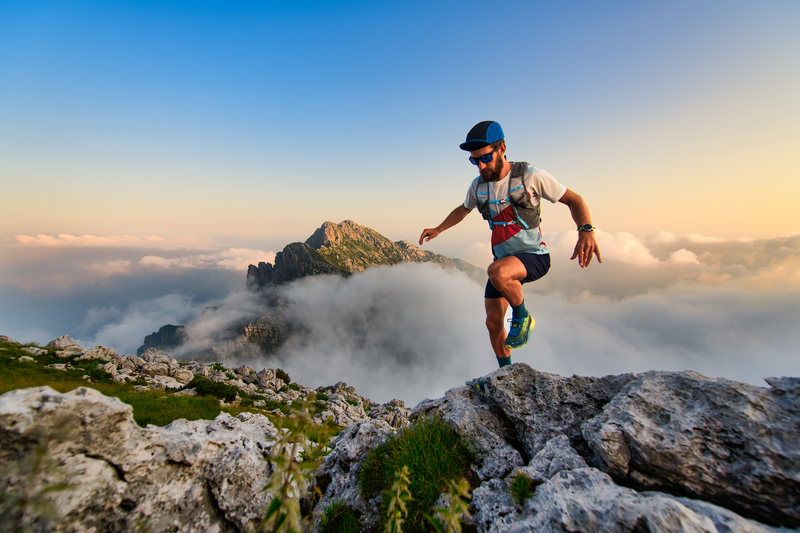
94% of researchers rate our articles as excellent or good
Learn more about the work of our research integrity team to safeguard the quality of each article we publish.
Find out more
REVIEW article
Front. Microbiol. , 07 June 2013
Sec. Antimicrobials, Resistance and Chemotherapy
Volume 4 - 2013 | https://doi.org/10.3389/fmicb.2013.00145
This article is part of the Research Topic Mechanisms of antibiotic resistance View all 24 articles
Rates of infection with antibiotic-resistant bacteria have increased precipitously over the past several decades, with far-reaching healthcare and societal costs. Recent evidence has established a link between antibiotic resistance genes in human pathogens and those found in non-pathogenic, commensal, and environmental organisms, prompting deeper investigation of natural and human-associated reservoirs of antibiotic resistance. Functional metagenomic selections, in which shotgun-cloned DNA fragments are selected for their ability to confer survival to an indicator host, have been increasingly applied to the characterization of many antibiotic resistance reservoirs. These experiments have demonstrated that antibiotic resistance genes are highly diverse and widely distributed, many times bearing little to no similarity to known sequences. Through unbiased selections for survival to antibiotic exposure, functional metagenomics can improve annotations by reducing the discovery of false-positive resistance and by allowing for the identification of previously unrecognizable resistance genes. In this review, we summarize the novel resistance functions uncovered using functional metagenomic investigations of natural and human-impacted resistance reservoirs. Examples of novel antibiotic resistance genes include those highly divergent from known sequences, those for which sequence is entirely unable to predict resistance function, bifunctional resistance genes, and those with unconventional, atypical resistance mechanisms. Overcoming antibiotic resistance in the clinic will require a better understanding of existing resistance reservoirs and the dissemination networks that govern horizontal gene exchange, informing best practices to limit the spread of resistance-conferring genes to human pathogens.
It is estimated that infection with antibiotic-resistant pathogens incurs over $25 billion in societal and healthcare costs annually in the United States (CDC, 2011). More than 70% of hospital-acquired bacterial infections are currently resistant to at least one of the major antibiotics used as standard treatment (Stone, 2009), and patient deaths as a result of hospital-acquired infections have increased by over 675% during the past 20 years (NIAID, 2012). As the incidence and spectrum of antibiotic resistance increases (Arias and Murray, 2009), antibiotic development has slowed to a trickle (Spellberg et al., 2008), further hindering the effectiveness of antibiotics to treat infectious disease. The majority of acquired antibiotic resistance genes in bacterial pathogens are obtained via horizontal gene transfer (HGT) (Ochman et al., 2000), likely with environmental origins (Benveniste and Davies, 1973; Wright, 2010; D'Costa et al., 2011). Accordingly, an increasing impetus has been placed on cataloging antibiotic resistance reservoirs (Allen et al., 2009b, 2010), determining how resistance genes are most readily transferred to the clinic (Marshall and Levy, 2011; Smillie et al., 2011), and identifying resistance mechanisms heretofore unseen in clinical settings (Jeon et al., 2011; Tao et al., 2012).
An antibiotic-resistant phenotype can be either an acquired trait or intrinsic to the bacterium in question. In the case of intrinsic resistance, antibiotic therapy is rendered ineffective due to a pre-existing physiological trait of the species, such as reduced accessibility to or absence of a drug target (Alekshun and Levy, 2007; Martinez, 2008; Davies and Davies, 2010; Dantas and Sommer, 2012). Examples of intrinsic antibiotic resistance include vancomycin tolerance in Gram-negative bacteria (the outer cell membrane reduces access to the peptidoglycan target) (Arthur and Courvalin, 1993) and biofilm formation in numerous organisms (perhaps providing resistance via reduced drug penetration and/or altered microenvironments) (Stewart and Costerton, 2001). Conversely, acquired antibiotic resistance stems from the expression of a specific resistance gene and is commonly the result of de novo mutation or the acquisition of resistance-conferring genes on mobile genetic elements (e.g., plasmids, transposons, integrons) (Walsh, 2003). The antibiotic resistance genes present in a microbial community that are capable of transfer to a new host are collectively referred to as the “transferable resistome.” Intrinsic resistance is, by definition, limited to the context of the parent organism, whereas acquired resistance represents a more flexible phenotype, and its prevalence is more immediately responsive to selection pressure (Martinez, 2008). As nearly all infectious bacteria were antibiotic-susceptible prior to the introduction of antibiotic therapy (Houndt and Ochman, 2000; Davies and Davies, 2010), the exceeding majority of resistance in human pathogens is acquired, either through mutation or HGT (Alekshun and Levy, 2007). This resistance represents a diversity of biochemical mechanisms that break down into three general categories (Walsh, 2000, 2003): (1) inactivation of the antibiotic, (2) reducing intracellular antibiotic concentration through efflux or permeability barriers, and (3) altering the cellular target of the antibiotic, reducing their association.
Perhaps the most intuitive of resistance mechanisms, antibiotic inactivation, is sub-categorized into two groups: enzymes that inactivate drugs via degradation (e.g., the β-lactamases) vs. those that function via chemical modification. The β-lactamases are characterized by their ability to cleave the four-membered ring present in all β-lactam antibiotics and are some of the best-studied and widely-distributed antibiotic resistance genes (for review, see Jacoby and Munoz-Price, 2005). These enzymes confer high-level antibiotic resistance and are found associated with mobile DNA elements and integrated into bacterial chromosomes. β-lactamases function via either a serine active site or metal cation cofactor (Jacoby and Munoz-Price, 2005) and can be found across bacterial phyla. Antibiotic-modifying enzymes are also phylogenetically widespread, as well as mechanistically diverse. These enzymes can confer tolerance toward numerous drugs, including the aminoglycoside (Davies and Wright, 1997), tetracycline (Yang et al., 2004), amphenicol (Schwarz et al., 2004), and macrolide-lincosamide-streptogramin (Weisblum, 1998) antibiotics, typically functioning via covalent modification of the drug with some functional moiety (e.g., acetyl, phosphoryl, nucleotidyl, glycosyl, and hydroxyl groups) (Alekshun and Levy, 2007).
