- Biosciences Division, Argonne National Laboratory, Argonne, IL, United States
Microbial communities that inhabit environments such as soil can contain thousands of distinct taxa, yet little is known about how this diversity is maintained in response to environmental perturbations such as changes in the availability of carbon. By utilizing aerobic substrate arrays to examine the effect of carbon amendment on microbial communities taken from six distinct environments (soil from a temperate prairie and forest, tropical forest soil, subalpine forest soil, and surface water and soil from a palustrine emergent wetland), we examined how carbon amendment and inoculum source shape the composition of the community in each enrichment. Dilute subsamples from each environment were used to inoculate 96-well microtiter plates containing triplicate wells amended with one of 31 carbon sources from six different classes of organic compounds (phenols, polymers, carbohydrates, carboxylic acids, amines, amino acids). After incubating each well aerobically in the dark for 72 h, we analyzed the composition of the microbial communities on the substrate arrays as well as the initial inocula by sequencing 16S rRNA gene amplicons using the Illumina MiSeq platform. Comparisons of alpha and beta diversity in these systems showed that, while the composition of the communities that grow to inhabit the wells in each substrate array diverges sharply from that of the original community in the inoculum, these enrichment communities are still strongly affected by the inoculum source. We found most enrichments were dominated by one or several OTUs most closely related to aerobes or facultative anaerobes from the Proteobacteria (e.g., Pseudomonas, Burkholderia, and Ralstonia) or Bacteroidetes (e.g., Chryseobacterium). Comparisons within each substrate array based on the class of carbon source further show that the communities inhabiting wells amended with a carbohydrate differ significantly from those enriched with a phenolic compound. Selection therefore seems to play a role in shaping the communities in the substrate arrays, although some stochasticity is also seen whereby several replicate wells within a single substrate array display strongly divergent community compositions. Overall, the use of highly parallel substrate arrays offers a promising path forward to study the response of microbial communities to perturbations in a changing environment.
Introduction
From the soil under our feet to the deepest sedimentary basins, microbial life inhabits nearly every environment on Earth (Whitman et al., 1998). The abundance and activity of the individual populations that comprise these communities change dynamically in response to changes in their local environment (Nemergut et al., 2013). The composition of microbial communities has been linked to specific parameters like water chemistry in environments such as lakes (Youngblut et al., 2014), streams (Zeglin, 2015), wetlands (Baldwin et al., 2006; Peralta et al., 2010; Dalcin Martins et al., 2017), and aquifers (Flynn et al., 2013; Hug et al., 2015; Kirk et al., 2015). In seawater, for example, the abundance of individual populations of bacteria have been shown to oscillate in sync with changes in light, temperature, and salinity (Eren et al., 2013; Ottesen et al., 2014). Soil, however, possesses such extreme physical, chemical, and biological heterogeneity that understanding the environmental forces that shape the structure and function of microbial communities there remains an outstanding challenge (Tiedje et al., 1999; Roesch et al., 2007; O'Brien et al., 2016; Bailey et al., 2017).
As the number of taxonomic groups within a particular soil often exceeds several thousand distinct clades, there is considerable interest in using simplified microbial communities to test hypotheses related to soil ecology. By “minimizing” a native microbial community from soil or elsewhere through enrichment in the laboratory, noise from the myriad co-existing metabolic networks and structural heterogeneities present in the parent environment can be pared down to focus on a particular process of interest, allowing the power of modern omics technology to be brought to bear on specific questions in microbial ecology (Prosser, 2015). This microcosm approach is frequently used to examine a subset of a native community such as sulfate reducers (Raskin et al., 1996; Kirk et al., 2013; Kwon et al., 2014), denitrifiers (Laverman et al., 2010; Kraft et al., 2014), or organisms capable of degrading specific compounds of interest (Brennan et al., 2006; Sutton et al., 2013; Luo et al., 2014; Onesios-Barry et al., 2014). Conducting such experiments as high-throughput, parallel replicates across a broad variety of environments has the potential to provide greater insight into how microbial communities respond to changing conditions.
Physiological profiling using microtiter plates has been frequently used as a method of examining the functional diversity of mixed microbial communities by monitoring the production of NADH using a redox-active dye (Bochner, 1989; Bochner et al., 2001). This approach allows the utilization of an array of carbon compounds by microbial communities to be monitored in parallel using 96-well plates. These substrate arrays are frequently used to test the metabolic capabilities of the microbiome inhabiting soil, water, and other environments (Bartscht et al., 1999; King, 2003; Weber and Legge, 2009; Gryta et al., 2014; Zhang Y. et al., 2014). Given that the composition of the communities that grow from the inocula in these arrays is rarely, if ever, characterized directly, the extent to which the “active” populations utilizing a particular substrate are representative of the community at large remains unclear (Konopka et al., 1998; Haack et al., 2004). Substrate arrays also offer an opportunity to study in parallel the response of a single inoculum to the addition of a wide array of nutrients.
In this study, we employed these substrate arrays as mini-bioreactors to monitor the response of microbial communities from six distinct environments to enrichment on 31 individual carbon compounds. We hypothesize that the fractionation of the complex natural communities will be more influenced by the nature of the carbon source utilized and select for a specific subset of microorganism(s) regardless of source environment. To this end, we selected as inocula soil, water and sediment from a variety of terrestrial environments including a wetland, a grassland, and three types of forest (temperate, subalpine, and tropical). We sought to test (A) the extent to which communities enriched in these substrate arrays are representative of the initial community as a whole, (B) the degree to which the initial structure of a community determines its response to a press disturbance (increase in nutrient levels) for different classes of environmentally-relevant (Hitzl et al., 1997) carbon compounds and (C) whether the composition of the communities that grow to inhabit the substrate arrays are more influenced by the composition of the inoculum or the type of carbon source upon which they are enriched.
Materials and Methods
Experimental Setup
Substrate array experiments were conducted by inoculating 96-well plates with soil suspensions or natural waters from six distinct environments. For these substrate arrays we chose the EcoPlate array (Biolog, Hayward, CA). Each well of this substrate array contains a proprietary minimal media (see Bochner et al., 2001) as well as one of 31 carbon sources representing five distinct categories: amines, amino acids, carbohydrates, polymers, and phenolic compounds (Table 1). While an exact formulation of the media is not publicly available, the manufacturer's documentation states that medium components are present at concentration of 2–20 mM for the carbon source, 1–5 mM for N, 0.1–1 mM for P, 0.1–1 mM for S, and <2 μM of a vitamin solution. A tetrazolium dye that reacts irreversibly with NADH is also present in each well, forming a distinct purple color in the presence of actively respiring cells. The intensity of this color change is proportional to the amount of NADH produced and was quantified by measuring absorption at 592 nm using a plate reader to approximate the level of metabolic activity (Bochner, 1989).
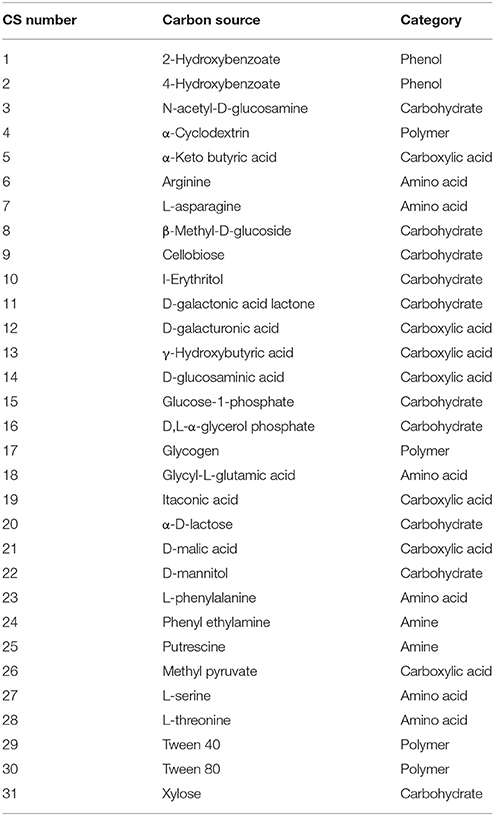
Table 1. List of 31 carbon substrates present in substrate arrays (one substrate per well, three replicate wells per substrate).
Given the spatial heterogeneity that can exist in even closely adjacent soil environments (O'Brien et al., 2016), we chose as inocula samples collected across several scales of geographic separation. Soil from a temperate climate was collected from a restored prairie grassland (FLP) and an adjacent forest (FLF) on site at the Fermi National Accelerator Laboratory (Fermilab) in Batavia, Illinois, USA (41.842888, −88.264180). Surface water (AWW) and soil (AWS) was obtained from a palustrine emergent wetland populated by Typha and Phragmites spp. on site at Argonne National Laboratory in Lemont, Illinois, USA (41.710022, −87.986241). Tropical forest soil (CRP14) was obtained from a Caribbean lowland rainforest (10.183333, −84.666667) within the EARTH University Forest Reserve in Costa Rica (Alvarez-Clare et al., 2013). Subalpine forest soil (SodaSpr) was taken from a pine forest near Soda Springs, California, USA (39.306120, −120.381295) at an elevation of 2,063 m. Soil samples were taken during the summer by removing ~100 g of soil to a depth of 5 cm with a clean, ethanol-sterilized hand trowel after clearing off any surface litter. Aquatic samples were taken at the water's surface using a sterile, 1 L container. Samples were used to inoculate substrate arrays within 72 h of collection to minimize any potential long-term storage effects.
