Exploring the avian gut microbiota: current trends and future directions
- Centre for Microbial Innovation, School of Biological Sciences, Faculty of Science, The University of Auckland, Auckland, New Zealand
Birds represent a diverse and evolutionarily successful lineage, occupying a wide range of niches throughout the world. Like all vertebrates, avians harbor diverse communities of microorganisms within their guts, which collectively fulfill important roles in providing the host with nutrition and protection from pathogens. Although many studies have investigated the role of particular microbes in the guts of avian species, there has been no attempt to unify the results of previous, sequence-based studies to examine the factors that shape the avian gut microbiota as a whole. In this study, we present the first meta-analysis of the avian gut microbiota, using 16S rRNA gene sequences obtained from a range of publicly available clone-library and amplicon pyrosequencing data. We investigate community membership and structure, as well as probe the roles of some of the key biological factors that influence the gut microbiota of other vertebrates, such as host phylogeny, location within the gut, diet, and association with humans. Our results indicate that, across avian studies, the microbiota demonstrates a similar phylum-level composition to that of mammals. Host bird species is the most important factor in determining community composition, although sampling site, diet, and captivity status also contribute. These analyses provide a first integrated look at the composition of the avian microbiota, and serve as a foundation for future studies in this area.
Introduction
The role of the gut microbiota in shaping the health and physiology of vertebrate hosts is a well-established, highly exciting area in microbiology. The diversity and function of microbes in the gastrointestinal (GI) tract is an area of ongoing research, with recognized roles for the vertebrate microbiota in nutrition (Jin et al., 1998; Preest et al., 2003; Turnbaugh et al., 2006; Angelakis and Raoult, 2010; Stanley et al., 2012), gut development (Stappenbeck et al., 2002; Rahimi et al., 2009; Zhang et al., 2011; Cao et al., 2012) and regulation of host physiology (Bäckhed et al., 2004; Björkholm et al., 2009; Meinl et al., 2009). 16S rRNA gene sequencing has been employed in a range of studies to assess the diversity and phylogenetic relationships of gut microbes and this has proven to be a powerful tool for understanding the factors that shape microbial communities, due to both its informative and predictive potential. A secondary benefit of the 16S rRNA gene is that, in addition to reporting the results of findings in scientific journals, it is customary to deposit the primary sequence data into publicly available databases which allow for a second wave of meta-study. By aggregating data from a variety of sources or environments, researchers have been able to discern large-scale patterns in microbial ecology, analysing the bacterial communities of mammalian (Ley et al., 2008a) and fish (Sullam et al., 2012) guts, as well as across other non-biological factors (Lozupone and Knight, 2007; Chu et al., 2010; Shade et al., 2013). One area that has arguably not undergone such a revolution is that of the avian microbiota. While several notable exceptions exist, such as commercially farmed broiler chickens and turkeys as well as the South American hoatzin, the majority of avian systems have not been studied outside of immediate pathogenic concerns.
Similar to other vertebrates, the GI tract of birds is colonized by a community of microbes, with a density as high as 1011 c.f.u/g in the hindgut (Barnes, 1972). The role of microbes in the avian gut has long been a topic of study, with ground-breaking research throughout the 1960's identifying the role of bacteria in starch degradation and volatile fatty-acid production within the bird gut (Bolton, 1965; Annison et al., 1968; Pritchard, 1972). From a microbiological perspective, there are two major areas of interest in the bird gut. The crop, a muscular pouch located at the start of the alimentary tract, is associated with the breakdown of starch (Shaw, 1913; Pritchard, 1972; Vispo and Karasov, 1997; Pacheco et al., 2004), and microbially mediated fermentation of lactate (Bolton, 1962, 1965; Pritchard, 1972; Moore et al., 2004). Cellulolytic microbes have occasionally been observed in avian crops (Shetty et al., 1990; Domínguez-Bello et al., 1993), but significant bacterial cellulolysis has only been reported in the hoatzin (Grajal et al., 1989; Domínguez-Bello et al., 1993), with only low levels of cellulose fermentation reported for other birds (Clemens et al., 1975; Cutler et al., 2005). The ceca are the sites of recycling of urea (Barnes, 1972; Mead, 1989; Vispo and Karasov, 1997; Preest et al., 2003), retention of water (McNab, 1973) and fermentation of carbohydrates (Józefiak et al., 2004). It has been observed that a cellulose-rich diet leads to increased size of the ceca (Leopold, 1953; McNab, 1973; Miller, 1976; Duke et al., 1984; Redig, 1989; Stevens and Hume, 1998), but there is contradictory evidence for the direct utilization of cellulose in the avian hindgut (Barnes, 1972; McNab, 1973; Mead, 1989).
With the rise of 16S rRNA gene sequencing a large portion of avian microbiology has shifted from microbial physiology to the diversity and phylogeny of avian gut microbes. Specific studies have addressed areas of avian microbial ecology, such as the variation in microbial diversity along the GI tract (Bjerrum et al., 2006; Gong et al., 2007; Torok et al., 2008; Waite et al., 2012), the influence of diet (Rubio et al., 1998; Blanco et al., 2006; Torok et al., 2008; Janczyk et al., 2009; Hammons et al., 2010), age (Van Der Wielen et al., 2002; Godoy-Vitorino et al., 2010; Van Dongen et al., 2013) or other host-specific factors (Zhu et al., 2002; Lucas and Heeb, 2005; Banks et al., 2009; Benskin et al., 2010; Wienemann et al., 2011). While there is extensive evidence that microbial colonization of the GI tract brings benefits to the host bird (Jin et al., 1998; Torok et al., 2008; Angelakis and Raoult, 2010; Torok et al., 2011; Zhang et al., 2011; Cao et al., 2012; Stanley et al., 2012), there are also pathways through which the normal colonization of microbes can be of detriment to the host (Ford and Coates, 1971; Potti et al., 2002; Cao et al., 2012; Singh et al., 2013). Although there are many published studies exploring aspects of the avian microbiota, it has evidently been uncommon for authors to publish their sequence data to an archive, somewhat limiting the potential for avian metastudies. As an example of this, in their 2008 meta-analysis of the vertebrate microbiota Ley et al. had access to rich clone-library data from insects (19 studies), humans (20 studies) and other vertebrate species (23 studies, including five from birds) (Ley et al., 2008b). In 2012, Sullam et al. identified for analysis 24 pre-existing clone-libraries derived from fish guts (Sullam et al., 2012). By contrast, in the same year Kohl only identified eight avian libraries with any significant microbiota data (Kohl, 2012). A survey of the recent literature has shown that the picture of the avian microbiota has since improved significantly, with the continued usage of clone-libraries and incorporation of amplicon pyrosequencing into existing study systems (Table 1).
In order to gain new insights into the avian gut microbiota, we sought to amalgamate the existing knowledge and determine whether patterns detected in individual studies were consistent across avians as a whole. To achieve this goal we collected publicly available data from NCBI GenBank and MG-RAST and reanalyzed the data using established bioinformatics pipelines.
Methods
Data Acquisition and Quality Control
Clone-library data were obtained from GenBank through a comprehensive literature survey, followed by the retrieval of clone-library sequence data of interest. Short amplicon data from next-generation sequencing studies were obtained from MG-RAST and the NCBI Sequence Read Archive (hereafter referred to as short-read data) by browsing for the publicly available data sets. Data sources are as reported in Table 1, with the exception of the database provided by Wei and colleagues (Wei et al., 2013), which was excluded from analysis as their data overlapped significantly with sequences obtained from original studies.
All downloaded data were re-analyzed using mothur version 1.32.1 (Schloss et al., 2009). For short-read data, flowgrams were trimmed to a single length then denoised. Where flowgrams were not available, sequences were trimmed using the trim.seqs command, removing the barcode and primer sequences and discarding sequences with an average quality score of less than 25, or sequences with a homopolymer run of greater than eight bases. All sequence data were then aligned, screened for chimeras with uchime (Edgar et al., 2011) and classified against the Greengenes taxonomy using the naïve Bayesian method (Desantis et al., 2006; Wang et al., 2007). Sequences that could not be classified to domain level, or were classified as Cyanobacteria, were removed from the dataset as they likely represent ingested plant material. Chimeric sequences and sequences that could not be aligned were also removed from the data set.
For data obtained from clone libraries it is common practice to simply upload representative sequences to GenBank, rather than the complete dataset. In order to account for the loss of abundance information from the original clone libraries, taxonomic classification was reported by calculating operational taxonomic units (OTUs) of 97% sequence similarity for each sample and assigning taxonomy using the classify.otu command in mothur. Although short-read data does contain the data from the complete sequencing run, studies did not always utilize the same 16S rRNA gene region and so could not be directly compared. In lieu of OTU generation, genus-level phylotypes were constructed using the sequence classification. For short-read data, the phylotype table was rarefied to a depth of 1500 data points and Shannon and Simpson diversity indices calculated.
