- Biological Oceanography Division, Helmholtz-Zentrum für Ozeanforschung Kiel (GEOMAR), Kiel, Germany
Iron (Fe) is an essential micronutrient for many processes in all living cells. Dissolved Fe (dFe) concentrations in the ocean are of the order of a few nM, and Fe is often a factor limiting primary production. Bioavailability of Fe in aquatic environments is believed to be primarily controlled through chelation by Fe-binding ligands. Marine microbes have evolved different mechanisms to cope with the scarcity of bioavailable dFe. Gradients in dFe concentrations and diversity of the Fe-ligand pool from coastal to open ocean waters have presumably imposed selection pressures that should be reflected in the genomes of microbial communities inhabiting the pelagic realm. We applied a hidden Markov model (HMM)-based search for proteins related to cellular iron metabolism, and in particular those involved in Fe uptake mechanisms in 164 microbial genomes belonging to diverse taxa and occupying different aquatic niches. A multivariate statistical approach demonstrated that in phototrophic organisms, there is a clear influence of the ecological niche on the diversity of Fe uptake systems. Extending the analyses to the metagenome database from the Global Ocean Sampling expedition, we demonstrated that the Fe uptake and homeostasis mechanisms differed significantly across marine niches defined by temperatures and dFe concentrations, and that this difference was linked to the distribution of microbial taxa in these niches. Using the dN/dS ratios (which signify the rate of non-synonymous mutations) of the nucleotide sequences, we identified that genes encoding for TonB, Ferritin, Ferric reductase, IdiA, ZupT, and Fe2+ transport proteins FeoA and FeoB were evolving at a faster rate (positive selection pressure) while genes encoding ferrisiderophore, heme and Vitamin B12 uptake systems, siderophore biosynthesis, and IsiA and IsiB were under purifying selection pressure (evolving slowly).
Introduction
Iron-containing metalloenzymes are essential for many life processes, including photosynthesis, respiration, and nitrogen fixation. Due to the tendency of Fe3+ to form ferric hydroxides and oxyhydroxide polymers in the presence of oxygen, the dFe concentration in surface seawater is <0.5 nM (Johnson et al., 1997). Aeolian dust deposition is the dominant external source of iron in the open ocean surface waters (Duce and Tindale, 1991; Jickells et al., 2005) of the North Atlantic and North-East Pacific accounting for 48 and 22% of total Fe deposition respectively (Gao et al., 2001). In around 40% of the world’s oceans where surface waters are high in nutrient and low in chlorophyll (HNLC regions), low Fe supply limits the growth of resident eukaryotic phytoplankton and cyanobacteria responsible for primary production (Martin et al., 1994; Coale et al., 1996; Boyd et al., 2000; Gall et al., 2001; Tsuda et al., 2003; de Baar et al., 2005).
Diverse strategies have evolved to competitively acquire enough iron for survival in various oceanic habitats. This competition is intensified by the fact that >99.9% of dFe is complexed to Fe-binding ligands of diverse nature (Gledhill and van den Berg, 1994; Rue and Bruland, 1995; Gledhill and Buck, 2012). Thus, microorganisms have developed systems to take up Fe from a wide range of Fe-binding ligands. Although ubiquitous in nature, Fe-binding ligands vary in their Fe-binding affinities and their distribution from surface to deep waters (Rue and Bruland, 1995; Hunter and Boyd, 2007) and from coastal to open ocean waters (Boye et al., 2003; Buck and Bruland, 2007).
The ability to produce siderophores in the open ocean is apparently, largely confined to heterotrophic bacteria (Reid et al., 1993; Martinez et al., 2000; Butler, 2005; Martinez and Butler, 2007; Homann et al., 2009a,b). While siderophore biosynthesis pathways have been found in some coastal (Ito and Butler, 2005) or fresh water (Ito et al., 2004) cyanobacteria, they appear to be absent in open ocean cyanobacteria. Coastal strains of Synechococcus that have been reported to produce siderophores (Ito and Butler, 2005) have high Fe-quotas compared to oceanic strains (Palenik et al., 2006). Even though only a few marine microorganisms can synthesize siderophores, the ability to take up siderophores may be more widespread, as evidenced by the abundance of TonB-dependent (TBD) siderophore uptake systems observed in terrestrial and freshwater microorganisms devoid of siderophore synthesis pathways (Plessner et al., 1993; Katoh et al., 2001; Poole and McKay, 2003; Joshi et al., 2008). Additionally, various heme-acquisition systems have been identified in bacteria for the utilization of Fe bound to heme (Stojiljkovic and Hantke, 1994; Cope et al., 1995; Thompson et al., 1999; Ochsner et al., 2000; Hopkinson et al., 2008). Notably, siderophore biosynthesis and TBD siderophore/heme uptake receptors are absent from the genomes of Prochlorococcus and strains of Synechococcus that dominate the microbial communities of open ocean surface waters (Waterbury et al., 1979; Liu et al., 1997, 1999; Hopkinson and Morel, 2009). The reduction of cellular Fe requirements provides an alternate adaptive strategy for surviving Fe limitation in open ocean surface waters. This strategy has been observed in the Prochlorococcus ecotypes (Thompson et al., 2011) dominant in HNLC waters, which have decreased their Fe-quotas by eliminating several Fe-requiring proteins (Rusch et al., 2010).
The availability of a large number of marine prokaryotic and eukaryotic microbial genomes and the Global Ocean Sampling (GOS) metagenomes, have greatly enhanced our understanding of the Fe-acquisition strategies used by various groups of microorganisms. Recent studies by Hopkinson and Barbeau (2012) and Toulza et al. (2012) presented sequence-based approaches to analyze the differences in occurrence patterns of proteins involved in Fe-metabolism in marine prokaryotic genomes and metagenomes, respectively. Hopkinson and Barbeau (2012) reported a dominance of TBD uptake systems in Gammaproteobacteria, and identified a novel heme TBDT in Prochlorococcus which may have been acquired by horizontal gene transfer, providing niche specific adaptation in this organism. Their study further revealed the widespread occurrence of Fe3+ ABC transporters in all groups of marine bacteria except for Flavobacteria, and a lack of the specific Fe2+ uptake system (FeoAB) in picocyanobacteria and Alphaproteobacteria. They identified that the TBDTs were less common in the metagenomes than in the genomes, an observation that reflects the numerical dominance of Pelagibacter and Prochlorococcus (Rusch et al., 2007) in the current collection of GOS metagenomes. Both genera have small genomes and non-specialized iron uptake systems (Smith et al., 2010; Thompson et al., 2011). A more detailed analysis of the Fe-metabolism proteins in the GOS metagenomes revealed a distribution pattern influenced by dFe concentrations (Toulza et al., 2012). The frequencies of occurrence of Fe3+ transporters and of Fe2+ uptake systems were negatively correlated with each other, the former being more abundant in the open ocean environments and the latter in the coastal environments, respectively. The taxonomic diversity and Fe-pathway prevalence differed significantly with habitat or niche type (Open Ocean or Coastal). However the GOS samples are distributed along a wide range of latitudes possibly confounding the effect of temperature, which varies widely within both Open Ocean and Coastal niches. In addition, prior studies did not directly investigate the exact nature of the link between taxonomic diversity and Fe-pathway prevalence within the metagenomes.