A conceptual diagram of our experimental design is shown in Figure 1. Solid suspensions used to inoculate the bioreactors were prepared by adding 1 g of soil to 5 mL of sterile, DNA-free ultrapure water (HyClone HyPure molecular grade, Thermo Scientific). Suspensions were then homogenized using an ultrasonic dismembrator at low intensity for 30 s, breaking up any aggregates and removing bacteria from organic and mineral particles. The slurry was then diluted 1:100 (v/v) by adding additional sterile water and dispensed into a substrate array (150 μL per well). When using an aqueous medium as an inoculum in the case of the wetland surface water, the inoculum was amended directly to the array without dilution. Triplicate subsamples of the inocula were set aside and frozen immediately at −80°C. After 72 h of incubation at 30°C, 100 μL aliquots were taken from each well of the substrate array and stored at −80°C until analyzed by sequencing 16S rRNA gene amplicons.
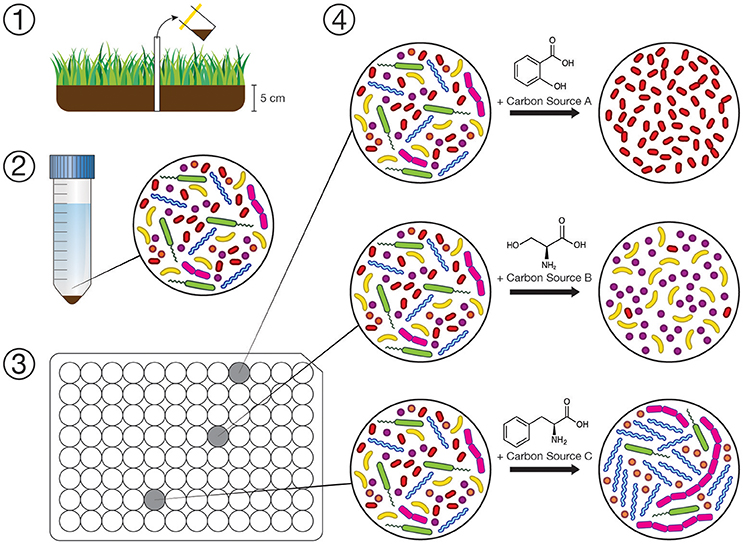
Figure 1. Conceptual diagram of our experimental workflow. (1) Collection of inocula for substrate arrays. (2) Dilute suspension of soil/water microbes prepared within 72 h. (3) Substrate arrays where each well is amended with one of 31 individual carbon sources (in triplicate), inoculated with suspension (1:100 m/v dilution), and incubated for 72 h. (4) Changes in composition of microbial communities in the substrate array is assayed by sequencing 16S rRNA gene amplicons on the Illumina MiSeq platform.
Molecular Microbiology and Bioinformatics
DNA was extracted from both environmental inocula and aliquots from the substrate arrays using MO BIO PowerSoil kits following the manufacturer's instructions. The concentration of DNA extracted was quantified using Quant-iT PicoGreen dsDNA assay (Invitrogen). 16S rRNA genes from each of these samples were amplified by the polymerase chain reaction (PCR) using a primer set (515F-806R) targeting the V4 region of this gene in both bacteria and archaea (Bates et al., 2011; Caporaso et al., 2011). These primers were barcoded to allow for sample multiplexing on the Illumina MiSeq (Caporaso et al., 2012). PCR reactions were carried out using 5 PRIME MasterMix (Gaithersburg, MD). PCR conditions used an initial denaturation step of 95°C for 3 min followed by 35 cycles of 95°C for 30 s, 55°C for 45 s, then 72°C for 1.5 min and finalized by a single extension step 72°C for 10 min. Pooled product for each sample was then quantified using the PicoGreen assay. Primer dimers were removed using the UltraClean PCR Clean-Up Kit (MO BIO Laboratories, Inc.) and the amount of DNA in each sample was normalized to a final concentration of 2 ng μL−1.
Paired-end amplicons (151 × 12 × 151 bp) were sequenced on an Illumina MiSeq at the Environmental Sample Preparation and Sequencing Facility at Argonne National Laboratory following procedures described in Caporaso et al. (2012). In all cases, sequencing workflows included both experimental and PCR/library preparation control blanks that failed to generate sequencing libraries of sufficient quality to provide any usable sequences. These controls were put through the same sequencing pipeline as the samples, but consistently failed to generate usable sequences. Any apparent sequences that were produced from these controls were all eliminated during the sequence screening process and likely arose from the formation of primer dimers or mispriming, rather than contamination.
A total of 19.0 million paired-end sequences were generated from 594 samples (576 substrate array samples and six triplicate inocula samples) for a depth of 32,552 ± 20,696 sequences per amplicon library. Paired-end reads were joined using PEAR (Zhang J. et al., 2014), and combined libraries were processed together using QIIME version 1.9.1 (Caporaso et al., 2010b). Poor-quality sequences were discarded based on Phred score, primer mismatches, divergence from expected amplicon length (253 base pairs) using the default settings in QIIME (split_libraries_fastq.py). Sequences were clustered into de novo operational taxonomic units (OTUs) at 97% similarity using QIIME's pick_de_novo_otus.py command. Singleton OTUs (defined as OTUs represented by only a single representative sequence across all samples) were discarded. Representative sequences from each OTU were aligned using PyNAST (Caporaso et al., 2010a) and classified according to the Greengenes taxonomy (version gg_13_8; McDonald et al., 2012). A phylogenetic tree of representative sequences was constructed with FastTree (Price et al., 2010) and used to calculate UniFrac distances between each sample as a measure of beta diversity (Hamady et al., 2009; Lozupone et al., 2011). Beta diversity was visualized in R using non-metric multidimensional scaling (NMDS) using the phyloseq package (McMurdie and Holmes, 2013). Analysis of similarity (ANOSIM) was calculated between groups of samples using Primer-7 (Clarke and Warwick, 2001). The differential abundance of specific OTUs was calculated in R using the package edgeR (Robinson et al., 2010) implemented in phyloseq. Significance testing for edgeR was done with tagwise tests using the exact negative binomial test (Robinson and Smyth, 2008) and a False Discovery Rate (FDR; Benjamini-Hochberg) significance threshold of 0.05 (Benjamini and Hochberg, 1995). Alpha diversity calculations (Shannon index of diversity) for each sample were made in R using phyloseq. Raw sequence data is freely available to download over the internet through the Argonne Scientific Publications portal and can be accessed using the Digital Object Identifier (DOI) 10.17038/1371460.
Results
We observed that, following incubation for 72 h at 30°C, the structures of the microbial communities in the wells of each substrate array deviated sharply from the initial composition of the inoculum. As shown in Figure 2, the average alpha diversity as measured by the Shannon index decreased sharply in the enrichments of all six substrate arrays, falling substantially below the average diversity of the inocula. Comparisons using the Mann-Whitney U-test between the Shannon indices of the inoculum samples and those of the substrate array samples for each environment showed a significant decrease (P < 0.005) in diversity for all six environments [AWS (0.003); AWW (0.003); CRP14 (0.003); FLF (0.003); FLP (0.003); SodaSpr (0.004)].
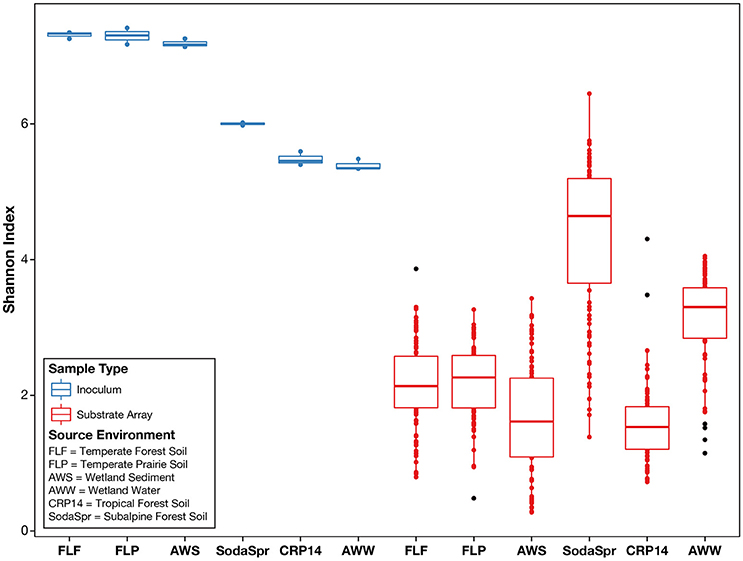
Figure 2. Alpha diversity of environmental inocula and EcoPlate enrichments as measured by the Shannon index of diversity. On average, the total diversity of environmental communities decreases substantially following incubation in the substrate array, regardless of carbon source.
Beta diversity comparisons using NMDS analysis found large differences in the composition of these communities compared to the inoculum based on the weighted UniFrac distance (Figure S1). While the microbial communities in each well of the substrate array diverged from the parent material, they differed substantially from one another as well (Figure 3). The degree of differences between substrate arrays largely recapitulate the observed differences between inocula (Figure S2), with one notable exception. The most divergent communities are from substrate arrays inoculated with soil from the SodaSpr and CRP14 sites. These enrichments differ substantially both from the arrays inoculated with material from temperate environments (AWW, AWS, FLF, and FLP) and from each other. Within those inoculated with materials from Illinois, the FLF and FLP substrate array communities overlap almost entirely. AWS and AWW communities are more separated from each other, but still cluster together with the enrichments inoculated with other Illinois materials (Figure 3). Comparing measurements of growth (qPCR quantification of 16S rRNA gene copies) and activity (color development of NADH-reactive tetrazolium dye) showed a generally positive trend between the two in the substrate arrays but no relationship was observed between either measurement and community composition (data not shown).
