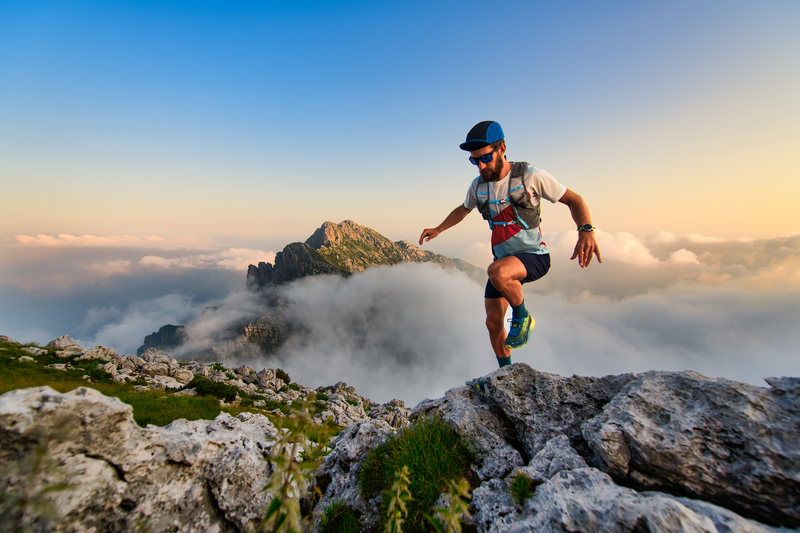
95% of researchers rate our articles as excellent or good
Learn more about the work of our research integrity team to safeguard the quality of each article we publish.
Find out more
ORIGINAL RESEARCH article
Front. Microbiol. , 03 March 2014
Sec. Extreme Microbiology
Volume 5 - 2014 | https://doi.org/10.3389/fmicb.2014.00080
Marine subsurface sediments on the Pacific margin harbor diverse microbial communities even at depths of several hundreds meters below the seafloor (mbsf) or more. Previous PCR-based molecular analysis showed the presence of diverse reductive dehalogenase gene (rdhA) homologs in marine subsurface sediment, suggesting that anaerobic respiration of organohalides is one of the possible energy-yielding pathways in the organic-rich sedimentary habitat. However, primer-independent molecular characterization of rdhA has remained to be demonstrated. Here, we studied the diversity and frequency of rdhA homologs by metagenomic analysis of five different depth horizons (0.8, 5.1, 18.6, 48.5, and 107.0 mbsf) at Site C9001 off the Shimokita Peninsula of Japan. From all metagenomic pools, remarkably diverse rdhA-homologous sequences, some of which are affiliated with novel clusters, were observed with high frequency. As a comparison, we also examined frequency of dissimilatory sulfite reductase genes (dsrAB), key functional genes for microbial sulfate reduction. The dsrAB were also widely observed in the metagenomic pools whereas the frequency of dsrAB genes was generally smaller than that of rdhA-homologous genes. The phylogenetic composition of rdhA-homologous genes was similar among the five depth horizons. Our metagenomic data revealed that subseafloor rdhA homologs are more diverse than previously identified from PCR-based molecular studies. Spatial distribution of similar rdhA homologs across wide depositional ages indicates that the heterotrophic metabolic processes mediated by the genes can be ecologically important, functioning in the organic-rich subseafloor sedimentary biosphere.
Previous microbiological studies conducted in conjunction with scientific ocean drilling have demonstrated remarkably high microbial cell abundance in deep and old organic carbon-rich marine subsurface sediments in the margins of the Pacific Ocean (Parkes et al., 1994, 2000; Lipp et al., 2008). The biomass in the open Pacific Ocean sediments, where primary photosynthetic production in the overlying water column is very small (e.g., South Pacific Gyre), is generally several orders of magnitude lower than that in the marginal sedimentary areas (D'Hondt et al., 2009). The current estimate of the global subseafloor microbial biomass is 2.9 × 1029 cells, comprising ca. 0.6% of the total carbon in living biomass on our planet (Kallmeyer et al., 2012). These facts mean that microbial communities in organic carbon-rich sediments in the margins of the Pacific Ocean contribute significantly to the global subseafloor microbial biomass.
In general, microbial activity in deep marine sediments, regardless of location, is extraordinarily low because of the limitation of bio-available electron donors and acceptors (D'Hondt et al., 2004; Røy et al., 2012; Hoehler and Jørgensen, 2013). However, there are metabolically active and/or alive microbial populations in deep marine sediments. Using nano-scale secondary ion-mass spectrometry (NanoSIMS), it has been demonstrated that a large fraction of deep subseafloor microbial components are physiologically alive, i.e., incorporate stable isotope-labeled carbon and nitrogen substrates (Morono et al., 2011). Successful extraction and sequencing of RNA and visualization of RNA by fluorescent in situ hybridization have also indicated the presence of metabolically active microbial components in deep marine sediments (Schippers et al., 2005; Sørensen and Teske, 2006; Mills et al., 2012; Orsi et al., 2013b).
In the deep subseafloor sedimentary biosphere, microbial communities comprise phylogenetically diverse bacteria and archaea (Inagaki et al., 2003, 2006; Teske, 2006; Fry et al., 2008), as well as eukaryotes (Orsi et al., 2013a) and viruses (Engelhardt et al., 2013; Yanagawa et al., 2013). Since the microbial constituents found in deep marine sediments are phylogenetically distinct from known isolates, their metabolic and physiological functions also remain largely unknown. In fact, the metagenomic analysis of the Peru Margin sediments showed that a large fraction (86–94%) of the sequences did not code any homologs of known protein-coding genes (Biddle et al., 2008), suggesting that microbial communities in marine subsurface are functionally and evolutionarily quite distinct from other microbial ecosystems of the Earth's surface biosphere (Biddle et al., 2006, 2011). A genomic study of single cells isolated from marine subsurface sediments has revealed extracellular protein-degrading enzymes, suggesting that previously unidentified metabolic functions related to protein degradation and recycling are present in the uncultured but predominant archaeal constituents in the marine subsurface sediments, such as members of the Miscellaneous Crenarchaeotic Group (Lloyd et al., 2013). In addition, stable isotope probing of the benthic microbial community showed that archaeal membrane lipids are recycled for new biomass production without energy-consuming lipid synthesis steps (Takano et al., 2010). These observations consistently suggest that deep subseafloor microbial constituents have unique metabolic and physiological functions that make them well suited for long-term survival under energy-limited conditions (Hoehler and Jørgensen, 2013).
One of the possible electron-acceptor systems in anoxic marine sediments is an organohalide respiration pathway (Bossert et al., 2003). Previous microbiological studies of various terrestrial environments and isolates demonstrated that the members of the genus Dehalococcoides are known to utilize organohalides as the sole terminal electron accepter by means of reductive dehalogenases (Löffler et al., 2003), in which reductive dehalogenase genes (rdhA) are key functional genes for organohalide respiration pathways (Bossert et al., 2003). In marine sedimentary habitats, culture-independent molecular ecological surveys of 16S rRNA genes showed that members of the phylum Chloroflexi, including Dehalococcoides-relatives, are the predominant bacterial components (e.g., Biddle et al., 2006; Inagaki et al., 2006; adrian, 2009). In addition, dehalogenation activities were observed in marine sediments (Häggblom et al., 2003), e.g., reductive dehalogenation of 2,4,6-tribromophenol was detected in sediment such as at 4.7 m below the seafloor in the Nankai Trough and somewhere else (Futagami et al., 2009, 2013). PCR-based analysis using a conventional primer set (i.e., RRF2 and B1R; Krajmalnik-Brown et al., 2004) revealed the widespread presence of phylogenetically diverse rdhA-homologous genes. However, because of potential bias caused by primer sequence mismatches (Teske and Sørensen, 2008; Hoshino and Inagaki, 2013), it is assumed that there are as-yet-unexplored functional genes related to the dehalogenation pathway in marine subsurface sediments.
In this study, we focused on the diversity, frequency and distribution of rdhA-homologs in organic carbon-rich marine subsurface sediments off the Shimokita Peninsula of Japan. The samples used in this study were obtained during the Chikyu Shakedown Expedition CK06-06 in 2006 (Aoike, 2007), in which relatively high cell numbers (>107 cells/cm3) were observed using an image-based cell count technique (Morono et al., 2009).
To obtain a comprehensive molecular overview of rdhA-homologous genes in marine subsurface sediments, we performed metagenomic analysis of sediment core samples at five depths to 107 m below the seafloor. This study allowed us to quantitatively assess the metagenomic pools through primer-independent phylogenetic analysis of rdhA-homologous genes, extending our current knowledge of the functional and evolutional contexts of deep subseafloor microbial communities.
Sediment samples used in this study were collected from Site C9001 Hole C (41° 10.6380′ N, 142° 12.081′ E), which is approximately 80 km from the coast of Shimokita Peninsula, northeastern Japan, during the Chikyu Shakedown Expedition CK06-06 in 2006 (Aoike, 2007). This site is at the same location of the Integrated Ocean Drilling Program (IODP) Expedition 337 hole designated as IODP Site C0020 Hole A (Inagaki et al., 2012). The water depth at Site C9001 is 1180.5 m. The sample depths examined in this metagenomic study are 0.8 (Core 1H-1), 5.1 (Core 1H-4), 18.6 (Core 3H-2), 48.5 (Core 6H-3), and 107.0 (Core 12H-4) mbsf. Environmental parameters such as pore-water chemistry and total organic carbon have been measured (Aoike, 2007; Tomaru et al., 2009). After core recovery onboard the vessel, 20-cm-long whole round scores were immediately sampled, and then the innermost part of the core was sub-sampled using an autoclaved tip-cut-syringe. The samples were placed in a −80°C freezer until DNA extraction.
