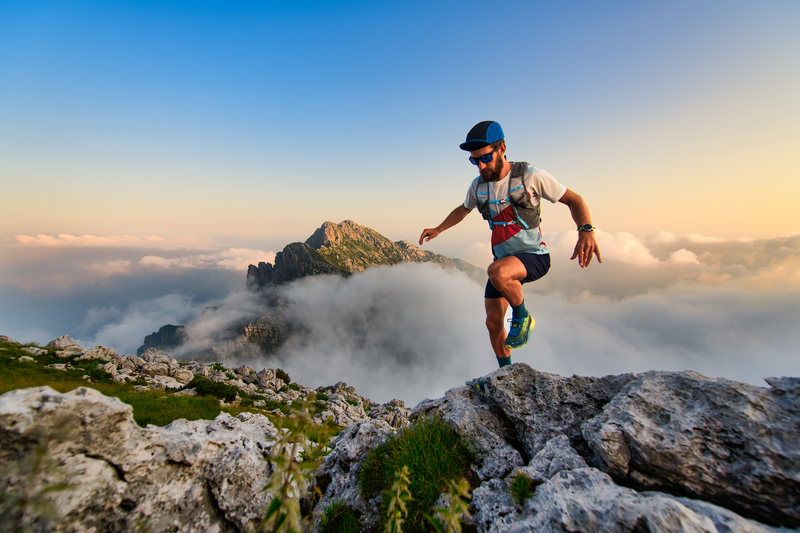
95% of researchers rate our articles as excellent or good
Learn more about the work of our research integrity team to safeguard the quality of each article we publish.
Find out more
ORIGINAL RESEARCH article
Front. Microbiol. , 20 November 2018
Sec. Evolutionary and Genomic Microbiology
Volume 9 - 2018 | https://doi.org/10.3389/fmicb.2018.02717
This article is part of the Research Topic Evolution of Genetic Mechanisms of Antibiotic Resistance View all 16 articles
Candida krusei is a notable pathogenic fungus that causes invasive candidiasis, mainly due to its natural resistance to fluconazole. However, to date, there is limited research on the genetic population features of C. krusei. We developed a set of microsatellite markers for this organism, with a cumulative discriminatory power of 1,000. Using these microsatellite loci, 48 independent C. krusei strains of clearly known the sources, were analyzed. Furthermore, susceptibility to 9 antifungal agents was determined for each strain, by the Clinical and Laboratory Standards Institute broth microdilution method. Population structure analyses revealed that C. krusei could be separated into two clusters. The cluster with the higher genetic diversity had wider MIC ranges for six antifungal agents. Furthermore, the highest MIC values of the six antifungal agents belonged to the cluster with higher genetic diversity. The higher genetic diversity cluster might have a better adaptive capacity when C. krusei is under selection pressure from antifungal agents, and thus is more likely to develop drug resistance.
Invasive candidiasis is the most common fungal disease among hospitalized patients, and affects more than 250,000 people worldwide annually, with more than 50,000 deaths reported (Kullberg and Arendrup, 2015). In the Candida genus, Candida krusei attracts much medical attention because it is intrinsically resistant to fluconazole (Akova et al., 1991; Schuster et al., 2013). In addition, C. krusei exhibits resistance to other antifungal drugs such as voriconazole, echinocandins, and amphotericin B (Fukuoka et al., 2003; Hakki et al., 2006; Pfaller et al., 2008). It has been known for some time that mutations in ERG11 and FKS 1 genes are the major mechanisms responsible for azole- and echinocandin-resistance in Candida species, including C. krusei (Jensen et al., 2014; Forastiero et al., 2015; Feng et al., 2016; Perlin et al., 2017). In addition, antifungal resistance can be acquired by over-expression of efflux pump e.g., Abc1p (Lamping et al., 2009; Ricardo et al., 2014). However, there have been some C. krusei antifungal resistant phenotypes, including resistance to azoles other than fluconazole and to enchinocandins e.g., caspofungin, that cannot be explained by currently known mechanisms of resistance (Hakki et al., 2006; Whaley et al., 2017).