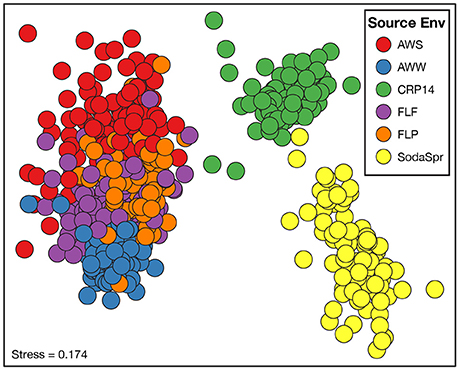
Figure 3. Non-metric multidimensional scaling (NMDS) analysis based on the weighted UniFrac distance metric showing the difference in microbial community structure between the substrate array enrichments.
Based on ANOSIM shown in Figure 4, the clustering by environment observed in the NMDS ordination shown in Figure 3 is statistically significant for pairwise comparisons between nearly all six substrate arrays. The CRP14 communities differ the most from the other substrate arrays, with R-values (RANOSIM) ranging from 0.685 to 0.876 (p < 0.0001%). An RANOSIM > 0.75 indicates two groups of microbial communities are almost entirely distinct, while a value between 0.25 and 0.75 indicates some degree of overlap (Ramette, 2007). Values of RANOSIM < 0.25 indicate the two groups of communities being compared are considered barely separable. Based on this metric, CRP14 enrichments are most similar to (although still mostly distinct from) the SodaSpr enrichments (RANOSIM = 0.685, p < 0.0001%). SodaSpr enrichments fall on a gradient of distinction between the tropical and temperate enrichments (RANOSIM = 0.377–0.685, p < 0.0001%), while the temperate soil enrichments show almost complete overlap with one another (RANOSIM = 0.136–0.288, p < 0.0001%). The two wetland substrate arrays (AWW and AWS) are distinct from one another but share some overlap (RANOSIM = 0.408, p < 0.0001%).
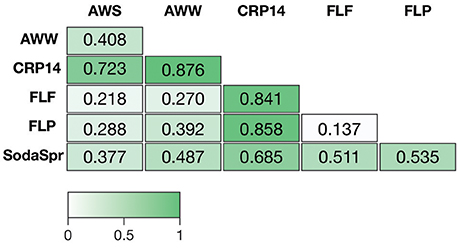
Figure 4. Analysis of similarity (ANOSIM) R-values showing differences in microbial community composition between each of the six substrate arrays. The value of p for all comparisons was <0.0001%.
Inspection of the most abundant organisms in each substrate arrays reveals that many of the enrichments in each substrate array are inhabited by one or several dominant taxa (Figure 5). These taxa, of which OTUs classified as Pseudomonas, Ralstonia, the family (fa.) Enterobacteriaceae, Burkholderia, and Agrobacterium are the most abundant, are not the most abundant taxa in the inocula. Sequences classified in the genus Pseudomonas account for 36% of all sequences across all six substrate arrays, but have an average abundance of only 0.26 ± 0.17% in the inocula. In five of the six substrate arrays (except CRP14), the relative abundance of Pseudomonas is on average 61–300 fold greater relative to the inoculum. In CRP14 Pseudomonas was only enriched four-fold, on average, and comprised <0.3% of the total diversity seen across all wells and was of >1% abundance in only three wells (3−11% in those wells). Others, like Ralstonia, fa. Enterobacteriaceae, Burkholderia, and Agrobacterium are considerably enriched in many if not most of the wells within individual substrate arrays, but are largely or entirely absent in others (Figure 5).
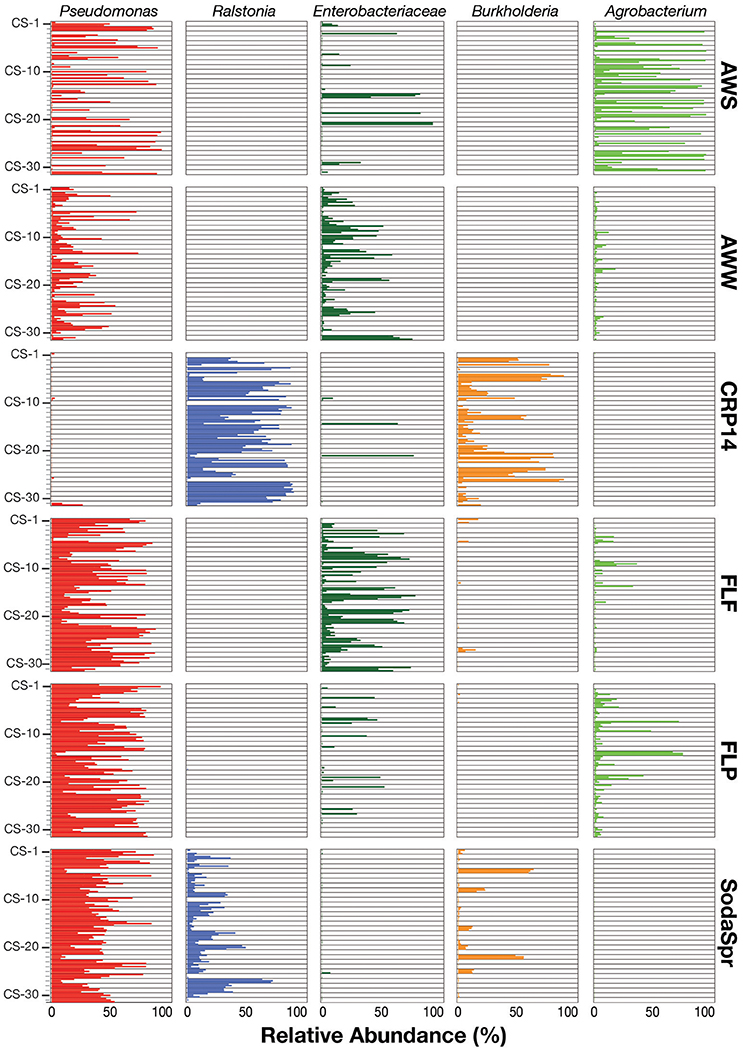
Figure 5. Relative abundance of the top five taxa (Pseudomonas, Ralstonia, Agrobacterium, Burkholderia, and Enterobacteriaceae) in the EcoPlate enrichments, with triplicate samples grouped by carbon amendment.
This pattern is also true for less broadly abundant taxa like Comamonas and the fa. Xanthomonadaceae. The distribution of all OTUs enriched or depleted (based on edgeR) in the substrate arrays compared to the inocula is shown in Figure 6. Overall, these data confirm the observational data shown in Figure 5, that the OTUs most abundant in the enrichments are largely members of the Proteobacteria (Pseudomonas, Ralstonia, Agrobacterium) and the Bacteroidetes (Chryseobacterium, Flavobacterium, Sphingobacterium, Pedobacter, and Wautersiella). OTUs classified as other phyla (e.g., Actinobacteria, Verrucomicrobia, and Acidobacteria) are considerably less abundant in the enrichments than they are in the original environments. The distribution of specific OTUs also varies within taxonomic groups. As seen in Figure 7, in four of the five substrate arrays where Pseudomonas is abundant (AWS, AWW, FLF, and FLP), a single OTU predominates (labeled OTU A). While OTU A is present in some enrichments inoculated with material from the SodaSpr site, a different OTU (OTU H) is much more dominant. Overall, of the 10 taxonomic groups that were found to be above 1% average abundance in the substrate arrays (Pseudomonas, Ralstonia, fa. Enterobacteriaceae, Agrobacterium, Burkholderia, fa. Aeromonadaceae, Chryseobacterium, fa. Xanthomonadaceae, Delftia, Comamonas), sequences from all these together comprise only 1.1–2.5% of the total diversity of the inocula in the five soil arrays and 8.0% of the diversity in the wetland water array.
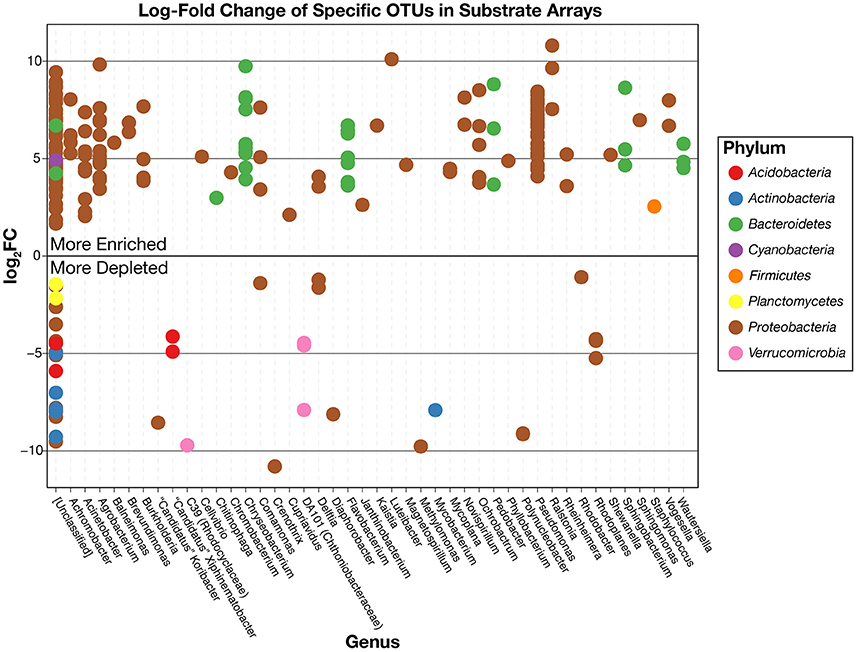
Figure 6. Log2-fold change in the relative abundance of individual OTUs in the substrate array enrichments relative to the original inoculum based on the edgeR package. Positively enriched OTUs are more abundant, on average, in the substrate arrays than in the inocula while negatively enriched OTUs are more abundant in the inocula. OTUs are separated horizontally by genus and colored by phylum.
