- 1Faculdade de Ciências Agrárias e Veterinárias, Universidade Estadual Paulista (UNESP), Jaboticabal, Brazil
- 2Centro de Energia Nuclear na Agricultura, Universidade de São Paulo (USP), Piracicaba, Brazil
Cyanobacteria, or oxyphotobacteria, are primary producers that establish ecological interactions with a wide variety of organisms. Although their associations with eukaryotes have received most attention, interactions with bacterial and archaeal symbionts have also been occurring for billions of years. Due to these associations, obtaining axenic cultures of cyanobacteria is usually difficult, and most isolation efforts result in unicyanobacterial cultures containing a number of associated microbes, hence composing a microbial consortium. With rising numbers of cyanobacterial blooms due to climate change, demand for genomic evaluations of these microorganisms is increasing. However, standard genomic techniques call for the sequencing of axenic cultures, an approach that not only adds months or even years for culture purification, but also appears to be impossible for some cyanobacteria, which is reflected in the relatively low number of publicly available genomic sequences of this phylum. Under the framework of metagenomics, on the other hand, cumbersome techniques for achieving axenic growth can be circumvented and individual genomes can be successfully obtained from microbial consortia. This review focuses on approaches for the genomic and metagenomic assessment of non-axenic cyanobacterial cultures that bypass requirements for axenity. These methods enable researchers to achieve faster and less costly genomic characterizations of cyanobacterial strains and raise additional information about their associated microorganisms. While non-axenic cultures may have been previously frowned upon in cyanobacteriology, latest advancements in metagenomics have provided new possibilities for in vitro studies of oxyphotobacteria, renewing the value of microbial consortia as a reliable and functional resource for the rapid assessment of bloom-forming cyanobacteria.
Introduction
Next generation DNA sequencing technologies became widely available in the middle 2000's, acting synergistically with advances in computer sciences and instigating a revolution in genomics (Koboldt et al., 2013). These technological advancements are much faster in acquiring data and enabled the analysis of much larger biological datasets than possible with the methodology of Sanger et al. (1977), which had become the standard DNA sequencing method for over three decades. The bioinformatics community has kept up with these advancements and developed a considerable number of computer software for analyzing this ever-growing amount of biological information. Following these new methods, not only has genome characterization become quicker, but large-scale projects involving genomics and metagenomics have also become feasible.
Despite the huge advances recent technologies have brought to microbiology, several obstacles still need to be overcome for a broader characterization of the domain Bacteria, as several phyla are still insufficiently covered by genomics. The field of cyanobacterial genomics holds an interesting example of a research subject that has been moving forward at a pace that is relatively slower than currently observed for some of the other bacterial phyla. Although an increasing number of laboratories around the world are interested in entering this research field, the availability of genomic sequences from cyanobacteria is still relatively low (Figure 1). Therefore, cyanobacteria are severely underrepresented in genomic databases when compared to other bacteria, and even archaea. Additionally, the currently available cyanobacterial genome databases are still lacking in taxonomic, environmental, and geographical diversity, thus providing an incomplete picture of this phylum.
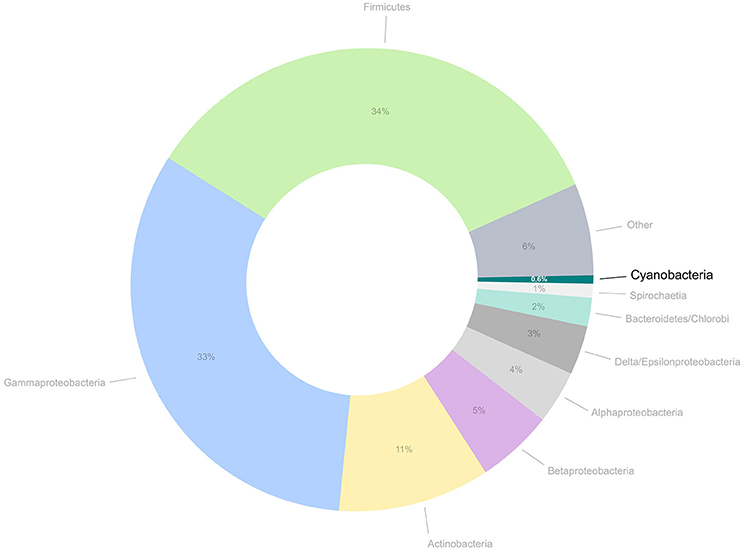
Figure 1. Proportion of public genomes from cyanobacteria in comparison to the total number of genomes currently available for bacteria and archaea. The number of cyanobacterial genomes amounts for approximately 0.6% of all prokaryotic genomes available at this moment.
The phylum Cyanobacteria groups oxygenic phototrophic bacteria, or oxyphotobacteria, the likely descendants of the pioneers of oxygenic photosynthesis (Fischer et al., 2016; Shaw, 2016). Most research on cyanobacteria is guided by evolutionary, ecological, ecotoxicological, biochemical, and taxonomic concerns (for a review on common investigation topics regarding cyanobacteria see Sciuto and Moro, 2015). Cyanobacteria were amongst the earliest organisms on the planet and synthesized important molecules for primitive life (Banack et al., 2012; Schirrmeister et al., 2016). Later, microorganisms of this phylum were responsible for oxygenating Earth's atmosphere (Shih et al., 2017) and originating chloroplasts (Alda et al., 2014). Currently, oxyphotobacteria (also known as blue-green bacteria) are important primary producers, with some taxa capable of fixing both atmospheric carbon and nitrogen (Hartmann et al., 2014; Karlson et al., 2015). Some of these organisms cause ecological disturbances after blooming in natural and eutrophic waters, an event that is becoming more frequent as the climate changes (Paerl and Otten, 2013; Costa et al., 2016; Visser et al., 2016). In addition, cyanobacteria are a subject of scientific investigation in regard to their production of toxic and non-toxic secondary metabolites (Merel et al., 2013; Micallef et al., 2015; Pearson et al., 2016). Their troubled taxonomic history has also left an ongoing need for revisiting the systematics of this phylum (Hoffmann et al., 2005; Komárek et al., 2014).
With the help of genomic methods, these topics are now under deeper scrutiny. It is now easier to gather information for understanding the evolution, organization, and distribution of genes involved in cyanotoxin biosynthesis (Stucken et al., 2010; Dittmann et al., 2013; D'Agostino et al., 2016), as well as investigating their possible ecophysiological functions and evolutionary advantages (Holland and Kinnear, 2013; Neilan et al., 2013); to acquire a better view of secondary metabolism and discover new molecules (Baran et al., 2013; Méjean and Ploux, 2013; Calteau et al., 2014; Dittmann et al., 2015; Moss et al., 2016); to verify the structure of DNA packaging (Lehmann et al., 2014); to better comprehend the relationship between morphology and genetics (Dagan et al., 2013; Gonzalez-Esquer et al., 2016); to advance the evolutionary history of the phylum and early life on Earth (Cardona et al., 2015; Harel et al., 2015; Schirrmeister et al., 2015); to study geological and biogeochemical alterations that occurred concurrently with changes in microbiota (Kaufman, 2014; Hamilton et al., 2016); and to refine systematics (Komárek et al., 2014; Thompson et al., 2015). Metagenomics has also made important discoveries, advancing our knowledge on macroevolution (Zaremba-Niedzwiedzka et al., 2017) and revealing a huge diversity yet to be explored (Hug et al., 2016), including the melainabacteria, the closest known relatives of oxyphotobacteria (Di Rienzi et al., 2013; Soo et al., 2014).
These investigations demonstrate that research on cyanobacteria gathers valuable knowledge on a broad range of subjects, and that genomics is well-suited for achieving breakthroughs. Therefore, the small number of available genome sequences for this phylum does not necessarily reflect irrelevance of the topic or lack of interest in either cyanobacteria or genomics; rather, it is likely a consequence of peculiarities inherent to current research methods on cyanobacteria. Difficulty in obtaining pure cyanobacterial cultures, technical challenges in the study of mixed cultures, and the very idiosyncrasies of cyanobacterial genomes are the main factors contributing to the complexity of this research theme, and they must be better understood and adequately addressed for more genomes of these microorganisms to become available. This review explores these factors and highlights joint genomics/metagenomics workflows that aim to overcome some of the challenges commonly found in cyanobacteriology.
Current State of Cyanobacterial Genomics
In 1996, Synechocystis sp. PCC 6803 became the first cyanobacterium to have its genome published (Kaneko et al., 1996). Yet, more than 20 years later, just a few over 400 cyanobacterial genomes are available in public databases, a number that pales in comparison to more than 30,000 complete genomes available for strains classified in 50 bacterial and 11 archaeal phyla (Land et al., 2015). Additionally, most available cyanobacterial genomes were retrieved from sea or freshwater strains deposited at the Pasteur Culture Collection (PCC) in Paris, France. Although the importance and quality of the PCC is undeniable, this predominance means that public databases are lacking in geographical diversity, as the majority of cyanobacterial strains deposited in the PCC are European. Also worrisome is the fact that some cyanobacterial taxa are overrepresented. As of this moment, 166 genomes were obtained from Prochlorococcus spp., among which 45 belong to a single species, P. marinus. Thus, the current public cyanobacterial genomes dataset is a biased sample of natural diversity in this phylum. Nevertheless, this dataset has helped to uncover fundamental information about these microorganisms.
The most recent ancestral genome for the cyanobacteria was estimated as having approximately 4.5 Mb and somewhere between 1,678 and 3,291 genes, with only around 4–6% remaining exclusive to the genomes of modern cyanobacteria, which have innovated in sequences for filament development, heterocyte differentiation, diazotrophic metabolism, and symbiotic competence (Larsson et al., 2011). Nonetheless, some of these innovations, like multicellularity, appear to have been acquired and lost many times during the evolutionary history of this phylum and may have been inherited from its common ancestor (Schirrmeister et al., 2011). By looking at the complete genomes published, it can be observed that the genetic material in cyanobacteria is composed of one or two chromosomes (Wang H. et al., 2012), ranging from 1.4 to 8.2 Mb (Meeks et al., 2001; Zehr et al., 2008), with up to 12 plasmids (Hirose et al., 2015), and occasionally an incision element (Thiel et al., 2014). Nevertheless, molecular analyses of the number of chromosomes in cyanobacteria indicate this phylum also presents polyploidy, so that some cyanobacteria contain up to 218 chromosomes during exponential growth (Griese et al., 2011). Akinetes may contain up to 450 chromosomes, most likely due to the necessity of a fast comeback for metabolic activities and cell division after dormancy (Sukenik et al., 2012).
Genomic content can be either the result of neutral processes or the reflection of adaptation to the different conditions an organism is subjected (Barrick et al., 2009; Koonin, 2009; Tenaillon et al., 2016). Basically, two adaptation strategies can be inferred from genomic analyses of cyanobacteria; broad adaptation potential through the increase of gene families as a result of genomic expansion, and elimination of genes that are dispensable for adaptation to a certain niche via mechanisms of genomic reduction (Larsson et al., 2011). Selection pressures may cause changes in genetic factors such as genome size, G-C percentage, gene number, and evolutionary rates. While cyanobacteria may develop individual strategies for interacting with the environment, several of their systems are globally conserved (Simm et al., 2015). Similar to what occurs in other microorganisms, a set of essential genes is found in cyanobacteria presenting considerably high conservation and resistance to horizontal transfer. This conserved gene set, or their core genome, consists mostly of sequences coding for complex protein structures and indispensable biochemical pathways (Shi and Falkowski, 2008; Larsson et al., 2011).
Non-essential genes, part of the accessory genome, are more frequently subject to horizontal gene transfer, which plays an important role in generating molecular diversity in cyanobacteria (Zhaxybayeva et al., 2006). Genome plasticity in these microorganisms is evidenced by the broad distribution and hypervariability of mobile genetic elements, mainly represented by insertion sequences, which can amount up to 10.95% of some genomes (Lin et al., 2010). The relatively high amount of repeated sequences found in the genomes of a number of cyanobacteria is a limiting factor for bioinformatic assembling, even after increasing sequencing depth. Therefore, these repeated sequences directly impact the cyanobacterial genome completeness during assembly. As is well known, large amounts of repeats deliver great challenges for assembling algorithms (Wang H. et al., 2012) and hence are an important factor to explain why approximately 90% of the genomes thus far available are permanent or temporary drafts (Land et al., 2015). Even for cyanobacterial strains in axenic cultures, genome sequencings with high depth and varying library strategies may prove insufficient for reconstructing chromosomes and plasmids into single sequences or even attending the minimum assembly quality for acceptance into the NCBI RefSeq genomes database (N50 above 5,000, L50 under 200, and less than 1,000 contigs) (Tatusova et al., 2013, 2015).
