISME J. 7, 2259–2273. doi: 10.1038/ismej.2013.122
Cindrić, A. M., Cukrov, N., Durrieu, G., Garnier, C., Pižeta, I., and Omanović, D. (2017). Evaluation of discrete and passive sampling (Diffusive Gradients in Thin-films–DGT) approach for the assessment of trace metal dynamics in marine waters–a case study in a small harbor. Croat. Chem. Acta 90, 1–9. doi: 10.5562/cca3163
Clarke, K. R. (1993). Non-parametric multivariate analyses of changes in community structure. Aust. J. Ecol. 18, 117–143. doi: 10.1111/j.1442-9993.1993.tb00438.x
Cloern, J. E. (2001). Our evolving conceptual model of the coastal eutrophication problem. Mar. Ecol. Prog. Ser. 210, 223–253. doi: 10.3354/meps210223
Coclet, C., Garnier, C., Delpy, F., Jamet, D., Durrieu, G., and Le Poupon, C. (2018). Trace metal contamination as a toxic and structuring factor impacting ultraphytoplankton communities in a multicontaminated Mediterranean coastal area. Prog. Oceanogr. 163, 196–213. doi: 10.1016/j.pocean.2017.06.006
Comte, J., and Del Giorgio, P. A. (2011). Composition influences the pathway but not the outcome of the metabolic response of bacterioplankton to resource shifts. PLoS One 6:e25266. doi: 10.1371/journal.pone.0025266
Comte, J., Lindström, E. S., Eiler, A., and Langenheder, S. (2014). Can marine bacteria be recruited from freshwater sources and the air? ISME J. 8, 2423–2430. doi: 10.1038/ismej.2014.89
Cram, J. A., Chow, C. E. T., Sachdeva, R., Needham, D. M., Parada, A. E., Steele, J. A., et al. (2015). Seasonal and interannual variability of the marine bacterioplankton community throughout the water column over ten years. ISME J. 9, 563–580. doi: 10.1038/ismej.2014.153
Dang, D. H., Lenoble, V., Durrieu, G., Omanović, D., Mullot, J. U., Mounier, S., et al. (2015). Seasonal variations of coastal sedimentary trace metals cycling: insight on the effect of manganese and iron (oxy) hydroxides, sulphide and organic matter. Mar. Pollut. Bull. 92, 113–124. doi: 10.1016/j.marpolbul.2014.12.048
Dinsdale, E. A., Edwards, R. A., Hall, D., Angly, F., Breitbart, M., Brulc, J. M., et al. (2008). Functional metagenomic profiling of nine biomes. Nature 452, 629–632. doi: 10.1038/nature06810
Dufresne, C., Duffa, C., and Rey, V. (2014). Wind-forced circulation model and water exchanges through the channel in the Bay of Toulon. Ocean Dyn. 64, 209–224. doi: 10.1007/s10236-013-0676-3
Edgar, R. C. (2010). Search and clustering orders of magnitude faster than BLAST. Bioinformatics 26, 2460–2461. doi: 10.1093/bioinformatics/btq461
Eiler, A., Heinrich, F., and Bertilsson, S. (2012). Coherent dynamics and association networks among lake bacterioplankton taxa. ISME J. 6, 330–332. doi: 10.1038/ismej.2011.113
Faust, K., Sathirapongsasuti, J. F., Izard, J., Segata, N., Gevers, D., Raes, J., et al. (2012). Microbial co-occurrence relationships in the human microbiome. PLoS Comput. Biol. 8:e1002606. doi: 10.1371/journal.pcbi.1002606
Fortunato, C. S., Herfort, L., Zuber, P., Baptista, A. M., and Crump, B. C. (2012). Spatial variability overwhelms seasonal patterns in bacterioplankton communities across a river to ocean gradient. ISME J. 6, 554–563. doi: 10.1038/ismej.2011.135
Franklin, N. M., Stauber, J. L., Lim, R. P., and Petocz, P. (2002). Toxicity of metal mixtures to a tropical freshwater alga (Chlorella sp.): the effect of interactions between copper, cadmium, and zinc on metal cell binding and uptake. Environ. Toxicol. Chem. 21, 2412–2422. doi: 10.1038/ismej.2011.135
Fuhrman, J. A., and Azam, F. (1980). Bacterioplankton secondary production estimates for coastal waters of British Columbia, Antarctica, and California. Appl. Environ. Microbiol. 39, 1085–1095. doi: 10.1002/etc.5620211121
Fuhrman, J. A., Cram, J. A., and Needham, D. M. (2015). Marine microbial community dynamics and their ecological interpretation. Nat. Rev. Microbiol. 13, 133–146. doi: 10.1038/nrmicro3417