Additional pairwise ANOSIM calculations were conducted to test for systematic differences in community composition between enrichments amended with a specific substrate category (amines, amino acids, carbohydrates, polymers, and phenolic compounds) and within each individual substrate itself (Supplementary Table 1). This test compared whether communities from across all six substrate arrays amended with a specific class of carbon compound (e.g., amines) were, on average, more similar to each other than to communities amended with a different class of compounds (e.g., carbohydrates). While no statistically significant correlations held observed across all six substrate arrays, several statistically significant ANOSIM-values were observed for several substrate categories within a given environment. Most significantly, comparing enrichments amended with a carbohydrate with those amended with a phenolic compound yield statistically significant ANOSIM values (RANOSIM = 0.483–0.597) in three of the six environmental enrichments: wetland water, temperate forest soil, and subalpine forest soil. None of the other within-environment comparisons yielded significant results for more than one of the six environmental sources.
Additional comparisons between phenolic compound-amended and carbohydrate-amended enrichments made using edgeR found that 42 OTUs above the chosen total variance threshold of 10−5 (McMurdie and Holmes, 2013) were differentially abundant (Figure 8). Overall, we found that OTUs within the phylum Bacteroidetes were generally more abundant in the phenolic-amended enrichments, while OTUs classified within the Proteobacteria were generally more abundant in carbohydrate-amended enrichments. OTUs classified in the genera Wautersiella, Comamonas, Sphingobacterium, Chitinophaga, Sphingobium, and Staphylococcus were more abundant in the phenolic-amended microcosms.
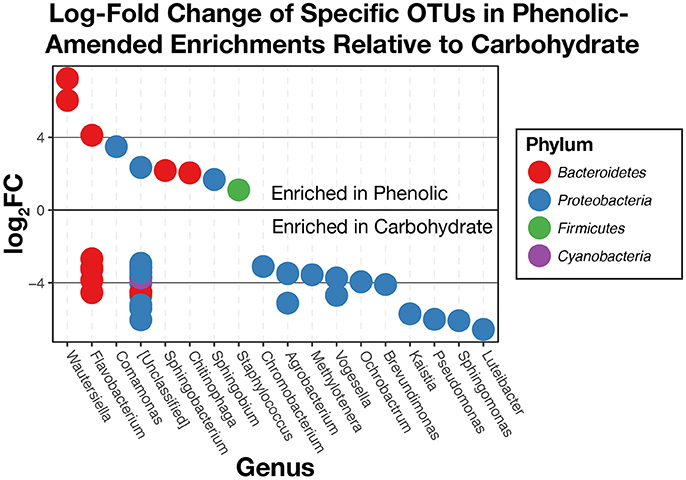
Figure 8. Log2-fold changes of differentially abundant operational taxonomic units (OTUs) in substrate arrays wells given phenolic substrates compared to carbohydrates. OTUs that are positively enriched are more abundant in phenolic enrichments. Those that are negatively enriched are more abundant in carbohydrate enrichments.
Discussion
The distinct communities of microorganisms that grow to dominate each enrichment within the substrate arrays bear the strong influence of the initial composition of the soil or water used as inoculum. As can be seen from the NMDS plot in Figure 3, the source of inoculum is by far the strongest signal in differentiating the microbial communities in these substrate arrays. The statistical significance of this result is confirmed by ANOSIM based on weighted UniFrac1, where the resulting R-values recapitulate the trends observed in the NMDS plot (Figure 4). Furthermore, these results show that, for the most part, the communities in the substrate arrays from the most divergent inocula [tropical (CRP14) and subalpine (SodaSpr) forest soil] remain divergent from those sourced from temperate soil inocula (AWS, FLP, FLF), which beta diversity comparisons indicate are more similar to each other (Figure S2). The exception is the wetland surface water (AWW), which Figure S2 shows has the most divergent community composition of the six inocula prior to enrichment. Following the 72-h incubation, however, the communities that arise in the substrate arrays inoculated with AWW material appear much more similar to the arrays inoculated with temperate soil than to those inoculated with soil from CRP14 or SodaSpr (Figure 3). This suggests that the generalists like Pseudomonas, fa. Enterobacteriaceae, and Agrobacterium that grow to dominate the substrate arrays share a similar baseline activity across all the temperate environments and are therefore able to be enriched to a similar degree in the arrays. Still, the smaller but still significant RANOSIM-values between some of the terrestrial enrichments (e.g., AWW and AWS) indicate the existence of smaller but significant differences between these environments.
Despite the strong influence of the initial inoculum, the overall structure of the microbial communities that grow to inhabit these substrate arrays bears little resemblance to that of the parent material, as measured by both alpha (Figure 2) and beta diversity (Figure S1). In most cases, the enrichments in each substrate array are primarily dominated by one or several OTUs most closely related to aerobic, heterotrophic generalists like Pseudomonas, Burkholderia, Ralstonia, fa. Enterobacteriaceae, and others (Figures 5, 6). Pseudomonas in particular dominated five of the six substrate arrays, although it was nearly absent from enrichments in the array inoculated with soil from site CRP14. Pseudomonas and its relatives are well-known as metabolic generalists and are frequently found in aerobic soil environments. Initially, “pseudomonads” were defined largely by “their most striking and ecologically significant group character… namely, the ability to use a wide variety of organic compounds as carbon and energy sources for aerobic growth” (Stanier et al., 1966). Numerous microcosm and enrichment studies from soils under aerobic conditions have found abundant growth of Pseudomonas (Greene et al., 2000; Eriksson et al., 2003; Ma et al., 2006), although these are often limited in scope to a few replicates or the utilization of a particular compound of interest.
An exception to this repeated occurrence of Pseudomonas was in the apparent swapping of Pseudomonas for Burkholderia and Ralstonia across the CRP14-inoculated enrichments. Like Pseudomonas, Burkholderia is a metabolic generalist well-distributed in soil environments (Cho and Tiedje, 2000; Salles et al., 2004; Janssen, 2006; Compant et al., 2008; Lauber et al., 2009; Stopnisek et al., 2014). Ralstonia also is a genus commonly found in soil environments (Janssen, 2006), although most studies of this genus focus on its role as soil-borne plant pathogen (Castillo and Greenberg, 2007; Leonard et al., 2017) with a wide distribution in warm and tropical climates (Genin and Boucher, 2004). Both Burkholderia and Ralstonia share many characteristics with Pseudomonas; both were at one time themselves classified as part of that genus (Yabuuchi et al., 1992, 1995) although they are now recognized as Betaproteobacteria while Pseudomonas is classified as a member of the Gammaproteobacteria. The abundance of these two genera in the CRP14-amended substrate array suggests an optimization of the community from the tropical forest system to the set of niches found in the source environment to the exclusion of Pseudomonas. There is likely some consistent factor associated with those niches, however, so that a “distinct generalist” is enriched consistently over time in one environment but not another.
When comparing the substrate array enrichments by the chemical class of substrate (amines, amino acids, carbohydrates, polymers, or phenolic compounds) rather than source environment, we found that significant differences in community composition exist primarily between those wells given a carbohydrate as a carbon source and those enriched with a phenolic compound (Figure 8). These differences were observed across three of the six environmental inocula: AWW, FLF, and SodaSpr, while none of the other pairwise comparisons between carbon source class showed a significant difference in more than one substrate array. Taken together, these results suggest that the co-occurrence of minimized community members within these three environments and grown on carbohydrates or phenolics are likely non-random associations. Like the most abundant taxa across the substrate arrays, most of the differentially-abundant OTUs (Figure 8) between phenolic- and carbohydrate-amended enrichments are most closely related to aerobic heterotrophs. Interestingly, the closest neighbors of several of the OTUs that are differentially abundant in phenolic-amended enrichments are organisms that are specifically known for degrading aromatic compounds. For example, members of the genus Comamonas have previously been isolated and studied based on their ability to degrade polycyclic aromatic hydrocarbons (Goyal and Zylstra, 1996) while isolated members of the genus Sphingobium are known for the ability to degrade phenolic compounds such as nonylphenols (Ushiba et al., 2003) and chlorophenols (Cai and Xun, 2002) as well as other aromatic hydrocarbons (Cunliffe and Kertesz, 2006). Carbohydrate-amended enrichments were more enriched with OTUs classified as Luteibacter, Sphingomonas, Pseudomonas, Kaistia, Brevundimonas, Ochrobactrum, Vogesella, and others. Unsurprisingly, known isolates from these groups are primarily aerobic heterotrophs with wide metabolic diversity, including the ability to grow on carbohydrates as an energy source (Lessie and Phibbs, 1984; Lebuhn et al., 2000; Im et al., 2004; de Boer et al., 2007) and are commonly found in a variety of terrestrial environments (Fredrickson et al., 1999; Cho and Tiedje, 2000; Spiers et al., 2000; Lauber et al., 2009). Despite being more enriched in carbohydrate-amended wells, Sphingomonas spp. have also shown the ability to grow on aromatic compounds (Fredrickson et al., 1995).
