- 1Department of Basic Sciences, College of Veterinary Medicine, Mississippi State University, Starkville, MS, United States
- 2Amity Institute of Biotechnology, Amity University, Noida, India
- 3Department of Animal and Dairy Sciences, Mississippi State University, Starkville, MS, United States
Metagenomic analyses of microbial communities from aquatic sediments are relatively few, and there are no reported metagenomic studies on sediment from inland ponds used for aquaculture. Catfish ponds in the southeastern U.S. are eutrophic systems. They are fertilized to enhance algae growth and encourage natural food production, and catfish are fed with commercial feed from spring to fall. As result, catfish pond sediment (CPS) contains a very dense, diverse microbial community that has significant effects on the physiochemical parameters of pond dynamics. Here we conducted an in-depth metagenomic analysis of the taxonomic and metabolic capabilities of a catfish pond sediment microbiome from a southeastern U.S. aquaculture farm in Mississippi using Illumina next-generation sequencing. A total of 3.3 Gbp of sequence was obtained, 25,491,518 of which encoded predicted protein features. The pond sediment was dominated by Proteobacteria sequences, followed by Bacteroidetes, Firmicutes, Chloroflexi, and Actinobacteria. Enzyme pathways for methane metabolism/methanogenesis, denitrification, and sulfate reduction appeared nearly complete in the pond sediment metagenome profile. In particular, a large number of Deltaproteobacteria sequences and genes encoding anaerobic functional enzymes were found. This is the first study to characterize a catfish pond sediment microbiome, and it is expected to be useful for characterizing specific changes in microbial flora in response to production practices. It will also provide insight into the taxonomic diversity and metabolic capabilities of microbial communities in aquaculture. Furthermore, comparison with other environments (i.e., river and marine sediments) will reveal habitat-specific characteristics and adaptations caused by differences in nutrients, vegetation, and environmental stresses.
Introduction
Channel catfish production is the largest aquaculture industry in the United States with total sales of $380 million in 2017 (USDA, 2018). Aquaculture is one of the most rapidly growing food production sectors (Food Agriculture Organization of the United Nations and Fisheries and Aquaculture, 2014), but it faces several production and environmental challenges to maintain its economic viability (Schreier et al., 2010). Fish production ponds are rich in dissolved nutrients due to intensive feeding and fecal waste. Unconsumed feed, fish feces, and senescent phytoplankton are deposited into aquaculture sediments, which can enhance the microbial flora in the sediments and lead to anoxic conditions (Holmer and Kristensen, 1992). Medicated feed, such as Terramycin (oxytetracycline), Romet-30 (sulfadimethoxine-ormetoprim), and Aquaflor (florfenicol), as well as fertilizers may also impact pond sediment microflora. In turn, changes in sediment microflora impact fish health in aquaculture systems.
Fish excrete nitrogen in the form of ammonia, which is toxic to fish; nitrification by chemolithoautotrophic bacteria in pond sediments is an important process that prevents toxic buildup. The microbes contributing to nitrification consist of two functional groups: the ammonia-oxidizing bacteria (AOB) and ammonia-oxidizing archaea (AOA) that convert toxic ammonia to nitrite, and the nitrite-oxidizing bacteria (NOB) that oxidize nitrite to the less toxic nitrate. AOB include the genera Nitrosomonas, Nitrosococcus, and Nitrosospira (Kowalchuk and Stephen, 2001). AOA include Nitrosopumilus from marine water (Konneke et al., 2005). The NOB group includes genera Nitrobacter, Nitrospira, and Nitrospina (Wang et al., 2014). Pond sediments also have potential to serve as a reservoir for fish pathogens. Therefore, it is important to understand microbial flora in aquaculture pond sediments because of its impact on the pond ecosystem, especially in nutrient cycling, concentrations of organic and inorganic nutrients and toxins, and effects on fish health.
Metagenomic analysis allows assessment of mixed environmental microbial communities by directly sequencing DNA from environmental samples (Dinsdale et al., 2008). This approach provides a picture of the diversity and microbiome structure present in the environment (Simon and Daniel, 2009), and it enables studies to understand how microbial diversity is modulated in response to environmental or anthropogenic impacts (Larsen et al., 2012). Thus, it is particularly appropriate for assessing the taxonomic and functional microbial diversity in a pond sediment biome and monitoring community changes over space and time (Nogales et al., 2011).
Previous culture-independent studies investigating sediment microbial phylogenetic structure showed that microbial communities are indicators of both the physicochemical status of freshwater sediments (Logue et al., 2008; Gibbons et al., 2014) and ecological degradation (Feris et al., 2009). A few metagenomes have been published from deep-sea sediment (Kimes et al., 2013), mangrove (Andreote et al., 2012), and river systems (Staley et al., 2013), and recent studies have examined the response of fish gut-associated microbial communities from aquaculture systems in response to lifestyle and dietary preference (Wu et al., 2010; Xing et al., 2013). Signatures of bacterial composition were found in shrimp farming (Sousa et al., 2006), and pyrosequencing was used to explore bacterial diversity and detect potential fish pathogens during production of Scophthalmus maximus (turbot) and Solea senegalensis (sole) (Martins et al., 2013).
In pond sediments, the microbiome is critical for maintenance of homeostasis conditions, including toxin removal and cycling of carbon, nitrogen, and phosphorus (Brock, 1970; Leung et al., 1994). In particular, nitrogen is very important in aquaculture as a nutrient and potential toxicant, and it is an essential requirement for phytoplankton and bacterial growth in anaerobic conditions. In the current study, we present a description of the microbiome found in sediment from a catfish research pond maintained under commercial production conditions. Our results are culture-independent and based on metagenomic sequence of total DNA extracted directly from the pond sediment and analyzed by Illumina sequencing. We describe the microbial taxa present in catfish pond sediment and the potential metabolic processes that appear to be occurring in this ecosystem affecting carbon, nitrogen, and sulfur cycling. This analysis also provided a fundamental baseline profile of the catfish pond sediment microbiome for comparison with sediments from other environments.
Materials and Methods
Sediment Sampling, DNA Extraction, and Sequencing
Sediment sampling was performed in October 2012 (water temperature approximately 20°C) in sediments from a 0.8-hectare aquaculture research pond stocked with approximately 6,000–8,000 catfish (Ictalurus punctatus) with average size of 0.5 kg at the Delta Research and Extension Center, Stoneville, MS, United States. The pond was maintained at typical stocking and feeding parameters used for catfish aquaculture. Three samples were collected at 10 am using a modification of a previously published method (Schneegurt et al., 2003). In brief, ∼500 g per sample of pond sediment was collected at 5–10 cm of sediment depth ∼8 m from the pond bank in a water depth of ∼1.2 m using a sterile spatula and immediately transferred to sterile 50 ml tubes kept on ice.
Genomic DNA was extracted from each sediment sample separately using MoBio PowerSoil DNA Isolation Kit (Mo Bio Laboratories, Carlsbad, CA, United States) according to manufacturer’s protocol with 5.0 g of sediment per extraction. A NanoDrop (Thermo Scientific, Wilmington, DE, United States) spectrometer was used to quantify extracted DNA and to assess DNA quality. Illumina library preparation and sequencing followed manufacturer’s protocols (Illumina, San Diego, CA, United States). Briefly, genomic DNA was sheared by sonication and separated by electrophoresis on a 1% agarose gel. Gel slices corresponding to ∼300 and ∼500 bp were excised and purified using QIAquick Gel Extraction Kit (Qiagen). The blunt-ended DNA fragments were A-tailed using the Quick Blunting Kit (New England BioLabs) and purified. Sheared DNA was ligated to sequence adapters. All ligated libraries were enriched using standard PCR (11–12 cycles) with Illumina paired end primers before library quantification and validation. One microgram DNA was used in library construction. The amplified libraries were pooled in an equimolar ratio and sequenced using an Illumina HiSeq 2000 (Illumina, San Diego, CA, United States) using paired end reads of 100 bases. Raw reads containing three or more “N” bases or contaminated by adapter (>15 bp overlap) were removed by Trimmomatic (Bolger et al., 2014), and the filtered clean reads were used for metagenomic analysis.
Taxonomic Distribution and Functional Analysis of Metagenomic Sequence
The taxonomic analysis was performed using BLASTX against the SEED and Pfam databases (Altschul et al., 1997) on the MG-RAST server1 using a cut-off E-value of 1e-5, minimum identity of 60%, and a minimum alignment length of 15 bp (Meyer et al., 2008). BLASTX was also conducted using MetaGenome Analyzer software (MEGAN v5) with the lowest common ancestor (LCA) algorithm used to visualize results (Huson et al., 2007). Using BLASTX and BLASTN, reads were compared against the NR and NT NCBI databases. Analysis was performed comparing distinct hierarchical levels, and a directed homogeneity test was used to identify significant differences in sample comparisons. Multiple testing correction analysis was not applied, and all unassigned reads were ignored.
Statistical analysis was performed using results from the MG-RAST annotation system, and results were visualized using Statistical Analyses of Metagenomic Profiles (STAMP) (Parks and Beiko, 2010) to detect biologically relevant differences in the relative proportion of sequences. Paired metagenomic samples were used for the analysis, and statistical significance of the differences between samples was assessed by the Two-sided Fisher’s Exact test. Story’s false discovery rate (FDR) was used for multiple test correction as recommended by STAMP. Results with q-value (<0.05) were considered significant, and unclassified reads were removed from the analysis.