Here, we build on the results presented in the studies of Hopkinson and Barbeau (2012) and Toulza et al. (2012), by extending the analyses to marine eukaryotic genomes of phytoplankton. We established a link between the Fe-metabolism profiles and taxonomic diversity prevalent in the metagenomes by comparing the Fe-metabolism protein occurrence profiles (Table 1) from the genomes of various taxa and those obtained from various aquatic niches defined by environmental characterization of metagenomes. Marine prokaryotic and eukaryotic microorganisms were grouped according to their location of isolation (Open Ocean, Coastal, or Freshwater) to check for niche specific adaptations reflected in their genomes. The GOS metagenomes were subjected to a more complex grouping in order to account for differences in Fe-metabolism protein profiles of the microbial community, which could be attributed to temperature, dFe concentration, or a coastal versus Open Ocean sampling location. Thus, we defined three contrasting niche group pairs in the GOS metagenomes such that the groups of samples in a pair differed with respect to only one of the above environmental factors. A multivariate statistical approach was used to study the differential distribution of Fe-metabolism protein profiles in genomes and in the above GOS metagenomic groupings to establish whether the Fe-metabolism strategies were correlated with the taxonomic distribution in the GOS metagenomes. We found a set of proteins that were statistically discriminating between the aquatic niches. Calculations of the non-synonymous mutation rates (dN/dS) for this set of proteins indicated that they were under positive selection pressure and therefore were evolving rapidly.
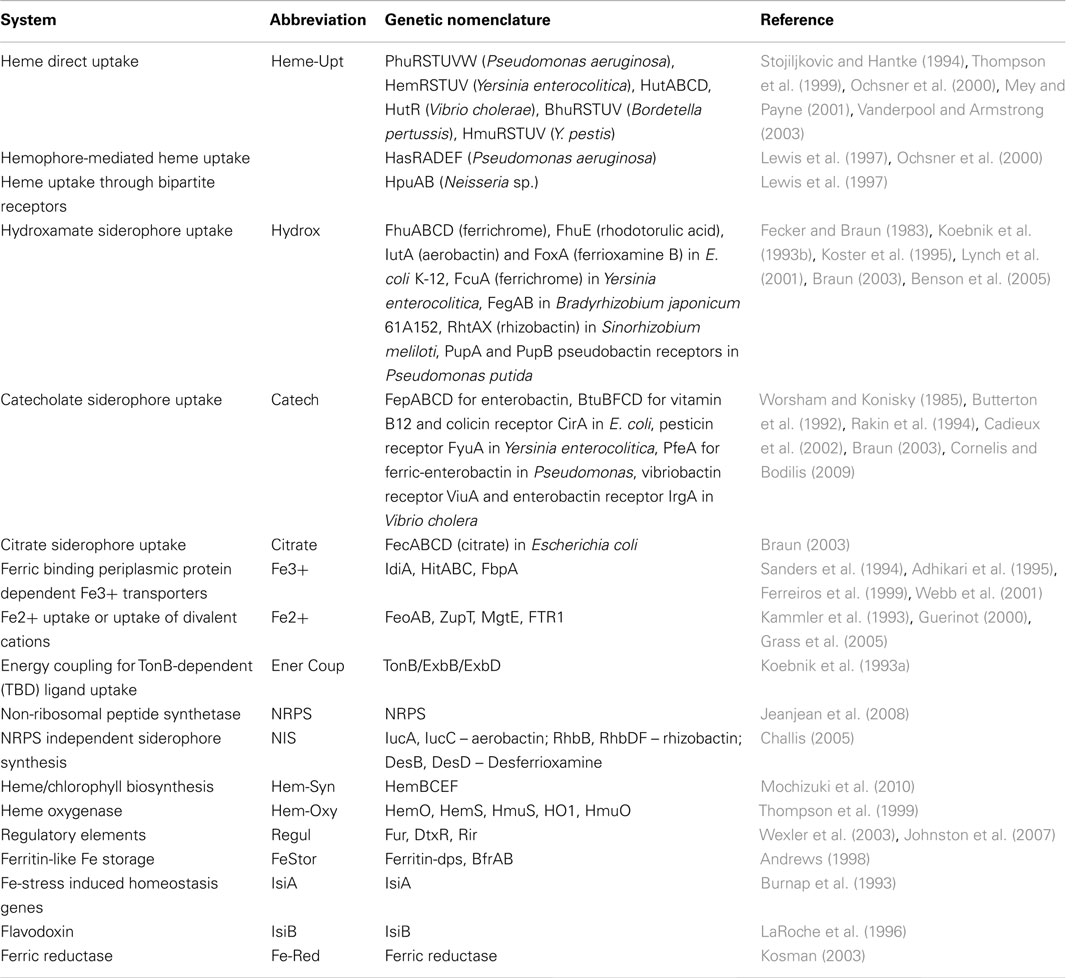
Table 1. List of proteins involved in Fe-ligand (siderophore and heme) uptake, siderophore, and heme biosynthesis and Fe homeostasis in microorganisms.
Materials and Methods
Hidden Markov Model-ModE Profiles of Iron Metabolism Genes
The set of non-redundant (Uniref 100) protein sequences for the genes listed in Table 1 were downloaded from Uniprot and HMM-ModE profiles were created as described earlier (Srivastava et al., 2007). The HMM-ModE protocol allows the construction of HMM profiles with increased specificity by using negative training sequences.
The training sequences for each protein were first clustered using the Markov Clustering Algorithm (MCL) (Enright et al., 2002). For each subgroup of each protein, the training sequences were aligned with MUSCLE (Edgar, 2004) and HMMs were generated using hmmbuild from the HMMER2 package (Eddy, 1998). The discrimination threshold of each protein HMM was optimized by an n-fold cross-validation exercise. The training sequences for each were divided into n test sets such that each sequence is part of at least one test set. For each test set t, the remaining (n-1) sets were combined to form the train set and used to build an HMM. The sequences in t were scored using this HMM by hmmsearch program to get a True Positive (TP) score distribution. False positives (FP) were identified from the negative training set (in this case the entire UniProt database excluding the training sequences for the gene in question). The sensitivity, specificity, and Matthews Correlation Coefficient (MCC) distribution for each of n sets was calculated (Hannenhalli and Russell, 2000). The optimal discrimination threshold was identified as the mid-point of the MCC distribution averaged over the n sets. Further increase in specificity was obtained by modifying the emission probabilities of the gene HMM by using the FP alignment as described earlier (Srivastava et al., 2007).
These HMM-ModE profiles with their optimized threshold were used with the program hmmsearch to scan the protein sequences from the marine microbial genomes as well as from the GOS metagenomes. All the above steps were performed using customized Perl scripts that are available for download from https://sites.google.com/site/dhwanidesai/home/bioinformatics.
Obtaining the Complete Genome Sequences of Marine Microbes
Complete genome sequences of marine microbes whose sequencing projects were commissioned by the Gordon and Betty Moore Foundation under their Marine Microbiology Initiative1 were downloaded from NCBI in the form of protein FASTA files. Complete genomes of six eukaryotic marine microorganisms including diatoms (Thalassiosira pseudonana, T. oceanica, and Phaeodactylum tricornutum), a pelagophyte (Aureococcus anophagefferens), green algae (Ostreococcus lucimarinus and Ostreococcus tauri), and a prymnesiophyte (Emiliania huxleyii) were also downloaded from NCBI as FASTA files of protein sequences. We also analyzed 12 Freshwater microbial genomes mentioned in (Hopkinson and Morel, 2009) making a total of 164 genomes.
Obtaining the Metagenome Sequences
The GOS metagenomic sequences and the corresponding metadata were downloaded from the CAMERA portal (Seshadri et al., 2007). The nucleotide sequences were translated in all six frames and all translations with a length less than 25 amino acids were discarded. Since there was a large variation in the number of sequences in each sample, we used the Daisychopper2 strategy to randomly select an equal number of sequences from all the samples. From the 44 GOS samples, we selected 30 samples that were obtained from a 0.1–0.8 μm filter and were classified as “Open Ocean” or “Coastal” (Table S2 in Supplementary Material). The sample GS07, from the Northern Gulf of Maine (43.63°N, 66.84°W), had the least number of sequences (50980). Hence, 50980 sequences were randomly selected from each of the other samples for the hmmsearch. The taxonomic profiles for these samples were downloaded from the MG-RAST server3.