DNA was extracted with an ISOIL for Bead Beating kit (Nippon Gene, Tokyo, Japan) according to manufacturer protocol, except for an additional treatment with lytic enzyme (Morita et al., 2007). Briefly, 5 g of sediment sample was suspended in 9 mL of five-fold diluted lytic buffer solution and vortexed vigorously. The suspension was transferred into a new 50 mL Falcon tube containing zirconia/silica beads and then shaken with a ShakeMaster Auto (ver. 2.0, Bio Medical Science Inc., Tokyo, Japan) for 5 min. After adding 0.5 mL of lysozyme solution (2.0 mg/ml) to the tube, the suspension was incubated for 1 h at 37°C with gentle shaking and further incubated for 1 h at 55°C after the addition of 0.5 mL of proteinase K solution (20 mg/ml) and 0.6 ml of lytic buffer 20S. The suspension treated by bead-beating and lytic enzymes was centrifuged at 6500 × g for 15 min at 4°C. DNA in the supernatant was purified twice according to manufacturer instructions. The DNA was further purified using a MagExtractor Plant Genome kit (Toyobo, Osaka, Japan). The concentration of the extracted DNA was measured using a Qubit fluorometer with a Quant-iT dsDNA HS Assay kit (Life Technologies, Carlsbad, CA, USA).
To increase amount of high-molecular DNA to construct shotgun genome libraries from marine subsurface sediments, it was found to be necessary that all DNA samples except for Core 1H-1 (0.8 mbsf) were amplified with multiple displacement amplification (MDA) using a GenomiPhi DNA Amplification kit (ver. 2, GE Healthcare, Uppsala, Sweden) according to manufacturer instructions (Lipp et al., 2008). To decrease potential amplification bias, we conducted multiple reactions (10–60 reactions per sample) with reaction time as short as 1.5 h, then the product was pooled, and used for sequencing analysis. The incubation was performed at 30°C after denaturing DNA at 95°C for 3 min. The Phi29 DNA polymerase and exonuclease were then inactivated by incubation at 65°C for 10 min.
Shotgun genomic libraries were constructed from extracted DNA that was randomly sheared (2–3 kb in length) using a HydroShear (Digilab Inc., Marlborough, MA, USA) and the pCR-BluntII-TOPO vector (Life Technologies). Approximately 10 μg of DNA was used to produce ca. 40,000 shotgun clones from each sediment sample. Template DNA for sequencing was prepared by PCR amplification of the insert DNA using an ExTaq kit (TaKaRa Bio Inc., Otsu, Japan) and a GeneAmp PCR System 9700 (Life Technologies). Sequencing was carried out from both ends of the cloned fragment using a BigDye v3.1 kit on ABI3730 (Life Technologies) and DeNOVA (Shimadzu Co., Kyoto, Japan) DNA sequencers.
The shotgun reads without 16S rRNA genes were filtered for low quality residues, so that the longest subsequence of a read is kept where Phred quality score is 20 or more for 80% in 20 bp window with sliding window size of 20 bp. The trimmed reads of each metagenomic sample were assembled to generate a consensus sequence for a contig based on an alignment of reads in the contig using the PCAP program (Huang et al., 2003) with default parameters.
The MetaGeneAnnotator program (Noguchi et al., 2008) was employed to predict potential protein-coding sequences (CDS ≥ 20 amino acids) from the assembled sequences. Prior to gene prediction, low-quality sequences (Phred quality score <15) were masked by “X”s. The results were described below and summarized in Table S1.
Assignment of metagenomic genes to orthologous gene groups was carried out using a pre-calculated ortholog table constructed from genomic sequences available from databases in the National Center for Biotechnology Information (NCBI; http://www.ncbi.nlm.nih.gov/). The table was constructed from 1806 available genome sequences without redundant representation of species (Table S2) using the DomClust program (Uchiyama, 2006). The metagenomic genes from the sediments were then assigned to the ortholog table using the MergeTree program in the RECOG package (http://mbgd.nibb.ac.jp/RECOG/), which is based on the clustering tree of each orthologous group in the table. Distance rather than score was used to construct the ortholog table and to merge the metagenomic genes into the table in order to ensure proper evaluation of evolutionary distance (Kawai et al., 2011). Distance values were calculated from identity values by a BLASTP (Altschul et al., 1997) search using Kimura's correction formula for protein sequence distances (Kimura, 1983). Homology pairs considered for calculation were unidirectional best hits with E ≤ 0.001 and score ≥60.0. Parameters used for the DomClust and MergeTree programs were as follows: -d (use distance as a measure of relatedness), −Ohorizweight = 0.0 (skip estimation of horizontal transfer), −C80.0 (cutoff score for domain split), −V0.6 (alignment coverage for domain split), −n1 (minimum number of organisms in clusters to be output), −ne1 (minimum number of entries in clusters to be output), −p0.5 (ratio of phylogenetic pattern overlap for tree cutting), −HO (both homology clustering, i.e., skip the tree cutting, and ortholog clustering), −ai0.95 (member overlap for absorbing adjacent small clusters), and −ao0.8 (member overlap for merging adjacent clusters).
To normalize the differences in the total number of sequences in each horizon, an “average coverage ratio” for each gene was defined as the ratio of the average coverage value of the gene to the average of average coverage values of 35 universally conserved single-copy genes.
Nucleotide sequence coverage of a gene in a metagenomic sequence (“average coverage” value in short) was defined as the average number of sequence reads mapped to each nucleotide position of the gene and the value is calculated as the total number of nucleotides that overlapped the gene divided by the length of the gene. The expected length of a gene in metagenomes is taken as the average length of the orthologous genes identified in the reference genomes based on the fact that metagenomic genes are largely truncated at the ends of sequence reads. Average coverage was based on clones rather than on individual reads; two reads from the same clone were counted as one if they overlapped with each other on a single contig.
Average coverage value is dependent on the sequencing depth of a metagenomic sample (i.e., the total amount of metagenomic sequences determined). Hence, the different sequencing depth among different samples (in our case, five different depths) must be normalized to compare the amount of genes among different metagenomes. To normalize the different sequencing depths among samples, we used average of the average coverage of universally conserved single-copy genes, which are conserved in almost all of the archaeal and bacterial genomes, to represent the estimated sequencing depth defined by the number of estimated genome equivalent. The 35 universally conserved single-copy genes used in this study (Table S3) were selected from the ortholog table based on the following criteria: (i) universally conserved among archaeal genomes, i.e., present in 95% of 110 complete archaeal genomes among 122 archaeal genomes used in this study which includes 12 incomplete genomes (Table S2), (ii) universally conserved among bacterial genomes, i.e., present in 95% of 1055 complete bacterial genomes among 1657 bacterial genomes used in this study, and (iii) present as a single-copy gene in most of the genomes, i.e., the average copy number among complete archaeal and bacterial genomes is less than 1.2 for both (Table S3). The average coverage ratio of the universally conserved single-copy genes for the five metagenomes was generally around one (Table S3).
Thus, the calculated average coverage ratio indicates the number of copies of a gene per a genome equivalent in the metagenomic sequences.
Metagenomic sequences from the Peru margin sediment samples (Biddle et al., 2008) [GenBank:SRR001322-SRR001326] were analyzed similarly as those of the present study. Low quality reads and duplicated reads were eliminated using PRINSEQ (Schmieder and Edwards, 2011). Comparison of reads and the reference genomes was carried out using TBLASTN (Altschul et al., 1997). Phylogenetic assignment of each read was based on the top hit among the rdhA genes detailed in the present study.
Statistical tests under hypergeometric distribution were conducted with Benjamini and Hochberg multi test correction to extract orthologous groups, in which the number of metagenomic genes assigned to the orthologous group was overrepresented compared to the number of reference genomes assigned to the group. In the test, the probability of deviation of the sample (the number of genes of the metagenomes) from the control (the number of genes of the reference genomes) was calculated based on the hypergeometric distribution, which is shown to be equivalent to the Fisher exact test (Rivals et al., 2007). If we randomly select k genes, where k equals the number of the metagenomic genes, from the all genes of the reference genomes and the metagenomic pools that are labeled as orthologous group X (m) or non-X (n), the distribution tells us the probability of getting q or more genes labeled as X. The sum of the average coverage of each gene (or that of a domain of the gene) of an orthologous group was rounded down to the nearest integer and used as the number of the metagenomic sample for the statistical test, while the number of genes assigned to the orthologous group was used for the reference genomes. Adjustment of p-values for multiple comparisons (multi-test correction) was performed using the Benjamini and Hochberg controlling procedure of false discovery rate (FDR) (Benjamini and Hochberg, 1995). All statistical analyses were conducted using the R statistical package (R Core Team, 2013).
Ribosomal rRNA genes were searched for SILVA ribosomal RNA gene database (version 111) (Quast et al., 2013) using BLASTN (Altschul et al., 1997) with E ≤ 0.001 and -dust no. Count of fragments was based on clones rather than on individual reads; two reads from the same clone were counted as one if they overlapped with each other. If a region of a sequence matched both large subunit ribosomal rRNA and small subunit ribosomal rRNA genes, higher score hit was used. An rRNA gene fragment was identified when it had equal or more than 100 bp homology with an rRNA gene. In addition, if the matched region is internal part of a sequence, the whole part of the hit should match. This case is a match between a query and a hit shorter than the query (e.g., archaeal PCR clones in the database can be less than approximately 1 kb). Taxonomy information was assigned to each 16S rRNA gene fragment using the mother program (Schloss et al., 2009). Coverage value was calculated as the ratio of the total length of 16S rRNA gene fragments to the length of the top hit 16S rRNA gene in the database.