Techniques based on polyphasic taxonomy were introduced to cyanobacterial systematics in an attempt to overcome shortcomings brought by traditional emphasis on morphological features (Vandamme et al., 1996; Komárek, 2005, 2016). Genomics is currently the most promising framework for correcting mistakes caused by traditional taxonomics and clearing out the complicated evolutionary relationships of several polyphyletic taxa persisting in cyanobacterial classification. Potentially, even misleading errors caused by horizontal gene transfer or other processes that obscure the phylogenetic signal could be solved by phylogenomics (Kauff and Büdel, 2011). While the refinement of polyphasic taxonomy is still being discussed (Palinska and Surosz, 2014; Mishra et al., 2015), comparative genomics is its next logical step, and phylogenomics and synapomorphy analyses may redefine our understanding of the evolution of cyanobacteria (Gupta, 2009; Gupta and Mathews, 2010; Komárek et al., 2014).
Phylogenetically, the unbalanced availability of genomic sequences from cyanobacteria causes an unsatisfactory representation of their genomic potential. This prevents the expansion of knowledge of the molecular biology of the phylum, since sequences from neglected taxa may bring to light answers to meaningful questions (Richards, 2015). Sequencing genomes from cyanobacteria of lesser known taxa allows not only increasing knowledge of the molecular genetics of the phylum, but also of the evolution and diversity of aspects such as morphology, photosynthesis, secondary metabolism, and endosymbiosis (Dagan et al., 2013; Shih et al., 2013). There is a clearly observable tendency in more recent genomic projects, to recognize the necessity of studying cyanobacteria from taxa that were poorly investigated or that come from less explored environments. Due to the relatively low number of available genomes from cyanobacteria, applying genomic approaches to some topics was not yet successful on a larger scale. However, even if at this moment there are technical questions preventing a broader use of genomics in cyanobacterial research, genome sequencing is becoming so much faster and cheaper, that it is likely to eventually become a standard procedure.
Ecological Associations Involving Cyanobacteria
Cyanobacteria present a broad range of metabolic capacities that lead them to perform important ecological roles and to establish mutualistic interactions with a wide variety of organisms. Epi- or endobiotic symbioses between cyanobacteria and eukaryotes such as animals (ascidia, echiuroid worms, midge larvae, sponges), chromalveolata (diatoms, dinoflagellates), fungi (lichens, Geosiphon), and plants (cycads, hornworths, liverworts, mosses, Azolla, Gunnera) have been documented (Adams, 2000; Bergman et al., 2007; Adams et al., 2013). On the other hand, associations between cyanobacteria and other bacteria or archaea have not received similar attention.
Several heterotrophic microorganisms benefit from associations with bacteria capable of oxygenic photosynthesis, nitrogen fixation, and biosynthesis of secondary metabolites, characteristics found in many cyanobacteria. Indeed, cyanobacteria often present heterotrophic microbes in symbiotic association with their cells (Figure 2). Some of these associates are in intimate contact with their cell envelope or even reside inside their glycocalyx (Zhubanova et al., 2013). The interacting interface between cyanobacteria and heterotrophic microbes in cyanobacteria-dominated communities could even be considered a “cyanosphere” in analogy to what is observed in plant rhizospheres and phyllospheres.
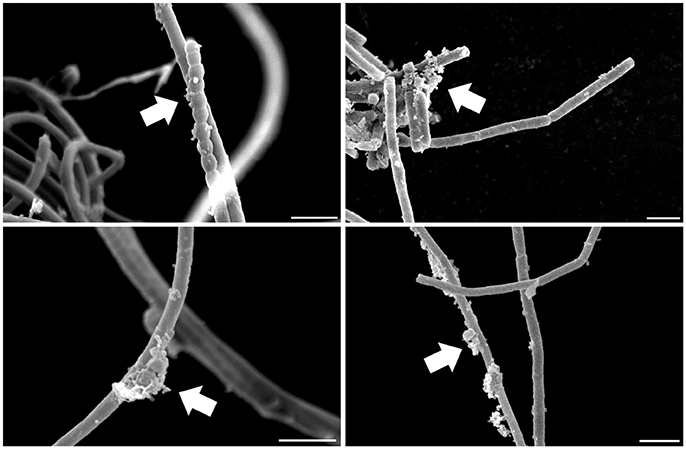
Figure 2. Scanning electron microscopy of a cyanobacterial strain after standard isolation procedures showing microbes associated with its filaments in a carbon- and nitrogen-free culture medium. Arrows highlight microbial assemblages attached to cyanobacterial sheaths. Scale: 5 μm.
Associations between cyanobacteria and other microorganisms have been presumed to exist even amongst the oldest known forms of life, engaging in important ecological interactions for billions of years. Stromatolites are a classical example of cyanobacteria-dominated mats supporting highly-developed microbial communities that establish complex interactions (Cohen and Gurevitz, 2006). Exopolysaccharides secreted by cyanobacteria are constantly colonized by microbes and may originate biofilms and microbial mats with high richness and abundance, where autotrophs and heterotrophs find diverse opportunities for interacting (Paerl et al., 2000; Cole et al., 2014). Biofilms dominated by cyanobacteria usually have high nutritional quality and may support large biomasses of primary consumers (Yamamuro, 1999; Nagarkar et al., 2004). Similarly, cyanobacterial blooms occurring in response to eutrophic conditions in water bodies may also be followed by associations with heterotrophic bacteria, several being capable of enhancing cyanobacterial growth (Berg et al., 2009).
In addition to bioavailable carbon and nitrogen, microbes interacting with cyanobacteria may benefit from the secondary metabolites they produce. For instance, some cyanotoxins and cyanopeptides may be degraded and taken up by associated bacteria (Kormas and Lymperopoulou, 2013; Briand et al., 2016). A wide range of chemicals are synthesized by cyanobacteria both under axenic and symbiotic conditions, either in artificial or natural habitats. Molecules with antibacterial, antiprotozoan, antitumor, immunomodulatory, and protease-inhibiting activities have been described, pointing to cyanobacteria as a prolific source for the production of bioactive compounds (Singh et al., 2011). Some authors consider the potential for biosynthesis of secondary metabolites from these organisms as matched only by the potential of myxobacteria and the actinobacterial genus Streptomyces (Nunnery et al., 2010). Though the ecological or physiological role of a considerable number of these metabolites is not yet understood, the chemical ecology of this phylum has been an increasingly explored topic (Leão et al., 2012).
Non-Axenic Cultures in Cyanobacteriology
Especially because of the associations between cyanobacteria and other microorganisms, the process for obtaining axenic cyanobacterial cultures is very challenging. Even with the use of cyanobacteria-specific media, heterotrophs may quickly overcome cells of the target cyanobacterium during isolation, and consequently culture purification becomes a very complex and time-consuming process (Waterbury, 2006). Although several techniques for the purification of cyanobacterial cultures including both mechanical and chemical methods have been published, axenic cyanobacterial cultures are still very hard to achieve, since most methodologies for axenity are somewhat specific to a few strains and have low rates of success (Choi et al., 2008; Sena et al., 2008). Some commonly-employed techniques, such as washing, centrifugation, or filtering are merely capable of reducing the number of unattached microorganisms that are either smaller or larger than the target cells (Vázquez-Martínez et al., 2004; Sena et al., 2008), and they usually cannot remove microbes strongly connected to cyanobacterial sheaths. In certain instances, an axenic culture is considered virtually impossible to obtain due to strong associations between cyanobacteria and symbiont microbes. In addition, fibrous carbohydrate structures in cyanobacterial mucilage may establish firm aggregates with microbes, resulting in strong connections capable of even protecting from antibiotic action (Vázquez-Martínez et al., 2004). Therefore, recalcitrant heterotrophs are a constant concern during the process of isolating cyanobacteria.
Most conventional efforts for the isolation of cyanobacteria result in non-axenic cultures, consisting of microbial consortia composed of a single cyanobacterial species and a number of closely associated non-cyanobacterial organisms, or a unicyanobacterial culture. These cultures are almost like “in vitro blooms” in the sense that they provide essential nutrients and conditions for cyanobacteria to massively reproduce and dominate their communities while bringing forward a number of ecological associations with microbes that are potentially host-specific, as commonly observed in environmental blooms (Pope and Patel, 2008; Berg et al., 2009; Bagatini et al., 2014). Most research labs keep non-axenic, unicyanobacterial cultures for routine work, and only dedicate time for trying to achieve an axenic culture when a specific strain is scheduled to be studied in further detail, e.g., for genomic sequencing. Furthermore, traditional methods for evaluating axenity in cyanobacterial cultures may be misleading, as they check for the persistence of cultured bacteria while ignoring uncultured symbionts, causing a mixed culture to be mistaken for an axenic one (Heck et al., 2016).
Another fact adding to the routine use of non-axenic cultures in cyanobacteriology is that these microbes were initially studied in botany, and for legacy reasons the International Code of Nomenclature for algae, fungi, and plants (Botanical Code) (McNeill et al., 2012) is still used for describing cyanobacteria in addition to the International Code of Nomenclature of Prokaryotes (Prokaryotic Code) (Parker et al., 2015). Even today, decades after cyanobacteria were proved to be prokaryotes and not algae, very few cyanobacteria have been described under the Prokaryotic Code (Oren, 2011), and one of the reasons for this is that the Botanical Code does not require axenic cultures as type material, unlike the Prokaryotic Code. As knowledge of microbial ecology advances, the requirement of axenic cultures in the Prokaryotic Code may come to be viewed as unnecessary, unrealistic, or anachronistic in the advancement of microbial systematics, since the great majority of microorganisms are likely to remain uncultured in the near future, due not only to the lack of knowledge of their physiological demands, but also to the incredible amount of work that would be necessary for culturing them, even if it were possible to remove these organisms from their ecological context.
Discussions about the Prokaryotic Code validating cyanobacterial names validly published under the Botanical Code (Pinevich, 2015) and adopting alternative type materials for the description of new microbes (Chun and Rainey, 2014; Sutcliffe, 2015; Whitman, 2015, 2016) are still in the beginning, thus it is likely the current practices will still be kept for quite some time. It is important nevertheless to also include in this discussion the availability of mixed cultures. Most botanical collections that accept cyanobacterial samples require either dried biomass in exsicata or liquid cultures preserved in formaldehyde, which make samples unsuitable for further work. Microbiological collections, on the other hand, are better equipped to receive in vitro microbial consortia, but typically only accept axenic cultures. Consequently, the only available option to obtain non-axenic cyanobacterial cultures mentioned in publications is to ask authors directly. The expansion of type material possibilities should therefore be accompanied by official avenues for depositing microbial consortia, which would greatly favor experiment reproducibility.
By using non-axenic cultures, it is possible to significantly speed up research and achieve breakthroughs faster, since the results are obtained drastically quicker when the time that would be necessary for removing the last remaining associated microbes is eliminated. In addition, if the culture is transferred to fresh media on a frequent basis, the relative abundance of cyanobacterial cells is maintained at higher levels than cells of other individual species in the community, as the conditions offered by the culture medium are directed toward the necessities of cyanobacterial physiology, which reduces interference from associates.
Advantages of Cyanobacteria in Microbial Consortia
Microbial consortia are usually obtained from the enrichment of environmental samples, which are based on the investigation of microbial assemblages after exposure to conditions that stimulate the growth of certain microorganisms from within the community. Depending on the research objectives, such an approach may present some advantages over the analyses of raw, unrefined environmental samples. Datasets coming from the high-throughput sequencing of non-axenic cyanobacterial cultures can be very similar to metagenomic sequences obtained from enriched microbial consortia.