The intracellular concentration of any given antibiotic can be reduced by either efflux mechanisms to remove the drug from the cytosol or permeability barriers that limit the drug's uptake. Many antibiotics have poor activity against Gram-negative pathogens due to efflux systems (Levy, 1992), most notably the RND superfamily transporters (Li and Nikaido, 2004, 2009). Other major families of efflux systems include the MFS, SMR, and ABC superfamily transporters, which are present in both Gram-negative and -positive organisms (Li and Nikaido, 2004, 2009). Although commonly chromosomal, many efflux systems are found on plasmids and other mobile elements and can confer drug-specific, class-specific, or multidrug resistance (Poole, 2005). Some permeability barriers, such as the Gram-negative outer membrane (Arthur and Courvalin, 1993), represent intrinsic antibiotic resistance, while in other instances, permeability barriers are acquired. Examples include multidrug-resistance via the altered expression of Gram-negative porin proteins (e.g., OmpF in Escherichia coli and OprD in Pseudomonas) (Delcour, 2009) and glycopeptide resistance due to thickened Gram-positive cell walls (Cui et al., 2006).
Antibiotic resistance via cellular target modification often occurs via chromosomal mutation and represents a common means by which the fluoroquinolone, sulfonamide, and trimethroprim antibiotics, among others, are tolerated (Alekshun and Levy, 2007). In the case of the fluoroquinolones, mutations to a variety of residues within the quinolone-resistance-determining-region (QRDR) of the DNA gyrase GyrA or topoisomerase IV ParC/GrlA prevent the interaction of the synthetic antibiotic with its target, facilitating resistance (Hooper, 1999). Importantly, resistance to fluoroquinolones via mutation is typically recessive, suppressing the acquisition of a resistant phenotype in the presence of wild-type GyrA or ParC/GrlA and thus preventing widespread horizontal dissemination of resistant gene variants (Wolfson and Hooper, 1989; Soussy et al., 1993). However, a plasmid-borne GyrA protection protein, Qnr, has been discovered that confers low-level fluoroquinolone resistance (Tran and Jacoby, 2002) and can potentiate the incidence of QRDR mutations, which combined provide high levels of resistance (Jacoby, 2005). Both the sulfonamides and trimethroprim competitively inhibit enzymes within the folate biosynthesis pathway: mutations to the dihydropteroate synthase and dihydrofolate reductase (DHFR) enzymes can reduce affinity for sulfonamides and trimethroprim, respectively, and provide tolerance to the antibiotics (Huovinen et al., 1995). In addition to arising de novo, both sulfonamide- and trimethroprim-resistant enzymes are present on mobile DNA elements, providing resistance to numerous bacteria via HGT (Alekshun and Levy, 2007). Other highly mobile mechanisms of target-modification include vancomycin resistance via the modification of peptidoglycan precursors (Bugg et al., 1991), aminoglycoside resistance via methylation of the 16S rRNA subunit (Galimand et al., 2003), and macrolide resistance from 23S rRNA methylases (Zhanel et al., 2001).
Research on antibiotic resistance over the past 70 years has focused on traditionally pathogenic bacteria isolated in a clinical setting and the role of antibiotic resistance genes already present in those species (Sommer et al., 2009; Davies and Davies, 2010). The resistance phenotype, however, is an ancient function of environmental bacteria (D'Costa et al., 2011), despite being largely absent from human pathogens prior to the antibiotic age (Hughes and Datta, 1983; Houndt and Ochman, 2000), with estimates that β-lactamases have existed for over 2 billion years (Hall and Barlow, 2004; Hall et al., 2004). Moreover, diverse mechanisms of antibiotic resistance have been discovered in nearly all environments (D'Costa et al., 2006; Allen et al., 2010; Davies and Davies, 2010; Wright, 2010); seemingly each new metagenomic study uncovers numerous examples of resistance genes previously unreported in public databases (Allen et al., 2009b; Sommer et al., 2009; Donato et al., 2010; Forsberg et al., 2012). In short, the diversity and abundance of antibiotic resistance in commensal microbiota and environmental settings dwarfs that which is seen in the context of human pathogens.
Importantly, environmental antibiotic resistance is not only widespread, but also represents the likely origins of the resistance seen in human pathogens. It has been known for 40 years that environmental bacteria share the same resistance mechanisms as those seen in pathogens (Benveniste and Davies, 1973), with documented examples of environmental resistance genes moving from natural settings into human pathogens (Poirel et al., 2002, 2005). It is becoming increasingly evident that human pathogens and environmental organisms share antibiotic resistance genes; a recent study described seven resistance genes from non-pathogenic soil organisms, conferring tolerance to five antibiotic classes, with perfect identity to genes from phylogenetically and geographically diverse pathogens (Forsberg et al., 2012). Given the staggering diversity of environmental resistance, high adaptability of bacteria, and strong selection pressure for antibiotic resistance, the question of antibiotic resistance is “not a matter of if but only a matter of when” (Walsh, 2000).
To understand when novel resistance will appear in human pathogens and perhaps diminish its impact, one must understand how new resistance genes are most frequently acquired by pathogenic bacteria. Since the answer is, most commonly, via HGT (Hughes and Datta, 1983; Ochman et al., 2000; Alekshun and Levy, 2007), understanding the complement of resistance genes most likely to be transferred to pathogens is crucial to predicting resistance acquisition. Although cataloging the repertoire of resistance genes on Earth remains a prohibitively large undertaking, techniques for interrogating the resistance properties of complex microbial communities exist and are being applied toward the identification of diverse and novel resistance from numerous settings. Importantly, these studies are focused not only on environmental locales, but also on the resistomes associated with human and animal microbiota (Shoemaker et al., 2001; Sommer et al., 2010b; Sommer and Dantas, 2011). Although antibiotic resistance may have its origins in the environment (Benveniste and Davies, 1973; Wright, 2010; D'Costa et al., 2011), the commensal resistome shares many resistance genes with both pathogens (Sommer et al., 2010b) and environmental organisms (Forsberg et al., 2012) and represents a likely route through which these populations exchange resistance genes (Smillie et al., 2011; Sommer and Dantas, 2011). Focused efforts to understand how pathogens acquire antibiotic resistance will require a greater appreciation for the diversity of resistance genes and in which environments and under what conditions these resistance genes are most accessible to human pathogens.
Traditionally, either culture-based (D'Costa et al., 2006) or PCR-based approaches (Perez-Perez and Hanson, 2002) have been used to study antibiotic resistance properties from microbial communities. While both techniques have led to major discoveries (Galan et al., 2013), both have inherent limitations that have contributed to an under-sampling of resistance genes from diverse microbial habitats. The majority of bacteria remain recalcitrant to culturing (Daniel, 2005) and are therefore not interrogated when culture-dependent techniques are employed. Additionally, linking a resistance phenotype to a causal genotype is a time-consuming process, often necessitating experimental scope to be limited to a small number of organisms, rather than whole communities. PCR screens are an effective means to identify or quantify resistance genes of known sequence, circumventing the need for culture (Knapp et al., 2010), but are only able to detect previously described genes and often require expression cloning and subsequent experimentation to verify function. In addition, annotation of antibiotic resistance genes in shotgun-sequenced microbial communities has proven challenging, as homology-based functional gene comparisons often fail due to low sequence similarity to previously discovered resistance genes, and in silico analyses are unable to confirm resistance function.
In contrast with standard techniques, functional metagenomics is a culture- and sequence-independent means of identifying transferrable antibiotic resistance in complex metagenomes. This method (Figure 1) involves shotgun-cloning total community DNA into an expression vector and transforming the library into an indicator host (commonly the model organism E. coli). The resulting transformants are then selected for the desired function (e.g., antibiotic resistance), and metagenomic DNA fragments are sequenced and annotated to identify causal survival-conferring genes (Allen et al., 2009b; Sommer et al., 2009). Functional metagenomics offers three classical advantages for the unbiased interrogation of complex resistomes (Daniel, 2005; Sommer and Dantas, 2011): (1) no need to culture organisms, (2) no required knowledge of resistance gene sequence, and (3) direct association between a genotype and a demonstrated resistance phenotype. Additionally, functional metagenomic selections specifically identify those genes within a metagenome capable of conferring antibiotic tolerance to the indicator host when expressed exogenously (i.e., they distinguish transferrable resistance from intrinsic resistance) (Dantas and Sommer, 2012). Recent improvements to the throughput of functional metagenomics (Forsberg et al., 2012) unlock the potential for the experiments of scale needed identify the specific sequences, and environments, most readily able to confer resistance to human pathogens, frequently represented by the opportunistic pathogen E. coli.