Fuhrman, J. A., Hewson, I., Schwalbach, M. S., Steele, J. A., Brown, M. V., and Naeem, S. (2006). Annually reoccurring bacterial communities are predictable from ocean conditions. Proc. Natl. Acad. Sci. U.S.A. 103, 13104–13109. doi: 10.1073/pnas.0602399103
Fuhrman, J. A., Steele, J. A., Hewson, I., Schwalbach, M. S., Brown, M. V., Green, J. L., et al. (2008). A latitudinal diversity gradient in planktonic marine bacteria. Proc. Natl. Acad. Sci. U.S.A. 105, 7774–7778. doi: 10.1073/pnas.0803070105
Garris, H. W., Baldwin, S. A., Taylor, J., Gurr, D. B., Denesiuk, D. R., Van Hamme, J. D., et al. (2018). Short-term microbial effects of a large-scale mine-tailing storage facility collapse on the local natural environment. PLoS One 13:e0196032. doi: 10.1371/journal.pone.0196032
Ghiglione, J. F., Conan, P., and Pujo-Pay, M. (2009). Diversity of total and active free-living vs. particle-attached bacteria in the euphotic zone of the NW Mediterranean Sea. FEMS Microbiol. Lett. 299, 9–21. doi: 10.1111/j.1574-6968.2009.01694.x
Ghiglione, J. F., Galand, P. E., Pommier, T., Pedrós-Alió, C., Maas, E. W., and Bakker, K. (2012). Pole-to-pole biogeography of surface and deep marine bacterial communities. Proc. Natl. Acad. Sci. U.S.A. 109, 17633–17638. doi: 10.1073/pnas.1208160109
Gilbert, J. A., Field, D., Swift, P., Newbold, L., Oliver, A., and Smyth, T. (2009). The seasonal structure of microbial communities in the Western English Channel. Environ. Microbiol. 11, 3132–3139. doi: 10.1111/j.1462-2920.2009.02017.x
Gilbert, J. A., Steele, J. A., Caporaso, J. G., Steinbrück, L., Reeder, J., and Temperton, B. (2012). Defining seasonal marine microbial community dynamics. ISME J. 6, 298–308. doi: 10.1038/ismej.2011.107
Gillan, D. C., Danis, B., Pernet, P., Joly, G., and Dubois, P. (2005). Structure of sediment-associated microbial communities along a heavy-metal contamination gradient in the marine environment. Appl. Environ. Microbiol. 71, 679–690. doi: 10.1128/AEM.71.2.679-690.2005
Goecks, J., Nekrutenko, A., and Taylor, J. (2010). Galaxy: a comprehensive approach for supporting accessible, reproducible, and transparent computational research in the life sciences. Genome Biol. 11:R86. doi: 10.1186/gb-2010-11-8-r86
Gough, H. L., and Stahl, D. A. (2011). Microbial community structures in anoxic freshwater lake sediment along a metal contamination gradient. ISME J. 5, 543–558. doi: 10.1038/ismej.2010.132
Halpern, B. S., Walbridge, S., Selkoe, K. A., Kappel, C. V., Micheli, F., and D’agrosa, C. (2008). A global map of human impact on marine ecosystems. Science 319, 948–952. doi: 10.1126/science.1149345
Hemme, C. L., Deng, Y., Gentry, T. J., Fields, M. W., Wu, L., and Barua, S. (2010). Metagenomic insights into evolution of a heavy metal-contaminated groundwater microbial community. ISME J. 4, 660–672. doi: 10.1038/ismej.2009.154
Jeanbille, M., Gury, J., Duran, R., Tronczynski, J., Ghiglione, J. F., and Agogué, H. (2016). Chronic polyaromatic hydrocarbon (PAH) contamination is a marginal driver for community diversity and prokaryotic predicted functioning in coastal sediments. Front. Microbiol. 7:1303. doi: 10.3389/fmicb.2016.01303
Jeffries, T. C., Schmitz Fontes, M. L., Harrison, D. P., Van-Dongen-Vogels, V., Eyre, B. D., Ralph, P. J., et al. (2016). Bacterioplankton dynamics within a large anthropogenically impacted urban estuary. Front. Microbiol. 6:1438. doi: 10.3389/fmicb.2015.01438
Kang, S., Van Nostrand, J. D., Gough, H. L., He, Z., Hazen, T. C., Stahl, D. A., et al. (2013). Functional gene array–based analysis of microbial communities in heavy metals-contaminated lake sediments. FEMS Microbiol. Ecol. 86, 200–214. doi: 10.1111/1574-6941.12152
Korlević, M., Zucko, J., Dragić, M. N., Blažina, M., Pustijanac, E., and Zeljko, T. V. (2015). Bacterial diversity of polluted surface sediments in the northern Adriatic Sea. Syst. Appl. Microbiol. 38, 189–197. doi: 10.1016/j.syapm.2015.03.001
