- 1State Key Laboratory of Bioreactor Engineering and Institute of Applied Chemistry, East China University of Science and Technology, Shanghai, China
- 2Shanghai Collaborative Innovation Center for Biomanufacturing Technology, Shanghai, China
- 3School of Biological Sciences, The University of Hong Kong, Hong Kong, Hong Kong
Deep subsurface petroleum reservoir ecosystems harbor a high diversity of microorganisms, and microbial influenced corrosion is a major problem for the petroleum industry. Here, we used high-throughput sequencing to explore the microbial communities based on genomic 16S rDNA and metabolically active 16S rRNA analyses of production water samples with different extents of corrosion from a high-temperature oil reservoir. Results showed that Desulfotignum and Roseovarius were the most abundant genera in both genomic and active bacterial communities of all the samples. Both genomic and active archaeal communities were mainly composed of Archaeoglobus and Methanolobus. Within both bacteria and archaea, the active and genomic communities were compositionally distinct from one another across the different oil wells (bacteria p = 0.002; archaea p = 0.01). In addition, the sulfate-reducing microorganisms (SRMs) were specifically assessed by Sanger sequencing of functional genes aprA and dsrA encoding the enzymes adenosine-5′-phosphosulfate reductase and dissimilatory sulfite reductase, respectively. Functional gene analysis indicated that potentially active Archaeoglobus, Desulfotignum, Desulfovibrio, and Thermodesulforhabdus were frequently detected, with Archaeoglobus as the most abundant and active sulfate-reducing group. Canonical correspondence analysis revealed that the SRM communities in petroleum reservoir system were closely related to pH of the production water and sulfate concentration. This study highlights the importance of distinguishing the metabolically active microorganisms from the genomic community and extends our knowledge on the active SRM communities in corrosive petroleum reservoirs.
Introduction
Metal corrosion is a major problem for oil production systems, leading to serious economic and safety as well as human health problems (Koch et al., 2002; Guan et al., 2014). It is well-recognized that the majority of corrosion of oil pipelines is associated with microorganisms (Almahamedh et al., 2011). Microbial groups of sulfate-reducing microorganism (SRM), acid-producing fermentative microorganisms, metal-reducers and methanogens are frequently detected in oil transporting pipeline systems (Duncan et al., 2009; Stevenson et al., 2011; Liang et al., 2014). The presence of hydrocarbons and their degradation intermediates, such as volatile fatty acids, as electron donors and carbon sources, and the supply of sulfate from injection water as electron acceptors promote the proliferation of microorganisms in anoxic subsurface oil reservoirs and related oil drilling equipment and transport pipeline system (Biderre-Petit et al., 2011; Vigneron et al., 2016).
Bastin et al. (1926) first reported the presence of sulfate-reducing bacteria in oil field waters. Sulfate reduction can have a deleterious impact on crude oil quality by reservoir souring and biocorrosion of the oil infrastructures (Lewandowski and Beyenal, 2008). The SRM, encompassing members affiliated with four bacterial [Proteobacteria (class Deltaproteobacteria), Nitrospira, Firmicutes, Thermodesulfobacteria] and two archaeal phyla (Euryarchaeota, Crenarchaeota) are commonly considered to be main culprits of MIC under anaerobic conditions (Beech and Sunner, 2004; Enning and Garrelfs, 2013; Müller et al., 2014). SRM contribute to chemical microbial influenced corrosion (CMIC) by reducing sulfate to sulfide using organic electron donors such as organic acids (Dinh et al., 2004; Venzlaff et al., 2012). The produced sulfide can then react with carbon steel to form FeS, resulting in dissolution of metal iron and formation of hydrogen which is then also used by SRM as an electron donor (Voordouw et al., 2016). Recent studies showed that some SRM were capable of iron corrosion via electron extraction from Fe0 and the process is referred to as electrical microbial influenced corrosion (EMIC) (Enning et al., 2012; Enning and Garrelfs, 2013). Other microorganisms, such as metal-reducers, acid-producing fermentative microorganisms and methanogens may also contribute to corrosion through different biochemical processes (Zhang et al., 2003; Enning and Garrelfs, 2013; Kip and Veen, 2014; Usher et al., 2014). The acid-producing fermentative organisms and methanogens might indirectly increase corrosion through the production of organic acids or syntrophy with corrosion promoting microorganisms (Kip and Veen, 2014). Studies have found high numbers of methanogens associated with pitting corrosion of steel pipes (Uchiyama et al., 2010; Park et al., 2011). Furthermore, the anaerobic biodegradation of labile fuel components coupled with sulfate respiration greatly contributed to the biocorrosion of carbon steel (Liang et al., 2016). Moreover, it has been reported that thiosulfate-utilizing, sulfide-producing fermentative bacteria such as Anaerobaculum sp. were implicated with the biocorrosion of a high-temperature petroleum facility (Liang et al., 2014).
Recently, multiplex dsrA and dsrB amplicon sequencing approach by using new primers and mock community-optimized bioinformatics have been demonstrated to be adequate for monitoring the spatial distribution and temporal abundance dynamics of sulfite- and SRMs (Pelikan et al., 2015). The molecular approach to track SRM is primarily based on functional genes encoding the key selective enzymes including adenosine-5′-phosphosulfate (APS) reductase (Apr) (Meyer and Kuever, 2007) and dissimilatory sulfite reductase (Dsr) (Muyzer and Stams, 2008). The application of these two different genes (aprA and dsrA) is complementary for the characterization of sulfate-reducing communities and have been studied in different environmental conditions, such as marine sediments (Blazejak and Schippers, 2011), mangrove sediments (Varon-Lopez et al., 2014), and hydrothermal vents (Frank et al., 2013). The composition and activity of microbial communities may depend on a range of factors, including temperature, and availability of electron donors and acceptors (Duncan et al., 2009; Davidova et al., 2012). Accurate and more specific detection and characterization of microbial communities associated with oil souring and pipeline corrosion are crucial to the development of management strategies to minimize or prevent biocorrosion.
In this study, the diversity and composition of microbial community in production water of Jiangsu oil reservoir were explored by analysis of both 16S rDNA and 16S rRNA. In addition, potentially active SRM were also investigated by using the aprA and dsrA gene transcripts to gain insights for SRM. Three blocks (six different oil wells) with known reservoir souring and pipeline corrosion history were selected in this investigation for a better understanding of microbial communities and SRM in a high temperature and corrosive petroleum reservoir.
Materials and Methods
Sampling and Chemical Analysis
Production water samples were obtained on 19 November 2015 from three different blocks (six wells) of Jiangsu oilfield (Yangzhou, Jiangsu, China), including Block W2 (2-53, 2-71), W9 (9-18, 9-14) and W11 (11-7, 11-6). The depths of these wells ranged from 1499 to 2143 m below ground surface, with temperature from 66.2 to 79.6°C. Blocks W2 (2-53, 2-71) and W11 (11-7, 11-6) oil wells have been water-flooded for about 18 and 10 years, respectively. At W9 block, 9-14 has been water-flooded for about 15 years, while 9-18 has never been water-flooded. Corrosion rates of the wells are shown in Table 1.
Twenty liters of the production water from each production oil well were collected directly from the production valve at the well head into sterile bottle. Production water of each sample for RNA analysis was filtered through 0.1-μm-pore-size polycarbonate membrane filters (Whatman, United Kingdom). Samples were collected within 30 min and stored with a stop solution (95% ethanol, 5% Trizol) for RNA preservation. All samples were stored on ice and immediately transported back to laboratory for further analysis.
Cations and anions and volatile fatty acids in the water samples were quantified by Ion Chromatography (IC DX-600, Dionex, United States). The detailed description of the method used is available elsewhere (Li C.-Y. et al., 2016). The oil well-characteristics were obtained from Jiangsu Oilfield Company. The corrosion rate (at the oil well-temperature) was determined according to the weight loss method for 1 week. Briefly, carbon steel coupons (20#) were immersed in 250 ml serum bottles filled with each of the well-production water (70 ml) under anaerobic conditions. Serum bottles with 70 ml of filtered production water (by 0.22-μm-pore-size polycarbonate membranes) were used as a control. The bottles were closed with butyl stoppers and aluminum seals after purging with pure N2 to remove the O2 from the corrosion test. The samples were incubated at oil well-temperature in the dark (Okoro et al., 2014).
Detection and Enumeration of Bacterial Cells
The number of microorganisms in these samples was quantified after fluorescent staining with 4′-6-diamidino-2-phenylindole (DAPI). 5 ml of production water sample was stained with 25 μL of DAPI (5.0 mg mL-1) as described before (Purkamo et al., 2013). Stained samples were concentrated on a black 0.2 μm pore-size polycarbonate membrane filter (Millipore, United States) and rinsed twice with 1 ml of sterilized 0.9% NaCl and examined with an epifluorescence microscope (Olympus BX60, China). The number of cells was enumerated from 30 randomly chosen fields on the filter. The total cell number in the samples was then calculated based on filtering sample volume, the observed area of the filter and total surface area of the filter as described in Nyyssönen et al. (2012).
DNA, RNA Extraction, and cDNA Synthesis and Sequencing
Total DNA and RNA were extracted from each sample using the genomic DNA Kit (Axygen Biosciences, United States) and High Pure RNA Isolation Kit (Roche, United States) according to the manufacturer’s instructions, respectively. Genomic DNA was removed during the RNA extraction with RNase-Free DNase reagents (TakaRa Bio, Japan). Total extracted RNA was checked for residual genomic DNA by performing a polymerase chain reaction (PCR) using the primers 8F (5′-AGAGTTTGATYMTGGCTCAG-3′) and 805R (5′-GACTACCAGGGTATCTAATCC-3′) to ensure that no amplified product was detectable after running on 1.5% agarose gels (Savage et al., 2010). Total RNA was reverse-transcribed to cDNA using M-MLV reverse transcriptase and random hexamer primers (Sangon Biotech, China). The quality and quantity of cDNA was determined using a NanoDrop2000 spectrophotometer (Thermo Fisher Scientific, United States). All DNA and cDNA were stored at -80°C before PCR amplification and sequencing were performed sequentially.
The bacterial hypervariable regions V4–V5 of the 16S rRNA genes were amplified using the primers 515F (5′-GTGCCAGCMGCCGCGG-3′) and 907R (5′-CCGTCAATTCMTTTRAGTTT-3′) containing a barcode sequences (Ren et al., 2014). Archaeal libraries were built using the primers 344F (5′-ACGGGGYGCAGCAGGCGCGA-3′) and 915R (5′-GTGCTCCCCCGCCAATTCCT-3′) (Ohene-Adjei et al., 2007). PCRs were carried out in triplicate of 25-μl reaction volume using 30–40 ng of template DNA or cDNA. The amplifications were run under the following thermocycling conditions: initial denaturation at 94°C for 3 min; 30 (bacteria) or 42 (archaea) cycles of denaturation at 94°C for 30 s, annealing at 55°C (bacteria) or 58°C (archaea) for 30 s, elongation at 72°C for 30 s, and a final extension at 72°C for 10 min. Triplicate reactions were pooled for each sample prior to purification with AxyPrep DNA Kit (Axygen, United States) and quantification with QuantiFluorTM -ST (Promega, United States). Paired-end sequencing was performed on Illumina Miseq platform (Majorbio, China).
Sequences were quality filtered and processed using the UPARSE (Edgar, 2013) and QIIME software package (Caporaso et al., 2010). Paired-end reads were first merged by using FLASH (minimum overlap 10 bp, maximum mismatch 0.25) (Magoč and Salzberg, 2011). PCR primers were detected and trimmed using Cutadapt (Martin, 2011) allowing for one mismatch. Reads not matching the primers or with read lengths below 200 bp were discarded. Trimmed reads were quality-filtered using USEARCH fastq_filter function with a maximum predicted sequences errors rate (maxee) of one (Edgar, 2013). High-quality sequences were aligned against the SILVA reference database and clustered into operational taxonomic units (OTUs) at a 97% similarity levels using uclust (Edgar, 2010). OTUs with only one sequences (singleton) were removed from the dataset for all downstream analyses.