Correlating Metadata to Community Structure
For clone data, sequences were trimmed to an 800 bp overlapping region and a phylogenetic tree constructed using the clearcut neighbor-joining algorithm (Evans et al., 2006) for UniFrac analysis. Sequences less than 800 bp in length were discarded, resulting in the loss of three avian samples compared with the previous classification. Due to the potential bias in relative abundance incurred by the selective uploading of data, only unweighted UniFrac distance was calculated. For short-read data there was no contiguous region of sequence common to all samples, so analysis was performed by constructing genus-level phylotypes of the classified data. Community differences were calculated using Jaccard (presence/absence) and Yue-Clayton theta (abundance) distance by randomly subsampling each community to 1500 sequences 20,000 times and averaging the community distances across iterations.
Metadata regarding the host, sample type, animal diet and captivity status were recorded and their impact on community differences compared using the vegan package (version 2.0–8) (Oksanen et al., 2013) in the R software environment (R. Core Team, 2012). Samples were grouped according to the following categories: host animal, diet and captivity status. Diet consisted of three categories—carnivore, herbivore and grain-fed—that reflected a “typical” diet of the host. When dividing animals based on diet, the distinction was made between an herbivorous diet (leaves and green plant material, such as eaten by the kakapo and hoatzin) and grain-fed diet (pelleted feed, such as found in farmed chickens) due to the different nutrient content and availability in these diets. Captivity status consisted of simply dividing samples into those animals that are wild or farm-raised. For short-read data the study that provided the data was also used as a test for how much the dynamics of the study itself shaped the data. This factor could not be applied to the clone-library data as not every original study uploaded sequences with sufficient information to recapture biological replication with the sequence data.
Permutational multivariate analysis of variance (PERMANOVA) with linear model fitting was performed (Anderson, 2001; McArdle and Anderson, 2001) in R. Samples were grouped according to each metadata factor and tested for how well the grouping accounted for the variation between samples using the “Adonis” function of the vegan package (Oksanen et al., 2013), measured as R2. A significance value (p-value) was generated by comparing the obtained R2 to that obtained from 1000 random permutations of the data. For factors with a statistically significant fit, constrained canonical analysis (CCA) was performed (Ter Braak, 1986) using the factor as the constraining variable to isolate the contribution of that factor to the microbial community.
Functional Prediction of Gut Microbiota
Following quality control of short-read data, sequences were mapped to OTUs using closed-reference OTU picking in QIIME 1.80 (Caporaso et al., 2010). 16S rRNA gene abundance levels were then normalized against the known gene copy number for that OTU and function predictions made based on OTU membership using PICRUSt (Langille et al., 2013). Functional predictions were categorized into KEGG pathways and statistical analysis performed using STAMP v2.0 (Parks and Beiko, 2010). Data were partitioned by metadata factors and differences in relative abundance tested using ANOVA, followed by post-hoc Games-Howell test with the Benjamini-Hochberg FDR used as a multiple testing correction (Benjamini and Hochberg, 1995). For testing the presence of genes involved in cellulose digestion, KEGG data were screened for pathways that mapped to COGs involved in cellulolysis and data extracted. Pair-wise comparisons were performed using Welch's t-test (Welch, 1947) with the Benjamini-Hochberg FDR.
Results and Discussion
Taxonomic Classification of OTUs
Quality-control of sequence data yielded a high number of high-quality sequences, of varying length, from a subset of the studies reported in Table 1, (Tables 2A,B). Consistent with the microbiota of vertebrates in general, the avian gut microbiota appears to harbor mostly OTUs belonging to Bacteroidetes, Firmicutes, and Proteobacteria (Figure 1). Members of the phylum Firmicutes were present in all samples analyzed, while Proteobacteria and Bacteroidetes were also widespread (Proteobacteria: 90% of clone samples, 100% of short-read samples; Bacteroidetes: 80% of clone samples, 87% of short-read samples). These three phyla are commonly observed within gut environments, and specific lineages of these phyla are frequently studied for their symbiotic roles, for example Bacteroides thetaiotaomicron starch degradation in humans (Dongowski et al., 2000; Xu et al., 2003; Sears, 2005), and Lactobacilli-associated bile salt hydrolase activity in mice and chickens (Tannock et al., 1989; Tanaka et al., 1999; Knarreborg et al., 2002). To a lesser extent, Actinobacteria (65% of clone samples, 89% short-read samples) and Tenericutes (65% of clone samples, 58% short-read samples) were also reasonably common throughout the data. Within the short-read data, a higher proportion of unclassified OTUs was observed, which may be due to a lack of phylogenetic resolution due to shorter read length. Alternatively, it has been shown that the use of the adapter/barcode construct in a single-step PCR, as is commonplace in pyrosequencing studies, can negatively affect taxonomic classification (Berry et al., 2011).
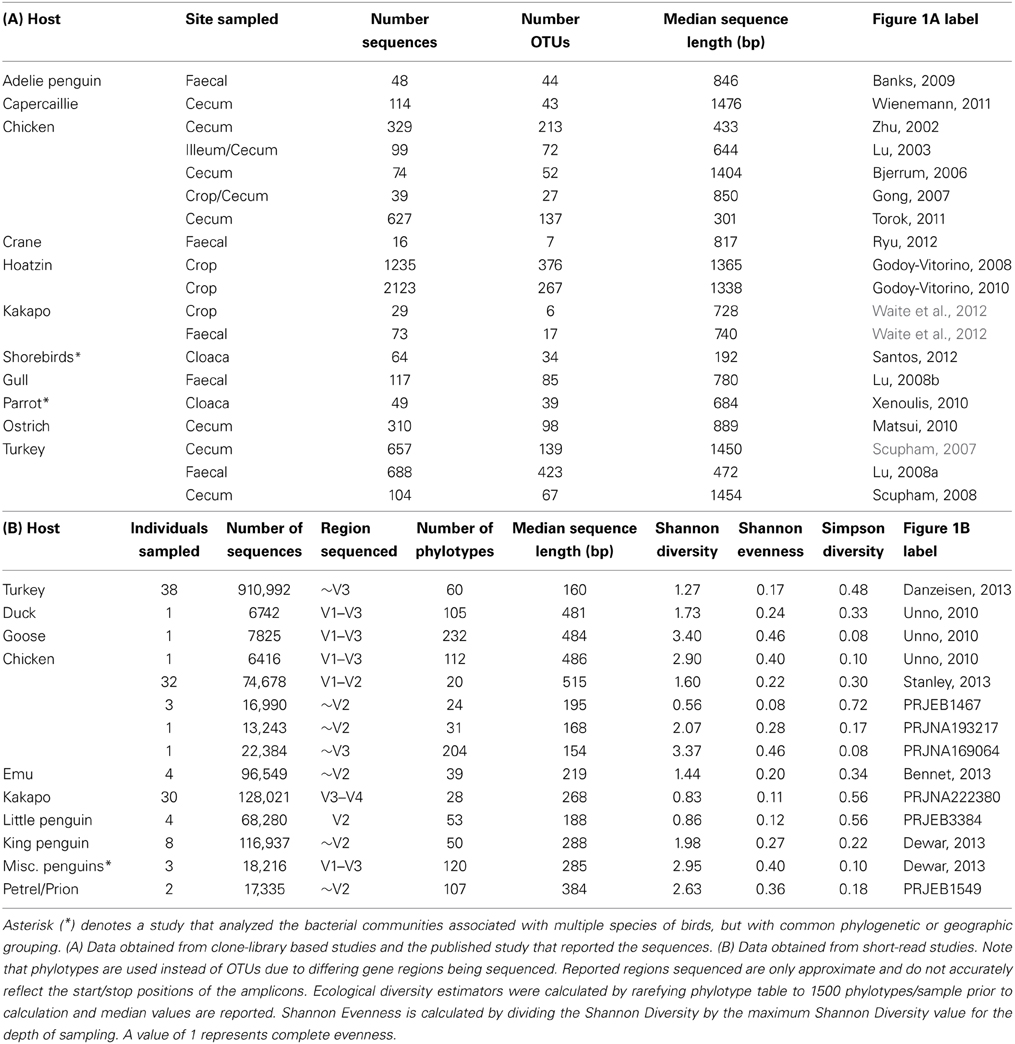
Table 2. Number of reads used, OTUs generated and average sequence length for 16S rRNA gene data utilized in the study.