From an evolutionary perspective, drug resistance in a microorganism is part of the adaptive evolutionary response of a species to environmental pressures (Salmond and Welch, 2008). Nowadays, the environmental pressure of antifungal drugs comes not only from the use of clinical drugs, but also from the use of agricultural drugs (Sanglard, 2016).
The adaptive capacity is usually related to the level of genetic diversity. From the points of molecular ecology, genetic diversity can allow species or populations to adapt quickly to changing environment conditions and different habitats (Freeland et al., 2011). Similarly, from the perspective of conservation genetics, genetic diversity allows species or populations to tolerate a wider range of environmental changes, including bacteria, fungi and so on. Also, genetic diversity is helpful to maintain the evolutionary vigor (Frankham et al., 2010). In general, a higher genetic diversity enables the organism to respond better to new selection pressures (McDonald and Linde, 2002). When there was a selection pressure for exogenous antifungal agents, the more genetic diversity the fungal populations had, the higher the probability of survival. In other words, antifungal agents were the directional selection factors from Darwin's theory of Evolution. If the genetic diversity of the fungal population was high, there might be some individual death under the pressure of drug selection, but some individuals carrying different genes would survive. Therefore, it is reasonable to hypothesize that microbial populations with higher genetic diversity are more likely to develop antimicrobial drug resistance.
However, to our best knowledge, no research targeting the genetic population features of C. krusei has been carried out to date. This is partly due to the lack of a flexible molecular typing method. Therefore, in this study, we (1) developed a novel set of microsatellite markers for molecular typing and population genetic analysis of C. krusei; (2) used the developed assay to type 48 multicenter collected C. krusei clinical strains; and (3) analyzed the correlation between genetic diversity and drug susceptibility among the studied strains.
This study was reviewed and approved by the ethics committee of the National Institute for Communicable Disease Control and Prevention, Chinese CDC. Written informed consent was obtained from patients for use of the samples in research.
A total of 48 C. krusei isolates, each from a single patient, were collected from 15 hospitals distributed in 10 cities across China during the period 2009–2012, as part of the national surveillance program for invasive fungal infections (the CHIF-NET study, Figure 1 and Supplemental A). The isolates were stored at −80°C until use at Peking Union Medical College Hospital, Beijing, China (PUMCH). Before testing, the isolates were inoculated on CHROMagar™ Candida medium (Difco Laboratories, Detroit, MI, USA) and incubated at 37°C for 24 h. Species identification of the isolates was confirmed by matrix-assisted laser desorption/ionization-time of flight mass spectrometry (MALDI-TOF MS, Vitek MS, bioMérieux, Marcy-l'E'toile, France) as per manufacturer's instructions, and by sequence analysis of their rDNA internal transcribed spacer (ITS) regions (Wang et al., 2012). The identities of all the isolates was confirmed by sequencing.
Figure 1. Geographical locations of sampled Candida krusei populations in China. The figure is generated by ArcGIS Desktop (version 9.3, ESRI, Redlands, USA).
All the fungal isolates were grown on potato dextrose agar at 37°C for 24 h. DNA extraction was performed using a QIAamp DNA Mini Kit (Qiagen, Hilden, Germany).
The software SciRoKo was used to identify microsatellites in the C. krusei genome (GenBank assembly accession: GCA_001983325.1, Kofler et al., 2007; Cuomo et al., 2017). Primers were designed using Primer premier 5.0 (PREMIER Biosoft International, Palo Alto, CA, USA) in regions flanking microsatellite loci, and annealing temperatures were optimized with a gradient PCR. Polymorphic microsatellite loci were selected for molecular typing and population genetic analysis of C. krusei. There were two criteria used for selection of microsatellite loci: first, the locus had to have a relatively high genetic polymorphism (the number of alleles was >3); second, the locus could be amplified relatively stable. The microsatellite loci would be abandoned if the loci was not amplified in more than two strains.