Construction and Analysis of aprA and dsrA Gene Transcripts Clone Libraries
Clone libraries of aprA and dsrA genes were constructed from cDNA samples obtained from the six different oil wells. aprA gene was amplified using primer pair aprA-1-FW (5′-TGGCAGATCATGATYMAYGG-3′) and aprA-5-RV′ (5′-GCGCCAACNGGDCCRTA-3′, a slightly modified version of aprA-5-RV) (Aoki et al., 2015) with initial denaturation at 95°C for 2 min followed by 35 cycles of 94°C for 30 s, annealing at 55°C for 30 s, and extension at 72°C for 30 s. Similarly, the dsrA gene was amplified with the primers DSR-1Fdeg (5′-ACSCAYTGGAARCACG-3′) and PJdsr853Rdeg (5′-CGGTGMAGYTCRTCCTG-3′) (Quillet et al., 2012). Thermal cycling was performed as follows: initial denaturation at 94°C for 2 min; 30 cycles of denaturation at 94°C for 30 s, annealing at 54°C for 30 s, elongation at 72°C for 30 s, and a final extension at 72°C for 10 min. The PCR products (approximately 400 and 450 bp for aprA and dsrA, respectively) were confirmed by electrophoresis in a 1% (w/v) agarose gel in 1× TAE, and the expected-size PCR products were purified using gel extraction kit. After purification, the products were cloned into Escherichia coli using pMD®19-T simple vector kit (Takara Biotechnology, Japan) according to the manufacturer instructions. White clones were picked into 1 ml Luria Broth (LB) medium added with ampicillin and incubated at 37°C for 24 h. Primer set M13-47 (5′-CGCCAGGGTTTTCCCAGTCACGAC-3′) and RV-M (5′-GAGCGGATAACAATTTCACACAGG-3′) was used to determine the positive clones. Sequencing of the positive clones was performed on an ABI 377 automated sequencer. Vector sequences were removed and nucleotide sequences were checked for possible chimeric artifacts by BLASTn alignment analysis (Nilsson et al., 2010). OTUs were classified using the BlastClust tool1 with the 97% similarity and one representative sequence from each OTU was selected for phylogenetic tree construction. The OTUs of aprA and dsrA functional genes were translated into amino acids using EMBOSS Transeq2 and aligned using MAFFT version 7 (Katoh and Standley, 2013). A neighbor-joining tree was constructed using MEGA version 6 (Tamura et al., 2013). The resulting trees were displayed using the FigTree version 1.4.23.
Quantification of 16S rRNA and dsrA Gene Transcripts by Real-Time PCR on cDNA
The total number of bacterial 16S rRNA and dsrA gene transcripts was determined for each sample of this study. The quantification was performed using a CFX96 thermalcycler (Bio-Rad, United States) with the SYBR Green system. The reactions for the two genes were performed using 25 μl reaction volume with 12.5 μl SYBR Green Master Mix (Takara, Japan), 9.5 μl of ddH2O, 0.5 μl of each PCR primers, and 2 μl of cDNA sample. The 16S rRNA gene transcript was amplified using the following primer sets: 338F (5′-ACTCCTACGGGAGGCAG-3′) and 805R (5′-GACTACCAGGGTATCTAATCC-3′) (Yu et al., 2005). The amplification was carried out as follows: an initial denaturation step of 4 min at 94°C, 40 cycles of denaturation at 94°C for 30 s, annealing at 57°C for 30 s and extension at 72°C for 1 min. The dsrA gene transcripts were amplified with the same primers (Quillet et al., 2012) as described above for the clone library. The PCR reaction conditions were as follows: initial denaturation at 94°C for 2 min; 40 cycles of denaturation at 94°C for 30 s, annealing at 54°C for 30 s, extension at 72°C for 30 s, and a final extension step at 72°C for 5 min. Annealing temperature was experimentally optimized to maximize the specificity of amplification (data not shown). The specificity of this primer pair was checked using the ProbeCheck4 against dsrAB database which contains 7,695 publicly available partial (6403) and full length (1,292) sequences (Müller et al., 2014). The standard curves for RT-qPCR were generated through 10-fold serial dilutions of plasmids carrying the specific target gene inserts (each run in triplicate). No-template negative controls were used to check for cross contamination. The size of the PCR product was checked with agarose gel electrophoresis. Melting curve (range: from 65 to 95°C) analysis was also conducted following each assay to confirm the specificity of the primer pairs. Amplification efficiency was calculated based on the respective standard curve. Results were expressed in gene copy numbers per ml of production water.
Bioinformatics and Statistical Analysis
aprA and dsrA diversity calculations were performed with the EstimateS (Colwell et al., 2012) including Shannon and reciprocal of Simpson index. Sampling coverage was evaluated by using non-parametric richness estimators ACE (abundance-based coverage estimator) (Chao and Yang, 1993), Chao1 (Chao, 1984; Hughes et al., 2001) and Coverage. Rarefaction curves were generated using GraphPad Prism 6. Bubble plots were made using the software R v3.2.4 (R Core Team, 2013) with the ggplot2 (Wickham, 2009) and reshape2 (Wickham, 2012) package. The Pearson correlation coefficients (r) between the gene copy numbers and environmental variables and Spearman′s rank correlations (rs) between the relative abundance of individual taxa and environmental factors were investigated via in R software with Hmisc package (Harrell, 2012). Differences in total and active microbial community composition were visualized through Non-metric Multi-Dimensional Scaling (NMDS) using Bray–Curtis similarity index by PAST software (version 3.09) (Hammer et al., 2001). OTU abundances for bacteria and archaea underwent square-root transformation to reduce the stress. Two-way PERMANOVA was performed to tests the differences in bulk and active bacterial and archaeal communities and location of community. The Bray–Curtis dissimilarity was calculated for each bacterial and archaeal genomic and active community based on OTU abundance and analyzed via PERMANOVA (999 permutations) in the PAST. Canonical correspondence analysis (CCA) was performed in CANOCO 4.5 for windows to identify the relationships between sulfate-reducing community structure and environmental parameters (Braak, 1988).
Nucleotide Sequence Accession Numbers
High-throughput raw sequences data have been deposited in the NCBI BioProject database under accession number SRP075241. The 966 nucleotide sequences of the aprA and dsrA genes reported in this study were deposited in GenBank under the accession numbers KX299071 to KX300036.
Results
Geochemical Characteristics of Production Water
The production water samples possessed high Na+ (3.36–5.68 g l-1), Cl- (4.03–8.23 g l-1), and (1.10–3.12 g l-1). The concentrations of the six production water samples ranged from 83.4 (2-71) to 533.7 mg l-1 (11-7), and the concentrations from 4.11 to 149.6 mg l-1. The amount of S2- ranged from 6.86 to 9.87 mg l-1. Volatile fatty acids including formate, acetate, propionate, and butyrate were detected in all samples (Table 1).
Total Microbial Cell Numbers and Gene Transcript Copy Numbers
Bacterial cell numbers were high in samples 2-53 (4.8 × 107 cells ml-1) and 9-18 (4.3 × 107 cells ml-1) and they ranged from 2.5 × 106 to 5.4 × 106 cells ml-1 in other samples (Figure 1A). The gene transcript levels of bacterial 16S rRNA and dsrA were assessed with quantitative PCR. DSR-1Fdeg is either a perfect match or contains only a single mismatch and is likely to amplify to 6228/7695 = 81% of sequences, PJdsr853Rdeg matches perfectly with almost all sequences in the Müller database and the coverage was 92%. Therefore, the exclusion of some sequences by these primers could result in a slight underestimate of dsrA transcript abundance. The efficiency values for 16S rRNA and dsrA gene transcripts were 93.7 and 80.5% with R2 values of 0.996 and 0.993, respectively. The abundance ranged from 1.46 × 107 to 3.85 × 107 copies ml-1 for 16S rRNA gene transcripts and from 2.06 × 105 to 3.73 × 105 copies ml-1 for dsrA gene transcripts (Figures 1B,C). The ratios of dsrA to the 16S rRNA gene transcripts copy numbers were used to determine the relative abundances of active SRMs in the whole community, and the ratios ranged from 0.54 to 1.02% in these samples (Figure 1D). The correlation analyses showed that the log-transformed dsrA gene transcripts were negatively correlated with the concentration of acetate and propionate significantly (r < -0.7, p ≤ 0.05). The dsrA/16S rRNA ratios were negatively correlated with the concentration of S2- (r = -0.85, p = 0.03) and propionate (r = -0.86, p = 0.03) (Supplementary Table S1).
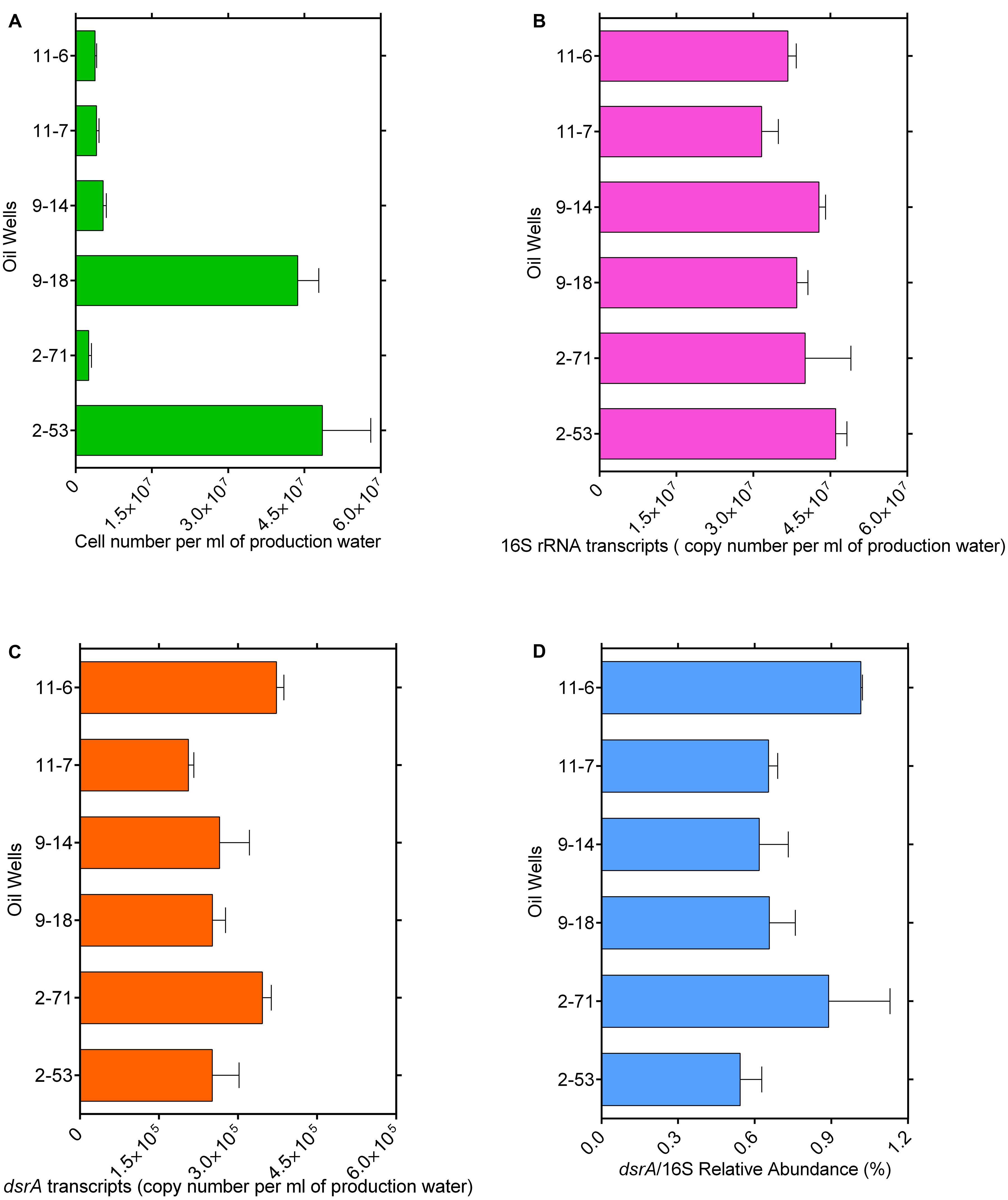
FIGURE 1. Total microbial cell numbers (A), gene transcript levels of bacterial 16S rRNA (B) and dsrA (C) from each well with different corrosion rate, ratios of dsrA/16S rRNA copy number (D). Error bars represent standard errors of the means across three replications.
Bacterial and Archaeal Sequencing and Richness
Supplementary Table S2 shows the results of sequencing of the genomic community (16S rRNA gene amplicons, DNA) and the active community (16S rRNA amplicons, RNA) in the production water of the six oil wells. Our data set consisted of 28,3246 bacterial reads, which clustered into 5,923 bacterial OTUs. Shannon indexes were ranged from 2.15 to 5.75 and Simpson indexes between 0.50 and 0.95 in bacterial libraries. The highest DNA diversity was measured in sample 9-18 and the lowest diversity in sample 11-7 with Shannon indexes 5.75 and 3.15, respectively. The highest RNA diversity was found in sample 11-6 (Shannon index: 4.99) and the lowest in sample 9-14 (1.79). Genomic DNA-based bacterial communities exhibited higher diversity than those of RNA-based active bacterial communities (pairwise t-test: t = 2.39, p = 0.037). The archaeal DNA and cDNA dataset had 26,2116 reads, 1,589 archaeal OTUs. The biodiversity (Shannon index) in genomic DNA-based archaeal communities were significantly more diverse than RNA-based active communities (p = 0.01). The Chao 1 richness were between 76 and 276, the Simpson indexes were 0.60–0.93 (Supplementary Table S2).