Lawes, J. C., Neilan, B. A., Brown, M. V., Clark, G. F., and Johnston, E. L. (2016). Elevated nutrients change bacterial community composition and connectivity: high throughput sequencing of young marine biofilms. Biofouling 32, 57–69. doi: 10.1080/08927014.2015.1126581
LeBrun, E. S., King, R. S., Back, J. A., and Kang, S. (2018). Microbial community structure and function decoupling across a phosphorus gradient in streams. Microb. Ecol. 75, 64–73. doi: 10.1007/s00248-017-1039-2
Levin, L. A., Boesch, D. F., Covich, A., Dahm, C., Erséus, C., and Ewel, K. C. (2001). The function of marine critical transition zones and the importance of sediment biodiversity. Ecosystems 4, 430–451. doi: 10.1007/s10021-001-0021-4
Lima-Mendez, G., Faust, K., Henry, N., Decelle, J., Colin, S., and Carcillo, F. (2015). Determinants of community structure in the global plankton interactome. Science 348:1262073. doi: 10.1126/science.1262073
Lorenzo, J. I., Nieto, O., and Beiras, R. (2002). Effect of humic acids on speciation and toxicity of copper to Paracentrotus lividus larvae in seawater. Aquat. Toxicol. 58, 27–41. doi: 10.1016/S0166-445X(01)00219-3
Louca, S., Parfrey, L. W., and Doebeli, M. (2016). Decoupling function and taxonomy in the global ocean microbiome. Science 353, 1272–1277. doi: 10.1126/science.aaf4507
Louis, Y., Garnier, C., Lenoble, V., Omanović, D., Mounier, S., and Pižeta, I. (2009). Characterisation and modelling of marine dissolved organic matter interactions with major and trace cations. Mar. Environ. Res. 67, 100–107. doi: 10.1016/j.marenvres.2008.12.002
Lozupone, C. A., and Knight, R. (2007). Global patterns in bacterial diversity. Proc. Natl. Acad. Sci. U.S.A. 104, 11436–11440. doi: 10.1073/pnas.0611525104
Marisol, G.-U., Hélène, M., Céline, L., Claire, C., Marc, B., Asma, S. H., et al. (2018). Consequences of contamination on the interactions between phytoplankton and bacterioplankton. Chemosphere 195, 212–222. doi: 10.1016/j.chemosphere.2017.12.053
Meziti, A., Kormas, K. A., Moustaka-Gouni, M., and Karayanni, H. (2015). Spatially uniform but temporally variable bacterioplankton in a semi-enclosed coastal area. Syst. Appl. Microbiol. 38, 358–367. doi: 10.1016/j.syapm.2015.04.003
Misson, B., Garnier, C., Lauga, B., Dang, D. H., Ghiglione, J. F., and Mullot, J. U. (2016). Chemical multi-contamination drives benthic prokaryotic diversity in the anthropized Toulon Bay. Sci. Total Environ. 556, 319–329. doi: 10.1016/j.scitotenv.2016.02.038
Morley, N. H., Burton, J. D., Tankere, S. P. C., and Martin, J. M. (1997). Distribution and behaviour of some dissolved trace metals in the western Mediterranean Sea. Deep Sea Res. Part II Top. Stud. Oceanogr. 44, 675–691. doi: 10.1016/S0967-0645(96)00098-7
Nayar, S., Goh, B. P. L., and Chou, L. M. (2004). Environmental impact of heavy metals from dredged and resuspended sediments on phytoplankton and bacteria assessed in in situ mesocosms. Ecotoxicol. Environ. Saf. 59, 349–369. doi: 10.1016/j.ecoenv.2003.08.015
Nelson, C. E., and Wear, E. K. (2014). Microbial diversity and the lability of dissolved organic carbon. Proc. Natl. Acad. Sci. U.S.A. 111, 7166–7167. doi: 10.1073/pnas.1405751111
Ni, C., Horton, D. J., Rui, J., Henson, M. W., Jiang, Y., Huang, X., et al. (2016). High concentrations of bioavailable heavy metals impact freshwater sediment microbial communities. Ann. Microbiol. 66, 1003–1012. doi: 10.1007/s13213-015-1189-8
Nies, D. H. (1999). Microbial heavy-metal resistance. Appl. Microbiol. Biotechnol. 51, 451–460. doi: 10.1007/s002530051457
Odum, E. P. (1985). Trends expected in stressed ecosystems. Bioscience 35, 419–422. doi: 10.1098/rstb.2010.0171
Oksanen, J., Blanchet, F., Kindt, R., Legendre, P., and O’Hara, R. (2016). Vegan: Community Ecology Package. R package 2.3–3.
