- 1Cell and Molecular Biology Laboratory, Center for Nuclear Energy in Agriculture, University of São Paulo, Piracicaba, Brazil
- 2Department of Biology, Institute of Ecology and Evolution, University of Oregon, Eugene, OR, USA
- 3Department of Plant, Soil and Microbial Sciences, Center for Microbial Ecology, Michigan State University, East Lansing, MI, USA
- 4Department of Microbiology, University of Massachusetts, Amherst, MA, USA
- 5Department of Land, Air and Water Resources, University of California, Davis, Davis, CA, USA
Members of the phylum Acidobacteria are among the most abundant soil bacteria on Earth, but little is known about their response to environmental changes. We asked how the relative abundance and biogeographic patterning of this phylum and its subgroups responded to forest-to-pasture conversion in soils of the western Brazilian Amazon. Pyrosequencing of 16S rRNA genes was employed to assess the abundance and composition of the Acidobacteria community across 54 soil samples taken using a spatially nested sampling scheme at the landscape level. Numerically, Acidobacteria represented 20% of the total bacterial community in forest soils and 11% in pasture soils. Overall, 15 different Acidobacteria subgroups of the current 26 subgroups were detected, with Acidobacteria subgroups 1, 3, 5, and 6 accounting together for 87% of the total Acidobacteria community in forest soils and 75% in pasture soils. Concomitant with changes in soil chemistry after forest-to-pasture conversion—particularly an increase in properties linked to soil acidity and nutrient availability—we observed an increase in the relative abundances of Acidobacteria subgroups 4, 10, 17, and 18, and a decrease in the relative abundances of other Acidobacteria subgroups in pasture relative to forest soils. The composition of the total Acidobacteria community as well as the most abundant Acidobacteria subgroups (1, 3, 5, and 6) was significantly more similar in composition across space in pasture soils than in forest soils. These results suggest that preponderant responses of Acidobacteria subgroups, especially subgroups 1, 3, 4, 5, and 6, to forest-to-pasture conversion effects in soils could be used to define management-indicators of agricultural practices in the Amazon Basin. These acidobacterial responses are at least in part through alterations on acidity- and nutrient-related properties of the Amazon soils.
Introduction
Land use change driven by human activities is considered the most important factor for biodiversity losses in the tropics (Sala et al., 2000) and a large number of studies have documented the negative effects of land use change for plants, animals (Gibson et al., 2011; Wearn et al., 2012), and most recently, microorganisms (Cenciani et al., 2009; Jesus et al., 2009; Navarrete et al., 2010, 2011, 2013, 2015; Taketani and Tsai, 2010; Rodrigues et al., 2013; Mirza et al., 2014; Mueller et al., 2014; Paula et al., 2014; Ranjan et al., 2015). For example, Rodrigues et al. (2013) reported that forest-to-pasture conversion resulted in a substantial decrease in the abundance of members of the bacterial phylum Acidobacteria.
Acidobacteria are among the most common bacteria in soils worldwide, including in Amazon soils (Kim et al., 2007; Jesus et al., 2009; Navarrete et al., 2010, 2013, 2015). The analysis of 16S rRNA gene sequences has demonstrated that acidobacterial abundance within a community may be regulated by soil pH (Fierer et al., 2007; Lauber et al., 2008; Jones et al., 2009; Rousk et al., 2010; Kuramae et al., 2011) and nutrient availability (Zhao et al., 2014). Genomic and physiological traits indicate characteristics that may contribute to Acidobacteria survival and growth in soil, such as the presence of membrane transporters and the ability to use carbon sources that span from simple sugars to more complex substrates such as hemicellulose, cellulose, and chitin; the reduction of nitrate, nitrite, and possibly nitric oxide; iron scavenging; and production of antimicrobial compounds (Ward et al., 2009; Rawat et al., 2012). In addition, Greening et al. (2015) proposed that consumption of trace gases such as H2 provides a dependable general mechanism for Acidobacteria to generate maintenance energy required for long-term survival in soils.
Recently, increased attention has been paid to the response of Acidobacteria to environmental changes (George et al., 2009; Naether et al., 2012; Catão et al., 2014). Despite this appreciation for the phylum Acidobacteria, little is still known about the differential response at subgroup level to alterations in soil chemical properties and fertility, and how their community similarity change with distance in mosaic landscapes. Navarrete et al. (2013) reported the impact of agricultural management of soybean in Amazon forest soils on the composition of the Acidobacteria community, and they revealed that the abundance of Acidobacteria subgroups was related to soil chemical properties, which were clearly affected by agricultural management. These findings opened the possibility that subgroups of Acidobacteria could be used as management-indicators for the consequences of agricultural practices in the Amazon region.
The present study was designed to assess the Acidobacteria subgroup response at different geographic scales in primary forest and pasture soils. Firstly, we hypothesized that different subgroups of Acidobacteria respond differently to forest conversion into pastures in Amazon soils. Because of the substantial effects that land use change may have on soil chemical characteristics, we evaluated the differential response of Acidobacteria subgroups through the prism of the expected changes in soil chemical properties after forest-to-pasture conversion in the Amazon. In a corollary hypothesis, we tested whether taxonomic similarity of total Acidobacteria community and of their most abundant subgroups varies across space in forest and pasture soil samples in the western Brazilian Amazon. To address these hypotheses, we used pyrosequencing of the region V4 of the bacterial 16S rRNA gene to analyze the relative abundance and composition of the Acidobacteria community inhabiting soil from primary forests and pastures collected from the Amazon Rainforest Microbial Observatory, a model site representing the current expansive agricultural development of the region. We correlated the relative abundances of Acidobacteria at the taxonomic levels phylum and subgroup with soil chemical properties to explore group-specific responses to agricultural conversion. Furthermore, we explored the relationship between group-specific biogeographic patterns and land use change by comparing distance-decay relationship patterns.
Materials and Methods
Site Description and Soil Sampling
This study was performed at the Fazenda Nova Vida (10°10′5″S and 62°49′27″W), located in the central region of the Brazilian state of Rondônia at the Amazon Rainforest Microbial Observatory (ARMO). Soils are classified as red-yellow podzolic latosol (Kandiudult). The climate is humid tropical, with an annual average temperature of 25.5°C and an average precipitation of 2200 mm (Bastos and Diniz, 1982). Local farmers employ slash-and-burn practices, i.e., clearing of primary forest followed by burning, in order to support livestock and farming systems in this region.
Soil samples were collected at the end of the rainy season (April 2009) from three primary forest sites and three pasture sites that had been continuously managed since 1987. At each site, a nested sampling scheme was established, centered on a 100 × 100 m (100 m2) quadrat, with 10 × 10 m (10 m2), and 1 × 1 m (1 m2) quadrats nested within and adjacent to one corner of the 100 m2 quadrat, for a total of nine sampling points per 100 m2 quadrat (Figure S1). At each point, after the removal of the litter layer, the soil was sampled from 0 to 10 cm depth in the topsoil layer, gently homogenized, and subdivided. Samples were transported to the laboratory on ice. A portion of each sample was stored at −80°C for molecular analysis and another portion was stored at 4°C for soil chemical analysis.