A phylogenetic tree was constructed with the putative rdhA genes in the metagenomes and the reference genomes, as well as PCR products of the rdhA genes detected in subseafloor sediments (Futagami et al., 2009) and several functionally characterized reductive dehalogenase genes (Table S4). The rdhA-homologous sequences in the metagenomes were defined by two steps; (1) choosing orthologous groups of rdhA genes, and (2) refinement based on phylogenetic tree, as described below. Included orthologous groups were those of which half or more of the genes in the cluster have the RdhA domain [TIGR02486 model in TIGRFAMs database (Haft et al., 2003)]. Identification of RdhA domain (TIGR02486) was conducted using the Hmmer3 program (Eddy, 2011) against the TIGRFAMs (Haft et al., 2003) using the noise cutoff bit score thresholds (–cut_nc). Amino acid sequences of these gene products were aligned using the einsi command of the MAFFT program (Katoh et al., 2005). To construct phylogenetic trees from the protein alignment, because the rdhA homologous fragments from the metagenomes were not all overlapping each other, we used the FastTree program (Price et al., 2010). This program can generate a reasonable topology of an approximately-maximum-likelihood phylogenetic tree using an alignment with some of the sequences do not overlap each other. The default parameters of the program were used as follows: Jones-Taylor-Thornton model of amino acid evolution, a single rate for each site (the “CAT” approximation) to account for the varying rates of evolution across sites, and support values with the Shimodaira-Hasegawa test to estimate the reliability of each split in the tree (Price et al., 2010). Specifically, the FastTree uses the SH test to compare (A,B), (C,D) to alternate topologies (A,C), (B,D) or (A,D), (B,C), given a topology (A,B), (C,D), where A, B, C, D may be subtrees rather than leaves. It has been recommended that the selection threshold for SH value should be in the 0.8–0.9 range (Guindon et al., 2010). The result showed that SH 0.85 as a threshold of branch selection have similar power as bootstrap (BP) 0.75 in the sense that about 85% of correct branches are selected. The results of simulated data sets also validated the common practice to use “a rule of thumb,” whereby sufficient evidence is indicated by BP value above 0.7–0.8, discarding most of the incorrect branches (for details, see Guindon et al., 2010). At the end, SH values 0.8 and 0.9 were used in this study to indicate the support value of each split. A second phylogenetic tree was constructed after eliminating the genes from subtrees for which the RdhA domain (TIGR02486) was not detected in any of the genes. Orthologous groups that included dsrA and dsrB genes of Archaeoglobus fulgidus (Dahl et al., 1993) were defined as dsrA and dsrB, respectively.
Identification of the protein domain was conducted using the Hmmer3 program (Eddy, 2011) against the TIGRFAMs (Haft et al., 2003) and Pfam (Finn et al., 2010) databases using the noise cutoff bit score thresholds (–cut_nc). Protein families in TIGRFAMs are intended to detect orthologous proteins to provide information with the maximum utility for the annotation process (Haft et al., 2003). On the other hand, protein families in Pfam typically achieve broad coverage across distant homologs but end at the boundaries of conserved structural domains (Finn et al., 2010). Hence, we complementarily used these two well-maintained protein family databases.
rdhA genes characterized to date sometimes accompany an short rdhB–homologous gene, which encodes a hydrophobic protein (Smidt and de Vos, 2004). Gene sequences within 2 kb of the rdhA-homologous genes were searched for putative rdhB genes. Orthologous groups defined as putative rdhB in this study were those of which genes have predicted amino acid sequences shorter than 150 amino acids and two to three transmembrane regions. Initial screening with amino acid sequences shorter than 200 amino acids revealed that homologous in the ortholog table of the previously described rdhB genes were below 150 amino acids in length [Table S5; the previously described rdhB genes are as follows: pceB.Smu from Sulfurospirillum multivorans (Neumann et al., 1998), cprB.Dde from Desulfitobacterium dehalogenans (van de Pas et al., 1999; Smidt et al., 2000), vcrB.Dba from Dehalococcoidia bacterium (Müller et al., 2004), pceB.Dha from Desulfitobacterium hafniense (Futagami et al., 2006), dcaB.Ddi from Desulfitobacterium dichloroeliminans (Marzorati et al., 2007)]. Homologs of these rdhB genes were also listed, as some of flanking genes of putative rdhA genes derived from the metagenomes were truncated at the end of a nucleotide sequence and unable to check the exact length and the number of transmembrane regions. The transmembrane domains were predicted using the TMHMM Server 2.0 program (Krogh et al., 2001). TMHMM is based on a hidden Markov model (HMM) approach. HMM is well suited for prediction of transmembrane helices because it can incorporate hydrophobicity, charge bias, helix lengths, and grammatical constraints into one model.
The nucleotide sequence data have been submitted to the DDBJ database under the following accession numbers: DDBJ:BARS01000001-DDBJ:BARS01060478 for 1H-1, DDBJ:BART01000001-DDBJ:BART01044241 for 1H-4, DDBJ:BARU01000001-DDBJ:BARU01051978 for 3H-2, DDBJ:BARV01000001-DDBJ:BARV01048200 for 6H-3, and DDBJ:BARW01000001-DDBJ:BARW01045500 for 12H-4.
The samples examined in this study were obtained from 0.8 (Core 1H-1), 5.1 (Core 1H-4), 18.6 (Core 3H-2), 48.5 (Core 6H-3), and 107.0 (Core 12H-4) meters below the seafloor (mbsf) at Site C9001 Hole C, off Shimokita Peninsula of Japan, during the drilling vessel Chikyu Shakedown Expedition CK06-06 in 2006 (Aoike, 2007). The amount of DNA extracted from samples at each depth was as follows: 0.42 μg/g (0.8 mbsf), 0.24 μg/g (5.1 mbsf), 0.18 μg/g (18.6 mbsf), 0.05 μg/g (48.5 mbsf), and 0.03 μg/g (107.0 mbsf). The depth profile of cell numbers reported earlier (in the order of 108–109 cells/cm3 at a few mbsf to 107 cells/cm3 at 100 mbsf) (Morono et al., 2009) is consistent to the decreasing trend of the extracted DNA amount. The paired-end sequences of ca. 40,000 shotgun clones from each horizon were determined. The length of sequence reads ranged from 650 to 800 bp. The sum of sequence length of 76,000–77,000 reads was ca. 50 Mbp per each sample. The ratio of the total sequence length after assembly to that before assembly was 84 to 98% (Table S1). N50 of contigs was 1.5 to 1.7 kb (Table S1), suggesting that most contigs comprised two reads. Consensus sequences of the contigs were used in further analyses. The number of predicted genes in each sample was 86,362 (0.8 mbsf), 67,494 (5.1 mbsf), 73,184 (18.6 mbsf), 67,201 (48.5 mbsf), and 63,711 (107.0 mbsf) (Table S1). Sum of the nucleotide length of assembled nucleotide sequences that did not encode any genes homologous to those of reference genomes was less than 25% of the total assembled nucleotide sequences in length [16.9% (0.8 mbsf), 15.2% (5.1 mbsf), 17.2% (18.6 mbsf), 20.2% (48.5 mbsf), and 21.8% (107.0 mbsf)] (Table S1). The genes identified in the metagenomic sequences were then assigned to orthologous groups. The ortholog table and the merged table consisted of 666,560 and 748,988 orthologous groups, respectively. Orthologous groups that contained metagenomic genes were 129,471, among which 47,043 and 82,428 groups were with and without genes from the reference genomes, respectively.
All of the orthologous groups were plotted using two parameters, S-value (“S” stands for sum) and F-value (“F” stands for fold change) (Figure 1). S-value is the sum of the average coverage of genes of the five metagenomic pools assigned to an orthologous group, and F-value is a fold change of the estimated average copy number of metagenomic genes per genome (average coverage ratio) to the average copy number of genes of reference genomes per genome. The value of average coverage ratio, indicator of the relative copy number of the rdhA-homologous genes in the metagenomic samples, is calculated by dividing average coverage of rdhA by average of average coverage of the 35 genes (see section Calculation of Average Coverage Ratio). The large F-value of a gene indicates the number of the gene found in the metagenomic samples is more frequent than that expected from the distribution of the gene in the present reference genomes. Overrepresented genes in the metagenomic samples were identified using the test for hypergeometric distribution (see section Statistical Analysis), and the overrepresented orthologous groups are indicated in red on the plot (p = 10−4; false discovery rate-controlled adjusted p-value for multiple comparisons). It means the number of false positives under the p-value is 1 among 10,000. As 11,167 overrepresented groups were identified in total, only a very few would be false positives.
Figure 1. Comparison of metagenomic samples to previously sequenced genomes for overall orthologous groups. All groups of genes (orthologous groups) were plotted according to the number of genes assigned to an orthologous group in the metagenomes (S for sum) on the X-axis and the ratio of the frequency of metagenomic genes in an orthologous group to the frequency of genes of reference genomes in the orthologous group (F for fold change) on the Y-axis, with the size of the dot proportional to the number of orthologous groups with the same S-and F-values. The overrepresented orthologous groups are indicated in red (p < 1 × 10−4). The star in blue indicates the orthologous group of rdhA-homologous genes; the circle in magenta indicates the orthologous group of dsrA and dsrB; the diamond in yellow indicates the average of the 35 universally conserved single-copy genes.
Interestingly, the orthologous group of rdhA genes was one of the highly overrepresented groups in the metagenomic pools (p = 1.4 × 10−07; Figure 1). This overrepresentation of rdhA genes can be ascribed to two factors: the F-value (fold change) of rdhA is large and the S-value (the number of rdhA genes) is also considerably large. For comparison, the values of the universally conserved single-copy genes are plotted in Figure 1. The S-value of rdhA (15.3) was about the half of the average of the S-value of the universally conserved single-copy genes (30.4), while the F-value of rdhA (10.7) was larger than the average of the F-value of the universally conserved single-copy genes (by definition, 1). This discrepancy in the S- and F-values of rdhA relative to the universally conserved single-copy genes is ascribed to the fact that the frequency of the rdhA genes in the previously sequenced reference genomes was low (less than 0.04 copies per genome in 1806 reference genomes) compared to that in the metagenomic pools (0.5 copies per genome; average coverage ratio defined as the ratio of the S-value of rdhA to that of the universally conserved single-copy genes). Although the rdhA genes were overrepresented among other genes in the metagenomic pools, the average copy number, 0.5 copies per genome, is rather small, considering the fact that it is commonly observed among sequenced genomes that multiple rdhA genes are coded in one genome (Richardson, 2013).