Functional classification was conducted using BLASTX (cut-off E-value of 1e-5) against COGs (Tatusov et al., 2001), which was downloaded from hierarchical classification in MG-RAST server using NCBI database. BLASTX and subsystem analysis were used against the SEED-NR database in MG-RAST for functional sequence annotation with the same parameters as a taxonomic distribution. A functional analysis using the SEED (Overbeek et al., 2005) and KEGG (Kanehisa et al., 2004) databases was conducted using MG-RAST sever. Each sequence was associated with its SEED functional role using the best BLAST score to protein sequences without known functional roles. A similar procedure was used to match each sequence to a KEGG orthology (KO) accession number. Results were matched with each protein’s RefSeq database, and relative abundance was used to identify enzymes in important metabolic pathways. Matches with alignment scores higher than 80 were retained.
To the best of our knowledge, there is no previous information about structure and function of bacterial communities in aquaculture pond sediments. Therefore, the microbial community from aquaculture sediment was compared to freshwater sediment from the Tongue River in Southeastern Montana (MG-RAST ID 4481977.3) (Gibbons et al., 2014) and deep-sea sediment of the Gulf of Mexico (MG-RAST ID 4465489.3) (Kimes et al., 2013). A classification was used to determine the sample that most closely clustered to the taxonomic composition or metabolic potential of the sediment metagenome (E-value of 1e-5, the minimum identity of 60%, and a minimum alignment length of 15 bp).
Results and Discussion
Sequence Generation
Whole community microbial DNA from catfish pond sediment (CPS) was sequenced, making this study the first metagenomic survey of a catfish aquaculture environment (MG-RAST ID 4583113.3). Of the 29,278,265 sequences (totaling 2,927,826,500 bps) that passed quality control, 3,690,631 sequences (11.2% of total) were identified as artificial duplicate reads (ADRs), which are nearly identical sequences that result from sequencing two or more copies of the exact DNA fragment (Niu et al., 2010). Of the sequences without rRNA genes, 26,127,903 contained predicted protein features, 4,424,138 (16.9%) of which were assigned an annotation using at least one protein database, and 21,703,765 (82.9% of features) contain predicted proteins with unknown function. A total of 2,855,527 sequences (64.5% of annotated proteins) were assigned to functional categories (Supplementary Table S1). At the domain level, Bacteria (96.8%) dominated, while the Archaea (1.2%) and the Eukaryota (1.5%) contributed substantially less to the CPS community.
Taxonomic Profiles
The numbers of sequences affiliated with each bacterial taxon in CPS were similar with other sediment samples, with a dominance of Proteobacteria (46.0%) and an abundance of Bacteroidetes (15.9%), Firmicutes (8.6%), Chlorflexi (5.1%), and Actinobacteria (5.0%) (Table 1). Minor groups represented at the phylum level included Cyanobacteria, Verrucomicrobia, Planctomycetes, Chlorobi, and Acidobacteria. The four most abundant classes of bacteria were Deltaproteobacteria, Bacteroidia, Clostridia, and Actinobacteria according to MG-RAST analysis (Table 1).
The Proteobacteria associated with each sample were examined more closely to evaluate the potential of both aerobic and anaerobic biodegradation. In aquaculture ponds and lakes, the top sediment layer down to a few millimeters is typically aerobic, but below this depth sediment is anaerobic (Boyd and Tucker, 1998). The high occurrence of Deltaproteobacteria in CPS, which is not commonly observed in metagenomes from water or sediment samples (Figure 1), might be related to the catfish habitat, where eutrophic and anaerobic conditions could drive selection for specific microbial groups such as sulfate associated bacteria (Harrison et al., 2009). The Deltaproteobacteria in our sample was mostly comprised of a branch of strictly anaerobic genera containing many of the known sulfate- and sulfur-reducing bacteria including Desulfovibrio, Desulfobacter, Desulfococcus, Desulfonema, and Desulfuromonas spp. High organic loads and anaerobic conditions in pond sediments yield ideal conditions for sulfate reduction and sulfide production (Boyd and Tucker, 1998). Many of the Deltaproteobacteria were also species involved in methane transformation, which was paralleled by the presence of other bacteria with anaerobic physiology such as ferric iron-reducing Geobacter spp. (Supplementary Table S2).
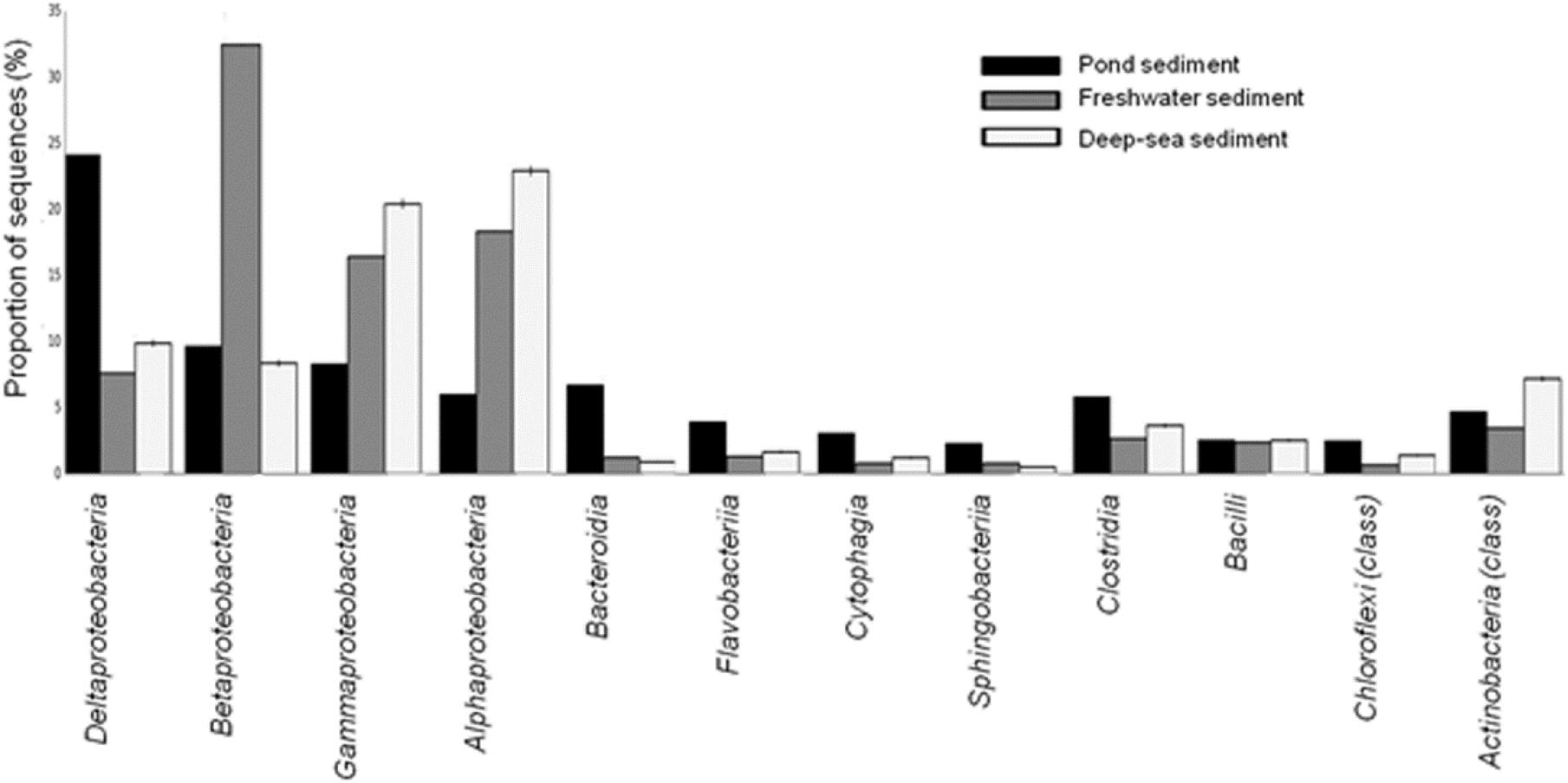
FIGURE 1. Taxonomic distribution of bacterial classes from the CPS and other aquatic sediments from freshwater and deep-sea environments.
Deltaproteobacteria, in particular, are capable of fumarate addition to both aromatic and aliphatic hydrocarbons, hence activating the anaerobic hydrocarbon biodegradation pathway. The increases in Deltaproteobacteria correlated with an increase in other protein-coding genes involved in anaerobic degradation of hydrocarbons, such as benzylsuccinate synthase (BSS), acetyl-CoA acetyltransferase, and benzoyl-CoA reductase (Kimes et al., 2013). Anthropogenic hydrocarbon loading from catfish feed may enrich for microbial species with anaerobic hydrocarbon degradation capabilities. Other types of Proteobacteria, including Beta-, Gamma-, and Alphaproteobacteria had relatively lower representation compared with microbial populations from other aquatic sediments from river or deep sea environments (Figure 1). Moreover, the CPS had a higher prevalence of Epsilonproteobacteria than the river and deep-sea sediments. Epsilonproteobacteria are prevalent in the digestive tracts of animals and serve as symbionts or pathogens; their energy metabolism involves oxidizing reduced sulfur, formate, or hydrogen coupled with the reduction of nitrate or oxygen (Takai et al., 2005).