Soil Chemical Properties and Statistical Analysis
The soil samples were dried and passed through a sieve (149 μm size). Total carbon (C) and nitrogen (N) were measured on a LECO CN elemental analyzer (St. Joseph, MI, USA) at the Soil Biogeochemistry Laboratory, Center for Nuclear Energy in Agriculture, University of São Paulo, Brazil. Soil chemical properties for each sample were analyzed at the Laboratory of Soil Fertility, Luiz de Queiroz College Agriculture, University of São Paulo, Brazil. Soil pH was measured from a soil/water (1:2.5) suspension. Aluminum (Al), calcium (Ca), and magnesium (Mg) were extracted with 1 M potassium chloride. Ca and Mg were determined by atomic absorption spectrometry, while Al was determined by acid-base titration. Phosphorous (P) and potassium (K) were extracted by ion-exchange resin, and determined by colorimetry and atomic emission spectroscopy, respectively. Combined results were used for calculation of exchangeable bases (SB) as the sum of Ca, Mg, and K; cation-exchange capacity (CEC) as the sum of Ca, Mg, K, Al, and H; base saturation (V) as the percent relation between SB and CEC; aluminum saturation (m) as the percent relation between exchangeable Al and CEC; and potential acidity (H+Al), by an equation based on the pH determined in Shoemaker-McLean-Pratt (SMP) buffer solution. Analysis of similarity (ANOSIM) statistics was calculated to test for differences between forest and pasture soil chemical properties. A distance matrix (Euclidean metric) was constructed using non-transformed data. ANOSIM was carried out using Primer six (version 6.1.5, Primer-E Ltd., Plymouth, UK).
Isolation of DNA from Soil, Amplification, and Pyrosequencing of Bacterial 16S rRNA Genes
Total genomic DNA for each soil sample was extracted in triplicate using the Power Soil DNA Isolation Kit (Mo Bio Laboratories Inc., Carlsbad, CA, USA), according to the manufacturer's instructions. The extractions for each sample were combined and DNA was quantified spectrophotometrically (Nanodrop ND-1000, NanoDrop Technologies, Inc., Wilmington, DE, USA). All DNA samples were stored at −20°C. The primer set 577F (5′-AYTGGGYDTAAAGNG-3′) and 926R (5′-CCGTCAATTCMTTTRAGT-3′) targeting the V4 region of bacterial 16S rRNA gene was used for the amplification. Group-specific primers for Acidobacteria such as Acid31F (Barns et al., 1999) and ACIDO (Lee and Cho, 2011) were not used in order to avoid the selective amplification and not detection of members of the phylum Acidobacteria such as 2, 22, and 25 as reported in many studies (Sait et al., 2006; Barns et al., 2007; George et al., 2009; Jones et al., 2009; Kielak et al., 2009; Lee and Cho, 2011). Adapter sequence was added to the primers as recommended by Roche (Table S1). Barcodes of 8 bp and AC linker were added to forward primers only. Each reaction was carried out in 50 μl reactions containing 1 × buffer, 1.8 mM of MgCl2, 0.2 μM of each primer, 200 μM of deoxynucleoside triphosphate, 300 ng/μl of bovine serum albumin, 10 ng of DNA template and 1 μl of the enzyme FastStart High Fidelity PCR System (Roche Applied Sciences, Indianapolis, IN, USA), subjected to the following conditions: 95°C for 3 min; 30 cycles of 94°C for 45 s, 57°C for 45 s and 72°C for 1 min; and 72°C for 4 min. Each soil sample was amplified in triplicate, and reaction products were pooled and purified using the Qiagen PCR purification kit (Qiagen, Valencia, CA, USA). PCR products were sequenced on a 454 GS FLX Sequencer (454 Life Sciences, Branford, CT, USA) at the Michigan State University Research Technology Support Facility. To prevent the possibility of sequencing errors (Huse et al., 2007), all reads were removed that either contained one or more ambiguous bases (N), had lengths outside the main distribution, or presented inexact matches to the primers used in the study. The high-quality bacterial 16S rRNA gene sequences are available through FigShare, http://dx.doi.org/10.6084/m9.figshare.1547935.
Sequence Analysis and Statistics
Sequences were processed using the bioinformatics platform QIIME version 1.7 (Caporaso et al., 2010). Sequences were removed from the analysis if they did not have the primer sequence, were less than 300 nt or more than 400 nt in length, contained a homopolymer run exceeding twenty nucleotides, or had ambiguous characters. The remaining sequences were assigned to samples by matching them to barcode sequences. Sequences that passed these quality filters were clustered into OTUs with a similarity cutoff of 97% using UCLUST (Edgar, 2010). Taxonomy was assigned to representative sequences from each OTU using the Ribosomal Database Project (Wang et al., 2007) web-based taxonomy assignment tool (http://rdp.cme.msu.edu/index.jsp) version 2.6 against the RDP 16S rRNA training set 9. The OTU table was filtered for specific taxonomic groups, and the relative abundance of Acidobacteria was estimated by comparing the number of sequences classified as belonging to the phylum with the number of classified bacterial sequences in each sample. Similarly, the relative abundance of Acidobacteria subgroups was estimated across all individual samples by comparing the number of sequences classified as belonging to each subgroup with the number of classified Acidobacteria sequences. Explicit relationships between the relative abundance of Acidobacteria subgroups and soil chemical properties were examined using constrained ordination generated by redundancy analysis (RDA) with the software CANOCO 4.5 (ter Braak and Šmilauer, 2002). Spearman's rank correlation coefficients were calculated between the relative abundance of Acidobacteria subgroups and soil properties using the “multtest” package (Pollard et al., 2005) in R (R Core Team, 2015). P-values were corrected for multiple testing, using the false discovery rate controlling procedure (Benjamini and Hochberg, 1995).
Distance-Decay of Similarity Analyses
The pairwise geographic distances between cores were calculated based on geographic coordinates and physical measurements. Community turnover (i.e., the distance-decay of similarity) was determined by regressing the pairwise community similarity against the pairwise logarithm of geographic distance using linear regression. Distance-decay slopes within taxonomic groups were compared between land types using the function diffslope in the software package “simba” (Jurasinski and Retzer, 2012) in R (R Core Team, 2015).
Results
Soil Chemical Properties
Overall, statistical comparison of soil chemical properties for forest and pasture soils indicated that forest conversion to pasture resulted in an increase in properties linked to soil acidity and nutrient availability in soil (Table S2). The chemical composition (Table S2) of forest and pasture soils differed significantly (ANOSIM, R = 0.680, P = 0.002). Potential acidity (H+Al) was significantly lower in forest soils compared to the pasture soils. Forest soils had significantly lower total C, N, S, and Mg contents and C/N ratios than pasture soils (Table S2).
