- 1Instituto de Patología Experimental, CONICET-UNSa, Salta, Argentina
- 2Department of Biochemistry and Molecular Biology, University of Texas Medical Branch (UTMB), Galveston, TX, United States
- 3Institute for Human Infections and Immunity, University of Texas Medical Branch (UTMB), Galveston, TX, United States
- 4Department of Preventive Medicine and Community Health, University of Texas Medical Branch (UTMB), Galveston, TX, United States
- 5Department of Pathology, University of Texas Medical Branch (UTMB), Galveston, TX, United States
- 6Servicio de Cardiología, Hospital San Bernardo, Salta, Argentina
- 7Servicio de Cardiología, Programa de Medicina Interna, Hospital Papa Francisco, Salta, Argentina
- 8Department of Microbiology and Immunology, University of Texas Medical Branch (UTMB), Galveston, TX, United States
Trypanosoma cruzi (Tc) infection causes Chagas disease (ChD) presented by dilated cardiomyopathy and heart failure. During infection, oxidative and nitrosative stresses are elicited by the immune cells for control the pathogen; however, excess nitric oxide and superoxide production can result in cysteine S-nitrosylation (SNO) of host proteins that affects cellular homeostasis and may contribute to disease development. To identify the proteins with changes in SNO modification levels as a hallmark of ChD, we obtained peripheral blood mononuclear cells (PBMC) from seronegative, normal healthy (NH, n = 30) subjects, and from seropositive clinically asymptomatic (ChD CA, n = 25) or clinically symptomatic (ChD CS, n = 28) ChD patients. All samples were treated (Asc+) or not-treated (Asc−) with ascorbate (reduces nitrosylated thiols), labeled with the thiol-labeling BODIPY FL-maleimide dye, resolved by two-dimensional electrophoresis (total 166 gels), and the protein spots that yielded significant differences in abundance or SNO level at p-value of ≤ 0.05t−test/Welch/BH were identified by MALDI-TOF/TOF MS or OrbiTrap LC-MS/MS. Targeted analysis of a new cohort of PBMC samples (n = 10–14/group) was conducted to verify the differential abundance/SNO levels of two of the proteins in ChD (vs. NH) subjects. The multivariate adaptive regression splines (MARS) modeling, comparing differences in relative SNO level (Asc−/Asc+ ratio) of the protein spots between any two groups yielded SNO biomarkers that exhibited ≥90% prediction success in classifying ChD CA (582-KRT1 and 884-TPM3) and ChD CS (426-PNP, 582-KRT1, 486-ALB, 662-ACTB) patients from NH controls. Ingenuity Pathway Analysis (IPA) of the SNO proteome dataset normalized to changes in protein abundance suggested the proteins belonging to the signaling networks of cell death and the recruitment and migration of immune cells were most affected in ChD CA and ChD CS (vs. NH) subjects. We propose that SNO modification of the select panel of proteins identified in this study have the potential to identify ChD severity in seropositive individuals exposed to Tc infection.
Introduction
Chagas disease (ChD), a neglected parasitic disease recognized as one of the top public health concern in the world, is endemic in Latin America and Mexico (Bonney, 2014). World Health Organization estimates suggest that ~6–7 million people worldwide are infected with Trypanosoma cruzi (T. cruzi or Tc) (World Health Organization, 2010). With an increase in migratory movements, additional millions of people are at risk of infection not only in Latin America, Mexico, and southern regions of the United States, but also in many countries of Europe where three million of migrants from American endemic areas are living (Bern et al., 2011; Tanowitz et al., 2016a; Monge-Maillo and Lopez-Velez, 2017). The presentation of high parasitemia and general febrile illness during acute phase can result in myocarditis related death in ~5% of the infected individuals. An asymptomatic phase occurs indeterminately, although ~30% of the infected people could develop chronic Chagas disease (ChD) that results in cardiomyopathy and heart failure (Machado et al., 2012; Bonney et al., 2019). Some patients also exhibit megacolon and digestive disorders that affect the quality of life. Currently, T. cruzi infection is treated with benznidazole or nifurtimox. These drugs are effective in children presenting the acute infection phase (Perez-Molina and Molina, 2018), but exhibit limited efficacy and high toxicity in infected adults that are at risk of developing heart failure (Viotti et al., 2014). The current methods of detecting infection is by microscopic examination of blood smears, serology, or PCR (Ribeiro et al., 2012), but no methods currently exist to track or predict ChD progression (Balouz et al., 2017).
T. cruzi activates the production of oxidants (e.g., superoxide, nitric oxide) from enzymatic and mitochondrial sources in immune and non-immune cells (Lopez et al., 2018). While the immune activation of reactive oxygen species (ROS) and nitric oxide are important for controlling T. cruzi, non-resolution of these responses can damage the host (Tanowitz et al., 2016b; Lopez et al., 2018). Indeed, we have reported the markers of chronic oxidative and nitrosative stress were increased in both, the heart and peripheral blood, tissues of the T. cruzi-infected rodents and humans during the development and progression of ChD (Wen et al., 2006; Wan et al., 2016). Nevertheless, the host proteins that are susceptible to oxidative/nitrosative stress-mediated changes in structure, function, or stability during ChD progression are not known.
The protein S-nitrosylation (SNO) is an ubiquitous, redox reversible, post-translational modification of cysteine residues that occurs in presence of excessive oxidative and nitrosative stress (Htet Hlaing and Clement, 2014). This modification is shown to influence the translocation, localization, and stability of proteins, and thus can drive the gain as well as loss of function in biological and disease conditions (Moldogazieva et al., 2018).
We previously reported that peripheral blood mononuclear cells (PBMC) carry differential protein abundance signatures of ChD (Garg et al., 2016) and idiopathic heart failure (Koo et al., 2016). Protein profiling of ChD patients' PBMC suggested that proteins related to immune cell migration and free radical synthesis and catabolism were differentially abundant by disease state (Garg et al., 2016). Further, a select number of differentially abundant proteins had the predictive value in distinguishing ChD patients vs. normal healthy contols. Such protein signatures of easily accessible cells may have potential to serve as biomarkers of ChD severity and the patients' response to treatment, to be verified in future studies.
In this study, we report the cysteine SNO fingerprint associated with Chagas disease development. For this, enrolled volunteers were evaluated by cardiologists and grouped according to the severity of their cardiac anomalies following the modified Kuschnir classification criteria based on physical exam, electrocardiography, and transthoracic echocardiography (Sanchez-Montalva et al., 2016). The PBMC samples of ChD patients and normal healthy volunteers were treated (or not treated) with ascorbate, and labeled with thiol-labeling BODIPY® FL N- (2-aminoethyl) maleimide (BD) dye. BD labels all proteins containing one or more cysteine residues, and it is capable of detecting >92% of the human proteins (Tyagarajan et al., 2003; Wiktorowicz et al., 2017). The advantage of this approach is the quantitative labeling of protein cysteine residues in a stable and specific fashion to analyze them through two-dimension gel electrophoresis (2D-GE) and mass spectrometry (Wiktorowicz et al., 2017). Our predictive modeling of the proteome datasets suggest that proteins belonging to the signaling networks of (a) cell death and (b) proliferation and recruitment/migration of immune and fibroblast cells were most affected by S-nitrosylation in ChD CA and ChD CS (vs. NH) subjects. We discuss the potential significance of differential SNO protein profile in identifying the severity of ChD in infected patients.
Materials and Methods
Study Population and Statement of Ethics
The institutional review board at the University of Texas Medical Branch at Galveston (IRB 04-257), the ethics committee of the School of Health Sciences at Universidad Nacional de Salta, and the institutional review board of Health Ministry of Salta, Argentina reviewed the study protocol, and all studies were carried out after approval of the human subjects study protocol by the three entities. A written informed consent was obtained from the individuals invited to participate before collection of blood samples. The study enrolled volunteers that came to seek medical assistance at the public hospitals in Salta city, Salta, Argentina. All samples were coded and de-identified before being provided for research purposes.
Human Samples
Sera samples from all enrolled volunteers were analyzed for anti-T. cruzi antibodies by two tests. Chagatest-ELISA recombinante v.4.0 assay detects antibodies to six recombinant proteins expressed in a variety of T. cruzi isolates. Chagatest-HAI assay detects sera antibody based heamaglutination of red blood cells sensitized with cytoplasmic and membrane antigens of T. cruzi. Both assays were carried out following protocols provided by the manufacturer (Wiener, Rosario, Argentina). Samples qualified as positive by both tests were identified as seropositive, and these individulas were grouped as ChD subjects. Later, chest X-ray, electrocardiography (ECG, 12-lead at rest, and 3-lead with exercise) and transthoracic echocardiography were performed to assess the heart pathology and function to categorize enrolled individuals according to the Kuschnir classification (Sanchez-Montalva et al., 2016). Briefly, seropositive individuals with minor to no ECG abnormalities, no changes in ventricular walls, and normal ejection fraction (range: 55–70%) were grouped as ChD clinically asymptomatic (CA) subjects. Seropositive individuals with a degree of ECG abnormalities, cardiomegaly, systolic dysfunction (ejection fraction: < 55%), left ventricular dilatation (diastolic diameter ≥57 mm), and/or potential signs of heart failure were categorized as ChD clinically symptomatic (CS) patients. Seronegative subjects with no history or clinical symptoms of heart disease were enrolled as normal healthy (NH) controls (Garg et al., 2016).
Purification of PBMC, Labeling With BODIPY, and Protein Resolution by Two-Dimensional Gel Electrophoresis (2D-GE)
We enrolled ChD CA (n = 25, 46% males, age: 49.8 ± 9 years) and ChD CS (n = 28, 53% males, age: 53 ± 10.6) patients and NH (n = 30, 50% males, age: 39 ± 16 years) subjects in the study. Blood samples (10–15 ml) were collected from all enrolled patients in the heparinized, BD Vacutainer CPT Cell Preparation Tubes. The tubes were centrifuged in a swing bucket rotor at 400 × g, 4°C for 10 min. After removing the upper plasma layer, packed blood cells were diluted with ice-cold phosphate buffered saline (PBS), layered on to The FICOLL Hypaque™ density gradient, and centrifuged at 4°C at 400 × g for 40 min. The PBMC layer at the plasma-Ficoll interface was transferred to a new tube, washed with ice-cold Hank's balanced salt solution, and stored at −80°C until used (Garg et al., 2016; Koo et al., 2016).