The phylum Bacteroidetes is very diverse and includes Cytophaga, Flexibacter, and Bacteroides (Woese, 1987; Woese et al., 1990). The Bacteroidetes phylum is comprised of four classes: Bacteroidia, Flavobacteria, Cytophage, and Sphingobacteria, which include around 7,000 different species (Bergey et al., 2011). The Bacteroidetes phylum in CPS included the Flavobacteria, which has many aquatic species (Table 1), and it also contained opportunistic human pathogens (Bernardet and Nakagawa, 2006), including the genera Elizabethkingia, Weeksella and Capnocytophaga (Kim et al., 2005; Leadbetter, 2006) (Supplementary Table S2). F. psychrophilum, F. columnare, and F. branchiophilum are some Bacteroidetes species that have economic impacts on freshwater fish, causing infections that can have severe effects on farmed and wild fish (Hawke and Thune, 1992; Loch and Faisal, 2015). Flavobacterium infections were first reported a century ago in aquaria (Davis, 1922).
Previously, Firmicutes was observed as a dominant phylum in the digestive tract of many marine and freshwater fish species (Austin, 2006; Wu et al., 2012). In the present study, Firmicutes was found to be prevalent in CPS. In particular, Clostridium sp. was the most abundant genus within Firmicutes and represented 5.6% of the identified sequences (Table 1), making it more abundant in CPS compared to marine and river sediments (Figure 1). Clostridium sp. are commonly found in human and animal guts and can form endospores, allowing survival under unfavorable environments (Davies et al., 1995; Mueller-Spitz et al., 2010). Clostridium sp. contribute to hydrolytic enzyme production, suggesting a possible role in degradation of organic matter. In eutrophic marine cage aquaculture sediments, metabolism is dominated by anaerobic decomposition (Holmer and Kristensen, 1992); therefore, enrichment of Clostridium sp. may be a good indicator of the impact of organic matter in aquatic sediments. Interestingly, some lactic acid bacteria were detected in the Firmicutes phylum in CPS, including the genera Lactococcus, Streptococcus, and Enterococcus (Supplementary Table S2). Lactic acid bacteria are generally considered to be non-pathogenic (Ringø and Gatesoupe, 1998). However, some species including Lactococcus garvieae, Streptococcus shiloi, and Streptococcus difficile were reported as fish pathogens (Eldar et al., 1994, 1996).
The highest proportion of archaeal reads within the CPS metagenome was Euryarchaeota (87.6%), which was composed of several classes: Archaeoglobi (1,159 reads), Halobacteria (3,001 reads), Methanobacteria (1,956 reads), Methanococci (2,334 reads), Methanomicrobia (12,467 reads), and Thermococci (1,593 reads) (Figure 2). Methanosarcina was the most abundant genus and accounted for 31% of the total archaeal sequences. This genus includes many methanogens involved in both acetotrophic and hydrogenotrophic methanogenesis, which is a type of anaerobic respiration that generates methane and is considered the terminal step in organic decomposition. Most Methanosarcina are non-motile and mesophilic, and they are unique among archaeal bacteria in that most can utilize multiple substrates as electron acceptors, including methanol (Kandler and Hippe, 1977). Thus, Methanosarcina is among the most adaptable and flexible of the methanogens (Maeder et al., 2006).
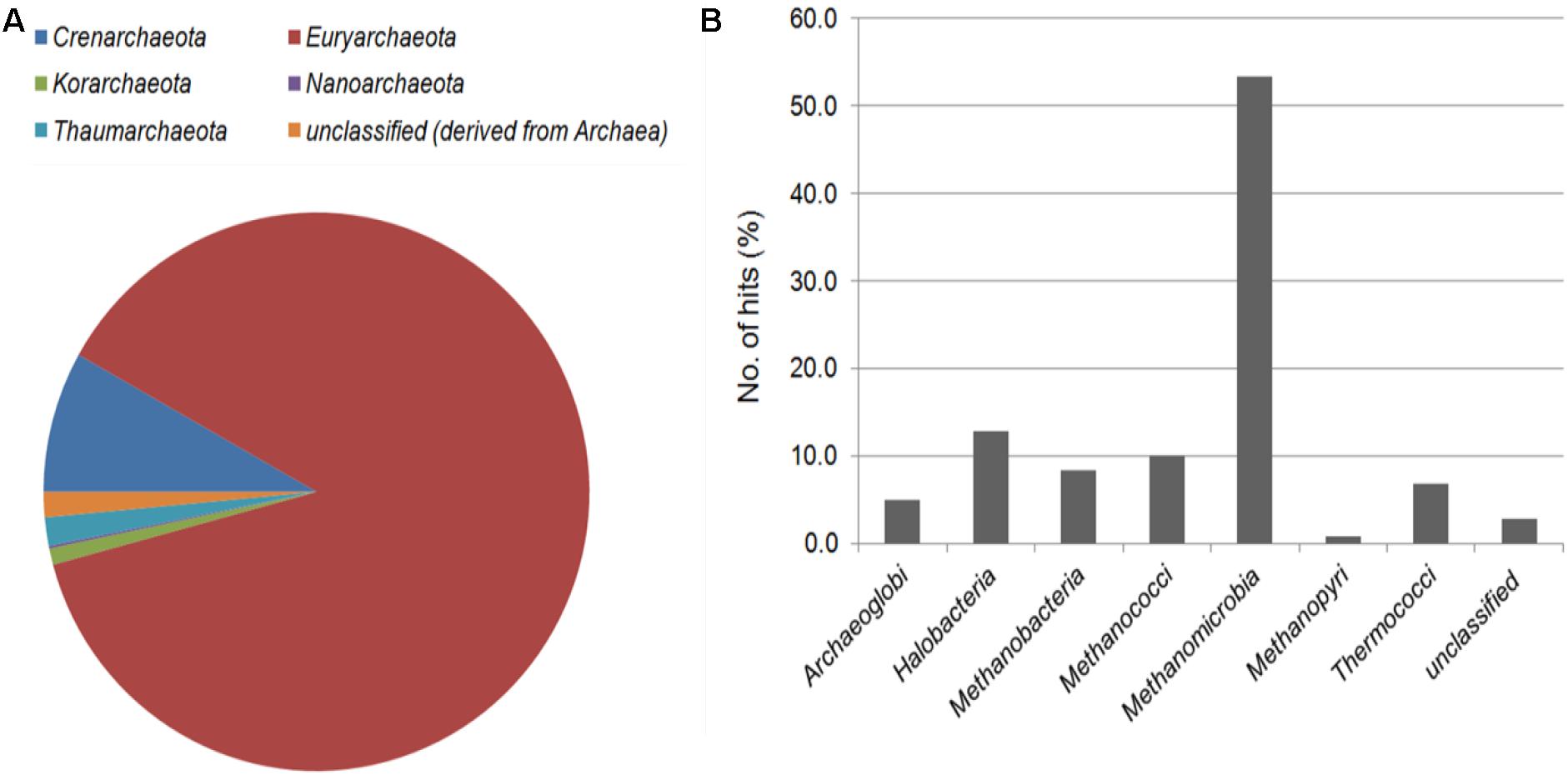
FIGURE 2. Taxonomic affiliation of archaeal reads in the CPS metagenome at the phylum (A) and class (B) level.
The eukaryotic sequences represented 23 phyla from the Animalia, Fungi, Plantae, and Protista. The Animalia phylum Chordata (20.76 – 28.52%) showed predominant abundance in the three sediments, followed by Athropoda, Ascomycota, and Steptophyta. Chordata was the most abundant in deep-sea sediment, but the Arthropoda was lower compared to CPS and river sediment. The Bacillariophyta, Cnidaria, and Echinodermata phyla had greater abundance in CPS compared to river sediment, and Apicomplexa was in greater abundance in river sediment (Figure 3).