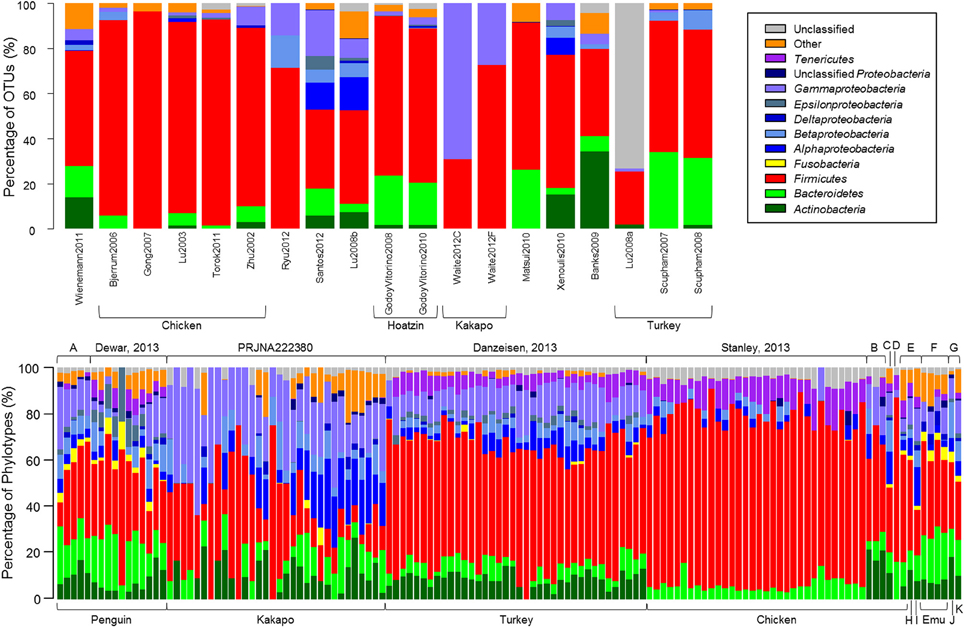
Figure 1. The relative proportion of OTUs represented in each study. OTUs were constructed by calculating average-neighbor distance between aligned 16S rRNA gene sequences in mothur and classified as a cluster of sequences with ≥97% similarity. Taxonomic classification for each OTU was derived from a consensus taxonomic classification of each sequence assigned to the OTU. (Top) Samples from clone-library data. (Bottom) Next-generation sequencing samples obtained from Sequence Read Archive. Top labels identify the study from which sequences were downloaded; bottom labels identify the host bird. Top letters denote studies PRJEB3384 (A), PRJEB1467 (B), PRJNA169064 (C) PRJNA193217 (D), Unno, 2010 (E), Bennet, 2013 (F), and PRJEB1549 (G). Bottom letters denote host organisms duck (H), goose (I), fairy prion (J), and petrel (K).
Factors Shaping the Avian Microbiota: Study vs. Host
PERMANOVA testing of the short-read data set revealed that the largest factor contributing to the shaping of the microbiota was the study itself (Table 3). This finding may be a real result, as most studies focused on a single bird geographically isolated from other studies (i.e., the “study” variable is the product of host and location), or may be an artefact resulting from the specific DNA extraction and PCR techniques involved (Boom et al., 1990; Suzuki and Giovannoni, 1996; Martin-Laurent et al., 2001; Sipos et al., 2007; Berry et al., 2011; Kennedy et al., 2014). In order to resolve this issue, we hypothesized that if the host species was truly driving the differences observed between studies, then the phylogenetic differences between taxonomically similar bacterial lineages within each study would be smaller between studies with a closely related host bird. Alternatively, a study that investigated a range of host birds would have greater within-study variation than a study that investigated a single host.
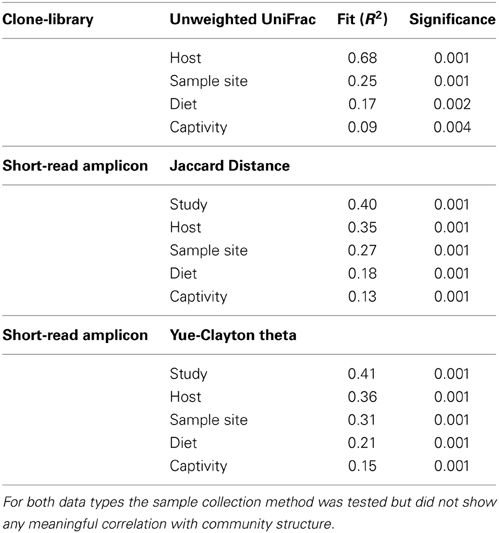
Table 3. Calculated fit of metadata factors to community distances using PERMANOVA with linear model fitting.
We identified three studies that sequenced overlapping regions of the bacterial 16S rRNA gene (Table 1, Unno, 2010, Dewar, 2013 and PRJEB3384) and observed that two bacterial genera were conserved across all three studies, namely Bacteroides and Clostridium. Sequences associated with these taxa were extracted from the main dataset and unweighted UniFrac distances were calculated between each biological replicate. The within- and between-study UniFrac distances are reported in Figure 2 and, consistent with our prediction, the within-study and between-study difference was similar when the data originated from a closely related host (Figure 2, Dewar, 2013, LittlePenguin and Dewar, 2013. LittlePenguin). By contrast, the differences between Dewar, 2013 and Unno, 2010, and LittlePenguin and Unno, 2010, were higher than the within-group difference for Clostridium and elevated compared to the penguin/penguin comparisons for Bacteroides. The within-group differences were higher for Unno, 2010-Bacteroides than for other groups, but this may be a result of the Unno, 2010 study itself analysing several different birds. Although the different methodologies employed in the various studies are likely to have some impact on the results, we concluded that this was overshadowed by the impact of the host organism and proceeded to analyse other metadata factors.
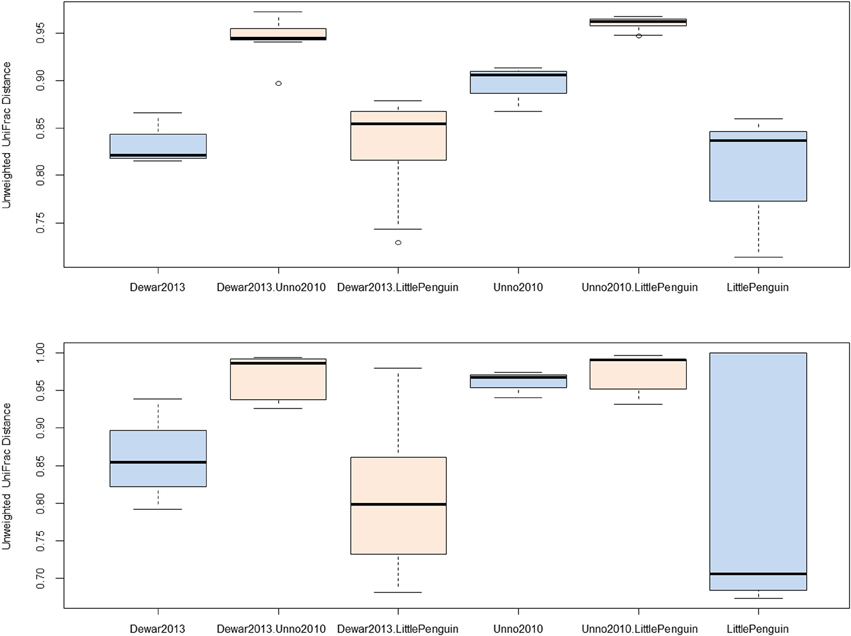
Figure 2. Unweighted UniFrac distances for within- and between-study comparisons. Distances were calculated by extracting reads classified as Clostridium (top) and Bacteroidetes (bottom) from each sample and constructing neighbor-joining phylogenetic trees based on average-neighbor distances between aligned sequences. Differences between each pair of samples were categorized as being the distance between samples from the same study or from different studies and plotted accordingly (blue = within study, orange = between study). The study “Dewar, 2013” investigated the faecal microbiota from little, king, macaroni, and gentoo penguins. The study “LittlePenguin” investigated the faecal microbiota of little penguins, and “Unno, 2010” the microbiota of a chicken, duck, and goose from a farm.