Othman, H. B., Pringault, O., Louati, H., Hlaili, A. S., and Leboulanger, C. (2017). Impact of contaminated sediment elutriate on coastal phytoplankton community (Thau lagoon, Mediterranean Sea, France). J. Exp. Mar. Biol. Ecol. 486, 1–12. doi: 10.1016/j.jembe.2016.09.006
Oursel, B., Garnier, C., Durrieu, G., Mounier, S., Omanović, D., and Lucas, Y. (2013). Dynamics and fates of trace metals chronically input in a Mediterranean coastal zone impacted by a large urban area. Mar. Pollut. Bull. 69, 137–149. doi: 10.1016/j.marpolbul.2013.01.023
Paquin, P. R., Gorsuch, J. W., Apte, S., Batley, G. E., Bowles, K. C., and Campbell, P. G. (2002). The biotic ligand model: a historical overview. Comp. Biochem. Physiol. C Toxicol. Pharmacol. 133, 3–35.
Parada, A. E., Needham, D. M., and Fuhrman, J. A. (2016). Every base matters: assessing small subunit rRNA primers for marine microbiomes with mock communities, time series and global field samples. Environ. Microbiol. 18, 1403–1414. doi: 10.1111/1462-2920.13023
Pedler, B. E., Aluwihare, L. I., and Azam, F. (2014). Single bacterial strain capable of significant contribution to carbon cycling in the surface ocean. Proc. Natl. Acad. Sci. U.S.A. 111, 7202–7207. doi: 10.1073/pnas.1401887111
Pommier, T., Canbäck, B., Riemann, L., Boström, K. H., Simu, K., and Lundberg, P. (2007). Global patterns of diversity and community structure in marine bacterioplankton. Mol. Ecol. 16, 867–880. doi: 10.1111/j.1365-294X.2006.03189.x
Pringault, O., Lafabrie, C., Avezac, M., Bancon-Montigny, C., Carre, C., and Chalghaf, M. (2016). Consequences of contaminant mixture on the dynamics and functional diversity of bacterioplankton in a southwestern Mediterranean coastal ecosystem. Chemosphere 144, 1060–1073. doi: 10.1016/j.chemosphere.2015.09.093
Pringault, O., Viret, H., and Duran, R. (2012). Interactions between Zn and bacteria in marine tropical coastal sediments. Environ. Sci. Pollut. Res. 19, 879–892. doi: 10.1007/s11356-011-0621-2
Pruesse, E., Quast, C., Knittel, K., Fuchs, B. M., Ludwig, W., Peplies, J., et al. (2007). SILVA: a comprehensive online resource for quality checked and aligned ribosomal RNA sequence data compatible with ARB. Nucleic Acids Res. 35, 7188–7196. doi: 10.1093/nar/gkm864
Qian, J., Ding, Q., Guo, A., Zhang, D., and Wang, K. (2017). Alteration in successional trajectories of bacterioplankton communities in response to co-exposure of cadmium and phenanthrene in coastal water microcosms. Environ. Pollut. 221, 480–490. doi: 10.1016/j.envpol.2016.12.020
Quast, C., Pruesse, E., Yilmaz, P., Gerken, J., Schweer, T., and Yarza, P. (2013). The SILVA ribosomal RNA gene database project: improved data processing and web-based tools. Nucleic Acids Res. 41, D590–D596. doi: 10.1093/nar/gks1219
Quero, G. M., Cassin, D., Botter, M., Perini, L., and Luna, G. M. (2015). Patterns of benthic bacterial diversity in coastal areas contaminated by heavy metals, polycyclic aromatic hydrocarbons (PAHs) and polychlorinated biphenyls (PCBs). Front. Microbiol. 6:1053. doi: 10.3389/fmicb.2015.01053
R Core Team (2015). R: A Language and Environment for Statistical Computing. Vienna: R Foundation for Statistical Computing.