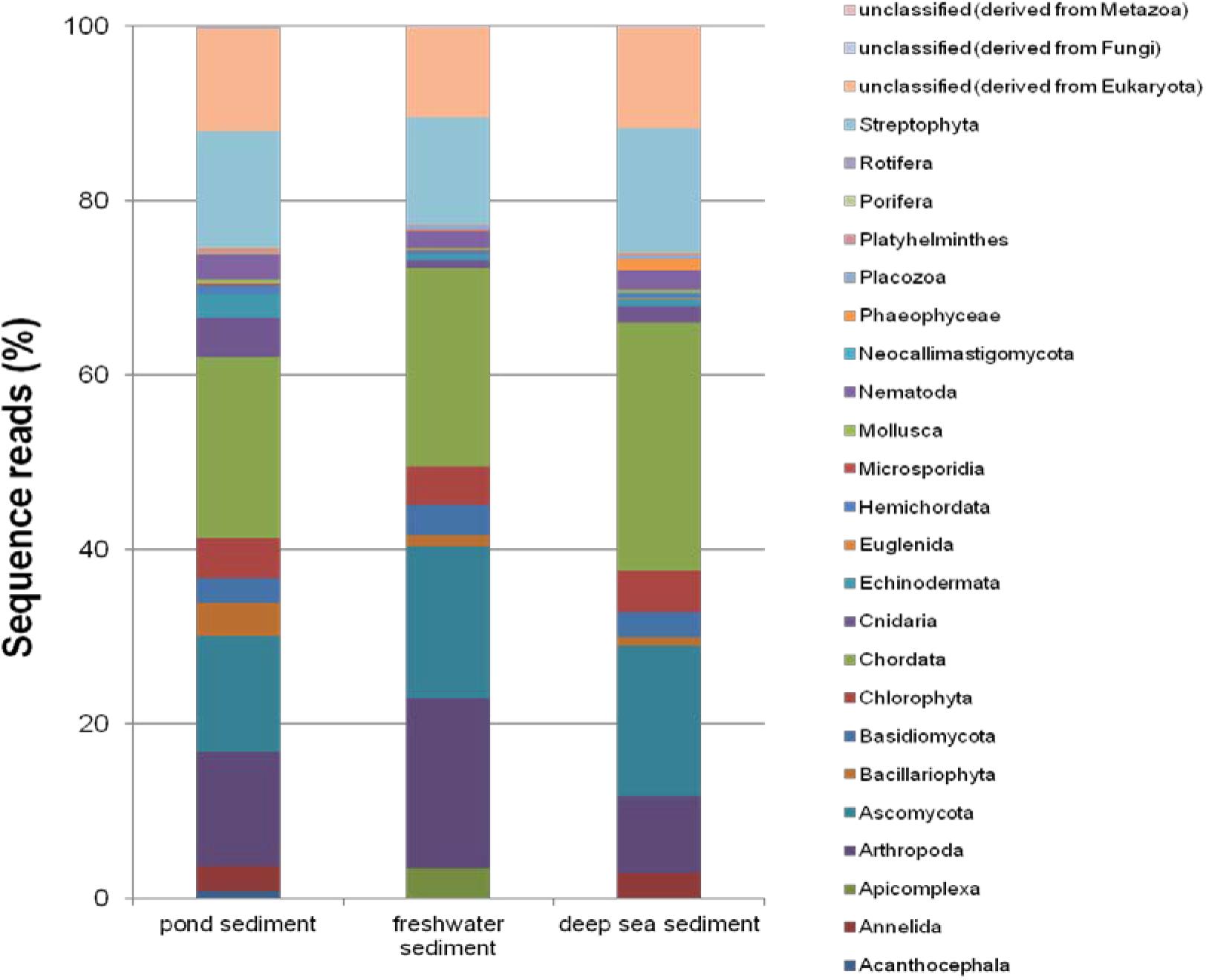
FIGURE 3. Comparison of the relative abundance of eukaryotic reads within the 23 represented phyla in CPS and two previously published marine sediments.
Functional Categories
Environmental DNA from CPS had matches in 24 COG and 28 KEGG functional categories, respectively (Supplementary Table S3). The dominant COG functions were prokaryotic, with high abundance of sequence reads in energy production and conversion as well as amino acid transport and metabolism. In particular, CPS had a high number of sequences in signal transduction mechanisms, carbohydrate transport and metabolism, inorganic ion transport and metabolism, and general function prediction. A lower percent of reads was found for functions associated with eukaryotic organisms (RNA procession and modification, chromatin structure and dynamics, cell motility, and cytoskeleton and extracellular structures) (Figure 4A). The most abundant KEGG functional categories were carbohydrate metabolism, clustering-based subsystems, miscellaneous, amino acids and derivatives, and protein metabolism (Figure 4B).
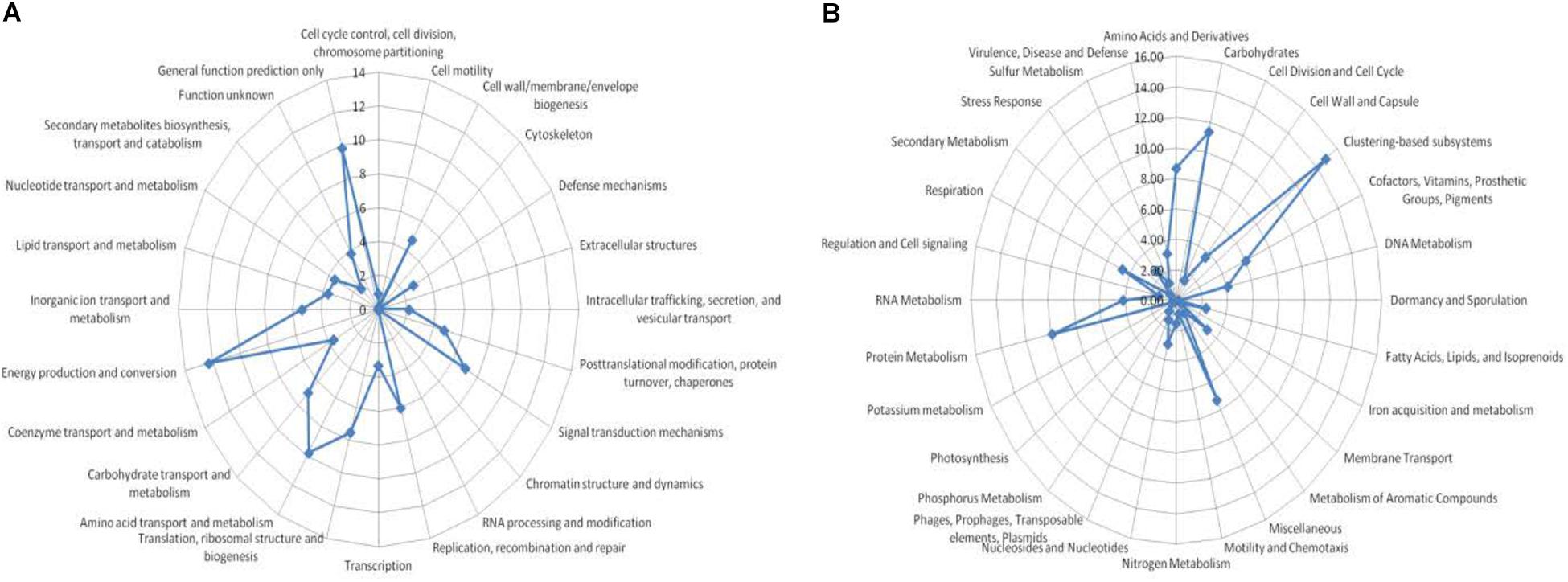
FIGURE 4. Functional assignment of metagenome sequences. (A) BLASTX analysis against the COGs database: percent was assigned to specific COG functional categories, and (B) BLASTX analysis against GenBank conducted using MG-RAST; percent abundance was assigned to specific KEGG identifiers.
The metabolic potential of the CPS metagenome was compared with two freshwater and deep-sea sediment metagenomes publicly available on the MG-RAST server. A heat map showed that the CPS metagenome is similar to the deep-sea sediment metagenome. CPS had more sequences within phosphorous metabolism, protein metabolism, and membrane transport than the other aquatic sediments (Figure 5). Phosphorus is relatively abundant in catfish ponds because producers often fertilize ponds to encourage algal blooms, and protein is also relatively abundant due to application of commercial feeds.