Although a mixed culture may be initially seen as an undesired outcome, microorganisms in consortia may act synergistically and become more efficient than axenic cultures for some processes, or even perform complex ecological functions with multiple steps, which are only possible with the co-culturing of distinct populations (Brenner et al., 2008). Microbial consortia also allow researchers to carry out studies on ecological interaction and co-evolution (Brenner et al., 2008). Mutualism, competition, predation/parasitism, commensalism, amensalism, and neutralism play a central role in the modeling of the stability and dynamics of communities in consortia; therefore, co-culturing enables the discovery of complex interaction networks, including contact-dependent or -independent mediating molecules (Faust and Raes, 2012; Song et al., 2014).
In a microbial consortium, cyanobacteria may enhance the conditions for the community to perform a certain task (Zhubanova et al., 2013). Furthermore, some microbial consortia supported by cyanobacteria potentially enable the growth of otherwise uncultured microbes. Most bacteria are not currently subject to growth under culturing conditions (Rappé and Giovannoni, 2003). Among these bacteria, obligatory symbionts depend on nutrients or cell interactions provided by other microorganisms, which explains the low success rates in attempts of axenic culturing (Wilson and Piel, 2013). Consequently, co-culturing has been suggested as an alternative for carrying out in vitro studies in previously uncultured bacteria (Vartoukian et al., 2010; Stewart, 2012). Low emphasis has been given to cyanobacteria as culturing partners, as research has focused mostly on other photosynthetic microorganisms, such as chlorophytes (Otsuka et al., 2008). Nonetheless, as a consequence of their extraordinary metabolic capacities, cyanobacteria have great potential for promoting the in vitro growth of currently uncultured bacteria.
Whereas high-throughput sequencing of non-axenic cultures delivers a great challenge to the genomic study of specific strains, it also provides the researcher with access to the genomes of its symbiotic microorganisms, allowing for a broader investigation. In contrast to conventional microbial genomics approaches, which assume datasets originating from axenic samples, metagenomics deals with data from mixed samples, composed of distinct microbial populations (McHardy and Rigoutsos, 2010). Metagenomic approaches have proved useful for unveiling the composition, structure, genetics, and metabolism of natural and artificial microbial communities and are appropriate for the evaluation of microbial consortia (Song et al., 2014). Metagenomics also allow to successfully retrieve draft, near-complete, or even complete microbial genomes from mixed samples (Tyson et al., 2004; Sharon and Banfield, 2013; Sangwan et al., 2016).
Metagenomic assembly is more efficient under conditions of lower richness and higher genomic coherence, when it faces lower interference of less relevant sequences (Teeling and Glöckner, 2012), and it is also favored by samples composed of distantly-related species (De Filippo et al., 2012), as commonly found in non-axenic cyanobacterial cultures. In addition, genomes obtained by metagenomics commonly represent a hybrid population genome, i.e., a chimeric sequence constructed with sequences from different strains of the same species. This problem is not found in a cyanobacterial genome retrieved from the metagenome of a non-axenic culture, as isolation and culturing procedures are responsible for ensuring a single, monoclonal strain.
Sequences obtained by this method must be subjected to either pre- or post-assembly separation into groups representing the distinct genomes present in the dataset. This separation, called binning, can be carried out based either on methods relying on comparisons to databases constructed from genome references or on unsupervised algorithms that consider differences in sequence composition and/or the differential profiling of genomic coverage (Drögue and McHardy, 2012; Mande et al., 2012; Albertsen et al., 2013; Alneberg et al., 2014; Nielsen et al., 2014). Metagenomic binning algorithms now enable the identification and retrieval of cyanobacterial genomes among the metagenomes of microbial consortia, and thus allow to bypass requirements for axenic cultures. This strategy has great potential for accelerating genomic research and has been successfully employed in the characterization of cyanobacterial genomes in mixed cultures in some recent work (Grim and Dick, 2016; Uyl et al., 2016).
Strategies for the Genomics of Axenic Cultures
If all associated microorganisms have been removed and a cyanobacterium has been successfully isolated into an axenic culture, a relatively straightforward approach can be employed in its genomic characterization (Figure 3A). Virtually, the only challenges that need to be overcome are those brought by the unique characteristics of the target genome, such as total genomic size, presence and number of extrachromosomal elements, occurrence of repeated regions, and type and abundance of mobile genetic elements, among others. Thus, standard practices in microbial genomics can be readily applied without major modifications, except for some techniques for genome assembly finishing and gap closing, since reference genomes are likely unavailable or too phylogenetically distant. Even when reference genomes have been published, they are likely to present significant rearrangements (Humbert et al., 2013). Consequently, reference assemblies may produce sequences that are neither more accurate nor more complete than those obtained from de novo assemblies (Fadeev et al., 2016).
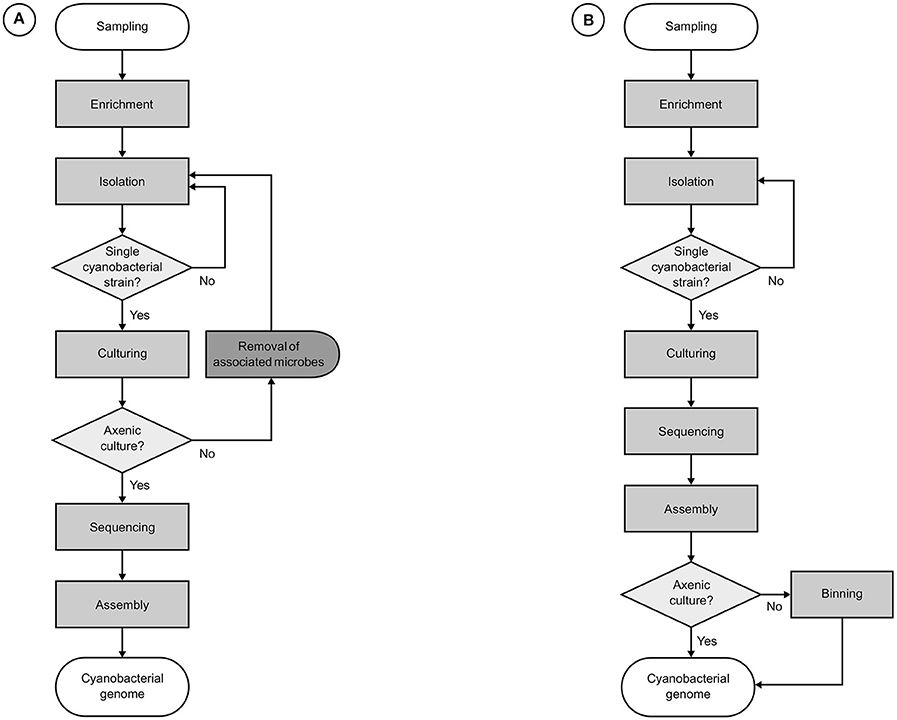
Figure 3. Workflows for the genomics of cultured cyanobacterial strains. (A) Usual roadmaps in the traditional approach, relying on the genomic sequencing of axenic cyanobacterial strains. (B) Common progression in the genomic characterization of cyanobacterial strains in non-axenic cultures. Both approaches start with the enrichment of an environmental sample by inoculation in cyanobacteria-specific culture media followed by the elimination of the majority of other organisms, leaving only a monoclonal cyanobacterial strain and its most strongly attached associates. For the workflow (A), additional procedures are required for the removal of associated microbes before genome sequencing can be carried out, usually causing major delays. Next, genomic DNA is extracted for the construction of libraries, which are submitted to a high-throughput sequencing platform. Finally, remaining sequencing reads in the filtered datasets are assembled. Workflow (B) sidesteps requirements for axenity by performing an additional binning step for the identification and retrieval of cyanobacterial sequences after assembly.
A satisfactory strategy for the genomics of axenic cyanobacterial cultures will be composed basically of read quality control, de novo assembly, scaffolding, gap closing, assembly statistics, and genome annotation. First, it is necessary to verify sequencing quality and remove from the reads regions containing bases of low quality scores or unknown bases (represented as “N” in the datasets). Acceptable sequence quality scores depend mainly on sequencing depth and technology, but Phred 20 as the very minimum quality is advised, whereas Phred 28 or higher is recommended for most analyses. We suggest using FastQC (http://www.bioinformatics.babraham.ac.uk/projects/fastqc/) for evaluating sequence quality and PRINSEQ (Schmieder and Edwards, 2011a) for filtering reads, as it is a powerful and flexible software enabling a large number of filtering parameters. FLASH (Magoč and Salzberg, 2011) can be used for merging read pairs with overlapping ends if paired-ends libraries constructed with shorter fragments are available. For genome assembly, SPAdes (Bankevich et al., 2014) currently appears to achieve the best results for most microbial genomes and metagenomes datasets obtained by Illumina sequencing. Platanus (Kajitani et al., 2014) can be employed after SPAdes assemblies for enhancing assembly by carrying out additional scaffolding and gap closing steps. QUAST (Gurevich et al., 2013) is interesting for verifying assembly statistics and comparing results from different workflows. Finally, the assembled genome can be annotated with Prokka (Seemann, 2014), which generates all files necessary for submitting the genome to NCBI, or RAST, which also provides subsystems information (Aziz et al., 2008; Overbeek et al., 2014). We also recommend using the NCBI Prokaryotic Genome Annotation Pipeline (PGAP) (Tatusova et al., 2016), since it is capable of properly identifying and annotating pseudogenes, which are commonly missed by Prokka and RAST.
Although this is viewed as the best scenario for characterizing the genome of a cyanobacterium, this strategy may still come short of producing finished chromosome and plasmid sequences. Nevertheless, draft genomes are suited to a number of analyses, including comparative genomics, as they can contain most of the genetic information of the genome (Humbert et al., 2013; Fadeev et al., 2016). Paired-ends libraries resulting in draft genomes are good enough for several applications, but differing sequencing strategies should be adopted if a finished genome is particularly indispensable for research, including mate-pair libraries, longer sequencing reads, or even BAC cloning.
Single-cell genomics has been recently reviewed as an alternative approach for sequencing cyanobacteria in natural environments (Davison et al., 2015). This strategy consists basically of the capture of a single-cell followed by routines that are similar to the ones employed for axenic strains. Unfortunately, this technology still faces methodological challenges and very few laboratories have access to or familiarity with its methods. In addition, it is also worth considering if it is really desirable to look at the genome of an individual strain and ignore the genomes of its most intimate associates, since ecological interactions are usually an important factor for determining evolutionary influence on genomic content.
Strategies for the Genomics of Non-Axenic Cultures
The main problem with the genomic sequencing of non-axenic cultures is the high number of “contaminating” sequences, which reduce the coverage of the target genome and disturb assembly by introducing increased complexity and noise. In most cases, the conventional genomic approach will be very hard to apply in conjunction with standard practices in the culturing of cyanobacteria, which mostly rely on microbial consortia. For cyanobacteria-dominated microbial consortia, it is more adequate to employ a metagenomics-like approach (Figure 3B). This strategy has to consider additional issues besides the particularities of the target genomes, urging for precautions to be taken both before and after sequencing.
Since microbial consortia contain a mixture of genomes, metagenome sequencing must have higher throughput than genome sequencing in order to obtain satisfactory depth for individual genomes. Several platforms currently provide enough depth and coverage for metagenome sequencing. However, when the cyanobacterial genome is the main target and sequencing is performed either under budget constraints or on a platform that provides longer sequences at the cost of lower depth, it is advisable to ascertain by microbiological methods that the abundance of the cyanobacterium is high enough for its genome to be present among the sequences. In this case, a great deal of caution must be taken in the maintenance of non-axenic cultures so that the abundance of associated bacteria is kept at controllable levels. This is achieved by frequently transferring the culture to fresh media (at a frequency that varies according to the growth rates of the strain) and using mechanical techniques that eliminate the organisms not firmly attached to the cyanobacteria (like streaking and washing, among others). Time spent on further cleaning up a cyanobacterial culture eventually pays off even if it does not result in axenity when faced with limited sequencing.