Reductive dehalogenase genes have been reported from relatively limited taxa (Hug et al., 2013). In order to get additional information whether these metagenomic rdhA-homologous genes can be derived from known rdhA-harboring taxa, 16S rRNA gene fragments detected in the metagenomic pools (Table S1) were classified to taxonomy, allowing us to show the taxonomic distribution pattern (Figure 2). The taxonomic distribution of 16S rRNA gene fragments suggests that about 20–50% of microbial community was derived from phyla that include known rdhA-coding organisms (i.e., Chloroflexi and Proteobacteria) (Figure 2). Organisms of these phyla may code the observed rdhA-homologous genes, although we cannot exclude the possibility that organisms of other phyla, from which rdhA-coding cultured organisms have not been reported, code the rdhA-homologs.
Figure 2. Taxonomic distribution of 16S rRNA gene fragments. Plotted is frequency of 16S rRNA gene fragments in the metagenomes to each taxon.
Examination of the relative copy number of rdhA-homologous genes showed that the frequency of rdhA homologs is generally similar in metagenomic pools at five sediment depths (Figure 3A). The average coverage ratio indicates the relative copy number of rdhA-homologous genes. The value of average coverage ratio is calculated by dividing average coverage of rdhA by average of average coverage of the 35 universally conserved single-copy genes, as described in detail in section Calculation of Average Coverage Ratio. In this case, the average coverage of the rdhA genes at each horizon was 5.4 (0.8 mbsf), 2.0 (5.1 mbsf), 3.6 (18.6 mbsf), 2.5 (48.5 mbsf), and 4.6 (107.0 mbsf), while the average of the average coverage for the universally conserved single-copy genes was 8.0, 5.7, 7.3, 5.7, 3.8, respectively. Thus, the copy number of rdhA genes per genome (average coverage ratio), which is expected to be equivalent to the relative amount of the genes in one cell, was 0.68, 0.35, 0.50, 0.44, 1.2, respectively (Figure 3A).
Figure 3. Distribution of putative rdhA and dsrAB genes by sediment horizons. The average coverage ratio, or the ratio of the number of genes relative to universally conserved single-copy genes, is plotted against collection depth. (A) rdhA-homologous genes. (B) dsrAB genes.
To compare the gene frequency of rdhA-homologous genes to other functional genes as the reference, we studied dissimilatory sulfite reductase genes (dsrAB), key genes for microbial sulfate reduction. The orthologous groups of dsrA and dsrB were plotted in Figure 1. The concentration of sulfate in porewater was reported in Tomaru et al. (2009). The amount of dsrAB genes indicated by S-value is generally small in all samples, which are about one tenth of that of rdhA-homologous genes (Figure 3B). It was also found that the frequency of dsrA and dsrB is not always consistent each other.
A phylogenetic tree was constructed with the rdhA-homologous genes in the metagenomes and the public genomes. Sequences obtained from PCR-based molecular ecological surveys as well as several functionally characterized reductive dehalogenase genes were also included in the phylogenetic analysis (Figure 4; the genes used are listed in Table S4).
Figure 4. Diversity of rdhA-homologous genes in the sediment samples. Phylogenetic tree was constructed based on the multiple sequence amino-acid alignment of rdhA genes using the FastTree program. Genes included were those detected from the metagenomes of the sediment samples in this study, those detected from the reference genomes, those that are experimentally characterized and are derived from cloned nucleotide sequences, and PCR clones from sediment samples. The previously identified rdhA and rdhA-homologous genes in the figure are listed in Table S4. PCR clones of the putative rdhA genes from sediment samples were described previously (Futagami et al., 2009). These genes were assigned to clusters as indicated in the figure when the support value was ≥0.90, except for Ds3 (0.71), Smkt4 (0.88), miscellaneous (0.15), Smkt1 (0.32), Sh (0.88), Al1 (0.50) to which a cluster name was tentatively assigned. The abbreviation of the cluster names was named after a taxon of which most genes in a cluster belong to, as follows: Smkt, a metagenome from the Shimokita subseafloor sediments in this study; Al, class Alphaproteobacteria; De, Dehalobacter (phylum Firmicutes, class Clostridia); Dh, class Dehalococcoidia (phylum Chloroflexi); Ds, Desulfitobacterium (phylum Firmicutes, class Clostridia); Ep, class Epsilonproteobacteria; Ge, Geobacter (class Deltaproteobacteria); Sh, Shewanella (class Gammaproteobacteria). A number after the abbreviation denotes the ordinal number of clusters with the abbreviation. A cluster that includes one or more genes with experimental evidence/support is indicated by asterisk at the head and the representative gene name characterized in the parentheses. The references of experimentally characterized RdhA proteins are also listed in Table S4. Taxonomic classifications in the inset indicate phylum names followed by class names. Solid circles and open circles indicate support values of the branch for major splits in the range of 0.90–1.00 and 0.80–0.89, respectively.
It has been reported that rdhA genes of phylum Chloroflexi affiliate to a single large cluster or some distinct small clusters whereas those of phyla Firmicutes and Proteobacteria are scattered to affiliate within different clusters (Hug et al., 2013).
The tree revealed several clusters, of which some were newly identified, whereas the others correspond to known clusters or a few genes in the previous report. Notably most of the newly identified clusters (labeled Smkt1, Smkt2, Smkt3, and Smkt4 in Figure 4; Smkt, a metagenome from the Shimokita subseafloor sediments in this study; a number after the abbreviation denotes the ordinal number of clusters with the abbreviation) were solely composed of genes from the present metagenomes. These clusters are phylogenetically distinct from the clusters to which previously known genes are classified. On the other hand, the PCR products of rdhA genes from the subseafloor sediments all clustered with those from the genera Dehalococcoides and Dehalogenimonas of the class Dehalococcoidia likely due to the bias of PCR primers, which is somewhat consistent with the findings of a previous report (Futagami et al., 2009). On the phylogenetic tree, we identified genes that form a cluster (a subtree), whether they are metagenomic genes or not. For example, a cluster that does not include genes from the present metagenomes [labeled as Al2 in Figure 4; the abbreviation of the cluster name was named after the taxon (class Alphaproteobacteria) of which most genes in the cluster belong to; a number after the abbreviation denotes the ordinal number of clusters with the abbreviation] corresponds to that described recently (Chen et al., 2013) where bhbA gene is proven to be a reductive dehalogenase for brominated aromatic compounds.
It is clear from the phylogenetic tree that the number of rdhA genes within the new metagenomic cluster Smkt1 is larger than the number classified to the Dh/PCR cluster (only four genes for the latter were detected from the metagenomes (Figure 4); Dh, the class Dehalococcoidia (within the phylum Chloroflexi). The frequency of genes of the cluster Smkt1 was more or less similar at all five depths examined (Figure 5A). Other clusters were generally composed of fewer genes.
Figure 5. Distribution of rdhA-homologous genes at Site C9001 off Shimokita Peninsula and at Peru-margin. (A) Distribution of rdhA-homologous genes of clusters indicated in Figure 4 among the five horizons from different depths of the Shimokita metagenomes in the present study. The number is the average coverage ratio as in Figure 3. The order of clusters corresponds to that in Figure 4. The abbreviation of cluster names are defined in Figure 4 as follows: Smkt, a metagenome from the Shimokita subseafloor sediments in this study; Al, class Alphaproteobacteria; Dh, class Dehalococcoidia (phylum Chloroflexi); Ds, Desulfitobacterium (phylum Firmicutes, class Clostridia); Ep, class Epsilonproteobacteria; Sh, Shewanella (class Gammaproteobacteria). A number after the abbreviation denotes the ordinal number of clusters with the abbreviation. (B) Distribution of rdhA-homologous genes in the Peru-margin subseafloor metagenomes. Asterisk (*) denotes original, unamplified sample (Biddle et al., 2008). (C) Distribution of rdhA-homologous genes of clusters in the Peru-margin subseafloor metagenomes.
The rdhA genes in the same clusters were derived from both shallow and deep sediments (Smkt1, Smkt4, Dh/PCR, Sh, miscellaneous; Figure 5A; Sh, Shewanella the class Gammaproteobacteria; Ep, the class Epsilonproteobacteria). Even subclusters Smkt1.1 and Smkt1.2 included genes from both shallow and deep sediments (Figure 4).
Amino acid sequence motifs in the predicted products for rdhA genes were examined to further characterize metagenome-specific rdhA gene products (sequences within clusters Smkt1, Smkt2, Smkt3, Smkt4, and Smkt5). Experimentally characterized typical reductive dehalogenase sequence has an RdhA domain that consists of 2 × Fe-S binding motif and other conserved regions at the middle of the gene product [Figure 6A; denoted as FeS (FeS1 and FeS2) and C1 through C5, respectively] (Smidt and de Vos, 2004; Hug et al., 2013). Most RdhA sequences known so far also encode twin arginine motif (TAT motif) at the N-terminus (Smidt and de Vos, 2004). The lack of TAT signal in the rdhA homolog is reported in Anaeromyxobacter dehalogenans 2CP-C (Thomas et al., 2008) and Dehalogenimonas lykanthroporepellens BL-DC-9 (Siddaramappa et al., 2012).