Bacterial and Archaeal Community Structure
Non-metric Multi-Dimensional Scaling ordination shows a clear separation for both genomic DNA and the active RNA based communities as shown in Figure 2. Bacterial community composition differed between the genomic DNA and the active RNA based communities (F = 7.9, p = 0.002). No significant difference was detected in bacterial community structures between sites across the oil well blocks of this study (F = 1.6, p = 0.1) (Figure 2A). A two-way PERMANOVA analysis also revealed significant differences between the genomic and active archaeal communities (F = 4.3, p = 0.01) as well as between oil wells across the oil well block (F = 4.1, p = 0.003) (Figure 2B).
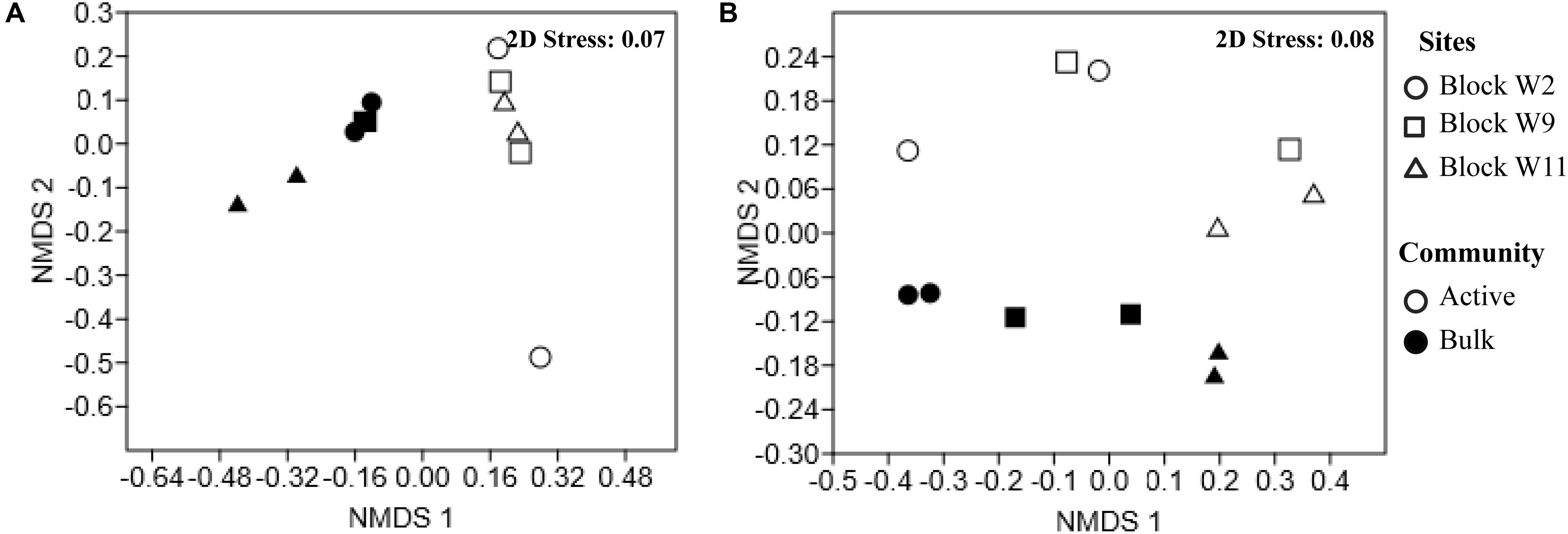
FIGURE 2. Non-metric Multi-Dimensional Scaling (NMDS) of the Bray–Curtis similarity of the relative abundance of bacterial (A) and archaeal (B) communities reveal spatial variation by reservoir blocks and between the genomic and active communities. Each point represents an individual sample in the NMDS charts. Full circles represent the genomic communities (DNA-based); empty circles symbol active communities (cDNA-based). Sites are represented by different shapes, block W2 (circle), block W9 (square), block W11 (triangle).
The Genomic and Active Communities and Composition
Figure 3 shows that the genomic bacterial communities (DNA-based) were dominated by Proteobacteria (44–69% of sequences) and Firmicutes (7.1–38%). Other phyla, such as Bacteroidetes (1.4–8.0%), Deferribacteres (0.050–0.23%), Chloroflexi (0.45–2.6%), Thermotogae (0.51–2.5%), and Spirochaetes (0.29–1.7%) were also represented in the samples. Desulfotignum, Desulfovibrio, Desulfarculus, and Thermodesulforhabdus dominated in both the genomic and the active SRM detected in oil reservoirs. Desulfotomaculum, Thermodesulfovibrio, and Desulfoglaeba were detected with low abundance (Supplementary Figure S1). A total of 17 genera of the genomic SRM were inferred from the genomic bacterial community accounting for 19.89, 15.51, 17.47, 17.14, 4.55, and 11.91% of total bacteria in 2-53, 2-71, 9-18, 9-14, 11-7, and 11-6 oil wells (Supplementary Table S3), respectively. The genomic archaeal communities were dominated by Archaeoglobi (26–86%), Methanobacteria (1.7–10%), and Methanomicrobia (3.3–57%). In addition, minor amounts of sequences related to Thermococci (0.97–2.1%), Crenarchaeota (0.070–13%), Woesearchaeota (0.14–3.9%), and Thermoplasmata (0.37–6.7%) were also obtained from the production waters.
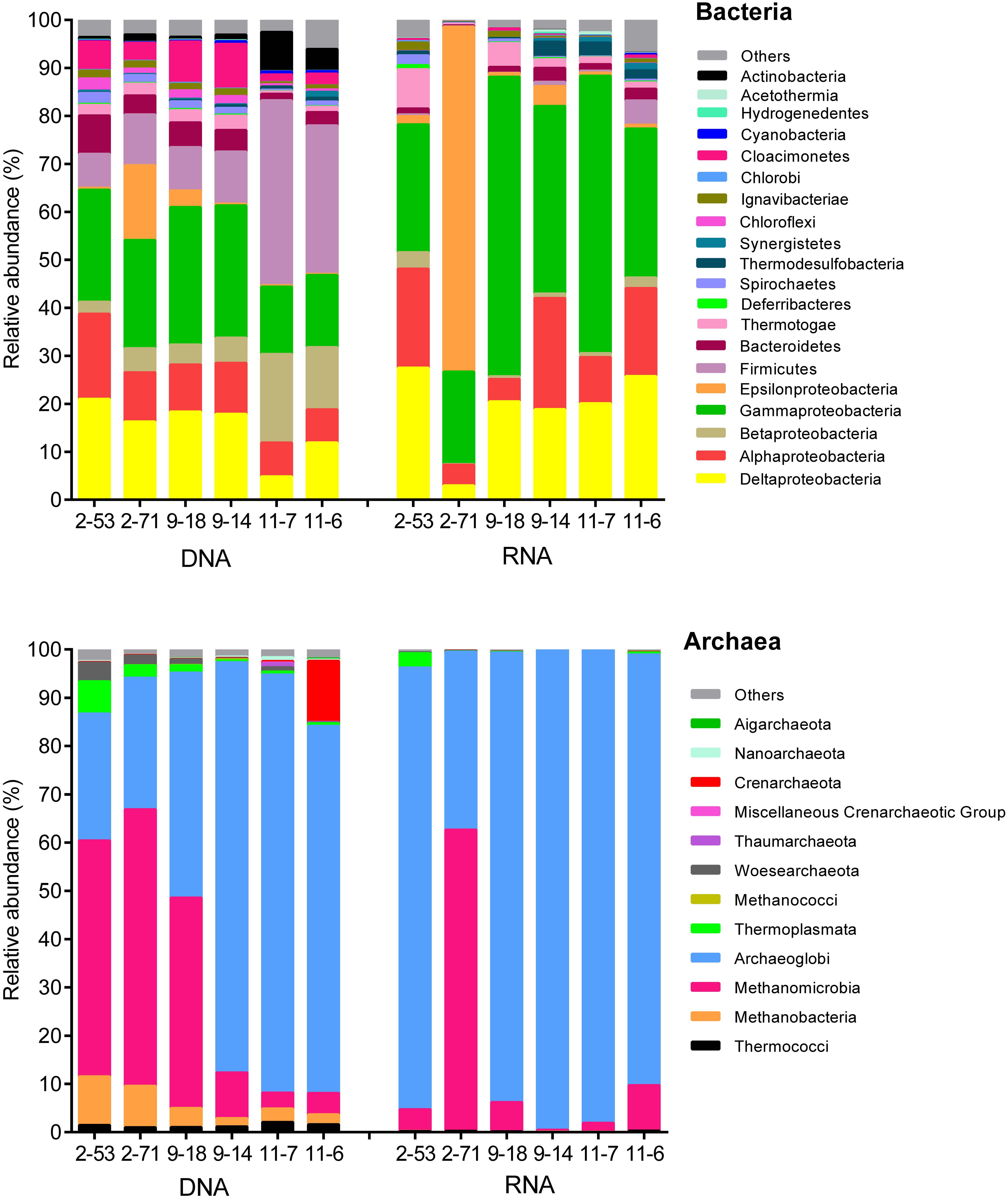
FIGURE 3. Composition of the genomic (DNA) and active (RNA) communities of production water from six different oil wells. DNA and RNA represent the abundance of rDNA and rRNA-derived 16S sequences of bacteria and archaea.
The potentially active bacterial communities (RNA-based) were dominated by Proteobacteria, which represented 78–98% of the total sequences, followed by Firmicutes (0.050–5.1%), Bacteroidetes (0.23–2.9%), and Thermotogae (0.34–8.2%) (Figure 3). The 16S rRNA bacterial (RNA-based) sequencing also resulted in 17 genera of active SRM. Those SRM occupied 23.90, 2.99, 19.97, 18.57, 20.62, and 25.53% in 2-53, 2-71, 9-18, 9-14, 11-7, and 11-6 oil wells, respectively (Supplementary Table S5). Active archaeal communities were dominated by Archaeoglobi (37–99%) and Methanomicrobia (0.31–62%) as shown in Figure 3.
Comparison of Relative Abundances in the Genomic and Active Communities
At bacterial class level, Gammaproteobacteria, Deltaproteobacteria, and Alphaproteobacteria dominated the production water communities (Figure 4). The relative abundances of class in the active and the genomic bacterial communities were correlated (Spearman correlation: r = 0.63, p < 0.005), Gammaproteobacteria were more represented in the RNA amplicon libraries compared with the genomic DNA amplicon libraries (pairwise t-test: t = 2.42, p = 0.035), while Betaproteobacteria were more represented in the genomic DNA amplicon libraries (t = 3.82, p = 0.003). Bacilli (t = 5.54, p < 0.001), Clostridia (t = 4.63, p < 0.001), Anaerolineae (t = 4.96, p < 0.001) were less abundant in the RNA amplicon libraries compared to the genomic DNA amplicon libraries. At genus level, the correlation of relative abundances between the genomic and the active communities was weaker (Spearman correlation: r = 0.50, p < 0.005) (Figures 4B,D). Desulfotignum and Roseovarius were highly abundant in both the genomic and the active communities. Thermodesulfobacterium (t = 2.27, p = 0.046) and Tepidiphilus (t = 2.39, p = 0.038) were more abundant in RNA amplicon libraries, while Pseudomonas (t = 4.84, p < 0.001), Alcanivorax (t = 2.59, p = 0.027), Achromobacter (t = 6.74, p < 0.001), Bacillus (t = 3.74, p = 0.0038), and Arthrobacter (t = 3.37, p = 0.0071) were more abundant in the genomic DNA amplicon libraries than the RNA amplicon libraries.