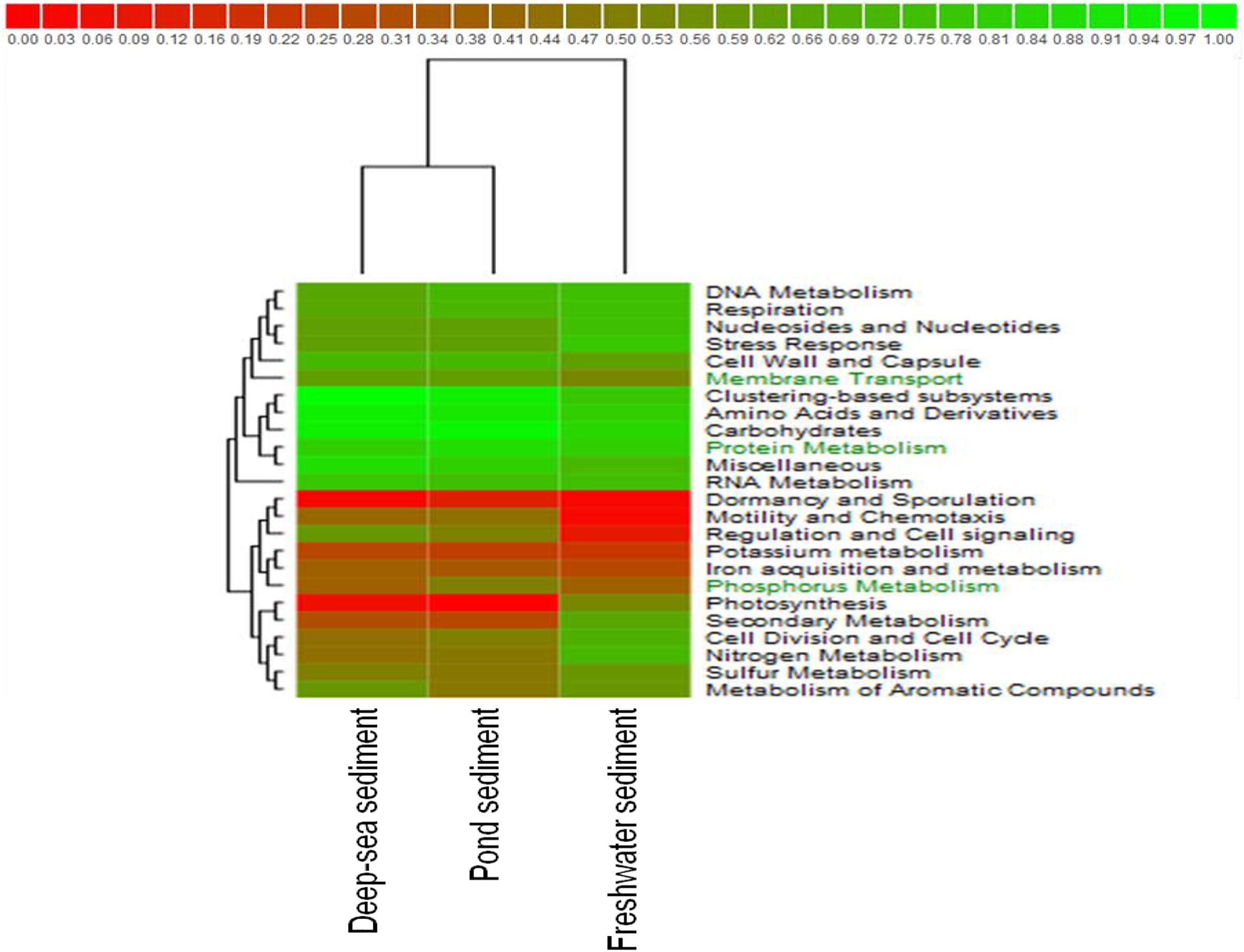
FIGURE 5. Hierarchical clustering combined with heat mapping based on functional subsystem classifications for CPS, freshwater sediment of the Tongue River in Southeastern Montana, and deep-sea sediment of the Gulf of Mexico.
Based on our taxonomic analysis, we expected to detect genes encoding enzymes involved in methanogenesis, which is considered the final step in decomposition. As expected, we detected methanogenesis genes encoding an F420-dependent N(5),N(10)-methylenetetrahydromethanopterin reductase (EC 1.5.99.11), N(5),N(10)-methenyltetrahydromethanopterin cyclohydrolase (EC 3.5.4.27), formylmethanofuran dehydrogenase (EC1.2.99.5), CoB–CoM heterodisulfide reductase (EC1.8.98.1), coenzyme F420 hydrogenase (EC 1.12.98.1), N5-methyltetrahydromethanopterin (EC 2.1.1.86), and the enzyme responsible for the last step of methanogenesis, methyl coenzyme M reductase (EC 2.8.4.1) (Table 2).
The transformation of methane and methanol into formaldehyde and then formate in CPS was suggested by metabolic reconstruction, mainly from the activity of particulate methane monooxygenase (EC 1.14.13.25), methanol dehydrogenase (EC 1.1.99.8), and S-formylglutathione hydrolase (EC 3.1.2.12). We also detected genes encoding enzymes involved in formaldehyde fixation, including genes for enzymes that incorporate methane into organic compounds via the serine pathway or the ribulose monophosphate pathway, indicating that the CPS microbiome is able to metabolize methane as their source of carbon and energy to survive. Compared to the metagenome of freshwater sediment from the Tongue River, both CPS and the freshwater sediment microbiomes encode processes for formaldehyde fixation; the distinct sediments differ only in the particular pathways used. In CPS, methane metabolism is encoded by the Gammaproteobacteria using the ribulose monophosphate pathway to assimilate carbon; in addition, part of the Alphaproteobacteria utilized the serine pathway of carbon assimilation. Oxidation of formate yields carbon dioxide, and a high abundance of genes encoding proteins involved in the conversion of carbon dioxide into carbon monoxide and later into acetyl-CoA was detected (Figure 6A). Formate also contributes to oxidative stress response; oxidase stress catalase (EC 1.11.1.6) and peroxidase (EC 1.11.1.7) were detected in CPS, both of which are antioxidant enzymes that contribute to limiting oxidative damage by reactive oxgen species (ROS) such as H2O2 (Table 2).
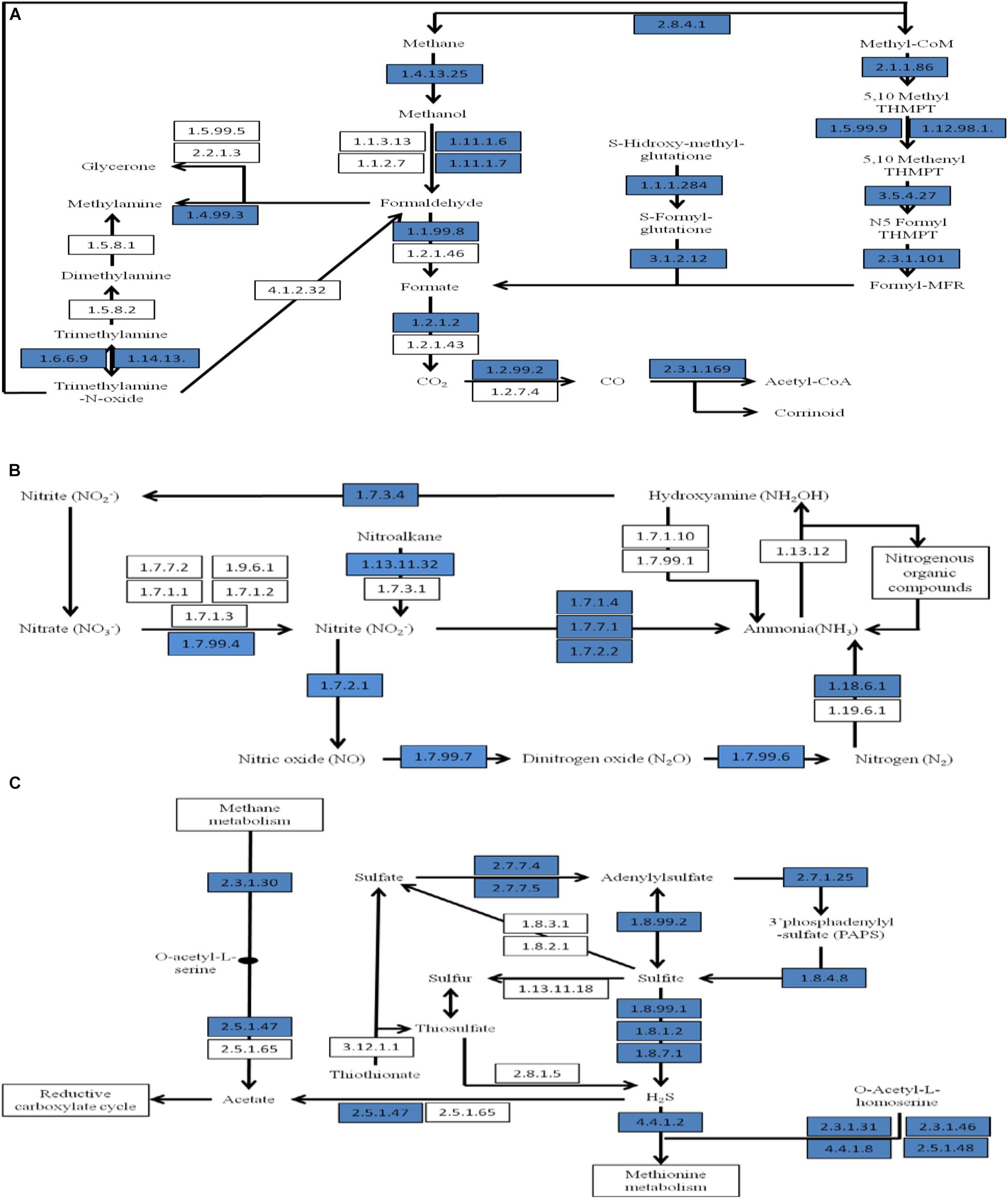
FIGURE 6. Part of a SEED-based functional analysis of the CPS metagenome. (A) Carbon fixation and methane metabolism; (B) nitrogen metabolism; and (C) sulfur metabolism. Blue boxes are proteins that were represented in CPS.
Analysis of nitrogen metabolism revealed genes encoding nitrogen immobilization and mineralization in CPS (Figure 6B). Sequences encoding nitrogen fixation were detected, including atmospheric nitrogen fixation using a nitrogenase (EC 1.18.6.1) that converts nitrogen gas to ammonia. A high abundance of nitrification species was detected in the metagenome (Supplementary Table S3) such as Nitrosomonas spp., Nitrobacter spp., and Nitrococcus spp. These species oxidize ammonia to nitrate, preventing toxic buildup of ammonia that can affect fish health. Genes encoding nitrification such as hydroxylamine oxidoreductase (EC 1.7.3.4) for oxidation of hydroxylamine were found. Genes encoding denitrification enzymes, including nitrate reductase (EC 1.7.99.4), nitrite reductase (EC 1.7.1.4, EC 1.7.7.1 and 1.7.2.1), cytochrome c552 precursor (EC 1.7.2.2), nitric-oxide reductase (EC 1.7.99.7) and nitrous-oxide reductase (EC 1.7.99.6) were observed (Table 2). Denitrification is the dissimilatory reduction of nitrate into nitric oxide, dinitrogen oxide, and nitrogen. The balance among these pathways is affected greatly by environmental conditions including oxygenation, temperature, nitrate concentration, and organic matter content in the sediment (Saunders and Kalff, 2001).