Statistical Analysis
The results of the hmmsearch program were parsed and tabulated as m × n matrix (m genomes or metagenomes in rows × n genes in columns). This matrix was used for making the non-parametric multidimensional scaling (NMDS) plots using the Primer-E v6 software (Clarke, 2006). Discarding gene columns which did not have any hits in any of the genomes, we obtained a 164 × 85 matrix. For the metagenomic samples, an environmental matrix was also created using the metadata provided in CAMERA for the GOS samples. Apart from the geographical coordinates of the samples, the environmental matrix contained “sample depth,” “water column depth,” “temperature,” and “dFe deposition” as variables. The dissolved iron concentration at the surface for the sample coordinates was obtained from the PELAGOS model simulation (Vichi et al., 2007a,b; data kindly provided by Dr. Marcello Vichi). We used the yearly mean concentration of dissolved iron, averaged over the entire period of simulation, i.e., from 1980 to 2002.
Analysis of Similarities (ANOSIM) test for statistically significant differences between prior groupings of the samples made according to taxonomy or location and the Similarity Percentages (SIMPER) analysis comparing relative abundances of genes in the said prior groupings to identify discriminating genes were carried out using Primer-E. Principal Components Analysis (PCA) of the GOS samples using the environmental matrix (Tables S1 and S2 in Supplementary Material) was also performed using Primer-E. Following is a brief description of the non-parametric statistical methods implemented in Primer-E (Clarke, 1993) that we have used here.
Data transformations
Whereas the abundance matrices were log-transformed, for the environmental matrix, the variables were individually transformed to reduce the collinearity as much as possible. So, Latitude and Longitude were square-root transformed, “Water column depth” was log-transformed and “dFe deposition” was exponential-transformed.
Bray–Curtis similarity
The first step in the analysis of multivariate data was the calculation of a similarity measure between the samples. The similarities between all pairs of samples (the similarity matrix) were then used for a number of analyses. The Bray–Curtis similarity coefficient is the most common measure for comparing ecological samples with species abundance data. The Bray–Curtis measure is independent of scale of measurements (counts, biomass etc.) and joint absences of variables in a pair of samples have no effect on the similarity between them. For two samples j and k the Bray–Curtis similarity is described by
where yij and yik are the abundance of the ith variable in the jth sample and kth sample respectively and p is the total number of variables. The Bray–Curtis dissimilarity is then simply represented as 100 − Sjk.
Non-metric multidimensional scaling
Ordination plots visually display the similarity between ecological samples by mapping the high-dimensional community structure to two or three dimensions such that the physical distance between samples on the plot reflects the similarity between their communities.
In an NMDS ordination plot, the distances between the samples (in this case genomes or metagenomes) are first calculated using complete profiles of occurrence of the variables (in our case the iron metabolism genes). The sample objects are then placed randomly in a 2-d space and the Euclidean or physical distance between the objects in 2-d is calculated. This distance matrix is then non-parametrically regressed on to the original distance matrix to calculate a stress value (goodness-of-fit of the regression) that gives an indication of the best fit between the two matrices. The samples are then iteratively rearranged such that the stress value is minimized. The NMDS plot thus is a 2-d representation of the distances between the samples in a high-dimensional space. The distance between two genomes in such an ordination diagram gives an indication of the similarity of their gene profiles. A stress value less than 0.2 combined with an overlay of pre-defined group names provides reliable inferences about the clustering of the samples.
Analysis of similarities
The ANOSIM test is the non-parametric multivariate analog of the Analysis of Variance tests for univariate, normally distributed data. Instead of the group means as in the univariate case, here only the rank similarities between the samples in the underlying similarity matrix are considered. For n samples having replicates for two or more categories (in our case the taxonomic or ecological niche groups) a test statistic R is calculated as follows
where is average of rank similarities in the replicates within a category, is the average of rank similarities among all pairs of replicates between the categories, M = n(n − 1)/2 and n is the total number of samples. The statistical significance of the observed R value is evaluated using the null hypothesis H0 that there are no differences between the groups of samples. This is accomplished by a permutation test where all the group labels are sequentially applied to all the samples and the R statistic recalculated for each permutation. The null hypothesis H0 is rejected if the observed R value lies outside of the distribution of R values from the permutation test. For instance if t R values of the T total permutations are greater than or equal to the observed R value then we can reject H0 at a significance level of (t + 1)/(T + 1). This is what is referred to as the Global R test, i.e., between all the groupings of the samples. Pairs of the groups were also similarly compared to each other in terms of the R statistic and its significance value. Following convention, we tolerated a significance value of up to 5% (Type I error, i.e., rejecting the null hypothesis when it is true) as being small enough to rule out the possibility of H0 being true.
Similarity percentages
This method disaggregates the Bray–Curtis similarity matrix in order to identify the species that contribute most to the differences (average dissimilarities) between the prior groupings of the samples. For two samples j and k SIMPER calculates the contribution for the ith species as follows:
The terms yij and yik are defined as before for Eq. (1). The average contribution of the ith species to the overall dissimilarity is just the average δjk(i) for all pairs (j,k) such that j is from the first group and k is from the second group. If is high and the standard deviation SD (δi) of the δjk (i) values is low, it implies that this species i has a significant contribution to the overall dissimilarity in a majority of pairwise comparisons between the two groups. A high ratio therefore means that species i is a good discriminator.
Principal components analysis
Principal components analysis is an ordination where the high-dimensional data is represented in terms of two or three orthogonal axes (Principal Components). The procedure involves finding a linear combination of the original variables (first PC) such that the variance of the sample points projected perpendicularly on this new axis is maximized. The second PC is restricted to be perpendicular to the first PC and again chosen in the direction that maximizes the variance of the sample points and so on. The percentage of the variance explained by the first three PCs gives an idea about the loss of information resulting from reducing the dimensions. The variable vectors can be plotted on top of the PCA ordination to visualize the directions of the variable gradients.
Phylogenetic and Positive Selection Analysis
The 16S rRNA gene sequences for the genomes were retrieved from GenBank along with the E. coli 16S rRNA sequence. These were aligned using MUSCLE and imported into the ARB software (Ludwig et al., 2004). A Maximum Likelihood tree was calculated with the FastDNAML (Olsen et al., 1994) implementation in ARB using a filter for base 800 to base 1300 encompassing and extending on both sides, the v6 hypervariable region in E. coli. An in-house script was used to calculate the average phylogenetic distance of a gene as follows:
where Dp(g) is the set of pairwise phylogenetic distances between all pairs of genomes where gene g occurs.
For the genes discriminating between taxa or locations, the nucleotide sequences were retrieved from GenBank and the Maximum Likelihood estimations of average pairwise non-synonymous by synonymous mutation (dN/dS) ratios were calculated using CodeML (runmode = −2) from the PAML package (Yang, 2007).
Results
A set of proteins involved in iron metabolism (Table 1) was recovered from 164 marine microbial genomes belonging to Cyanobacteria, eukaryotic phytoplankton, Alphaproteobacteria, Gammaproteobacteria, and Flavobacteria (Figure 1A) using HMMs with optimized thresholds and modified emission probabilities as described earlier (Srivastava et al., 2007). The heme biosynthesis system, the TonB/ExbB/ExbD system, ferredoxin, and the iron-sulfur cluster assembly protein Isca1 (Table 1) were present in almost all prokaryotic genomes and therefore removed from further analysis. The observed abundances of TBD Fe-siderophore uptake systems, components of Fe2+ or divalent cation uptake and Fe3+ transporters were in agreement with previous reports (Hopkinson and Barbeau, 2012). The TBD uptake systems for catecholate, hydroxamate, and citrate siderophores were more widespread in Gammaproteobacteria (60, 55, and 37% of the genomes, respectively) as compared to Alphaproteobacteria (24, 16, and 13%, respectively), Flavobacteria (13, 8, and 30%, respectively), and Cyanobacteria (2, 19, and 19%, respectively). Fe2+ or divalent cation transporters were abundant in all the taxa but were most abundant in the eukaryotic phytoplankton genomes (39%). Ferric reductase was characteristic of the eukaryotic phytoplankton group (71%), but was also present in Cyanobacteria (2%), Alphaproteobacteria (16%), and Gammaproteobacteria (10%). Fe3+ transporters occurred in Cyanobacteria (37%), Alphaproteobacteria (33%), and Gammaproteobacteria (27%), but were uncommon in Flavobacteria (2%) and absent from eukaryotic phytoplankton. NRPS and NIS components involved in siderophore biosynthesis were present in Alphaproteobacteria (14 and 16%), Gammaproteobacteria (14 and 39%), Flavobacteria (14 and 22%), Cyanobacteria (32 and 11%), and also in eukaryotic phytoplankton (24 and 11% respectively).