Factors Shaping the Avian Microbiota: Biological Factors
Standard ecological diversity indices revealed varying degrees of microbial diversity among the birds studied (Table 2B). In agreement with our previous observations of low microbial diversity within the kakapo hindgut (Waite et al., 2012, 2013), the diversity estimators for kakapo were among the lowest observed. Consistent with previously reported mammalian findings (Ley et al., 2008a), and with more targeted avian studies (Zhu et al., 2002; Lucas and Heeb, 2005; Banks et al., 2009; Benskin et al., 2010), the host organism was the strongest driver of community structure in the clone-library data and second strongest in the short-read data (Table 3). Other factors were still significantly associated with shaping the gut community but their fit to the data was lower. The fit for any particular factor across the data was quite low (Table 3), which is likely a result of compounding variables from the individual studies, rather than a real lack of influence of these factors. In order to account for this variation, CCA was used to visualize patterns in the data that could be accounted for by the factor of interest. Results are summarized in Figure 3 and show clear clustering of data for clone samples, but weak clustering for short-read data (Figure 4). This lack of resolution within the short-read data is likely due to the loss of OTU phylogenetic information due to non-overlapping 16S rRNA gene regions between studies. Due to the lack of phylogenetic relationship between OTUs, each OTU is considered equally different from every other OTU (Lozupone and Knight, 2005) and hence evolutionary information is lost.
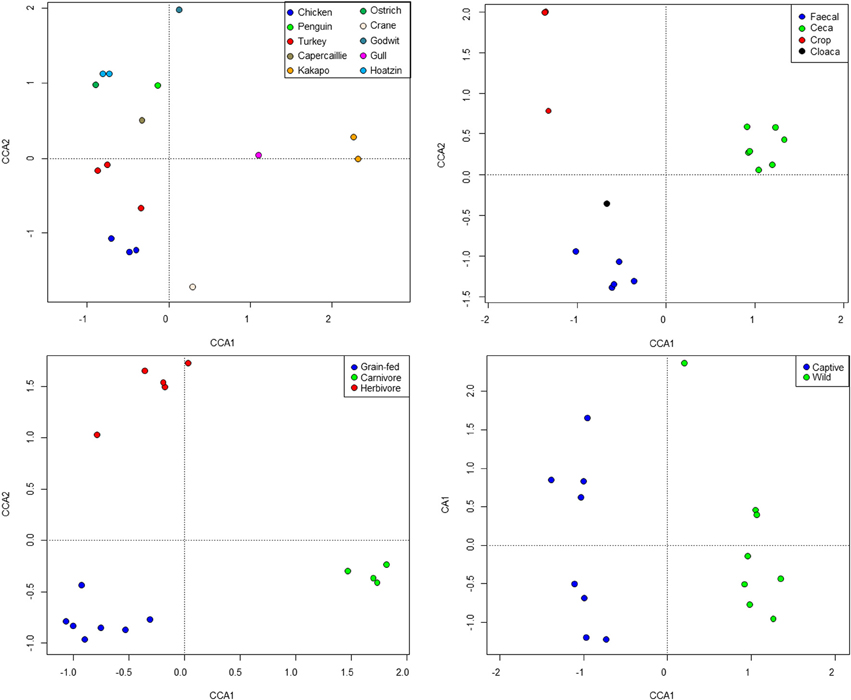
Figure 3. Constrained Canonical Analysis of community structure based on fitting of metadata factors to the clone-library sequence data. Images represent host (top left), sample site (top right), diet (bottom left), and captivity status (bottom right).
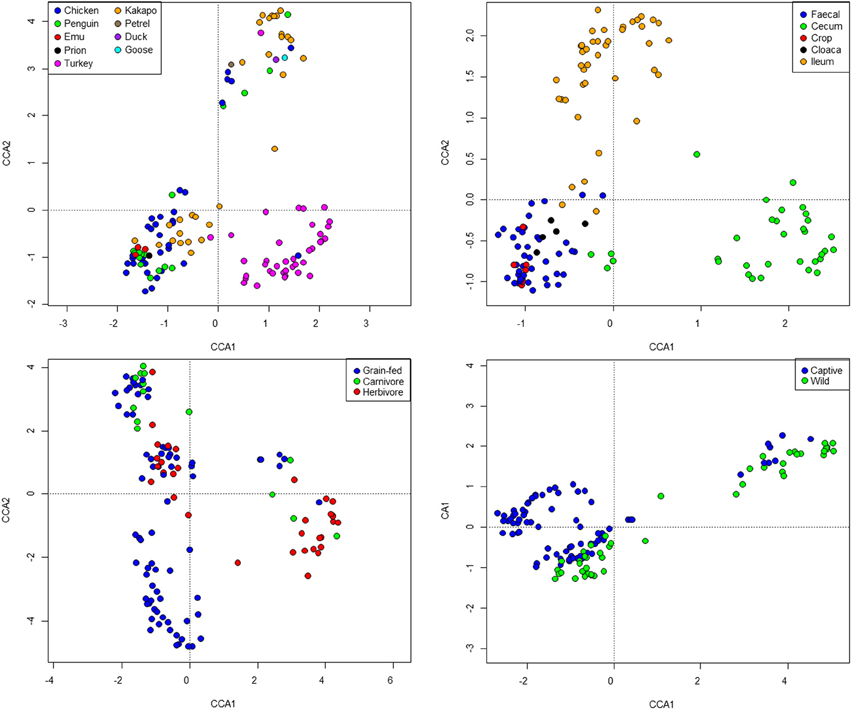
Figure 4. Constrained Canonical Analysis of community structure based on fitting of metadata factors to the short-read sequence data. Images represent host (top left), sample site (top right), diet (bottom left), and captivity status (bottom right). Note that the “herbivore” grouping represents exclusively kakapo.
Functional Prediction of the Gut Microbiota
Ultimately, the study of microbial communities is of little biological value unless the functional potential of the community, or individual members, is considered. Statistical testing revealed differences in many predicted functional pathways when data were partitioned by host, but this finding was ignored as it is a likely side-effect of 16S rRNA prediction (i.e., if the 16S rRNA-defined communities differ between hosts, the metagenomic prediction based on 16S rRNA community is also likely to differ). Metagenomes were instead partitioned by diet, captivity and gut location sampled and these categorizations of data revealed interesting differences in functional capability (Table 4). Captive birds were predicted to have a microbiota with enhanced capability for carbohydrate metabolism and a lower rate of microbial genes associated with infectious disease. When comparing predicted metagenomes by diet, the microbiota of carnivores was predicted to have a greater capability for amino acid and energy metabolism when compared to herbivores, a finding previously reported in mammals (Muegge et al., 2011). The grain-fed microbiota was predicted to have a higher capability for carbohydrate metabolism than that of herbivores. Genes involved in lactate production were predicted in all samples, which is not surprising as lactate is a known by-product of microbial activity in the ceca and is a major metabolic precursor for glucose in avians (Brady et al., 1979; Ogata et al., 1982; Franson et al., 1985). These findings provide support for the fitting of metadata categories to the samples, as the factors that contribute to shaping the microbiota were also supported by known functional roles of these microorganisms. Partitioning of data by sample site revealed several key influences on the predicted functionality of the microbiota. For example, genes grouping into the KEGG grouping “signaling molecules and interaction” were lowest in faecal samples. This grouping includes an array of genes involved in cell adhesion molecules and cytokine receptors and is likely to be involved in host/bacteria interactions. Genes involved in carbohydrate metabolism were at their lowest in foregut samples from kakapo, and elevated in the hindgut, consistent with the fact that most birds utilize their hindgut/cecum for carbohydrate fermentation (McNab, 1973; Mead, 1989).
Interestingly, the influence of diet did not match differences in the predicted ability of the microbiota to degrade cellulose. Between the three diet groupings, β-1,4-endoxylanase was more abundant in carnivorous birds than herbivorous birds. β-xylosidase activity was predicted to be higher in grain-fed birds than strictly herbivorous birds, while xylanase was higher in herbivorous birds than grain-fed (Table 4). When taken as a proportion of the total cellulolytic potential, the microbiota of carnivorous birds had a higher predicted occurrence of β-xylosidase than that of herbivorous birds, and a higher occurrence of Cellulase M than grain-fed birds. Between the non-carnivorous birds, Cellulase M and xylanase accounted for a higher proportion of cellulolytic potential in the herbivorous birds, and β-glucosidase and β-xylosidase in grain-fed birds. These genes were detected in a range of bacterial phyla within the avian gut, but particular bacterial families were enriched in the gut microbiota, likely contributing to these differences in relative gene abundance. Of the PICRUSt OTUs that carried cellulolytic potential, members of the Bifidobacteriaceae, Bacteroidaceae, and Lactobacillaceae were highly represented in metagenomes which exhibited elevated β-xylosidase and β-glucosidase levels. Leuconostocaceae were enriched in predicted metagenomes with elevated Cellulase M and β-xylosidase. Interestingly, higher abundance of xylanase genes was pre-dominantly associated with abundance of the Enterobacteriaceae, which may reflect the influence of the Proteobacteria-rich kakapo microbiota. When normalized to a proportion of the total cellulolytic gene abundance, predicted proportions of β-1,4-endoxylanase were not significantly different between dietary groupings. Although not necessarily intuitive, these findings are supported by previous observations that the cellulolytic potential of the avian hindgut is minimal (Barnes, 1972; McNab, 1973; Mead, 1989), and correlates with the observation that cellulolytic pre-digestion of feed boosts energy harvest and weight gain (Józefiak et al., 2006; Yu et al., 2008; Cowieson et al., 2010; Mathlouthi et al., 2011; Ghahri et al., 2012; Ribeiro et al., 2012) in farmed broiler chickens. Caution must be taken in interpreting these predictions, as a recent study has shown that the functional capabilities of the gut microbiota are dependent on community membership as well as genetic potential (Berry et al., 2013). Furthermore, the PICRUSt prediction framework can only account for sequences that can be accurately mapped to the existing database, with no provision for sequences representing novel, or unstudied, bacterial lineages. Nevertheless, the framework provided high-level predictions that were consistent with the known state of avian microbiology and therefore represents an excellent pathway for generation of novel hypotheses and for general annotation of 16S rRNA gene amplicon studies.