The diversity of associated microbes can be initially explored in unassembled datasets, so that the relative abundance of operational taxonomic units in the datasets is estimated. FOCUS (Silva et al., 2014) and SUPER-FOCUS (Silva et al., 2015) are useful for quickly verifying diversity in the dataset before assembly and allow the previewing of the relative abundances of the different genomes in the metagenome. Due to the less than satisfactory current state of public databases, most metagenomics software will state there are several different cyanobacterial taxa in the sample instead of a single one, either in the sequencing of a new cyanobacterium or in the resequencing of a known taxon. In the case of unicyanobacterial cultures, the level of phylum should be considered for these analyses, as the still low number of related genomes, if available, most likely do not encompass the whole genomic diversity of the referenced taxon and similar sequences may be found in the genomes of some phylogenetically distant taxa, resulting in the overestimation of cyanobacterial diversity in the sample.
After sequencing, it is fundamental to pre-process the data set for eliminating not only low quality sequences with PRINSEQ as previously mentioned, but also contamination. DeconSeq (Schmieder and Edwards, 2011b) is a very useful tool for removing usual contaminants from metagenomic sequences. If the number of associated microbes is low and reference genomes are available, their sequences can be dealt with in the same way contaminating sequences are, such as mapping sequencing reads to references with Bowtie (Langmead et al., 2009) or BWA (Li and Durbin, 2009) and collecting unmapped reads. If successful, downstream analyses may follow some of the methods employed for axenic cultures. However, if the associated community is richer, binning will need to be carried out either before or after assembly. QUAST can be used for comparing results and evaluating at which point binning has to be carried out for the best results, and CheckM (Parks et al., 2014) is useful for verifying binning reliability.
A relatively intuitive approach for pre-assembly selection of cyanobacterial sequences is to fetch available reference cyanobacterial genomes and format them as a Bowtie database. Reads that are mapped to this database can then be retrieved and de novo assembled as if they were originated from an axenic culture. Although this method is commonly brought up by some researchers new to cyanobacterial genomics, most often it only produces meaningful results for taxa that have a considerable number of references available and low genomic variation. However, even in this case, binning the assembled metagenome after assembly will most often achieve better results, including when using a reference-based method. This occurs because of the likely presence of regions exclusive to that novel strain, whose reads may escape selection because of their absence in the reference genomes or of the distance of their evolutionary relationship; reference-based binning of reads before assembly would lead to the removal of a significant amount of unknown sequences, which might otherwise be connected to known sequences into contigs or scaffolds.
Metagenome reconstruction from non-axenic cyanobacterial cultures may also be carried out successfully by using SPAdes for initial assembly and Platanus for additional scaffolding and gap closing steps. After a satisfactory assembly is achieved, metagenome binning should be carried out for separating cyanobacterial sequences and sequences from associated microbes. Binnning is a crucial step in strategies that work with non-axenic cultures, as correct identification of genome sequences ensures the success of downstream annotation. The retrieval of population genomes among metagenomes and their validation has been recently reviewed (Sangwan et al., 2016), and similar methods may apply to the metagenomics of microbial consortia. A considerable diversity of bioinformatic tools is now available for performing metagenomic binning (Drögue and McHardy, 2012; Mande et al., 2012; Sangwan et al., 2016; Sedlar et al., 2017), and yet it is still hard to point to an optimal method for this step (Sangwan et al., 2016), so this step asks for increased attention.
The simplest scenario is presented when sequencing a cyanobacterium with publicly available references. However, if a sequence from a closely related species or genus is not available, references belonging to related taxonomic levels up to phylum might be used for supervised binning (Thomas et al., 2012), which allows for sequences from unreferenced cyanobacteria in unicyanobacterial cultures to be processed by a reference-based strategy. For post-assembly taxonomic assignment, Kraken (Wood and Salzberg, 2014) is usually a good choice, since it has shown good accuracy and very low levels of false positives when compared to other binning software (Lindgren et al., 2016). To facilitate genome retrieval after taxonomic assignment of the assembled sequences by Kraken, we have developed a custom Python script that enables the collection of genome sequences from select taxa among the assembled metagenome based on the Kraken output. This script is freely available at https://www.github.com/danillo-alvarenga/zeuss and can be used for retrieving either the target cyanobacterium genome, the associated community metagenome, or the genome from an associated microbe in particular that has been identified by Kraken.
Depending on the available sequencing data and genomic information, software employing reference-based methods might be more suited to resequencing, while novel taxa could be more adequately addressed by methods based on reference-free algorithms. Reference-based binning is frequently limited by poor databases, which greatly favors unsupervised strategies. Since even genomes from the same species present considerable variation (Humbert et al., 2013), reference-based assembly may not be an optimal methodology even for genomes with references available. Furthermore, modern reference-free binning software can distinguish between genomes until the taxonomic rank of species (Strous et al., 2012; Kang et al., 2015), and thus might be useful even for multicyanobacterial cultures. There are lots of binning software alternatives implementing differing algorithms and methodologies that may be employed in metagenome binning (see Tables 1, 2), and it is hard to favor one over another, but the results of different binning software may be combined and refined for more accurate results (Song and Thomas, 2017). Additionally, some of these methodologies, including those based on differential coverage, benefit from sequencing the cultures at different phases of their growth cycle, such as during log, lag, and early or late stationary phases.
After separation of the cyanobacterium genome, diversity and function analyses can be easily performed on the associated community sequences, as well as functional analyses comparing cyanobacterium and community sequences. The associated community genome can then be automatically annotated with the MG-RAST server (Meyer et al., 2008) or another metagenome annotation software. Finally, manual curation of the cyanobacterial genome after annotation by Prokka, RAST, PGAP or other resource assures the assembly of a single genome. Several pipeline options are available for the analysis and annotation of mobile genetic elements, which have been reviewed elsewhere (Alvarenga et al., in press).
Figure 4 illustrates a possible workflow for the initial genomic investigation of cultured cyanobacteria. The indications provided are based on free and open source bioinformatics tools that are readily available for use on modern GNU/Linux distributions. Please keep in mind that there is now a large diversity of bioinformatics software that can be employed in this effort, and thus many alternative programs could replace the examples given, and some steps could be repeated and reiterated for improved results. Therefore, this suggestion should be taken merely as a starting point.
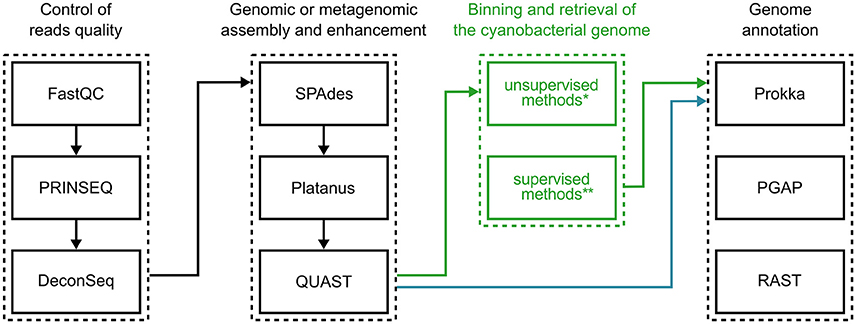
Figure 4. Suggested software for research on cyanobacterial genomics. Typical bioinformatics procedures for characterizing cyanobacterial genomes may be divided into three main steps: (1) assessment and filtering of sequencing read quality; (2) assembly of filtered reads; (3) annotation of genomic sequences. When sequencing axenic cultures, genome sequences can be evaluated right after assembly (blue arrows), while the sequencing of mixed cultures depend on binning the assembled consortium metagenome (green arrows). *For a list of software options implementing unsupervised methods, see Table 1. **See Table 2 for a list of software based on supervised methods.
Concluding Remarks
The assembly of high-throughput reads becomes easier for both genomics and metagenomics when sequencing technologies become capable of generating longer reads. New methods of library preparation, such as Illumina synthetic long reads (McCoy et al., 2014) and developing platforms from the 10x Genomics and Dovetail startups (Eisenstein, 2015), are becoming available for achieving longer reads. Likewise, new generations of sequencing technologies, including Pacific BioSciences SMRT (Rhoads and Au, 2015) and Oxford Nanopore (Laver et al., 2015; Lu et al., 2016), are focused on generating reads of increasing lengths. As sequencing technologies, assembly algorithms, metagenomics tools, and genomic databases advance, so confidence and reliability in mixed-culture assemblies increase, virtually rendering axenity dispensable. Whether this is a desirable outcome or an unfortunate side effect is arguable. If on the one hand it is likely that a smaller number of research labs will keep satisfying traditional microbiology demands and pursue axenity in cyanobacterial cultures, this change may also bring more cyanobacterial genomes to light and advance our comprehension of the molecular biology of this phylum. Nonetheless, it appears to be inevitable that metagenomics becomes a subject of central interest in cyanobacteriology, not only for the study of ecological interactions, but also for advancing knowledge on the genomics and evolution of oxyphotobacteria at an increased pace.
Author Contributions
DA, MF, and AV conceived the review. DA wrote the draft version of the manuscript. MF and AV revised the manuscript critically and provided substantial contributions. All authors read and approved the final version of the article.
Funding
This work was supported by a grant from the São Paulo Research Foundation (FAPESP) to MF (#2013/50425-8). DA was supported by a FAPESP graduate fellowship (#2011/08092-6) and a post-doctoral fellowship (#2015/14600-5). MF and AV thank the Conselho Nacional de Desenvolvimento Científico e Tecnológico (CNPq) for research fellowships (#310244/2015-3 and #302599/2016-9, respectively).
Conflict of Interest Statement
The authors declare that the research was conducted in the absence of any commercial or financial relationships that could be construed as a potential conflict of interest.
Acknowledgments
We would like to thank Prof. Dr. Célia Regina Montes from CENA-USP for assisting with scanning electron microscopy and Emily McKinney for proofreading the manuscript. We would also like to thank the peer reviewers for their critical comments, which have greatly improved the manuscript.
References
Adams, D. G. (2000). “Symbiotic interactions,” in The Ecology of Cyanobacteria, eds B. A. Whitton and M. Potts (Dordrecht: Kluwer Academic), 523–561.
Adams, D. G., Bergman, B., Nierzwicki-Bauer, S. A., Duggan, P. S., Rai, A. N., and Schüssler, A. (2013). “Cyanobacterial-plant symbioses,” in The Prokaryotes 1, eds E. Rosenberg, E. F. Delong, S. Lory, E. Stackbrandt, and F. Thompson (Heidelberg: Springer), 359–400.
Ainsworth, D., Sternberg, M. J. E., Raczy, C., and Butcher, S. A. (2016). k-SLAM: accurate and ultra-fast taxonomic classification and gene identification for large metagenomic data sets. Nucleic Acids Res. 45, 1649–1656. doi: 10.1093/nar/gkw1248
Albertsen, M., Hugenholtz, P., Skarshewski, A., Nielsen, K. L., Tyson, G. W., and Nielsen, P. H. (2013). Genome sequences of rare, uncultured bacteria obtained by differential coverage binning of multiple metagenomes. Nat. Biotechnol. 31, 533–538. doi: 10.1038/nbt.2579
Alda, J. A. G. O., Esteban, R., Diago, M. L., and Houmard, J. (2014). The plastid ancestor originated among one of the major cyanobacterial lineages. Nat. Comm. 5:4937. doi: 10.1038/ncomms5937
Alneberg, J., Bjarnason, B. S., De Bruijn, I., Schirmer, M., Quick, J., Ijaz, U. Z., et al. (2014). Binning metagenomic contigs by coverage and composition. Nat. Meth. 11, 1144–1146. doi: 10.1038/nmeth.3103
Alvarenga, D. O., Moreira, L. M., Chandler, M., and Varani, A. M. (in press). “A practical guide for comparative genomics of mobile genetic elements in prokaryotic genomes,” in Methods in Molecular Biology: Comparative Genomics, eds J. C. Setubal, J. Stoye, P. F. Stadler (Totowa, NJ: Humana Press).