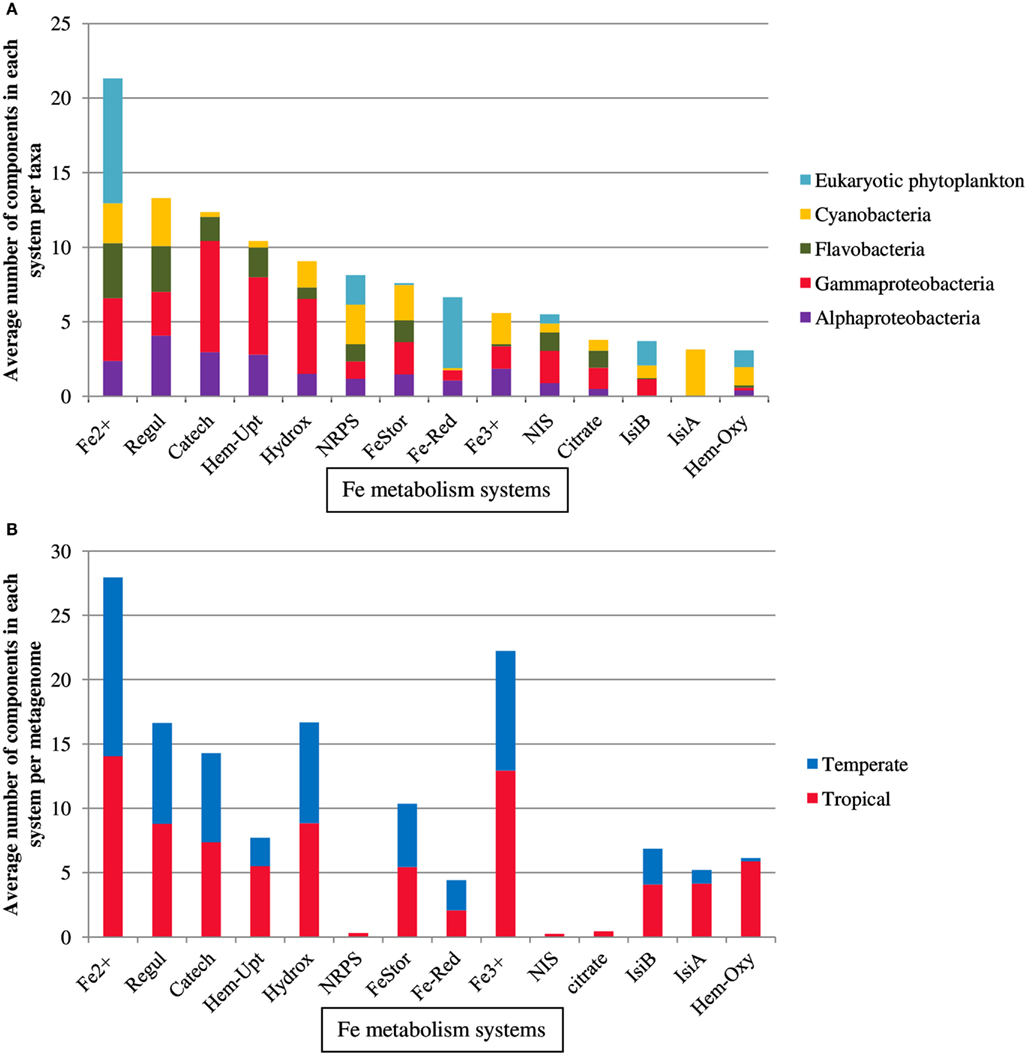
Figure 1. Distribution of components of Fe-metabolism systems in (A) marine microbial taxa and (B) Temperature groupings of metagenomes. For detailed description of the components of each system see Table 1.
Siderophore Biosynthesis Components in Phototrophic Genomes
Recent surveys involving searches of NIS components represented by PFAM domains AlcB (Acetyl transferase) and IucA_IucC (siderophore synthetase for Aerobactin) suggest that none of the eukaryotic phytoplankton and only around 4% of marine picocyanobacteria possess this system (Hopkinson and Morel, 2009; Hopkinson and Barbeau, 2012). Here our HMM search utilized a more extensive set of NIS proteins involved in the biosynthesis of aerobactin, desferrioxamine, and rhizobactin 1021 siderophores (Challis, 2005; Table 1). NRPS was detected in picocyanobacteria P. marinus MIT9303 and NRPS along with the NIS component RhbB (a PLP dependent decarboxylase) were detected in P. marinus MIT 9303 and MIT 9313. It is possible that the high specificity of our HMM-ModE models led to a slight drop in sensitivity. To confirm whether the other components of this pathway were indeed present in the phototrophic genomes and were being missed due to this lowered sensitivity of HMM-ModE, we used the Search Tool for Interacting Genes/Proteins (STRING) database (Szklarczyk et al., 2011). For a given query sequence, this database identifies a set of proteins that repeatedly co-occur with the query in the genomes of many different organisms. In addition to P. marinus MIT9303 and MIT9313, using the S. meliloti RhbB sequence as the query, the STRING database showed the co-occurrence of RhbB and RhbA (diaminobutyrate aminotransferase involved in rhizobactin biosynthesis) in P. marinus CCMP1375, NATL1A, CCMP1986, MIT9211, MIT9515, MIT9215, MIT9312, NATL2A, AS9601, and MIT9301. A corresponding siderophore uptake gene was not detected in the Prochlorococcus genomes. Our profiles detected a putative gene for NRPS in eukaryotic phytoplankton A. anophagefferens, E. huxleyi, O. tauri, P. tricornutum, and T. pseudonana, and the NIS component RhbB in E. huxleyi, F. cylindrus, and O. lucimarinus. Using the STRING database we detected genes similar to rhizobactin biosynthesis components RhbA and RhbB in O. tauri, O. lucimarinus, T. pseudonana, and P. tricornutum along with RhtX, a special permease involved in the uptake of rhizobactin 1021. The sequences identified as RhbA, RhbB, and RhtX from these genomes shared 48.18, 35.48, and 30% identity at the protein level within each group, respectively. A neighbor-joining tree calculated from the multiple sequence alignments of these sequences showed a higher similarity of the Freshwater organisms with the Sinorhizobium genes while the eukaryotic sequences clustered with the Prochlorococcus sequences (Figure 2). We also detected the NRPS as well as NIS components in the metagenomes, though their abundances were low (Figure 1B).