The predominant type of sulfur metabolism encoded in the CPS generates the reductive form of sulfite and hydrogen sulfide (H2S) (Figure 6C). Most of genes observed were involved in conversion of sulfate into adenylylsulfate and to sulfite and H2S, including sulfate adenylyltransferase (EC 2.7.7.4), adenylylsulfate kinase (EC 2.7.1.25), phosphoadenylyl sulfate reductase (EC 1.8.4.8), adenylylsulfate reductase (EC 1.8.99.2), and sulfite reductase (EC 1.8.99.1, 1.8.1.2 and 1.8.7.1) (Table 2). Enzymes mediating reduction of sulfate and adenylylsulfate into H2S dominated sulfur metabolism in CPS (Figure 6C). Generated H2S can influence the reductive carboxylate cycle (CO2 assimilation) and might be released by volatilization, producing the typical smell of mangrove swamps (Andreote et al., 2012). Sulfate-reducing bacteria obtain energy by oxidizing organic matter or hydrogen using sulfate (or other sulfur molecules) as electron acceptors, yielding H2S. They are prevalent in environments such as swamps and standing waters that have low oxygen. Sulfur-reducing bacteria and some archaea are similar, but they use elemental sulfur as an electron acceptor, and they also produce H2S. During catabolism of organic matter, hydrogen sulfide is also released by other anaerobic bacteria when sulfur-containing amino acids are digested. In CPS, Deltaproteobacteria was the most abundant, possibly indicating the importance of sulfate reduction in this environment (Wrighton et al., 2014).
Overall, the metabolism of carbon, nitrogen, and sulfur are interlinked within the microbial population, and this is particularly true for the metabolism of sulfur and carbon. The abundance of organic matter in the anaerobic environment of CPS yields an optimal environment for several anaerobic bacteria such as sulfate-reducing bacteria and methanogens (Dar et al., 2008). These groups share similar environmental niches, and their relative abundance is controlled by substrate availability (Oremland and Polcin, 1982). Simple substrates are important for methanogens, while sulfate-reducing bacteria are capable of degrading more complex substrates, including long chain and aromatic hydrocarbons (Muyzer and Stams, 2008).
Genes encoding resistance to antibiotics and toxic compounds (RATC) represents a subset of virulence genes that made up 3.45% of the classified metagenome in CPS. By comparison, genes encoding RATC proteins generally make up ∼2–2.24% of the classified metagenome in other aquatic ecosystems. Compared to other aquatic sediments, the CPS metagenome encoded a higher proportion of proteins in copper homeostasis, cobalt-zinc-cadmium resistance, multidrug resistance efflux pumps, and resistance to fluoroquinolones; however, the CPS metagenome encoded a lower proportion of arsenic resistance, beta-lactamase, erythromycin resistance, methicillin resistance, and resistance to vancomycin (Table 3). In particular, genes encoding cobalt-zinc-cadmium energy-dependent efflux pump had the highest proportion in the RATC category in CPS. By contrast, the fish gut microbiome encodes a much higher proportion of mercury resistance, mercuric reductase, and cobalt-zinc-cadmium genes than other metagenomes (Durso et al., 2012). Fluoroquinolone is of particular interest regarding the use of antibiotics in animal agriculture. Two enzymes are the principal targets for the antibacterial activity of fluoroquinolone: DNA gyrase and topoisomerase IV, which introduce negative supercoiling and prevent the accumulation of excess supercoiling (Collignon and Angulo, 2006). The DNA gyrase subunit B gene and topoisomerase IV subunit A were most frequently associated with Clostridia, Actinobacteria, Bacteroidetes and with Proteobacteria in the CPS metagenome, but whether they carry mutations associated with resistance is not currently known. Beta-lactamase genes were most frequently associated with Alpha- and Gammaproteobacteria, which encode resistance to beta-lactam antibiotics (penicillins and cephalosporins). The true risk to public health from antimicrobial use and subsequent resistance in aquaculture is speculative. However, the presence of antibiotic resistance genes and elements in pond sediments is a threat to public health if the resistance genes are transferrable to clinically significant pathogens.
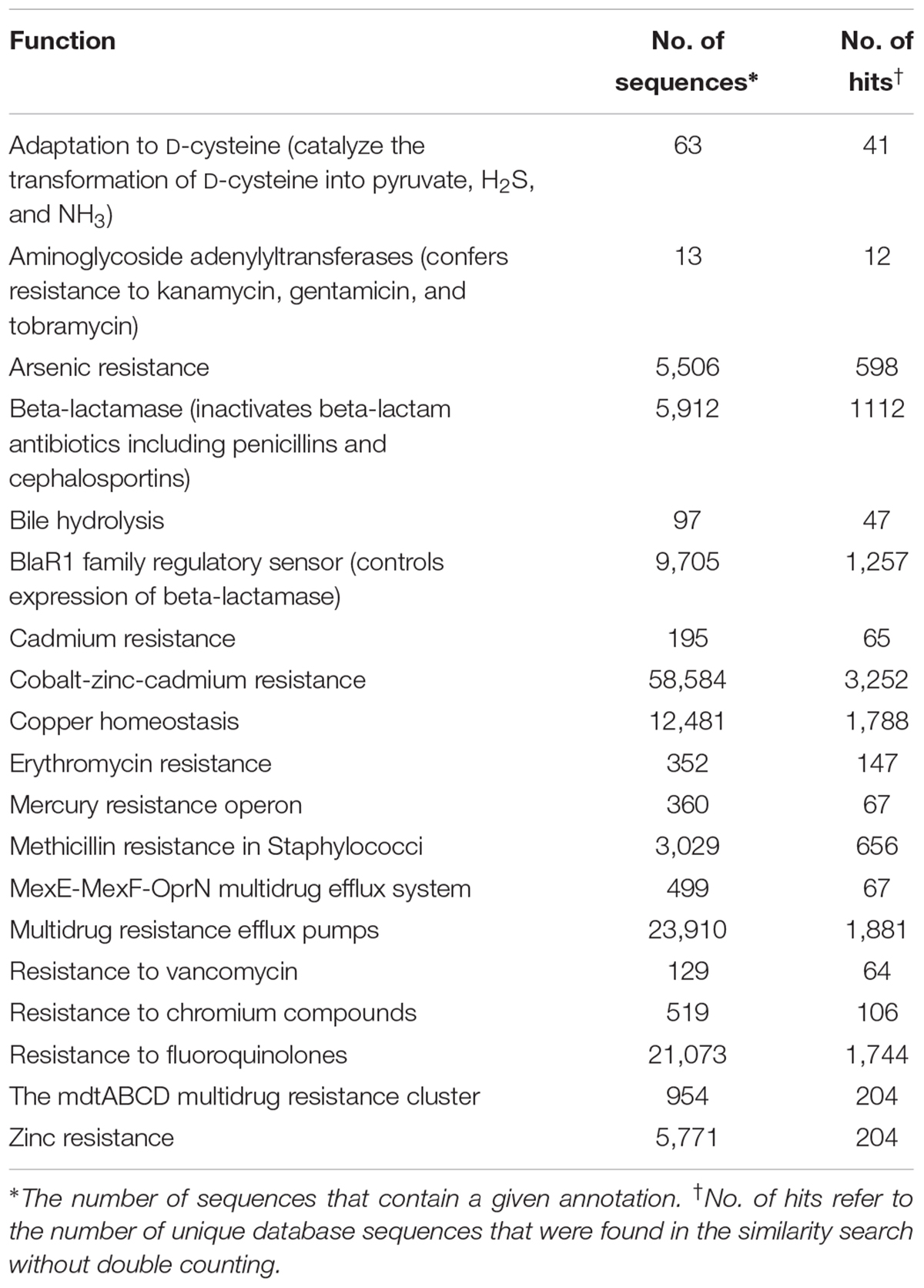
TABLE 3. Proteins in the CPS metagenome in the resistance to antibiotics and toxic compounds (RATC) category.
Of these RATC categories, beta-lactamase resistance, multidrug resistance efflux pumps, fluoroquinolone resistance, cobalt/zinc/cadmium resistance, and acriflavine resistance genes are present in other metagenomes such as lake sediment, soil, feces, and marine environment (Durso et al., 2012). This broad distribution across agricultural, environmental, and human-associated samples indicates that these mechanisms are generally distributed and suggests they are functionally important in diverse habitats.
Conclusion
Catfish pond sediment is a highly eutrophic, nutrient-rich, and diverse ecosystem that has significant effects on the physiochemical parameters of pond dynamics. This study is a pacesetting metagenomics analysis using Illumina sequencing for sediment in an intensive aquaculture system, and it revealed significant coupling between phylogeny and functional potential. The community structure suggests that the distribution of particular taxa is driven by their metabolic capabilities in response to the environment. For example, Deltaproteobacteria was the most abundant class, which is unique in the CPS metagenome compared to other aquatic sediments, and it demonstrates capability of anaerobic hydrocarbon metabolism. Functionally, our analysis revealed that the metagenome likely has significant impacts on nitrogen, phosphorus, and sulfur dynamics in catfish production ponds.