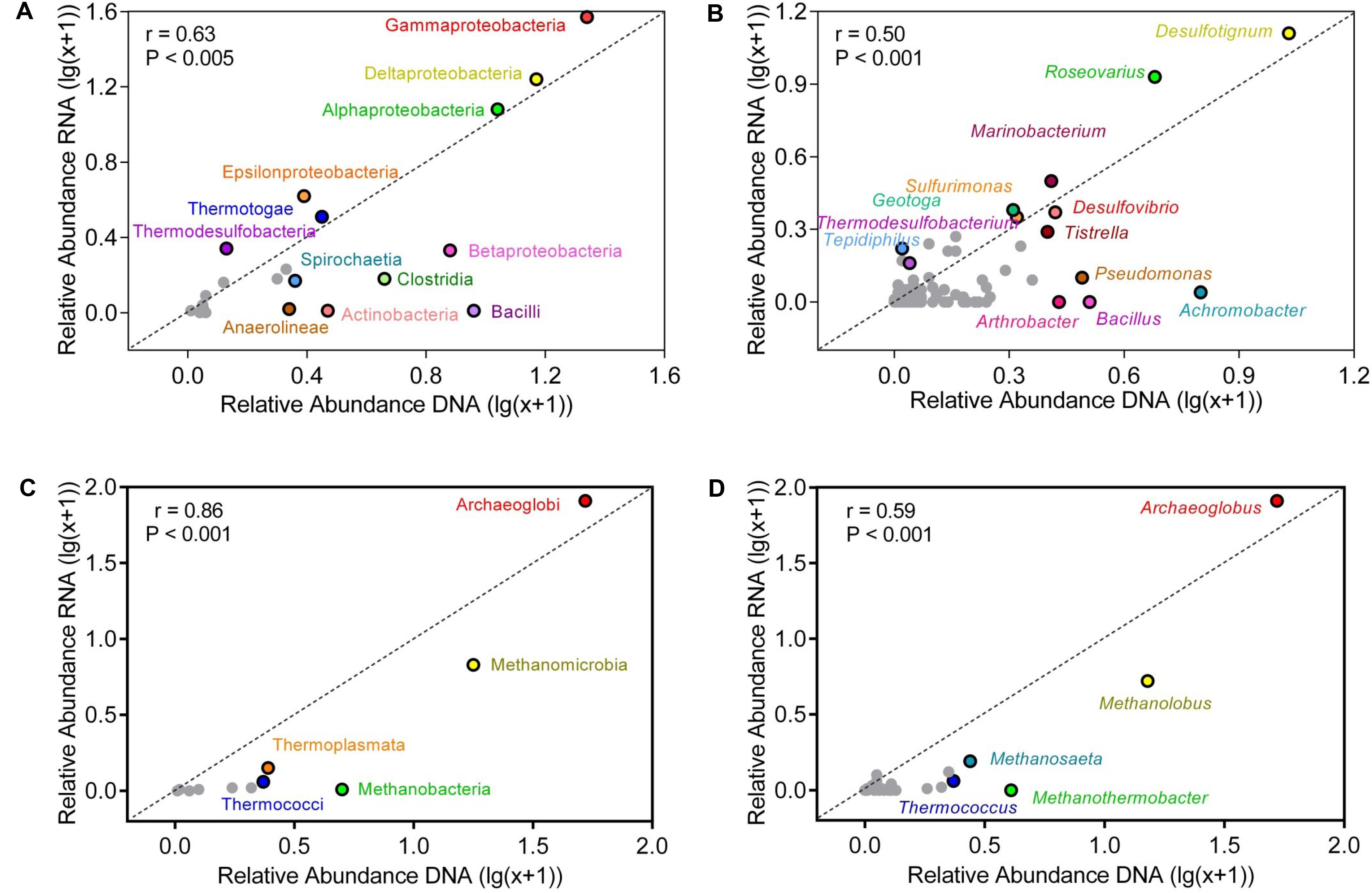
FIGURE 4. Correlation of relative abundances of taxa in bulk and active microbial communities at class (A,C) and genus (B,D) level. (A,B) represent bacterial communities (C,D) represent archaeal communities. The abundance shown was averaged across all samples. Relative abundances were log-transformed to reduce skewness of the data. Points above the diagonal indicated those taxa enriched in the RNA amplicon libraries. Gray points indicate taxa, which occurred in low numbers.
Compared with the bacterial communities, the correlation of relative abundances between genomic and active archaeal communities was stronger (Spearman correlation: r = 0.86, p < 0.001) (Figure 4). Thermococci (t = 9.25, p < 0.001) and Methanobacteria (t = 6.54, p < 0.001) were more represented in the genomic DNA amplicon libraries compared with the RNA amplicon libraries. Relative abundances of all detected archaeal genera are shown in Supplementary Table S3. Archaeoglobus and Methanolobus dominated the archaeal community at genus level, and the correlation of relative abundances between the genomic and the active communities was weak (r = 0.59, p < 0.001). Thermococcus (t = 9.24, p < 0.001) and Methanothermobacter (t = 4.83, p < 0.001) were more abundant in genomic DNA amplicon libraries than RNA amplicon libraries.
Relationships between Environmental Variables and Microbial Taxa
Correlations between taxa and geochemical data provide insight into the relationship between microbial taxa and environmental factors. Supplementary Table S4 shows that Gammaproteobacteria, Bacteroidia, Chlorobia, and Thermotogae of the genomic DNA were positively correlated with S2- and (rs > 0.75) and negatively correlated with corrosion rate (rs < -0.75). Thermodesulfobacteria were negatively correlated with pH and (rs < -0.80) and positively correlated with oil reservoirs depth (rs = 0.94). Deferribacteres were positively correlated with S2- (rs = 0.88), Betaproteobacteria were positively correlated with TDS and Ca2+ (rs > 0.75) and negatively correlated with (rs = -0.94). Methanobacteria, Thermoplasmata, and Woesearchaeota were positively correlated with pH (rs > 0.75). For genomic DNA, Archaeoglobi were positively correlated with oil reservoirs depth, TDS, Mg2+, Ca2+, and Cl- and negatively correlated with (rs = 0.89). Methanococci were negatively correlated with corrosion rate (rs = -0.88), whereas Crenarchaeota were positively correlated with corrosion rate (rs = 0.90).
Supplementary Table S5 shows the active Betaproteobacteria and Deferribacteres were negatively correlated with (rs < -0.75). Active Thermodesulfobacteria were positively correlated with (rs = 0.89) and negatively correlated with pH (rs = -0.89). Thermococci, Methanomicrobia, and Crenarchaeota were negatively correlated with formate and acetate (rs < -0.80). Active Archaeoglobi were positively correlated with Mg2+, , Na+, formate, and acetate (rs > 0.80).
Sequence Analysis of aprA Gene
A total of 391 aprA gene clones were clustered into 29 OTUs based on sequence similarity at 97% threshold value. The rarefaction curves for samples 9-18 and 11-7 aprA gene clone libraries reached saturation (100% coverage) and others did not plateau with coverage values ranged from 93.5 to 99.1% (Supplementary Figure S2A and Table 2). aprA sequences related to known SRM comprised 92.5% of total aprA gene sequences (23 OTUs), only four OTUs (4.1% of all aprA gene sequences) corresponded to sulfur-oxidizing microorganisms (SOM), and they all fell within cluster genus Thiobacillus. The remaining 13 clones, 2 OTUs (3.3%) without close affiliation to either SOM or SRM lineage, were grouped into aprA11-6-24 and aprA11-7-10, only found in Block W11 as shown in Figure 5.
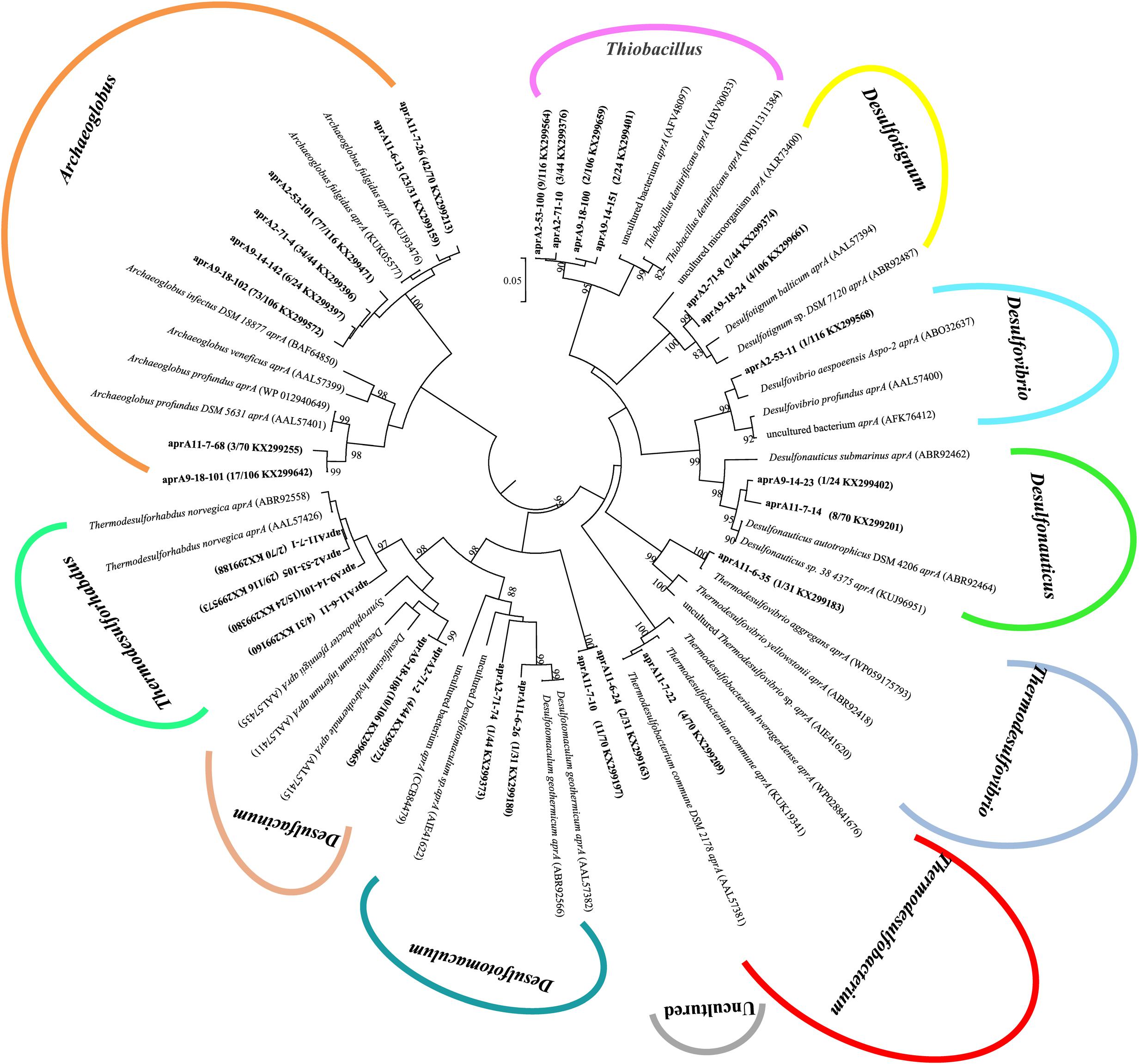
FIGURE 5. Phylogenetic tree of deduced amino acid sequences of aprA gene amplicons from six different oil wells of production water. The topology of the tree was obtained by the neighbor-joining method. Bold black letters indicated the OTUs detected in this study. The OTUs are shown with clone names, the number of clones of each OTU and accession numbers. The bootstrap values at the nodes of >80% (n = 1000 replicates) are reported. The scale bar represents 5% sequence divergence.
At the genus level, the relative abundance of Archaeoglobus-affiliated aprA genes comprised 66, 77, 85, 25, 64, and 74% of the clone libraries from the production water of 2-53, 2-71, 9-18, 9-14, 11-7, and 11-6, respectively (Figure 6A). Desulfotignum-affiliated aprA genes were detected in 2-71 (4.5%) and 9-18 (3.8%) samples. aprA2-53-11 and aprA2-53-105 which belonged to the genus Desulfovibrio and Thermodesulforhabdus were detected in sample 2-53. In addition, sequences related to Desulfacinum (9.1%) and Desulfotomaculum (2.3%) were detected in 2-71. Desulfonauticus, Thermodesulfobacterium, and Thermodesulforhabdus were also found in 11-7 with a relatively low frequency, resulting in 11, 5.7, and 2.9% of all clones, respectively. Sequences related to Desulfotignum and Desulfacinum were less abundant, but were detected at 9-18, accounting for about 3.8 and 9.4% of the clones, respectively. aprA9-14-101 and aprA9-14-23 related to Thermodesulforhabdus and Desulfonauticus were detected in sample 9-14, which represented 63 and 4.2% of total aprA gene sequences, respectively.
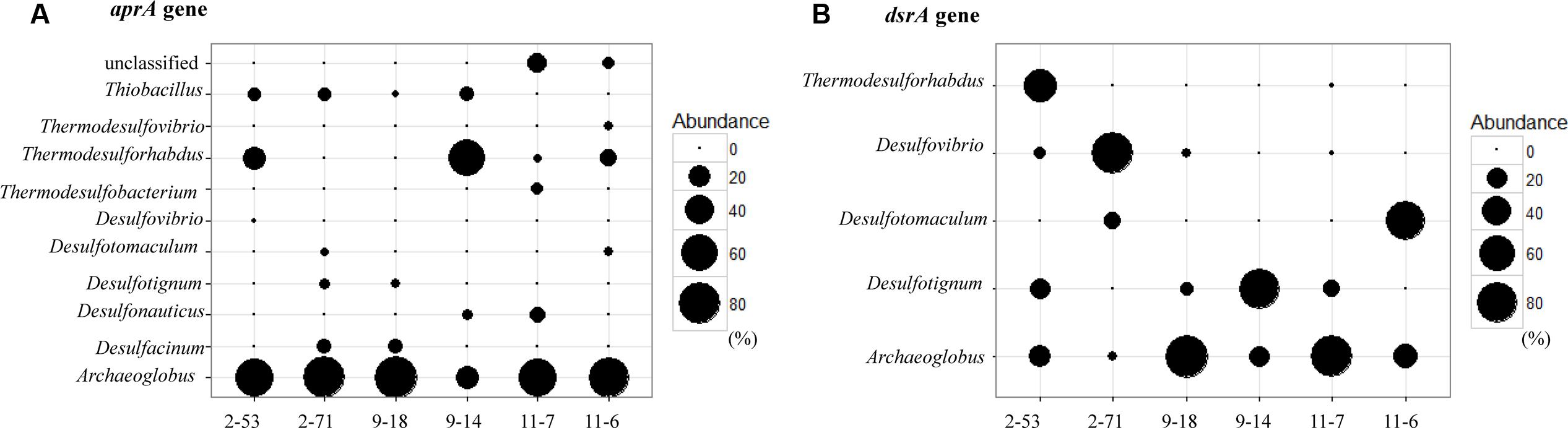
FIGURE 6. Bubble plots of aprA (A) and dsrA (B) gene community structures showing the relative abundances across samples. The size of each bubble indicate the relative abundance (percentage) of identified aprA and dsrA gene sequences falling within taxonomic group (at the genus level).