Figure 1. Overview of functional metagenomic selections. Total metagenomic DNA is extracted from a microbial community sample, sheared, and ligated into an expression vector (Step 1) and is subsequently transformed into a suitable library host (Step 2) to create a metagenomic library. The library is then plated on media containing antibiotics inhibitory to the wild-type host (Step 3) to select for metagenomic fragments conferring antibiotic resistance. Metagenomic fragments present in colonies growing on antibiotic selection media are then PCR-amplified (Step 4) and sequenced using either traditional Sanger sequencing or next-generation sequencing methods (Step 5). Finally, reads are assembled and annotated in order to identify the causative antibiotic resistance genes (Step 6).
The following sections review novel resistance mechanisms and genes uncovered by functional metagenomic selections; these studies are summarized in Table 1.
PCR-based screens for known resistance genes are able to detect novel variants with minor sequence differences, but due to the requirement for conserved primer binding sites, they are often unable to detect genes that have diverged significantly from the canonical example. In comparison, functional metagenomic selections for antibiotic resistance frequently identify genes that are less than 65% identical at the amino acid level to known resistance genes. In this way, functional metagenomics expands our knowledge of what sequence variants are tolerated in a resistance gene while preserving the resistance mechanism, as well as what mutations lead to expanded specificity profiles.
β-lactamases are one of the largest and best studied classes of resistance determinants, and yet, novel β-lactamases are regularly uncovered with sequence-independent techniques (Allen et al., 2009b; Sommer et al., 2009; Donato et al., 2010; Cheng et al., 2012; Forsberg et al., 2012). In the first functional metagenomic selection for antibiotic resistance in the human gut microbiome, Sommer et al. identified ten novel β-lactamase families whose eleven members were only 35–61% identical to known genes (Sommer et al., 2009). Based on phylogenetic analysis with PhyloPythia, (McHardy et al., 2007) these genes originated primarily from the Firmicutes and Bacteroidetes phyla, which comprise the majority of the human gut microbiota but have been undersampled because they are less readily culturable in aerobic environments than Proteobacteria. Functional selection for β-lactam resistance in metagenomic libraries constructed from remote Alaskan soil revealed 13 novel β-lactamases of all four Ambler classes that were ≤67% identical to any known, functionally characterized gene (Allen et al., 2009b). In the case of the class B β-lactamases, several genes appear to be more closely related to the ancestral gene of that class than to clinically isolated genes, providing context for the evolution of clinical isolates. The study also confirmed that overall sequence similarity does not necessarily confer similar drug susceptibility profiles, supporting discovery techniques that confirm the function of identified genes.
Functional metagenomic selections also enable the detection of highly diverse classes of resistance genes, defined by substrate specificity more than shared sequence identity. For instance, aminoglycoside 6′-N-acetyltransferases [AAC(6′)s] are difficult to identify via PCR because of their high sequence diversity (Riesenfeld et al., 2004). Sommer et al. identified six AAC(6′)s with less than 48% amino acid identity to known genes and a methyltransferase conferring resistance to sisomycin with only 26.3% shared identity. Riesenfeld et al. and other studies have identified many aminoglycoside resistance genes less than 70% identical to previously reported sequences (Riesenfeld et al., 2004; Donato et al., 2010; Torres-Cortes et al., 2011; Cheng et al., 2012; McGarvey et al., 2012). Similarly, two novel bleomycin resistance genes were isolated from an activated sludge metagenomic library (Mori et al., 2008). Bleomycin resistance proteins act primarily by sequestering the antibiotic through electrostatic interactions, so their sequences tolerate an extreme amount of divergence while still maintaining the resistance function. Nevertheless, the study confirmed that all known bleomycin resistance proteins contain a proline near the N-terminus and have an acidic pI of <5, improving computational annotation of this class. Finally, McGarvey et al. identified an ADP-ribosyltransferase that was much longer any previously characterized using functional metagenomic selections (McGarvey et al., 2012). This full-length gene may not have been identified by PCR-based screens, but its discovery, enabled by functional metagenomics, expands our knowledge of the sequence diversity tolerated within this resistance class.
A major limitation of sequence-based metagenomics is its restriction to antibiotic resistance genes that are recognizable as members of a previously characterized class. BLAST, the most commonly used annotation tool, requires a threshold of shared sequence identity for a gene to be considered a member of an established gene class. Therefore, by definition, this method limits the ability to identify novel resistance genes. In addition, while profile hidden Markov model (HMM)-based annotation provides a sensitive, statistically sound analysis method capable of identifying remote homologs, these models still rely on underlying multiple sequences alignments of previously characterized proteins. In addition, current profile HMM databases provide high-level classification of antibiotic resistance genes (e.g., “Beta-Lactamase,” rather than “TEM Beta-Lactamase”), providing little functional information about the gene and its potential resistance profile. Therefore, while these techniques have the potential to identify genes that have low sequence identity to known resistance genes, in the absence of additional functional confirmation, they cannot identify novel resistance mechanisms or verify that sequence variants are functional.
Functional metagenomics identifies resistance-conferring elements without prior knowledge of the sequence, circumventing this limitation. For instance, in a selection for tetracycline resistance encoded by the human oral metagenome, Diaz-Torres et al. discovered a novel tetracycline resistance gene, tet(37) (Diaz-Torres et al., 2003). In vitro analysis indicated that tet(37) inactivates tetracycline, making it one of only three tetracycline resistance proteins to utilize this mechanism (Thaker et al., 2010). tet(37) resembles flavoproteins, oxidoreductases, and NAD(P)-requiring enzymes in sequence and conserved motifs, but has no identity to tet(X), the first tetracycline-inactivating gene identified. It is therefore unlikely that computational annotation would have identified it as a resistance gene (Diaz-Torres et al., 2003).
Similarly, a study of soil microbiota identified a dihydrofolate-reducing gene, Tm8-3, that resembles 3-oxoacyl-(acyl-carrier-protein) reductases but not dhfr, the target of trimethoprim (Torres-Cortes et al., 2011). A screen of an activated sludge metagenome also identified six resistance genes that appear to inactivate chloramphenicol through enzymatic modification, but which share no significant identity with known chloramphenicol acetyltransferases (Parsley et al., 2010). Although these genes may become important for clinical resistance in the future, their existence would likely have been overlooked without high-throughput functional selections independent of previous sequence knowledge.
Drug efflux is a widespread resistance mechanism common to many antibiotic classes, but individual transporters identified through computational annotation methods cannot be assigned antibiotic efflux properties without functional validation. Small changes in protein structure can change the drug specificity profile of an efflux pump and confer or eliminate resistance. Therefore, functional metagenomic selections are an attractive alternative for high-throughput characterization of transporters with tentative resistance annotations (Torres-Cortes et al., 2011). For instance, in their study of soil isolate metagenomes, Forsberg et al. identified a novel gene with only low identity to a drug/metabolite transporter. Were it not for functional selection of D-cycloserine resistance, this putative transporter would never have been identified as an antibiotic resistance gene (Forsberg et al., 2012). McGarvey et al. identified five novel MFS transporters and two ABC transporters from an urban soil that conferred resistance to tetracycline, chloramphenicol, or trimethoprim (McGarvey et al., 2012). Lang et al. successfully identified pexA, a novel amphenicol MFS transporter, despite its low identity (33%) to other drug resistance transporters, including any known chloramphenicol exporters (Lang et al., 2010).
Broad-spectrum resistance as a result of bifunctional resistance genes, the fusion of two complementary enzymatic functions into a single gene, is recognized as an increasing occurrence in many pathogens (Kim et al., 2006; Perez et al., 2007; Chandrakanth et al., 2008). Functional metagenomics provides an opportunity to identify fusion genes that are functionally active against multiple classes of antibiotic agents from natural resistance reservoirs and commensal organisms, and which have the potential to appear in clinically relevant bacteria.