Ames, S. K., Hysom, D. A., Gardner, S. N., Lloyd, G. S., Gokhale, M. B., and Allen, J. E. (2013). Scalable metagenomic taxonomy classification using a reference genome database. Bioinformatics 29, 2253–2260. doi: 10.1093/bioinformatics/btt389
Aziz, R. K., Bartels, D., Best, A. A., Dejongh, M., Disz, T., Edwards, R. A., et al. (2008). The RAST server: rapid annotations using subsystems technology. BMC Genomics 9:75. doi: 10.1186/1471-2164-9-75
Bagatini, I. L., Eiler, A., Bertilsson, S., Klaveness, D., Tessarolli, L. P., and Vieira, A. A. H. (2014). Host-specificity and dynamics in bacterial communities associated with bloom-forming freshwater phytoplankton. PLoS ONE 9:e85950. doi: 10.1371/journal.pone.0085950
Banack, S. A., Metcalf, J. A., Jiang, L., Craighead, D., Ilag, L. L., and Cox, P. A. (2012). Cyanobacteria produce N-(2-aminoethyl)glycine, a backbone for peptide nucleic acids which may have been the first genetic molecules for life on Earth. PLoS ONE 7:e49043. doi: 10.1371/journal.pone.0049043
Bankevich, A., Nurk, S., Antipov, D., Gurevich, A. A., Dvorkin, M., Kulikov, A. S., et al. (2014). SPAdes: a new genome assembly algorithm and its applications to single-cell sequencing. J. Comput. Biol. 19, 455–477. doi: 10.1089/cmb.2012.0021
Baran, R., Ivanova, N. N., Jose, N., Garcia-Pichel, F., Kyrpides, N. C., Gugger, M., et al. (2013). Functional genomics of novel secondary metabolites from diverse cyanobacteria using untargeted metabolomics. Mar. Drugs 11, 3617–3631. doi: 10.3390/md11103617
Barrick, J. E., Yu, D. S., Yoon, S. H., Jeong, H., Oh, T. K., Schneider, D., et al. (2009). Genome evolution and adaptation in a long-term experiment with Escherichia coli. Nature 461, 1243–1249. doi: 10.1038/nature08480
Berg, K. A., Lyra, C., Sivonen, K., Paulin, L., Suomalainen, S., Tuomi, P., et al. (2009). High diversity of cultivable heterotrophic bacteria in association with cyanobacterial water blooms. ISME J. 3, 314–325. doi: 10.1038/ismej.2008.110
Bergman, B., Rai, A. N., and Rasmussen, U. (2007). “Cyanobacterial associations,” in Associative and Endophytic Nitrogen-Fixing Bacteria and Cyanobacterial Associations, eds C. Elmerich and W. E. Newton (New York, NY: Springer), 257–301.
Brady, A., and Salzberg, S. L. (2011). PhymmBL expanded: confidence scores, custom databases, parallelization and more. Nat. Meth. 8:367. doi: 10.1038/nmeth0511-367
Brenner, K., You, L., and Arnold, F. H. (2008). Engineering microbial consortia: a new frontier in synthetic biology. Trends Biotechnol. 26, 483–489. doi: 10.1016/j.tibtech.2008.05.004
Briand, E., Humbert, J. F., Tambosco, K., Bormans, M., and Gerwick, W. H. (2016). Role of bacteria in the production and degradation of Microcystis cyanopeptides. Microbiology Open 5, 469–478. doi: 10.1002/mbo3.343
Calteau, A., Fewer, D. P., Latifi, A., Coursin, T., Laurent, T., Jokela, J., et al. (2014). Phylum-wide comparative genomics unravel the diversity of secondary metabolism in Cyanobacteria. BMC Genomics 15:977. doi: 10.1186/1471-2164-15-977
Cardona, T., Murray, J. W., and Rutherford, A. W. (2015). Origin and evolution of water oxidation before the last common ancestor of the cyanobacteria. Mol. Biol. Evol. 32, 1310–1328. doi: 10.1093/molbev/msv024
Chatterji, S., Yamazaki, I., Bai, Z., and Eisen, J. A. (2008). “CompostBin: a DNA composition-based algorithm for binning environmental shotgun reads,” in Lecture Notes in Computer Science: Research in Computational Molecular Biology, eds M. Vingron and L. Wong (Berlin: Springer), 17–28.
Choi, G. G., Bae, M. S., Ahn, C. Y., and Oh, H. M. (2008). Induction of axenic culture of Arthrospira (Spirulina) platensis based on antibiotic sensitivity of contaminating bacteria. Biotechnol. Lett. 30, 87–92. doi: 10.1007/s10529-007-9523-2
Chun, J., and Rainey, F. A. (2014). Integrating genomics into the taxonomy and systematics of the Bacteria and Archaea. Int. J. Syst. Evol. Microbiol. 64, 316–324. doi: 10.1099/ijs.0.054171-0
Cohen, Y., and Gurevitz, M. (2006). “The cyanobacteria—ecology, physiology and molecular genetics,” in The Prokaryotes, Vol. 4, eds M. Dworkin, S. Falkow, E. Rosenberg, K. H. Schleifer, and E. Stackebrandt (New York, NY: Springer), 1074–1098.
Cole, J. K., Hutchison, J. R., Renslow, R. S., Kim, Y. M., Chrisler, W. B., Engelmann, H. E., et al. (2014). Phototrophic biofilm assembly in microbial-mat-derived unicyanobacterial consortia: model systems for the study of autotroph-heterotroph interactions. Front. Microbiol. 5:109. doi: 10.3389/fmicb.2014.00109
Costa, N. B., Kolman, M. A., and Giani, A. (2016). Cyanobacteria diversity in saline alkaline lakes in the Brazilian Pantanal wetland: a polyphasic approach. J. Plankton Res. 38, 1389–1403. doi: 10.1093/plankt/fbw066
D'Agostino, P., Woodhouse, J. N., Makower, A. K., Yeung, A. C. Y., Ongley, S. E., Micallef, M. L., et al. (2016). Advances in genomics, transcriptomics and proteomics of toxin-producing cyanobacteria. Environ. Microbiol. Rep. 8, 3–13. doi: 10.1111/1758-2229.12366
Dagan, T., Roettger, M., Stucken, K., Landan, G., Koch, R., Major, P., et al. (2013). Genomes of stigonematalean cyanobacteria (subsection V) and the evolution of oxygenic photosynthesis from prokaryotes to plastids. Genome Biol. Evol. 5, 31–44. doi: 10.1093/gbe/evs117
Davenport, C. F., Neugebauer, J., Beckmann, N., Friedrich, B., Kameri, B., Kokott, S., et al. (2012). Genometa – a fast and accurate classifier for short metagenomic shotgun reads. PLoS ONE 7:e41224. doi: 10.1371/journal.pone.0041224
Davison, M., Hall, E., Zare, R., and Bhaya, D. (2015). Challenges of metagenomics and single-cell genomics approaches for exploring cyanobacterial diversity. Photosynth. Res. 126, 135–146. doi: 10.1007/s11120-014-0066-9
De Filippo, C., Ramazzotti, M., Fontana, P., and Cavalieri, D. (2012). Bioinformatic approaches for functional annotation and pathway inference in metagenomics data. Brief. Bioinform. 13, 696–710. doi: 10.1093/bib/bbs070
Di Rienzi, S. C., Sharon, I., Wrighton, K. C., Koren, O., Hug, L. A., Thomas, B. C., et al. (2013). The human gut and groundwater harbor non-photosynthetic bacteria belonging to a new candidate phylum sibling to Cyanobacteria. eLife 2:e01102. doi: 10.7554/eLife.01102
Dittmann, E., Fewer, D. P., and Neilan, B. A. (2013). Cyanobacterial toxins: biosynthetic routes and evolutionary roots. FEMS Microbiol. Rev. 37, 23–43. doi: 10.1111/j.1574-6976.2012.12000.x
Dittmann, E., Gugger, M., Sivonen, K., and Fewer, D. P. (2015). Natural product biosynthetic diversity and comparative genomics of the cyanobacteria. Trends Microbiol. 23, 642–652. doi: 10.1016/j.tim.2015.07.008
Dröge, J., Gregor, I., and McHardy, A. C. (2014). Taxator-tk: precise taxonomic assignment of metagenomes by fast approximation of evolutionary neighborhoods. Bioinformatics 31, 817–824. doi: 10.1093/bioinformatics/btu745
Drögue, J., and McHardy, A. C. (2012). Taxonomic binning of metagenome samples generated by next-generation sequencing technologies. Brief. Bioinform. 13, 646–655. doi: 10.1093/bib/bbs031
Eisenstein, M. (2015). Startups use short-read data to expand long-read sequencing market. Nat. Biotechnol. 33, 433–435. doi: 10.1038/nbt0515-433
Fadeev, E., De Pascale, F., Vezzi, A., Hübner, S., Aharonovich, D., and Sher, D. (2016). Why close a bacterial genome? The plasmid of Alteromonas macleodii HOT1A3 is a vector for inter-specific transfer of a flexible genomic island. Front. Microbiol. 7:248. doi: 10.3389/fmicb.2016.00248
Faust, K., and Raes, J. (2012). Microbial interactions: from networks to models. Nat. Rev. Microbiol. 10:538–550. doi: 10.1038/nrmicro2832
Fischer, W. W., Hemp, J., and Johnson, J. E. (2016). Evolution of oxygenic photosynthesis. Annu. Rev. Earth Planet Sci. 44, 647–683. doi: 10.1146/annurev-earth-060313-054810
Freitas, T. A., Li, P. E., Scholz, M. B., and Chain, P. S. G. (2015). Accurate read-based metagenome characterization using a hierarchical suite of unique signatures. Nucleic Acids Res. 43:e69. doi: 10.1093/nar/gkv180
Gerlach, W., and Stoye, J. (2011). Taxonomic classification of metagenomic shotgun sequences with CARMA3. Nucleic Acids Res. 39:e91. doi: 10.1093/nar/gkr225
Gonzalez-Esquer, C. R., Smarda, J., Rippka, R., Axen, S. D., Guglielmi, G., Gugger, M., et al. (2016). Cyanobacterial ultrastructure in light of genomic sequence data. Photosynth. Res. 129, 147–157. doi: 10.1007/s11120-016-0286-2
Graham, E., Heidelberg, J., and Tully, B. (2017). BinSanity: unsupervised clustering of environmental microbial assemblies using coverage and affinity propagation. PeerJ 5:e3035. doi: 10.7717/peerj.3035
Gregor, I., Dröge, J., Schirmer, M., Quince, C., and McHardy, A. C. (2016). PhyloPythiaS+: a self-training method for the rapid reconstruction of low-ranking taxonomic bins from metagenomes. PeerJ 4:e1603. doi: 10.7717/peerj.1603
Griese, M., Lange, C., and Soppa, J. (2011). Ploidy in cyanobacteria. FEMS Microbiol. Lett. 323, 124–131. doi: 10.1111/j.1574-6968.2011.02368.x
Grim, S. L., and Dick, G. J. (2016). Photosynthetic versatility in the genome of Geitlerinema sp. PCC 9228 (formely Oscillatoria limnetica ‘Solar Lake’), a model anoxygenic photosynthetic cyanobacterium. Front. Microbiol. 7:1546. doi: 10.3389/fmicb.2016.01546
Gupta, R. S. (2009). Protein signatures (molecular synapomorphies) that are distinctive characteristics of the major cyanobacterial clades. Int. J. Syst. Evol. Microbiol. 59, 2510–2526. doi: 10.1099/ijs.0.005678-0
Gupta, R. S., and Mathews, D. W. (2010). Signature proteins for the major clades of Cyanobacteria. BMC Evol. Biol. 10:24. doi: 10.1186/1471-2148-10-24