Sequence Analysis of dsrA Genes
A total of 575 dsrA gene clones were grouped into 20 OTUs, and the rarefaction curves for dsrA gene clone libraries reached clear saturation except for W11-7 (98.5% coverage) (Supplementary Figure S2B). Richness and diversity as supported by Shannon index and Chao 1 values were low in samples from different wells (Table 2). The majority of dsrA sequences were affiliated to those of Archaeoglobus (286 sequences, 8 OTUs). 109 sequences (4 OTUs) were related to Desulfotignum and 51 sequences (3 OTUs) clustered with Desulfovibrio. 41 sequences (2 OTUs) were related to Thermodesulforhabdus and 88 sequences (2 OTUs) belonged to Desulfotomaculum as shown in Figure 7.
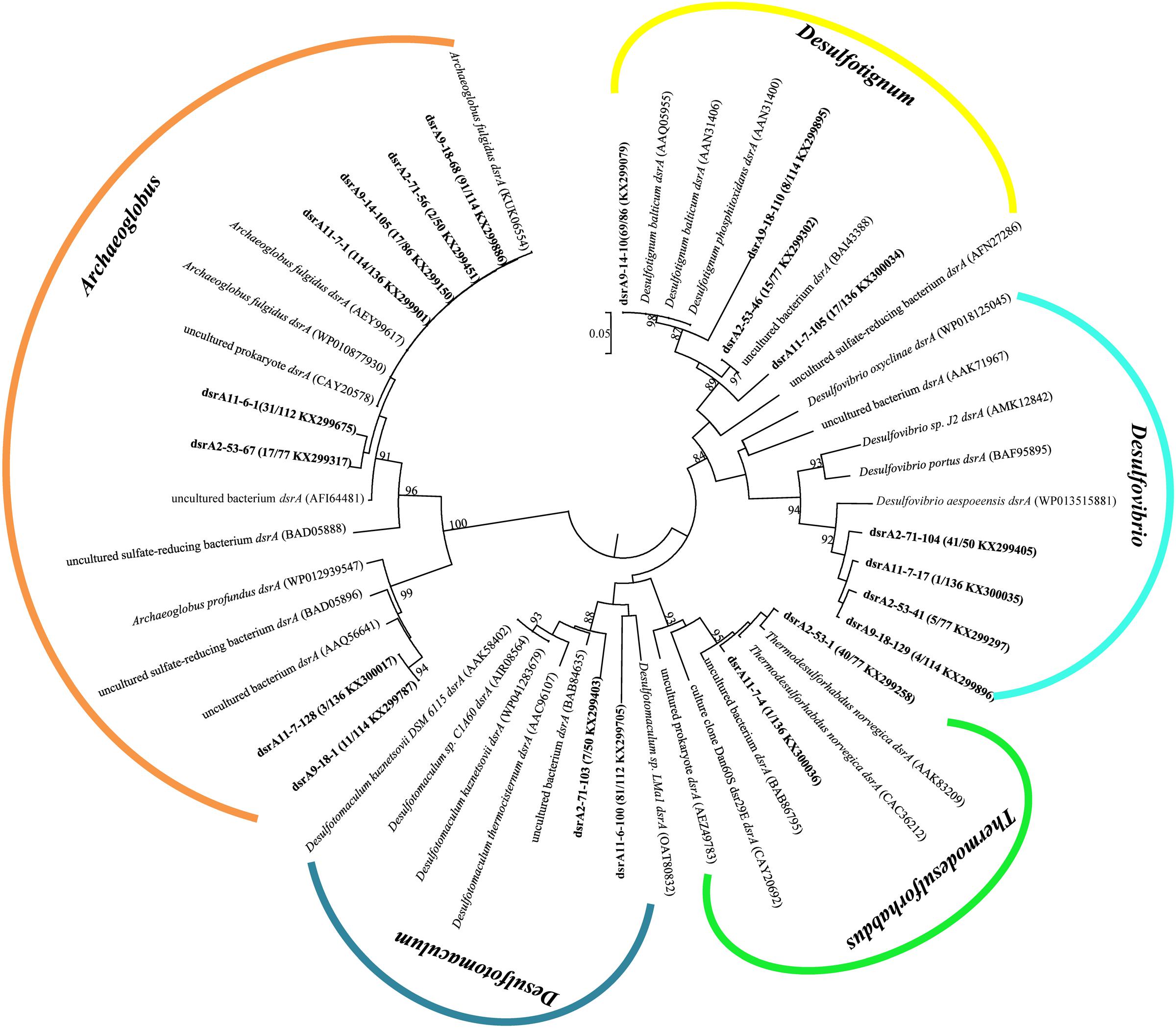
FIGURE 7. Phylogenetic tree of deduced amino acid sequences of dsrA gene of production water from six different oil wells. The topology of the tree was obtained by the neighbor-joining method. Bold black letters indicated the OTUs detected in this study. The OTUs are shown with clone names, the number of clones of each OTU and accession numbers. The bootstrap values at the nodes of >80% (n = 1000 replicates) are reported. The scale bar represents 5% sequence divergence.
dsrA9-18-1 and dsrA11-7-128 were 95% identical to Archaeoglobus profundus, other OTUs belonging to Archaeoglobus were 97–99% identical to Archaeoglobus fulgidus, which is known to reduce both sulfate and thiosulfate (Lenhart et al., 2014). Sequences related to Archaeoglobus were detected in all samples and also identified as the major component in 9-18 and 11-7 with 89 and 86% of the clone library, respectively. Sequences affiliated to Desulfotignum were detected in 9-18, 9-14, 2-53, and 11-7 (Figure 6B). Their closest relative was Desulfotignum balticum, and Desulfotignum group was dominant in 9-14 (80% of dsrA clones in library). Four samples (2-53, 2-71, 9-18, and 11-7) were detected for sequences affiliated to Desulfovibrio, with the highest abundance (82% of the dsrA gene clone library) detected in 2-71. In contrast, in 2-53, Thermodesulforhabdus was the most abundant (52% of all clones), which was also detected in W11-7 (0.74% of the clone library). The genus Desulfotomaculum comprised of 14 and 72% of the dsrA gene clone libraries obtained from 2-71 and 11-6, respectively (Figure 6B).
Relationships of Sulfate-Reducing Communities with Environmental Parameters
Based on variance inflation factors with 999 Monte Carlo permutations, five environmental variables, temperature, pH, concentration of S2-, , and were selected in the CCA biplot. The length of an environmental factors arrow in the ordination plot indicates the strength of the relationship of that parameter to community composition. In the aprA gene CCA plot (Figure 8A), concentration of appeared to be the most important environmental parameter, and the genus Desulfonauticus was closely associated with the . Desulfovibrio were associated with and S2- levels. The genera Desulfotignum and Desulfacinum were correlated with pH. The relationship of dsrA gene-based community with environmental factors (Figure 8B) showed that Archaeoglobus were positively correlated to temperature and Thermodesulforhabdus were associated with both and S2- levels.
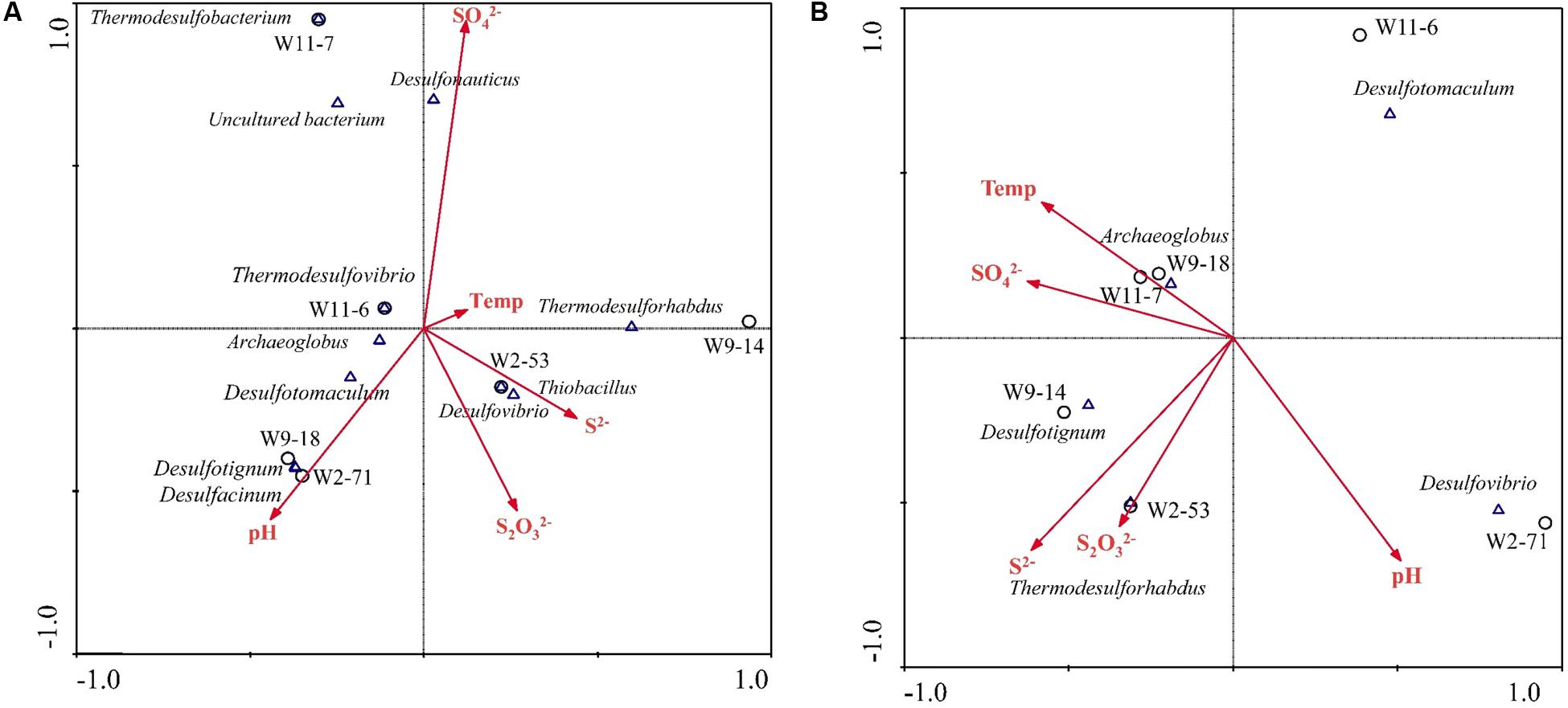
FIGURE 8. Canonical correspondence analysis (CCA) for the relationships between community structure based on aprA (A) and dsrA (B) genes and environmental factors in the six samples. Arrows indicate the direction and magnitude of measurable variables associated with sulfate-reducing community structures. Each circle represents sampling sites.
Discussion
Oil reservoirs are generally characterized with a high content of hydrocarbons and anoxic conditions. Previous studies of oil reservoirs reported that this unique ecosystem harbored diverse and variable microbial communities (Pham et al., 2009; Mayumi et al., 2011; Mbadinga et al., 2012; Tang et al., 2012; Wang et al., 2014; Li X.-X. et al., 2016). This study aimed to characterize genomic and active microbial populations, especially sulfate reducer in oil reservoir production water collected from Jiangsu oilfield which is known for reservoir souring and pipeline corrosion, and contains high sulfate (Guan et al., 2014). Results broaden our understanding on the microorganisms contributing to MIC in extreme environment and development of more specific treatment strategies for mitigation of problems with SRM in such environments.