Recently, functional metagenomic selections of a remote Alaskan soil revealed a novel bifunctional β-lactamase (Allen et al., 2009b). The 609-amino-acid protein is nearly double the length of a typical β-lactamase and is a natural fusion of genes for two different β-lactamase subclasses. The C-terminal domain (356 residues) aligns with class C β-lactamases, while the N-terminal domain (253 residues) aligns with class D β-lactamases, each contributing to the resistance profile of the full-length gene. The class C homologue confers resistance to cephalexin, while the class D homologue is responsible for resistance to amoxicillin, ampicillin, and carbenicillin. The bifunctional fusion gene therefore expands the resistance profile of the full-length gene beyond what either domain is responsible for alone (Allen et al., 2009b).
While this was the first bifunctional β-lactamase to be discovered, bifunctional resistance has been documented in the past, particularly against the aminoglycoside antibiotics (Daigle et al., 1999; Centrón and Roy, 2002; Dubois et al., 2002; Mendes et al., 2004; Robicsek et al., 2006). The most common mechanism of aminoglycoside resistance is deactivation of the molecule through modification by cytoplasmic enzymes: aminoglycoside acetyltransferases (AACs), aminoglycoside phosphotransferases (APHs), or aminoglycoside nucleotidyltransferases (ANTs). Four bifunctional enzymes, combining two complementary aminoglycoside-modifying enzymes as separate domains into a single open reading frame, significantly broadening the resistance profile, are known. Ferretti et al. reported the first bifunctional enzyme, AAC(6′)/APH(2″), demonstrating that it is capable of both acetylation (ACC activity found in N-terminal domain) and phosphorylation (APH activity found in C-terminal domain) of aminoglycosides (Ferretti et al., 1986). This bifunctional enzyme was also discovered in a functional metagenomics study of the human gut microbiota (Cheng et al., 2012). The ability to doubly modify aminoglycosides enables this enzyme to confer resistance to nearly all clinically relevant aminoglycosides except streptomycin and spectinomycin (Daigle et al., 1999). Similarly, the bifunctional resistance enzymes characterized as an AAC(3)-Ib/AAC(6′)-Ib′ (Dubois et al., 2002), an ANT(3″)-Ii/AAC(6′)-IId (Centrón and Roy, 2002), and an AAC(6′)-30/AAC(6′)-Ib′ (Mendes et al., 2004) all exhibit an expanded resistance profile by combining two different aminoglycoside-modifying enzymes into a single gene.
AAC(6′)-Ib-cr is the only bifunctional resistance enzyme identified capable of conferring resistance to two different structural classes of antibiotics, aminoglycosides and fluoroquinolones (Vetting et al., 2008). A variant of a common aminoglycoside acetyltransferase AAC(6′)-Ib, AAC(6′)-Ib-cr is also capable of N-acetylation of fluoroquinolones. Only two codon changes in the original enzyme are responsible for the fluoroquinolone-resistance phenotype (Vetting et al., 2008).
The merger of two genes with complementary enzymatic activities represents a novel mechanism for overcoming the increasing challenge by antibiotics, resulting in extremely broad resistance to entire antibiotic structural classes. It is likely that more examples of bifunctional resistance exist in the environment, and functional metagenomics provides a powerful technique for the discovery of functionally active bifunctional enzymes that may have great clinical implications.
Functional metagenomic selections have the potential to identify another important class of resistance genes, those that have a primary role in the cell other than antibiotic resistance. This includes housekeeping genes whose overexpression provides resistance, general stress response transcription factors, and enzymes with promiscuous activity.
Selections for trimethoprim resistance, for example, frequently identify genes encoding DHFR, the cellular target of trimethoprim (Torres-Cortes et al., 2011; McGarvey et al., 2012). Cloning a dhfr homolog into a high copy-number plasmid results in overexpression of DHFR, which sequesters the antibiotic (Flensburg and Skold, 1987). McGarvey et al. identified 19 highly divergent DHFRs from a soil metagenome. The proteins shared only 7–44% amino acid identity in pairwise alignments, but a subset selected for further investigation conferred similar levels of trimethoprim resistance on the host when cloned into the same position of a high-copy number vector (McGarvey et al., 2012). Although clinical isolates of E. coli that massively overproduce DHFR as a result of promoter mutations and intrinsically resistant dhfr homologs have been identified (Flensburg and Skold, 1987), in most instances, resistance conferred by overexpression of a heterologous gene is likely independent of its ability to replace the host homolog. Rather, the introduced gene may sequester enough of the antibiotic to allow the native homolog to continue functioning. Selections for D-cycloserine resistance that identify D-alanine-D-alanine ligase, the target of the antibiotic, likely fall under the same class (Cheng et al., 2012). Creating and screening libraries in low-copy number vectors is essential in these instances to identify genes that are naturally resistant to the antibiotic, rather than simply selecting for overexpression.
In contrast, antibiotic resistance conferred by transcriptional regulators represents the successful interaction of a heterologous gene with existing host cellular pathways. The AraC transcriptional stress response regulators marA, soxS, and rob share a highly overlapping regulon and mediate low-level antibiotic resistance in E. coli and its close relative E. fergusonii (Alekshun and Levy, 1997; Martin and Rosner, 2002). As might be expected, these genes are often identified in functional metagenomic selections against chloramphenicol, tetracyclines, and other antibiotics when E. coli is the indicator host. A homolog of the AraC transcriptional regulator ramA, from Enterobacter sp., was also identified from a gypsy moth larvae gut isolate metagenomic library as conferring resistance to E. coli against multiple antibiotics of different classes when overexpressed (Allen et al., 2009a). These results provide valuable information about the ability of horizontally acquired genes from closely related phyla to incorporate into a cell at the regulatory level, a mechanism of antibiotic resistance that has been previously under-considered.
Certain genes identified by functional metagenomic selections are surprising in that they more closely resemble protein families that perform unrelated functions in the cell than previously identified resistance genes. They may represent instances of expanded substrate specificity, where mutation to a gene with an unrelated function confers resistance. By first screening a soil metagenomic library for esterase activity, Jeon et al. identified a family VIII carboxylesterase that hydrolyzes both esters and the amide bond of β-lactams, apparently utilizing the same catalytic site residues for both reactions (Jeon et al., 2011). The protein changes that enable it to also hydrolyze β-lactams are currently unidentified. A novel chloramphenicol acetate esterase, EstDL136, isolated from a soil metagenome, hydrolyzes the amide linkage of both chloramphenicol and its synthetic derivative florfenicol (Tao, 2011; Tao et al., 2012). Many of the surrounding genes in the metagenomic fragment are most closely related to Sphingomonadaceae, which are frequently considered for bioremediation for their ability to degrade many compounds (Stolz, 2009). EstDL136 may have originally evolved to detoxify another compound, later expanding its substrate specificity to chloramphenicol.
Functional metagenomic selections have proven to be an excellent technique for the discovery of novel antibiotic resistance mechanisms and genes encoded by varied environmental and human-associated microbial communities. As library sizes continue to increase, diverse indicator hosts are established, and techniques for improved functional selection and mechanistic determination are developed, functional metagenomics will only become better powered to explore the transferable resistome of complex microbial communities.
All of the metagenomic libraries discussed above were constructed in E. coli. The commercial availability of competent E. coli with extremely high transformation efficiencies has made this organism the preferred host for library construction, and these studies show that E. coli permits the heterologous expression of genes from many phyla. However, there are many clinically important classes of antibiotics that are intrinsically inactive against this Gram-negative species (e.g., glycopeptides, macrolides, oxazolidinones), prohibiting functional metagenomic selections in this host from identifying antibiotic resistance genes active against these classes. Development of a Gram-positive indicator host will represent a major step forward for the field (Riesenfeld et al., 2004; Sommer et al., 2009). This host must also exhibit a high transformation efficiency to enable the creation of metagenomic libraries large enough to permit the discovery of novel resistance genes and should lack restriction-modification systems or other host defenses that would prevent the expression of foreign DNA. E. coli mutants that mimic Gram-positive species through the alteration of cell membrane and wall structure are another possible alternative (Tamae et al., 2008).