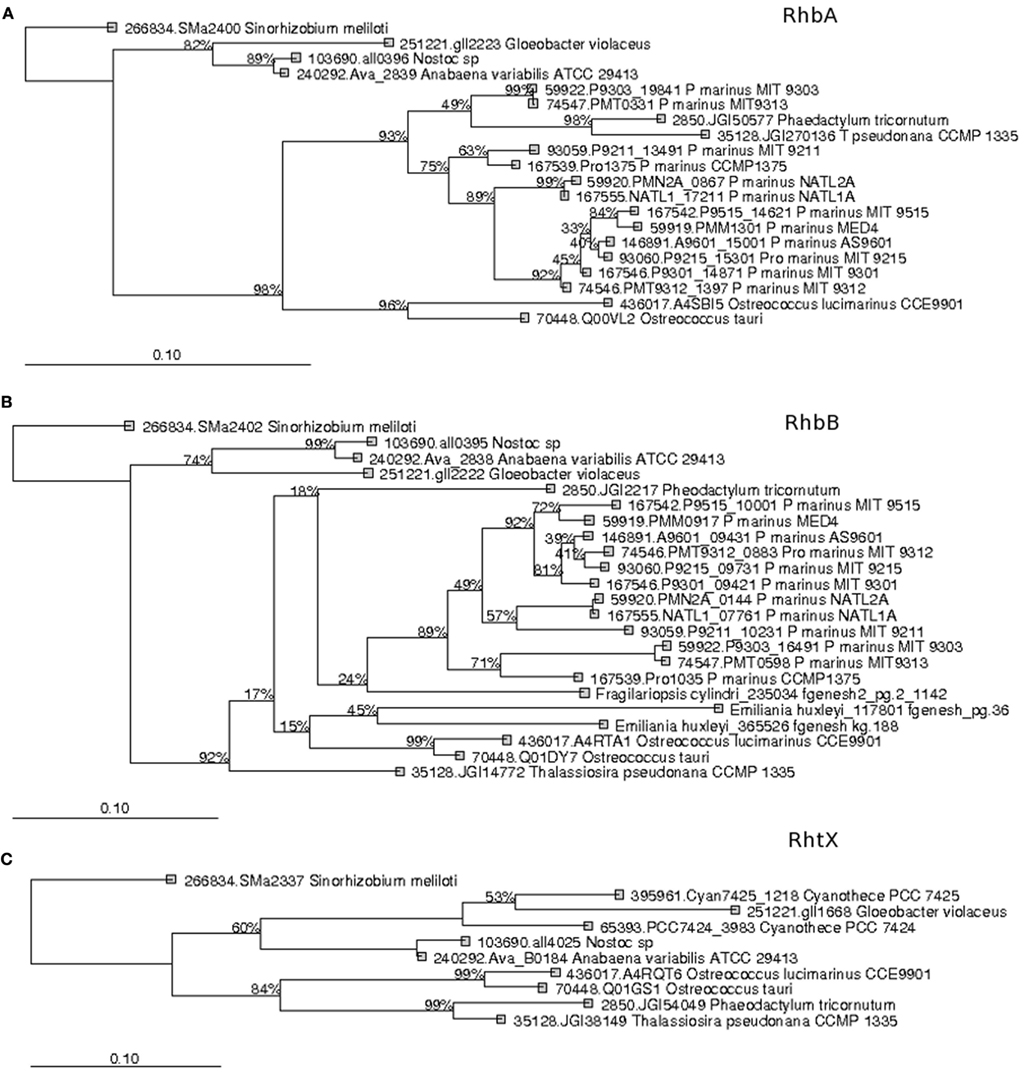
Figure 2. Phylogenetic trees of (A) RhbA, (B) RhbB, and (C) RhtX protein sequences from Open Ocean picocyanobacteria and eukaryotic phytoplankton recovered in our study. The tree was constructed using a Neighbor-Joining method, using the Jukes–Cantor correction and a bootstrap test was conducted with 1000 replicates. The scale bar represents 100% estimated sequence divergence.
Analysis of Genomes in Terms of Their Taxonomic Affiliation
We generated occurrence matrices showing the abundance of Fe-metabolism proteins (variables) in each genome or metagenome (samples) and used NMDS plots to visualize the clustering of the samples based on similarities of their gene occurrence profiles. The distance between the sample points on a NMDS plot is indicative of the extent to which samples share species (or proteins in this case). We grouped the individual genomes according to either their taxonomic class or to their ecological niches and applied a multivariate non-parametric test (ANOSIM) to check for differences in distribution of the Fe uptake systems across these groupings. For groups that showed a significant difference (a positive ANOSIM R value with significance <5%) in the type and frequencies of occurrence of Fe uptake systems, the SIMPER method was used to identify the proteins that contributed the most to this difference (see Materials and Methods for details). The taxonomic groups took into account the heterotrophic genomes, comprised of Alphaproteobacteria, Gammaproteobacteria, and Flavobacteria, and the phototrophic genomes consisting of picocyanobacteria (Synechococcus and Prochlorococcus), other-Cyanobacteria (Cyanobacteria excluding picocyanobacteria), and the eukaryotic phytoplankton. The genomes were grouped into niches based on only the isolation location of the source organism, e.g., Open Ocean, Coastal, or Freshwater (Table S1 in Supplementary Material).
The NMDS plot of 110 heterotrophic genomes showed three distinct clusters corresponding to the three taxa (Figure 3A). The differences in Fe-metabolism systems among the three groups were statistically significant (Table 2). The greatest diversity of TBD hydroxamate/catecholate siderophore and heme uptake components, and occurrence frequency of bacterioferritin and NIS biosynthesis component RhbB, as identified by SIMPER, was seen in Gammaproteobacteria (Table 3). The Alphaproteobacteria genomes had the highest occurrence frequencies of Ferric reductase, the Zinc uptake protein ZupT (free Fe2+and other divalent cations), and FbpA (Fe3+ transporter component) as well as regulatory elements Fur and RirA. The ferric citrate uptake protein FecA, the FeoAB proteins (Fe2+ uptake), and Ferritin were amongst the most abundant in Flavobacteria and infrequent in the other two groups of heterotrophic bacteria. The regulatory element DtxR was only present in Flavobacteria and absent in Alphaproteobacteria and Gammaproteobacteria.
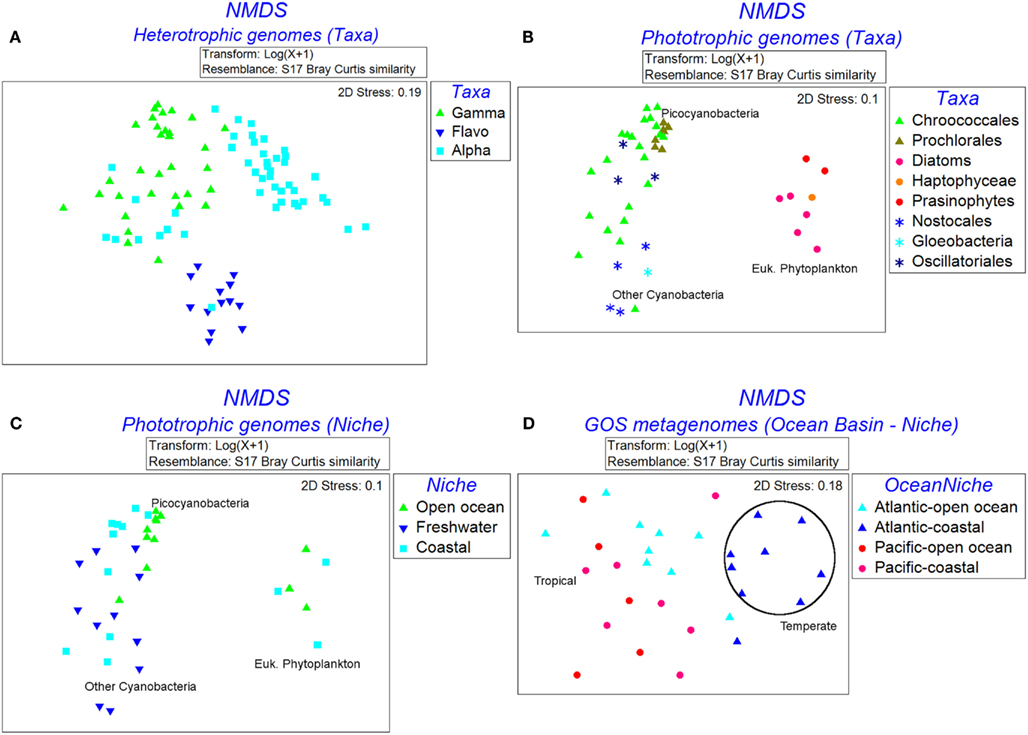
Figure 3. Non-parametric multidimensional scaling plots showing the clustering of genomes and metagenomes based on the similarities of occurrence patterns of Fe uptake systems. (A) Heterotrophic genomes labeled by taxa, (B) phototrophic genomes labeled by taxa, (C) phototrophic genomes labeled by niche, and (D) GOS metagenomes labeled by marine niche groups.