The PBMC pellets were lysed in urea buffer (50 mM Tris pH 7.5 containing 7 M urea, 2 M thiourea, and 2% CHAPS), and all PBMC lysates were analyzed by Lowry method to evaluate the protein concentrations, and by L8800 amino acid analyzer (Hitachi High Technologies, Pleasanton, CA) to determine the cysteine (cysteic acid) levels (Koo et al., 2016). The samples were briefly centrifuged to remove cellular debris, and then split into two aliquots. Aliquot A was treated with 6 mM ascorbate (Asc+) for 1 h to reduce SNO, and aliquot B was treated with 100-μM neocuproine for 1 h to preserve SNO (Asc−). Both aliquots of each sample were separately dialyzed in dialysis tubes (3.5 kDa MWCO) against urea buffer. The dialyzed samples were labeled for 2 h with BODIPY® FL N- (2-aminoethyl) maleimide (BD, Life Technologies, Grand Island, NY) that was added at saturating concentration (BD: cysteine concentration, 60: 1 ratio). All incubations were carried out at room temperature while protecting the samples from light, and the reaction was stopped with ß-mercaptoethanol added in 10: 1 molar excess to the BD dye (Pretzer and Wiktorowicz, 2008; Wiktorowicz et al., 2011, 2017).
The BD-labeled, Asc+ and Asc− PBMC lysates (100-μg each) were separated by 2D-GE, employing an IPGphor multiple sample isoelectric focusing (IEF) device (GE Healthcare, Chicago, IL) in 1st dimension, and the Criterion Dodeca cell (Bio-Rad, Hercules, CA) in 2nd dimension, following the protocol previously described by us (Garg et al., 2016).
Gel Fixing, BD Imaging, and Image Processing
All gels were fixed in 20% methanol/7% acetic acid/10% acetonitrile for 1 h, washed with 20% ethanol / 10% acetonitrile overnight, washed with dH2O, and BD-labeled proteins were imaged at 100 μm resolution (Ex488nm/Em520nm) by using the Typhoon Trio Variable Mode Imager (GE Healthcare). The voltage was set to result in 85–99% of the saturation level for the most abundant protein on the gel. Gel images taken under the BD-specific filters were used to obtain the spot-specific data (Wiktorowicz et al., 2017).
A reference gel from the set of Asc+ gels was selected by Totallabs Ltd SameSpots software (Newcastle, UK). The reference gel was stained overnight with Sypro Ruby Stain (Life Technologies), and imaged at Ex488nm/Em560LPnm. The reference gel image (100 μM resolution) was used to ensure the detection of all protein spots irrespective of presence or absence of cysteine residues and to define the spot boundaries. The matching spot volumes from BD-stained Asc+ and Asc− gels were used to obtain the quantitative spot data (Wiktorowicz et al., 2017).
Quantification of SNO Levels
In total, 166 BODIPY-stained 2DE gels [two gels with either Asc+ or Asc− protein lysates for each sample from the NH (n = 30), ChD CA (n = 25), and ChD CS (n = 28) subjects] were scanned and anayzed using the Totallabs Progenesis SameSpots™ software. The spot volumes were subjected to statistical analysis by using built-in tools of Totallab SameSpots software. The data were found to be normally distributed, and the differential protein abundance and/or SNO levels for all protein spots between any two groups was subjected to statistical analysis by Student's t-tests with Welch's correction for unequal variances. Also, to account for the false discovery rate, Benjamini–Hochberg (B-H) multiple hypotheses testing correction was applied and significance was accepted at p-value ≤ 0.05 (Koo et al., 2016; Wiktorowicz et al., 2017).
The SNO modification levels per protein spot from each cohort (NH, ChD CA, ChD CS) were quantified by calculation of the ratio of fluorescence units from Asc− and Asc+ signal for each spot (SNO signal/total protein signal = Asc−/Asc+) (Wiktorowicz et al., 2011). Because SNO modification prevents Cys from being labeled by the BD fluor; a Asc−/Asc+ ratio of < 1 or a negative value (after log transformation) indirectly reflects the “percentage” of SNO modified protein in the spot.
The final calculation of the SNO modification per unit of protein was carried out according to the following formula, called Ratio of ratios (RoR):
By adjusting for abundance changes, the RoR is used to establish true SNO-specific changes in experimental sample with respect to controls. Moreover, it is important to note that since SNO modification prevents the Cys-BODIPY labeling, a negative value indicates an increase in SNO level (and vice versa) in the sample (Wiktorowicz et al., 2017).
Matrix Assisted Laser Desorption Ionization-Time of Flight (MALDI-TOF)/Mass Spectrometry (MS) for Protein Identification
The protein spots that were identified to be differentially abundant and/or differentially SNO-modified (p < 0.05) between any two groups were subjected for mass spectrometry identification as we have previously described (Dhiman et al., 2012; Wen et al., 2012). Briefly, protein spots (1 mm) on the 2D gels were picked robotically (ProPick II, Digilab, Ann Arbor, MI). Proteins were in-gel digested with 1% trypsin/25 mM NH4HCO3 at 37°C for 6 h. Peptide mixtures (1 μl) were directly spotted onto the MALDI TOF MS/MS target plate with 1 μl of alpha-cyano-4-hydroxycinnamic acid matrix solution (5 mg/ml in 50% acetonitrile) and analyzed by using an AB Sciex, TOF/TOF 5800 Proteomic Analyzer (Foster City, CA). The MS and MS/MS spectral data were acquired and analyzed by Applied Biosystems software package that included 4000 Series Explorer (v.3.6 RC1) with Oracle Database Schema (v.3.19.0) and Data Version (3.80.0) (Foster City, CA). The instrument was operated in a positive ion reflectron mode (focus mass: 1,700 Da, mass range: 850–3,000 Da), and 1,000–2,000 laser shots were acquired and averaged from each protein spot. A peptide mixture with the reference masses 904.468, 1296.685, 1570.677, and 2465.199 was utilized for automatic external calibration. Following MALDI MS, MALDI MS/MS was performed on 5–10 abundant ions from each protein spot. A 1 kV positive ion MS/MS method was used to acquire data under post-source decay (PSD) conditions. The instrument precursor selection window was ±3 Da. Automatic external calibration was performed by using reference fragment masses 175.120, 480.257, 684.347, 1056.475, and 1441.635 (from precursor mass 1570.700) (Dhiman et al., 2012; Wen et al., 2012).
The MS and MS/MS spectral data were searched against the UniProt human protein database (35,208,664 residues; 87,656 sequences; last accessed: July 12, 2016) by using AB Sciex GPS Explorer (v.3.6) software in conjunction with MASCOT (v.2.2.07, Matrix Science, London, UK) (Garg et al., 2016; Koo et al., 2016). The parameters used for MS peak filtering included a mass range of 800–3,000 Da, minimum S/N filter = 10, mass exclusion list tolerance = 0.5 Da, and exclusion of trypsin and keratin-containing compounds with masses of 842.51, 870.45, 1045.56, 1179.60, 1277.71, 1475.79, and 2211.1 daltons. The parameters for MS/MS peak filtering included a minimum S/N filter = 10, maximum missed cleavages = 1, fixed modification of carbamidomethyl (C), variable modifications due to oxidation (M), precursor tolerance = 0.2 Da, MS/MS fragment tolerance = 0.3 Da, mass = monoisotopic, and peptide charges = +1. The significance of a protein match, based on the MS and the MS/MS data from several precursor ions, was presented as a Protein Score with a confidence cutoff of ≥ 62. Some of the protein spots (|Fold change|≥ 1.5) identified with low confidence by MS/MS were subjected to LTQ OrbiTrap Fusion LC-MS analysis (Thermo Fisher Scientific, Waltham, MA) for protein identification (Garg et al., 2016; Koo et al., 2016).
Enzyme-Linked Immunosorbent Assay (ELISA) and Biotin Switch Assay
A new set of PBMC samples from NH, ChD CA, and ChD CS subjects (n = 10–14 per group) were lysed by agitation for 30 min at 4°C in S-nitrosylation block buffer (Cayman Chemicals, Ann Arbor MI), and protein concentrations were determined by the Bradford method (Bio-Rad). The samples were divided into two aliquots; aliquot A was used to quantify the abundance of the target protein and aliquot B was processed to measure its SNO level.
Sandwich ELISA kits were used to quantify the changes in abundance of actin gamma (Reddot Biotech, Kelowna, Canada; detection range: 0.015–10 ng/mL) and filamin A (LifeSpan Biosciences, Seattle WA; detection range: 1.88–120 ng/mL) polypeptides by following manufacturer's instructions. In brief, aliquot A of PBMC lysates (2 μg in 100 μL/well) were loaded onto 96-well plates pre-coated with target-specific antibody. After 2 h incubation at 4°C, plates were aspirated and sequentially incubated with biotin-conjugated anti-target 2nd antibody (1: 100 dilution), avidin-conjugated horseradish peroxidase (HRP) (1: 100 dilution). The plates were washed between each reagent addition, color was developed with TMB substrate, and the change in absorbance was recorded at 450 nm by using a spectrophotometer (Spectramax 190, Molecular Devices, Sunnyvale, CA). A standard curve was prepared by using recombinant proteins and target protein concentration was plotted as pg per μg total protein (Koo et al., 2016).
The SNO modification levels of actin gamma and filamin A were assessed in aliquot B of each sample by the biotin switch assay. Briefly, free SH (thiol) groups in each sample were blocked with methyl methanethiosulfonate (MMTS), and then protein SNO bonds present in the sample were converted to thiols via transnitrosation with ascorbate. The newly formed SH groups were then labeled by S-biotinylation with biotin-HPDP by using a biotin switch-based, S-Nitrosylated Protein Detection Assay Kit (Cayman Chemicals) following the instructions provided by the manufacturer. Then, 96-well plates pre-coated with antibodies against actin gamma or filamin A were incubated for 2 h with biotin-derivatized protein lysates (2 μg in 100 μL/well). Plates were washed to remove the unbound proteins and then incubated for 10 min at room temperature with the avidin-conjugated HRP (1:3,000 dilution, BioLegend, San Diego, CA). The TMB substrate was added, and the change in absorbance reflecting the levels of biotin-bound SNO-modified actin gamma or filamin A was measured by spectrophotometry (Koo et al., 2016).