Gurevich, A., Saveliev, V., Vyahhi, N., and Tesler, G. (2013). QUAST: quality assessment tool for genome assemblies. Bioinformatics 29, 1072–1075. doi: 10.1093/bioinformatics/btt086
Hamilton, T. L., Bryant, D. A., and Macalady, J. L. (2016). The role of biology in planetary evolution: cyanobacterial primary production in low-oxygen Proterozoic oceans. Environ. Microbiol. 18, 325–340. doi: 10.1111/1462-2920.13118
Harel, A., Karkar, S., Cheng, S., Falkowski, P. G., and Bhattacharya, D. (2015). Deciphering primordial cyanobacterial genome functions from protein network analysis. Curr. Biol. 25, 628–634. doi: 10.1016/j.cub.2014.12.061
Hartmann, M., Gomez-Pereira, P., Grob, C., Ostrowski, M., Scanlan, D. J., and Zubkov, M. V. (2014). Efficient CO2 fixation by surface Prochlorococcus in the Atlantic ocean. ISME J. 8, 2280–2289. doi: 10.1038/ismej.2014.56
Heck, K., Machineski, G. S., Alvarenga, D. O., Vaz, M. G. M. V., Varani, A. M., and Fiore, M. F. (2016). Evaluating methods for purifying cyanobacterial cultures by qPCR and high-throughput Illumina sequencing. J. Microbiol. Meth. 129, 55–60. doi: 10.1016/j.mimet.2016.07.023
Hirose, Y., Katayama, M., Ohtsubo, Y., Misawa, N., Iioka, E., Suda, W., et al. (2015). Complete genome sequence of cyanobacterium Geminocystis sp. strain NIES-3709, which harbors a phycoerythrin-rich phycobilisome. Genome Announc. 3:e00385-15. doi: 10.1128/genomeA.00385-15
Hoffmann, L., Komárek, J., and Kaštovský, J. (2005). System of cyanoprokaryotes (Cyanobacteria) – state in 2004. Algol. Stud. 117, 95–115. doi: 10.1127/1864-1318/2005/0117-0095
Holland, A., and Kinnear, S. (2013). Interpreting the possible ecological role(s) of cyanotoxins: compounds for competitive advantage and/or physiological aide? Mar. Drugs 11, 2239–2258. doi: 10.3390/md11072239
Hug, L. A., Baker, B. J., Anantharaman, K., Brown, C. T., Probst, A. J., Castelle, C. J., et al. (2016). A new view of the three of life. Nat. Microbiol. 1:16048. doi: 10.1038/nmicrobiol.2016.48
Humbert, J. F., Barbe, V., Latifi, A., Gugger, M., Calteau, A., Coursin, T., et al. (2013). A tribute to the disorder in the genome of the bloom-forming freshwater cyanobacterium Microcystis aeruginosa. PLoS ONE 8:e70747. doi: 10.1371/journal.pone.0070747
Huson, D. H., Beier, S., Flade, I., Górska, A., El-Hadidi, M., Mitra, S., et al. (2016). MEGAN Community Edition – interactive exploration and analysis of large-scale microbiome sequencing data. PLoS Comput. Biol. 12:e1004957. doi: 10.1371/journal.pcbi.1004957
Imelfort, M., Parks, D., Woodcroft, B. J., Dennis, P., Hugenholtz, P., and Tyson, G. W. (2014). GroopM: an automated tool for the recovery of population genomes from related metagenomes. PeerJ 2:e603. doi: 10.7717/peerj.603
Kajitani, R., Toshimoto, K., Noguchi, H., Toyoda, A., Ogura, Y., Okuno, M., et al. (2014). Efficient de novo assembly of highly heterozygous genomes from whole-genome shotgun short reads. Genome Res. 24, 1384–1395. doi: 10.1101/gr.170720.113
Kaneko, T., Sato, S., Kotani, H., Tanaka, A., Asamizu, E., Nakamura, Y., et al. (1996). Sequence analysis of the genome of the unicellular cyanobacterium Synechocystis sp. strain PCC6803. II. Sequence determination of the entire genome and assignment of potential protein-coding regions. DNA Res. 3, 109–136. doi: 10.1093/dnares/3.3.109
Kang, D. D., Froula, K., Egan, R., and Wang, Z. (2015). MetaBAT, an efficient tool for accurately reconstructing single genomes from complex microbial communities. PeerJ 3:e1165. doi: 10.7717/peerj.1165
Karlson, A. M. L., Duberg, J., Motwani, N. H., Hogfors, H., Klawonn, I., Ploug, H., et al. (2015). Nitrogen fixation by cyanobacteria stimulates production in Baltic food webs. AMBIO 44, S413–S426. doi: 10.1007/s13280-015-0660-x
Kauff, F., and Büdel, B. (2011). Phylogeny of cyanobacteria: an overview. Prog. Bot. 72, 209–224. doi: 10.1007/978-3-642-13145-5_8
Kaufman, A. J. (2014). Early Earth: cyanobacteria at work. Nat. Geosci. 7, 253–254. doi: 10.1038/ngeo2128
Kelley, D. R., and Salzberg, S. L. (2010). Clustering metagenomic sequences with interpolated Markov models. BMC Bioinformatics 11:544. doi: 10.1186/1471-2105-11-544
Kim, D., Song, L., Breitwieser, F. P., and Salzberg, S. K. (2016). Centrifuge: rapid and sensitive classification of metagenomic sequences. Genome Res. 26, 1721–1729. doi: 10.1101/gr.210641.116
Kislyuk, A., Bhatnagar, S., Dushoff, J., and Weitz, J. S. (2009). Unsupervised statistical clustering of environmental shotgun sequences. BMC Bioinformatics 10:316. doi: 10.1186/1471-2105-10-316
Koboldt, D. C., Steinberg, K. M., Larson, D. E., Wilson, R. K., and Mardis, E. R. (2013). The next-generation sequencing revolution and its impact on genomics. Cell 155, 27–38. doi: 10.1016/j.cell.2013.09.006
Komárek, J. (2005). The modern classification of cyanoprokaryotes (Cyanobacteria). Oceanol. Hidrobiol. Stud. 234, 5–17. doi: 10.1080/09670262.2016.1163738
Komárek, J. (2016). A polyphasic approach for the taxonomy of cyanobacteria: principles and applications. Eur. J. Phycol. 51, 346–353. doi: 10.1080/09670262.2016.1163738
Komárek, J., Kaštovský, J., Mareš, J., and Johansen, J. R. (2014). Taxonomic classification of cyanoprokaryotes (cyanobacterial genera) 2014, using a polyphasic approach. Preslia 86, 295–335.
Koonin, E. V. (2009). Evolution of genome architecture. Int. J. Biochem. Cell Biol. 41, 298–306. doi: 10.1016/j.biocel.2008.09.015
Kormas, K., and Lymperopoulou, D. S. (2013). Cyanobacterial toxin degrading bacteria: who are they? BioMed. Res. Int. 2013:463894. doi: 10.1155/2013/463894
Laczny, C. C., Sternal, T., Plugaru, V., Gawron, P., Atashpendar, A., Margossian, H. H., et al. (2015). VizBin – an application for reference-independent visualization and human-augmented binning of metagenomic data. Microbiome 3:1. doi: 10.1186/s40168-014-0066-1
Land, M., Hauser, L., Jun, S. R., Nookaew, I., Leuze, M. R., Ahn, T. H., et al. (2015). Insights from 20 years of bacterial genome sequencing. Funct. Integr. Genomics 15, 141–161. doi: 10.1007/s10142-015-0433-4
Langmead, B., Trapnell, C., Pop, M., and Salzberg, S. L. (2009). Ultrafast and memory-efficient alignment of short DNA sequences to the human genome. Genome Biol. 10:R25. doi: 10.1186/gb-2009-10-3-r25
Larsson, J., Nylander, J. A. A., and Bergman, B. (2011). Genome fluctuations in cyanobacteria reflect evolutionary developmental and adaptive traits. BMC Evol. Biol. 11:187. doi: 10.1186/1471-2148-11-187
Laver, T., Harrison, J., O'Neill, P. A., Moore, K., Farbos, A., Paszkiewicz, K., et al. (2015). Assessing the performance of the Oxford Nanopore Technologies MinION. Biomol. Detec. Quantif. 3, 1–8. doi: 10.1016/j.bdq.2015.02.001
Leão, P. N., Engene, N., Antunes, A., Gerwick, W. H., and Vasconcelos, V. (2012). The chemical ecology of cyanobacteria. Nat. Prod. Rep. 29, 372–391. doi: 10.1039/c2np00075j
Lehmann, R., Machné, R., and Herzel, H. (2014). The structural code of cyanobacterial genomes. Nucleic Acids Res. 42, 8873–8883. doi: 10.1093/nar/gku641
Li, H., and Durbin, R. (2009). Fast and accurate short read alignment with Burrows-Wheeler transform. Bioinformatics 25, 1754–1760. doi: 10.1093/bioinformatics/btp324
Lin, H. H., and Liao, Y. C. (2016). Accurate binning of metagenomic contigs via automated clustering sequences using information of genomic signatures and marker genes. Sci. Rep. 6:24175. doi: 10.1038/srep24175
Lin, S., Hass, S., Zemojtek, T., Xiao, P., Vingron, M., and Li, R. (2010). Genome-wide comparison of cyanobacterial transposable elements, potential genetic diversity indicators. Gene 473, 139–149. doi: 10.1016/j.gene.2010.11.011
Lindgren, S., Adair, K. L., and Gardner, P. P. (2016). An evaluation of the accuracy and speed of metagenome analysis tools. Sci. Rep. 6:19233. doi: 10.1038/srep19233
Liu, B., Gibbons, T., Ghodsi, M., Treangen, T., and Pop, M. (2011). Accurate and fast estimation of taxonomic profiles from metagenomic shotgun sequences. BMC Genomics 12:S4. doi: 10.1186/1471-2164-12-S2-S4
Lu, H., Giordano, F., and Ning, Z. (2016). Oxford Nanopore MinION sequencing and genome assembly. Genom. Proteom. Bioinform. 14, 265–279. doi: 10.1016/j.gpb.2016.05.004
Lu, Y. Y., Chen, T., Fuhrman, J. A., and Sun, F. (2017). COCACOLA: binning metagenomic contigs using sequence COmposition, read CoverAge, CO-alignment and paired-end read LinkAge. Bioinformatics 33, 791–798. doi: 10.1093/bioinformatics/btw290
MacDonald, N. J., Parks, D. H., and Beiko, R. G. (2012). Rapid identification of high-confidence taxonomic assignments for metagenomic data. Nucleic Acids Res. 14:e111. doi: 10.1093/nar/gks335
Magoč, T., and Salzberg, S. L. (2011). FLASH: fast length adjustment of short reads to improve genome assemblies. Bioinformatics 27, 2957–2963. doi: 10.1093/bioinformatics/btr507
Mande, S. S., Mohammed, M. H., and Ghosh, T. S. (2012). Classification of metagenomic sequences: methods and challenges. Brief. Bioinform. 13, 669–681. doi: 10.1093/bib/bbs054
McCoy, R. C., Taylor, R. W., Blauwkamp, T. A., Kelley, J. L., Kertesz, M., Pushkarev, D., et al. (2014). Illumina TruSeq Synthetic Long-Reads empower de novo assembly and resolve complex, highly-repetitive transposable elements. PLoS ONE 9:e106689. doi: 10.1371/journal.pone.0106689
McHardy, A. C., and Rigoutsos, I. (2010). What's in the mix: phylogenetic classification of metagenome sequence samples. Curr. Opin. Microbiol. 10, 499–503. doi: 10.1016/j.mib.2007.08.004
McNeill, J., Barrie, F. R., Buck, W. R., Demoulin, V., Greuter, W., Hawksworth, D. L., et al. (2012). International Code of Nomenclature of algae, fungi, and plants (Melbourne Code). Regnum Vegetabile 154, 208.