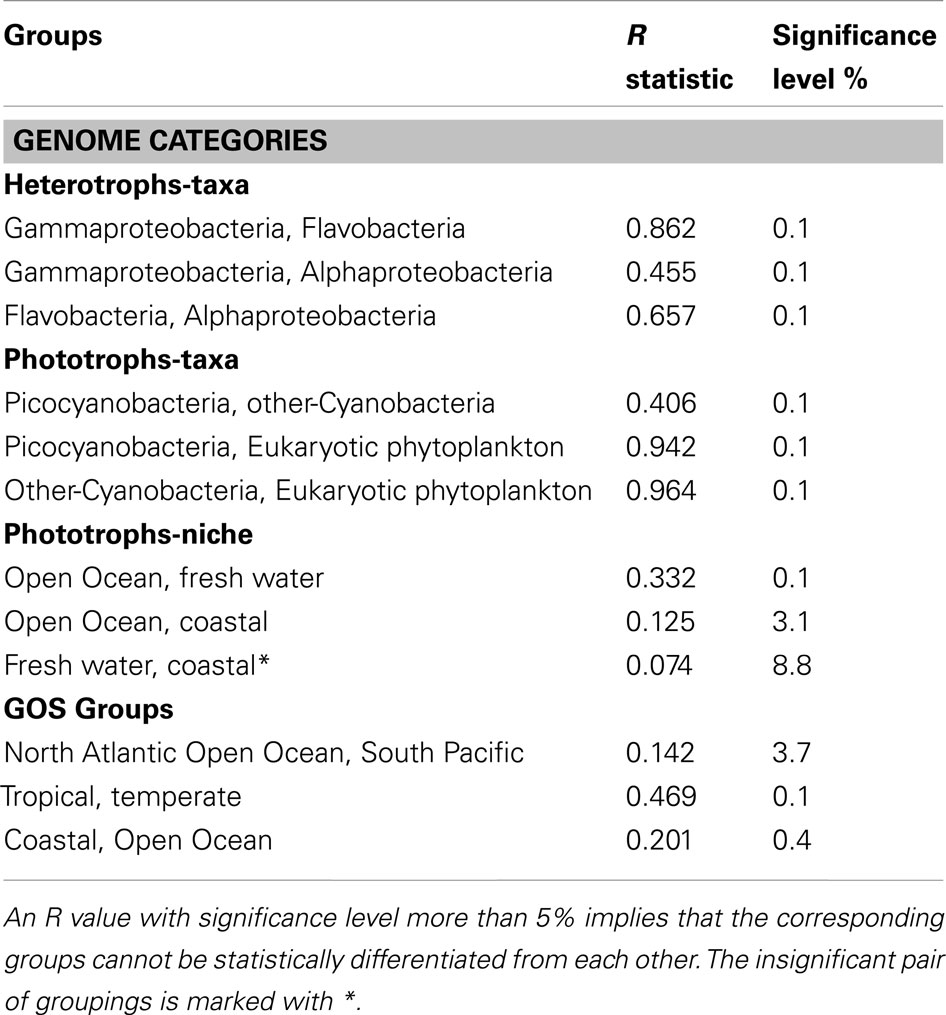
Table 2. Statistical comparison (ANOSIM) of Fe-metabolism systems in various groupings of genomes and metagenomes.
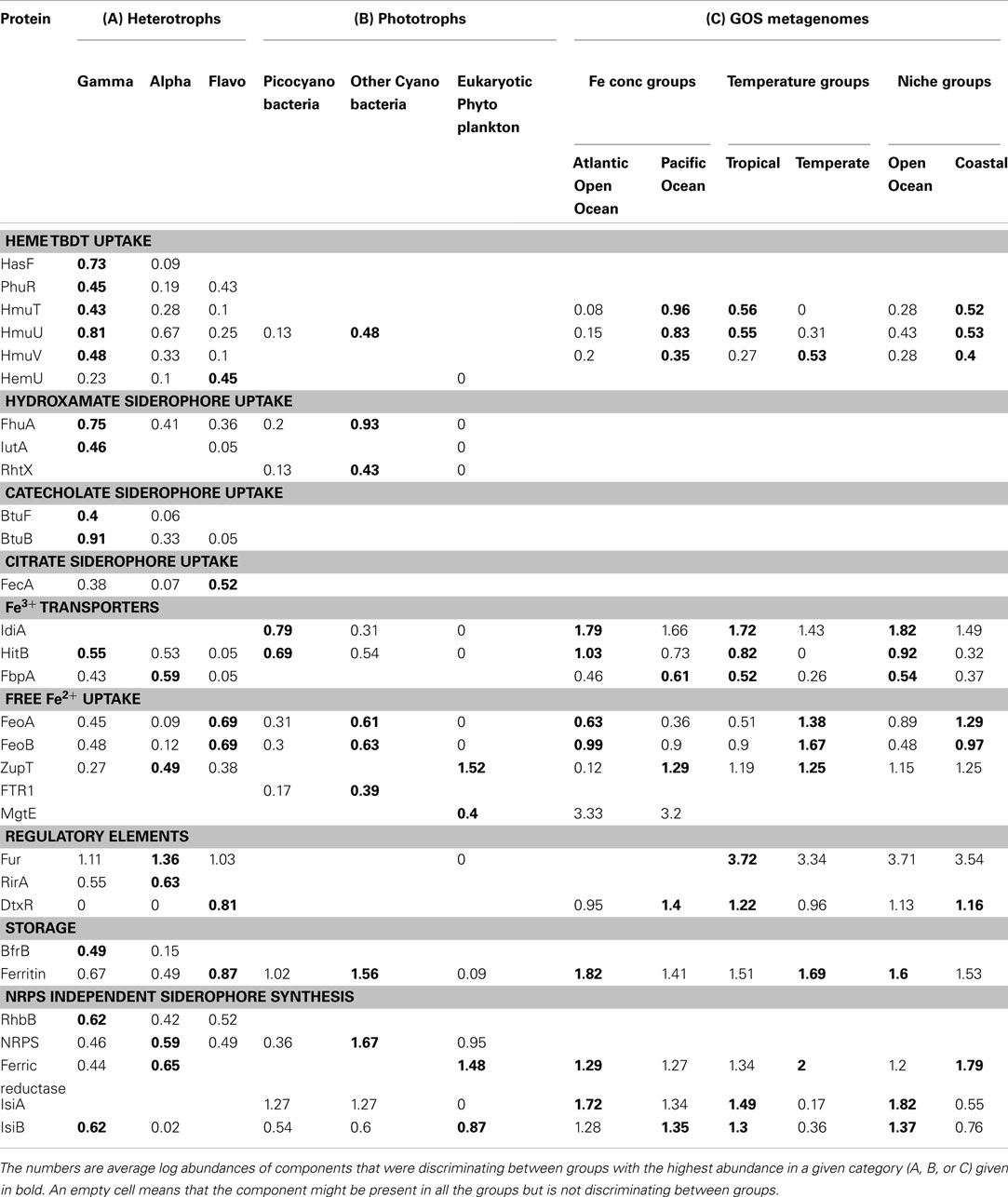
Table 3. Fe-metabolism protein components discriminating between the three major taxonomic groups of heterotrophs (A) and phototrophs (B) and groupings of the GOS metagenomes (C) identified using the Similarity Percentages (SIMPER) method.