Multivariate Adaptive Regression Splines (MARS) Analysis of the Proteome Datasets
MARS is a non-parametric regression procedure that automatically models non-linearities and interactions between variables (Friedman and Roosen, 1995; Austin, 2007). The input data were the Asc−/Asc+ (i.e., DSNO) values for the protein spots derived from each of the Asc− and ASC+ gels of the NH (n = 30), ChD CA (n = 25), and ChD CS (n = 28) PBMC lysates. By using the R (R Foundation, Vienna, Austria) and SPSS (v.20, IBM Corporation, Armonk, NY) software, datasets were log2 transformed, screened by Student's t-test with Welch's correction (for non-equal variances amongst the groups), and Benjamini–Hochberg multiple hypothesis testing corrections were ran for the adjusted p-values. The significantly different protein spots between any two groups with p-value of ≤ 0.05t−test/Welch/BH were used as input for MARS to model the predictive value of the selected variables in identifying ChD (vs.NH) patients. To avoid overfitting of the data, we employed two approaches: (1) 10-fold cross-validation (CV), allowing the same number of maximum basis functions as were the differentially SNO-modified spots at p-value of ≤ 0.05 (with 1 max interaction term) (Liu et al., 2017); and (2) training/testing approach in which randomly selected proteome datasets from 80% of the individuals in each group were utilized to create the model and the datasets from the remaining 20% of the subjects in each group were used to assess the fit of the model (Dobbin and Simon, 2011). The receiver operator characteristics (ROC) curves were developed to examine the sensitivity and specificity of the identified models.
Ingenuity Pathway Analysis (IPA)
To assess the biological meaning of the proteome datasets, we used the IPA web-based application (Ingenuity Systems, Redwood city, CA) (Thomas and Bonchev, 2010). Briefly, the RoR values for SNO-modified proteins in ChD and control PBMCs were uploaded in the IPA to retrieve biological information, such as gene name, subcellular location, tissue specificity, function, and association with disease, etc. from the literature. Then the datasets were integrated to define networks and signaling pathways allowing us to understand the significance of data or candidate biomarkers in the context of a larger biological system (Garg et al., 2016; Koo et al., 2016).
Results
2DE/MS Identification of Changes in PBMC SNO Proteome in Human Chagas Disease
A scheme of the steps involved in SNO proteomic analysis is presented in Figure 1. Each PBMC lysate was divided into two aliquots; aliquot A being reduced with ascorbate (Asc+, makes all cysteine residues available for BD labeling) and aliquot B (Asc−) was treated with neocuproine to preserve the SNO-modified cysteines. Labeling of the PBMC lysates with BD at saturating concentrations resulted in a no non-specific labeling. Further, BD had no effect on the isoelectric point and mobilities of the proteins/peptides, and it offered a highly sensitive method (detection limit: 5 fmol) to detect and quantitate the protein spots over a linear dynamic range of four orders of magnitude. The BD-labeled Asc+ and Asc− PBMC lysates of NH controls (n = 30), and of ChD, clinically asymptomatic (CA, n = 25) and ChD, clinically symptomatic (CS, n = 28) patients were resolved on a total of 166 2D gels. Representative Asc+ and Asc− gel images from each group are shown in Figures 2A–F. A reference gel stained with Sypro Ruby detected 635 protein spots within the relative molecular sizes of 10 to 250 kDa. The protein spot intensities on BD-labeled Asc+ and Asc− NH, ChD CA, and ChD CS gels were normalized against the reference gel, and the data were analyzed in pair-wise manner. The protein spots that were changed in abundance and/or in SNO modification levels at p ≤ 0.05t−test/Welch/BH between any of the two groups were submitted to MALDI-TOF/TOF MS analysis for protein identification.
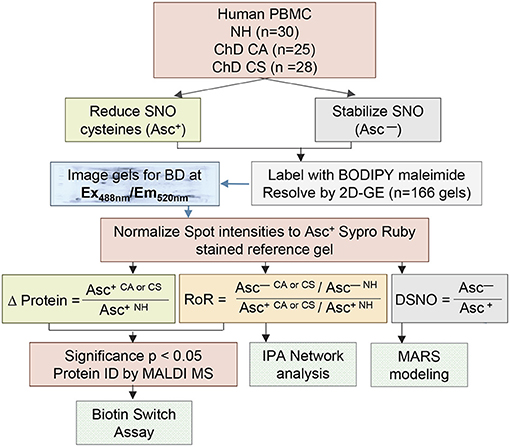
Figure 1. Schematic of work flow. Human PBMCs were obtained from volunteers categorized as seropositive, Chagas disease (ChD), clinically asymptomatic (ChD CA, n = 25) patients, seropositive, ChD clinically symptomatic (ChD CS, n = 28) patients, and seronegative, normal healthy (NH, n = 30) controls. Each sample was divided into two aliquots, and SNO cysteines were reduced with ascorbate (Asc+) in one aliquot, and stabilized with neocuproine in the 2nd aliquot (Asc −). All sample aliquots were labeled with BODIPY FL N- (2-aminoethyl) maleimide (labels reduced cysteine) and resolved by 2-dimensional gel electrophoresis. Gel images were obtained with BD specific filter. A reference gel stained with Sypro Ruby was used to mark the spot boundaries, and the spot boundaries on all the BD labeled gels were normalized to Sypro Ruby stained reference gel. Ratiometric calculation of differential protein abundance from BODIPY-fluorescence units in Asc+ aliquots (CA or CS vs. NH) was performed for all protein spots. The relative percentage of each protein spot that was S-NO modified was quantitated by calculation of the ratio of fluorescence units from Asc–/Asc+ aliquots for each experimental condition (DSNO = Asc−/Asc+). The ratio of ratios (RoR) was calculated to obtain the change in S-NO levels in ChD patients (vs. NH controls) after normalizing for the changes in protein abundance. Protein spots that changed in abundance or S-NO modification at p ≤ 0.05 were submitted to mass spectrometry analysis for protein identification. Log2 transformed DSNO datasets were used for MARS modeling and RoR datasets were analyzed by Ingeunity pathway analysis (IPA). Selected proteins were confirmed for differential abundance and S-NO modification levels by ELISA/ Biotin switch assay.
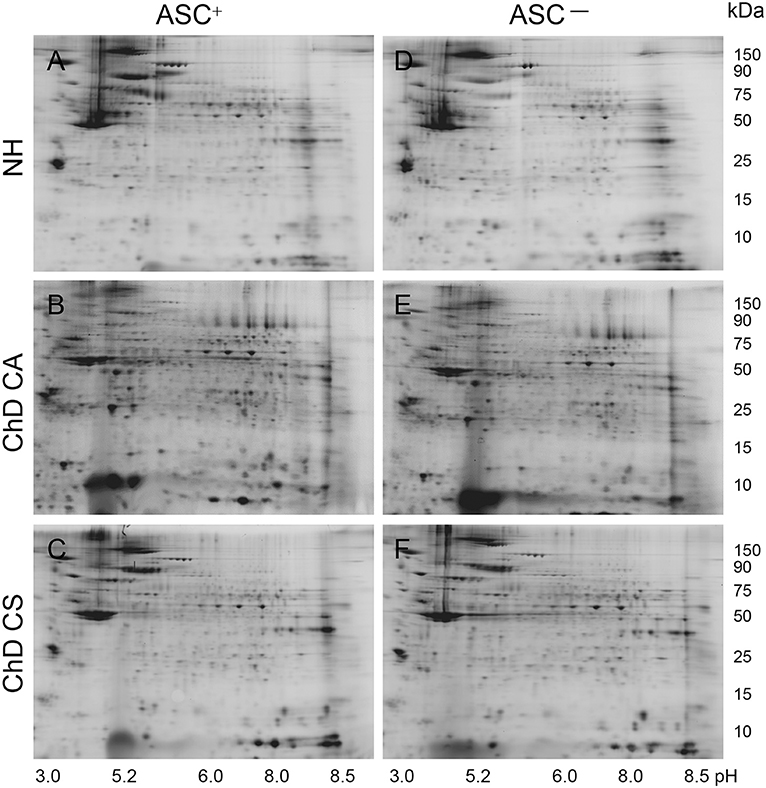
Figure 2. Representative two-dimensional gel images of protein spots in PBMC of Chagas disease subjects. BD-labeled PBMC lysates were separated in 1st dimension by isoelectric focusing on 11 cm non-linear pH 3–11 immobilized pH gradient strips, and in 2nd dimension by sodium dodecyl sulfate polyacrylamide gel electrophoresis (SDS-PAGE) on an 8–16% gradient gel. Gel images were obtained at 100 μm resolution to quantify BD-labeled proteins (Ex488nm/Em520 ± 15nm). Shown are the representative gel images of Asc+ (A–C) and Asc− (D–F) PBMC lysates from NH (A,D) controls, and ChD CA (B,E), and ChD CS (C,F) patients. Approximate size of the proteins in kDa (vertical) and pI ranges (horizontal) are noted.