Links between the Phylum Acidobacteria, Relative Abundances of Subgroup-Levels, and Soil Chemical Properties
The taxonomic analysis of the soil acidobacterial community was based on the retrieval of approximately 45,000 and 20,000 sequences of acidobacterial 16S rRNA gene fragments from forest soils and pasture soils, respectively (Table S3). The relative abundance of Acidobacteria sequences within an individual soil bacterial community represented on average 20% (±3.5%) in forest soil samples and 11% (±3.3%) in pasture soil samples. Overall, 15 different Acidobacteria subgroups of the current 26 subgroups (Hugenholtz et al., 1998; Zimmermann et al., 2005; Barns et al., 2007) were detected across the 54 soil samples, with Acidobacteria subgroups 1, 3, 5, and 6 accounting together for 87% of the total Acidobacteria community in forest soils and 75% in pasture soils (Table 1). A redundancy analysis of the relative abundance of Acidobacteria subgroups (1–7, 9–11, 13, 17, 18, 22, and 25) showed that the subgroups 1–3, 5, 9, 11, and 13 were significantly associated with forest soils while subgroups 4, 7, 10, 17, 18, and 25 were associated with pasture soils (Figure 1). Acidobacteria subgroup 6 was more related to pasture soils than forest soils. Statistically significant differences between forest vs. pasture soils were found for the relative abundances of the Acidobacteria subgroups 2 (P < 0.005), 4 (P < 0.05), 7 (P < 0.0005), 10 (P < 0.05), 13 (P < 0.0005), 17 (P < 0.0005) and 18 (P < 0.005) (Table 1). A correlation between the relative abundances of Acidobacteria subgroups and soil chemical properties revealed two distinct groups. Acidobacteria subgroups 1, 2, 3, and 13 were negatively correlated with total C and N content, C/N ratio, and P, S, K, Ca, and Mg content, and positively correlated with properties linked to soil acidity such as pH, Al, H+Al, and m; while subgroups 4, 5, 6, 7, 17, and 25 were positively correlated to nutrient availability and negatively correlated to properties linked to soil acidity (Table 2).
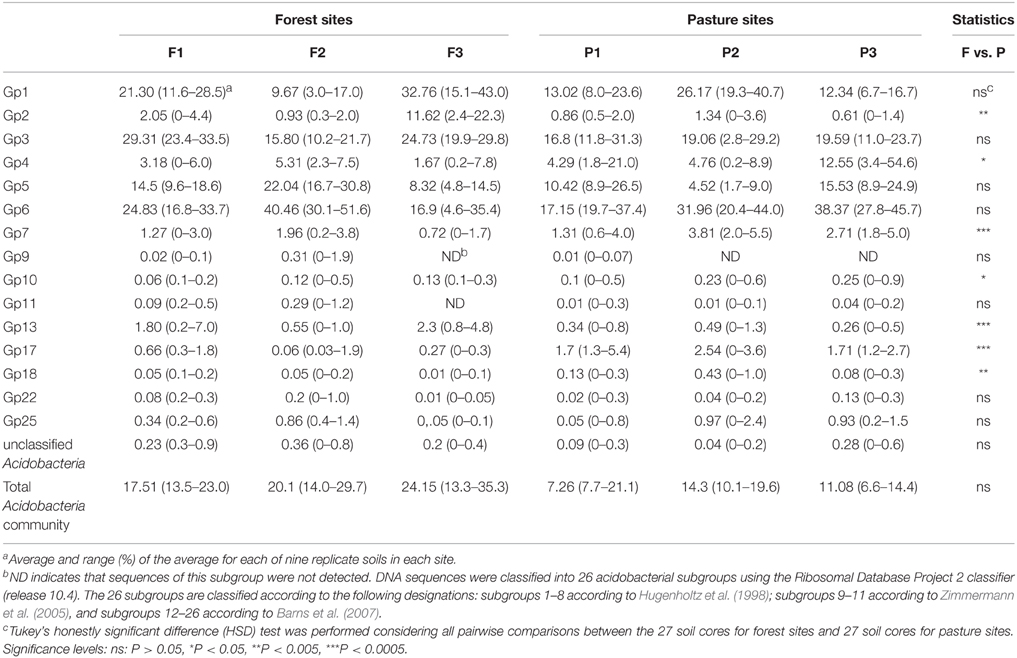
Table 1. Percentage of Acidobacteria subgroups relative to all Acidobacteria and of these to all Bacteria in forest and pasture sites.
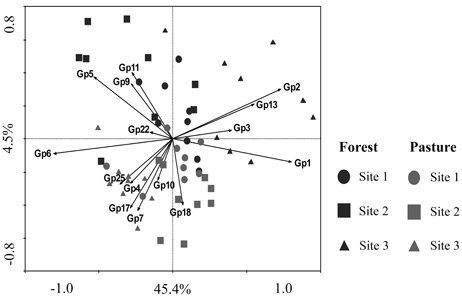
Figure 1. Constrained ordination diagram for sample plots in the first two redundancy analysis (RDA) axes based on the soil chemical characteristics of the different sampling sites and their relationship with the relative abundance of Acidobacteria subgroups (1–7, 9–11, 13, 17, 18, 22, and 25). Each vector points to the direction of increase for a given Acidobacteria subgroup (Gp) and its length indicates the strength of the correlation between this variable and the ordination scores.
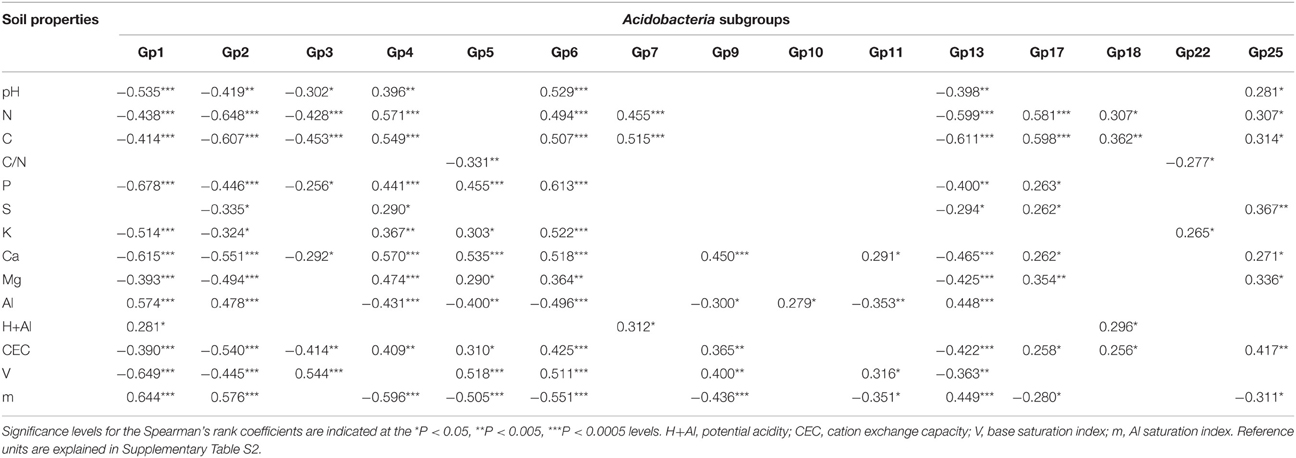
Table 2. Spearman's rank correlation coefficients and statistical significance between abundance of Acidobacteria subgroups relative to all Acidobacteria and soil properties.