In summary, we have conducted a comprehensive meta-analysis of publicly available avian microbiota sequences and tested whether, despite notable differences in physiology between avians and mammals, the factors that drive community structure are the same. We show that the avian host species is the strongest factor in determining community composition and decoupled this effect from potential study bias where the data allowed. Finally, we have analyzed the potential functional profiles of 16S rRNA gene amplicon data and found that the genomic potential predicted of the communities fits well with the existing literature, and is therefore an excellent platform to leverage these data into new hypotheses and lines of inquiry.
Conflict of Interest Statement
The authors declare that the research was conducted in the absence of any commercial or financial relationships that could be construed as a potential conflict of interest.
Acknowledgments
This work was supported by funding from The University of Auckland Faculty Research Development Fund (grant 9841 3626187). David W. Waite was supported by a University of Auckland Doctoral Scholarship.
References
Anderson, M. J. (2001). A new method for non-parametric multivariate analysis of variance. Austral Ecol. 26, 32–46. doi: 10.1111/j.1442-9993.2001.01070.pp.x
Angelakis, E., and Raoult, D. (2010). The increase of Lactobacillus species in the gut flora of newborn Broiler chicks and ducks is associated with weight gain. PLoS ONE 5:e10463. doi: 10.1371/journal.pone.0010463
Annison, E. F., Hill, K. J., and Kenworthy, R. (1968). Volatile fatty acids in the digestive tract of the fowl. Br. J. Nutr. 22, 207–216. doi: 10.1079/BJN19680026
Bäckhed, F., Ding, H., Wang, T., Hooper, L. V., Gou, Y. K., Nagy, A., et al. (2004). The gut microbiota as an environmental factor that regulates fat storage. Proc. Natl. Acad. Sci. U.S.A. 101, 15718–15723. doi: 10.1073/pnas.0407076101
Banks, J. C., Cary, S. C., and Hogg, I. D. (2009). The phylogeography of Adelie penguin faecal ?ora. Environ. Microbiol. 11, 577–588. doi: 10.1111/j.1462-2920.2008.01816.x
Barnes, E. M. (1972). The avian intestinal flora with particular reference to the possible ecological significance of the cecal anaerobic bacteria. Am. J. Clin. Nutr. 25, 1475–1479.
Benjamini, Y., and Hochberg, Y. (1995). Controlling the false discovery rate: a practical and powerful approach to multiple testing. J. R. Stat. Soc. Series B Stat. Methodol. 57, 289–300. doi: 10.2307/2346101
Bennett, D. C., Tun, H. M., Kim, J. E., Leung, F. C., and Cheng, K. M. (2013). Characterization of cecal microbiota of the emu (Dromaius novaehollandiae). Vet. Microbiol. 166, 304–310. doi: 10.1016/j.vetmic.2013.05.018
Benskin, C. M., Rhodes, G., Pickup, R. W., Wilson, K., and Hartley, I. R. (2010). Diversity and temporal stability of bacterial communities in a model passerine bird, the zebra finch. Mol. Ecol. 19, 5531–5544. doi: 10.1111/j.1365-294X.2010.04892.x
Berry, D., Mahfoudh, K. B., Wagner, M., and Loy, A. (2011). Barcoded primers used in multiplex amplicon pyrosequencing bias amplification. Appl. Environ. Microbiol. 77, 7846–7849. doi: 10.1128/AEM.05220-11
Berry, D., Stecher, B., Schintlmeister, A., Reichert, J., Brugiroux, S., Wild, B., et al. (2013). Host-compound foraging by intestinal microbiota revealed by single-cell stable isotope probing. Proc. Natl. Acad. Sci. U.S.A. 110, 4720–4725. doi: 10.1073/pnas.1219247110
Bjerrum, L., Engberg, R. M., Leser, T. D., Jensen, B. B., Finster, K., and Pedersen, K. (2006). Microbial community composition of the ileum and cecum of broiler chickens as revealed by molecular and culture-based techniques. Poult. Sci. 85, 1151–1164. doi: 10.1093/ps/85.7.1151
Björkholm, B., Bok, C. M., Lundin, A., Rafter, J., Hibberd, M. L., and Pettersson, S. (2009). Intestinal microbiota regulate xenobiotic metabolism in the liver. PLoS ONE 4:e6958. doi: 10.1371/journal.pone.0006958
Blanco, G., Lemus, J. A., and Grande, J. (2006). Faecal bacteria associated with different diets of wintering red kites: Influence of livestock carcass dumps in microflora alteration and pathogen acquisition. J. Appl. Ecol. 43, 990–998. doi: 10.1111/j.1365-2664.2006.01200.x
Bolton, W. (1965). Digestion in the crop of the fowl. Br. Poult. Sci. 6, 97–102. doi: 10.1080/00071666508415561
Boom, R., Sol, C. J. A., Salimans, M. M. M., Jansen, C. L., Wertheim-Van Dillen, P. M. E., and Van Der Noordaa, J. (1990). Rapid and simple method for purification of nucleic acids. J. Clin. Microbiol. 28, 495–503.
Brady, L. J., Romsos, D. R., and Leveille, G. A. (1979). Gluconeogenesis in isolated chicken (Gallus domesticus) liver cells. Comp. Biochem. Physiol. B Biochem. Mol. Biol. 63, 193–198. doi: 10.1016/0305-0491(79)90029-4
Cao, G. T., Xiao, Y. P., Yang, C. M., Chen, A. G., Liu, T. T., Zhou, L., et al. (2012). Effects of Clostridium butyricum on growth performance, nitrogen metabolism, intestinal morphology and cecal microflora in broiler chickens. J. Anim. Vet. Adv. 11, 2665–2671. doi: 10.3923/javaa.2012.2665.2671
Caporaso, J. G., Kuczynski, J., Stombaugh, J., Bittinger, K., Bushman, F. D., Costello, E. K., et al. (2010). QIIME allows analysis of high-throughput community sequencing data. Nat. Methods 7, 335–336. doi: 10.1038/nmeth.f.303
Chu, H., Fierer, N., Lauber, C. L., Caporaso, J. G., Knight, R., and Grogan, P. (2010). Soil bacterial diversity in the Arctic is not fundamentally different from that found in other biomes. Environ. Microbiol. 12, 2998–3006. doi: 10.1111/j.1462-2920.2010.02277.x
Clemens, E. T., Stevens, C. E., and Southworth, M. (1975). Sites of organic acid production and pattern of digesta movement in the gastrointestinal tract of geese. J. Nutr. 105, 1341–1350.
Cowieson, A. J., Bedford, M. R., and Ravindran, V. (2010). Interactions between xylanase and glucanase in maize-soy-based diets for broilers. Br. Poult. Sci. 51, 246–257. doi: 10.1080/00071661003789347
Cutler, S. A., Rasmussen, M. A., Hensley, M. J., Wilhelms, K. W., Griffith, R. W., and Scanes, C. G. (2005). Effects of Lactobacilli and lactose on Salmonella typhimurium colonisation and microbial fermentation in the crop of the young turkey. Br. Poult. Sci. 46, 708–716. doi: 10.1080/00071660500393694
Danzeisen, J. L., Calvert, A. J., Noll, S. L., McComb, B., Sherwood, J. S., Loque, C. M., et al. (2013). Succession of the turkey gastrointestinal bacterial microbiome related to weight gain. PeerJ 1:e237. doi: 10.7717/peerj.237
Desantis, T. Z., Hugenholtz, P., Larsen, N., Rojas, M., Brodie, E. L., Keller, K., et al. (2006). Greengenes, a chimera-checked 16S rRNA gene database and workbench compatible with ARB. Appl. Environ. Microbiol. 72, 5069–5072. doi: 10.1128/AEM.03006-05
Dewar, M. L., Arnould, J. P., Dann, P., Trathan, P., Groscolas, R., and Smith, S. (2013). Interspecific variations in the gastrointestinal microbiota in penguins. Microbiologyopen 2, 195–204. doi: 10.1002/mbo3.66
Domínguez-Bello, M. G., Lovera, M., Suarez, P., and Michelangeli, F. (1993). Microbial digestive symbionts of the crop of the hoatzin (Opisthocomus hoazin): an avian foregut fermenter. Physiol. Zool. 66, 374–383.