Of the 312 protein spots submitted to mass spectrometry, 249 protein/peptide spots were correctly identified (Table 1). Of these 12, 6 and 8 protein spots exhibited a change in Asc−/Asc+ ratio (i.e., DSNO value) that indirectly reflects the “percentage” of SNO modified protein in the spot (fold change: ≥1.5 at p-value of ≤ 0.05t−test/Welch/BH) in the NH, ChD CA, and ChD CS subjects (Table 1). The RoR values for all protein spots were calculated to normalize the changes in SNO levels with respect to changes in abundance. The RoR values indicated that 30 (20 increased, –ve RoR/10 decreased, +ve RoR) and 28 (22 increased, –ve RoR/6 decreased, +ve RoR) protein spots were differentially SNO-modified (normalized to change in abundance) in ChD CA and ChD CS subjects, respectively, in comparison to NH controls (fold change: |≥1.5|, p ≤ 0.05t−test/Welch/BH, Figure 3A). The changes in Cys-SNO modification frequency (RoR values) of the protein spots ranged from 1.79- to −1.99-fold and 2.20- to −2.11-fold in ChD CA and ChD CS patients (vs. NH subjects), respectively (Figure 3A). Venn diagram showed that 11 protein spots changed in RoR values in both ChD CA and ChD CS patients (vs. NH controls), and 19 and 17 protein spots exhibited changes in RoR values uniquely associated with the ChD CA and ChD CS patients, respectively (Figure 3B). The top molecules that were significantly changed in RoR values in ChD CA and ChD CS subjects (vs. NH controls) are shown in Figures 3C,D. The top molecules that distinguished the RoR and abundance profiles of ChD CS patients with respect to ChD CA patients are presented in Figures 3E,F. Note that proteomic profile identified some of the protein spots (e.g., PSMB3, SH3BGRL2, ACTB, THBS1) that were significantly changed in RoR values in both ChD CA and ChD CS patient groups; and other proteins (e.g., ACTG, ALB, HNRNPA1, PTH2R, KRT83, MPO, FGB, and ACTB) exhibited significant differences in RoR values between ChD CA and ChD CS subjects (Figure 3E). Importantly, proteomic profiling identified FLNA and ACTG were uniquely changed in RoR values in ChD CA and ChD CS subjects, respectively (Figures 3C,D), and SNO ACTG (spot# 563) was also differentially expressed between CA and CS patients (Figure 3E), and appears to be a hallmark of ChD severity.
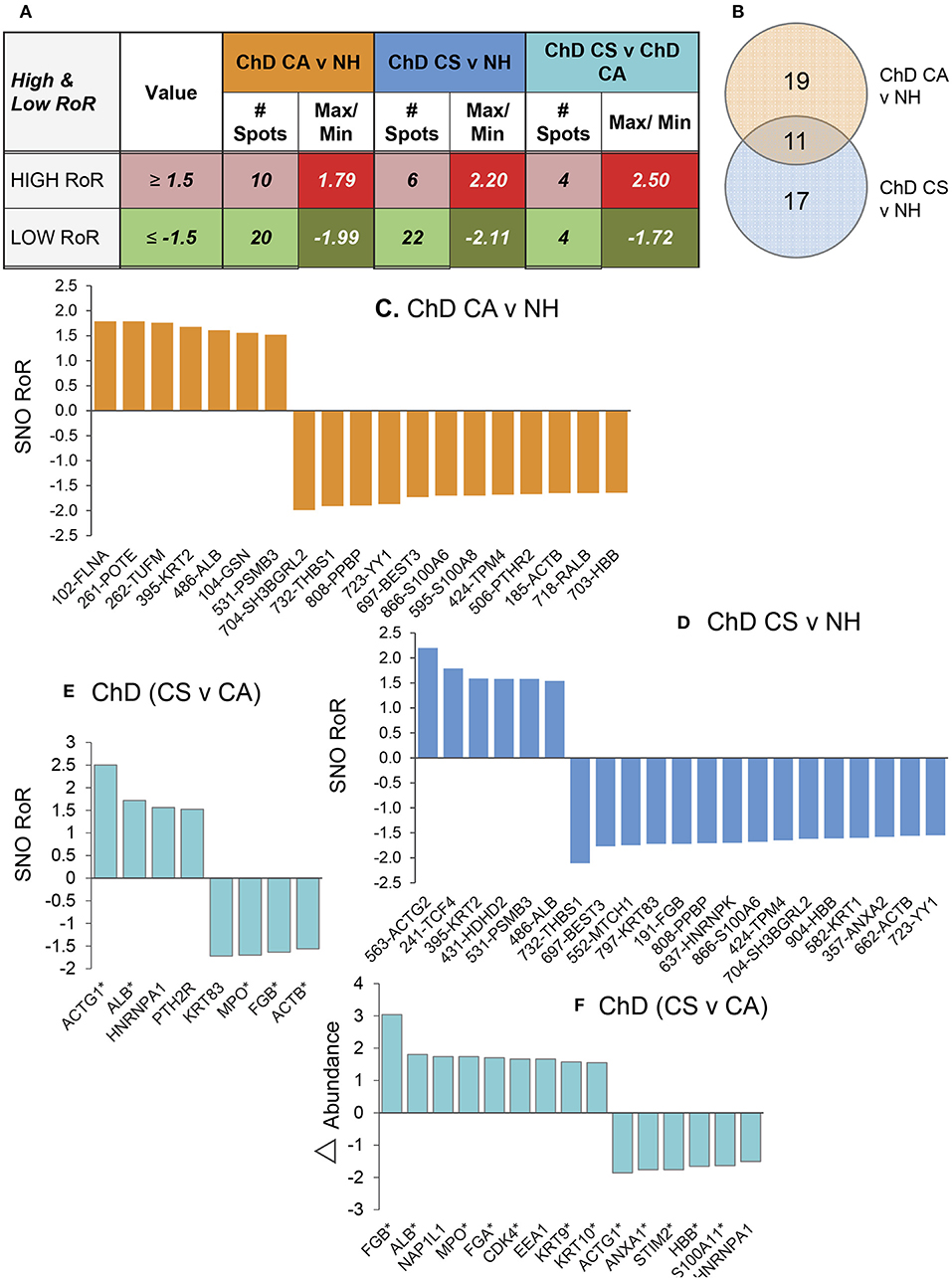
Figure 3. PBMC SNO signature in Chagas disease. (A) Number of protein spots that were changed in SNO levels (after normalizing for change in abundance) in ChD CA and ChD CS subjects with respect to NH controls (p ≤ 0.05) were calculated by ratio of ratio (RoR) method. Different intensity of red and green colors are in correspondence with Table 1. The darker shade means the higher values. (B) Venn diagram. Shown are the number of protein spots that were uniquely SNO modified (calculated by RoR method) in Chagas disease clinically asymptomatic and Chagas diease clinically symptomatic subjects. (C–E) Bar graphs show the RoR values of the top molecules in ChD CA vs. NH (C), ChD CS vs. NH (D) and ChD CS vs. ChD CA (E) patients. A negative RoR indicates increased SNO modification and a positive RoR indicates increased reduction of protein thiols. The * next to protein name indicates multiple protein spots. (F) Proteins spots that also changed in abundance between ChD CS vs. ChD CA subjects (≥1.5-fold) are shown.
Biotin Switch Assay for Verification of SNO-Modified Proteins in ChD
We utilized a new set of PBMC from NH, CA, and CS cohorts (n = 10–14 per group), and employed ELISA and biotin-switch assays to verify the changes in abundance and SNO levels of two proteins in Chagas disease. We chose filamin A and actin gamma for these studies because spot#102 and spot#563 for these proteins were found to have most changes at RoR level in ChD CA and ChD CS subjects, respectively (Figures 3C,D, 4A).
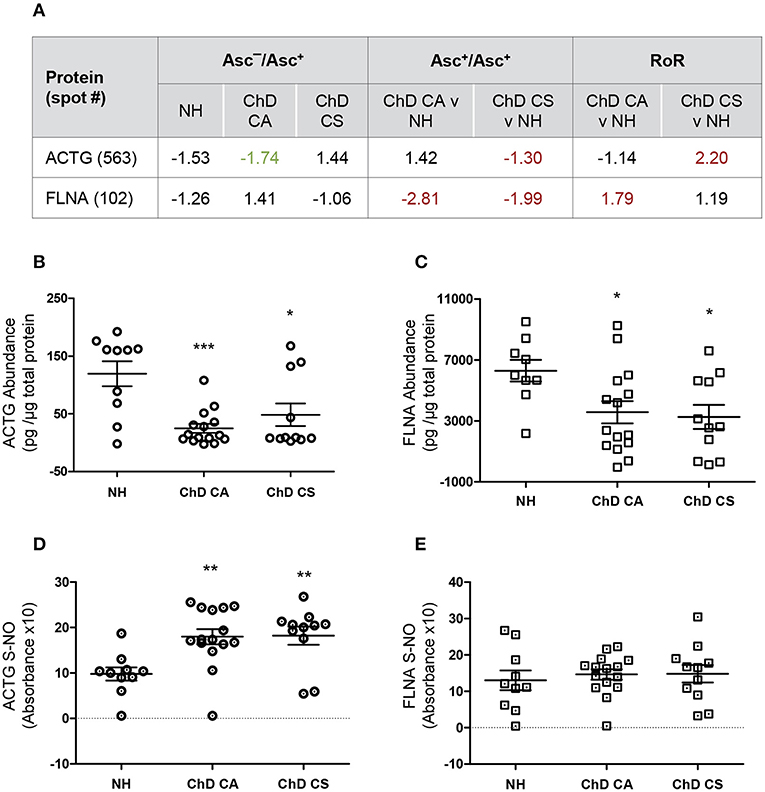
Figure 4. Biotin switch assay for verification of abundance and SNO levels of ACTG and FLNA in Chagas disease. (A) SNO proteome dataset for ACTG and FLNA are presented. (B–E) PBMC protein lysates (2 μg) from normal healthy (NH) controls and seropositive, Chagas Disease clinically asymptomatic (ChD CA) and Chagas disease clinically symptomatic (ChD CS) patients (n = 10–14 per group) were evaluated for abundance (B,C) and SNO (D,E) levels of ACTG (B,D) and FLNA (C,E) by using an ELISA-based method. For the analysis of SNO-modified proteins, PBMC lysates were subjected to biotin switch procedure prior to a modified ELISA protocol as described in section Materials and Methods. ANOVATukey test was performed to evaluate the significance (*p < 0.05, **p < 0.01, and ***p < 0.001).
We first examined the changes in abundance of ACTG and FLNA in ChD patients and NH controls by an ELISA. In ChD CA patients (vs. NH controls), we observed 79% (p < 0.001) and 49.6% (p < 0.05) decline in the ACTG and FLNA protein levels, respectively (Figures 4A,B). In ChD CS patients, ACTG and FLNA protein levels were decreased by 59.6 and 48%, respectively (vs. NH controls, p < 0.05, Figures 4A,B). The finding of a decline in FLNA in ChD CA and ChD CS patients and of ACTG in ChD CS patients by ELISA was in alignment with the finding of a decline in the abundance of these proteins in the proteomic study (Asc+ChD/Asc+NH, Figure 4A). The finding of an overall decline in ACTG level in ChD CA patients by ELISA did not corroborate the proteomic finding (compare Figure 4B and Figure 4A).