Comparative Analysis of DNA and RNA-Derived Communities
In this study, DNA- and RNA-based libraries revealed a diverse microbial community in the production water of six different oil wells which suffered reservoir souring and pipeline corrosion. The DNA-derived bacterial and archaeal communities analyzed by the 16S rRNA gene sequences represent the whole communities including groups that are dormant or inactive, spore and dead (Blagodatskaya and Kuzyakov, 2013; Blazewicz et al., 2013). In contrast, the RNA-based community represents the active community (Moeseneder et al., 2005; Angel et al., 2013; Blazewicz et al., 2013) using ribosomal RNA as an indicator of microbial activity has limitations, including non-linear relation between growth rate and cellular rRNA content, as well as ribosomal content in dormant cells that could be high in some bacteria (Blazewicz et al., 2013). However, although this analysis has several weaknesses, comparison of the DNA- and RNA-based libraries provides for more complete characterization of microorganisms and new insights into activity in environmental communities (Frenk et al., 2015; Nazina et al., 2017).
Compared with RNA libraries, a higher species richness in the bacterial and archaeal DNA libraries was observed (Supplementary Table S2), which is consistent with a study in hydrothermal vent (Lanzén et al., 2011). One major difference between DNA and RNA-based bacterial community composition was that Firmicutes were more abundant in DNA samples, while fewer sequences in RNA samples were identified as Firmicutes (Figure 3). It has been reported that Firmicutes is an important component of microbial communities in water-injected and pristine oil reservoirs in Russia based on 16S rRNA gene (Frank et al., 2016). In Dagang oilfield, most sequences belonged to members of the phyla Firmicutes and Proteobacteria according to DNA-derived bacterial clone library. While, in RNA-based clone library, Proteobacteria were the most abundant (Nazina et al., 2017), which is consistent with our results. The dominant bacterial genera were Desulfotignum and Roseovarius, with the relative abundances ranging from 7.0 to 18.1% and 0.8 to 17.7% respectively (Supplementary Table S3). Tian et al. (2017) also found Desulfotignum was one of the dominant populations with its relative abundances in the different high temperature petroleum reservoirs ranging from 9.7 to 43.2%. In archaeal community, the most noticeable difference was that Methanobacteria and Thermococci were more abundant in DNA amplicon datasets (Figure 3). The majority of the archaeal OTUs were related to the Archaeoglobus (26.1–99.4%) and Methanolobus (0.09–60.6%) (Supplementary Table S3) in DNA- and RNA-based libraries. In a hyper-temperature Japanese oil well, the major populations were Thermotoga, Thermodesulfobacterium, and Archaeoglobus (Yamane et al., 2011).
Archaeoglobus as Dominant Active Sulfate-Reducer
16S rDNA and rRNA tag sequencing, as well as phylogenetic analyses of aprA and dsrA gene transcripts showed that Archaeoglobus was the dominant and active sulfate-reducer in production water of corrosive petroleum reservoirs in this study. Archaeoglobus, with close relatedness to Archaeoglobus fulgidus and Archaeoglobus profundus, were found in all production water samples. Three Archaeoglobus species are known to contain the complete pathway for dissimilatory sulfate reduction: Archaeoglobus fulgidus, Archaeoglobus profundus, and Archaeoglobus lithotrophicus (Speich and Trüper, 1988; Dahl et al., 1990). The genus Archaeoglobus may play a major role in sulfate reduction in production waters of high temperature reservoirs because of the high numbers of aprA and dsrA gene transcript clones related to Archaeoglobus. Interestingly, Archaeoglobus was also found and dominated the sulfate-reducing communities in the production water of North Sea high-temperature oil reservoir (Gittel et al., 2009).
Sulfate-reducing members of Desulfotignum, Desulfovibrio, and Thermodesulforhabdus genera were also prevalent groups in the investigated samples. Desulfotignum related sequences were closely related to Desulfotignum balticum, which is known for anaerobic benzoate degradation coupled with sulfate reduction (Habe et al., 2009). Desulfovibrio species can use hydrogen, formate, and many other organic compounds to reduce sulfate (Voordouw, 1995). They have been reported to be strongly adapted to environmental stresses, such as heavy metal contamination (Quillet et al., 2012). It has been reported that Desulfovibrio species dominated the microbial communities of highly corrosive biofilms of an offshore oil production facility (Vigneron et al., 2016). Thermodesulforhabdus were closely related to Thermodesulforhabdus norvegicus which was isolated from hot water of North Sea oilfield (Beeder et al., 1995). We also identified sequences related to Desulfotomaculum, which can grow under a range of sulfate concentrations, use diverse organic substrates and participate in syntrophic metabolism with methanogens (Imachi et al., 2006; Aüllo et al., 2013). And members of Desulfotomaculum are known for their sporulation capability, which is considered to be one of the microbial strategies used to resist unfavorable temperatures or nutrient deprivation conditions (Aüllo et al., 2013). In addition, some representatives of SRM within Deltaproteobacteria were also observed, which were assigned to Desulfobotulus, Desulfatitalea, Desulfocella, Desulfobulbus, Desulfovibrio, Desulfofustis, Desulfomicrobium, Desulfonauticus, Desulfarculus, and Desulfoglaeba. Moreover, Thermodesulfobacterium (Thermodesulfobacteria) and Thermodesulfovibrio (Nitrospira) were also detected with relatively low abundances.
Strategies for Mitigation of Petroleum Reservoirs Souring and Pipeline Corrosion
Since the role of microorganisms in MIC was acknowledged, different methods have been applied in the oil and gas industries to control or prevent microbial reservoir souring. So far, control measures against souring in the oil industry include removing sulfate from the injection water or inhibition or killing of SRM by continuous amendment of injection water with biocides, and chemical inhibitors including nitrite and nitrate (Rabus et al., 2015). Due to the high investment and operational cost, sulfate removal from the injection water is not feasible (Liebensteiner et al., 2014). Biocides are commonly used to control SRM in the oil industry, but application of biocides is usually limited to above-ground infrastructures to control microbial souring and corrosion, and the use of biocides is generally a concern with the increased resistance to biocides for long-term applications (Xue and Voordouw, 2015). Amendment of injection water with nitrate is the most widely accepted strategy to control microbial reservoir souring due to the effective and the advantages over biocides (Voordouw, 2011). Nitrate injection is effective in controlling souring by (1) the competitive suppression of SRM by stimulate the growth of heterotrophic nitrate-reducing bacteria (HNRB) which are able to outcompete SRM for the same electron donors, such as organic acids; (2) the removal of generated sulfide by sulfide-oxidizing nitrate-reducing bacteria (SONRB); (3) inhibition of the dissimilatory sulfite reductase (dsr) by nitrite (the metabolic intermediate of nitrate reduction) (Liebensteiner et al., 2014). In spite of success with nitrate to control or prevent souring, problems exist for ineffectiveness in low-temperature oil reservoirs as a result of emergence of microbial zone (Callbeck et al., 2011). Recently, perchlorate and monofluorophosphate have been demonstrated as effective inhibitors of SRM (Carlson et al., 2015a,b). Perchlorate is effective in laboratory tests and also inhibits the sulfate reduction by Archaeoglobus (Engelbrektson et al., 2014). As oil reservoir properties have a dominant effect in determining the results of souring control measures, a better understanding on the microbial community composition and eco-physiology are important for the development of a specific mitigation strategy.
Dual Role of Sulfur-Oxidizer in Corrosion
Except for SRM, SOM were also detected in these oil wells by 16S rDNA and rRNA tag sequencing. The SOM could catalyze the inorganic compound sulfide to sulfate. Roseovarius and Sulfurimonas were the dominant SOM in the samples of this study, with the abundance ranged from 1.8 to 17.7% in the cDNA-based bacterial libraries. The abundance of Sulfurimonas in the active bacterial community reached as high as 72% in oil well 2-71. In addition, sulfur-oxidizing species of the Paracoccus, Rhodovulum, Sulfuritalea, Dechloromonas, Arcobacter, and Rhizobium genera were also detected with low abundances (Supplementary Table S5). Previous studies have pointed out that some SOM like Sulfurospirillum spp. could control SRM and their activity was the primary force to control in nitrate injection systems (Hubert and Voordouw, 2007). SONRB may compete with SRM for electron donors and reduce the concentration of sulfide by oxidizing the dissolved sulfide. However, recent study showed that members of Sulfuricurvum and Sulfurovum of SOM play a potential role in MIC in pipelines subjected to injection of bisulfate (An et al., 2016). Furthermore, it has been reported that the presence of SONRB can promote the formation of greigite, a product of corrosion, and the Sulfurospirillum and Arcobacter which have the metabolic capacity of sulfide oxidation with nitrate were enriched by the nitrate-amendment rig (connected to a water injection pipeline) suffering from serious corrosion compared with non-amendment control (Drønen et al., 2014). Sulfuric acid may also induce more serious corrosion and play a certain role in MIC. The SOM community should not be ignored in petroleum reservoirs (Tian et al., 2017).
Conclusion
The combined approach of 16S rDNA and 16S rRNA high-throughput sequencing and aprA and dsrA clone libraries provided evidence that a diverse microorganisms inhabited in the corrosive and high temperature petroleum reservoir. Desulfotignum and Roseovarius, Archaeoglobus and Methanolobus dominated the bacterial and archaeal communities, respectively. The metabolically active microorganisms differed from the genomic community, representing a subset of the taxa presented in the genomic community. Most detected SRM were affiliated to Archaeoglobus, Desulfotignum, Desulfovibrio and Thermodesulforhabdus, they were closely related to pH of the production water and sulfate concentration. The most abundant sequence which belonged to SRM was identified as the genus Archaeoglobus, indicating that archaea Archaeoglobus might play a major role in reservoir souring and pipeline corrosion in high temperature oil reservoirs.
Author Contributions
B-ZM and J-DG designed the experiments, X-XL conducted the experiments, X-XL and LZ carried out the microbial analysis. J-FL, S-ZY, and SM gave suggestions for the experiments and results analysis. X-XL prepared the manuscript with contribution from all co-authors.
Conflict of Interest Statement
The authors declare that the research was conducted in the absence of any commercial or financial relationships that could be construed as a potential conflict of interest.
Acknowledgments
This work was supported by the National Science Foundation of China (No. 41530318), the Research Foundation of Shanghai (No. 15JC1401400), and the Fundamental Research Funds for the Central Universities of China (No. 222201717017).
Supplementary Material
The Supplementary Material for this article can be found online at: http://journal.frontiersin.org/article/10.3389/fmicb.2017.01011/full#supplementary-material
Footnotes
- ^http://toolkit.tuebingen.mpg.de/blastclust
- ^http://www.ebi.ac.uk/Tools/st/emboss_transeq/
- ^http://tree.bio.ed.ac.uk/software/figtree/
- ^http://131.130.66.200/cgi-bin/probecheck/probecheck.pl
References
Almahamedh, H. H., Williamson, C., Spear, J. R., Mishra, B., and Olson, D. L. (2011). Identification of Microorganisms and their Effects on Corrosion of Carbon Steels Pipelines. Houston, TX: NACE International, 97–100.
An, D., Dong, X., An, A., Park, H. S., Strous, M., and Voordouw, G. (2016). Metagenomic analysis indicates Epsilonproteobacteria as a potential cause of microbial corrosion in pipelines injected with bisulfite. Front. Microbiol. 7:28. doi: 10.3389/fmicb.2016.00028
Angel, R., Pasternak, Z., Soares, M. I. M., Conrad, R., and Gillor, O. (2013). Active and total prokaryotic communities in dryland soils. FEMS Microbiol. Ecol. 86, 130–138. doi: 10.1111/1574-6941.12155
Aoki, M., Kakiuchi, R., Yamaguchi, T., Takai, K., Inagaki, F., and Imachi, H. (2015). Phylogenetic diversity of aprA genes in subseafloor sediments on the northwestern pacific margin off Japan. Microbes Environ. 30, 276–280. doi: 10.1264/jsme2.ME15023
Aüllo, T., Ranchou-Peyruse, A., Ollivier, B., and Magot, M. (2013). Desulfotomaculum spp. and related gram-positive sulfate-reducing bacteria in deep subsurface environments. Front. Microbiol. 4:362. doi: 10.3389/fmicb.2013.00362
Bastin, E. S., Greer, F. E., Merritt, C., and Moulton, G. (1926). The presence of sulphate reducing bacteria in oil field waters. Science 63, 21–24. doi: 10.1126/science.63.1618.21
Beech, I. B., and Sunner, J. (2004). Biocorrosion: towards understanding interactions between biofilms and metals. Curr. Opin. Biotechol. 15, 181–186. doi: 10.1016/j.copbio.2004.05.001
Beeder, J., Torsvik, T., and Lien, T. (1995). Thermodesulforhabdus norvegicus gen. nov., sp. nov., a novel thermophilic sulfate-reducing bacterium from oil field water. Arch. Microbiol. 164, 331–336. doi: 10.1007/BF02529979
Biderre-Petit, C., Boucher, D., Kuever, J., Alberic, P., Jézéquel, D., Chebance, B., et al. (2011). Identification of sulfur-cycle prokaryotes in a low-sulfate lake (Lake Pavin) using aprA and 16S rRNA gene markers. Microbial. Ecol. 61, 313–327. doi: 10.1007/s00248-010-9769-4
Blagodatskaya, E., and Kuzyakov, Y. (2013). Active microorganisms in soil: critical review of estimation criteria and approaches. Soil Biol. Biochem. 67, 192–211. doi: 10.1016/j.soilbio.2013.08.024
Blazejak, A., and Schippers, A. (2011). Real-time PCR quantification and diversity analysis of the functional genes aprA and dsrA of sulfate-reducing prokaryotes in marine sediments of the Peru continental margin and the Black Sea. Front. Microbiol. 2:253. doi: 10.3389/fmicb.2011.00253
Blazewicz, S. J., Barnard, R. L., Daly, R. A., and Firestone, M. K. (2013). Evaluating rRNA as an indicator of microbial activity in environmental communities: limitations and uses. ISME J. 7, 2061–2068. doi: 10.1038/ismej.2013.102
Braak, C. J. (1988). CANOCO—an extension of DECORANA to analyze species-environment relationships. Plant Ecol. 75, 159–160.