Figure 3B shows the NMDS plots labeled by taxonomy for the 54 phototrophic genomes. The Cyanobacteria and eukaryotic genomes formed separate clusters. Within the cyanobacterial cluster the picocyanobacteria, which had the highest number of representative genomes, and other-Cyanobacteria formed sub-clusters. The phototrophic genomes showed a statistically significant difference between the eukaryotic phytoplankton, picocyanobacteria, and other-Cyanobacteria groups (Table 2). The Fe uptake components which showed a marked difference in abundances across the phototrophic genome groups are given in Table 3. The picocyanobacteria were characterized by the periplasmic Fe+3 transport components IdiA and HitB which were infrequent in the other-Cyanobacteria and not detected at all in the available eukaryotic phytoplankton genomes (Table 3). On the other hand, GTP driven Fe2+ uptake components FeoAB, FTR1, and hydroxamate uptake components FhuA and RhtX were largely absent from picocyanobacteria and eukaryotic phytoplankton but were present in the other-Cyanobacteria group. Similarly, high occurrence frequencies of the Zinc transporter ZupT, Ferric reductase, IsiB, and NRPS were characteristic of the eukaryotic phytoplankton as compared to the Cyanobacteria.
Analysis of Genomes in Terms of Their Ecological Niches
The Open Ocean niche group of the phototrophic genomes (Figure 3C) was significantly different from the Coastal and Freshwater groups (Table 2). We observed that the TBD siderophore/heme uptake components as well as the Feo system for Fe2+ uptake were most widespread in the Freshwater niche, relatively less common in Coastal niche and rarely represented in the Open Ocean niche (Table 4). FTR1 (direct Fe2+ uptake), ferritin, HO1 (heme oxygenase), and NRPS were abundant in Freshwater niche. The Fe3+ transporter components (IdiA and HitB) were evenly present in all the three niches. The NIS biosynthesis protein RhbB was most abundant in the Coastal niche. The Open Ocean niche had a greater abundance of IsiA and IsiB than both other niches. The niche groups in the heterotrophic genomes were not distinguishable from each other in terms of the frequencies of iron uptake genes (Table 2).
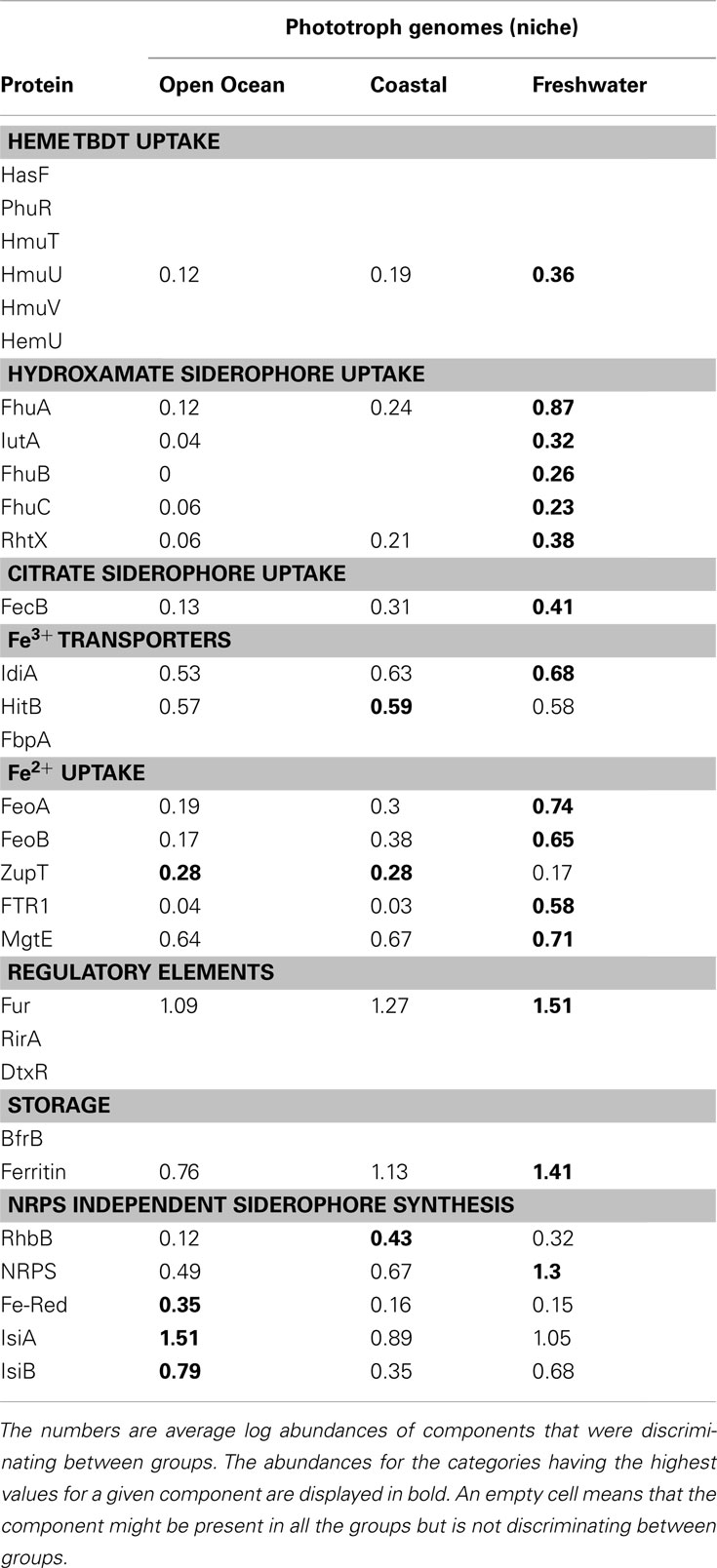
Table 4. Proteins discriminating between aquatic niche groups in phototrophic genomes identified using the Similarity Percentages (SIMPER) method.
Environmental Characterization of the GOS Metagenomes
Figure 4 shows the GOS sample locations overlayed on the annual mean surface bioavailable dFe from the PELAGOS model (Vichi et al., 2007a,b). This model couples the biogeochemical fluxes with global ocean general circulation models. The iron dynamics in PELAGOS include fluxes for uptake of bioavailable Fe by phytoplankton, loss by turnover/cell lysis, and predation. The only external function that forces these fluxes is the monthly deposition of atmospheric Fe and a dissolution fraction of the Fe dust which is set at 1%. We used the surface dFe concentration predictions from the PELAGOS model to characterize the aquatic niches. Since we were using model-derived dFe concentrations in place of actual observations, we compared the dFe concentrations used in this study with those used in Toulza et al. (2012). For the 22 GOS samples where dFe concentration predictions from both of these models were available, an R2 of 0.849 in a linear regression of the dFe values showed a good correlation between the outputs of the two models (Table S2 in Supplementary Material). There was a marked difference in the surface dFe concentration in samples collected from the Atlantic and the Pacific basins. However, apart from the dFe differences in the niches (Open Ocean or Coastal) defined in Toulza et al. (2012), the spatial distribution of the samples across a wide range of latitudes also resulted in a separation along a temperature gradient (Temperate vs. Tropical) (Rusch et al., 2007).
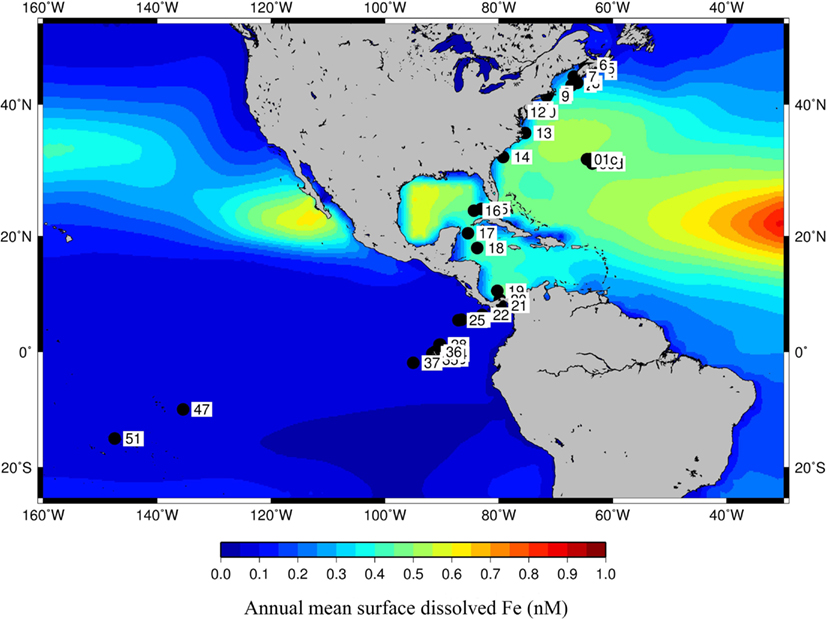
Figure 4. Coordinates of GOS metagenome samples overlayed on dissolved Fe concentrations as predicted by PELAGOS model.