Figure 6. Conserved regions of amino acids sequences of rdhA genes. (A) Regions conserved among experimentally characterized reductive dehalogenases. The members are indicated by their name and protein accession. A label in the parentheses indicates the cluster name as in Figures 4, 5. Amino acid residues are colored according to the similarity of their physico-chemical properties. Conserved regions and two Fe-S binding motifs are labeled as C1 through C5 and FeS (FeS1 and FeS2), respectively. Consensus residues are those conserved in ≥80% (10 or more among 13 enzymes); highlighted residues are those conserved in ≥60% (7 or more among 13). Experimental evidences have been reported for the following proteins: TceA.YP_180831.1 (Magnuson et al., 1998), VcrA.AAQ94119.1 (Müller et al., 2004), DcpA.AGS15112.1, CbrA.CAI82345.1 (Padilla-Crespo et al., 2014), CprA.AAD44542.1 (van de Pas et al., 1999; Smidt et al., 2000), PceA.CAD28790.2 (Maillard et al., 2003), PceA.AAC60788.1 (Neumann et al., 1998), Ssed_3769.YP_001475501.1 (Lohner and Spormann, 2013), CfrA.AFQ20272.1 (Tang and Edwards, 2013), BhbA.AFV28965.1 (Chen et al., 2013). Experimental support (transcription under organohalide compounds) has been reported for the following proteins: BvcA.AAT64888.1 (Krajmalnik-Brown et al., 2004). MbrA.ADF96893.1 (Chow et al., 2010), PrdA.BAE45338.1 (Tsukagoshi et al., 2006). (B) Residues of the rdhA-homologous genes in the sediment samples around the conserved regions defined in (A). The members are indicated by locus tag. A label in the parentheses indicates the cluster name as in Figures 4, 5. Double slash characters “//” denotes truncation at the end of the nucleotide sequence.
The Rdh domain was detected in metagenomic sequences of the present study (Table 1 and Figure 6B). Examination of multiple alignment revealed that two Fe-S cluster-binding motifs were found in those RdhA sequences as well as all the other conserved regions but C5 region in the clusters Smkt1 and Smkt5 (Figure 6B). Interestingly, however, genes of the cluster Smkt1 lacked twin arginine motif (TAT motif) (Table 1). Lack of a TAT signal sequence was confirmed by the N-terminal alignment of the gene products in the cluster Smkt1 to those from other clusters. As RdhA proteins are transported across the cytoplasmic membrane by action of the Tat transport system recognizing the TAT motif (Palmer and Berks, 2003), whether those rdhA-homologous genes lacking an apparent TAT motif are targeted to the membrane remains a further question. It does not necessarily mean that the product of those rdhA-homologous genes are not targeted to the membrane by the Tat transport system, because it is known that there are cases in which the physiological mechanism for targeting a protein to the Tat system is less obvious (Berks et al., 2005).
Most of the experimentally characterized rdhA genes are closely linked with a small open reading frame (designated rdhB) that codes for a hydrophobic membrane protein, typically about 100-amino acid hydrophobic protein (Smidt and de Vos, 2004), which is predicted to have three transmembrane α-helices (Maillard et al., 2003). It is conceivable that these helices anchor RdhA to the cytoplasmic membrane (Smidt and de Vos, 2004). Co-transcription of both genes has been shown for some cases such as pceAB (Neumann et al., 1998) and cprBA (Smidt et al., 2000), indicating a functional link of both gene products, although experimental characterization of association of these proteins is yet to be shown.
The examination of rdhB-homologous genes in the reference genomes of these experimentally supported rdhB homologs revealed that sequence similarity among them was mostly below the threshold used to construct the orthlog table in this study and they formed separate orthologous groups that consist of a single gene.
As an approach based only on sequence similarity turned out to be prone to miss rdhB–homologous genes, another approach based on gene-neighborhood was conducted to find rdhB homologs by introducing the following criteria: (1) genes within 2 kb of the rdhA-homologous genes, (2) genes with predicted amino acid sequences shorter than 150 amino acids, and (3) genes with predicted amino acid sequences of two to three transmembrane regions. The survey revealed that three rdhA-homologous genes in the metagenomes in this study had a gene that satisfied the above criteria, so was regarded as the rdhB-homologous genes (Tables 1, S5). We note that homologs of the genes that satisfied the criteria in the reference genomes were all flanked with a putative rdhA.
One of these three rdhB-homologous genes was adjacent to an rdhA of the cluster Smkt1 (Figure 4). The presence of rdhB homolog suggests that the function of the Smkt1 gene is similar to other rdhA-homologous genes, although frequency of the rdhB was apparently low as compared to that of the Smkt1 sequence. The other two rdhB-homologous genes from the metagenomes were adjacent to the rdhA gene of the cluster Dh/PCR. This result agrees well with the fact that rdhA genes from the reference genomes in this cluster are mostly adjacent to putative rdhB.
Other neighborhood genes, which are identified on the nucleotide sequences that code the metagenomic rdhA genes, did not code any genes related to mobile elements (transposases or phages) including integrases. It is worth noting that only very limited portion of the flanking region of the metagenomic rdhA homologs were sequenced (N50 was only 1.5–1.7 kb).
To compare the gene frequency of rdhA-homologous genes to geologically distinct location, we analyzed frequency of the rdhA homologs in metagenomic sequences from Peru margin (Biddle et al., 2008). It was analyzed similarly as above, although the Peru margin metagenomic sequence pools consist of relatively short sequence reads for the accurate assignment of orthologous groups. The analysis revealed that the frequency of rdhA-homologous genes in the Peru margin sediments was generally similar to that in the present Shimokita sediments (Figures 3, 5B). Distribution among different depths was rather even, as that in the present Shimokita sediments. Nevertheless, phylogenetic composition of the genes in Peruvian sediments was found to be slightly different from Shimokita, i.e., genes assigned to Smkt1 were fewer whereas those assigned to Smkt4 were higher (Figures 5A,C).
This study clearly demonstrated that the PCR-independent metagenomic approach is a powerful tool for characterizing diversity and distribution pattern of the target gene(s) from microbial communities in the deep subseafloor sedimentary biosphere (see, Biddle et al., 2008, 2011). Through the metagenomic approach, the wide range of previously undiscovered rdhA-like sequences could successfully be recovered, suggesting that reductive dehalogenase-related pathways were more genetically diverse than previously expected. It has also been evidenced that metagenomic pools from marine subsurface sediments contain novel and hence previously unidentified/uncharacterized rdhA-homologous genes, which were found to be more diverse than those detected by PCR-based molecular ecological survey (Futagami et al., 2009). The lack of a TAT motif in the sequences in new clusters can reasonably explain that no amplification of the new cluster genes occurs by PCR using a primer (RRF2) that covers the conserved TAT motif region (Krajmalnik-Brown et al., 2004).
The combined use of an ortholog table and statistical evaluation enabled quantitative assay of the target gene(s) (as an orthologous group) in metagenomic pools, revealing that rdhA-homologs are overrepresented among other genes. Further, the rdhA-homologous genes showed phylogenetically diverse and spatially distribute at five different depths from near the seafloor to a depth of 107.0 mbsf, at least 150,000-year-old sediment (Domitsu et al., 2010). The rdhA-homologous genes of the cluster Smkt1.1 and Smkt1.2 were consistently detected throughout the depths examined. The widespread occurrence of microorganisms that code rdhA-homologous genes in the Pacific margins is further supported by the comparison of the metagenomic pools of the sediments off Shimokita and off Peru. Taken together, our data indicate that the heterotrophic metabolic processes mediated by the rdhA-homologous genes can be ecologically important, functioning in the organic-rich subseafloor biosphere.
As anaerobic organohalide-respiring bacteria utilize organohalides as the electron acceptor through the enzymatic reaction of Rdh proteins (Smidt and de Vos, 2004), the high frequency of rdhA-homologous genes with relatively constant distribution in the sediment column suggests that there are still bio-available organohalides to sustain the organohalide respiring prokaryotes. The relatively constant value of total organic carbon at depths surrounding the 5 depths, around 1–2% [e.g., 2.3% (0.2 mbsf), 1.2% (18.0 mbsf), 1.3% (37.2 mbsf), 1.8% (56.3 mbsf), and 1.9% (113.2 mbsf)] (Aoike, 2007), does not contradict to this possibility. It is worth noting that the depositional environment thousands of years ago must predate the accumulation of anthropogenic organohalides; therefore, the subseafloor rdhA-homologs are not associated with polluted terrestrial environments. Possible sources of structurally diverse organohalides such as halogenated aromatic compounds and halogenated alkanes can be a benthic biological origin, such as hemichordates and marine sponges (King, 1986; Harper, 2000; Winterton, 2000; Öberg, 2002). One of other possible sources of naturally derived organohalides is the chlorinated degradation products of lignin, the most abundant aromatic substance on our planet (Boerjan et al., 2003). A not yet elucidated degradation process that is similar to lignin degradation could be taking place in seawater or in benthic depositional systems (i.e., transfer of chlorine from inorganic to organic forms). This could be similar to the process that produces massive amounts of organic carbon that are mineralized to refractory forms during depositional processes (Jiao et al., 2010). The rdhA-homologous genes were also detected in the open Pacific Ocean, far away from the continental margins (Futagami et al., 2009), suggesting the possibility of not yet elucidated mechanisms for the formation of organohalides in the seawater-sediment system.
In addition, we compared frequencies of rdhA homologs to dsrAB among different depths. Interestingly, the result showed that frequency of rdhA-homologous genes is generally much higher than that of dsrAB (Figures 3A,B). We observed either or both dsrA and dsrB genes in all the samples examined, which phenomenon is in good agreement with previous reports regarding the widespread occurrence of the genes, even in sulfate-depleted deep horizons (Schippers and Neretin, 2006). We have to note here that organohalide-respiring bacteria such as the members of Dehalococcoides code multiple copies of the rdhA-homologs while most of sulfate reducing bacteria do a single copy of the dsrAB genes. This indicates that remarkably high frequency of diverse rdhA-homologous does not necessarily correspond to the cell abundance of organohalide-respiring microbes.