For the 33 selected microsatellite loci (Table 1), PCR was performed on 48 clinical isolates. Amplification was carried out using a Taq polymerase kit (Takara, Dalian, China). Each of the amplification reactions was composed of 1 × PCR buffer, 0.2 μM dNTP, 0.5 U Taq polymerase, 0.2 μM each primer, and 2 μl genomic DNA (20–50 ng/μl). The thermocycler conditions were as follows: initial denaturation at 95°C for 5 min, followed by 35 cycles of denaturation at 94°C for 30 s, annealing at an optimized primer-specific annealing temperature for 30 s (Table 1), extension at 72°C for 30 s and final extension at 72°C for 10 min. The primers for these selected loci were fluorescently labeled with 6-carboxy-fluorescein (6-FAM). Allele length was determined by migration of PCR products on an ABI 3,700 automated capillary DNA sequencer (Applied Biosystems). Allele sizes were assigned with GeneMapper software (version 3.7) according to an internal size standard (LIZ 500, Applied Biosystems).
The in vitro susceptibility to nine antifungal drugs- fluconazole, voriconazole, itraconazole, posaconazole, caspofungin, micafungin, anidulafungin, amphotericin B, and 5-flucytosine, was determinedfor 48 isolates using the Clinical and Laboratory Standards Institute (CLSI) broth microdilution method22. Minimum inhibitory concentration (MIC) results for fluconazole, voriconazole, caspofungin, micafungin, and anidulafungin, were interpreted using clinical breakpoints in accordance with the CLSI guidelines (CLSI, 2017), and those for itraconazole, posaconazole, amphotericin B, and 5-flucytosine, were interpreted using epidemiological cut-off values (Xiao et al., 2014). The quality control strains used were C.krusei ATCC 6,258 and Candida parapsilosis ATCC 22,019.
Deviation from Hardy-Weinberg equilibrium was computed using GENEPOP version 4 (Rousset, 2008). Hardy-Weinberg equilibrium was tested using the score test for heterozygote deficiency and the significance was addressed by a Markov Chain algorithm (Markov chain parameters: dememorization number = 2,000, number of batches = 250, number of iterations per batch = 2,000).
The discriminatory power of markers was calculated according to the method of Hunter and Gaston (1988). Number of alleles (nA), effective number of alleles (ne), Shannon's Information Index (I), and Nei's unbiased gene diversity (HS), were calculated using GENALEX 6.5 (Peakall and Smouse, 2012). Allelic Richness (AR) was calculated by FSTAT 2.9.3 (Goudet, 1995). Ne, I, HS, and AR were used to measure genetic variability of populations.
Population composition was inferred for the C. krusei isolates using the program Structure 2.3 (Pritchard et al., 2000), which estimates the log probability of the data for each value of K (number of clusters or populations). A series of independent runs were performed by using K from 1 to 12 populations, a burn-in of 100,000 Markov chain Monte Carlo (MCMC) iterations, and a data collection period of 100,000 MCMC iterations. Each simulation of K was replicated 10 times. The method of Evanno et al. (2005) was used to estimate the most likely K given the data with Structure Harvester (Earl, 2012). The level of genetic differentiation at microsatellite loci among clusters was estimated as FST, which is simply a measure of how genetically similar populations are to one another. FST was calculated using Arlequin 3.5 (Excoffier and Lischer, 2010). Principal coordinates analysis (PCoA) of FST value among clusters was calculated using GenAlEx (Peakall and Smouse, 2012).
For antifungal susceptibility results, MIC50, MIC90, and geometric mean (GM) MIC values were calculated using WHONET software (version 5.6, WHO Collaborating Center for Surveillance of Antimicrobial Resistance, Boston, USA).