Callbeck, C. M., Dong, X., Chatterjee, I., Agrawal, A., Caffrey, S. M., Sensen, C. W., et al. (2011). Microbial community succession in a bioreactor modeling a souring low-temperature oil reservoir subjected to nitrate injection. Appl. Microbiol. Biotechnol. 91, 799–810. doi: 10.1007/s00253-011-3287-2
Caporaso, J. G., Kuczynski, J., Stombaugh, J., Bittinger, K., Bushman, F. D., Costello, E. K., et al. (2010). QIIME allows analysis of high-throughput community sequencing data. Nat. Methods 7, 335–336. doi: 10.1038/nmeth.f.303
Carlson, H. K., Kuehl, J. V., Hazra, A. B., Justice, N. B., Stoeva, M. K., Sczesnak, A., et al. (2015a). Mechanisms of direct inhibition of the respiratory sulfate-reduction pathway by (per)chlorate and nitrate. ISME J. 9, 1295–1305. doi: 10.1038/ismej.2014.216
Carlson, H. K., Stoeva, M. K., Justice, N. B., Sczesnak, A., Mullan, M. R., Mosqueda, L. A., et al. (2015b). Monofluorophosphate is a selective inhibitor of respiratory sulfate-reducing microorganisms. Environ. Sci. Technol. 49, 3727–3736. doi: 10.1021/es505843z
Chao, A. (1984). Nonparametric estimation of the number of classes in a population. Scand. J. Stat. 11, 265–270.
Chao, A., and Yang, M. C. (1993). Stopping rules and estimation for recapture debugging with unequal failure rates. Biometrika 80, 193–201. doi: 10.1093/biomet/80.1.193
Colwell, R. K., Chao, A., Gotelli, N. J., Lin, S.-Y., Mao, C. X., Chazdon, R. L., et al. (2012). Models and estimators linking individual-based and sample-based rarefaction, extrapolation and comparison of assemblages. J. Plant Ecol. 5, 3–21. doi: 10.1093/jpe/rtr044
Dahl, C., Koch, H.-G., Keuken, O., and Trüper, H. G. (1990). Purification and characterization of ATP sulfurylase from the extremely thermophilic archaebacterial sulfate-reducer, Archaeoglobus fulgidus. FEMS Microbiol. Lett. 67, 27–32. doi: 10.1111/j.1574-6968.1990.tb13830.x
Davidova, I. A., Duncan, K. E., Perezibarra, B. M., and Suflita, J. M. (2012). Involvement of thermophilic archaea in the biocorrosion of oil pipelines. Environ. Microbiol. 14, 1762–1771. doi: 10.1111/j.1462-2920.2012.02721.x
Dinh, H. T., Kuever, J., Mussmann, M., Hassel, A. W., Stratmann, M., and Widdel, F. (2004). Iron corrosion by novel anaerobic microorganisms. Nature 427, 829–832. doi: 10.1038/nature02321
Drønen, K., Roalkvam, I., Beeder, J., Torsvik, T., Steen, I. H., Skauge, A., et al. (2014). Modeling of heavy nitrate corrosion in anaerobe aquifer injection water biofilm: a case study in a flow rig. Environ. Sci. Technol. 48, 8627–8635. doi: 10.1021/es500839u
Duncan, K. E., Gieg, L. M., Parisi, V. A., Tanner, R. S., Tringe, S. G., Bristow, J., et al. (2009). Biocorrosive thermophilic microbial communities in Alaskan North Slope oil facilities. Environ. Sci. Technol. 43, 7977–7984. doi: 10.1021/es9013932
Edgar, R. C. (2010). Search and clustering orders of magnitude faster than BLAST. Bioinformatics 26, 2460–2461. doi: 10.1093/bioinformatics/btq461
Edgar, R. C. (2013). UPARSE: highly accurate OTU sequences from microbial amplicon reads. Nat. Methods 10, 996–998. doi: 10.1038/nmeth.2604
Engelbrektson, A., Hubbard, C. G., Tom, L. M., Boussina, A., Jin, Y. T., Wong, H., et al. (2014). Inhibition of microbial sulfate reduction in a flow-through column system by (per)chlorate treatment. Front. Microbiol. 5:315. doi: 10.3389/fmicb.2014.00315
Enning, D., and Garrelfs, J. (2013). Corrosion of iron by sulfate-reducing bacteria: new views of an old problem. Appl. Environ. Microb. 80, 1226–1236. doi: 10.1128/AEM.02848-13
Enning, D., Venzlaff, H., Garrelfs, J., Dinh, H. T., Meyer, V., Mayrhofer, K., et al. (2012). Marine sulfate-reducing bacteria cause serious corrosion of iron under electroconductive biogenic mineral crust. Environ. Microbiol. 14, 1772–1787. doi: 10.1111/j.1462-2920.2012.02778.x
Frank, K. L., Rogers, D. R., Olins, H. C., Vidoudez, C., and Girguis, P. R. (2013). Characterizing the distribution and rates of microbial sulfate reduction at Middle Valley hydrothermal vents. ISME J. 7, 1391–1401. doi: 10.1038/ismej.2013.17
Frank, Y., Banks, D., Avakian, M., Antsiferov, D., Kadychagov, P., and Karnachuk, O. (2016). Firmicutes is an important component of microbial communities in water-injected and pristine oil reservoirs, western Siberia, Russia. Geomicrobiol. J. 33, 387–400. doi: 10.1080/01490451.2015.1045635
Frenk, S., Dag, A., Yermiyahu, U., Zipori, I., Hadar, Y., and Minz, D. (2015). Seasonal effect and anthropogenic impact on the composition of the active bacterial community in Mediterranean orchard soil. FEMS Microbiol. Ecol. 91:fiv096. doi: 10.1093/femsec/fiv096
Gittel, A., Sørensen, K. B., Skovhus, T. L., Ingvorsen, K., and Schramm, A. (2009). Prokaryotic community structure and sulfate reducer activity in water from high-temperature oil reservoirs with and without nitrate treatment. Appl. Environ. Microb. 75, 7086–7096. doi: 10.1128/AEM.01123-09
Guan, J., Zhang, B.-L., Mbadinga, S. M., Liu, J.-F., Gu, J.-D., and Mu, B.-Z. (2014). Functional genes (dsr) approach reveals similar sulphidogenic prokaryotes diversity but different structure in saline waters from corroding high temperature petroleum reservoirs. Appl. Microbiol. Biotechnol. 98, 1871–1882. doi: 10.1007/s00253-013-5152-y
Habe, H., Kobuna, A., Hosoda, A., Kosaka, T., Endoh, T., Tamura, H., et al. (2009). Identification of the electron transfer flavoprotein as an upregulated enzyme in the benzoate utilization of Desulfotignum balticum. Biosci. Biotechnol. Biochem. 73, 1647–1652. doi: 10.1271/bbb.90160
Hammer,Ø, Harper, D., and Ryan, P. (2001). PAST-palaeontological statistics, ver. 1.89. Palaeontol. Electron 4, 1–9.
Harrell, F. (2012). Hmisc: Harrell Miscellaneous Package. R Package Version 3.10. Available at: http://CRAN.R-project.org/package=Hmisc
Hubert, C., and Voordouw, G. (2007). Oil field souring control by nitrate-reducing Sulfurospirillum spp. that outcompete sulfate-reducing bacteria for organic electron donors. Appl. Environ. Microbiol. 73, 2644–2652. doi: 10.1128/AEM.02332-06
Hughes, J. B., Hellmann, J. J., Ricketts, T. H., and Bohannan, B. J. (2001). Counting the uncountable: statistical approaches to estimating microbial diversity. Appl. Environ. Microb. 67, 4399–4406. doi: 10.1128/AEM.67.10.4399-4406.2001
Imachi, H., Sekiguchi, Y., Kamagata, Y., Loy, A., Qiu, Y.-L., Hugenholtz, P., et al. (2006). Non-sulfate-reducing, syntrophic bacteria affiliated with Desulfotomaculum cluster I are widely distributed in methanogenic environments. Appl. Environ. Microb. 72, 2080–2091. doi: 10.1128/AEM.72.3.2080-2091.2006
Katoh, K., and Standley, D. M. (2013). MAFFT multiple sequence alignment software version 7: improvements in performance and usability. Mol. Biol. Evol. 30, 772–780. doi: 10.1093/molbev/mst010
Kip, N., and Veen, J. A. V. (2014). The dual role of microbes in corrosion. ISME J. 9, 542–551. doi: 10.1038/ismej.2014.169
Koch, G. H., Brongers, M. P. H., Thompson, N. G., Virmani, Y. P., and Payer, J. H. (2002). Corrosion cost and preventive strategies in the United States. J. Endocrinol. 122, 23–31.
Lanzén, A., Jørgensen, S. L., Bengtsson, M. M., Jonassen, I., Øvreås, L., and Urich, T. (2011). Exploring the composition and diversity of microbial communities at the Jan Mayen hydrothermal vent field using RNA and DNA. FEMS Microbiol. Ecol. 77, 577–589. doi: 10.1111/j.1574-6941.2011.01138.x
Lenhart, T. R., Duncan, K. E., Beech, I. B., Sunner, J. A., Smith, W., Bonifay, V., et al. (2014). Identification and characterization of microbial biofilm communities associated with corroded oil pipeline surfaces. Biofouling 30, 823–835. doi: 10.1080/08927014.2014.931379
Lewandowski, Z., and Beyenal, H. (2008). Mechanisms of Microbially Influenced corrosion. Springer Series on Biofilms. Berlin: Springer, 35–37.