Acidobacterial Distance-Decay Relationships
Taxonomic similarity of the total Acidobacteria community was significantly correlated with geographic distance in both forest and pasture sites (Table 3). The slopes of the lines fitted to these relationships differed significantly between the forest and pasture soils with a significantly steeper slope for the total forest Acidobacteria community (Figure 2).
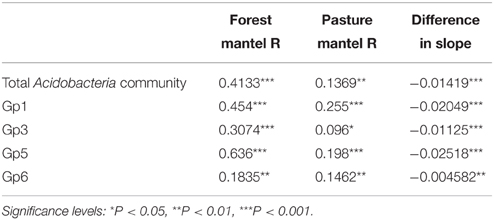
Table 3. Correlations of taxonomic similarity (Bray Curtis) and geographic distance of phylum Acidobacteria and subgroups with comparison of slope of linear model between land use types.
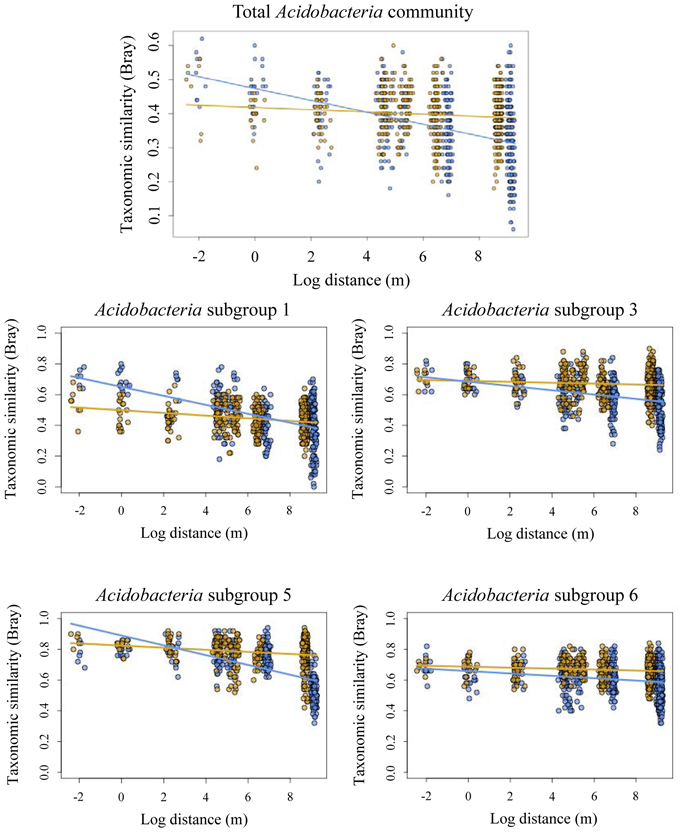
Figure 2. Decay of taxonomic similarity (Bray–Curtis) with geographic distance in forest (blue) and pasture (yellow) for total acidobacterial community and Acidobacteria subgroups 1, 3, 5, and 6.
Taxonomic similarity was significantly correlated with distance for Acidobacteria subgroups 1, 3, 5, and 6 in both the forest and pasture soils (Table 3). For each group, forest distance-decay slopes were significantly steeper than their pasture counterparts (Figure 2). The distance-decay linear model showed a better fit to community similarity over distance for forest Acidobacteria communities than for those from pasture. Similar biogeographic patterns were revealed for the total Acidobacteria community and total bacterial community when comparing slopes across all forest and pasture soils (Figure S2).
Discussion
The present study reports differential relative abundances for Acidobacteria at phylum and subgroup-levels in forest soils and in soils converted into pasture in the western Brazilian Amazon. These differences in abundances are correlated with soil acidity and nutrient availability. Total Acidobacteria community as well as the most abundant subgroups, namely 1, 3, 5, and 6, showed a divergence in spatial patterning between forest and pasture, with the pasture communities showing less spatial turnover than the forest communities.
Pasture establishment on acidic soils in the Amazon region is preceded by cutting and removing the economically important trees and burning the remaining above ground biomass (Fujisaka et al., 1996). As a result of these conversion and management practices, the thick organic layer of the forest is lost, the soil nutrient input is changed, and the topsoil is fertilized with alkaline ashes, thus increasing the soil pH (Juo and Manu, 1996; Giardina et al., 2000; Makeschin et al., 2008). Neye and Greenland (1960) proposed the “nutrient-rich ash” hypothesis to explain the observed short-term increase in soil nutrient availability after slash-and-burn clearing of forest. Although the slash-and-burn method of deforestation was applied 28 years before the soil sampling in our pasture sites, numerous studies of forest-to-pasture conversion in the Amazon reported increases in C and N stocks after several years of pasture establishment (Feigl et al., 1995; Neill et al., 1995, 1996; Cerri et al., 2004). Increases in C and N contents and nutrient availability in pasture soils can be also associated with a more decomposable litter (Rhoades et al., 2000; Potthast et al., 2010) and a dense fine-root system (Rhoades and Coleman, 1999) of the pasture grasses.
The chemical characteristics found in pasture soils can be a selective pressure for soil bacteria that prefer nutrient-rich habitats. Cultivation-dependent and -independent approaches have revealed adaptations of members of the phylum Acidobacteria to low substrate concentrations in soil, and their negative responses to increases in carbon and pH (Noll et al., 2005; Eichorst et al., 2007; Fierer et al., 2007; Ward et al., 2009). However, certain subgroups of the Acidobacteria are also known to have a preference for soil environments with increased available nutrients, i.e., copiotrophic environments (Navarrete et al., 2013). Despite the higher abundance of most Acidobacteria subgroups in forest soils, which may help to explain the strong decrease in the proportion of the total Acidobacteria community after forest-to-pasture conversion (Rodrigues et al., 2013), subgroups 7, 17, and 18 were significantly more abundant in pasture soils compared to the forest soils, with their abundances linked to high nutrient availability. Acidobacteria subgroup 7 showed similar response in soils from the Southeastern Brazilian Amazon converted into agricultural fields, with their abundances linked to high contents of nutrient in soil (Navarrete et al., 2013). Naether et al. (2012) also found higher relative abundances for members of Acidobacteria subgroups 17 in pasture soils in comparison to forest soils from three geographical regions in Germany. The selective advantage that allows microorganisms to respond rapidly in environments characterized by fluctuations in resource availability may be conferred by the number of rRNA gene copies in their genomes (Klappenbach et al., 2000; Stevenson and Schmidt, 2004). Genomes of Acidobacteria subgroups 1 and 3 were typified by a low number of rRNA gene copies (Ward et al., 2009). Although the number of rRNA gene copies is unknown for most of the Acidobacteria subgroups, the few number of ribossomal operons in acidobacterial genomes (Ward et al., 2009) is consistent with the higher abundance of this phylum in forest soils and has been postulated to be a characteristic marker of slow growth and a K-selected lifestyle (Klappenbach et al., 2000; Stevenson and Schmidt, 2004). Taken together, these findings suggest that different Acidobacteria subgroups have different life history patterns, with some preferring high nutrient concentrations and others preferring more oligotrophic environments.