Further work to assess pond sediment could yield a complete description of the potential metabolic pathways in the CPS metagenome. The current work establishes a critical baseline for the CPS metagenome for comparison with other distinct environments. Also, future work could assess the effects of environmental changes (for example, feeding changes or antimicrobial use) in catfish production ponds on the sediment metagenome.
Author Contributions
ML and MM supervised the study. ML, MM, and AK designed the experiments. HA, SN, SP, DP, and MM performed the experiments. SN, SP, and ML analyzed and interpreted the data. All authors wrote and approved the manuscript.
Funding
This project was supported by the U.S. Department of Agriculture-Catfish Health Research Initiative (MIS-371660) and the Mississippi State University College of Veterinary Medicine.
Conflict of Interest Statement
The authors declare that the research was conducted in the absence of any commercial or financial relationships that could be construed as a potential conflict of interest.
Acknowledgments
We thank Dr. Zhao Nan (Alan) for assistance in data analysis. We also thank Amanda Cooksey for help in library preparation and sequencing.
Supplementary Material
The Supplementary Material for this article can be found online at: https://www.frontiersin.org/articles/10.3389/fmicb.2018.02855/full#supplementary-material
TABLE S1 | Sequence data from the CPS metagenome.
TABLE S2 | Functional profiles (COG and KEGG) for genes identified in the CPS metagenome.
TABLE S3 | Relative abundances of bacteria by systematic classifications within the CPS metagenome.
Footnotes
References
Altschul, S. F., Madden, T. L., Schaffer, A. A., Zhang, J., Zhang, Z., Miller, W., et al. (1997). Gapped BLAST and PSI-BLAST: a new generation of protein database search programs. Nucleic Acids Res. 25, 3389–3402. doi: 10.1093/nar/25.17.3389
Andreote, F. D., Jimenez, D. J., Chaves, D., Dias, A. C., Luvizotto, D. M., Dini-Andreote, F., et al. (2012). The microbiome of Brazilian mangrove sediments as revealed by metagenomics. PLoS One 7:e38600. doi: 10.1371/journal.pone.0038600
Austin, B. (2006). The bacterial microflora of fish, revised. ScientificWorldJournal 6, 931–945. doi: 10.1100/tsw.2006.181
Bergey, D. H., Whitman, W. B., Krieg, N. R., and Staley, J. T. (2011). Bergey’s Manual of Systematic Bacteriology, Vol. 4. New York, NY: Springer.
Bernardet, J.-F., and Nakagawa, Y. (2006). An introduction to the family flavobacteriaceae. Prokaryotes 455–480. doi: 10.1007/0-387-30747-8_16
Bolger, A. M., Lohse, M., and Usadel, B. (2014). Trimmomatic: a flexible trimmer for Illumina sequence data. Bioinformatics 30, 2114–2120. doi: 10.1093/bioinformatics/btu170
Boyd, C. E., and Tucker, C. S. (1998). Pond Aquaculture Water Quality Management. Boston, MA: Kluwer Academic Publishers, 700. doi: 10.1007/978-1-4615-5407-3_8
Collignon, P., and Angulo, F. J. (2006). Fluoroquinolone-resistant Escherichia coli: food for thought. J. Infect. Dis. 194, 8–10. doi: 10.1086/504922
Dar, S. A., Kleerebezem, R., Stams, A. J., Kuenen, J. G., and Muyzer, G. (2008). Competition and coexistence of sulfate-reducing bacteria, acetogens and methanogens in a lab-scale anaerobic bioreactor as affected by changing substrate to sulfate ratio. Appl. Microbiol. Biotechnol. 78, 1045–1055. doi: 10.1007/s00253-008-1391-8
Davies, C. M., Long, J. A., Donald, M., and Ashbolt, N. J. (1995). Survival of fecal microorganisms in marine and freshwater sediments. Appl. Environ. Microbiol. 61, 1888–1896.
Davis, H. S. (1922). A New Bacterial Disease of Fresh-water Fishes. Washington, DC: Government Printing Office. doi: 10.5962/bhl.title.49773
Dinsdale, E. A., Edwards, R. A., Hall, D., Angly, F., Breitbart, M., Brulc, J. M., et al. (2008). Functional metagenomic profiling of nine biomes. Nature 3, 629–632. doi: 10.1038/nature06810
Durso, L. M., Miller, D. N., and Wienhold, B. J. (2012). Distribution and quantification of antibiotic resistant genes and bacteria across agricultural and non-agricultural metagenomes. PLoS One 7:e48325. doi: 10.1371/journal.pone.0048325
Eldar, A., Bejerano, Y., and Bercovier, H. (1994). Streptococcus shiloi andStreptococcus difficile: two new streptococcal species causing a meningoencephalitis in fish. Curr. Microbiol. 28, 139–143. doi: 10.1007/BF01571054
Eldar, A., Ghittino, C., Asanta, L., Bozzetta, E., Goria, M., Prearo, M., et al. (1996). Enterococcus seriolicida is a junior synonym of Lactococcus garvieae, a causative agent of septicemia and meningoencephalitis in fish. Curr. Microbiol. 32, 85–88. doi: 10.1007/s002849900015
Feris, K. P., Ramsey, P. W., Gibbons, S. M., Frazar, C., Rillig, M. C., Moore, J. N., et al. (2009). Hyporheic microbial community development is a sensitive indicator of metal contamination. Environ. Sci. Technol. 43, 6158–6163. doi: 10.1021/es9005465
Food Agriculture Organization of the United Nations and Fisheries and Aquaculture (2014). The State of World Fisheries and Aquaculture : Opportunities and Challenges. Rome: Food and Agriculture Organization of the United Nations.
Gibbons, S. M., Jones, E., Bearquiver, A., Blackwolf, F., Roundstone, W., Scott, N., et al. (2014). Human and environmental impacts on river sediment microbial communities. PLoS One 9:e97435. doi: 10.1371/journal.pone.0097435
Harrison, B. K., Zhang, H., Berelson, W., and Orphan, V. J. (2009). Variations in archaeal and bacterial diversity associated with the sulfate-methane transition zone in continental margin sediments (Santa Barbara Basin, California). Appl. Environ. Microbiol. 75, 1487–1499. doi: 10.1128/AEM.01812-08
Hawke, J. P., and Thune, R. L. (1992). Systemic isolation and antimicrobial susceptibility of Cytophaga columnaris from commercially reared channel catfish. J. Aquat. Anim. Health 4, 109–113. doi: 10.1577/1548-8667 (1992)004<0109:SIAASO>2.3.CO;2
Holmer, M., and Kristensen, E. (1992). Impact of marine fish cage farming on metabolism and sulfate reduction of underlying sediments. Mar. Ecol. Prog. Ser. 80, 191–201. doi: 10.3354/meps080191
Huson, D. H., Auch, A. F., Qi, J., and Schuster, S. C. (2007). MEGAN analysis of metagenomic data. Genome Res. 17, 377–386. doi: 10.1101/gr.5969107
Kandler, O., and Hippe, H. (1977). Lack of peptidoglycan in the cell walls of Methanosarcina barkeri. Arch. Microbiol. 113, 57–60. doi: 10.1007/BF00428580
Kanehisa, M., Goto, S., Kawashima, S., Okuno, Y., and Hattori, M. (2004). The KEGG resource for deciphering the genome. Nucleic Acids Res. 32, D277–D280. doi: 10.1093/nar/gkh063
Kim, K. K., Kim, M. K., Lim, J. H., Park, H. Y., and Lee, S. T. (2005). Transfer of Chryseobacterium meningosepticum and Chryseobacterium miricola to Elizabethkingia gen. nov. as Elizabethkingia meningoseptica comb. nov. and Elizabethkingia miricola comb. nov. Int. J. Syst. Evol. Microbiol. 55, 1287–1293. doi: 10.1099/ijs.0.63541-0
Kimes, N. E., Callaghan, A. V., Aktas, D. F., Smith, W. L., Sunner, J., Golding, B., et al. (2013). Metagenomic analysis and metabolite profiling of deep-sea sediments from the Gulf of Mexico following the Deepwater Horizon oil spill. Front. Microbiol. 4:50. doi: 10.3389/fmicb.2013.00050
Konneke, M., Bernhard, A. E., de la Torre, J. R., Walker, C. B., Waterbury, J. B., and Stahl, D. A. (2005). Isolation of an autotrophic ammonia-oxidizing marine archaeon. Nature 437, 543–546. doi: 10.1038/nature03911
Kowalchuk, G. A., and Stephen, J. R. (2001). Ammonia-oxidizing bacteria: a model for molecular microbial ecology. Annu. Rev. Microbiol. 55, 485–529. doi: 10.1146/annurev.micro.55.1.485
Larsen, P. E., Gibbons, S. M., and Gilbert, J. A. (2012). Modeling microbial community structure and functional diversity across time and space. FEMS Microbiol. Lett. 332, 91–98. doi: 10.1111/j.1574-6968.2012.02588.x
Leadbetter, E. R. (2006). “The genus Capnocytophaga,” in The Prokaryotes, eds M. Dworkin, S. Falkow, E. Rosenberg, K. H. Schleifer, and E. Stackebrandt (New York, NY: Springer,), 709–711.