Li, C.-Y., Zhang, D., Li, X.-X., Mbadinga, S. M., Yang, S.-Z., Liu, J.-F., et al. (2016). The biofilm property and its correlationship with high-molecular-weight polyacrylamide degradation in a water injection pipeline of Daqing oilfield. J. Hazard. Mater. 304, 388–399. doi: 10.1016/j.jhazmat.2015.10.067
Li, X.-X., Liu, J.-F., Yao, F., Wu, W.-L., Yang, S.-Z., Mbadinga, S. M., et al. (2016). Dominance of Desulfotignum in sulfate-reducing community in high sulfate production-water of high temperature and corrosive petroleum reservoirs. Int. Biodeter. Biodegr. 114, 45–56. doi: 10.1016/j.ibiod.2016.05.018
Liang, R., Aktas, D. F., Aydin, E., Bonifay, V., Sunner, J., and Suflita, J. M. (2016). Anaerobic biodegradation of alternative fuels and associated biocorrosion of carbon steel in marine environments. Environ. Sci. Technol. 50, 4844–4853. doi: 10.1021/acs.est.5b06388
Liang, R., Grizzle, R. S., Duncan, K. E., McInerney, M. J., and Suflita, J. M. (2014). Roles of thermophilic thiosulfate-reducing bacteria and methanogenic archaea in the biocorrosion of oil pipelines. Front. Microbiol. 5:89. doi: 10.3389/fmicb.2014.00089
Liebensteiner, M. G., Tsesmetzis, N., Stams, A. J. M., and Lomans, B. P. (2014). Microbial redox processes in deep subsurface environments and the potential application of (per)chlorate in oil reservoirs. Front. Microbiol. 5:428. doi: 10.3389/fmicb.2014.00428
Magoč, T., and Salzberg, S. L. (2011). FLASH: fast length adjustment of short reads to improve genome assemblies. Bioinformatics 27, 2957–2963. doi: 10.1093/bioinformatics/btr507
Martin, M. (2011). Cutadapt removes adapter sequences from high-throughput sequencing reads. EMBnet. J. 17, 10–12. doi: 10.14806/ej.17.1.200
Mayumi, D., Mochimaru, H., Yoshioka, H., Sakata, S., Maeda, H., Miyagawa, Y., et al. (2011). Evidence for syntrophic acetate oxidation coupled to hydrogenotrophic methanogenesis in the high-temperature petroleum reservoir of Yabase oil field (Japan). Environ. Microbiol. 13, 1995–2006. doi: 10.1111/j.1462-2920.2010.02338.x
Mbadinga, S. M., Li, K.-P., Zhou, L., Wang, L.-Y., Yang, S.-Z., Liu, J.-F., et al. (2012). Analysis of alkane-dependent methanogenic community derived from production water of a high-temperature petroleum reservoir. Appl. Microbiol. Biot. 96, 531–542. doi: 10.1007/s00253-011-3828-8
Meyer, B., and Kuever, J. (2007). Phylogeny of the alpha and beta subunits of the dissimilatory adenosine-5′-phosphosulfate (APS) reductase from sulfate-reducing prokaryotes–origin and evolution of the dissimilatory sulfate-reduction pathway. Microbiology 153, 2026–2044. doi: 10.1099/mic.0.2006/003152-0
Moeseneder, M. M., Arrieta, J. M., and Herndl, G. J. (2005). A comparison of DNA-and RNA-based clone libraries from the same marine bacterioplankton community. FEMS Microbiol. Ecol. 51, 341–352. doi: 10.1016/j.femsec.2004.09.012
Müller, A. L., Kjeldsen, K. U., Rattei, T., Pester, M., and Loy, A. (2014). Phylogenetic and environmental diversity of DsrAB-type dissimilatory (bi)sulfite reductases. ISME J. 9, 1152–1165. doi: 10.1038/ismej.2014.208
Muyzer, G., and Stams, A. J. (2008). The ecology and biotechnology of sulphate-reducing bacteria. Nat. Rev. Microbiol. 6, 441–454. doi: 10.1038/nrmicro1892
Nazina, T. N., Shestakova, N. M., Semenova, E. M., Korshunova, A. V., Kostrukova, N. K., Tourova, T. P., et al. (2017). Diversity of metabolically active bacteria in water-flooded high-temperature heavy oil reservoir. Front. Microbiol. 8:707. doi: 10.3389/fmicb.2017.00707
Nilsson, R. H., Veldre, V., Hartmann, M., Unterseher, M., Amend, A., Bergsten, J., et al. (2010). An open source software package for automated extraction of ITS1 and ITS2 from fungal ITS sequences for use in high-throughput community assays and molecular ecology. Fungal Ecol. 3, 284–287. doi: 10.1016/j.funeco.2010.05.002
Nyyssönen, M., Bomberg, M., Kapanen, A., Nousiainen, A., Pitkänen, P., and Itävaara, M. (2012). Methanogenic and sulphate-reducing microbial communities in deep groundwater of crystalline rock fractures in Olkiluoto, Finland. Geomicrobiol. J. 29, 863–878. doi: 10.1080/01490451.2011.635759
Ohene-Adjei, S., Teather, R. M., Ivan, M., and Forster, R. J. (2007). Postinoculation protozoan establishment and association patterns of methanogenic archaea in the ovine rumen. Appl. Environ. Microb. 73, 4609–4618. doi: 10.1128/AEM.02687-06
Okoro, C., Smith, S., Chiejina, L., Lumactud, R., An, D., Park, H. S., et al. (2014). Comparison of microbial communities involved in souring and corrosion in offshore and onshore oil production facilities in Nigeria. J. Ind. Microbiol. Biotechnol. 41, 665–678. doi: 10.1007/s10295-014-1401-z
Park, H. S., Chatterjee, I., Dong, X., Wang, S. H., Sensen, C. W., Caffrey, S. M., et al. (2011). Effect of sodium bisulfite injection on the microbial community composition in a brackish-water-transporting pipeline. Appl. Environ. Microbiol. 77, 6908–6917. doi: 10.1128/AEM.05891-11
Pelikan, C., Herbold, C. W., Hausmann, B., Müller, A. L., Pester, M., and Loy, A. (2015). Diversity analysis of sulfite- and sulfate-reducing microorganisms by multiplex dsrA and dsrB amplicon sequencing using new primers and mock community-optimized bioinformatics. Environ. Microbiol. 18, 2994–3009. doi: 10.1111/1462-2920.13139
Pham, V. D., Hnatow, L. L., Zhang, S., Fallon, R. D., Jackson, S. C., Tomb, J. F., et al. (2009). Characterizing microbial diversity in production water from an Alaskan mesothermic petroleum reservoir with two independent molecular methods. Environ. Microbiol. 11, 176–187. doi: 10.1111/j.1462-2920.2008.01751.x
Purkamo, L., Bomberg, M., Nyyssönen, M., Kukkonen, I., Ahonen, L., Kietäväinen, R., et al. (2013). Dissecting the deep biosphere: retrieving authentic microbial communities from packer-isolated deep crystalline bedrock fracture zones. FEMS Microbiol. Ecol. 85, 324–337. doi: 10.1111/1574-6941.12126
Quillet, L., Besaury, L., Popova, M., Paissé, S., Deloffre, J., and Ouddane, B. (2012). Abundance, diversity and activity of sulfate-reducing prokaryotes in heavy metal-contaminated sediment from a salt marsh in the Medway Estuary (UK). Mar. Biotechnol. 14, 363–381. doi: 10.1007/s10126-011-9420-5
R Core Team (2013). R: A Language and Environment for Statistical Computing. Vienna: The R Foundation for Statistical Computing.
Rabus, R., Venceslau, S. S., Wöhlbrand, L., Voordouw, G., Wall, J. D., and Pereira, I. A. (2015). Chapter two-a post-genomic view of the ecophysiology, catabolism and biotechnological relevance of sulphate-reducing prokaryotes. Adv. Microb. Physiol. 66, 55–321. doi: 10.1016/bs.ampbs.2015.05.002
Ren, G., Zhang, H., Lin, X., Zhu, J., and Jia, Z. (2014). Response of phyllosphere bacterial communities to elevated CO2 during rice growing season. Appl. Microbiol. Biotechnol. 98, 9459–9471. doi: 10.1007/s00253-014-5915-0
Savage, K. N., Krumholz, L. R., Gieg, L. M., Parisi, V. A., Suflita, J. M., Allen, J., et al. (2010). Biodegradation of low-molecular-weight alkanes under mesophilic, sulfate-reducing conditions: metabolic intermediates and community patterns. FEMS Microbiol. Ecol. 72, 485–495. doi: 10.1111/j.1574-6941.2010.00866.x
Speich, N., and Trüper, H. G. (1988). Adenylylsulphate reductase in a dissimilatory sulphate-reducing archaebacterium. Microbiology 134, 1419–1425. doi: 10.1099/00221287-134-6-1419
Stevenson, B. S., Drilling, H. S., Lawson, P. A., Duncan, K. E., Parisi, V. A., and Suflita, J. M. (2011). Microbial communities in bulk fluids and biofilms of an oil facility have similar composition but different structure. Environ. Microbiol. 13, 1078–1090. doi: 10.1111/j.1462-2920.2010.02413.x
Tamura, K., Stecher, G., Peterson, D., Filipski, A., and Kumar, S. (2013). MEGA6: molecular evolutionary genetics analysis version 6.0. Mol. Biol. Evol. 30, 2725–2729. doi: 10.1093/molbev/mst197
Tang, Y.-Q., Li, Y., Zhao, J.-Y., Chi, C.-Q., Huang, L.-X., Dong, H.-P., et al. (2012). Microbial communities in long-term, water-flooded petroleum reservoirs with different in situ temperatures in the Huabei Oilfield, China. PLoS ONE 7:e33535. doi: 10.1371/journal.pone.0033535
Tian, H., Gao, P., Chen, Z., Li, Y., Li, Y., Wang, Y., et al. (2017). Compositions and abundances of sulfate-reducing and sulfur-oxidizing microorganisms in water-flooded petroleum reservoirs with different temperatures in China. Front. Microbiol. 8:143. doi: 10.3389/fmicb.2017.00143
Uchiyama, T., Ito, K., Mori, K., Tsurumaru, H., and Harayama, S. (2010). Iron-corroding methanogen isolated from a crude-oil storage tank. Appl. Environ. Microbiol. 76, 1783–1788. doi: 10.1128/AEM.00668-09
Usher, K. M., Kaksonen, A. H., and Macleod, I. D. (2014). Marine rust tubercles harbour iron corroding archaea and sulphate reducing bacteria. Corros. Sci. 83, 189–197. doi: 10.1016/j.corsci.2014.02.014
Varon-Lopez, M., Dias, A. C. F., Fasanella, C. C., Durrer, A., Melo, I. S., Kuramae, E. E., et al. (2014). Sulphur-oxidizing and sulphate-reducing communities in Brazilian mangrove sediments. Environ. Microbiol. 16, 845–855. doi: 10.1111/1462-2920.12237
Venzlaff, H., Enning, D., Srinivasan, J., Mayrhofer, K. J. J., Hassel, A. W., Widdel, F., et al. (2012). Accelerated cathodic reaction in microbial corrosion of iron due to direct electron uptake by sulfate-reducing bacteria. Corros. Sci. 66, 88–96. doi: 10.1016/j.corsci.2012.09.006
Vigneron, A., Alsop, E. B., Chambers, B., Lomans, B. P., Head, I. M., and Tsesmetzis, N. (2016). Complementary microorganisms in highly corrosive biofilms from an offshore oil production facility. Appl. Environ. Microb. 82, 2545–2554. doi: 10.1128/AEM.03842-15
Voordouw, G. (1995). The genus Desulfovibrio: the centennial. Appl. Environ. Microbiol. 61, 2813–2819.
Voordouw, G. (2011). Production-related petroleum microbiology: progress and prospects. Curr. Opin. Biotechnol. 22, 401–405. doi: 10.1016/j.copbio.2010.12.005
Voordouw, G., Menon, P., Pinnock, T., Sharma, M., Shen, Y., Venturelli, A., et al. (2016). Use of homogeneously-sized carbon steel ball bearings to study microbially-influenced corrosion in oil field samples. Front. Microbiol. 7:351. doi: 10.3389/fmicb.2016.00351
Wang, L.-Y., Ke, W.-J., Sun, X.-B., Liu, J.-F., Gu, J.-D., and Mu, B.-Z. (2014). Comparison of bacterial community in aqueous and oil phases of water-flooded petroleum reservoirs using pyrosequencing and clone library approaches. Appl. Microbiol. Biotechnol. 98, 4209–4221. doi: 10.1007/s00253-013-5472-y
Wickham, H. (2009). ggplot2: Elegant Graphics for Data Analysis. New York, NY: Springer. doi: 10.1007/978-0-387-98141-3
Wickham, H. (2012). reshape2: Flexibly Reshape Data: A Reboot of the Reshape Package. R Package Version 1. Available at: https://cran.r-project.org/web/packages/reshape2/
Xue, Y., and Voordouw, G. (2015). Control of microbial sulfide production with biocides and nitrate in oil reservoir simulating bioreactors. Front. Microbiol. 6:1387. doi: 10.3389/fmicb.2015.01387
Yamane, K., Hattori, Y., Ohtagaki, H., and Fujiwara, K. (2011). Microbial diversity with dominance of 16S rRNA gene sequences with high GC contents at 74 and 98°C subsurface crude oil deposits in Japan. FEMS Microbiol. Ecol. 76, 220–235. doi: 10.1111/j.1574-6941.2011.01044.x
Yu, Y., Lee, C., Kim, J., and Hwang, S. (2005). Group-specific primer and probe sets to detect methanogenic communities using quantitative real-time polymerase chain reaction. Biotechnol. Bioeng. 89, 670–679. doi: 10.1002/bit.20347
Keywords: subsurface petroleum reservoirs, sulfate-reducing microorganisms (SRM), aprA, dsrA, RT-qPCR
Citation: Li X-X, Liu J-F, Zhou L, Mbadinga SM, Yang S-Z, Gu J-D and Mu B-Z (2017) Diversity and Composition of Sulfate-Reducing Microbial Communities Based on Genomic DNA and RNA Transcription in Production Water of High Temperature and Corrosive Oil Reservoir. Front. Microbiol. 8:1011. doi: 10.3389/fmicb.2017.01011
Received: 06 February 2017; Accepted: 22 May 2017;
Published: 07 June 2017.
Edited by:
Jesse G. Dillon, California State University, Long Beach, United StatesReviewed by:
Hiroyuki Imachi, Japan Agency for Marine-Earth Science and Technology, JapanMartin Krüger, Federal Institute for Geosciences and Natural Resources, Germany
Copyright © 2017 Li, Liu, Zhou, Mbadinga, Yang, Gu and Mu. This is an open-access article distributed under the terms of the Creative Commons Attribution License (CC BY). The use, distribution or reproduction in other forums is permitted, provided the original author(s) or licensor are credited and that the original publication in this journal is cited, in accordance with accepted academic practice. No use, distribution or reproduction is permitted which does not comply with these terms.
*Correspondence: Bo-Zhong Mu, YnptdUBlY3VzdC5lZHUuY24=