Segata, N., Izard, J., Waldron, L., Gevers, D., Miropolsky, L., Garrett, W. S., et al. (2011). Metagenomic biomarker discovery and explanation. Genome Biol. 12:R60. doi: 10.1186/gb-2011-12-6-r60
Sipler, R. E., Kellogg, C. T., Connelly, T. L., Roberts, Q. N., Yager, P. L., and Bronk, D. A. (2017). Microbial community response to terrestrially derived dissolved organic matter in the coastal arctic. Front. Microbiol. 8:1018. doi: 10.3389/fmicb.2017.01018
Sjöstedt, J., Koch-Schmidt, P., Pontarp, M., Canbäck, B., Tunlid, A., and Lundberg, P. (2012). Recruitment of members from the rare biosphere of marine bacterioplankton communities after an environmental disturbance. Appl. Environ. Microbiol. 78, 1361–1369. doi: 10.1128/AEM.05542-11
Sjöstedt, J., Langenheder, S., Kritzberg, E., Karlsson, C. M., and Lindström, E. S. (2018). Repeated disturbances affect functional but not compositional resistance and resilience in an aquatic bacterioplankton community. Environ. Microbiol. Rep. 10, 493–500. doi: 10.1111/1758-2229.12656
Smoot, M. E., Ono, K., Ruscheinski, J., Wang, P. L., and Ideker, T. (2011). Cytoscape 2.8: new features for data integration and network visualization. Bioinformatics 27, 431–432. doi: 10.1093/bioinformatics/btq675
Spring, S., Scheuner, C., Göker, M., and Klenk, H. P. (2015). A taxonomic framework for emerging groups of ecologically important marine gammaproteobacteria based on the reconstruction of evolutionary relationships using genome-scale data. Front. Microbiol. 6:281. doi: 10.3389/fmicb.2015.00281
Sun, M. Y., Dafforn, K. A., Brown, M. V., and Johnston, E. L. (2012). Bacterial communities are sensitive indicators of contaminant stress. Mar. Pollut. Bull. 64, 1029–1038. doi: 10.1016/j.marpolbul.2012.01.035
Sun, M. Y., Dafforn, K. A., Johnston, E. L., and Brown, M. V. (2013). Core sediment bacteria drive community response to anthropogenic contamination over multiple environmental gradients. Environ. Microbiol. 15, 2517–2531. doi: 10.1111/1462-2920.12133
Tessier, E., Garnier, C., Mullot, J. U., Lenoble, V., Arnaud, M., Raynaud, M., et al. (2011). Study of the spatial and historical distribution of sediment inorganic contamination in the Toulon bay (France). Mar. Pollut. Bull. 62, 2075–2086. doi: 10.1016/j.marpolbul.2011.07.022
The MerMex Group, Durrieu de Madron, X., Guieu, C., Sempéré, R., Conan, P., Cossa Fabrizio, D., et al. (2011). Marine ecosystems’ responses to climatic and anthropogenic forcings in the Mediterranean. Prog. Oceanogr. 91, 97–166. doi: 10.1016/j.pocean.2011.02.003
Thompson, L. R., Williams, G. J., Haroon, M. F., Shibl, A., Larsen, P., and Shorenstein, J. (2017). Metagenomic covariation along densely sampled environmental gradients in the Red Sea. ISME J. 11, 138–151. doi: 10.1038/ismej.2016.99
Treusch, A. H., Vergin, K. L., Finlay, L. A., Donatz, M. G., Burton, R. M., Carlson, C. A., et al. (2009). Seasonality and vertical structure of microbial communities in an ocean gyre. ISME J. 3, 1148–1163. doi: 10.1038/ismej.2009.60
Turner, A. (2010). Marine pollution from antifouling paint particles. Mar. Pollut. Bull. 60, 159–171. doi: 10.1016/j.marpolbul.2009.12.004
Wang, K., Ye, X., Zhang, H., Chen, H., Zhang, D., and Liu, L. (2016). Regional variations in the diversity and predicted metabolic potential of benthic prokaryotes in coastal northern Zhejiang, East China Sea. Sci. Rep. 6:38709. doi: 10.1038/srep38709