The taxa from which antibiotic resistance genes can be expressed in E. coli are also limited by the ability of the host to recognize gene promoters and ribosome binding sites and translate them into functional proteins. Engineering E. coli to produce tRNA that recognize rare codons and alternative sigma factors will expand the range of taxa interrogated (Riesenfeld et al., 2004; Sommer et al., 2009, 2010a).
Finally, genes of unknown function should be further investigated to determine their mechanisms of resistance. Functional metagenomic selections that uncover a multitude of confirmed resistance genes in a high-throughput manner often require complementary biochemical experimentation to understand novel mechanisms of antibiotic resistance. Many metagenomic functional selections have identified resistance-conferring inserts whose mechanisms remain obscure because they cannot be determined based on sequence alone (Riesenfeld et al., 2004; Diaz-Torres et al., 2006; Kazimierczak et al., 2009; Martiny et al., 2011; McGarvey et al., 2012). These genes are excellent candidates for future functional characterization.
The depth and diversity of antibiotic resistance genes uncovered by functional metagenomic selections, many of which are associated with mobile genetic elements, brings to light the need for novel strategies to combat antibiotic-resistant pathogens. Alternative approaches that modulate the host immune response (e.g., immunomodulatory peptides, vaccination, therapeutic antibodies) or target the pathogen (e.g., anti-virulence initiatives, phage therapy, antibiotic potentiators) have been explored with some success (Planson et al., 2011; Pieren and Tigges, 2012).
For instance, recent studies have suggested engineered bacteriophage as an adjuvant to enhance the bactericidal activity of antibiotics and avoid evolution of resistance to either (Lu and Collins, 2009; Parracho et al., 2012). In this case, bacteriophage is engineered to repress non-essential genes (e.g., the SOS system) not directly targeted by antibiotics, weakening the cell and potentiating antibiotic activity. Other adjuvant compounds that inhibit intrinsic repair pathways or cell tolerance mechanisms are being explored (Fischbach, 2011).
Several permeabilizing products capable of disturbing the cell membrane have been identified, thus allowing antibiotics to penetrate the cells more efficiently. One such product is the low molecular-weight oligosaccharide nanomedicine OligoG, which is able to disturb multidrug-resistant bacteria (Khan et al., 2012).
Drug efflux pumps, both specific and multidrug-resistant, may be inhibited through a variety of strategies. These include interfering with the expression of a functional transporter at different stages between transcription and final assembly, interfering with the assembly of channel proteins, designing inhibitors that compete with the antibiotic for efflux, disrupting the energy source, and blocking the efflux channel (Pages and Amaral, 2009). Deletion of genes involved in expression of porins has been shown to increase antibiotic resistance (Rodrigues et al., 2011). Therefore, strategies combining overexpression of porins used by the antibiotic to penetrate the cell with downregulation of efflux pumps may allow the antibiotic to overcome existing resistance mechanisms.
Functional metagenomic selections are a powerful technique for high-throughput characterization of the transferable resistome encoded by environmental and human-associated microbial communities, which have the potential to provide human pathogens with resistance genes through HGT. The advantages of this technique over traditional culture- and sequence-based screens are underlined when considering novel resistance mechanisms. Because resistance genes are selected on the basis of function regardless of sequence, the functional metagenomic selections reviewed above identified numerous genes with sequences highly divergent from other members of their class, as well as novel resistance mechanisms that would not have been recognized as resistance genes based on sequence alone. Conversely, they confirm antibiotic resistance function in putative drug transporters, which are frequently annotated as such regardless of true resistance ability, and permit the accurate annotation of resistance genes typically associated with other cellular functions. They have also led to the discovery of confirmed bifunctional enzymes, with broad resistance spectrums resulting from the fusion of two resistance genes. By providing a broader understanding of the resistance mechanisms currently in existence, functional metagenomic selections will aid the production of new antibiotics less susceptible to existing resistance mechanisms, as well as alternative strategies for combating antibiotic resistance.
The authors declare that the research was conducted in the absence of any commercial or financial relationships that could be construed as a potential conflict of interest.
This work was supported in part by The Children's Discovery Institute of Washington University and St. Louis Children's Hospital (grant MD-II-2011-117 to Gautam Dantas), The March of Dimes Foundation (grant 6-FY12-394 to Gautam Dantas), the Kenneth Rainin Foundation (grant 12H1 to Gautam Dantas), and the National Institutes of Health (grants DP2-DK098089 and R01-GM099538 to Gautam Dantas). Erica C. Pehrsson is supported by the Department of Defense (DoD) through the National Defense Science & Engineering Graduate Fellowship (NDSEG) Program, Kevin J. Forsberg is a National Science Foundation graduate research fellow (award DGE-1143954), and Molly K. Gibson is a National Science Foundation graduate research fellow (award DGE-1143954).
Alekshun, M. N., and Levy, S. B. (1997). Regulation of chromosomally mediated multiple antibiotic resistance: the mar regulon. Antimicrob. Agents Chemother. 41, 2067–2075.
Alekshun, M. N., and Levy, S. B. (2007). Molecular mechanisms of antibacterial multidrug resistance. Cell 128, 1037–1050. doi: 10.1016/j.cell.2007.03.004
Allen, H. K., Cloud-Hansen, K. A., Wolinski, J. M., Guan, C., Greene, S., Lu, S., et al. (2009a). Resident microbiota of the gypsy moth midgut harbors antibiotic resistance determinants. DNA Cell Biol. 28, 109–117. doi: 10.1089/dna.2008.0812
Allen, H. K., Moe, L. A., Rodbumrer, J., Gaarder, A., and Handelsman, J. (2009b). Functional metagenomics reveals diverse beta-lactamases in a remote Alaskan soil. ISME J. 3, 243–251. doi: 10.1038/ismej.2008.86
Allen, H. K., Donato, J., Wang, H. H., Cloud-Hansen, K. A., Davies, J., and Handelsman, J. (2010). Call of the wild: antibiotic resistance genes in natural environments. Nat. Rev. Microbiol. 8, 251–259. doi: 10.1038/nrmicro2312
Arias, C. A., and Murray, B. E. (2009). Antibiotic-resistant bugs in the 21st century—a clinical super-challenge. N. Engl. J. Med. 360, 439–443. doi: 10.1056/NEJMp0804651
Arthur, M., and Courvalin, P. (1993). Genetics and mechanisms of glycopeptide resistance in enterococci. Antimicrob. Agents Chemother. 37, 1563–1571.
Benveniste, R., and Davies, J. (1973). Aminoglycoside antibiotic-inactivating enzymes in actinomycetes similar to those present in clinical isolates of antibiotic-resistant bacteria. Proc. Natl. Acad. Sci. U.S.A. 70, 2276–2280.
Bugg, T. D., Wright, G. D., Dutka-Malen, S., Arthur, M., Courvalin, P., and Walsh, C. T. (1991). Molecular basis for vancomycin resistance in Enterococcus faecium BM4147: biosynthesis of a depsipeptide peptidoglycan precursor by vancomycin resistance proteins VanH and VanA. Biochemistry 30, 10408–10415. doi: 10.1021/bi00107a007
CDC. (2011). Antimicrobial Resistance Posing Growing Health Threat. Available online at: http://www.cdc.gov/media/releases/2011/p0407_antimicrobialresistance.html [Accessed]. Press Release: April 7, 2011.
Centrón, D., and Roy, P. H. (2002). Presence of a group II intron in a multiresistant Serratia marcescens strain that harbors three integrons and a novel gene fusion. Antimicrob. Agents Chemother. 46, 1402–1409.