Based on the genome of C. krusei, a total of 200 microsatellite loci were identified, and primers were designed (data not shown). Of these microsatellite loci, 33 polymorphic microsatellite loci (Cakr 001–Cakr 033) could be stably amplified in all C. krusei isolates (Table 1). The cumulative discriminatory power of the 33 loci was 1.000. If only 8 polymorphic sites with the highest polymorphism were selected (Cakr004, Cakr005, Cakr011, Cakr019, Cakr025, Cakr026, Cakr029, Cakr031), it was found that the cumulative discriminatory power would still be 1.000. This might mean that the molecular typing of strains could be achieved effectively by using only these 8 microsatellite loci. All loci showed significant deviation from Hardy-Weinberg equilibrium (P < 0.05).
In addition, it must be noted that many isolates were heterozygote (Supplemental A).
When performing STRUCTURE analyses, the clustering level K = 2 yielded the largest delta-K value (Figure 2A). At K = 2, individual isolates could be assigned to two clusters (Figure 2B). Cluster A included 17 strains and cluster B 31 strains. There was no clear relationship between cluster patterns and geographical source of the isolates, and between cluster patterns and disease clinical manifestations. The FST between the two clusters was 0.188 (P < 0.01). The principal coordinate analysis (PCoA) supported the result of the STRUCTURE analyses (Figure 3), suggesting that the population of C. krusei was divided into two lineages. The PCoA also suggested that two lineages would both consist of strains from different geographical origins and clinical manifestations of disease. However, if patients from whom the isolates were obtained is considered, there appears to be some differences between the two clusters. The hosts of Cluster A strains were mainly children younger than 10 years old or aged people older than 50 years old. In contrast, the hosts of Cluster B covered almost all age groups (Figure 4). Furthermore, the specimen types of Cluster B are also more complex than Cluster A (Figure 5).
Figure 2. STRUCTURE analyses of 48 C. krusei strains. (A) STRUCTURE analysis estimates that the optimal predicted number of populations K for our set of genotypes is two. (B) Bayesian estimation of the population structure of C. krusei by STRUCTURE. Each vertical bar represents one individual and is partitioned into colored segments that represent the individual's estimated membership fractions in K clusters.
Figure 3. Results of principal coordinate analysis (PCoA) of C. krusei clusters. Using estimates of Nei's unbiased genetic distance supports 2 main subgroups, which corresponded to the 2 clusters divided by STRUCTURE software.
The genetic diversity of C. krusei was assessed by Shannon's Information Index, Nei's unbiased gene diversity and allelic richness. These indices are shown in Table 2. Four indices (Mean number of effective alleles, Shannon's Information Index, Nei's unbiased gene diversity, allelic richness), all showed that there was a higher genetic diversity in cluster B.
All isolates were intrinsically resistant to fluconazole (MICs ≥ 16 mg/L; Figure 6, Supplemental A). Of the other eight antifungal agents tested, all isolates were susceptible or of wild-type phenotype to voriconazole, itraconazole, posaconazole, anidulafungin, micafungin, 5-flucytosine, and amphotericin B. Only two of 48 isolates (4.2%) were interpreted as intermediate to caspofungin, both of which belonged to microsatellite cluster B, while the rest 95.8% (46/48) isolates remained susceptible to caspofungin (Figure 6, Supplemental A).
The Geometric mean (GM) MIC, MIC50, and MIC90 of the two clusters were generally similar, while the MIC range differed between the two clusters (Table 3, Figure 6). For most antifungal agents (6/9) (including caspofungin, posaconazole, voriconazole, itraconazole, fluconazole, amphotericin B), cluster B had a wider MIC range. It is worth noting that the highest MIC values of all 6 antifungal agents were confined to cluster B.