Dongowski, G., Lorenz, A., and Anger, H. (2000). Degradation of pectins with different degrees of esterification by Bacteroides thetaiotaomicron isolated from human gut flora. Appl. Environ. Microbiol. 66, 1321–1327. doi: 10.1128/AEM.66.4.1321-1327.2000
Duke, G. E., Eccleston, E., Kirkwood, S., Louis, C. F., and Bedbury, H. P. (1984). Cellulose digestion by domestic turkeys fed low or high fiber diets. J. Nutr. 114, 95–102.
Edgar, R. C., Haas, B. J., Clemente, J. C., Quince, C., and Knight, R. (2011). UCHIME improves sensitivity and speed of chimera detection. Bioinformatics 27, 2194–2200. doi: 10.1093/bioinformatics/btr381
Evans, J., Sheneman, L., and Foster, J. (2006). Relaxed neighbor joining: a fast distance-based phylogenetic tree construction method. J. Mol. Evol. 62, 785–792. doi: 10.1007/s00239-005-0176-2
Ford, D. J., and Coates, M. E. (1971). Absorption of glucose and vitamins of the B complex by germ-free and conventional chicks. Proc. Nutr. Soc. 30, 10A–11A.
Franson, J. C., Murray, H. C., and Bunck, C. (1985). Enzyme activities in plasma, kidney, liver, and muscle of five avian species. J. Wildl. Dis. 21, 33–39. doi: 10.7589/0090-3558-21.1.33
Ghahri, H., Molodian, M., Habibian, R., and Namavar, M. (2012). Effects of xylanase and β-glucanase on performance and humoral immune response of broilers fed wheat-corn-soy based nutritionally marginal diets. Glob. Vet. 8, 264–269. Available online at: http://www.idosi.org/gv/gv8(3)12.htm
Godoy-Vitorino, F., Goldfarb, K. C., Brodie, E. L., Garcia-Amado, M. A., Michelangeli, F., and Domínguez-Bello, M. G. (2010). Developmental microbial ecology of the crop of the folivorous hoatzin. ISME J. 4, 611–620. doi: 10.1038/ismej.2009.147
Godoy-Vitorino, F., Ley, R. E., Gao, Z., Pei, Z., Ortiz-Zuazaga, H., Pericchi, L. R., et al. (2008). Bacterial community in the crop of the hoatzin, a neotropical folivorous flying bird. Appl. Environ. Microbiol. 74, 5905–5912. doi: 10.1128/AEM.00574-08
Gong, J., Weiduo, S., Forster, R. J., Huang, R., Yu, H., Yin, Y., et al. (2007). 16S rRNA gene-based analysis of mucosa-associated bacterial community and phylogeny in the chicken gastrointestinal tracts: from crops to ceca. FEMS Microbiol. Ecol. 59, 147–157. doi: 10.1111/j.1574-6941.2006.00193.x
Grajal, A., Strahl, S. D., Pabra, R., Dominguez, M. G., and Neher, A. (1989). Foregut fermentation in the hoatzin, a neotropical leaf-eating bird. Science 245, 1236–1238. doi: 10.1126/science.245.4923.1236
Hammons, S., Oh, P. L., Martínez, I., Clark, K., Schlegel, V. L., Sitorius, E., et al. (2010). A small variation in diet influences the Lactobacillus strain composition in the crop of broiler chickens. Syst. Appl. Microbiol. 33, 275–281. doi: 10.1016/j.syapm.2010.04.003
Janczyk, P., Halle, B., and Souffrant, W. B. (2009). Microbial community composition of the crop and ceca contents of laying hens fed diets supplemented with Chlorella vulgaris. Poult. Sci. 88, 2324–2332. doi: 10.3382/ps.2009-00250
Jin, L. Z., Ho, Y. W., Abdullah, N., Ali, M. A., and Jalaludin, S. (1998). Effects of adherent Lactobacillus cultures on growth, weight of organs and intestinal microflora and volatile fatty acids in broilers. Anim. Feed Sci. Technol. 70, 197–209. doi: 10.1016/S0377-8401(97)00080-1
Józefiak, D., Rutkowski, A., Jensen, B. B., and Engberg, R. M. (2006). The effect of β-glucanase supplementation of barley- and oat-based diets on growth performance and fermentation in broiler chicken gastrointestinal tract. Br. Poult. Sci. 47, 57–64. doi: 10.1080/00071660500475145
Józefiak, D., Rutkowski, A., and Martin, S. A. (2004). Carbohydrate fermentation in the avian ceca: a review. Anim. Feed Sci. Technol. 113, 1–15. doi: 10.1016/j.anifeedsci.2003.09.007
Kennedy, N. A., Walker, A. W., Berry, S. H., Duncan, S. H., Farquarson, F. M., Louis, P., et al. (2014). The impact of different DNA extraction kits and laboratories upon the assessment of human gut microbiota composition by 16s rRNA gene sequencing. PLoS ONE 9:e88982. doi: 10.1371/journal.pone.0088982
Knarreborg, A., Engberg, R. M., Jensen, S. K., and Jensen, B. B. (2002). Quantitative determination of bile salt hydrolase activity in bacteria isolated from the small intestine of chickens. Appl. Environ. Microbiol. 68, 6425–6428. doi: 10.1128/AEM.68.12.6425-6428.2002
Kohl, K. D. (2012). Diversity and function of the avian gut microbiota. J. Comp. Physiol. B 182, 591–602. doi: 10.1007/s00360-012-0645-z
Langille, M. G. I., Zaneveld, J., Caporaso, J. G., McDonald, D., Knights, D., Reyes, J. A., et al. (2013). Predictive functional profiling of microbial communities using 16S rRNA marker gene sequences. Nat. Biotechnol. 31, 814–821. doi: 10.1038/nbt.2676
Leopold, A. S. (1953). Intestinal morphology of gallinaceous birds in relation to food habits. J. Wildl. Manage. 17, 197–203. doi: 10.2307/3796715
Ley, R. E., Hamady, M., Lozupone, C., Turnbaugh, P. J., Ramey, R. R., Bircher, J. S., et al. (2008a). Evolution of mammals and their gut microbes. Science 320, 1647–1651. doi: 10.1126/science.1155725
Ley, R. E., Lozupone, C. A., Hamady, M., Knight, R., and Gordon, J. I. (2008b). Worlds within worlds: evolution of the vertebrate gut microbiota. Nat. Rev. Microbiol. 6, 776–788. doi: 10.1038/nrmicro1978
Lozupone, C., and Knight, R. (2005). UniFrac: a new phylogenetic method for comparing microbial communities. Appl. Environ. Microbiol. 71, 8228–8235. doi: 10.1128/AEM.71.12.8228-8235.2005
Lozupone, C., and Knight, R. (2007). Global patterns in bacterial diversity. Proc. Natl. Acad. Sci. U.S.A. 104, 11436–11440. doi: 10.1073/pnas.0611525104
Lu, J., and Domingo, J. S. (2008). Turkey fecal microbial community structure and functional gene diversity revealed by 16S rRNA gene and metagenomic sequences. J. Microbiol. 46, 469–477. doi: 10.1007/s12275-008-0117-z
Lu, J., Idris, U., Harmon, B., Hofacre, C., Maurer, J. J., and Lee, M. D. (2003). Diversity and succession of the intestinal bacterial community of the maturing broiler chicken. Appl. Environ. Microbiol. 69, 6816–6824. doi: 10.1128/AEM.69.11.6816-6824.2003
Lu, J., Santo-Domingo, J. W., Lamendella, R., Edge, T., and Hill, S. (2008). Phylogenetic diversity and molecular detection of bacteria in gull feces. Appl. Environ. Microbiol. 74, 3969–3976. doi: 10.1128/AEM.00019-08
Lucas, F. S., and Heeb, P. (2005). Environmental factors shape cloacal bacterial assemblages in great tit Parus major and blue tit P. caeruleus nestlings. J. Avian Biol. 36, 510–516. doi: 10.1111/j.0908-8857.2005.03479.x
Martin-Laurent, F., Philippot, L., Hallet, S., Chaussod, R., Germon, J. C., Soulas, G., et al. (2001). DNA extraction from soils: old bias for new microbial diversity analysis methods. Appl. Environ. Microbiol. 67, 2354–2359. doi: 10.1128/AEM.67.5.2354-2359.2001
Mathlouthi, N., Ballet, N., and Larbier, M. (2011). Influence of beta-glucanase supplementation of growth performances and digestive organs weights of broiler chickens fed corn, wheat and barley-based diet. Int. J. Poult. Sci. 10, 157–159. doi: 10.3923/ijps.2011.157.159
Matsui, H., Kato, Y., Chikaraishi, T., Moritani, M., Ban-Tokuda, T., and Wakita, M. (2010). Microbial diversity in ostrich ceca as revealed by 16S ribosomal RNA gene clone library and detection of novel Fibrobacter species. Anaerobe 16, 83–93. doi: 10.1016/j.anaerobe.2009.07.005
McArdle, B. H., and Anderson, M. J. (2001). Fitting multivariate models to community data: a comment on distance-based redundancy analysis. Ecology 82, 290–297. doi: 10.1890/0012-9658(2001)082[0290:FMMTCD]2.0.CO;2