Meeks, J. C., Elhai, J., Thiel, T., Potts, M., Larimer, F., Lamerdin, J., et al. (2001). An overview of the genome of Nostoc punctiforme, a multicellular, symbiotic cyanobacterium. Photosynth. Res. 70, 85–106. doi: 10.2172/841015
Méjean, A., and Ploux, O. (2013). A genomic view of secondary metabolite production in cyanobacteria. Adv. Bot. Res. 65, 189–234. doi: 10.1016/B978-0-12-394313-2.00006-8
Merel, S., Walker, D., Chicana, R., Snyder, S., Baurès, E., and Thomas, O. (2013). State of knowledge and concerns on cyanobacterial blooms and cyanotoxins. Environ. Int. 59, 303–327. doi: 10.1016/j.envint.2013.06.013
Meyer, F., Paarmann, D., D'Souza, M., Olson, R., Glass, E. M., Kubal, M., et al. (2008). The metagenomics RAST server – a public resource for the automatic phylogenetic and functional analysis of metagenomes. BMC Bioinformatics 9:386. doi: 10.1186/1471-2105-9-386
Micallef, M. L., D'Agostino, P. M., Al-Sinawi, B., Neilan, B. A., and Moffitt, M. C. (2015). Exploring cyanobacterial genomes for natural product biosynthesis pathways. Mar. Genomics 21, 1–12. doi: 10.1016/j.margen.2014.11.009
Mishra, S., Bhargava, P., Adhikary, S. P., Pradeep, A., and Rai, L. C. (2015). Weighted morphology: a new approach towards phylogenetic assessment of Nostocales (Cyanobacteria). Protoplasma 252, 145–163. doi: 10.1007/s00709-014-0629-9
Mohammed, M. H., Ghosh, T. S., Komanduri, D., and Mande, S. S. (2009). SOrt-ITEMS: sequence orthology based approach for improved taxonomic estimation of metagenomic sequences. Bioinformatics 25, 1722–1730. doi: 10.1093/bioinformatics/btp317
Moss, N. A., Bertin, M. J., Kleigrewe, K., Leão, T. F., Gerwick, L., and Gerwick, W. H. (2016). Integrating mass spectrometry and genomics for cyanobacterial metabolite discovery. J. Ind. Microbiol. Biotechnol. 43, 313–324. doi: 10.1007/s10295-015-1705-7
Nagarkar, S., Williams, G. A., Subramanian, G., and Saha, S. K. (2004). Cyanobacteria-dominated biofilms: a high quality food resource for intertidal grazers. Hydrobiologia 512, 89–95. doi: 10.1023/B:HYDR.0000020313.09924.c1
Nalbantoglu, O. U., Way, S. F., Hinrichs, S. H., and Sayood, K. (2011). RAIphy: phylogenetic classification of metagenomics samples using iterative refinement of relative abundance index profiles. BMC Bioinformatics 12:41. doi: 10.1186/1471-2105-12-41
Neilan, B. A., Pearson, L. A., Muenchhoff, J., Moffitt, M. C., and Dittmann, E. (2013). Environmental conditions that influence toxin biosynthesis in cyanobacteria. Environ. Microbiol. 15, 1239–1253. doi: 10.1111/j.1462-2920.2012.02729.x
Nielsen, H. B., Almeida, M., Juncker, A. S., Rasmussen, S., Li, J., Sunagawa, S., et al. (2014). Identification and assembly of genomes and genetic elements in complex metagenomic samples without using reference genomes. Nat. Biotechnol. 32, 822–828. doi: 10.1038/nbt.2939
Nunnery, J. K., Mevers, E., and Gerwick, W. H. (2010). Biologically active secondary metabolites from marine cyanobacteria. Curr. Opin. Biotechnol. 21, 787–793. doi: 10.1016/j.copbio.2010.09.019
Oren, A. (2011). Cyanobacterial systematics and nomenclature as featured in the international bulletin of bacteriological nomenclature and taxonomy / international journal of systematic bacteriology / international journal of systematic and evolutionary microbiology. Int. J. Syst. Evol. Microbiol. 61, 10–15. doi: 10.1099/ijs.0.018838-0
Otsuka, S., Abe, Y., Fukui, R., Nishiyama, M., and Senoo, K. (2008). Presence of previously undescribed bacterial taxa in non-axenic Chlorella cultures. J. Gen. Appl. Microbiol. 54, 187–193. doi: 10.2323/jgam.54.187
Ounit, R., and Lonardi, S. (2016). Higher classification sensitivity of short metagenomic reads with CLARK-S. Bioinformatics 32, 2823–3825. doi: 10.1093/bioinformatics/btw542
Overbeek, R., Olson, R., Pusch, G. D., Olsen, G. J., Davis, D. J., Disz, T., et al. (2014). The SEED and the rapid annotation of microbial genomes using subsystems technology (RAST). Nucleic Acids Res. 42, D206–D214. doi: 10.1093/nar/gkt1226
Paerl, H. W., and Otten, T. G. (2013). Harmful cyanobacterial blooms: causes, consequences, and controls. Microb. Ecol. 65, 995–1010. doi: 10.1007/s00248-012-0159-y
Paerl, H. W., Pinckney, J. L., and Steppe, T. F. (2000). Cyanobacterial-bacterial mat consortia: examining the functional unit of microbial survival and growth in extreme environments. Environ. Microbiol. 2, 11–26. doi: 10.1046/j.1462-2920.2000.00071.x
Palinska, K. A., and Surosz, W. (2014). Taxonomy of cyanobacteria: a contribution to consensus approach. Hydrobiologia 740, 1–11. doi: 10.1007/s10750-014-1971-9
Parker, C. T., Tindall, B. J., and Garrity, G. M. (2015). International code of nomenclature of prokaryotes. Int. J. Syst. Evol. Microbiol. doi: 10.1099/ijsem.0.000778
Parks, D. H., Imelfort, D., Skennerton, C. T., Hugenholtz, P., and Tyson, G. W. (2014). CheckM: assessing the quality of microbial genomes recovered from isolates, single cells, and metagenomes. Genome Res. 25, 1043–1055. doi: 10.1101/gr.186072.114
Pati, A., Heath, L. S., Kyrpides, N. C., and Ivanova, N. (2011). ClaMS: a classifier for metagenomic sequences. Stand. Genomic Sci. 5, 248–253. doi: 10.4056/sigs.2075298
Pearson, L. A., Dittmann, E., Mazmouz, R., Ongley, S. E., D'Agostino, P. M., and Neilan, B. A. (2016). The genetics, biosynthesis and regulation of toxic specialized metabolites of cyanobacteria. Harmful Algae 54, 98–111. doi: 10.1016/j.hal.2015.11.002
Pinevich, A. V. (2015). Proposal to consistently apply the International Code of Nomenclature of Prokaryotes (ICNP) to names of the oxygenic photosynthetic bacteria (cyanobacteria), including those validly published under the International Code of Botanical Nomenclature (ICBN)/International Code of Nomenclature for algae, fungi and plants (ICN), and proposal to change Principle 2 of the ICNP. Int. J. Syst. Evol. Microbiol. 65, 1070–1074. doi: 10.1099/ijs.0.000034
Pope, P. B., and Patel, B. K. C. (2008). Metagenomic analysis of a freshwater toxic cyanobacteria bloom. FEMS Microbiol. Ecol. 64, 9–27. doi: 10.1111/j.1574-6941.2008.00448.x
Rappé, M. S., and Giovannoni, S. J. (2003). The uncultured microbial majority. Annu. Rev. Microbiol. 57, 369–394. doi: 10.1146/annurev.micro.57.030502.090759
Reddy, R. M., Mohammed, M. H., and Mande, S. S. (2012). TWARIT: an extremely rapid and efficient approach for phylogenetic classification of metagenomic sequences. Gene 505, 259–265. doi: 10.1016/j.gene.2012.06.014
Rhoads, A., and Au, K. F. (2015). PacBio sequencing and its applications. Genom. Proteom. Bioinform. 13, 278–289. doi: 10.1016/j.gpb.2015.08.002
Richards, S. (2015). It's more than stamp collecting: how genome sequencing can unify biological research. Trends Genet. 31, 411–421. doi: 10.1016/j.tig.2015.04.007
Rosen, G. L., Reichenberger, E. R., and Rosenfeld, A. M. (2010). NBC: the naïve Bayes classification tool webserver for taxonomic classification of metagenomic reads. Bioinformatics 27, 127–129. doi: 10.1093/bioinformatics/btq619
Sanger, F., Nicklen, S., and Coulson, A. R. (1977). DNA sequencing with chain-terminating inhibitors. Proc. Natl. Acad. Sci. U.S.A. 74, 5463–5467. doi: 10.1073/pnas.74.12.5463
Sangwan, N., Xia, F., and Gilbert, J. A. (2016). Recovering complete and draft population genomes from metagenome datasets. Microbiome 4:8. doi: 10.1186/s40168-016-0154-5
Schirrmeister, B. E., Antonelli, A., and Bagheri, H. C. (2011). The origin of multicellularity in cyanobacteria. BMC Evol. Biol. 11:45. doi: 10.1186/1471-2148-11-45
Schirrmeister, B. E., Gugger, M., and Donoghue, P. C. J. (2015). Cyanobacteria and the great oxidation event: evidence from genes and fossils. Palaeontology 58, 769–785. doi: 10.1111/pala.12178
Schirrmeister, B. E., Sanchez-Baracaldo, P., and Wacey, P. (2016). Cyanobacterial evolution during the Precambrian. Int. J. Astrobiol. 15, 187–204. doi: 10.1017/S1473550415000579
Schmieder, R., and Edwards, R. (2011a). Quality control and preprocessing of metagenomic datasets. Bioinformatics 27, 863–864. doi: 10.1093/bioinformatics/btr026
Schmieder, R., and Edwards, R. (2011b). Fast identification and removal of sequence contamination from genomic and metagenomic datasets. PLoS ONE 6:e17288. doi: 10.1371/journal.pone.0017288
Schreiber, F., Gumrich, P., Daniel, R., and Meinicke, P. (2010). Treephyler: fast taxonomic profiling of metagenomes. Bioinformatics 26, 960–961. doi: 10.1093/bioinformatics/btq070
Sciuto, K., and Moro, I. (2015). Cyanobacteria: the bright and dark sides of a charming group. Biodivers. Conserv. 24, 711–738. doi: 10.1007/s10531-015-0898-4
Sedlar, K., Kupkova, K., and Provaznik, I. (2017). Bioinformatics strategies for taxonomy independent binning and visualization of sequences in shotgun metagenomics. Comput. Struct. Biotechnol. J. 15, 48–55. doi: 10.1016/j.csbj.2016.11.005
Seemann, T. (2014). Prokka: rapid prokaryotic genome annotation. Bioinformatics 30, 2068–2069. doi: 10.1093/bioinformatics/btu153
Segata, N., Waldron, L., Ballarini, A., Narasimhan, V., Jousson, O., and Huttenhower, C. (2012). Metagenomic microbial community profiling using unique clade-specific marker genes. Nat. Meth. 9, 811–814. doi: 10.1038/nmeth.2066
Sena, L., Rojas, D., Montiel, E., Gonzáles, H., Moret, J., and Naranjo, L. (2008). A strategy to obtain axenic cultures of Arthrospira spp. cyanobacteria. World J. Microbiol. Biotechnol. 27, 1045–1053. doi: 10.1007/s11274-010-0549-6
Sharon, I., and Banfield, J. F. (2013). Genomes from metagenomics. Science 342, 1057. doi: 10.1126/science.1247023
Shaw, G. H. (2016). “The origin of oxygenic photosynthesis and its impact on the atmosphere,” in Earth's Early Atmosphere and Oceans, and The Origin of Life, ed G. H. Shaw (Cham: Springer), 75–93.
Shi, T., and Falkowski, P. G. (2008). Genome evolution in cyanobacteria: the stable core and the variable shell. Proc. Natl. Acad. Sci. U.S.A. 105, 2510–2515. doi: 10.1073/pnas.0711165105
Shih, P. M., Hemp, J., Ward, L. M., Matzke, N. J., and Fischer, W. W. (2017). Crown group oxyphotobacteria postdate the rise of oxygen. Geobiology 15, 19–29. doi: 10.1111/gbi.12200
Shih, P. M., Wu, D., Latifi, A., Axen, S. D., Fewer, D. P., Talla, E., et al. (2013). Improving the coverage of the cyanobacterial phylum using diversity-driven genome sequencing. Proc. Natl. Acad. Sci. U.S.A. 110, 1053–1058. doi: 10.1073/pnas.1217107110
Silva, G. G. Z., Cuevas, D. A., Dutilh, B. E., and Edwards, R. A. (2014). FOCUS: an alignment-free model to identify organisms in metagenomes using non-negative least squares. PeerJ 2:e425. doi: 10.7717/peerj.425
Silva, G. G. Z., Green, K. T., Dutilh, B. E., and Edwards, R. A. (2015). SUPER-FOCUS: a tool for agile functional analysis of shotgun metagenomic data. Bioinformatics 32, 354–361. doi: 10.1093/bioinformatics/btv584
Simm, S., Keller, M., Selymesi, M., and Schleiff, E. (2015). The composition of the global and feature specific cyanobacterial core-genomes. Front. Microbiol. 6:219. doi: 10.3389/fmicb.2015.00219
Singh, R. K., Tiwari, S. P., Rai, A. K., and Mohapatra, T. M. (2011). Cyanobacteria: an emerging source for drug discovery. J. Antibiot. 64, 401–412. doi: 10.1038/ja.2011.21
Song, H., Ding, M. Z., Jia, X. Q., Ma, Q., and Yuan, Y. J. (2014). Synthetic microbial consortia: from systematic analysis to construction and applications. Chem. Soc. Rev. 43, 6954–6981. doi: 10.1039/C4CS00114A
Song, W. H., and Thomas, T. (2017). Binning_refiner: improving genome bins through the combination of different binning programs. Bioinformatics. doi: 10.1093/bioinformatics/btx086. [Epub ahead of print].