The correlation between genetic diversity and adaptive capacity of the population has long been studied in the field of molecular ecology. For example, it was found that the Arabidopsis thaliana population with higher genetic diversity had better colonization success (Crawford and Whitney, 2010). In principle, the process of fungal infection and clinical manifestation of disease is also considered a colonization success. Unfortunately, very few studies have been done on fungal infections and drug-resistance from the perspective of population genetics. In this study, we carried out a comprehensive analysis of the population genetic features and drug resistance, and attempted to elucidate the drug resistance of C. krusei from an evolutionary perspective.
Although an important pathogenic fungal species, the population genetic parameters of C. krusei have remained largely unknown. In this study, a novel array of microsatellite markers was developed for molecular typing and population genetic analysis of the species. The discriminatory index of the new method (1.000) was slightly higher than MLST, which exhibited a discriminatory index of 0.998 (Jacobsen et al., 2007). It has been shown by Cuomo et al. (2017) that the genome of C. krusei is highly heterozygous, and this was also confirmed in the present study. For all microsatellite loci, there were some heterozygous individual isolates. As previously described in Candida albicans, a significant departure from Hardy-Weinberg equilibrium expectations was found (Sampaio et al., 2003). This finding supports the previous conclusions that reproduction in C. krusei is mainly clonal.
Based on the STRUCTURE software analysis, the C. krusei population in China appears to be divided into two clusters, a result which was also supported by PCoA. The two clusters showed no obvious differences with respect to geographical distribution of the isolates. These findings are very similar to those of another pathogenic fungus, Trichophyton rubrum, which was also divided into two clusters with similar geographical distributions of the clusters (Gong et al., 2016). For pathogenic fungi, it might be a common phenomenon that different clusters of the same organism co-exist in the same geographical locale. Dispersal of pathogenic fungi is generally affected by host activity. It is highly possible that C. krusei strains of different clusters existed in different geographical areas and were carried to the same geographical area by hosts including humans. When the survival capacity of different clusters is similar, it indicates that the different clusters co-existed in the same area. However, there were significant differences in host age between the two clusters, which might suggest that cluster B strains have a higher pathogenicity. Moreover, the type of specimens in Cluster B were also more complicated and seemed to confirm this. However, this is only a hypothesis, and more work needs to be done to demonstrate these findings in animal infection model experiments.
Cluster B had a higher genetic diversity which suggests a better adaptive capacity for survival in challenging conditions. As previously mentioned, the population with a higher genetic diversity is more likely to develop antimicrobial drug resistance. In our study, cluster B had a wider MIC range for 6 antifungal drugs, although there was no obvious difference in GM MIC, MIC50, and MIC90 between the two clusters. Specifically, the strains with the highest MIC value were either in the current group B (including 6 drugs: caspofungin, posaconazole, voriconazole, itraconazole, fluconazole, and amphotericin B), or both in group A and group B (including 3 drugs: anidulafungin, micafungin, and 5-flucytosine). Meanwhile, there was no strain with the highest MIC value was observed in Cluster A. These findings suggest that the population with higher genetic diversity may have more diverse phenotypes, including drug resistance. When subjected to selective pressure from antifungal drugs, cluster B might have a better adaptive capacity, and thus would be more likely to develop drug resistance. This suggests that the C. krusei population or lineage with higher genetic diversity needs more attention in terms of fungal drug resistance.
In conclusion, C. krusei was divided into two clusters by novel high-resolution microsatellite markers. The cluster with higher genetic diversity had wider MIC ranges for six antifungal agents, and the highest MIC values of the six antifungal agents belonged to the cluster of higher genetic diversity. It is plausible that the C. krusei cluster with higher genetic diversity might have better adaptive capacity when under the selection pressure of antifungal agents.
JZ and Y-CX designed the experiments. JG, MX, HW, FZ, LH, and WW collected the samples and performed the experiments. JG, MX, TK, and YW analyzed data. JG, MX, and TK wrote the manuscript. All authors read and approved the final manuscript.