Taken together, these findings provide substantial insight into how microbial communities respond to changes in nutrient conditions. Microbial communities, like all communities of living organisms, are thought to be shaped by two types of ecological factors: those that are more deterministic are dubbed “niche” while those that are more stochastic are “neutral” (Vellend, 2010; Stegen et al., 2012). Niche factors that influence microbial community dynamics include environmental factors such as temperature or pH, the presence or absence of a particular nutrient (e.g., carbon, nitrogen, phosphorus, etc.) or the physical structure of the environment itself (e.g., the porosity or mineralogy of the soil matrix). Neutral forces, conversely, include random birth/death events, migrations of a particular population from one area to another, and other probabilistic events (Chase and Myers, 2011; Stegen et al., 2013). Evidence of the significance of both processes in shaping microbial communities has been observed throughout a variety of environments including soil, seawater, and aquifers (Gilbert et al., 2012; Shade et al., 2012; Handley et al., 2014; Graham et al., 2017). Understanding the relative importance of these factors is of particular importance in microbial ecosystems, where physiologically-relevant gradients can occur on the scale of micrometers. This heterogeneity at the microscale can be found in biofilms (Battin et al., 2016), soil pores (Bailey et al., 2013, 2017), and other sedimentary environments (Jakobsen, 2007), potentially imposing both niche and dispersal limitations at scales far smaller than that of the typical environmental sample. This poses a significant challenge to creating phylogenetically-resolved predictive models of microbial activity (Graham et al., 2016), particularly considering the breathtaking diversity of natural microbial communities (Howe et al., 2014; Hug et al., 2016).
While these experiments were not designed to explicitly test the extent to which niche and neutral factors lead to the composition of microbial communities within the substrate arrays, we see qualitative evidence of both processes shaping the overall composition of these communities. The degree to which either of these two processes influences the resultant minimized community, however, likely depends on the class of substrate. For example, niche selection clearly determines the overall trajectory of the response of the initial inoculum to changes in environmental conditions, as the organisms that grow to dominate the wells of the substrate arrays are aerobic heterotrophs, matching the oxic and carbon-rich conditions found in the wells. Further, niche selection appears evident in the distinction between carbohydrate- and phenolic-amended enrichments, as specific populations closely related to isolates known for degrading aromatic compounds become significantly more abundant in the presence of phenolic compounds (Figure 8).
Still, despite the fact that the source of inoculum is a significant determinant of the overall community structure (Figure 3), probabilistic events still appear to control some of the finer aspects of the composition of the substrate array communities. The extent to which triplicate wells on the same substrate array ended up with the same community composition varied depending on both the substrate and the population. For example, when populations classified as Burkholderia became abundant in wells inoculated with soil from the SodaSpr site, the abundance was observed uniformly across all three replicates (Figure 6). Conversely for some of carbon sources in wells amended with AWS soil, two of the three triplicates contained >90% Pseudomonas while the third well was similarly dominated by sequences classified as either fa. Enterobacteriaceae or Agrobacterium (Figure 6). Because the inocula were rigorously diluted, dispersed, and homogenized prior to being added to the substrate arrays, it appears unlikely that this is an artifact of sample preparation. Instead, stochasticity and imperceptible differences in the initial inoculum likely play at least some role in determining the overall trajectory of change in community composition. This has been seen elsewhere, as in Kwon et al.'s (2016) observation of two distinct mineralogical and microbiological trajectories in replicate microcosms amended with glucose and ferric iron.
The extent to which the communities within the substrate arrays represent the environments from which they were enriched depends upon how one looks at the question. In one sense, the communities in the substrate arrays retain components (populations) of the initial community as evidenced by the clustering by environment observed in Figure 3. However, the composition of these communities bears little resemble to that of the source inoculum when compared by measures of alpha (Figure 2) and beta (Figure S1) diversity. This is likely primarily due to the differences in the physicochemical makeup within the substrate arrays compared to that of the source environments. Whereas, soil and sedimentary environments display a complex, heterogeneous three-dimensional structure with dynamic changes in temperature, nutrient availability, and other variables, the substrate arrays are relatively homogeneous in comparison. In fact, the substrate arrays that shared the most taxa with their initial inoculum were those inoculated with wetland surface water, where shared taxa comprised 8.0% of the inoculum diversity compared to 1.1–2.5% in the five substrate arrays inoculated with soil suspensions. While still different in many respects, the wetland surface water does most closely resemble the aqueous environment of the substrate array wells.
The relative physical homogeneity of the substrate arrays compared to the source environments might be one explanation for the rapid dominance of one or few taxa in most of the substrate arrays over the 72-h incubation period. Previous work has shown that physical structure in laboratory microcosms enhances the stable coexistence of multiple interacting soil bacteria (Kim et al., 2008). Other work, however, has found many co-existing microbial populations can exist stably in homogeneous liquid medium (Kraft et al., 2014; Sutton et al., 2015; Chen et al., 2017). For example, recent work has shown that found that co-existence of bacterial isolates could be tied to mortality under different growth conditions within the same media (Friedman et al., 2017). While the goal of our experiments was not to replicate natural environments, it is clear from these results and others that both initial community composition and physical structure are likely both substantive controls on the composition of mixed environmental communities, as are intraspecific competition, mutualism, and other forms of organismal interaction (Konopka et al., 2015).
The parallelized enrichment strategy we employ here provides a framework to capture some of the underlying and potentially novel interactions between microbial community members found in soil and water in a high-throughput manner. This work also has the potential to inform the synthetic design of microbial communities, an area of considerable interest (Shou et al., 2007; Mee et al., 2014; Stenuit and Agathos, 2015). Current work in our laboratory is focused on testing the stability of these “minimal communities” in continuous culture systems with the hope of creating hybrid synthetic communities that are sourced directly from specific environments but streamlined in the laboratory. The isolation of a consistently reoccurring generalist provides an anchor within the community for genetic engineering. A variety of genetic tools are available for Pseudomonas and other taxa seen here, providing a potential platform for customization of minimal communities for industrial and biomedical applications. Moving forward, as desire increases to engineer microbial communities either by strain selection or outright genetic manipulation (Johnson et al., 2012; Lindemann et al., 2016), the use of highly parallelized microbial enrichments promises to provide novel insights that will allow us to predict with greater accuracy the interactions that contribute to emergent properties of microbial communities, such as community stability.
Author Contributions
DA, TF, and KK: designed the project; TF: collected samples and conducted the experiments; JK: extracted DNA and prepared sequencing libraries; SG and SO: carried out DNA sequencing; TF and DA: analyzed the data and wrote the manuscript; All authors reviewed and approved the final manuscript.
Funding
This material is based upon work supported by Laboratory Directed Research and Development (LDRD) funding from Argonne National Laboratory, provided by the Director, Office of Science, of the U.S. Department of Energy under contract DE-AC02-06CH11357.
Conflict of Interest Statement
The authors declare that the research was conducted in the absence of any commercial or financial relationships that could be construed as a potential conflict of interest.
Acknowledgments
The authors thank T. Beemer for his assistance with wetland classification. We also thank S. O'Brien, E. O'Loughlin, S. Alvarez-Clare, and P. Weisenhorn, for helpful discussions and insights that substantially improved this manuscript.
Supplementary Material
The Supplementary Material for this article can be found online at: https://www.frontiersin.org/articles/10.3389/fmicb.2017.02321/full#supplementary-material
Footnotes
1. ^ANOSIM was also performed using the Bray-Curtis similarity coefficient and exhibited similar trends (data not shown).
References
Alvarez-Clare, S., Mack, M. C., and Brooks, M. (2013). A direct test of nitrogen and phosphorus limitation to net primary productivity in a lowland tropical wet forest. Ecology 94, 1540–1551. doi: 10.1890/12-2128.1
Bailey, V. L., McCue, L. A., Fansler, S. J., Boyanov, M. I., DeCarlo, F., Kemner, K. M., et al. (2013). Micrometer-scale physical structure and microbial composition of soil macroaggregates. Soil Biol. Biochem. 65, 60–68. doi: 10.1016/j.soilbio.2013.02.005
Bailey, V. L., Smith, A. P., Tfaily, M., Fansler, S. J., and Bond-Lamberty, B. (2017). Differences in soluble organic carbon chemistry in pore waters sampled from different pore size domains. Soil Biol. Biochem. 107, 133–143. doi: 10.1016/j.soilbio.2016.11.025
Baldwin, D. S., Rees, G. N., Mitchell, A. M., Watson, G., and Williams, J. (2006). The short-term effects of salinization on anaerobic nutrient cycling and microbial community structure in sediment from a freshwater wetland. Wetlands 26, 455–464. doi: 10.1672/0277-5212(2006)26[455:TSEOSO]2.0.CO;2
Bartscht, K., Cypionka, H., and Overmann, J. (1999). Evaluation of cell activity and of methods for the cultivation of bacteria from a natural lake community. FEMS Microbiol. Ecol. 28, 249–259. doi: 10.1111/j.1574-6941.1999.tb00580.x
Bates, S. T., Berg-Lyons, D., Caporaso, J. G., Walters, W. A., Knight, R., and Fierer, N. (2011). Examining the global distribution of dominant archaeal populations in soil. ISME J. 5, 908–917. doi: 10.1038/ismej.2010.171
Battin, T. J., Besemer, K., Bengtsson, M. M., Romani, A. M., and Packmann, A. I. (2016). The ecology and biogeochemistry of stream biofilms. Nat. Rev. Microbiol. 14, 251–263. doi: 10.1038/nrmicro.2016.15
Benjamini, Y., and Hochberg, Y. (1995). Controlling the false discovery rate: a practical and powerful approach to multiple testing. J. R. Stat. Soc. B 57, 289–300.