Leung, K., England, L. S., Cassidy, M. B., Trevors, J. T., and Weir, S. (1994). Microbial diversity in soil: effect of releasing genetically engineered micro-organisms. MEC Mol. Ecol. 3, 413–422. doi: 10.1111/j.1365-294X.1994.tb00081.x
Loch, T. P., and Faisal, M. (2015). Emerging flavobacterial infections in fish: a review. J. Adv. Res. 6, 283–300. doi: 10.1016/j.jare.2014.10.009
Logue, J. B., Bürgmann, H., and Robinson, C. T. (2008). Progress in the ecological genetics and biodiversity of freshwater bacteria. Bioscience 58, 103–113. doi: 10.1641/B580205
Maeder, D. L., Anderson, I., Brettin, T. S., Bruce, D. C., Gilna, P., Han, C. S., et al. (2006). The Methanosarcina barkeri genome: comparative analysis with Methanosarcina acetivorans and Methanosarcina mazei reveals extensive rearrangement within methanosarcinal genomes. J. Bacteriol. 188, 7922–7931. doi: 10.1128/JB.00810-06
Martins, P., Cleary, D. F., Pires, A. C., Rodrigues, A. M., Quintino, V., Calado, R., et al. (2013). Molecular analysis of bacterial communities and detection of potential pathogens in a recirculating aquaculture system for Scophthalmus maximus and Solea senegalensis. PLoS One 8:e80847. doi: 10.1371/journal.pone.0080847
Meyer, F., Paarmann, D., D’Souza, M., Olson, R., Glass, E. M., Kubal, M., et al. (2008). The metagenomics RAST server - a public resource for the automatic phylogenetic and functional analysis of metagenomes. BMC Bioinformatics 9:386. doi: 10.1186/1471-2105-9-386
Mueller-Spitz, S. R., Stewart, L. B., Klump, J. V., and McLellan, S. L. (2010). Freshwater suspended sediments and sewage are reservoirs for enterotoxin-positive Clostridium perfringens. Appl. Environ. Microbiol. 76, 5556–5562. doi: 10.1128/AEM.01702-09
Muyzer, G., and Stams, A. J. (2008). The ecology and biotechnology of sulphate-reducing bacteria. Nat. Rev. Microbiol. 6, 441–454. doi: 10.1038/nrmicro1892
Niu, B., Fu, L., Sun, S., and Li, W. (2010). Artificial and natural duplicates in pyrosequencing reads of metagenomic data. BMC Bioinformatics 11:187. doi: 10.1186/1471-2105-11-187
Nogales, B., Lanfranconi, M. P., Pina-Villalonga, J. M., and Bosch, R. (2011). Anthropogenic perturbations in marine microbial communities. FEMS Microbiol. Rev. 35, 275–298. doi: 10.1111/j.1574-6976.2010.00248.x
Oremland, R. S., and Polcin, S. (1982). Methanogenesis and sulfate reduction: competitive and noncompetitive substrates in estuarine sediments. Appl. Environ. Microbiol. 44, 1270–1276.
Overbeek, R., Begley, T., Butler, R. M., Choudhuri, J. V., Chuang, H. Y., Cohoon, M., et al. (2005). The subsystems approach to genome annotation and its use in the project to annotate 1000 genomes. Nucleic Acids Res. 33, 5691–5702. doi: 10.1093/nar/gki866
Parks, D. H., and Beiko, R. G. (2010). Identifying biologically relevant differences between metagenomic communities. Bioinformatics 26, 715–721. doi: 10.1093/bioinformatics/btq041
Ringø, E., and Gatesoupe, F.-J. (1998). Lactic acid bacteria in fish: a review. Aquaculture 160, 177–203. doi: 10.1016/S0044-8486(97)00299-8
Saunders, D. L., and Kalff, J. (2001). Denitrification rates in the sediments of Lake Memphremagog, Canada USA. Water Res. 35, 1897–1904. doi: 10.1016/S0043-1354(00)00479-6
Schneegurt, M. A., Dore, S. Y., and Kulpa, C. F. Jr. (2003). Direct extraction of DNA from soils for studies in microbial ecology. Curr. Issues Mol. Biol. 5, 1–8.
Schreier, H. J., Mirzoyan, N., and Saito, K. (2010). Microbial diversity of biological filters in recirculating aquaculture systems. Curr. Opin. Biotechnol. 21, 318–325. doi: 10.1016/j.copbio.2010.03.011
Simon, C., and Daniel, R. (2009). Achievements and new knowledge unraveled by metagenomic approaches. Appl. Microbiol. Biotechnol. 85, 265–276. doi: 10.1007/s00253-009-2233-z
Sousa, O. V., Macrae, A., Menezes, F. G., Gomes, N. C., Vieira, R. H., and Mendonca-Hagler, L. C. (2006). The impact of shrimp farming effluent on bacterial communities in mangrove waters. Ceara, Brazil. Mar. Pollut. Bull. 52, 1725–1734. doi: 10.1016/j.marpolbul.2006.07.006
Staley, C., Unno, T., Gould, T. J., Jarvis, B., Phillips, J., Cotner, J. B., et al. (2013). Application of Illumina next-generation sequencing to characterize the bacterial community of the Upper Mississippi River. J. Appl. Microbiol. 115, 1147–1158. doi: 10.1111/jam.12323
Takai, K., Campbell, B. J., Cary, S. C., Suzuki, M., Oida, H., Nunoura, T., et al. (2005). Enzymatic and genetic characterization of carbon and energy metabolisms by deep-sea hydrothermal chemolithoautotrophic isolates of Epsilonproteobacteria. Appl. Environ. Microbiol. 71, 7310–7320. doi: 10.1128/AEM.71.11.7310-7320.2005
Tatusov, R. L., Natale, D. A., Garkavtsev, I. V., Tatusova, T. A., Shankavaram, U. T., Rao, B. S., et al. (2001). The COG database: new developments in phylogenetic classification of proteins from complete genomes. Nucleic Acids Res. 29, 22–28. doi: 10.1093/nar/29.1.22
USDA (2018). USDA, 2018, Catfish production, by the National Agricultural Statistics Service (NASS), Agricultural Statistics Board. Washington, DC: United States Department of Agriculture (USDA).
Wang, X., Wang, C., Bao, L., and Xie, S. (2014). Abundance and community structure of ammonia-oxidizing microorganisms in reservoir sediment and adjacent soils. Appl. Microbiol. Biotechnol. 98, 1883–1892. doi: 10.1007/s00253-013-5174-5
Woese, C. R., Yang, D., Mandelco, L., and Stetter, K. O. (1990). The Flexibacter-Flavobacter connection. Syst. Appl. Microbiol. 13, 161–165. doi: 10.1016/S0723-2020(11)80163-3
Wrighton, K. C., Castelle, C. J., Wilkins, M. J., Hug, L. A., Sharon, I., Thomas, B. C., et al. (2014). Metabolic interdependencies between phylogenetically novel fermenters and respiratory organisms in an unconfined aquifer. ISME J. 8, 1452–1463. doi: 10.1038/ismej.2013.249
Wu, S., Gao, T., Zheng, Y., Wang, W., Cheng, Y., and Wang, G. (2010). Microbial diversity of intestinal contents and mucus in yellow catfish (Pelteobagrus fulvidraco). Aquaculture 303, 1–7. doi: 10.1016/j.aquaculture.2009.12.025
Wu, S., Wang, G., Angert, E. R., Wang, W., Li, W., and Zou, H. (2012). Composition, diversity, and origin of the bacterial community in grass carp intestine. PLoS One 7:e30440. doi: 10.1371/journal.pone.0030440
Keywords: metagenome, sediment, aquaculture pond, catfish, eutrophic, nitrogen metabolism, sulfur metabolism, methanogenesis
Citation: Nho SW, Abdelhamed H, Paul D, Park S, Mauel MJ, Karsi A and Lawrence ML (2018) Taxonomic and Functional Metagenomic Profile of Sediment From a Commercial Catfish Pond in Mississippi. Front. Microbiol. 9:2855. doi: 10.3389/fmicb.2018.02855
Received: 06 March 2018; Accepted: 06 November 2018;
Published: 22 November 2018.
Edited by:
Jaime Romero, Universidad de Chile, ChileReviewed by:
Virginia Helena Albarracín, Center for Electron Microscopy (CIME), ArgentinaSigmund Jensen, University of Bergen, Norway
Copyright © 2018 Nho, Abdelhamed, Paul, Park, Mauel, Karsi and Lawrence. This is an open-access article distributed under the terms of the Creative Commons Attribution License (CC BY). The use, distribution or reproduction in other forums is permitted, provided the original author(s) and the copyright owner(s) are credited and that the original publication in this journal is cited, in accordance with accepted academic practice. No use, distribution or reproduction is permitted which does not comply with these terms.
*Correspondence: Mark L. Lawrence, bGF3cmVuY2VAY3ZtLm1zc3RhdGUuZWR1