For the detection of SNO modification levels of proteins in PBMC lysates, the reduced thiol groups were blocked, and then SNO-modified proteins were reduced to make these available for binding with biotin, and detection by avidin-conjugated horseradish peroxidase method. These data showed >80% changes in SNO levels of ACTG in PBMC from ChD CA and ChD CS patients (vs. NH subjects, Figure 4D). No significant changes in the SNO levels of FLNA were detected between PBMC of the three groups (Figure 4E).
The differences in the observations between ELISA/Biotin Switch Assay and BD-fluor/2D-GE based proteomic study can at least partially be explanined by the fact that 2D-GE/proteomic approach identifies the full length protein as well as derived peptides as individual spots that may change in concentration depending on their stability and/or degradation, while ELISA did not discriminate between the full length and smaller peptides of these proteins; the level of detection will depend on the epitopes that the coated antibody recognizes. Secondly, Biotin switch assay simply detects the SNO-modified proportion of a protein but does not take into consideration the changes in its abundance, while RoR approach normalizes SNO level against the protein concentration in experimental sample and then also derives the values in comparison to control data. At present no other reliable and easy to use assays exist that can detect the SNO modification level per unit protein. Despite the discrepancies between the two approaches, our results in Figure 4 suggest that use of the coating antibodies against specific ACTG and FLNA peptides that are SNO-modified will offer a more reliable diagnostic approach in distinguishing the disease state in Chagas patients.
MARS Predictive Modeling of SNO-Modified Proteins in Chagas Disease
For this analysis, we log2 transformed the protein spot intensities to ensure that biologically relevant proportional changes are captured between the different groups (Feng et al., 2013). The log2 transformed protein spot intensities on each of the Asc− and Asc+ gels of PBMC lysates from NH (n = 30), ChD CA (n = 25), and ChD CS (n = 28) subjects were used to calculate the Asc−/Asc+ ratios for each spot, and the protein spots that exhibited significant changes in the Asc−/Asc+ ratio (indicates relative proportion of the SNO-modified protein in the spot) at p ≤ 0.05t−test/Welch/BH between any of the two groups were subjected to MARS analysis to develop the classification model.
Comparing Asc−/Asc+ values of ChD CA vs. NH groups, we identified nine protein spots that exhibited differential SNO modification level (p ≤ 0.05t−test/Welch/BH, Figure 5A). These nine spots were used as input to MARS modeling with 10-fold CV and 80/20 approaches. The CV MARS model ranked the nine spots with high to low priority and allocated predictive values to top ranked molecules (spot#582-KRT1 and spot#884-TPM3). The CV training (AUC/ROC = 1.0) and testing [(AUC/ROC = 1.0) models exhibited high confidence in correctly identifying the ChD CA patients (vs. NH controls] with 100% prediction success and no mistakes (Figure 5B). The 80/20 approach built the predictive model based on top ranked molecule (spot#884-TPM3). The training of this model by using datasets from randomly selected 80% of the samples from NH and ChD CA groups (AUC/ROC = 1.0) and testing of this model by using datasets from remaining 20% of the samples from the same groups (AUC/ROC = 1.0) also yielded high confidence in correctly identifying the NH and ChD CA subjects with 100% prediction success (Figure 5B).
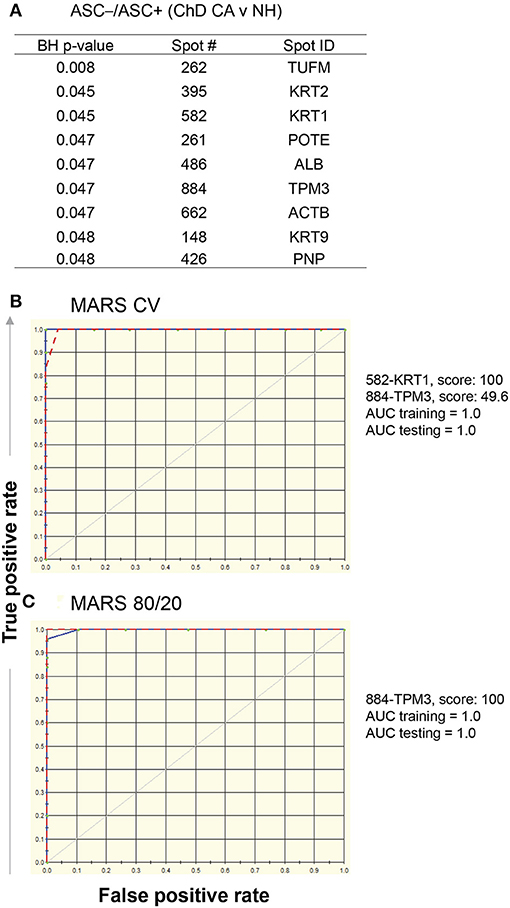
Figure 5. MARS modeling of SNO-modified proteins for classification of clinically asymptomatic ChD patients. For this, log2 transformed protein spot intensities on each of the Asc+ and Asc− gels of PBMC lysates from ChD clinically asymptomatic (ChD CA, n = 25) and normal healthy (NH, n = 30) controls were used to calculate Asc−/Asc+ ratio (indicates relative proportion of the protein spot that is SNO modified). (A) List of protein spots showing significant changes in Asc−/Asc+ ratio in ChD CA patients vs. NH controls at p-value of <0.05t−test/Welch/BH. The 9 molecules listed in (A) were used as input for MARS analysis. We employed (B) 10-fold cross-validation (CV) and (C) 80% testing / 20% training approaches to assess the fit of the model for training and testing dataset. The ROC curves show the prediction success of the CV (B) and 80/20 (C) models is high for both training (blue curve) and testing (red curve) data.
Comparing Asc−/Asc+ values of ChD CS vs. NH groups, we identified 11 protein spots that exhibited significant differences in SNO modification level (p < 0.05t−test/Welch/BH, Figure 6A). These eleven spots were used as input to MARS modeling with 10-fold CV and 80/20 approaches. The CV MARS model ranked the eleven spots with high to low priority and allocated predictive values to top ranked molecules (spot#426-PNP, spot#904-HBB, and spot#662-ACTB). The CV training model correctly identified 90% and 92.86% of the NH and ChD CS subjects, respectively, with overall prediction success of 91.38% (AUC/ROC = 0.95, Figure 6B). However, the CV model performed poorly in testing phase, and correctly identified only 73.3 and 64.3% of the NH and ChD CS subjects, respectively (AUC/ROC = 0.79, Figure 6B). The 80/20 approach chose four top-ranked molecules (spot#426-PNP, spot#582-KRT1, spot#486-ALB, and spot#662-ACTB), and this model performed better than the CV model in predicting the ChD CS vs. NH subjects. The training of this model by using datasets from 80% of the NH and ChD CS subjects yielded high confidence (AUC/ROC = 0.99, Figure 6C) with an overall prediction success of 82.61% and correctly identified 100% of ChD CS subjects. The testing of this model with datasets from 20% of the NH and ChD CS subjects also yielded good confidence (AUC/ROC = 0.89, Figure 6C) with an overall prediction success of 83.33%.
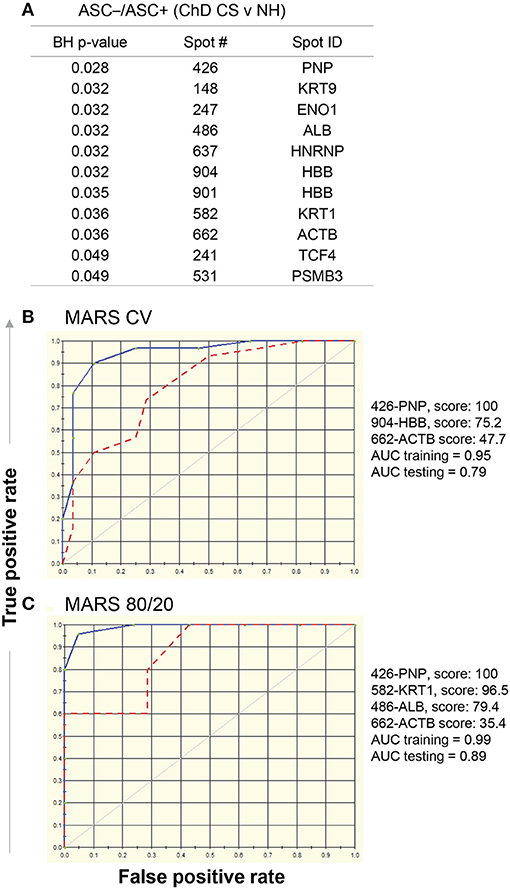
Figure 6. MARS modeling of SNO-modified proteins for classification of clinically symptomatic ChD patients. As in Figure 4, log2 transformed protein spot intensities on each of the Asc+ and Asc− gels of PBMC lysates from ChD clinically symptomatic (ChD CS, n = 28) patients and normal healthy (NH, n = 30) subjects were used to calculate Asc−/Asc+ ratios for each protein spot (A) List of protein spots showing significant changes in Asc−/Asc+ ratio in ChD CS patients (vs. NH controls at p-value of <0.05t−test/Welch/BH. The 11 molecules listed in (A) were used as input for MARS analysis. (B) The ROC curves show the prediction success of the 10-fold cross validation model is high for the training data but not for the testing data. (C) The ROC curves for 80/20 model show good prediction success for the training and testing data. Blue curve: training data, red curve: testing data.
Together, the results presented in Figures 5, 6 suggest that a) SNO modification of KRT1 and TPM3 peptides will have high predictive value in diagnosing the ChD CA status of infected patients; and b) SNO modification of PNP HBB, KRT1, ACTB, and ALB peptides will have high predictive value in identifying the ChD CS status of infected patients. Further, the predictive modeling worked more robustly in identifying the ChD CA subjects than in recognizing the ChD CS status. Furthermore, the protein spots predicted by this analysis were in accordance with significative RoR levels showed in Figures 3C,D (vs. Figure 5 and Figure 6, respectively). The MARS did not yield high degree of success in distinguishing the ChD CA vs. ChD CS groups (data not shown).