Chandrakanth, R. K., Raju, S., and Patil, S. A. (2008). Aminoglycoside-resistance mechanisms in multidrug-resistant Staphylococcus aureus clinical isolates. Curr. Microbiol. 56, 558–562. doi: 10.1007/s00284-008-9123-y
Cheng, G., Hu, Y., Yin, Y., Yang, X., Xiang, C., Wang, B., et al. (2012). Functional screening of antibiotic resistance genes from human gut microbiota reveals a novel gene fusion. FEMS Microbiol. Lett. 336, 11–16. doi: 10.1111/j.1574-6968.2012.02647.x
Cui, L., Iwamoto, A., Lian, J. Q., Neoh, H. M., Maruyama, T., Horikawa, Y., et al. (2006). Novel mechanism of antibiotic resistance originating in vancomycin-intermediate Staphylococcus aureus. Antimicrob. Agents Chemother. 50, 428–438. doi: 10.1128/AAC.50.2.428-438.2006
D'Costa, V. M., King, C. E., Kalan, L., Morar, M., Sung, W. W. L., Schwarz, C., et al. (2011). Antibiotic resistance is ancient. Nature 477, 457–461. doi: 10.1038/nature10388
D'Costa, V. M., McGrann, K. M., Hughes, D. W., and Wright, G. D. (2006). Sampling the antibiotic resistome. Science 311, 374–377. doi: 10.1126/science.1120800
Daigle, D. M., Hughes, D. W., and Wright, G. D. (1999). Prodigious substrate specificity of AAC(6′)-APH(2″), an aminoglycoside antibiotic resistance determinant in enterococci and staphylococci. Chem. Biol. 6, 99–110. doi: 10.1016/S1074-5521(99)80006-4
Daniel, R. (2005). The metagenomics of soil. Nat. Rev. Microbiol. 3, 470–478. doi: 10.1038/nrmicro1160
Dantas, G., and Sommer, M. O. (2012). Context matters - the complex interplay between resistome genotypes and resistance phenotypes. Curr. Opin. Microbiol. 15, 577–582. doi: 10.1016/j.mib.2012.07.004
Davies, J., and Davies, D. (2010). Origins and evolution of antibiotic resistance. Microbiol. Mol. Biol. Rev. 74, 417–433. doi: 10.1128/MMBR.00016-10
Davies, J., and Wright, G. D. (1997). Bacterial resistance to aminoglycoside antibiotics. Trends Microbiol. 5, 234–240. doi: 10.1016/S0966-842X(97)01033-0
Delcour, A. H. (2009). Outer membrane permeability and antibiotic resistance. Biochim. Biophys. Acta 1794, 808–816. doi: 10.1016/j.bbapap.2008.11.005
Diaz-Torres, M. L., McNab, R., Spratt, D. A., Villedieu, A., Hunt, N., Wilson, M., et al. (2003). Novel tetracycline resistance determinant from the oral metagenome. Antimicrob. Agents Chemother. 47, 1430–1432.
Diaz-Torres, M. L., Villedieu, A., Hunt, N., McNab, R., Spratt, D. A., Allan, E., et al. (2006). Determining the antibiotic resistance potential of the indigenous oral microbiota of humans using a metagenomic approach. FEMS Microbiol. Lett. 258, 257–262. doi: 10.1111/j.1574-6968.2006.00221.x
Donato, J. J., Moe, L. A., Converse, B. J., Smart, K. D., Berklein, F. C., McManus, P. S., et al. (2010). Metagenomic analysis of apple orchard soil reveals antibiotic resistance genes encoding predicted bifunctional proteins. Appl. Environ. Microbiol. 76, 4396–4401. doi: 10.1128/AEM.01763-09
Dubois, V., Poirel, L., Marie, C., Arpin, C., Nordmann, P., and Quentin, C. (2002). Molecular characterization of a novel class 1 integron containing bla(GES-1) and a fused product of aac3-Ib/aac6′-Ib′ gene cassettes in Pseudomonas aeruginosa. Antimicrob. Agents Chemother. 46, 638–645.
Ferretti, J. J., Gilmore, K. S., and Courvalin, P. (1986). Nucleotide sequence analysis of the gene specifying the bifunctional 6′-aminoglycoside acetyltransferase 2>″-aminoglycoside phosphotransferase enzyme in Streptococcus faecalis and identification and cloning of gene regions specifying the two activities. J. Bacteriol. 167, 631–638.
Fischbach, M. A. (2011). Combination therapies for combating antimicrobial resistance. Curr. Opin. Microbiol. 14, 519–523. doi: 10.1016/j.mib.2011.08.003
Flensburg, J., and Skold, O. (1987). Massive overproduction of dihydrofolate reductase in bacteria as a response to the use of trimethoprim. Eur. J. Biochem. 162, 473–476. doi: 10.1111/j.1432-1033.1987.tb10664.x
Forsberg, K. J., Reyes, A., Wang, B., Selleck, E. M., Sommer, M. O., and Dantas, G. (2012). The shared antibiotic resistome of soil bacteria and human pathogens. Science 337, 1107–1111. doi: 10.1126/science.1220761
Galan, J. C., Gonzalez-Candelas, F., Rolain, J. M., and Canton, R. (2013). Antibiotics as selectors and accelerators of diversity in the mechanisms of resistance: from the resistome to genetic plasticity in the beta-lactamases world. Front. Microbiol. 4:9. doi: 10.3389/fmicb.2013.00009
Galimand, M., Courvalin, P., and Lambert, T. (2003). Plasmid-mediated high-level resistance to aminoglycosides in Enterobacteriaceae due to 16S rRNA methylation. Antimicrob. Agents Chemother. 47, 2565–2571.