The Acidobacteria subgroups 4 and 10 were also predominant in pasture soils and positively linked to soil pH. Previously, the abundance of the Acidobacteria subgroup 4 has been linked to increases in soil pH (Jones et al., 2009; Lauber et al., 2009). In addition, Blastocatella fastidiosa, the only known isolate from Acidobacteria subgroup 4, recovered from a savanna soil with a moderate acidic pH (i.e., close to 6.0) in Namibia, grows at even higher pHs (up to 10.0) (Foesel et al., 2013). Although soil pH has been demonstrated to explain a significant degree of microbial community variation in different spatial scales (Lauber et al., 2009), few studies have characterized the specific effects of pH on rare Acidobacteria subgroups in soil.
A large fraction of the total Acidobacteria community was composed of members of subgroup 1 in both forest and pasture soils. Sait et al. (2006) identified moderately acidic pH values as an important factor driving the abundance of members of this Acidobacteria subgroup in different soils, with Acidobacteria subgroup 1 increasing in relative abundance as the soil pH decreases. Li et al. (2014) showed significant negative correlations between Acidobacteria subgroup 1 and pH, and a positive correlation with C/N ratio. Rawat et al. (2013, 2014) and Ward et al. (2009) reported that members of Acidobacteria subgroup 1 are versatile heterotrophs that hydrolyze a suite of sugars and complex polysaccharides, contributing to carbon availability in certain ecosystems, including oligotrophic environments. This consideration was based on genomic data from Granulicella mallensis MP5ACTX8T and Granulicella tundricola type strain MP5ACTX9T, members of Acidobacteria subgroup 1 from tundra soil, and two acidobacterial subgroup 1 strains (Acidobacterium capsulatum), isolated from sediments in acidic drainage from the Yanahara pyrite mine in Japan. Isolation, cultivation and genome analysis of Acidobacteria subgroup 1 community members has revealed sugars as their preferred growth substrates (Männistö et al., 2011), and metabolic versatility with genes involved in metabolism and transport of carbohydrates, utilization and biosynthesis of diverse structural and storage polysaccharides such as plant based carbon polymers (Rawat et al., 2014).
The spatial turnover of a community (i.e., the rate of the distance-decay relationship) has been used as a proxy to estimate biotic homogenization at the landscape scale (Olden and Poff, 2003; Rodrigues et al., 2013). Through our approach we were able to detect changes to the spatial patterning of the Acidobacteria community as well as the most abundant Acidobacteria subgroups. In all cases, the directionality of change was the same; forest communities showed a steeper distance-decay relationship relative to pasture communities and that pasture communities were more similar to each other at larger distances than forest communities. We take these patterns to be suggestive of biotic homogenization. Changes to distance-decay patterns could result from alterations to several community assembly processes. For example, forest soils may have a more diverse or spatially variable array of microbial niches that may get broken down through the change in aboveground plant communities or alterations to the soil environment associated with land use change. It has been shown that Acidobacteria are one of the most abundant members of the phyllosphere of tropical trees, and that the distribution of Acidobacteria follows host plant phylogeny (Kim et al., 2012). Hence the removal and subsequent replacement of the tree community by low diversity grassland could be a strong driver in the changes to Acidobacteria biogeography.
These differential responses in relative abundance and biogeographic patterning of the Acidobacteria phylum and its subgroups to forest conversion into pastures in the Amazon rainforest expand the known possibilities to explore these subgroups to define management-indicators of agricultural practices. When conditions related to specific soil properties change owing to soil management practices, the proportion of different subgroups may be used to as an indicator of the soil status (Holt and Miller, 2011; Kuramae et al., 2011).
In conclusion, this study expands the understanding of ecological characteristics of Acidobacteria subgroups in Amazon soils by reporting differential responses of Acidobacteria and their subgroups to forest-to-pasture conversion and the associated biogeographic patterns in a western Brazilian Amazon area. The forest clear-cutting and burning in the Amazon primarily to yield cattle pastures play a role in the assembly of the Acidobacteria communities in soil, especially in Acidobacteria subgroups 1, 3, 4, 5, and 6. Preponderant responses of Acidobacteria subgroups to forest-to-pasture conversion effects in soils are at least in part through effects on soil acidity and nutrient availability. The results also showed more similar composition of the total Acidobacteria community as well as the most abundant Acidobacteria subgroups across space in pasture soils than in forest soils. Taken together, these findings could assist to define management-indicators to judge the impacts from the forest-to-pasture conversion on soil ecosystem in the Amazon Basin.
Author Contributions
AN and JR designed research; AN, AV, KM, AK, JT, KN, BB, ST, and JR performed research; JT, BB, ST, KN, and JR contributed new reagents/analytic tools; AN, AV, KM, AK, and JR analyzed data; and AN, KM, AK, BB, KN, and JR wrote the paper.
Conflict of Interest Statement
The authors declare that the research was conducted in the absence of any commercial or financial relationships that could be construed as a potential conflict of interest.
Acknowledgments
This project was supported by the National Science Foundation—Dimensions of Biodiversity 2014 (DEB—14422214) and NSF-FAPESP (2014/50320-4), Agriculture and Food Research Initiative Competitive Grant 2009-35319-05186 from the US Department of Agriculture National Institute of Food and Agriculture, Conselho Nacional de Desenvolvimento Científico (CNPq—152084/2011-8, 485801/2011-6), and Fundação de Amparo à Pesquisa do Estado de São Paulo (FAPESP—2008/58114-3, 2011/517449-6, 2012/13321-7).
Supplementary Material
The Supplementary Material for this article can be found online at: http://journal.frontiersin.org/article/10.3389/fmicb.2015.01443
References
Barns, S. M., Cain, E. C., Sommerville, L., and Kuske, C. R. (2007). Acidobacteria phylum sequences in uranium-contaminated subsurface sediments greatly expand the known diversity within the phylum. Appl. Environ. Microbiol. 73, 3113–3116. doi: 10.1128/AEM.02012-06
Barns, S. M., Takala, S. L., and Kuske, C. R. (1999). Wide distribution and diversity of members of the bacterial kingdom Acidobacterium in the environment. Appl. Environ. Microbiol. 65, 1731–1737.