McNab, J. M. (1973). The avian caeca: a review. Worlds Poult. Sci. J. 29, 251–263. doi: 10.1079/WPS19730014
Mead, G. C. (1989). Microbes of the avian cecum: types present and substrates utilized. J. Exp. Zool. 252, 48–54. doi: 10.1002/jez.1402520508
Meinl, W., Sczesny, S., Brigelius-Flohé, R., Blaut, M., and Glatt, H. (2009). Impact of gut microbiota on intestinal and hepatic levels of phase 2 xenobiotic-metabolizing enzymes in the rat. Drug Metab. Dispos. 37, 1179–1186. doi: 10.1124/dmd.108.025916
Miller, M. R. (1976). Cecal fermentation in mallards in relation to diet. Condor 78, 107–111. doi: 10.2307/1366928
Moore, R. W., Park, S. Y., Kubena, L. F., Byrd, J. A., McReynolds, J. L., Burnham, M. R., et al. (2004). Comparison of zinc acetate and propionate addition on gastrointestinal tract fermentation and susceptibility of laying hens to Salmonella enteritidis during forced molt. Poult. Sci. 83, 1276–1286. doi: 10.1093/ps/83.8.1276
Muegge, B. D., Kuczynski, J., Knights, D., Clemente, J. C., González, A., Fontana, L., et al. (2011). Diet drives convergence in gut microbiome functions across mammalian phylogeny and within humans. Science 332, 970–974. doi: 10.1126/science.1198719
Nawrot, R., Barylski, J., Tomaszewski, Ł, Jerzak, L., GoŸdzicka-Józefiak, A., Jêdrzejewski, S., et al. (2009). Identification of bacterial species in white stork chicks in Poland using PCR method and sequencing of bacterial 16S rRNA. Polish J. Environ. Stud. 18, 301–304. Available online at: http://www.cabdirect.org/abstracts/20093110993.html;jsessionid=EDBEAC7C7143C15C66F1B8324CE80C0E
Ogata, K., Watford, M., Brady, L. J., and Hanson, R. W. (1982). Mitochondrial phosphoenolpyruvate carboxykinase (GTP) and the regulation of gluconeogenesis and ketogenesis in avian liver. J. Biol. Chem. 257, 5385–5391.
Oksanen, J., Blanchet, F. G., Kindt, R., Legendre, P., Minchin, P. R., O'Hara, R. B., et al. (2013). Vegan: Community Ecology. Available online at: http://CRAN.R-project.org/package=vegan
Pacheco, M. A., Alexandra García-Amado, M., Bosque, C., and Domínguez-Bello, M. G. (2004). Bacteria in the crop of the seed-eating green-rumped parrotlet. Condor 106, 139–143. doi: 10.1650/7406
Parks, D. H., and Beiko, R. G. (2010). Identifying biologically relevant differences between metagenomic communities. Bioinformatics 26, 715–721. doi: 10.1093/bioinformatics/btq041
Potti, J., Moreno, J., Yorio, P., Briones, V., García-Borboroglu, P., Villar, S., et al. (2002). Bacteria divert resources from growth for magellanic penguin chicks. Ecol. Lett. 5, 709–714. doi: 10.1046/j.1461-0248.2002.00375.x
Preest, M. R., Folk, D. G., and Beuchat, C. A. (2003). Decomposition of nitrogenous compounds by intestinal bacteria in hummingbirds. Auk 120, 1091–1101. doi: 10.1642/0004-8038(2003)120[1091:DONCBI]2.0.CO;2
Pritchard, P. J. (1972). Digestion of sugars in the crop. Comp. Biochem. Physiol. A Mol. Integr. Physiol. 43, 195–205. doi: 10.1016/0300-9629(72)90482-3
Rahimi, S., Grimes, J. L., Fletcher, O., Oviedo, E., and Sheldon, B. W. (2009). Effect of a direct-fed microbial (Primalac) on structure and ultrastructure of small intestine in turkey poults. Poult. Sci. 88, 491–503. doi: 10.3382/ps.2008-00272
R.Core Team. (2012). R: A Language and Environment for Statistical Computing. Vienna, Austria. Available online at: http://www.R-project.org/
Redig, P. T. (1989). The avian ceca: obligate combustion chambers or facultative afterburners? - The conditioning influence of diet. J. Exp. Zool. 252, 66–69. doi: 10.1002/jez.1402520511
Ribeiro, T., Lordelo, M. M. S., Prates, J. A. M., Falcão, L., Freire, J. P. B., Ferreira, L. M. A., et al. (2012). The thermostable β-1,3-1,4-glucanase from Clostridium thermocellum improves the nutritive value of highly viscous barley-based diets for broilers. Br. Poult. Sci. 53, 224–234. doi: 10.1080/00071668.2012.674632
Rubio, L. A., Brenes, A., Setién, I., De La Asunción, G., Durán, N., and Cutuli, M. T. (1998). Lactobacilli counts in crop, ileum and caecum of growing broiler chickens fed on practical diets containing whole or dehulled sweet lupin (Lupinus angustifolius) seed meal. Br. Poult. Sci. 39, 354–359. doi: 10.1080/00071669888890
Ryu, H., Lu, J., Vogel, J., Elk, M., Chávez-Ramírez, F., Ashbolt, N., et al. (2012). Development and evaluation of a quantitative PCR assay targeting sandhill crane (Grus canadensis) fecal pollution. Appl. Environ. Microbiol. 78, 4338–4345. doi: 10.1128/AEM.07923-11
Santos, S. S., Pardal, S., Proença, D. N., Lopes, R. J., Ramos, J. A., Mendes, L., et al. (2012). Diversity of cloacal microbial community in migratory shorebirds that use the Tagus estuary as stopover habitat and their potential to harbor and disperse pathogenic microorganisms. FEMS Microbiol. Ecol. 82, 63–74. doi: 10.1111/j.1574-6941.2012.01407.x
Schloss, P. D., Westcott, S. L., Ryabin, T., Hall, J. R., Hartmann, M., Hollister, E. B., et al. (2009). Introducing mothur: open-source, platform-independent, community-supported software for describing and comparing microbial communities. Appl. Environ. Microbiol. 75, 7537–7541. doi: 10.1128/AEM.01541-09
Scupham, A. J. (2007). Succession in the intestinal microbiota of preadolescent turkeys. FEMS Microbiol. Ecol. 60, 136–147. doi: 10.1111/j.1574-6941.2006.00245.x
Scupham, A. J., Patton, T. G., Bent, E., and Bayles, D. O. (2008). Comparison of the cecal microbiota of domestic and wild turkeys. Microb. Ecol. 56, 322–331. doi: 10.1007/s00248-007-9349-4
Sears, C. L. (2005). A dynamic partnership: celebrating our gut flora. Anaerobe 11, 247–251. doi: 10.1016/j.anaerobe.2005.05.001
Shade, A., Caporaso, J. G., Handelsman, J., Knight, R., and Fierer, N. (2013). A meta-analysis of changes in bacterial and archaeal communities with time. ISME J. 7, 1493–1506. doi: 10.1038/ismej.2013.54
Shetty, S., Sridhar, K. R., Shenoy, K. B., and Hegde, S. N. (1990). Observations on bacteria associated with pigeon crop. Folia Microbiol. (Praha) 35, 240–244. doi: 10.1007/BF02820491
Singh, P., Karmi, A., Devendra, K., Waldroup, P. W., Cho, K. K., and Kwon, Y. M. (2013). Influence of penicillin on microbial diversity of the cecal microbiota in broiler chickens. Poult. Sci. 92, 272–276. doi: 10.3382/ps.2012-02603
Sipos, R., Székely, A. J., Palatinszky, M., Révész, S., Márialigeti, K., and Nikolausz, M. (2007). Effect of primer mismatch, annealing temperature and PCR cycle number on 16S rRNA gene-targetting bacterial community analysis. FEMS Microbiol. Ecol. 60, 341–350. doi: 10.1111/j.1574-6941.2007.00283.x
Stanley, D., Denman, S. E., Hughes, R. J., Geier, M. S., Crowley, T. M., Chen, H., et al. (2012). Intestinal microbiota associated with differential feed conversion efficiency in chickens. Appl. Microbiol. Biotechnol. 96, 1361–1369. doi: 10.1007/s00253-011-3847-5
Stanley, D., Geier, M. S., Hughes, R. J., Denman, S. E., and Moore, R. J. (2013). Highly variable microbiota development in the chicken gastrointestinal tract. PLoS ONE 8:e84290. doi: 10.1371/journal.pone.0084290
Stappenbeck, T. S., Hooper, L. V., and Gordon, J. I. (2002). Developmental regulation of intestinal angiogenesis by indigenous microbes via Paneth cells. Proc. Natl. Acad. Sci. U.S.A. 99, 15451–15455. doi: 10.1073/pnas.202604299
Stevens, C. E., and Hume, I. D. (1998). Contributions of microbes in vertebrate gastrointestinal tract to production and conservation of nutrients. Physiol. Rev. 78, 393–427.