Hall, B. G., and Barlow, M. (2004). Evolution of the serine beta-lactamases: past, present and future. Drug Resist. Updat. 7, 111–123. doi: 10.1016/j.drup.2004.02.003
Hall, B. G., Salipante, S. J., and Barlow, M. (2004). Independent origins of subgroup Bl + B2 and subgroup B3 metallo-beta-lactamases. J. Mol. Evol. 59, 133–141. doi: 10.1007/s00239-003-2572-9
Hooper, D. C. (1999). Mechanisms of fluoroquinolone resistance. Drug Resist. Updat. 2, 38–55. doi: 10.1054/drup.1998.0068
Houndt, T., and Ochman, H. (2000). Long-term shifts in patterns of antibiotic resistance in enteric bacteria. Appl. Environ. Microbiol. 66, 5406–5409. doi: 10.1128/AEM.66.12.5406-5409.2000
Hughes, V. M., and Datta, N. (1983). Conjugative plasmids in bacteria of the ‘pre-antibiotic’ era. Nature 302, 725–726. doi: 10.1038/302725a0
Huovinen, P., Sundstrom, L., Swedberg, G., and Skold, O. (1995). Trimethoprim and sulfonamide resistance. Antimicrob. Agents Chemother. 39, 279–289. doi: 10.1128/AAC.39.2.279
Jacoby, G. A. (2005). Mechanisms of resistance to quinolones. Clin. Infect. Dis. 41(Suppl. 2), S120–S126. doi: 10.1086/428052
Jacoby, G. A., and Munoz-Price, L. S. (2005). The new beta-lactamases. N. Engl. J. Med. 352, 380–391. doi: 10.1056/NEJMra041359
Jeon, J. H., Kim, S. J., Lee, H. S., Cha, S. S., Lee, J. H., Yoon, S. H., et al. (2011). Novel metagenome-derived carboxylesterase that hydrolyzes beta-lactam antibiotics. Appl. Environ. Microbiol. 77, 7830–7836. doi: 10.1128/AEM.05363-11
Kazimierczak, K. A., Scott, K. P., Kelly, D., and Aminov, R. I. (2009). Tetracycline resistome of the organic pig gut. Appl. Environ. Microbiol. 75, 1717–1722. doi: 10.1128/AEM.02206-08
Khan, S., Tondervik, A., Sletta, H., Klinkenberg, G., Emanuel, C., Onsoyen, E., et al. (2012). Overcoming drug resistance with alginate oligosaccharides able to potentiate the action of selected antibiotics. Antimicrob. Agents Chemother. 56, 5134–5141. doi: 10.1128/AAC.00525-12
Kim, C., Hesek, D., Zajícek, J., Vakulenko, S. B., and Mobashery, S. (2006). Characterization of the Bifunctional Aminoglycoside-Modifying Enzyme ANT(3″)-Ii/AAC(6′)-IId from Serratia marcescens. Biochemistry 45, 8368–8377. doi: 10.1021/bi060723g
Kim, S. J., Lee, C. M., Han, B. R., Kim, M. Y., Yeo, Y. S., Yoon, S. H., et al. (2008). Characterization of a gene encoding cellulase from uncultured soil bacteria. FEMS Microbiol. Lett. 282, 44–51. doi: 10.1111/j.1574-6968.2008.01097.x
Knapp, C. W., Dolfing, J., Ehlert, P. A., and Graham, D. W. (2010). Evidence of increasing antibiotic resistance gene abundances in archived soils since 1940. Environ. Sci. Technol. 44, 580–587. doi: 10.1021/es901221x
Lang, K. S., Anderson, J. M., Schwarz, S., Williamson, L., Handelsman, J., and Singer, R. S. (2010). Novel florfenicol and chloramphenicol resistance gene discovered in Alaskan soil by using functional metagenomics. Appl. Environ. Microbiol. 76, 5321–5326. doi: 10.1128/AEM.00323-10
Levy, S. B. (1992). Active efflux mechanisms for antimicrobial resistance. Antimicrob. Agents Chemother. 36, 695–703. doi: 10.1128/AAC.36.4.695
Li, X. Z., and Nikaido, H. (2009). Efflux-mediated drug resistance in bacteria: an update. Drugs 69, 1555–1623. doi: 10.2165/11317030-000000000-00000
Lu, T. K., and Collins, J. J. (2009). Engineered bacteriophage targeting gene networks as adjuvants for antibiotic therapy. Proc. Natl. Acad. Sci. U.S.A. 106, 4629–4634. doi: 10.1073/pnas.0800442106
Marshall, B. M., and Levy, S. B. (2011). Food animals and antimicrobials: impacts on human health. Clin. Microbiol. Rev. 24, 718–733. doi: 10.1128/CMR.00002-11
Martin, R. G., and Rosner, J. L. (2002). Genomics of the marA/soxS/rob regulon of Escherichia coli: identification of directly activated promoters by application of molecular genetics and informatics to microarray data. Mol. Microbiol. 44, 1611–1624. doi: 10.3410/f.1008106.102720
Martinez, J. L. (2008). Antibiotics and antibiotic resistance genes in natural environments. Science 321, 365–367. doi: 10.1126/science.1159483
Martiny, A. C., Martiny, J. B., Weihe, C., Field, A., and Ellis, J. C. (2011). Functional metagenomics reveals previously unrecognized diversity of antibiotic resistance genes in gulls. Front. Microbiol. 2:238. doi: 10.3389/fmicb.2011.00238
McGarvey, K. M., Queitsch, K., and Fields, S. (2012). Wide variation in antibiotic resistance proteins identified by functional metagenomic screening of a soil DNA library. Appl. Environ. Microbiol. 78, 1708–1714. doi: 10.1128/AEM.06759-11
McHardy, A. C., Martin, H. G., Tsirigos, A., Hugenholtz, P., and Rigoutsos, I. (2007). Accurate phylogenetic classification of variable-length DNA fragments. Nat. Methods 4, 63–72. doi: 10.1038/NMETH976
Mendes, R. E., Toleman, M. A., Ribeiro, J., Sader, H. S., Jones, R. N., and Walsh, T. R. (2004). Integron carrying a novel metallo-beta-lactamase gene, blaIMP-16, and a fused form of aminoglycoside-resistant gene aac(6′)-30/aac(6′)-Ib′: report from the SENTRY Antimicrobial Surveillance Program. Antimicrob. Agents Chemother. 48, 4693–4702. doi: 10.1128/AAC.48.12.4693-4702.2004
Mori, T., Mizuta, S., Suenaga, H., and Miyazaki, K. (2008). Metagenomic screening for bleomycin resistance genes. Appl. Environ. Microbiol. 74, 6803–6805. doi: 10.1128/AEM.00873-08
NIAID. (2012). Antimicrobial (Drug) Resistance: Quick Facts. Available online at: http://www.niaid.nih.gov/topics/antimicrobialResistance/Understanding/Pages/quickFacts.aspx [Accessed]. Last Updated: January 05, 2012
Ochman, H., Lawrence, J. G., and Groisman, E. A. (2000). Lateral gene transfer and the nature of bacterial innovation. Nature 405, 299–304. doi: 10.1038/35012500
Pages, J. M., and Amaral, L. (2009). Mechanisms of drug efflux and strategies to combat them: challenging the efflux pump of Gram-negative bacteria. Biochim. Biophys. Acta 1794, 826–833. doi: 10.1016/j.bbapap.2008.12.011
Parracho, H. M., Burrowes, B. H., Enright, M. C., McConville, M. L., and Harper, D. R. (2012). The role of regulated clinical trials in the development of bacteriophage therapeutics. J. Mol. Genet. Med. 6, 279–286.
Parsley, L. C., Consuegra, E. J., Kakirde, K. S., Land, A. M., Harper, W. F. Jr., and Liles, M. R. (2010). Identification of diverse antimicrobial resistance determinants carried on bacterial, plasmid, or viral metagenomes from an activated sludge microbial assemblage. Appl. Environ. Microbiol. 76, 3753–3757. doi: 10.1128/AEM.03080-09
Perez-Perez, F. J., and Hanson, N. D. (2002). Detection of plasmid-mediated AmpC beta-lactamase genes in clinical isolates by using multiplex PCR. J. Clin. Microbiol. 40, 2153–2162. doi: 10.1128/JCM.40.6.2153-2162.2002
Perez, F., Hujer, A. M., Hujer, K. M., Decker, B. K., Rather, P. N., and Bonomo, R. A. (2007). Global challenge of multidrug-resistant Acinetobacter baumannii. Antimicrob. Agents Chemother. 51, 3471–3484. doi: 10.1128/AAC.01464-06
Pieren, M., and Tigges, M. (2012). Adjuvant strategies for potentiation of antibiotics to overcome antimicrobial resistance. Curr. Opin. Pharmacol. 12, 551–555. doi: 10.1016/j.coph.2012.07.005
Planson, A. G., Carbonell, P., Grigoras, I., and Faulon, J. L. (2011). Engineering antibiotic production and overcoming bacterial resistance. Biotechnol. J. 6, 812–825. doi: 10.1002/biot.201100085
Poirel, L., Kampfer, P., and Nordmann, P. (2002). Chromosome-encoded Ambler class A beta-lactamase of Kluyvera georgiana, a probable progenitor of a subgroup of CTX-M extended-spectrum beta-lactamases. Antimicrob. Agents Chemother. 46, 4038–4040.