Bastos, T. X., and Diniz, T. D. (1982). Avaliação do Clima do Estado de Rondônia Para Desenvolvimento Agrícola. Belém: Embrapa-CPATU.
Benjamini, Y., and Hochberg, Y. (1995). Controling the false discovery rate - a practical and powerful approach to multiple testing. J. R. Stat. Soc. B Stat. Methodol. 57, 289–300.
ter Braak, C. J. F., and Šmilauer, P. (2002). CANOCO Reference Manual and CanoDraw for Windows User's Guide: Software for Canonical Community Ordination (Version 4.5). New York, NY: Microcomputer Power.
Caporaso, J. G., Kuczynski, J., Stombaugh, J., Bittinger, K., Bushman, F. D., Costello, E. K., et al. (2010). QIIME allows analysis of high-throughput community sequencing data. Nat. Methods 7, 335–336. doi: 10.1038/nmeth.f.303
Catão, E. C. P., Lopes, F. A. C., Araújo, J. F., Castro, A. P., Barreto, C. C., Bustamante, M. M. C., et al. (2014). Soil acidobacterial 16S rRNA gene sequences reveal subgroup level differences between savanna-like Cerrado and Atlantic forest brazilian biomes. Int. J. Microbiol. 2014:156341. doi: 10.1155/2014/156341
Cenciani, K., Lambais, M. R., Cerri, C. C., De Azevedo, L. C. B., and Feigl, B. J. (2009). Bacteria diversity and microbial biomass in forest, pasture and fallow soils in the southwestern Amazon Basin. Rev. Bras. Cienc. Solo 33, 907–916. doi: 10.1590/S0100-06832009000400015
Cerri, C. E. P., Paustian, K., Bernoux, M., Victoria, R. L., Melillos, J. M., and Cerri, C. C. (2004). Modeling changes in soil organic matter in Amazon forest to pasture conversion with the Century model. Glob. Change Biol. 10, 815–832. doi: 10.1111/j.1365-2486.2004.00759.x
Edgar, R. C. (2010). Search and clustering orders of magnitude faster than BLAST. Bioinformatics 26, 2460–2461. doi: 10.1093/bioinformatics/btq461
Eichorst, S. A., Breznak, J. A., and Schmidt, T. M. (2007). Isolation and characterization of soil bacteria that define Terriglobus gen. nov., in the phylum Acidobacteria. Appl. Environ. Microb. 73, 2708–2717. doi: 10.1128/AEM.02140-06
Feigl, B. J., Melillo, J. M., and Cerri, C. C. (1995). Changes in the origin and the quality of soil organic matter after pasture introduction in Rondônia (Brazil). Plant Soil 175, 21–29. doi: 10.1007/BF02413007
Fierer, N., Bradford, M. A., and Jackson, R. B. (2007). Toward an ecological classification of soil bacteria. Ecology 88, 135–1364. doi: 10.1890/05-1839
Foesel, B. U., Rohde, M., and Overmann, J. (2013). Blastocatella fastidiosa gen. nov., sp. nov., isolated from semiarid savanna soil - the first described species of Acidobacteria subdivision 4. Syst. Appl. Microbiol. 36, 82–89. doi: 10.1016/j.syapm.2012.11.002
Fujisaka, S., Bell, W., Thomas, N., Hurtad, L., and Crawford, E. (1996). Slash-and-burn agriculture, conversion to pasture, and deforestation in two Brazilian Amazon colonies. Agr. Ecosyst. Environ. 59, 115–130. doi: 10.1016/0167-8809(96)01015-8
George, I. F., Liles, M. R., Hartmann, M., Ludwig, W., Goodman, R. M., and Agathos, S. N. (2009). Changes in soil Acidobacteria communities after 2,4,6-trinitrotoluene contamination. FEMS Microbiol. Lett. 296, 159–166. doi: 10.1111/j.1574-6968.2009.01632.x
Giardina, C. P., Sanford, R. L., Døckersmith, I. C., and Jaramillo, V. J. (2000). The effects of slash burning on ecosystem nutrients during the land preparation phase of shifting cultivation. Plant Soil 220, 247–260. doi: 10.1023/A:1004741125636
Gibson, L., Lee, T. M., Koh, L. P., Brook, B. W., Gardner, T. A., Barlow, J., et al. (2011). Primary forests are irreplaceable for sustaining tropical biodiversity. Nature 478, 378–381. doi: 10.1038/nature10425
Greening, C., Carere, C. R., Rushton-Green, R., Harold, L. K., Hards, K., Taylor, M. C., et al. (2015). Persistence of the dominant soil phylum Acidobacteria by trace gas scavenging. Proc. Natl. Acad. Sci. U.S.A. 112, 10497–10502. doi: 10.1073/pnas.1508385112
Holt, E. A., and Miller, S. W. (2011). Bioindicators: using organisms to measure environmental impacts. Nat. Educ. Knowl. 3, 8.
Hugenholtz, P., Goebel, B. M., and Pace, N. R. (1998). Impact of culture-independent studies on the emerging phylogenetic view of bacterial diversity. J. Bacteriol. 180, 4765–4774.