Through metagenomic analysis of the deep sedimentary habitats, we found that remarkably diverse putative rdhA genes are spatially widespread in shallow to deep and old sedimentary habitats on the Pacific margins. We identified four new phylogenetic clusters of putative rdhA genes that are solely composed of genes detectable only from PCR-independent metagenomic analysis. These findings suggest that the deeply buried organohalide compounds may play ecologically significant roles in the subseafloor biogeochemical carbon cycle, and the anaerobic organohalide respiration is potentially one of the key metabolic energy-yielding mechanisms of the organic compound-fueled deep subseafloor microbial ecosystem.
However, the ecological consequences of organohalide degradation in the deep subseafloor biosphere still remain largely unknown: why are such phylogenetically diverse rdhA-like genes present? What kinds of organohalides exist and in what amounts? To what depths and at what levels do such organohalide-utilizing microbes occur? If all bio-available organohalide compounds are consumed, do these microbes disappear? What are the in situ dehalogenation activities and the impacts of biogeochemical halogen cycles? What are the possible electron donors for subseafloor organohalide respiration? These questions need to be addressed through advanced microbiological and biogeochemical analyses in the future. In addition, metabolic functioning of diverse rdhA-homologous genes as well as the gene expression and activity in situ still remains largely unknown, which should be clarified by culture-dependent and -independent molecular ecological studies at single cells to community levels. In addition, metagenomic analysis of deep subseafloor microbial communities is one of the technological challenges. In this study, except for a near surface sample, we performed multiple MDA reactions for the preparation of metagenomic libraries to reduce possible bias randomly occurred by MDA, which may still remain uncertainty for the accurate estimate and even coverage of the environmental gene pool. This means that development of molecular ecological technologies, such as high-sensitivity, high-throughput, less-bias molecular tools, should be necessary for the future deep-biosphere exploration.
The authors declare that the research was conducted in the absence of any commercial or financial relationships that could be construed as a potential conflict of interest.
The authors thank all the crews, members of the drilling team, shipboard scientists and technical staff members of the Chikyu Shakedown Expedition CK06-06. We also thank the members of Comparative Genomics Laboratory at NIG for technical and computational assistance. This research was supported in part by Grant-in-Aid for Scientific Research from the Japan Society for the Promotion of Science (JSPS) to Mikihiko Kawai (No. 21710209), Yuki Morono (No. 24651018) and Hideto Takami (No. 20310124). This work was also supported in part by the Japan Society for the Promotion of Science (JSPS) Strategic Fund for Strengthening Leading-Edge Research and Development (to JAMSTEC and Fumio Inagaki), the JSPS Funding Program for Next Generation World-Leading Researchers (NEXT Program, to Fumio Inagaki), the Institute for Bioinformatics Research and Development, the Japan Science and Technology Agency (to Ikuo Uchiyama and Hideto Takami), Collaborative research program of National Institute for Basic Biology (NIBB, to Hideto Takami and Ikuo Uchiyama). Computational resources were partly provided by the Data Integration and Analysis Facility, NIBB.
The Supplementary Material for this article can be found online at: http://www.frontiersin.org/journal/10.3389/fmicb.2014.00080/abstract
Table S1. Summary of sequencing characteristics.
Table S2. List of genomic sequences used for the construction of the ortholog table.
Table S3. Universally conserved single-copy genes in the ortholog table.
Table S4. List of rdhA and its homologs used in Figure 4.
Table S5. List of putative rdhB gene sequences.
Adrian, L. (2009). ERC-group microflex: microbiology of Dehalococcoides-like Chloroflexi. Rev. Environ. Sci. Biotechnol. 8, 225–229. doi: 10.1007/s11157-009-9166-y
Altschul, S. F., Madden, T. L., Schäffer, A. A., Zhang, J., Zhang, Z., Miller, W., et al. (1997). Gapped BLAST and PSI-BLAST: a new generation of protein database search programs. Nucleic Acids Res. 25, 3389–3402. doi: 10.1093/nar/25.17.3389
Aoike, K. (ed.). (2007). “CK06-06 D/V Chikyu shakedown cruise offshore Shimokita,” in Laboratory Operation Report (Yokohama: JAMSTEC-CDEX).
Benjamini, Y., and Hochberg, Y. (1995). Controlling the false discovery rate: a practical and powerful approach to multiple testing. J. R. Statist. Soc. B 57, 289–300. doi: 10.2307/2346101
Berks, B. C., Palmer, T., and Sargent, F. (2005). Protein targeting by the bacterial twin-arginine translocation (Tat) pathway. Curr. Opin. Microbiol. 8, 174–181. doi: 10.1016/j.mib.2005.02.010
Biddle, J. F., Fitz-Gibbon, S., Schuster, S. C., Brenchley, J. E., and House, C. H. (2008). Metagenomic signatures of the Peru Margin subseafloor biosphere show a genetically distinct environment. Proc. Natl. Acad. Sci. U.S.A. 105, 10583–10588. doi: 10.1073/pnas.0709942105
Biddle, J. F., Lipp, J. S., Lever, M. A., Lloyd, K. G., Sørensen, K. B., Anderson, R., et al. (2006). Heterotrophic Archaea dominate sedimentary subsurface ecosystems off Peru. Proc. Natl. Acad. Sci. U.S.A. 103, 3846–3851. doi: 10.1073/pnas.0600035103
Biddle, J. F., White, J. R., Teske, A. P., and House, C. H. (2011). Metagenomics of the subsurface Brazos-Trinity Basin (IODP site 1320): comparison with other sediment and pyrosequenced metagenomes. ISME J. 5, 1038–1047. doi: 10.1038/ismej.2010.199
Boerjan, W., Ralph, J., and Baucher, M. (2003). Lignin biosynthesis. Annu. Rev. Plant Biol. 54, 519–546. doi: 10.1146/annurev.arplant.54.031902.134938
Bossert, I. D., Häggblom, M. M., and Young, L. Y. (2003). “Microbial ecology of dehalogenation,” in Diversity of Dechlorinating Bacteria. Dehalogenation, Microbial Processes and Environmental Applications, eds M. M. Häggblom and I. D. Bossert (Norwell, MA: Kluwer Academic Publisher Group), 33–52.
Chen, K., Huang, L., Xu, C., Liu, X., He, J., Zinder, S. H., et al. (2013). Molecular characterization of the enzymes involved in the degradation of a brominated aromatic herbicide. Mol. Microbiol. 89, 1121–1139. doi: 10.1111/mmi.12332
Chow, W. L., Cheng, D., Wang, S., and He, J. (2010). Identification and transcriptional analysis of trans-DCE-producing reductive dehalogenases in Dehalococcoides species. ISME J. 4, 1020–1030. doi: 10.1038/ismej.2010.27
D'Hondt, S., Jørgensen, B. B., Miller, D. J., Batzke, A., Blake, R., Cragg, B. A., et al. (2004). Distributions of microbial activities in deep subseafloor sediments. Science 306, 2216–2221. doi: 10.1126/science.1101155
D'Hondt, S., Spivack, A. J., Pockalny, R., Ferdelman, T. G., Fischer, J. P., Kallmeyer, J., et al. (2009). Subseafloor sedimentary life in the South Pacific Gyre. Proc. Natl. Acad. Sci. U.S.A. 106, 11651–11656. doi: 10.1073/pnas.0811793106
Dahl, C., Kredich, N. M., Deutzmann, R., and Trüper, H. G. (1993). Dissimilatory sulphite reductase from Archaeoglobus fulgidus: physico-chemical properties of the enzyme and cloning, sequencing and analysis of the reductase genes. J. Gen. Microbiol. 139, 1817–1828. doi: 10.1099/00221287-139-8-1817
Domitsu, H., Nishi, H., Uchida, J., Oda, M., Ogane, K., Taira, A., et al. (2010). Age model of core sediments taken by D/V Chikyu during the shakedown cruises off Shimokita Peninsula. Fossils 87, 47–64. Available online at: http://www.palaeo-soc-japan.jp/Japanese/fossil.html
Eddy, S. R. (2011). Accelerated profile HMM searches. PLoS Comput. Biol. 7:e1002195. doi: 10.1371/journal.pcbi.1002195
Engelhardt, T., Sahlberg, M., Cypionka, H., and Engelen, B. (2013). Biogeography of Rhizobium radiobacter and distribution of associated temperate phages in deep subseafloor sediments. ISME J. 7, 199–209. doi: 10.1038/ismej.2012.92
Finn, R. D., Mistry, J., Tate, J., Coggill, P., Heger, A., Pollington, J. E., et al. (2010). The Pfam protein families database. Nucleic Acids Res. 38, D211–D222. doi: 10.1093/nar/gkp985
Fry, J. C., Parkes, R. J., Cragg, B. A., Weightman, A. J., and Webster, G. (2008). Prokaryotic biodiversity and activity in the deep subseafloor biosphere. FEMS Microbiol. Ecol. 66, 181–196. doi: 10.1111/j.1574-6941.2008.00566.x