Ingenuity Pathway Analysis of Chagas Disease Associated SNO Proteome Signature
We employed Ingenuity Pathway Analysis software to determine molecular and biological functions, as well as the important pathways and networks involved in Chagas disease development. Input to IPA were the RoR values (p ≤ 0.05t−test/Welch/BH, Table 1) and we established a cut-off of |fold change|: ≥ 1.2 to ensure sufficient molecules are available to build the disease-related networks. IPA analysis showed that several molecules that are predicted to be associated with cell death were significantly changed in their RoR values in ChD CA (vs. NH) subjects (14 molecules, p = 1.89E-02, Figure 7A). Likewise cell death associated 15 molecules exhibited significant changes in RoR values in ChD CS (vs. NH) subjects (p = 9.6E-03, Figure 7B). The cell death network was predicted to be more responsive to SNO modification level of proteins (ANXA1, FGA, FLNA, GSTP1, HBB, MTPN, PRDX6, THBS1, VCL, YY1) in clinically symptomatic patients as compared to the clinically asymptomatic ChD subjects (p = 8.71E-03, Figure 7C).
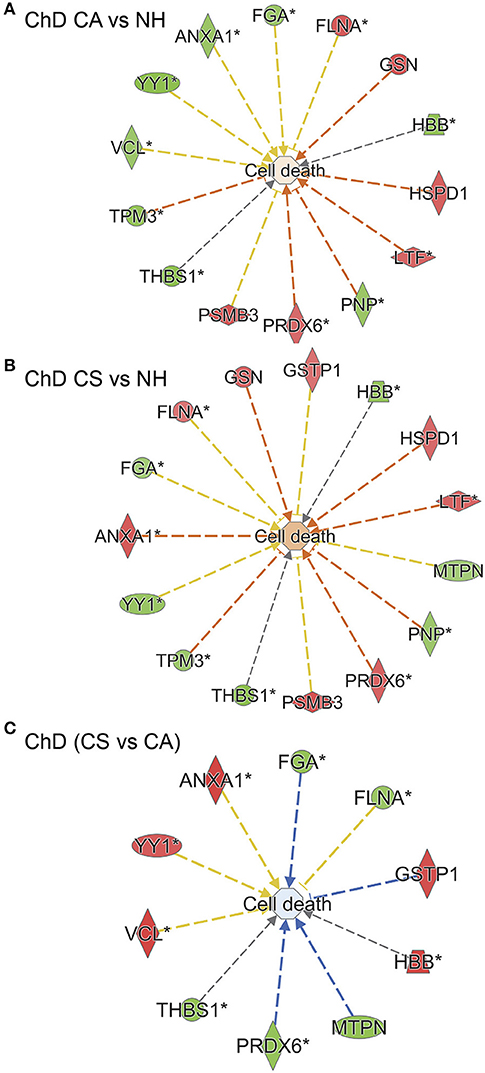
Figure 7. SNO profile of cell death network in Chagas disease (ChD). PBMC SNO proteome of ChD clinically asymptomatic (ChD CA, n = 25) and ChD clinically symptomatic (ChD CS, n = 28) patients and of normal healthy controls (NH, n = 30) was developed as described in section Materials and Methods. The RoR values for protein spots that were differentially SNO-modified (normalized to change in protein abundance) at p-value of <0.05 were uploaded in the ingenuity Pathway Analysis (IPA) software, and proteins that changed in RoR at fold change: |≥ 1.2| were included in biological modeling by IPA. Shown are molecules that are predicted to be associated with cell death and that were significantly changed in RoR values in (A) ChD CA patients vs. NH controls, (B) ChD CS patients vs. NH controls, and (C) ChD CS vs. ChD CA patients. In the networks, the intensity of red and green colors show the extent of decrease and increase in protein SNO modification, respectively. Brownish orange node/lines and blue node/lines show predicted activation and inhibition, respectively, of a pathway. Gray and yellow lines are used when the putative effect is not completely understood.
IPA analysis of RoR proteome datasets also predicted a putative differential SNO profile of proteins involved in recruitment of immune cells, i.e., leukocytes, neutrophils, and phagocytes, and overall development of inflammatory response in ChD CA patients (13 molecules, p: 1.84E-02 to 3.26E-04, z score range: 0.9–1.954, Figure 8A) and ChD CS patients (9 molecules, p: 2.37 E-03 to 4.52E-03, z score range: 0.8–1.387, Figure 8B) when compared to the NH controls. The extent of SNO modification of proteins predicted to contribute to the proliferation of HEMATOPOIETIC cells (ACTB, CDK4, GRB2, GSTP1, VCL, YY1, ANXA1, ANXA2, FLNA, THBS1, HBB, and TCF4) was more pronounced in ChD CS (vs. ChD CA) patients (p: 5.12E-03, z score: 1.0, Figure 8C). However, a –ve z score values indicated that S-nitrosyation of proteins in ChD CS (vs. ChD CA) patients may be used to control the acute phase like signaling (FGA, GRB2, RALB, VCL, z score: −2.449, p = 5.28E-07), cell proliferation of fibroblasts and migration of cells (z scores: −1.471 and −1.659, respectively, p: 3.37E-02). Together the results presented in Figures 6, 7 suggest that changes in SNO modification levels might serve as an important mechanism in regulating cell death, cell proliferation, and the development of inflammatory and fibrotic responses with progression of heart disease in Chagas disease patients.
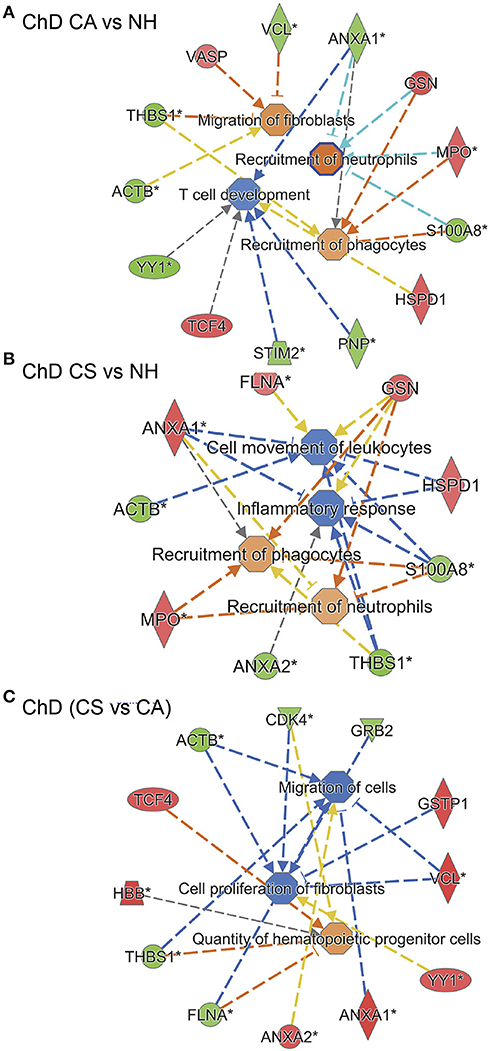
Figure 8. SNO profile of inflammation and immune responses network in Chagas disease. As in Figure 6, PBMC SNO proteome of ChD clinically asymptomatic (ChD CA, n = 25) and ChD clinically symptomatic (ChD CS, n = 28) patients and of normal healthy controls (NH, n = 30) was developed as described in section Materials and Methods. The RoR values for protein spots that were differentially SNO-modified (normalized to change in protein abundance) at p-value of <0.05 were uploaded in the ingenuity Pathway Analysis (IPA) software, and proteins that changed in RoR at fold change: |≥ 1.2| were included in biological modeling by IPA. Shown are molecules that are predicted to be associated with proliferation and recruitment of immune cells and fibroblasts and that were significantly changed in RoR values in (A) ChD CA patients vs. NH controls, (B) ChD CS patients vs. NH controls, and (C) ChD CS vs. ChD CA patients. In the networks, the intensity of red and green colors show the extent of decrease and increase in protein SNO modification, respectively. Brownish orange node/lines and blue node/lines show predicted activation and inhibition, respectively, of a pathway. Gray and yellow lines are used when the putative effect is not completely understood.
Discussion
In this discovery proteomic study, we have focused on identifying the S-nitrosylation profile of PBMC from seropositive ChD patients with or without clinical disease in comparison with seronegative healthy subjects (n = 25–30 per group). We ran 166 2D gels to resolve the protein samples and utilized BODIPY® FL N- (2-aminoethyl) maleimide labeling to detect changes in SNO modification in PBMC samples. Of the 635 protein spots that were detected by 2D-GE, 312 protein spots exhibited significant differences in abundance and/or SNO-modification levels (p ≤ 0.05) between any of the two groups, and 249 of these protein spots were successfully identified with high confidence (Table 1). Further, 30 and 28 protein spots were differentially SNO-modified (RoR fold change |≥ 1.5|, p < 0.05) in ChD CA and ChD CS patients, respectively (Table 1 and Figure 3). Eight of these molecules (732-THBS1, 697-BEST3, 808-PPBP, 866-S100A6, 424-TPM4, 704-SH3BGRL2, 582-KRT1, and 723-YY1) were significantly increased in SNO modification levels (indicated by negative RoR values) in ChD CA as well as ChD CS patients. We postulate that increase in SNO modification of these molecules in a ChD CA patient would serve as a warning for the development of Chagas cardiomyopathy, and absence of SNO of these eight molecules would indicate the seropositive subject is not at risk of heart disease development. This hypothesis will need to be tested in future studies.
We noted increased SNO modification (–ve RoR value) of three proteins (THBS1, S100A6, abd SH3BGRL2) in ChD patients in this study, as well in congestive heart failure (CHF) patients of idiopathic etiology in a previous study (Koo et al., 2016). Conversely, BEST3, PPBP, and YY1 exhibited opposing pattern, with a decreased s-nitrosylation in CHF patients (Koo et al., 2016) and an increase in SNO modification in ChD patients (Table 1). These findings indicate the common and distinct features of s-nitrosylation in the development of HF of diverse etiologies. Also interesting is the presence of PSMB3 as part of SNO-proteome signature of ChD subjects but not found in our previous CHF study. This is suggestive of a post-translational modification induced by the presence of the parasite. Further studies should be performed in the future in order to validate this hypothesis.