The authors declare that the research was conducted in the absence of any commercial or financial relationships that could be construed as a potential conflict of interest.
This work was supported by a Peking Union Medical College Hospital Out-standing Young Talents Program (JQ201703), a Chinese Academy of Medical Sciences Innovation Fund for Medical Sciences (2016-I2M-1-014), a Beijing Innovation Base Cultivation and Development Special Fund (Z171100002217068), and the Major Infectious Diseases Such as AIDS and Viral Hepatitis Prevention and Control Technology Major Projects (2018ZX10712001).
The Supplementary Material for this article can be found online at: https://www.frontiersin.org/articles/10.3389/fmicb.2018.02717/full#supplementary-material
Akova, M., Akalin, H., Uzun, O., and Gür, D. (1991). Emergence of Candida krusei infections after therapy of oropharyngeal candidiasis with fluconazole. Eur. J. Clin. Microbiol. 10, 598–599.
CLSI (2017). Performance Standards for Antifungal Susceptibility Testing of Yeasts. Document M60Ed1E. Wayne, PA: Clinical, and Laboratory Standards Institute.
Crawford, K., and Whitney, K. (2010). Population genetic diversity influences colonization success. Mol. Ecol. 19, 1253–1263. doi: 10.1111/j.1365-294X.2010.04550.x
Cuomo, C. A., Shea, T., Yang, B., Ra, R., and Forche, A. (2017). Whole genome sequence of the heterozygous clinical isolate Candida krusei 81-B-5. G3 7, 2883–2889. doi: 10.1534/g3.117.043547
Earl, D. A. (2012). Structure harvester: a website and program for visualizing structure output and implementing the evanno method. Conserv. Genet. Resour. 4, 359–361. doi: 10.1007/s12686-011-9548-7
Evanno, G., Regnaut, S., and Goudet, J. (2005). Detecting the number of clusters of individuals using the software structure: a simulation study. Mol. Ecol. 14, 2611–2620. doi: 10.1111/j.1365-294X.2005.02553.x
Excoffier, L., and Lischer, H. E. (2010). Arlequin suite ver 3.5: a new series of programs to perform population genetics analyses under Linux and Windows. Mol. Ecol. Resour. 10, 564–567. doi: 10.1111/j.1755-0998.2010.02847.x
Feng, W., Yang, J., Wang, Y., Chen, J., Xi, Z., and Qiao, Z. (2016). ERG11 mutations and upregulation in clinical itraconazole-resistant isolates of Candida krusei. Can. J. Microbiol. 62,938–943. doi: 10.1139/cjm-2016-0055
Forastiero, A., Garcia-Gil, V., Rivero-Menendez, O., Garcia-Rubio, R., Monteiro, M., Alastruey-Izquierdo, A., et al. (2015). Rapid development of Candida krusei echinocandin resistance during caspofungin therapy. Antimicrob. Agents. Chemother. 59, 6975–6982. doi: 10.1128/AAC.01005-15
Frankham, R., Ballow, J. D., and Briscoe, D. A. (2010). Introduction to Conservation Genetics, 2nd Edn. New York, NY: Cambridge University Press.
Freeland, J. R., Kirk, H., and Peterson, S. (2011). Molecular Ecology, 2nd Edn. West Sussex: John Wiley & Sons, Ltd.
Fukuoka, T., Johnston, D. A., Winslow, C. A., de Groot, M. J., Burt, C., Hitchcock, C. A., et al. (2003). Genetic basis for differential activities of fluconazole and voriconazole against Candida krusei. Antimicrob. Agents Chemother. 47, 1213–1219. doi: 10.1128/AAC.47.4.1213-1219.2003
Gong, J., Wu, W., Ran, M., Wang, X., Liu, W., Wan, Z., et al. (2016). Population differentiation and genetic diversity of Trichophyton rubrum as revealed by highly discriminatory microsatellites. Fungal. Genet. Biol. 95, 24–29. doi: 10.1016/j.fgb.2016.08.002
Goudet, J. (1995). FSTAT (version 1.2): a computer program to calculate F-statistics. J. Hered. 86, 485–486.