Futagami, T., Morono, Y., Terada, T., Kaksonen, A. H., and Inagaki, F. (2009). Dehalogenation activities and distribution of reductive dehalogenase homologous genes in marine subsurface sediments. Appl. Environ. Microbiol. 75, 6905–6909. doi: 10.1128/AEM.01124-09
Futagami, T., Morono, Y., Terada, T., Kaksonen, A. H., and Inagaki, F. (2013). Distribution of dehalogenation activity in subseafloor sediments of the Nankai Trough subduction zone. Philos. Trans. R. Soc. Lond,. B Biol. Sci. 368:20120249. doi: 10.1098/rstb.2012.0249
Futagami, T., Yamaguchi, T., Nakayama, S., Goto, M., and Furukawa, K. (2006). Effects of chloromethanes on growth of and deletion of the pce gene cluster in dehalorespiring Desulfitobacterium hafniense strain Y51. Appl. Environ. Microbiol. 72, 5998–6003. doi: 10.1128/AEM.00979-06
Guindon, S., Dufayard, J. F., Lefort, V., Anisimova, M., Hordijk, W., and Gascuel, O. (2010). New algorithms and methods to estimate maximum-likelihood phylogenies: assessing the performance of PhyML 3.0. Syst. Biol. 59, 307–321. doi: 10.1093/sysbio/syq010
Haft, D. H., Selengut, J. D., and White, O. (2003). The TIGRFAMs database of protein families. Nucleic Acids Res. 31, 371–373. doi: 10.1093/nar/gkg128
Häggblom, M. M., Ahn, Y. B., Fennell, D. E., Kerkhof, L. J., and Rhee, S. K. (2003). Anaerobic dehalogenation of organohalide contaminants in the marine environment. Adv. Appl. Microbiol. 53, 61–84. doi: 10.1016/S0065-2164(03)53002-7
Harper, D. B. (2000). The global chloromethane cycle: biosynthesis, biodegradation and metabolic role. Nat. Prod. Rep. 17, 337–348. doi: 10.1039/A809400D
Hoehler, T. M., and Jørgensen, B. B. (2013). Microbial life under extreme energy limitation. Nat. Rev. Microbiol. 11, 83–94. doi: 10.1038/nrmicro2939
Hoshino, T., and Inagaki, F. (2013). A comparative study of microbial diversity and community structure in marine sediments using poly(A) tailing and reverse transcription PCR. Front. Microbiol. 4:160. doi: 10.3389/fmicb.2013.00160
Huang, X., Wang, J., Aluru, S., Yang, S. P., and Hillier, L. (2003). PCAP: a whole-genome assembly program. Genome Res. 13, 2164–2170. doi: 10.1101/gr.1390403
Hug, L. A., Maphosa, F., Leys, D., Löffler, F. E., Smidt, H., Edwards, E. A., et al. (2013). Overview of organohalide-respiring bacteria and a proposal for a classification system for reductive dehalogenases. Philos. Trans. R. Soc. Lond. B Biol. Sci. 368:20120322. doi: 10.1098/rstb.2012.0322
Inagaki, F., Hinrichs, K. U., Kubo, Y., and the Expedition 337 Scientists. (2012). “Deep coalbed biosphere off Shimokita: microbial processes and hydrocarbon system associated with deeply buried coalbed in the ocean,” in IODP Preliminary Report (Tokyo: IODP Management International), 337. doi: 10.2204/iodp.pr.337.2012
Inagaki, F., Nunoura, T., Nakagawa, S., Teske, A., Lever, M., Lauer, A., et al. (2006). Biogeographical distribution and diversity of microbes in methane hydrate-bearing deep marine sediments on the Pacific Ocean Margin. Proc. Natl. Acad. Sci. U.S.A. 103, 2815–2820. doi: 10.1073/pnas.0511033103
Inagaki, F., Suzuki, M., Takai, K., Oida, H., Sakamoto, T., Aoki, K., et al. (2003). Microbial communities associated with geological horizons in coastal subseafloor sediments from the Sea of Okhotsk. Appl. Environ. Microbiol. 69, 7224–7235. doi: 10.1128/AEM.69.12.7224-7235.2003
Jiao, N., Herndl, G. J., Hansell, D. A., Benner, R., Kattner, G., Wilhelm, S. W., et al. (2010). Microbial production of recalcitrant dissolved organic matter: long-term carbon storage in the global ocean. Nat. Rev. Microbiol. 8, 593–599. doi: 10.1038/nrmicro2386
Kallmeyer, J., Pockalny, R., Adhikari, R. R., Smith, D. C., and D'Hondt, S. (2012). Global distribution of microbial abundance and biomass in subseafloor sediment. Proc. Natl. Acad. Sci. U.S.A. 109, 16213–16216. doi: 10.1073/pnas.1203849109
Katoh, K., Kuma, K., Toh, H., and Miyata, T. (2005). MAFFT version 5: improvement in accuracy of multiple sequence alignment. Nucleic Acids Res. 33, 511–518. doi: 10.1093/nar/gki198
Kawai, M., Furuta, Y., Yahara, K., Tsuru, T., Oshima, K., Handa, N., et al. (2011). Evolution in an oncogenic bacterial species with extreme genome plasticity: Helicobacter pylori East Asian genomes. BMC Microbiol. 11:104. doi: 10.1186/1471-2180-11-104
Kimura, M. (1983). The Neutral Theory of Molecular Evolution. Cambridge: Cambridge University Press.
King, G. M. (1986). Inhibition of microbial activity in marine sediments by a bromophenol from a hemichordate. Nature 323, 257–259. doi: 10.1038/323257a0
Krajmalnik-Brown, R., Hölscher, T., Thomson, I. N., Saunders, F. M., Ritalahti, K. M., and Löffler, F. E. (2004). Genetic identification of a putative vinyl chloride reductase in Dehalococcoides sp. strain BAV1. Appl. Environ. Microbiol. 70, 6347–6351. doi: 10.1128/AEM.70.10.6347-6351.2004
Krogh, A., Larsson, B., von Heijne, G., and Sonnhammer, E. L. (2001). Predicting transmembrane protein topology with a hidden Markov model: application to complete genomes. J. Mol. Biol. 305, 567–580. doi: 10.1006/jmbi.2000.4315
Lipp, J. S., Morono, Y., Inagaki, F., and Hinrichs, K. U. (2008). Significant contribution of Archaea to extant biomass in marine subsurface sediments. Nature 454, 991–994. doi: 10.1038/nature07174
Lloyd, K. G., Schreiber, L., Petersen, D. G., Kjeldsen, K. U., Lever, M. A., Steen, A. D., et al. (2013). Predominant archaea in marine sediments degrade detrital proteins. Nature 496, 215–218. doi: 10.1038/nature12033
Löffler, F. E., Cole, J. R., Ritalahti, K. M., and Tiedje, J. M. (2003). “Diversity of dechlorinating Bacteria,” in Diversity of Dechlorinating Bacteria. Dehalogenation, Microbial Processes and Environmental Applications, eds M. M. Häggblom and I. D. Bossert (Norwell, MA: Kluwer Academic Publisher Group), 53–87.
Lohner, S. T., and Spormann, A. M. (2013). Identification of a reductive tetrachloroethene dehalogenase in Shewanella sediminis. Philos. Trans. R. Soc. Lond. B Biol. Sci. 368:20120326. doi: 10.1098/rstb.2012.0326
Magnuson, J. K., Stern, R. V., Gossett, J. M., Zinder, S. H., and Burris, D. R. (1998). Reductive dechlorination of tetrachloroethene to ethene by a two-component enzyme pathway. Appl. Environ. Microbiol. 64, 1270–1275.
Maillard, J., Schumacher, W., Vazquez, F., Regeard, C., Hagen, W. R., and Holliger, C. (2003). Characterization of the corrinoid iron-sulfur protein tetrachloroethene reductive dehalogenase of Dehalobacter restrictus. Appl. Environ. Microbiol. 69, 4628–4638. doi: 10.1128/AEM.69.8.4628-4638.2003
Marzorati, M., de Ferra, F., Van Raemdonck, H., Borin, S., Allifranchini, E., Carpani, G., et al. (2007). A novel reductive dehalogenase, identified in a contaminated groundwater enrichment culture and in Desulfitobacterium dichloroeliminans strain DCA1, is linked to dehalogenation of 1,2-dichloroethane. Appl. Environ. Microbiol. 73, 2990–2999. doi: 10.1128/AEM.02748-06
Mills, H. J., Reese, B. K., Shepard, A. K., Riedinger, N., Dowd, S. E., Morono, Y., et al. (2012). Characterization of metabolically active bacterial populations in subseafloor Nankai Trough sediments above, within, and below the sulfate-methane transition zone. Front. Microbiol. 3:113. doi: 10.3389/fmicb.2012.00113
Morita, H., Kuwahara, T., Ohshima, K., Sasamoto, H., Itoh, K., Hattori, M., et al. (2007). An improved DNA isolation method for metagenomic analysis of the microbial flora of the human intestine. Microbes Environ. 22, 214–222. doi: 10.1264/jsme2.22.214
Morono, Y., Terada, T., Masui, N., and Inagaki, F. (2009). Discriminative detection and enumeration of microbial life in marine subsurface sediments. ISME J. 3, 503–511. doi: 10.1038/ismej.2009.1
Morono, Y., Terada, T., Nishizawa, M., Ito, M., Hillion, F., Takahata, N., et al. (2011). Carbon and nitrogen assimilation in deep subseafloor microbial cells. Proc. Natl. Acad. Sci. U.S.A. 108, 18295–18300. doi: 10.1073/pnas.1107763108
Müller, J. A., Rosner, B. M., Von Abendroth, G., Meshulam-Simon, G., McCarty, P. L., and Spormann, A. M. (2004). Molecular identification of the catabolic vinyl chloride reductase from Dehalococcoides sp. strain VS and its environmental distribution. Appl. Environ. Microbiol. 70, 4880–4888. doi: 10.1128/AEM.70.8.4880-4888.2004
Neumann, A., Wohlfarth, G., and Diekert, G. (1998). Tetrachloroethene dehalogenase from Dehalospirillum multivorans: cloning, sequencing of the encoding genes, and expression of the pceA gene in Escherichia coli. J. Bacteriol. 180, 4140–4145.