Sullam, K. E., Essinger, S. D., Lozupone, C. A., O'Connor, M. P., Rosen, G. L., Knight, R., et al. (2012). Environmental and ecological factors that shape the gut bacterial communities of ?sh: a meta-analysis. Mol. Ecol. 21, 3363–3378. doi: 10.1111/j.1365-294X.2012.05552.x
Suzuki, M. T., and Giovannoni, S. J. (1996). Bias caused by template annealing in the amplification of mixtures of 16S rRNA genes by PCR. Appl. Environ. Microbiol. 62, 625–630.
Tanaka, H., Doesburg, K., Iwasaki, T., and Mierau, I. (1999). Screening of lactic acid bacteria for bile salt hydrolase activity. J. Dairy Sci. 82, 2530–2535. doi: 10.3168/jds.S0022-0302(99)75506-2
Tannock, G. W., Deshkevicz, M. P., and Feighner, S. D. (1989). Lactobacilli and bile salt hydrolase in the murine intestinal tract. Appl. Environ. Microbiol. 55, 1848–1851.
Ter Braak, C. J. F. (1986). Canonical correspondence analysis: a new eigenvector technique for multivariate direct gradient analysis. Ecology 67, 1167–1179. doi: 10.2307/1938672
Torok, V. A., Hughes, R. J., Mikkelsen, L. L., Perez-Maldonado, R., Balding, K., Macalpine, R., et al. (2011). Identification and characterization of potential performance-related gut microbiotas in broiler chickens across various feeding trials. Appl. Environ. Microbiol. 77, 5868–5878. doi: 10.1128/AEM.00165-11
Torok, V. A., Ophel-Keller, K., Loo, M., and Hughes, R. J. (2008). Application of methods for identifying broiler chicken gut bacterial species linked with increased energy metabolism. Appl. Environ. Microbiol. 74, 783–791. doi: 10.1128/AEM.01384-07
Turnbaugh, P. J., Ley, R. E., Mahowald, M. A., Magrini, V., Mardis, E. R., and Gordon, J. I. (2006). An obesity-associated gut microbiome with increased capacity for energy harvest. Nature 444, 1027–1031. doi: 10.1038/nature05414
Unno, T., Jang, J., Han, D., Kim, J. H., Sadowsky, M. J., Kim, O. S., et al. (2010). Use of barcoded pyrosequencing and shared OTUs to determine sources of fecal bacteria in watersheds. Environ. Sci. Technol. 44, 7777–7782. doi: 10.1021/es101500z
Van Der Wielen, P. W. J. J., Keuzenkamp, D. A., Lipman, L. J. A., Van Knapen, F., and Biesterveld, S. (2002). Spatial and temporal variation of the intestinal bacterial community in commercially raised broiler chickens during growth. Microb. Ecol. 44, 286–293. doi: 10.1007/s00248-002-2015-y
Van Dongen, W. F. D., White, J., Brandl, H. B., Moodley, Y., Merkling, T., Leclaire, S., et al. (2013). Age-related differences in the cloacal microbiota of a wild bird species. BMC Ecol. 13:11. doi: 10.1186/1472-6785-13-11
Vispo, C., and Karasov, W. H. (1997). “The interaction of avian gut microbes and their host: an elusive symbiosis,” in Gastrointestinal Microbiology, eds R. I. Mackie and B. A. White (London: Chapmann & Hall), 116–155.
Waite, D. W., Deines, P., and Taylor, M. W. (2012). Gut microbiome of the critically endangered New Zealand parrot, the kakapo (Strigops habroptilus). PLoS ONE 7:e35803. doi: 10.1371/journal.pone.0035803
Waite, D. W., Deines, P., and Taylor, M. W. (2013). Quantifying the impact of storage procedures for faecal bacteriotherapy in the critically endangered New Zealand parrot, the kakapo (Strigops habroptilus). Zool. Biol. 32, 620–625. doi: 10.1002/zoo.21098
Wang, Q., Garrity, G. M., Tiedje, J. M., and Cole, J. R. (2007). Naïve Bayesian classifier for rapid assignment of rRNA sequences into the new bacterial taxonomy. Appl. Environ. Microbiol. 73, 5261–5267. doi: 10.1128/AEM.00062-07
Wei, S., Morrison, M., and Yu, Z. (2013). Bacterial census of poultry intestinal microbiome. Poult. Sci. 92, 671–683. doi: 10.3382/ps.2012-02822
Welch, B. L. (1947). The generalization of “Student's” problem when several different population variances are involved. Biometrika 34, 28–35.
Wienemann, T., Schmitt-Wagner, D., Meuser, K., Segelbacher, G., Schink, B., Brune, A., et al. (2011). The bacterial microbiota in the ceca of Capercaillie (Tetrao urogallus) differs between wild and captive birds. Syst. Appl. Microbiol. 34, 542–551. doi: 10.1016/j.syapm.2011.06.003
Wright, A. G., Northwood, K. S., and Obispo, N. E. (2009). Rumen-like methanogens identified from the crop of the folivorous South American bird, the hoatzin (Opisthocomus hoazin). ISME J. 3, 1120–1126. doi: 10.1038/ismej.2009.41
Xenoulis, P. G., Gray, P. L., Brightsmith, D., Palculict, B., Hoppes, S., Steiner, J. M., et al. (2010). Molecular characterization of the cloacal microbiota of wild and captive parrots. Vet. Microbiol. 146, 320–325. doi: 10.1016/j.vetmic.2010.05.024
Xu, J., Bjursell, M. K., Himrod, J., Deng, S., Carmichael, L. K., Chiang, H., et al. (2003). A genomic view of the human–Bacteroides thetaiotaomicron symbiosis. Science 299, 2074–2076. doi: 10.1126/science.1080029
Yu, B., Liu, J. R., Hsaio, F. S., and Chiou, P. W. S. (2008). Evaluation of Lactobacillus reuteri Pg4 strain expressing heterologous β-glucanase as a probiotic in poultry diets based on barley. Anim. Feed Sci. Technol. 141, 82–91. doi: 10.1016/j.anifeedsci.2007.04.010
Zhang, B., Yang, X., Guo, Y., and Long, F. (2011). Effects of dietary lipids and Clostridium butyricum on the performance and the digestive tract of broiler chickens. Arch. Anim. Nutr. 65, 329–339. doi: 10.1080/1745039X.2011.568274
Keywords: bird, avian, microbiota, bacteria, 16S rRNA gene, meta-analysis
Citation: Waite DW and Taylor MW (2014) Characterizing the avian gut microbiota: membership, driving influences, and potential function. Front. Microbiol. 5:223. doi: 10.3389/fmicb.2014.00223
Received: 20 March 2014; Accepted: 28 April 2014;
Published online: 16 May 2014.
Edited by:
Ute Hentschel, University of Wuerzburg, GermanyReviewed by:
Clarissa Schwab, ETH Zurich, SwitzerlandLucas Moitinho-Silva, University of Wuerzburg, Germany
Copyright © 2014 Waite and Taylor. This is an open-access article distributed under the terms of the Creative Commons Attribution License (CC BY). The use, distribution or reproduction in other forums is permitted, provided the original author(s) or licensor are credited and that the original publication in this journal is cited, in accordance with accepted academic practice. No use, distribution or reproduction is permitted which does not comply with these terms.
*Correspondence: Michael W. Taylor, School of Biological Sciences, University of Auckland, Thomas Building, Symonds Street, Private Bag 92019, Auckland, New Zealand e-mail: mw.taylor@auckland.ac.nz