Huse, S. M., Huber, J. A., Morrison, H. G., Sogin, M. L., and Welch, D. M. (2007). Accuracy and quality of massively parallel DNA pyrosequencing. Genome Biol. 8, R143. doi: 10.1186/gb-2007-8-7-r143
Jesus, E. C. D., Marsh, T. L., Tiedje, J. M., and Moreira, F. M. S. (2009). Changes in land use alter the structure of bacterial communities in Western Amazon soils. ISME J. 3, 1004–1011. doi: 10.1038/ismej.2009.47
Jones, R. T., Robeson, M. S., Lauber, C. L., Hamady, M., Knight, R., and Fierer, N. (2009). A comprehensive survey of soil acidobacterial diversity using pyrosequencing and clone library analyses. ISME J. 3, 442–453. doi: 10.1038/ismej.2008.127
Juo, A. S. R., and Manu, A. (1996). Chemical dynamics in slash-and-burn agriculture. Agric. Ecosyst. Environ. 58, 49–60. doi: 10.1016/0167-8809(95)00656-7
Jurasinski, G., and Retzer, V. (2012). Simba: A Collection of Functions for Similarity Analysis of Vegetation Data. R package Version 0.3-5. Available online at: http://CRAN.R-project.org/package=simba
Kielak, A., Pijl, A. S., van Veen, J. A., and Kowalchuk, G. A. (2009). Phylogenetic diversity of Acidobacteria in a former agricultural soil. ISME J. 3, 378–382. doi: 10.1038/ismej.2008.113
Kim, J. S., Sparovek, G., Longo, R. M., De Melo, W. J., and Crowley, D. (2007). Bacteria diversity of terra preta and pristine forest soil from the western Amazon. Soil Biol. Biochem. 39, 684–690. doi: 10.1016/j.soilbio.2006.08.010
Kim, M., Singh, D., Lai-Hoe, A., Go, R., Abdul Rahim, R., Ainuddin, A. N., et al. (2012). Distinctive phyllosphere bacterial communities in tropical trees. Microb. Ecol. 63, 674–681. doi: 10.1007/s00248-011-9953-1
Klappenbach, J. A., Dunbar, J. M., and Schmidt, T. M. (2000). rRNA operon copy number reflects ecological strategies of Bacteria. Appl. Environ. Microbiol. 66, 1328–1333. doi: 10.1128/AEM.66.4.1328-1333.2000
Kuramae, E. E., Yergeau, E., Wong, L. C., Pijl, A. S., van Veen, J. A., and Kowalchuk, G. A. (2011). Soil characteristics more strongly influence soil bacterial communities than land-use type. FEMS Microbiol. Ecol. 79, 12–24. doi: 10.1111/j.1574-6941.2011.01192.x
Lauber, C. L., Hamady, M., Knight, R., and Fierer, N. (2009). Pyrosequencing-based assessment of soil pH as a predictor of soil bacterial community composition at the continental scale. Appl. Environ. Microbiol. 75, 5111–5120. doi: 10.1128/AEM.00335-09
Lauber, C. L., Strickland, M. S., Bradford, M. A., and Fierer, N. (2008). The influence of soil properties on the structure of bacterial and fungal communities across land-use types. Soil Biol. Biochem. 40, 2407–2415. doi: 10.1016/j.soilbio.2008.05.021
Lee, S.-H., and Cho, J.-C. (2011). Group-specific PCR primers for the phylum Acidobacteria designed based on the comparative analysis of 16S rRNA gene sequences. J. Microbiol. Methods 86, 195–203. doi: 10.1016/j.mimet.2011.05.003
Li, H., Ye, D., Wang, X., Settles, M. L., Wang, J., Hao, Z., et al. (2014). Soil bacterial communities of different natural forest types in Northeast China. Plant Soil 383, 203–216. doi: 10.1007/s11104-014-2165-y
Makeschin, F., Haubrich, F., Abiy, M., Burneo, J. I., and Klinger, T. (2008). “Pasture management and natural soil regeneration,” in Gradients in a Tropical Mountain Ecosystem of Ecuador - Ecological Studies, eds E. Beck, J. Bendix, I. Kottke, F. Makeschin, and R. Mosandl (Berlin: Springer), 397–408. doi: 10.1007/978-3-540-73526-7_38
Männistö, M. K., Rawat, S., Starovoytov, V., and Häggblom, M. M. (2011). Terriglobus saanensis sp. nov., a novel Acidobacterium isolated from tundra soil of Northern Finland. Int. J. Syst. Evol. Microbiol. 61, 1823–1828. doi: 10.1099/ijs.0.026005-0
Mirza, B. S., Potisap, C., Nüsslein, K., Bohannan, B. J. M., and Rodrigues, J. L. M. (2014). Response of free-living nitrogen-fixing microorganisms to land use change in the Amazon rainforest. Appl. Environ. Microbiol. 80, 281–288. doi: 10.1128/AEM.02362-13
Mueller, R. C., Paula, F. S., Mirza, B. S., Rodrigues, J. L. M., Nüsslein, K., and Bohannan, B. J. M. (2014). Links between plant and fungal communities across a deforestation chronosequence in the Amazon rainforest. ISME J. 8, 1548–1550. doi: 10.1038/ismej.2013.253
Naether, A., Foesel, B. U., Naegele, V., Wüst, P. K., Weinert, J., Bonkowski, M., et al. (2012). Environmental factors affect acidobacterial communities below the subgroup level in grassland and forest soils. Appl. Environ. Microbiol. 78, 7398–7406. doi: 10.1128/AEM.01325-12
Navarrete, A. A., Cannavan, F. S., Taketani, R. G., and Tsai, S. M. (2010). A molecular survey of the diversity of microbial communities in different Amazonian agricultural model systems. Diversity 2, 787–809. doi: 10.3390/d2050787
Navarrete, A. A., Kuramae, E. E., de Hollander, M., Pijl, A. S., van Veen, J. A., and Tsai, S. M. (2013). Acidobacterial community responses to agricultural management of soybean in Amazon forest soils. FEMS Microbiol. Ecol. 83, 607–621. doi: 10.1111/1574-6941.12018
Navarrete, A. A., Taketani, R. G., Mendes, L. W., Cannavan, F. S., Moreira, F. M. S., and Tsai, S. M. (2011). Land-use systems affect archaeal community structure and functional diversity in western Amazon soils. Rev. Bras. de Cienc. Solo 35, 1527–1540. doi: 10.1590/s0100-06832011000500007
Navarrete, A. A., Tsai, S. M., Mendes, L. W., Faust, K., de Hollander, M., Cassman, N., et al. (2015). Soil microbiome responses to the short-term effects of Amazonian deforestation. Mol. Ecol. 24, 2433–2448. doi: 10.1111/mec.13172
Neill, C., Fry, B., Melillo, J. M., Steudler, P. A., Moraes, J. F. L., and Cerri, C. C. (1996). Forest and pasture derived carbon contributions to carbon stocks and microbial respiration of tropical pasture soils. Oecologia 107, 113–119. doi: 10.1007/BF00582241
Neill, C., Piccolo, M. C., Steudler, P. A., Melillo, J. M., Feigl, B. J., and Cerri, C. C. (1995). Nitrogen dynamics in soils of forests and active pastures in the western Brazilian Amazon basin. Soil Biol. Biochem. 27, 1167–1175. doi: 10.1016/0038-0717(95)00036-E
Neye, P., and Greenland, D. (1960). The soil under shifting cultivation. Tech. Commun. Common Wealth Agric. Bur. 51, 156.
Noll, M., Matthies, D., Frenzel, P., Derakshani, M., and Liesack, W. (2005). Succession of bacterial community structure and diversity in a paddy soil oxygen gradient. Environ. Microbiol. 7, 382–395. doi: 10.1111/j.1462-2920.2005.00700.x
Olden, J. D., and Poff, N. L. (2003). Toward a mechanistic understanding and prediction of biotic homogenization. Am. Nat. 162, 442–460. doi: 10.1086/378212
Paula, F. S., Rodrigues, J. L. M., Zhou, J., Wu, L., Mueller, R. C., Mirza, B. S., et al. (2014). Land use change alters functional gene diversity, composition and abundance in Amazon forest soil microbial communities. Mol. Ecol. 23, 2988–2999. doi: 10.1111/mec.12786
Pollard, K. S., Dudoit, S., and van der Laan, M. J. (2005). “Multiple testing procedures: R multtest package and applications to genomics” in Bioinformatics and computational biology solutions using R and bioconductor, eds R. Gentleman, V. Carey, S. Dudoit, R. Irizarry, and W. Huber (New York, NY: Springer), 251–272.