We performed MARS analysis of the datasets to understand the diagnostic potential of the SNO proteome datasets in identifying the disease status in Chagas patients (Figures 5, 6). MARS creates models based on piecewise linear regressions. It searches through all predictors to find those most useful for predicting outcomes and then creates an optimal model by a series of regression splines called basis functions (Sun et al., 2007; Benedet et al., 2018). For this, MARS uses a two-stage process; the first half of the process involves creating an overly large model by adding basis functions that represent either single variable transformations or multivariate interaction terms. In the second stage, MARS successively deletes basis functions, starting with the lowest contributor in order of least contribution to the model until the optimum model is reached. The end result is a classification model based on single variables and interaction terms that will optimally determine class identity (Sun et al., 2007; Benedet et al., 2018). The MARS analysis with two approaches to avoid overfitting of the datasets showed high-to-moderate confidence in predictive value of differential SNO modification of select proteins in identifying ChD CA (KRT1 and TPM3) and ChD CS (PNP, KRT1, ALB, HBB, ACTB) patients (Figures 4, 5). One limitation of these observations is that MARS models were built on the Asc−/Asc+ ratios of the protein spots, and did not consider the changes in abundance of the respective proteins. As yet, our data provide the framework for designing the multiplex diagnostic assays targeting change in abundance and SNO modification levels of the host proteins in diagnosing the exposure to parasite, disease state, and cure post-treatment. Indeed, in recent years, key s-nitrosylation targets important in cardiovascular pathophysiology of diverse etiologies were identified (reviewed in Maron et al., 2013). For example, SNO of several proteins of RyR2 and L-type Ca+2 channels was found to be associated with modulation of myocardial contractility, electromechanical function, and ventricular fibrillation in congestive heart failure (Burger et al., 2009), while others have shown the s-nitrosylation of mitochondrial proteins was protective against ischemia and reperfusion injury (Sun et al., 2007; Murray et al., 2011). Other investigators showed potential value of nitroyslation/denitrosylation status in identifying the clinical outcomes after septic shock in experimental models, though specific target proteins were not identified (Benedet et al., 2018). Some researchers have employed proteomic approach and found the s-nitrosylation of proteins important for synapse function, metabolism, and Alzheimer's disease pathology in the brain tissue during early stages of neurodegeneration (Seneviratne et al., 2016). We propose that the future longitudinal studies with larger patient cohorts will justly assess and confirm the potential value of the SNO-modified protein spots identified in this study in diagnosing the progression of cardiac disease in Chagas patients.
Ingenuity Pathway Analysis of the proteome datasets (RoR values, fold change |≥1.2|, p < 0.05) pointed to the importance of SNO modification of several molecules involved in proliferation, recruitment and migration of immune cells and fibroblasts, and in cell death pathway during the development and progression of Chagas disease (Figures 7, 8). A change in SNO modification of proteins involved in cellular disassembly and disorganization associated with disruption of filaments that is central to remodeling of the cytoskeleton and modulation of cell shape for migration was observed in PBMC of all ChD patients (Figure 8). Whether s-nitrosylation of proteins involved in immune cell proliferation, migration and cell death play a cardioprotective or cardiotoxic role in Chagas disease remains to be seen in future studies. However, we specifically discuss the proteome profile of two proteins. Our data showed the SNO as well as abundance profiles of beta and gamma isoforms of actins (ACTB, ACTG) that are the highly conserved proteins of the cytoskeleton and coexist in most cell types, that are responsible for maintaining the cell integrity, and also are mediators of internal cell motility (Chang and Goldman, 2004) were altered in PBMC of ChD patients (Table 1, Figure 8, Garg et al., 2016). Likewise, several isoforms of filamin A (FLNA), an actin binding protein that links actin filaments to membrane glycoproteins, that interacts with several molecules (e.g., integrins, transmembrane receptor complexes, and second messengers) (Nakamura et al., 2011), and that is shown to have effects on cell shape and cell migration, were also altered by s-nitrosylation in PBMC of ChD patients (Table 1). A recent study has also shown that filamin A, through its interaction with Drp1 (modulator of mitochondrial dynamics), attenuates the mitochondrial hyperfission and cardiomyocytes' senescence in an animal model of myocardial infarction (Nishimura et al., 2018). We have shown the mitochondrial dysfunction of electron transport chain, and mitochondrial production of reactive oxygen species (ROS) was exacerbated in Chagas disease (Wen and Garg, 2008, 2010; Lopez et al., 2018), and mtROS provided signal to NFκB-dependent activation of proinflammatory response in immune and non-immune cells (Ba et al., 2010; Gupta et al., 2011). These observations allow us to propose that (a) SNO modification of a select panel of proteins, specifically actins and filamins, determines the activation, migration and survival of immune cells in the circulatory system of Chagas patients, and (b) proinflammatory activation and/or senescence/death of immune cells by the ROS produced by mitochondria in the cardiac environment determines the clinical outcomes in the infected individuals with the progression of Chagas disease.
In summary, we have presented unbiased SNO proteomic analysis of PBMC of Chagas disease patients in this study. We have identified the possible pathologic mechanisms in disease progression that involve immune cell activation and cell death. MARS-modeling identified a panel of protein spots that if monitored in infected individuals, would have high degree of success in predicting risk of clinical disease development.
Author Contributions
MZ collected and processed the human samples. MZ, JW, HS, S-JK, and NG performed experiments, analyzed data, and wrote manuscript. NB, AN, JN, VB, FI and RL recruited patients, performed physical/clinical exam, serology, and other examination, and clinically characterized patients, everyone was involved in study design, and reviewing the manuscript. NG provided financial support for carrying out the experiments. RL and NB supported the patient exam.
Funding
This work was supported, in part, by grants from the National Institute of Allergy and Infectious Diseases, National Institutes of Health (R01AI054578; R01AI136031) to NG. S-JK was the recipient of a pre-doctoral fellowship from the UTMB McLaughlin Endowment, IHII and from the American Heart Association. MZ is supported by CONICET, Argentina.
Conflict of Interest Statement
The authors declare that the research was conducted in the absence of any commercial or financial relationships that could be construed as a potential conflict of interest.
Acknowledgments
We are thankful for the support provided in patients' sample collection and serology tests by Dr. Ines Vidal and lab technicians of the Central Laboratory at the San Bernardo Hospital and the Papa Francisco Hospital in Salta, Argentina.
References
Austin, P. C. (2007). A comparison of regression trees, logistic regression, generalized additive models, and multivariate adaptive regression splines for predicting AMI mortality. Stat. Med. 26, 2937–2957. doi: 10.1002/sim.2770
Ba, X., Gupta, S., Davidson, M., and Garg, N. J. (2010). Trypanosoma cruzi induces ROS-PARP-1-RelA pathway for up regulation of cytokine expression in cardiomyocytes. J. Biol. Chem. 285, 11596–11606. doi: 10.1074/jbc.M109.076984
Balouz, V., Aguero, F., and Buscaglia, C. A. (2017). Chagas disease diagnostic applications: present knowledge and future steps. Adv. Parasitol. 97, 1–45. doi: 10.1016/bs.apar.2016.10.001
Benedet, P. O., Menegatti, A. C. O., Goncalves, M. C., Terenzi, H., and Assreuy, J. (2018). The therapeutic value of protein (de)nitrosylation in experimental septic shock. Biochim. Biophys. Acta Mol. Basis Dis. 1864, 307–316. doi: 10.1016/j.bbadis.2017.10.029
Bern, C., Kjos, S., Yabsley, M. J., and Montgomery, S. P. (2011). Trypanosoma cruzi and Chagas' Disease in the United States. Clin. Microbiol. Rev. 24, 655–681. doi: 10.1128/CMR.00005-11
Bonney, K. M. (2014). Chagas disease in the 21st century: a public health success or an emerging threat? Parasite 21:11. doi: 10.1051/parasite/2014012
Bonney, K. M., Luthringer, D. J., Kim, S. A., Garg, N. J., and Engman, D. M. (2019). Pathology and pathogenesis of Chagas heart disease. Annu. Rev. Pathol. Mech. Dis. 14, 419–445. doi: 10.1146/annurev-pathol-020117-043711
Burger, D. E., Lu, X., Lei, M., Xiang, F. L., Hammoud, L., Jiang, M., et al. (2009). Neuronal nitric oxide synthase protects against myocardial infarction-induced ventricular arrhythmia and mortality in mice. Circulation 120, 1345–1354. doi: 10.1161/CIRCULATIONAHA.108.846402
Chang, L., and Goldman, R. D. (2004). Intermediate filaments mediate cytoskeletal crosstalk. Nat. Rev. Mol. Cell Biol. 5, 601–613. doi: 10.1038/nrm1438