Soo, R. M., Skennerton, C. T., Sekiguchi, Y., Imelfort, M., Paech, S. J., Dennis, P. G., et al. (2014). An expanded genomic representation of the phylum Cyanobacteria. Genome Biol. Evol. 6, 1031–1045. doi: 10.1093/gbe/evu073
Stark, M., Berger, S. A., Stamatakis, A., and von Mering, C. (2010). MLTreeMap – accurate maximum likelihood placement of environmental DNA sequences into taxonomic and functional reference phylogenies. BMC Genomics 11:461. doi: 10.1186/1471-2164-11-461
Stewart, E. J. (2012). Growing unculturable bacteria. J. Bacteriol. 194, 4151–4160. doi: 10.1128/JB.00345-12
Strous, M., Kraft, B., Bisdorf, R., and Tegetmeyer, H. E. (2012). The binning of metagenomic contigs for microbial physiology of mixed cultures. Front. Microbiol. 3:410. doi: 10.3389/fmicb.2012.00410
Stucken, K., John, U., Cembella, A., Murillo, A. A., Soto-Liebe, K., Fuentes-Valdés, J. J., et al. (2010). The smallest known genomes of multicellular and toxic cyanobacteria: comparison, minimal gene sets for linked traits and the evolutionary implications. PLoS ONE 5:e9235. doi: 10.1371/journal.pone.0009235
Sukenik, A., Kaplan-Levy, R. N., Welch, J. M., and Post, A. F. (2012). Massive multiplication of genome and ribosomes in dormant cells (akinetes) of Aphanizomenon ovalisporum (Cyanobacteria). ISME J. 6, 670–679. doi: 10.1038/ismej.2011.128
Sunagawa, S., Mende, D. R., Zeller, G., Izquierdo-Carrasco, F., Berger, S. A., Kultima, J. R., et al. (2013). Metagenomic species profiling using universal phylogenetic marker genes. Nat. Meth. 10, 1196–1199. doi: 10.1038/nmeth.2693
Sutcliffe, I. C. (2015). Challenging the anthropocentric emphasis on phenotypic testing in prokaryotic species descriptions: rip it up and start again. Front. Genet. 6:218. doi: 10.3389/fgene.2015.00218
Tatusova, T., Ciufo, S., Fedorov, B., O'Neill, K., and Tolstoy, I. (2013). RefSeq microbial genomes database: new representation and annotation strategy. Nucleic Acids Res. 42, D553–D559. doi: 10.1093/nar/gkt1274
Tatusova, T., Ciufo, S., Fedorov, B., O'Neill, K., and Tolstoy, I. (2015). RefSeq microbial genomes database: new representation and annotation strategy. Nucleic Acids Res. 43:3872. doi: 10.1093/nar/gkv278
Tatusova, T., DiCuccio, M., Badretdin, A., Chetvernin, V., Nawrocki, E. P., Zaslavsky, L., et al. (2016). NCBI prokaryotic genome annotation pipeline. Nucleic Acids Res. 44, 6614–6624. doi: 10.1093/nar/gkw569
Teeling, H., and Glöckner, O. (2012). Current opportunities and challenges in microbial metagenome analysis – a bioinformatic perspective. Brief. Bioinform. 13, 728–742. doi: 10.1093/bib/bbs039
Tenaillon, O., Barrick, J. E., Ribeck, N., Deatherage, D. E., Blanchard, J. L., Dasgupta, A., et al. (2016). Tempo and mode of genome evolution in a 50,000-generation experiment. Nature 536, 165–170. doi: 10.1038/nature18959
Thiel, T., Pratte, B. S., Zhong, J., Goodwin, L., Copeland, A., Lucas, S., et al. (2014). Complete genome sequence of Anabaena variabilis ATCC 29413. Stand. Genomic. Sci. 9, 562–573. doi: 10.4056/sigs.3899418
Thomas, T., Gilbert, J., and Meyer, F. (2012). Metagenomics – a guide from sampling to data analysis. Microb. Inform. Exp. 2:3. doi: 10.1186/2042-5783-2-3
Thompson, C. C., Amaral, G. R., Campeão, M., Edwards, R. A., Polz, M. E., Dutilh, B. E., et al. (2015). Microbial taxonomy in the post-genomic era: rebuilding from scratch? Arch. Microbiol. 197, 359–370. doi: 10.1007/s00203-014-1071-2
Tyson, G. W., Chapman, J., Hugenholtz, P., Allen, E. E., Ram, R. J., Richardson, P. M., et al. (2004). Community structure and metabolism through reconstruction of microbial genomes from the environment. Nature 428, 37–43. doi: 10.1038/nature02340
Uyl, P. A. D., Richardson, L. R., Jain, S., and Dick, G. J. (2016). Unraveling the physiological roles of the cyanobacterium Geitlerinema sp. BBD and other black band disease community members through genomic analysis of a mixed culture. PLoS ONE 6:e0157953. doi: 10.1371/journal.pone.0157953
Vandamme, P., Pot, B., Gillis, M., De Vos, P., Kersters, K., and Swings, J. (1996). Polyphasic taxonomy, a consensus approach to bacterial systematics. Microbiol. Rev. 60, 407–438.
Vartoukian, S. R., Palmer, R. M., and Wade, W. G. (2010). Strategies for the culture of “unculturable” bacteria. FEMS Microbiol. Lett. 309, 1–7. doi: 10.1111/j.1574-6968.2010.02000.x
Vázquez-Martínez, G., Rodriguez, M. H., Hernández-Hernández, F., and Ibarra, J. E. (2004). Strategy to obtain axenic cultures from field-collected samples of the cyanobacterium Phormidium animalis. J. Microbiol. Meth. 57, 115–121. doi: 10.1016/j.mimet.2003.12.003
Visser, P. M., Verspagen, J. M. H., Sandrini, G., Stal, L. J., Matthijs, H. C. P., Davis, T. W., et al. (2016). How rising CO2 and global warming may stimulate harmful cyanobacterial blooms. Harmful Algae 54, 145–159. doi: 10.1016/j.hal.2015.12.006
Wang, H., Sivonen, K., Rouhiainen, L., Fewer, D. P., Lyra, C., Rantala-Ylinen, A., et al. (2012). Genome-derived insights into the biology of the hepatotoxic bloom-forming cyanobacterium Anabaena sp. strain 90. BMC Genomics 13:613. doi: 10.1186/1471-2164-13-613
Wang, Y., Hu, H., and Li, X. (2015). MBBC: an efficient approach for metagenomic binning based on clustering. BMC Bioinformatics 16:36. doi: 10.1186/s12859-015-0473-8
Wang, Y., Leung, H. C. M., Yiu, S. M., and Chin, F. Y. L. (2012). MetaCluster 5.0: a two-round binning approach for metagenomic data for low-abundance species in a noisy sample. Bioinformatics 28, i356–i362. doi: 10.1093/bioinformatics/bts397
Waterbury, J. B. (2006). “The cyanobacteria – isolation, purification and identification,” in The Prokaryotes, Vol. 4, eds M. Dworkin, S. Falkow, E. Rosenberg, K. H. Schleifer, and E. Stackebrandt (New York, NY: Springer), 1053–1073.
Whitman, W. B. (2015). Genome sequences as the type material for taxonomic descriptions of prokaryotes. Syst. Appl. Microbiol. 38, 217–222. doi: 10.1016/j.syapm.2015.02.003
Whitman, W. B. (2016). Modest proposals to expand the type material for naming of prokaryotes. Int. J. Syst. Evol. Microbiol. 66, 2108–2112. doi: 10.1099/ijsem.0.000980
Wilson, M. C., and Piel, J. (2013). Metagenomic approaches for exploiting uncultivated bacteria as a resource for novel biosynthetic enzymology. Chem. Biol. 20, 636–647. doi: 10.1016/j.chembiol.2013.04.011
Wood, D. E., and Salzberg, S. L. (2014). Kraken: ultrafast metagenomic sequence classification using exact alignments. Genome Biol. 15:R46. doi: 10.1186/gb-2014-15-3-r46
Wu, Y. W., Tang, Y. H., Tringe, S. G., Simmons, B. A., and Singer, S. W. (2014). MaxBin: an automated binning method to recover individual genomes from metagenomes using an expectation-maximization algorithm. Microbiome 2:26. doi: 10.1186/2049-2618-2-26
Wu, Y. W., and Ye, Y. (2011). A novel abundance-based algorithm for binning metagenomic sequences using l-tuples. J. Comput. Biol. 18, 523–534. doi: 10.1089/cmb.2010.0245
Yamamuro, M. (1999). Importance of epiphytic cyanobacteria as food sources for heterotrophs in a tropical seagrass bed. Coral Reefs 18, 263–271. doi: 10.1007/s003380050191
Zaremba-Niedzwiedzka, K., Caceres, E. F., Saw, J. H., Bäckström, D., Juzokaite, L., Vancaester, E., et al. (2017). Asgard archaea illuminate the origin of eukaryotic cellular complexity. Nature 541, 353–358. doi: 10.1038/nature21031
Zehr, J. P., Bench, S. R., Carter, C. J., Hewson, I., Niazi, F., Shi, T., et al. (2008). Globally distributed uncultivated organic N2-fixing cyanobacteria lacks oxygenic photosystem II. Science 322, 1110–1112. doi: 10.1126/science.1165340
Zhaxybayeva, O., Gogarten, J. P., Charlebois, R. L., Doolittle, F., and Papke, R. T. (2006). Phylogenetic analyses of cyanobacterial genomes: quantification of horizontal gene transfer events. Genome Res. 16, 1099–1108. doi: 10.1101/gr.5322306
Zhubanova, A. A., Ernazarova, A. K., Kaiyrmanova, G. K., Zayadan, B. K., Savitskaya, I. S., Abdieva, G. Z., et al. (2013). Construction of cyanobacterial-bacterial consortium on the basis of axenic cyanobacterial cultures and heterotrophic bacteria cultures for bioremediation of oil-contaminated soils and water ponds. Russ. J. Plant. Phys. 60, 555–562. doi: 10.1134/S1021443713040183
Keywords: bioinformatics, microbial ecology, genome assembly, metagenome binning, symbiosis, microbial consortia, oxyphotobacteria
Citation: Alvarenga DO, Fiore MF and Varani AM (2017) A Metagenomic Approach to Cyanobacterial Genomics. Front. Microbiol. 8:809. doi: 10.3389/fmicb.2017.00809
Received: 14 March 2017; Accepted: 20 April 2017;
Published: 09 May 2017.
Edited by:
Ana Beatriz Furlanetto Pacheco, Federal University of Rio de Janeiro, BrazilCopyright © 2017 Alvarenga, Fiore and Varani. This is an open-access article distributed under the terms of the Creative Commons Attribution License (CC BY). The use, distribution or reproduction in other forums is permitted, provided the original author(s) or licensor are credited and that the original publication in this journal is cited, in accordance with accepted academic practice. No use, distribution or reproduction is permitted which does not comply with these terms.
*Correspondence: Marli F. Fiore, fiore@cena.usp.br
Alessandro M. Varani, amvarani@fcav.unesp.br