Potthast, K., Hamer, U., and Makeschin, F. (2010). Impact of litter quality on mineralization processes in managed and abandoned pasture soils in Southern Ecuador. Soil Biol. Biochem. 42, 56–64. doi: 10.1016/j.soilbio.2009.09.025
Ranjan, K., Paula, F. S., Mueller, R. C., Jesus, E. C., Cenciani, K., Bohannan, B. J., et al. (2015). Forest-to-pasture conversion increases the diversity of the phylum Verrucomicrobia in Amazon rainforest soils. Front. Microbiol. 6:779. doi: 10.3389/fmicb.2015.00779
Rawat, S. R., Männistö, M. K., Bromberg, Y., and Haggblom, M. M. (2012). Comparative genomic and physiological analysis provides insights into the role of Acidobacteria in organic carbon utilization in Arctic tundra soils. FEMS Microbiol. Ecol. 82, 341–355. doi: 10.1111/j.1574-6941.2012.01381.x
Rawat, S. R., Männistö, M. K., Starovoytov, V., Goodwin, L., Nolan, M., Hauser, L., et al. (2014). Complete genome sequence of Granulicella tundricola type strain MP5ACTX9T, an Acidobacteria from tundra soil. Stan. Genomic Sci. 9, 449–461. doi: 10.4056/sigs.4648353
Rawat, S. R., Männistö, M. K., Starovoytov, V., Goodwin, L., Nolan, M., Hauser, L. J., et al. (2013). Complete genome sequence of Granulicella mallensis type strain MP5ACTX8T, an acidobacterium from tundra soil. Stand. Genomic Sci. 9, 71–82. doi: 10.4056/sigs.4328031
R Core Team (2015). R: A Language and Environment for Statistical Computing. R Foundation for Statistical Computing. Vienna. Available online at: http://www.R-project.org/
Rhoades, C. C., and Coleman, D. C. (1999). Nitrogen mineralization and nitrification following land conversion in montane Ecuador. Soil Biol. Biochem. 31, 1347–1354. doi: 10.1016/S0038-0717(99)00037-1
Rhoades, C. C., Eckert, G. E., and Coleman, D. C. (2000). Soil carbon differences among forest, agriculture, and secondary vegetation in lower montane Ecuador. Ecol. Appl. 10, 497–505. doi: 10.1890/1051-0761(2000)010[0497:SCDAFA]2.0.CO;2
Rodrigues, J. L. M., Pellizari, V. H., Mueller, R., Baek, K., Jesus, E. C., Paula, F. S., et al. (2013). Conversion of the Amazon rainforest to agriculture results in biotic homogenization of soil bacterial communities. Proc. Natl. Acad. Sci. U.S.A. 110, 988–993. doi: 10.1073/pnas.1220608110
Rousk, J., Bååth, E., Brookes, P. C., Lauber, C. L., Lozupone, C., Caporaso, J. G., et al. (2010). Soil bacterial and fungal communities across a pH gradient in an arable soil. ISME J. 4, 1340–1351. doi: 10.1038/ismej.2010.58
Sait, M., Davis, K. E., and Janssen, P. H. (2006). Effect of pH on isolation and distribution of members of subdivision 1 of the phylum Acidobacteria occurring in soil. Appl. Environ. Microbiol. 72, 1852–1857. doi: 10.1128/AEM.72.3.1852-1857.2006
Sala, O. E., Chapin, F. S., Armesto, J. J., Berlow, E., Bloomfield, J., Dirzo, R., et al. (2000). Biodiversity - global biodiversity scenarios for the year 2100. Science 287, 1770–1774. doi: 10.1126/science.287.5459.1770
Stevenson, B. S., and Schmidt, T. M. (2004). Life history implications of rRNA gene copy number in Escherichia coli. Appl. Environ. Microbiol. 70, 6670–6677. doi: 10.1128/AEM.70.11.6670-6677.2004
Taketani, R. G., and Tsai, S. M. (2010). The influence of different land uses on the structure of archaeal communities in Amazonian anthrosols based on 16S rRNA and amoA genes. Microb. Ecol. 59, 734–743. doi: 10.1007/s00248-010-9638-1
Wang, Q., Garrity, G. M., Tiedje, J. M., and Cole, J. R. (2007). Naive Bayesian classifier for rapid assignment of rRNA sequences into the new bacterial taxonomy. Appl. Environ. Microbiol. 73, 5261–5267. doi: 10.1128/AEM.00062-07
Ward, N. L., Challacombe, J. F., Janssen, P. H., Henrissat, B., Coutinho, P. M., Wu, M., et al. (2009). Three genomes from the phylum Acidobacteria provide insight into the lifestyles of these microorganisms in soils. Appl. Environ. Microb. 75, 2046–2056. doi: 10.1128/AEM.02294-08
Wearn, O. R., Reuman, D. C., and Ewers, R. M. (2012). Extinction debt and windows of conservation opportunity in the Brazilian Amazon. Science 337, 228–232. doi: 10.1126/science.1219013
Zhao, J., Ni, T., Li, Y., Xiong, W., Ran, W., Shen, B., et al. (2014). Responses of bacterial communities in arable soils in a rice-wheat cropping system to different fertilizer regimes and sampling times. PLoS ONE 9:e85301. doi: 10.1371/journal.pone.0085301
Keywords: tropical rainforest, land-use change, spatial scale, 16S rRNA gene, community similarity, Acidobacteria
Citation: Navarrete AA, Venturini AM, Meyer KM, Klein AM, Tiedje JM, Bohannan BJM, Nüsslein K, Tsai SM and Rodrigues JLM (2015) Differential Response of Acidobacteria Subgroups to Forest-to-Pasture Conversion and Their Biogeographic Patterns in the Western Brazilian Amazon. Front. Microbiol. 6:1443. doi: 10.3389/fmicb.2015.01443
Received: 18 September 2015; Accepted: 03 December 2015;
Published: 22 December 2015.
Edited by:
Svetlana N. Dedysh, Russian Academy of Sciences, RussiaReviewed by:
Stephanie A. Eichorst, University of Vienna, AustriaBärbel Ulrike Fösel, Leibniz-Institut DSMZ-Deutsche Sammlung von Mikroorganismen und Zellkulturen GmbH, Germany
Copyright © 2015 Navarrete, Venturini, Meyer, Klein, Tiedje, Bohannan, Nüsslein, Tsai and Rodrigues. This is an open-access article distributed under the terms of the Creative Commons Attribution License (CC BY). The use, distribution or reproduction in other forums is permitted, provided the original author(s) or licensor are credited and that the original publication in this journal is cited, in accordance with accepted academic practice. No use, distribution or reproduction is permitted which does not comply with these terms.
*Correspondence: Jorge L. M. Rodrigues, am1yb2RyaWd1ZXNAdWNkYXZpcy5lZHU=