Wang, K., Zhang, D., Xiong, J., Chen, X., Zheng, J., and Hu, C. (2015). Response of bacterioplankton communities to cadmium exposure in coastal water microcosms with high temporal variability. Appl. Environ. Microbiol. 81, 231–240. doi: 10.1128/AEM.02562-14
Wang, L., Zhang, J., Li, H., Yang, H., Peng, C., Peng, Z., et al. (2018). Shift in the microbial community composition of surface water and sediment along an urban river. Sci. Total Environ. 627, 600–612. doi: 10.1016/j.scitotenv.2018.01.203
Ward, C. S., Yung, C. M., Davis, K. M., Blinebry, S. K., Williams, T. C., Johnson, Z. I., et al. (2017). Annual community patterns are driven by seasonal switching between closely related marine bacteria. ISME J. 11, 1412–1422. doi: 10.1038/ismej.2017.4
Wright, J. J., Konwar, K. M., and Hallam, S. J. (2012). Microbial ecology of expanding oxygen minimum zones. Nat. Rev. Microbiol. 10, 381–394. doi: 10.1038/nrmicro2778
Xu, Y., Sun, Q., Yi, L., Yin, X., Wang, A., Li, Y., et al. (2014). The source of natural and anthropogenic heavy metals in the sediments of the Minjiang River Estuary (SE China): implications for historical pollution. Sci. Total Environ. 493, 729–736. doi: 10.1016/j.scitotenv.2014.06.046
Yan, S., Fuchs, B. M., Lenk, S., Harder, J., Wulf, J., Jiao, N. Z., et al. (2009). Biogeography and phylogeny of the NOR5/OM60 clade of Gammaproteobacteria. Syst. Appl. Microbiol. 32, 124–139. doi: 10.1016/j.syapm.2008.12.001
Zhang, J., Kobert, K., Flouri, T., and Stamatakis, A. (2014). PEAR: a fast and accurate Illumina Paired-End reAd mergeR. Bioinformatics 30, 614–620. doi: 10.1093/bioinformatics/btt593
Keywords: coastal ecosystem, metal contamination gradients, bacterioplankton community structure, functional prediction, co-occurrence network
Citation: Coclet C, Garnier C, Durrieu G, Omanović D, D’Onofrio S, Le Poupon C, Mullot J-U, Briand J-F and Misson B (2019) Changes in Bacterioplankton Communities Resulting From Direct and Indirect Interactions With Trace Metal Gradients in an Urbanized Marine Coastal Area. Front. Microbiol. 10:257. doi: 10.3389/fmicb.2019.00257
Received: 04 September 2018; Accepted: 31 January 2019;
Published: 22 February 2019.
Edited by:
Stéphane Pesce, National Research Institute of Science and Technology for Environment and Agriculture (IRSTEA), FranceReviewed by:
Yonghong Bi, Institute of Hydrobiology (CAS), ChinaCopyright © 2019 Coclet, Garnier, Durrieu, Omanović, D’Onofrio, Le Poupon, Mullot, Briand and Misson. This is an open-access article distributed under the terms of the Creative Commons Attribution License (CC BY). The use, distribution or reproduction in other forums is permitted, provided the original author(s) and the copyright owner(s) are credited and that the original publication in this journal is cited, in accordance with accepted academic practice. No use, distribution or reproduction is permitted which does not comply with these terms.
*Correspondence: Benjamin Misson, bWlzc29uQHVuaXYtdGxuLmZy
Disclaimer: All claims expressed in this article are solely those of the authors and do not necessarily represent those of their affiliated organizations, or those of the publisher, the editors and the reviewers. Any product that may be evaluated in this article or claim that may be made by its manufacturer is not guaranteed or endorsed by the publisher.
Research integrity at Frontiers
Learn more about the work of our research integrity team to safeguard the quality of each article we publish.