Bochner, B. R. (1989). Sleuthing out bacterial identities. Nature 339, 157–158. doi: 10.1038/339157a0
Bochner, B. R., Gadzinski, P., and Panomitros, E. (2001). Phenotype microarrays for high-throughput phenotypic testing and assay of gene function. Genome Res. 11, 1246–1255. doi: 10.1101/gr.186501
Brennan, R. A., Sanford, R. A., and Werth, C. J. (2006). Chitin and corncobs as electron donor sources for the reductive dechlorination of tetrachloroethene. Water Res. 40, 2125–2134. doi: 10.1016/j.watres.2006.04.011
Cai, M., and Xun, L. (2002). Organization and regulation of pentachlorophenol-degrading genes in Sphingobium chlorophenolicum ATCC 39723. J. Bacteriol. 184, 4672–4680. doi: 10.1128/JB.184.17.4672-4680.2002
Caporaso, J. G., Bittinger, K., Bushman, F. D., DeSantis, T. Z., Andersen, G. L., and Knight, R. (2010a). PyNAST: a flexible tool for aligning sequences to a template alignment. Bioinformatics 26, 266–267. doi: 10.1093/bioinformatics/btp636
Caporaso, J. G., Kuczynski, J., Stombaugh, J., Bittinger, K., Bushman, F. D., Costello, E. K., et al. (2010b). QIIME allows analysis of high-throughput community sequencing data. Nat. Methods 7, 335–336. doi: 10.1038/nmeth.f.303
Caporaso, J. G., Lauber, C. L., Walters, W. A., Berg-Lyons, D., Huntley, J., Fierer, N., et al. (2012). Ultra-high-throughput microbial community analysis on the Illumina HiSeq and MiSeq platforms. ISME J. 6, 1621–1624. doi: 10.1038/ismej.2012.8
Caporaso, J. G., Lauber, C. L., Walters, W. A., Berg-Lyons, D., Lozupone, C. A., Turnbaugh, P. J., et al. (2011). Global patterns of 16S rRNA diversity at a depth of millions of sequences per sample. Proc. Natl. Acad. Sci. U.S.A. 108, 4516–4522. doi: 10.1073/pnas.1000080107
Castillo, J. A., and Greenberg, J. T. (2007). Evolutionary dynamics of Ralstonia solanacearum. Appl. Environ. Microbiol. 73, 1225–1238. doi: 10.1128/AEM.01253-06
Chase, J. M., and Myers, J. A. (2011). Disentangling the importance of ecological niches from stochastic processes across scales. Philos. Trans. R. Soc. B Biol. Sci. 366, 2351–2363. doi: 10.1098/rstb.2011.0063
Chen, G., Kleindienst, S., Griffiths, D. R., Mack, E. E., Seger, E. S., and Löffler, F. E. (2017). Mutualistic interaction between dichloromethane- and chloromethane-degrading bacteria in an anaerobic mixed culture. Environ. Microbiol. 19, 4784–4796. doi: 10.1111/1462-2920.13945
Cho, J. C., and Tiedje, J. M. (2000). Biogeography and degree of endemicity of fluorescent Pseudomonas strains in soil. Appl. Environ. Microbiol. 66, 5448–5456. doi: 10.1128/AEM.66.12.5448-5456.2000
Clarke, K. R., and Warwick, R. M. (2001). Change in Marine Communities: An Approach to Statistical Analysis and Interpretation. Plymouth: PRIMER-E, Ltd.
Compant, S., Nowak, J., Coenye, T., Clément, C., and Ait Barka, E. (2008). Diversity and occurrence of Burkholderia spp. in the natural environment. FEMS Microbiol. Rev. 32, 607–626. doi: 10.1111/j.1574-6976.2008.00113.x
Cunliffe, M., and Kertesz, M. A. (2006). Effect of Sphingobium yanoikuyae B1 inoculation on bacterial community dynamics and polycyclic aromatic hydrocarbon degradation in aged and freshly PAH-contaminated soils. Environ. Pollut. 144, 228–237. doi: 10.1016/j.envpol.2005.12.026
Dalcin Martins, P., Hoyt, D. W., Bansal, S., Mills, C. T., Tfaily, M., Tangen, B. A., et al. (2017). Abundant carbon substrates drive extremely high sulfate reduction rates and methane fluxes in prairie pothole wetlands. Glob. Change Biol. 23, 3107–3120. doi: 10.1111/gcb.13633
de Boer, W., Wagenaar, A. M., Klein Gunnewiek, P. J., and van Veen, J. A. (2007). In vitro suppression of fungi caused by combinations of apparently non-antagonistic soil bacteria. FEMS Microbiol. Ecol. 59, 177–185. doi: 10.1111/j.1574-6941.2006.00197.x
Eren, A. M., Maignien, L., Sul, W. J., Murphy, L. G., Grim, S. L., Morrison, H. G., et al. (2013). Oligotyping: differentiating between closely related microbial taxa using 16S rRNA gene data. Methods Ecol. Evol. 4, 1111–1119. doi: 10.1111/2041-210X.12114
Eriksson, M., Sodersten, E., Yu, Z., Dalhammar, G., and Mohn, W. W. (2003). Degradation of polycyclic aromatic hydrocarbons at low temperature under aerobic and nitrate-reducing conditions in enrichment cultures from northern soils. Appl. Environ. Microbiol. 69, 275–284. doi: 10.1128/AEM.69.1.275-284.2003
Flynn, T. M., Sanford, R. A., Ryu, H., Bethke, C. M., Levine, A. D., Ashbolt, N. J., et al. (2013). Functional microbial diversity explains groundwater chemistry in a pristine aquifer. BMC Microbiol. 13:146. doi: 10.1186/1471-2180-13-146
Fredrickson, J. K., Balkwill, D. L., Drake, G. R., Romine, M. F., Ringelberg, D. B., and White, D. C. (1995). Aromatic-degrading Sphingomonas isolates from the deep subsurface. Appl. Environ. Microbiol. 61, 1917–1922.
Fredrickson, J. K., Balkwill, D. L., Romine, M. F., and Shi, T. (1999). Ecology, physiology, and phylogeny of deep subsurface Sphingomonas sp. J. Ind. Microbiol. Biotechnol. 23, 273–283. doi: 10.1038/sj.jim.2900741
Friedman, J., Higgins, L. M., and Gore, J. (2017). Community structure follows simple assembly rules in microbial microcosms. 1:109. doi: 10.1038/s41559-017-0109
Genin, S., and Boucher, C. (2004). Lessons learned from the genome analysis of Ralstonia solanacearum. Annu. Rev. Phytopathol. 42, 107–134. doi: 10.1146/annurev.phyto.42.011204.104301
Gilbert, J. A., Steele, J. A., Caporaso, J. G., Steinbruck, L., Reeder, J., Temperton, B., et al. (2012). Defining seasonal marine microbial community dynamics. ISME J. 6, 298–308. doi: 10.1038/ismej.2011.107
Goyal, A. K., and Zylstra, G. J. (1996). Molecular cloning of novel genes for polycyclic aromatic hydrocarbon degradation from Comamonas testosteroni GZ39. Appl. Environ. Microbiol. 62, 230–236.