Hakki, M., Staab, J. F., and Marr, K. A. (2006). Emergence of a Candida krusei isolate with reduced susceptibility to caspofungin during therapy. Antimicrob. Agents Chemother. 50, 2522–2524. doi: 10.1128/AAC.00148-06
Hunter, P. R., and Gaston, M. A. (1988). Numerical index of the discriminatory ability of typing systems: an application of Simpson's index of diversity. J. Clin. Microbiol. 26, 2465–2466.
Jacobsen, M. D., Gow, N. A., Maiden, M. C., Shaw, D. J., and Odds, F. C. (2007). Strain typing and determination of population structure of Candida krusei by multilocus sequence typing. J. Clin. Microbiol. 45, 317–323. doi: 10.1128/JCM.01549-06
Jensen, R. H., Justesen, U. S., Rewes, A., Perlin, D. S., and Arendrup, M. C. (2014). Echinocandin failure case due to a previously unreported FKS1 mutation in Candida krusei. Antimicrob. Agents Ch. 58, 3550–3552. doi: 10.1128/AAC.02367-14
Kofler, R., Schlötterer, C., and Lelley, T. (2007). SciRoKo: a new tool for whole genome microsatellite search and investigation. Bioinformatics 23, 1683–1685. doi: 10.1093/bioinformatics/btm157
Kullberg, B. J., and Arendrup, M. C. (2015). Invasive candidiasis. N. Engl. J. Med. 373, 1445–1456. doi: 10.1056/NEJMra1315399
Lamping, E., Ranchod, A., Nakamura, K., Tyndall, J. D., Niimi, K., Holmes, A. R., et al. (2009). Abc1p is a multidrug efflux transporter that tips the balance in favor of innate azole resistance in Candida krusei. Antimicrob. Agents Chemother. 53, 354–369. doi: 10.1128/AAC.01095-08
McDonald, B. A., and Linde, C. (2002). Pathogen population genetics, evolutionary potential, and durable resistance. Annu. Rev. Phytopathol. 40, 349–379.doi: 10.1146/annurev.phyto.40.120501.101443
Peakall, R., and Smouse, P. (2012). GenAlEx 6.5: genetic analysis in Excel. Population genetic software for teaching and research: an update. Bioinformatics 28, 2537–2539. doi: 10.1093/bioinformatics/bts460
Perlin, D. S., Rautemaa-Richardson, R., and Alastruey-Izquierdo, A. (2017). The global problem of antifungal resistance: prevalence, mechanisms, and management. Lancet Infect. Dis. 17, e383–e392. doi: 10.1016/S1473-3099(17)30316-X
Pfaller, M. A., Diekema, D. J., Gibbs, D. L., Newell, V. A., Nagy, E., Dobiasova, S., et al. (2008). Candida krusei, a multidrug-resistant opportunistic fungal pathogen: geographic and temporal trends from the artemis disk antifungal surveillance program, 2001 to 2005. J. Clin. Microbiol. 46, 515–521. doi: 10.1128/JCM.01915-07
Pritchard, J. K., Stephens, M., and Donnelly, P. (2000). Inference of population structure using multilocus genotype data. Genetics 155, 945–959.