Noguchi, H., Taniguchi, T., and Itoh, T. (2008). MetaGeneAnnotator: detecting species-specific patterns of ribosomal binding site for precise gene prediction in anonymous prokaryotic and phage genomes. DNA Res. 15, 387–396. doi: 10.1093/dnares/dsn027
Öberg, G. (2002). The natural chlorine cycle—fitting the scattered pieces. Appl. Microbiol. Biotechnol. 58, 565–581. doi: 10.1007/s00253-001-0895-2
Orsi, W., Biddle, J. F., and Edgcomb, V. (2013a). Deep sequencing of subseafloor eukaryotic rRNA reveals active Fungi across marine subsurface provinces. PLoS ONE 8:e56335. doi: 10.1371/journal.pone.0056335
Orsi, W. D., Edgcomb, V. P., Christman, G. D., and Biddle, J. F. (2013b). Gene expression in the deep biosphere. Nature 499, 205–208. doi: 10.1038/nature12230
Padilla-Crespo, E., Yan, J., Swift, C., Wagner, D. D., Chourey, K., Hettich, R. L., et al. (2014). Identification and environmental distribution of dcpA encoding the 1,2-dichloropropane-to-propene reductive dehalogenase in organohalide-respiring Chloroflexi. Appl. Environ. Microbiol. 80, 808–818. doi: 10.1128/AEM.02927-13
Palmer, T., and Berks, B. C. (2003). Moving folded proteins across the bacterial cell membrane. Microbiology 149, 547–556. doi: 10.1099/mic.0.25900-0
Parkes, R. J., Cragg, B. A., Bale, S. J., Getlifff, J. M., Goodman, K., Rochelle, P. A., et al. (1994). Deep bacterial biosphere in Pacific Ocean sediments. Nature 371, 410–413. doi: 10.1038/371410a0
Parkes, R. J., Cragg, B. A., and Wellsbury, P. (2000). Recent studies on bacterial populations and processes in subseafloor sediments: a review. Hydrogeol. J. 8, 11–28. doi: 10.1007/pl00010971
Price, M. N., Dehal, P. S., and Arkin, A. P. (2010). FastTree 2-approximately maximum-likelihood trees for large alignments. PLoS ONE 5:e9490. doi: 10.1371/journal.pone.0009490
Quast, C., Pruesse, E., Yilmaz, P., Gerken, J., Schweer, T., Yarza, P., et al. (2013). The SILVA ribosomal RNA gene database project: improved data processing and web-based tools. Nucleic Acids Res. 41, D590–D596. doi: 10.1093/nar/gks1219
R Core Team (2013). R: A Language and Environment for Statistical Computing. Vienna: R Foundation for Statistical Computing.
Richardson, R. E. (2013). Genomic insights into organohalide respiration. Curr. Opin. Biotechnol. 24, 498–505. doi: 10.1016/j.copbio.2013.02.014
Rivals, I., Personnaz, L., Taing, L., and Potier, M. C. (2007). Enrichment or depletion of a GO category within a class of genes: which test? Bioinformatics 23, 401–407. doi: 10.1093/bioinformatics/btl633
Røy, H., Kallmeyer, J., Adhikari, R. R., Pockalny, R., Jørgensen, B. B., and D'Hondt, S. (2012). Aerobic microbial respiration in 86-million-year-old deep-sea red clay. Science 336, 922–925. doi: 10.1126/science.1219424
Schippers, A., and Neretin, L. N. (2006). Quantification of microbial communities in near-surface and deeply buried marine sediments on the Peru continental margin using real-time PCR. Environ. Microbiol. 8, 1251–1260. doi: 10.1111/j.1462-2920.2006.01019.x
Schippers, A., Neretin, L. N., Kallmeyer, J., Ferdelman, T. G., Cragg, B. A., Parkes, R. J., et al. (2005). Prokaryotic cells of the deep sub-seafloor biosphere identified as living bacteria. Nature 433, 861–864. doi: 10.1038/nature03302
Schloss, P. D., Westcott, S. L., Ryabin, T., Hall, J. R., Hartmann, M., Hollister, E. B., et al. (2009). Introducing mothur: open-source, platform-independent, community-supported software for describing and comparing microbial communities. Appl. Environ. Microbiol. 75, 7537–7541. doi: 10.1128/AEM.01541-09
Schmieder, R., and Edwards, R. (2011). Quality control and preprocessing of metagenomic datasets. Bioinformatics 27, 863–864. doi: 10.1093/bioinformatics/btr026
Siddaramappa, S., Challacombe, J. F., Delano, S. F., Green, L. D., Daligault, H., Bruce, D., et al. (2012). Complete genome sequence of Dehalogenimonas lykanthroporepellens type strain (BL-DC-9(T)) and comparison to “Dehalococcoides” strains. Stand. Genomic Sci. 6, 251–264. doi: 10.4056/sigs.2806097
Smidt, H., and de Vos, W. M. (2004). Anaerobic microbial dehalogenation. Annu. Rev. Microbiol. 58, 43–73. doi: 10.1146/annurev.micro.58.030603.123600
Smidt, H., van Leest, M., van der Oost, J., and de Vos, W. M. (2000). Transcriptional regulation of the cpr gene cluster in ortho-chlorophenol-respiring Desulfitobacterium dehalogenans. J. Bacteriol. 182, 5683–5691. doi: 10.1128/JB.182.20.5683-5691.2000
Sørensen, K. B., and Teske, A. (2006). Stratified communities of active Archaea in deep marine subsurface sediments. Appl. Environ. Microbiol. 72, 4596–4603. doi: 10.1128/AEM.00562-06
Takano, Y., Chikaraishi, Y., Ogawa, N. O., Nomaki, H., Morono, Y., Inagaki, F., et al. (2010). Sedimentary membrane lipids recycled by deep-sea benthic archaea. Nat. Geosci. 3, 858–861. doi: 10.1038/ngeo983
Tang, S., and Edwards, E. A. (2013). Identification of Dehalobacter reductive dehalogenases that catalyse dechlorination of chloroform, 1,1,1-trichloroethane and 1,1-dichloroethane. Philos. Trans. R. Soc. Lond. B Biol. Sci. 368:20120318. doi: 10.1098/rstb.2012.0318
Teske, A. P. (2006). Microbial communities of deep marine subsurface sediments: molecular and cultivation surveys. Geomicrobiol. J. 23, 357–368. doi: 10.1080/01490450600875613
Teske, A., and Sørensen, K. B. (2008). Uncultured archaea in deep marine subsurface sediments: have we caught them all? ISME J. 2, 3–18. doi: 10.1038/ismej.2007.90
Thomas, S. H., Wagner, R. D., Arakaki, A. K., Skolnick, J., Kirby, J. R., Shimkets, L. J., et al. (2008). The mosaic genome of Anaeromyxobacter dehalogenans strain 2CP-C suggests an aerobic common ancestor to the delta-proteobacteria. PLoS ONE 3:e2103. doi: 10.1371/journal.pone.0002103
Tomaru, H., Fehn, U., Lu, Z., Takeuchi, R., Inagaki, F., Imachi, H., et al. (2009). Dating of dissolved Iodine in pore waters from the gas hydrate occurrence offshore Shimokita Peninsula, Japan: 129I results from the D/V Chikyu shakedown cruise. Resour. Geol. 59, 359–373. doi: 10.1111/j.1751-3928.2009.00103.x
Tsukagoshi, N., Ezaki, S., Uenaka, T., Suzuki, N., and Kurane, R. (2006). Isolation and transcriptional analysis of novel tetrachloroethene reductive dehalogenase gene from Desulfitobacterium sp. strain KBC1. Appl. Microbiol. Biotechnol. 69, 543–553. doi: 10.1007/s00253-005-0022-x
Uchiyama, I. (2006). Hierarchical clustering algorithm for comprehensive orthologous-domain classification in multiple genomes. Nucleic Acids Res. 34, 647–658. doi: 10.1093/nar/gkj448
van de Pas, B. A., Smidt, H., Hagen, W. R., van der Oost, J., Schraa, G., Stams, A. J., et al. (1999). Purification and molecular characterization of ortho-chlorophenol reductive dehalogenase, a key enzyme of halorespiration in Desulfitobacterium dehalogenans. J. Biol. Chem. 274, 20287–20292. doi: 10.1074/jbc.274.29.20287
Winterton, N. (2000). Chlorine: the only green element—towards a wider acceptance of its role in natural cycles. Green Chem. 2, 173–225. doi: 10.1039/b003394O
Keywords: deep biosphere, dehalogenation, metagenome, rdhA, sedimentary habitat
Citation: Kawai M, Futagami T, Toyoda A, Takaki Y, Nishi S, Hori S, Arai W, Tsubouchi T, Morono Y, Uchiyama I, Ito T, Fujiyama A, Inagaki F and Takami H (2014) High frequency of phylogenetically diverse reductive dehalogenase-homologous genes in deep subseafloor sedimentary metagenomes. Front. Microbiol. 5:80. doi: 10.3389/fmicb.2014.00080
Received: 10 December 2013; Accepted: 13 February 2014;
Published online: 03 March 2014.
Edited by:
Jennifer F. Biddle, University of Delaware, USAReviewed by:
William D. Orsi, Woods Hole Oceanographic Institution, USACopyright © 2014 Kawai, Futagami, Toyoda, Takaki, Nishi, Hori, Arai, Tsubouchi, Morono, Uchiyama, Ito, Fujiyama, Inagaki and Takami. This is an open-access article distributed under the terms of the Creative Commons Attribution License (CC BY). The use, distribution or reproduction in other forums is permitted, provided the original author(s) or licensor are credited and that the original publication in this journal is cited, in accordance with accepted academic practice. No use, distribution or reproduction is permitted which does not comply with these terms.
*Correspondence: Hideto Takami, Microbial Genome Research Group, Extremobiosphere Research Program, Institute of Biogeosciences, Japan Agency for Marine-Earth Science and Technology, Natsushima 2-15, Yokosuka, Kanagawa, 237-0061, Japan e-mail:dGFrYW1paEBqYW1zdGVjLmdvLmpw
Disclaimer: All claims expressed in this article are solely those of the authors and do not necessarily represent those of their affiliated organizations, or those of the publisher, the editors and the reviewers. Any product that may be evaluated in this article or claim that may be made by its manufacturer is not guaranteed or endorsed by the publisher.
Research integrity at Frontiers
Learn more about the work of our research integrity team to safeguard the quality of each article we publish.