Graham, E. B., Crump, A. R., Resch, C. T., Fansler, S., Arntzen, E., Kennedy, D. W., et al. (2017). Deterministic influences exceed dispersal effects on hydrologically-connected microbiomes. Environ. Microbiol. 19, 1552–1567. doi: 10.1111/1462-2920.13720
Graham, E. B., Knelman, J. E., Schindlbacher, A., Siciliano, S., Breulmann, M., Yannarell, A., et al. (2016). Microbes as engines of ecosystem function: when does community structure enhance predictions of ecosystem processes? Front. Microbiol. 7:214. doi: 10.3389/fmicb.2016.00214
Greene, E. A., Kay, J. G., Jaber, K., Stehmeier, L. G., and Voordouw, G. (2000). Composition of soil microbial communities enriched on a mixture of aromatic hydrocarbons. Appl. Environ. Microbiol. 66, 5282–5289. doi: 10.1128/AEM.66.12.5282-5289.2000
Gryta, A., Frac, M., and Oszust, K. (2014). The application of the biolog ecoplate approach in ecotoxicological evaluation of dairy sewage sludge. Appl. Biochem. Biotechnol. 174, 1434–1443. doi: 10.1007/s12010-014-1131-8
Haack, S. K., Fogarty, L. R., West, T. G., Alm, E. W., McGuire, J. T., Long, D. T., et al. (2004). Spatial and temporal changes in microbial community structure associated with recharge-influenced chemical gradients in a contaminated aquifer. Environ. Microbiol. 6, 438–448. doi: 10.1111/j.1462-2920.2003.00563.x
Hamady, M., Lozupone, C., and Knight, R. (2009). Fast UniFrac: facilitating high-throughput phylogenetic analyses of microbial communities including analysis of pyrosequencing and PhyloChip data. ISME J. 4, 17–27. doi: 10.1038/ismej.2009.97
Handley, K. M., Wrighton, K. C., Miller, C. S., Wilkins, M. J., Kantor, R. S., Thomas, B. C., et al. (2014). Disturbed subsurface microbial communities follow equivalent trajectories despite different structural starting points. Environ. Microbiol. 17, 622–636. doi: 10.1111/1462-2920.12467
Hitzl, W., Rangger, A., Sharma, S., and Insam, H. (1997). Separation power of the 95 substrates of the BIOLOG system determined in various soils. FEMS Microbiol. Ecol. 22, 167–174. doi: 10.1111/j.1574-6941.1997.tb00368.x
Howe, A. C., Jansson, J. K., Malfatti, S. A., Tringe, S. G., Tiedje, J. M., and Brown, C. T. (2014). Tackling soil diversity with the assembly of large, complex metagenomes. Proc. Natl. Acad. Sci. U.S.A. 111, 4904–4909. doi: 10.1073/pnas.1402564111
Hug, L. A., Baker, B. J., Anantharaman, K., Brown, C. T., Probst, A. J., Castelle, C. J., et al. (2016). A new view of the tree of life. Nat. Microbiol. 1:16048. doi: 10.1038/nmicrobiol.2016.48
Hug, L. A., Thomas, B. C., Brown, C. T., Frischkorn, K. R., Williams, K. H., Tringe, S. G., et al. (2015). Aquifer environment selects for microbial species cohorts in sediment and groundwater. ISME J. 9, 1846–1856. doi: 10.1038/ismej.2015.2
Im, W.-T., Yokota, A., Kim, M.-K., and Lee, S.-T. (2004). Kaistia adipata gen. nov., sp. nov., a novel α-proteobacterium. J. Gen. Appl. Microbiol. 50, 249–254. doi: 10.2323/jgam.50.249
Jakobsen, R. (2007). Redox microniches in groundwater: a model study on the geometric and kinetic conditions required for concomitant Fe oxide reduction, sulfate reduction, and methanogenesis. Water Resour. Res. 43:W12S12. doi: 10.1029/2006WR005663
Janssen, P. H. (2006). Identifying the dominant soil bacterial taxa in libraries of 16S rRNA and 16S rRNA genes. Appl. Environ. Microbiol. 72, 1719–1728. doi: 10.1128/AEM.72.3.1719-1728.2006
Johnson, D. R., Goldschmidt, F., Lilja, E. E., and Ackermann, M. (2012). Metabolic specialization and the assembly of microbial communities. ISME J. 6, 1985–1991. doi: 10.1038/ismej.2012.46
Kim, H. J., Boedicker, J. Q., Choi, J. W., and Ismagilov, R. F. (2008). Defined spatial structure stabilizes a synthetic multispecies bacterial community. Proc. Natl. Acad. Sci. U.S.A. 105, 18188–18193. doi: 10.1073/pnas.0807935105
King, G. M. (2003). Contributions of atmospheric CO and hydrogen uptake to microbial dynamics on recent Hawaiian volcanic deposits. Appl. Environ. Microbiol. 69, 4067–4075. doi: 10.1128/AEM.69.7.4067-4075.2003
Kirk, M. F., Santillan, E. F. U., Sanford, R. A., and Altman, S. J. (2013). CO2-induced shift in microbial activity affects carbon trapping and water quality in anoxic bioreactors. Geochim. Cosmochim. Acta 122, 198–208. doi: 10.1016/j.gca.2013.08.018
Kirk, M. F., Wilson, B. H., Marquart, K. A., Zeglin, L. H., Vinson, D. S., and Flynn, T. M. (2015). Solute concentrations influence microbial methanogenesis in coal-bearing strata of the Cherokee basin, USA. Front. Microbiol. 6:1287. doi: 10.3389/fmicb.2015.01287
Konopka, A., Lindemann, S., and Fredrickson, J. (2015). Dynamics in microbial communities: unraveling mechanisms to identify principles. ISME J. 9, 1488–1495. doi: 10.1038/ismej.2014.251
Konopka, A., Oliver, L., and Turco, R. F. (1998). The use of carbon substrate utilization patterns in environmental and ecological microbiology. Microb. Ecol. 35, 103–115. doi: 10.1007/s002489900065
Kraft, B., Tegetmeyer, H. E., Meier, D., Geelhoed, J. S., and Strous, M. (2014). Rapid succession of uncultured marine bacterial and archaeal populations in a denitrifying continuous culture. Environ. Microbiol. 16, 3275–3286. doi: 10.1111/1462-2920.12552
Kwon, M. J., Boyanov, M. I., Antonopoulos, D. A., Brulc, J. M., Johnston, E. R., Skinner, K. A., et al. (2014). Effects of dissimilatory sulfate reduction on FeIII (hydr)oxide reduction and microbial community development. Geochim. Cosmochim. Acta 129, 177–190. doi: 10.1016/j.gca.2013.09.037
Kwon, M. J., O'Loughlin, E. J., Boyanov, M. I., Brulc, J. M., Johnston, E. R., Kemner, K. M., et al. (2016). Impact of organic carbon electron donors on microbial community development under iron- and sulfate-reducing conditions. PLoS ONE 11:e0146689. doi: 10.1371/journal.pone.0146689
Lauber, C. L., Hamady, M., Knight, R., and Fierer, N. (2009). Pyrosequencing-based assessment of soil pH as a predictor of soil bacterial community structure at the continental scale. Appl. Environ. Microbiol. 75, 5111–5120. doi: 10.1128/AEM.00335-09
Laverman, A. M., Garnier, J. A., Mounier, E. M., and Roose-Amsaleg, C. L. (2010). Nitrous oxide production kinetics during nitrate reduction in river sediments. Water Res. 44, 1753–1764. doi: 10.1016/j.watres.2009.11.050
Lebuhn, M., Achouak, W., Schloter, M., Berge, O., Meier, H., Barakat, M., et al. (2000). Taxonomic characterization of Ochrobactrum sp. isolates from soil samples and wheat roots, and description of Ochrobactrum tritici sp. nov. and Ochrobactrum grignonense sp. nov. Int. J. Syst. Evol. Microbiol. 50, 2207–2223. doi: 10.1099/00207713-50-6-2207
Leonard, S., Hommais, F., Nasser, W., and Reverchon, S. (2017). Plant–phytopathogen interactions: bacterial responses to environmental and plant stimuli. Environ. Microbiol. 19, 1689–1716. doi: 10.1111/1462-2920.13611
Lessie, T. G., and Phibbs, P. V. (1984). Alternative pathways of carbohydrate utilization in pseudomonads. Annu. Rev. Microbiol. 38, 359–388. doi: 10.1146/annurev.mi.38.100184.002043
Lindemann, S. R., Bernstein, H. C., Song, H. S., Fredrickson, J. K., Fields, M. W., Shou, W., et al. (2016). Engineering microbial consortia for controllable outputs. ISME J. 10, 2077–2084. doi: 10.1038/ismej.2016.26
Lozupone, C., Lladser, M. E., Knights, D., Stombaugh, J., and Knight, R. (2011). UniFrac: an effective distance metric for microbial community comparison. ISME J. 5, 169–172. doi: 10.1038/ismej.2010.133
Luo, F., Gitiafroz, R., Devine, C. E., Gong, Y., Hug, L. A., Raskin, L., et al. (2014). Metatranscriptome of an anaerobic benzene-degrading, nitrate-reducing enrichment culture reveals involvement of carboxylation in benzene ring activation. Appl. Environ. Microbiol. 80, 4095–4107. doi: 10.1128/AEM.00717-14
Ma, Y., Wang, L., and Shao, Z. (2006). Pseudomonas, the dominant polycyclic aromatic hydrocarbon-degrading bacteria isolated from Antarctic soils and the role of large plasmids in horizontal gene transfer. Environ. Microbiol. 8, 455–465. doi: 10.1111/j.1462-2920.2005.00911.x
McDonald, D., Price, M. N., Goodrich, J., Nawrocki, E. P., DeSantis, T. Z., Probst, A., et al. (2012). An improved Greengenes taxonomy with explicit ranks for ecological and evolutionary analyses of bacteria and archaea. ISME J. 6, 610–618. doi: 10.1038/ismej.2011.139
McMurdie, P. J., and Holmes, S. (2013). phyloseq: an R package for reproducible interactive analysis and graphics of microbiome census data. PLoS ONE 8:e61217. doi: 10.1371/journal.pone.0061217
Mee, M. T., Collins, J. J., Church, G. M., and Wang, H. H. (2014). Syntrophic exchange in synthetic microbial communities. Proc. Natl. Acad. Sci. U.S.A. 111, E2149–E2156. doi: 10.1073/pnas.1405641111
Nemergut, D. R., Schmidt, S. K., Fukami, T., O'Neill, S. P., Bilinski, T. M., Stanish, L. F., et al. (2013). Patterns and processes of microbial community assembly. Microbiol. Mol. Biol. Rev. 77, 342–356. doi: 10.1128/MMBR.00051-12
O'Brien, S. L., Gibbons, S. M., Owens, S. M., Hampton-Marcell, J., Johnston, E. R., Jastrow, J. D., et al. (2016). Spatial scale drives patterns in soil bacterial diversity. Environ. Microbiol. 18, 2039–2051. doi: 10.1111/1462-2920.13231
Onesios-Barry, K. M., Berry, D., Proescher, J. B., Sivakumar, I. K., and Bouwer, E. J. (2014). Removal of pharmaceuticals and personal care products during water recycling: microbial community structure and effects of substrate concentration. Appl. Environ. Microbiol. 80, 2440–2450. doi: 10.1128/AEM.03693-13
Ottesen, E. A., Young, C. R., Gifford, S. M., Eppley, J. M., Marin, R., Schuster, S. C., et al. (2014). Multispecies diel transcriptional oscillations in open ocean heterotrophic bacterial assemblages. Science 345, 207–212. doi: 10.1126/science.1252476
Peralta, A. L., Matthews, J. W., and Kent, A. D. (2010). Microbial community structure and denitrification in a wetland mitigation bank. Appl. Environ. Microbiol. 76, 4207–4215. doi: 10.1128/AEM.02977-09
Price, M. N., Dehal, P. S., and Arkin, A. P. (2010). FastTree 2 – approximately maximum-likelihood trees for large alignments. PLoS ONE 5:e9490. doi: 10.1371/journal.pone.0009490
Prosser, J. I. (2015). Dispersing misconceptions and identifying opportunities for the use of ‘omics’ in soil microbial ecology. Nat. Rev. Microbiol. 13, 439–446. doi: 10.1038/nrmicro3468
Ramette, A. (2007). Multivariate analyses in microbial ecology. FEMS Microbiol. Ecol. 62, 142–160. doi: 10.1111/j.1574-6941.2007.00375.x
Raskin, L., Rittmann, B. E., and Stahl, D. A. (1996). Competition and coexistence of sulfate-reducing and methanogenic populations in anaerobic biofilms. Appl. Environ. Microbiol. 62, 3847–3857.