Dhiman, M., Zago, M. P., Nunez, S., Nunez-Burgio, F., and Garg, N. J. (2012). Cardiac oxidized antigens are targets of immune recognition by antibodies and potential molecular determinants in Chagas disease pathogenesis. PLoS ONE 7:e28449. doi: 10.1371/journal.pone.0028449
Dobbin, K. K., and Simon, R. M. (2011). Optimally splitting cases for training and testing high dimensional classifiers. BMC Med. Genomics 4:31. doi: 10.1186/1755-8794-4-31
Feng, C., Wang, H., Lu, N., and Tu, X. M. (2013). Log transformation: application and interpretation in biomedical research. Stat. Med. 32, 230–239. doi: 10.1002/sim.5486
Friedman, J. H., and Roosen, C. B. (1995). An introduction to multivariate adaptive regression splines. Stat. Methods Med. Res. 4, 197–217. doi: 10.1177/096228029500400303
Garg, N. J., Soman, K. V., Zago, M. P., Koo, S. J., Spratt, H., Stafford, S., et al. (2016). Changes in proteome profile of peripheral blood mononuclear cells in chronic Chagas disease. PLoS Negl. Trop. Dis. 10:e0004490. doi: 10.1371/journal.pntd.0004490
Gupta, S., Dhiman, M., Wen, J. J., and Garg, N. J. (2011). ROS signalling of inflammatory cytokines during Trypanosoma cruzi infection. Adv. Parasitol. 76, 153–170. doi: 10.1016/B978-0-12-385895-5.00007-4
Htet Hlaing, K., and Clement, M. V. (2014). Formation of protein S-nitrosylation by reactive oxygen species. Free Radic. Res. 48, 996–1010. doi: 10.3109/10715762.2014.942842
Koo, S. J., Spratt, H. M., Soman, K. V., Stafford, S., Gupta, S., Petersen, J. R., et al. (2016). S-Nitrosylation proteome profile of peripheral blood mononuclear cells in human heart failure. Int. J. Proteomics 2016:1384523. doi: 10.1155/2016/1384523
Liu, C., Gu, X., and Jiang, Z. (2017). Identification of novel targets for multiple myeloma through integrative approach with Monte Carlo cross-validation analysis. J. Bone Oncol. 8, 8–12. doi: 10.1016/j.jbo.2017.08.001
Lopez, M., Tanowitz, H. B., and Garg, N. J. (2018). Pathogenesis of chronic Chagas disease: acrophages, mitochondria, and oxidative Stress. Curr. Clin. Microbiol. Rep. 5, 45–54. doi: 10.1007/s40588-018-0081-2
Machado, F. S., Dutra, W. O., Esper, L., Gollob, K. J., Teixeira, M. M., Factor, S. M., et al. (2012). Current understanding of immunity to Trypanosoma cruzi infection and pathogenesis of Chagas disease. Semin. Immunopathol. 34, 753–770. doi: 10.1007/s00281-012-0351-7
Maron, B. A., Tang, S. S., and Loscalzo, J. (2013). S-nitrosothiols and the S-nitrosoproteome of the cardiovascular system. Antioxid. Redox. Signal 18, 270–287. doi: 10.1089/ars.2012.4744
Moldogazieva, N. T., Mokhosoev, I. M., Feldman, N. B., and Lutsenko, S. V. (2018). ROS and RNS signalling: adaptive redox switches through oxidative/nitrosative protein modifications. Free Radic. Res. 52, 507–543. doi: 10.1080/10715762.2018.1457217
Monge-Maillo, B., and Lopez-Velez, R. (2017). Challenges in the management of Chagas disease in Latin-American migrants in Europe. Clin. Microbiol. Infect. 23, 290–295. doi: 10.1016/j.cmi.2017.04.013
Murray, C. I., Kane, L. A., Uhrigshardt, H., Wang, S. B., and Van Eyk, J. E. (2011). Site-mapping of in vitro S-nitrosation in cardiac mitochondria: implications for cardioprotection. Mol. Cell Proteomics 10:M110.004721. doi: 10.1074/mcp.M110.004721
Nakamura, F., Stossel, T. P., and Hartwig, J. H. (2011). The filamins: organizers of cell structure and function. Cell Adh. Migr. 5, 160–169. doi: 10.4161/cam.5.2.14401
Nishimura, A., Shimauchi, T., Tanaka, T., Shimoda, K., Toyama, T., Kitajima, N., et al. (2018). Hypoxia-induced interaction of filamin with Drp1 causes mitochondrial hyperfission-associated myocardial senescence. Sci. Signal 11:eaat5185. doi: 10.1126/scisignal.aat5185
Perez-Molina, J. A., and Molina, I. (2018). Chagas disease. Lancet 391, 82–94. doi: 10.1016/S0140-6736(17)31612-4
Pretzer, E., and Wiktorowicz, J. E. (2008). Saturation fluorescence labeling of proteins for proteomic analyses. Anal. Biochem. 374, 250–262. doi: 10.1016/j.ab.2007.12.014
Ribeiro, A. L., Nunes, M. P., Teixeira, M. M., and Rocha, M. O. (2012). Diagnosis and management of Chagas disease and cardiomyopathy. Nat. Rev. Cardiol. 9, 576–589. doi: 10.1038/nrcardio.2012.109
Sanchez-Montalva, A., Salvador, F., Rodriguez-Palomares, J., Sulleiro, E., Sao-Aviles, A., et al. (2016). Chagas cardiomyopathy: usefulness of EKG and echocardiogram in a non-endemic country. PLoS ONE 11:e0157597. doi: 10.1371/journal.pone.015759
Seneviratne, U., Nott, A., Bhat, V. B., Ravindra, K. C., Wishnok, J. S., Tsai, L. H., et al. (2016). S-nitrosation of proteins relevant to Alzheimer's disease during early stages of neurodegeneration. Proc. Natl. Acad. Sci. U.S.A. 113, 4152–4157. doi: 10.1073/pnas.1521318113
Sun, J., Morgan, M., Shen, R. F., Steenbergen, C., and Murphy, E. (2007). Preconditioning results in S-nitrosylation of proteins involved in regulation of mitochondrial energetics and calcium transport. Circ. Res. 101, 1155–1163. doi: 10.1161/CIRCRESAHA.107.155879
Tanowitz, H. B., Machado, F. S., Spray, D. C., Friedman, J. M., Weiss, O. S., Lora, J. N., et al. (2016a). Developments in the manangement of chagasic cardiomyopathy. Exp. Rev. Cardiovasc. Ther. 13, 1393–1409. doi: 10.1586/14779072.2015.1103648
Tanowitz, H. B., Wen, J. J., Machado, F. S., Desruisseaux, M. S., Robello, C., and Garg, N. J. (2016b). Trypanosoma cruzi and Chagas Disease: Innate Immunity, ROS, and Cardiovascular System. Waltham, MA: Academic Press Elsevier Inc. doi: 10.1016/B978-0-12-801078-5.00014-5
Thomas, S., and Bonchev, D. (2010). A survey of current software for network analysis in molecular biology. Hum. Genomics 4, 353–360. doi: 10.1186/1479-7364-4-5-353
Tyagarajan, K., Pretzer, E., and Wiktorowicz, J. E. (2003). Thiol-reactive dyes for fluorescence labeling of proteomic samples. Electrophoresis 24, 2348–2358. doi: 10.1002/elps.200305478
Viotti, R., Alarcon de Noya, B., Araujo-Jorge, T., Grijalva, M. J., Guhl, F., López, M. C., et al. (2014). Towards a paradigm shift in the treatment of chronic Chagas disease. Antimicrob. Agents Chemother. 58, 635–639. doi: 10.1128/AAC.01662-13
Wan, X., Wen, J. J., Koo, S. J., Liang, L. Y., and Garg, N. J. (2016). SIRT1-PGC1alpha-NFkappaB pathway of oxidative and inflammatory stress during Trypanosoma cruzi infection: benefits of SIRT1-targeted therapy in improving heart function in Chagas disease. PLoS Pathog. 12:e1005954. doi: 10.1371/journal.ppat.1005954
Wen, J.-J., and Garg, N. J. (2010). Mitochondrial complex III defects contribute to inefficient respiration and ATP synthesis in the myocardium of Trypanosoma cruzi-infected mice. Antioxid Redox Signal 12, 27–37. doi: 10.1089/ars.2008.2418
Wen, J.-J., Yachelini, P. C., Sembaj, A., Manzur, R. E., and Garg, N. J. (2006). Increased oxidative stress is correlated with mitochondrial dysfunction in chagasic patients. Free Radic. Biol. Med. 41, 270–276. doi: 10.1016/j.freeradbiomed.2006.04.009
Wen, J. J., and Garg, N. J. (2008). Mitochondrial generation of reactive oxygen species is enhanced at the Q(o) site of the complex III in the myocardium of Trypanosoma cruzi-infected mice: beneficial effects of an antioxidant. J. Bioenerg Biomembr. 40, 587–598. doi: 10.1007/s10863-008-9184-4
Wen, J. J., Zago, M. P., Nunez, S., Gupta, S., Nunez Burgos, F., and Garg, N. J. (2012). Serum proteomic signature of human chagasic patients for the identification of novel protein biomarkers of disease. Mol Cell Proteomics 11, 435–452. doi: 10.1074/mcp.M112.017640
Wiktorowicz, J. E., Stafford, S., Rea, H., Urvil, P., Soman, K., Kurosky, A., et al. (2011). Quantification of cysteinyl s-nitrosylation by fluorescence in unbiased proteomic studies. Biochemistry 50, 5601–5614. doi: 10.1021/bi200008b
Wiktorowicz, J. E., Stafford, S. J., and Garg, N. J. (2017). Protein cysteinyl-s-nitrosylation: analysis and quantification. Methods Enzymol. 586, 1–14. doi: 10.1016/bs.mie.2016.10.016
World Health Organization (2010). Chagas Disease: Control and Elimination. UNDP/World Bank/WHO. Available online at: http://apps.who.int/gb/ebwha/pdf_files/WHA63/A63_17-en.pdf
Keywords: infectious disease, Chagas cardiomyopathy, Trypanosoma cruzi, S-nitrosylation, peripheral blood mononuclear cells, 2DE, mass spectrometry
Citation: Zago MP, Wiktorowicz JE, Spratt H, Koo S-J, Barrientos N, Nuñez Burgos A, Nuñez Burgos J, Iñiguez F, Botelli V, Leon de la Fuente R and Garg NJ (2019) Potential Utility of Protein Targets of Cysteine-S-Nitrosylation in Identifying Clinical Disease Status in Human Chagas Disease. Front. Microbiol. 9:3320. doi: 10.3389/fmicb.2018.03320
Received: 09 July 2018; Accepted: 20 December 2018;
Published: 15 January 2019.
Edited by:
Thomas Dandekar, Universität Würzburg, GermanyReviewed by:
Claudia M. Calvet Alvarez, University of California, San Diego, United StatesIzabela Marques Dourado Bastos, Universidade de Brasília, Brazil;
Igor C. Almeida, The University of Texas at El Paso, United States
Copyright © 2019 Zago, Wiktorowicz, Spratt, Koo, Barrientos, Nuñez Burgos, Nuñez Burgos, Iñiguez, Botelli, Leon de la Fuente and Garg. This is an open-access article distributed under the terms of the Creative Commons Attribution License (CC BY). The use, distribution or reproduction in other forums is permitted, provided the original author(s) and the copyright owner(s) are credited and that the original publication in this journal is cited, in accordance with accepted academic practice. No use, distribution or reproduction is permitted which does not comply with these terms.
*Correspondence: Maria Paola Zago, bXB6YWdvQGNvbmljZXQuZ292LmFy
John E. Wiktorowicz, andpa3RvckBpbm5vdmFyZWdpLmNvbQ==
Nisha Jain Garg, bmlnYXJnQHV0bWIuZWR1