Poirel, L., Rodriguez-Martinez, J. M., Mammeri, H., Liard, A., and Nordmann, P. (2005). Origin of plasmid-mediated quinolone resistance determinant QnrA. Antimicrobi. Agents Chemother. 49, 3523–3525. doi: 10.1128/AAC.49.8.3523-3525.2005
Poole, K. (2005). Efflux-mediated antimicrobial resistance. J. Antimicrob. Chemother. 56, 20–51. doi: 10.1093/jac/dki171
Riesenfeld, C. S., Goodman, R. M., and Handelsman, J. (2004). Uncultured soil bacteria are a reservoir of new antibiotic resistance genes. Environ. Microbiol. 6, 981–989. doi: 10.1111/j.1462-2920.2004.00664.x
Robicsek, A., Jacoby, G. A., and Hooper, D. C. (2006). The worldwide emergence of plasmid-mediated quinolone resistance. Lancet Infect. Dis. 6, 629–640. doi: 10.1016/S1473-3099(06)70599-0
Rodrigues, L., Ramos, J., Couto, I., Amaral, L., and Viveiros, M. (2011). Ethidium bromide transport across Mycobacterium smegmatis cell-wall: correlation with antibiotic resistance. BMC Microbiol. 11:35. doi: 10.1186/1471-2180-11-35
Schwarz, S., Kehrenberg, C., Doublet, B., and Cloeckaert, A. (2004). Molecular basis of bacterial resistance to chloramphenicol and florfenicol. FEMS Microbiol. Rev. 28, 519–542. doi: 10.1016/j.femsre.2004.04.001
Shoemaker, N. B., Vlamakis, H., Hayes, K., and Salyers, A. A. (2001). Evidence for extensive resistance gene transfer among Bacteroides spp. and among Bacteroides and other genera in the human colon. Appl. Environ. Microbiol. 67, 561–568. doi: 10.1128/AEM.67.2.561-568.2001
Smillie, C. S., Smith, M. B., Friedman, J., Cordero, O. X., David, L. A., and Alm, E. J. (2011). Ecology drives a global network of gene exchange connecting the human microbiome. Nature 480, 241–244. doi: 10.1038/nature10571
Sommer, M. O., Church, G. M., and Dantas, G. (2010a). A functional metagenomic approach for expanding the synthetic biology toolbox for biomass conversion. Mol. Syst. Biol. 6, 360. doi: 10.1038/msb.2010.16
Sommer, M. O., Church, G. M., and Dantas, G. (2010b). The human microbiome harbors a diverse reservoir of antibiotic resistance genes. Virulence 1, 299–303. doi: 10.4161/viru.1.4.12010
Sommer, M. O., and Dantas, G. (2011). Antibiotics and the resistant microbiome. Curr. Opin. Microbiol. 14, 556–563. doi: 10.1016/j.mib.2011.07.005
Sommer, M. O., Dantas, G., and Church, G. M. (2009). Functional characterization of the antibiotic resistance reservoir in the human microflora. Science 325, 1128–1131. doi: 10.1126/science.1176950
Soussy, C. J., Wolfson, J. S., Ng, E. Y., and Hooper, D. C. (1993). Limitations of plasmid complementation test for determination of quinolone resistance due to changes in the gyrase A protein and identification of conditional quinolone resistance locus. Antimicrob. Agents Chemother. 37, 2588–2592. doi: 10.1128/AAC.37.12.2588
Spellberg, B., Guidos, R., Gilbert, D., Bradley, J., Boucher, H. W., Scheld, W. M., et al. (2008). The epidemic of antibiotic-resistant infections: a call to action for the medical community from the Infectious Diseases Society of America. Clin. Infect. Dis. 46, 155–164. doi: 10.1086/524891
Stewart, P. S., and Costerton, J. W. (2001). Antibiotic resistance of bacteria in biofilms. Lancet 358, 135–138.
Stolz, A. (2009). Molecular characteristics of xenobiotic-degrading sphingomonads. Appl. Microbiol. Biotechnol. 81, 793–811. doi: 10.1007/s00253-008-1752-3
Stone, P. W. (2009). Economic burden of healthcare-associated infections: an American perspective. Exp. Rev. Pharmacoecon Outcomes Res. 9, 417–422. doi: 10.1586/erp.09.53
Tamae, C., Liu, A., Kim, K., Sitz, D., Hong, J., Becket, E., et al. (2008). Determination of antibiotic hypersensitivity among 4, 000 single-gene-knockout mutants of Escherichia coli. J. Bacteriol. 190, 5981–5988. doi: 10.1128/JB.01982-07
Tao, W. (2011). Characterization of two metagenome-derived esterases that reactivate chloramphenicol by counteracting chloramphenicol acetyltransferase. J. Microbiol. Biotechnol. 21, 1203–1210.
Tao, W., Lee, M. H., Wu, J., Kim, N. H., Kim, J. C., Chung, E., et al. (2012). Inactivation of chloramphenicol and florfenicol by a novel chloramphenicol hydrolase. Appl. Environ. Microbiol. 78, 6295–6301. doi: 10.1128/AEM.01154-12
Thaker, M., Spanogiannopoulos, P., and Wright, G. D. (2010). The tetracycline resistome. Cell. Mol. Life Sci. 67, 419–431. doi: 10.1007/s00018-009-0172-6
Torres-Cortes, G., Millan, V., Ramirez-Saad, H. C., Nisa-Martinez, R., Toro, N., and Martinez-Abarca, F. (2011). Characterization of novel antibiotic resistance genes identified by functional metagenomics on soil samples. Environ. Microbiol. 13, 1101–1114. doi: 10.1111/j.1462-2920.2010.02422.x
Tran, J. H., and Jacoby, G. A. (2002). Mechanism of plasmid-mediated quinolone resistance. Proc. Natl. Acad. Sci. U.S.A. 99, 5638–5642. doi: 10.1073/pnas.082092899
Vetting, M. W., Park, C. H., Hegde, S. S., and Jacoby, G. A. (2008). Mechanistic and Structural Analysis of Aminoglycoside N-Acetyltransferase, AAC (6′)-Ib and Its Bifunctional, Fluoroquinolone-Active, AAC (6′)-Ib-cr Variant†‡. Biochemistry 47, 9825–9835. doi: 10.1021/bi800664x
Walsh, C. (2000). Molecular mechanisms that confer antibacterial drug resistance. Nature 406, 775–781. doi: 10.1038/35021219
Wolfson, J. S., and Hooper, D. C. (1989). Fluoroquinolone antimicrobial agents. Clin. Microbiol. Rev. 2, 378–424.
Wright, G. D. (2010). Antibiotic resistance in the environment: a link to the clinic? Curr. Opin. Microbiol. 13, 589–594. doi: 10.1016/j.mib.2010.08.005
Yang, W., Moore, I. F., Koteva, K. P., Bareich, D. C., Hughes, D. W., and Wright, G. D. (2004). TetX is a flavin-dependent monooxygenase conferring resistance to tetracycline antibiotics. J. Biol. Chem. 279, 52346–52352. doi: 10.1074/jbc.M409573200
Keywords: functional metagenomics, antibiotic resistance, bifunctional resistance gene, environmental resistance, resistance reservoir, transferable resistance
Citation: Pehrsson EC, Forsberg KJ, Gibson MK, Ahmadi S and Dantas G (2013) Novel resistance functions uncovered using functional metagenomic investigations of resistance reservoirs. Front. Microbiol. 4:145. doi: 10.3389/fmicb.2013.00145
Received: 04 April 2013; Paper pending published: 19 April 2013;
Accepted: 21 May 2013; Published online: 07 June 2013.
Edited by:
Marilyn C. Roberts, University of Washington, USACopyright © 2013 Pehrsson, Forsberg, Gibson, Ahmadi and Dantas. This is an open-access article distributed under the terms of the Creative Commons Attribution License, which permits use, distribution and reproduction in other forums, provided the original authors and source are credited and subject to any copyright notices concerning any third-party graphics etc.
*Correspondence: Gautam Dantas, Center for Genome Sciences and Systems Biology, Washington University School of Medicine, 4444 Forest Park Blvd., 6th floor, Campus Box 8510, St. Louis, MO 63108, USA e-mail:ZGFudGFzQHd1c3RsLmVkdQ==
Disclaimer: All claims expressed in this article are solely those of the authors and do not necessarily represent those of their affiliated organizations, or those of the publisher, the editors and the reviewers. Any product that may be evaluated in this article or claim that may be made by its manufacturer is not guaranteed or endorsed by the publisher.
Research integrity at Frontiers
Learn more about the work of our research integrity team to safeguard the quality of each article we publish.