Robinson, M. D., and Smyth, G. K. (2008). Small-sample estimation of negative binomial dispersion, with applications to SAGE data. Biostatistics 9, 321–332. doi: 10.1093/biostatistics/kxm030
Robinson, M. D., McCarthy, D. J., and Smyth, G. K. (2010). edgeR: a bioconductor package for differential expression analysis of digital gene expression data. Bioinformatics 26, 139–140. doi: 10.1093/bioinformatics/btp616
Roesch, L. F., Fulthorpe, R. R., Riva, A., Casella, G., Hadwin, A. K., Kent, A. D., et al. (2007). Pyrosequencing enumerates and contrasts soil microbial diversity. ISME J. 1, 283–290. doi: 10.1038/ismej.2007.53
Salles, J. F., van Veen, J. A., and van Elsas, J. D. (2004). Multivariate analyses of Burkholderia species in soil: effect of crop and land use history. Appl. Environ. Microbiol. 70, 4012–4020. doi: 10.1128/AEM.70.7.4012-4020.2004
Shade, A., Hogan, C. S., Klimowicz, A. K., Linske, M., McManus, P. S., and Handelsman, J. (2012). Culturing captures members of the soil rare biosphere. Environ. Microbiol. 14, 2247–2252. doi: 10.1111/j.1462-2920.2012.02817.x
Shou, W., Ram, S., and Vilar, J. M. (2007). Synthetic cooperation in engineered yeast populations. Proc. Natl. Acad. Sci. U.S.A. 104, 1877–1882. doi: 10.1073/pnas.0610575104
Spiers, A. J., Buckling, A., and Rainey, P. B. (2000). The causes of Pseudomonas diversity. Microbiology 146, 2345–2350. doi: 10.1099/00221287-146-10-2345
Stanier, R. Y., Palleroni, N. J., and Doudoroff, M. (1966). The aerobic pseudomonads: a taxonomic study. Microbiology 43, 159–271. doi: 10.1099/00221287-43-2-159
Stegen, J. C., Lin, X., Fredrickson, J. K., Chen, X., Kennedy, D. W., Murray, C. J., et al. (2013). Quantifying community assembly processes and identifying features that impose them. ISME J. 7, 2069–2079. doi: 10.1038/ismej.2013.93
Stegen, J. C., Lin, X., Konopka, A. E., and Fredrickson, J. K. (2012). Stochastic and deterministic assembly processes in subsurface microbial communities. ISME J. 6, 1653–1664. doi: 10.1038/ismej.2012.22
Stenuit, B., and Agathos, S. N. (2015). Deciphering microbial community robustness through synthetic ecology and molecular systems synecology. Curr. Opin. Biotechnol. 33, 305–317. doi: 10.1016/j.copbio.2015.03.012
Stopnisek, N., Bodenhausen, N., Frey, B., Fierer, N., Eberl, L., and Weisskopf, L. (2014). Genus-wide acid tolerance accounts for the biogeographical distribution of soil Burkholderia populations. Environ. Microbiol. 16, 1503–1512. doi: 10.1111/1462-2920.12211
Sutton, N. B., Atashgahi, S., Saccenti, E., Grotenhuis, T., Smidt, H., and Rijnaarts, H. H. (2015). Microbial community response of an organohalide respiring enrichment culture to permanganate oxidation. PLoS ONE 10:e0134615. doi: 10.1371/journal.pone.0134615
Sutton, N. B., van Gaans, P., Langenhoff, A. A., Maphosa, F., Smidt, H., Grotenhuis, T., et al. (2013). Biodegradation of aged diesel in diverse soil matrixes: impact of environmental conditions and bioavailability on microbial remediation capacity. Biodegradation 24, 487–498. doi: 10.1007/s10532-012-9605-2
Tiedje, J. M., Asuming-Brempong, S., Nüsslein, K., Marsh, T. L., and Flynn, S. J. (1999). Opening the black box of soil microbial diversity. Appl. Soil Ecol. 13, 109–122. doi: 10.1016/S0929-1393(99)00026-8
Ushiba, Y., Takahara, Y., and Ohta, H. (2003). Sphingobium amiense sp. nov., a novel nonylphenol-degrading bacterium isolated from a river sediment. Int. J. Syst. Evol. Microbiol. 53, 2045–2048. doi: 10.1099/ijs.0.02581-0
Vellend, M. (2010). Conceptual synthesis in community ecology. Q. Rev. Biol. 85, 183–206. doi: 10.1086/652373
Weber, K. P., and Legge, R. L. (2009). One-dimensional metric for tracking bacterial community divergence using sole carbon source utilization patterns. J. Microbiol. Methods 79, 55–61. doi: 10.1016/j.mimet.2009.07.020
Whitman, W. B., Coleman, D. C., and Wiebe, W. J. (1998). Prokaryotes: the unseen majority. Proc. Nat. Acad. Sci. U.S.A. 95, 6578–6583. doi: 10.1073/pnas.95.12.6578
Yabuuchi, E., Kosako, Y., Oyaizu, H., Yano, I., Hotta, H., Hashimoto, Y., et al. (1992). Proposal of Burkholderia gen. nov. and transfer of seven species of the genus Pseudomonas Homology Group II to the new genus, with the type species Burkholderia cepacia (Palleroni and Holmes 1981) comb. nov. Microbiol. Immunol. 36, 1251–1275. doi: 10.1111/j.1348-0421.1992.tb02129.x
Yabuuchi, E., Kosako, Y., Yano, I., Hotta, H., and Nishiuchi, Y. (1995). Transfer of two Burkholderia and an Alcaligenes species to Ralstonia gen. nov. Microbiol. Immunol. 39, 897–904. doi: 10.1111/j.1348-0421.1995.tb03275.x
Youngblut, N. D., Dell'Aringa, M., and Whitaker, R. J. (2014). Differentiation between sediment and hypolimnion methanogen communities in humic lakes. Environ. Microbiol. 16, 1411–1423. doi: 10.1111/1462-2920.12330
Zeglin, L. H. (2015). Stream microbial diversity in response to environmental changes: review and synthesis of existing research. Front. Microbiol. 6:454. doi: 10.3389/fmicb.2015.00454
Zhang, J., Kobert, K., Flouri, T., and Stamatakis, A. (2014). PEAR: a fast and accurate Illumina Paired-End reAd mergeR. Bioinformatics 30, 614–620. doi: 10.1093/bioinformatics/btt593
Keywords: microbial ecology, carbon cycling, soil microbiology, microcosm, enrichment culture
Citation: Flynn TM, Koval JC, Greenwald SM, Owens SM, Kemner KM and Antonopoulos DA (2017) Parallelized, Aerobic, Single Carbon-Source Enrichments from Different Natural Environments Contain Divergent Microbial Communities. Front. Microbiol. 8:2321. doi: 10.3389/fmicb.2017.02321
Received: 17 July 2017; Accepted: 10 November 2017;
Published: 28 November 2017.
Edited by:
Jorge L. M. Rodrigues, University of California, Davis, United StatesReviewed by:
Steven Singer, Lawrence Berkeley National Laboratory, United StatesLucas William Mendes, University of São Paulo, Brazil
Copyright © 2017 Flynn, Koval, Greenwald, Owens, Kemner and Antonopoulos. This is an open-access article distributed under the terms of the Creative Commons Attribution License (CC BY). The use, distribution or reproduction in other forums is permitted, provided the original author(s) or licensor are credited and that the original publication in this journal is cited, in accordance with accepted academic practice. No use, distribution or reproduction is permitted which does not comply with these terms.
*Correspondence: Theodore M. Flynn, dGZseW5uQGFubC5nb3Y=
Dionysios A. Antonopoulos, ZGlvbkBhbmwuZ292