Ricardo, E., Miranda, I. M., Faria-Ramos, I., Silva, R. M., Rodrigues, A. G., and Pina-Vaz, C. (2014). In vivo and in vitro acquisition of resistance to voriconazole by Candida krusei. Antimicrob. Agents Chemother. 58, 4604–4611. doi: 10.1128/AAC.02603-14
Rousset, F. (2008). Genepop'007: a complete re-implementation of the genepop software for Windows and Linux. Mol. Ecol. Resour. 8, 103–106. doi: 10.1111/j.1471-8286.2007.01931.x
Salmond, G. P., and Welch, M. (2008). Antibiotic resistance: adaptive evolution. Lancet 372, S97–S103. doi: 10.1016/S0140-6736(08)61888-7
Sampaio, P., Gusmão, L., Alves, C., Pina-Vaz, C., Amorim, A., and Pais, C. (2003). Highly polymorphic microsatellite for identification of Candida albicans strains. J. Clin. Microbiol. 41, 552–557. doi: 10.1128/JCM.41.2.552-557.2003
Sanglard, D. (2016). Emerging threats in antifungal-resistant fungal pathogens. Front. Med. 3:11. doi: 10.3389/fmed.2016.00011
Schuster, M. G., Meibohm, A., Lloyd, L., and Strom, B. (2013). Risk factors and outcomes of Candida krusei bloodstream infection: a matched, case-control study. J. Infection. 66, 278–284. doi: 10.1016/j.jinf.2012.11.002
Wang, H., Xiao, M., Chen, S. C., Kong, F., Sun, Z. Y., Liao, K., et al. (2012). In vitro susceptibilities of yeast species to fluconazole and voriconazole as determined by the 2010 national china hospital invasive fungal surveillance net (CHIF-NET) study. J. Clin. Microbiol. 50, 3952–3959. doi: 10.1128/JCM.01130-12
Whaley, S. G., Berkow, E. L., Rybak, J. M., Nishimoto, A. T., Barker, K. S., and Rogers, P. D. (2017). Azole antifungal resistance in Candida albicans and emerging non-albicans Candida species. Front. Microbiol. 7:2173. doi: 10.3389/fmicb.2016.02173
Xiao, M., Fan, X., Chen, S. C., Wang, H., Sun, Z. Y., Liao, K., et al. (2014). Antifungal susceptibilities of Candida glabrata species complex, Candida krusei, Candida parapsilosis species complex and Candida tropicalis causing invasive candidiasis in China: 3 year national surveillance. J. Antimicrob. Chemother. 70, 802–810. doi: 10.1093/jac/dku460
Keywords: Candida krusei, invasive candidiasis, genetic differentiation, genetic diversity, microsatellites, drug susceptibility
Citation: Gong J, Xiao M, Wang H, Kudinha T, Wang Y, Zhao F, Wu W, He L, Xu Y-C and Zhang J (2018) Genetic Differentiation, Diversity, and Drug Susceptibility of Candida krusei. Front. Microbiol. 9:2717. doi: 10.3389/fmicb.2018.02717
Received: 19 July 2018; Accepted: 24 October 2018;
Published: 20 November 2018.
Edited by:
Silvia Buroni, University of Pavia, ItalyReviewed by:
Paula Sampaio, University of Minho, PortugalCopyright © 2018 Gong, Xiao, Wang, Kudinha, Wang, Zhao, Wu, He, Xu and Zhang. This is an open-access article distributed under the terms of the Creative Commons Attribution License (CC BY). The use, distribution or reproduction in other forums is permitted, provided the original author(s) and the copyright owner(s) are credited and that the original publication in this journal is cited, in accordance with accepted academic practice. No use, distribution or reproduction is permitted which does not comply with these terms.
*Correspondence: Ying-Chun Xu, eHljcHVtY2hAMTM5LmNvbQ==
Jianzhong Zhang, WmhhbmdqaWFuemhvbmdAaWNkYy5jbg==
†These authors have contributed equally to this work
Disclaimer: All claims expressed in this article are solely those of the authors and do not necessarily represent those of their affiliated organizations, or those of the publisher, the editors and the reviewers. Any product that may be evaluated in this article or claim that may be made by its manufacturer is not guaranteed or endorsed by the publisher.
Research integrity at Frontiers
Learn more about the work of our research integrity team to safeguard the quality of each article we publish.