The environmental matrix for the 30 GOS samples belonging to the Open Ocean and Coastal niche groups was subjected to a PCA (Figure 5). The Atlantic and Pacific samples were separated along the first PC, where the environmental parameters accounting for the separation were Longitude, Latitude, and Temperature. Within each ocean basin group (Atlantic or Pacific), along the second PC, the water column depth gradient separated the samples into Open Ocean and Coastal groups. The dFe gradient separated the Atlantic Open Ocean samples from the Pacific Open Ocean and Coastal samples (Figure 5). The first PC, which included the temperature gradient in addition to the dFe, also separated the samples into Temperate and Tropical groups (Figure 5).
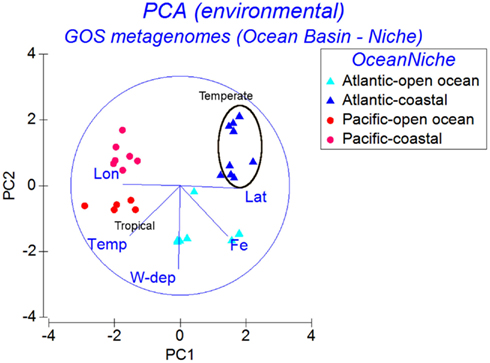
Figure 5. Principal Components Analysis of GOS samples using similarities of the environmental parameter profiles. The samples are labeled according to the ocean basins (Atlantic or Pacific) combined with niche groups (Open Ocean or Coastal).
To further understand the interaction between dFe and temperature and to define meaningful niche groups with contrasting parameters, we compared the median and range of the dFe concentrations as well as the temperatures (suitably transformed as described in the methods) for different group of GOS samples (Figures 6A,B respectively). There was a significant difference in dFe concentrations in the Pacific and Atlantic samples (single factor ANOVA, p value = 6.31E−09), and their median temperature difference was also significantly different (p value < 0.0005). The most noticeable difference of Fe concentrations was seen in the Atlantic Open Ocean samples and Pacific (Open Ocean and Coastal) samples (p value 1.3E−11) whereas the corresponding temperature difference between these groups was not significant (p value 0.27 at α = 0.01). Conversely, the Temperate, Coastal samples were primarily separated from the Tropical (Open Ocean and Coastal) samples by a temperature difference (p value 2.1E−07) with no difference in the corresponding average Fe concentrations (p value 0.3 at α = 0.01). Consequently, only these three pairs of groups (Atlantic Open Ocean vs. Pacific, Temperate vs. Tropical, and Open Ocean vs. Coastal) were further analyzed for the differences in their taxonomic profiles and their associated Fe-metabolism systems.
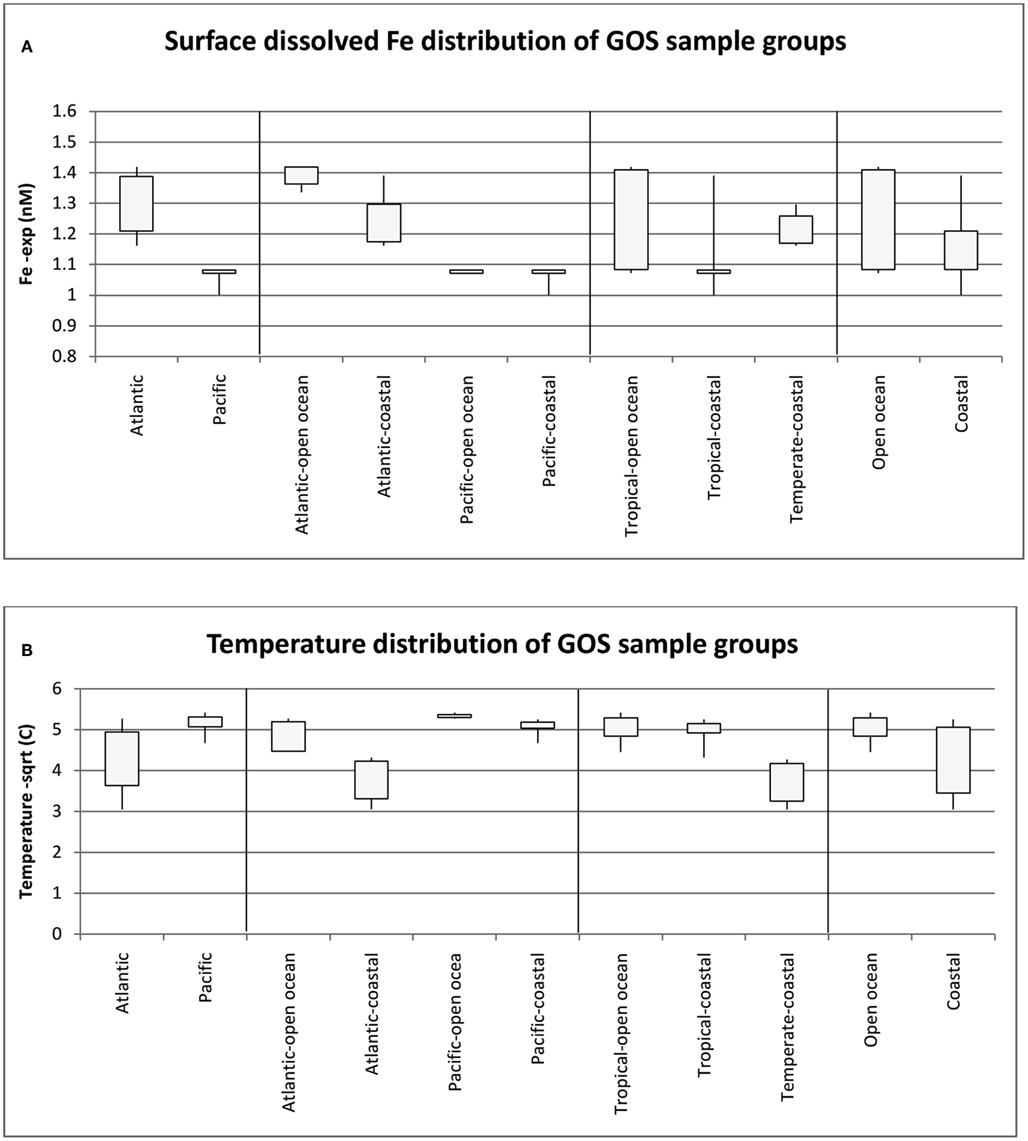
Figure 6. Median and range of dissolved Fe concentrations (A) and Temperature (B) for the GOS metagenome sample groups used in the study. Numbers of samples in the groups are as follows: Atlantic (18), Pacific (12), Atlantic Open Ocean (9), Pacific Open Ocean (5), Pacific-Coastal (7), Tropical-Open Ocean (11), Tropical-Coastal (11), Temperate-Coastal (8), Open Ocean (14), Coastal (16).
Taxonomic Distribution and Fe-metabolism Components Represented in the GOS Metagenome Groups
The taxonomic profiles (the occurrence of sequences from various taxonomic groups) of the aforementioned GOS metagenome groups were obtained from MG-RAST (Meyer et al., 2008) using an E-value cut-off of 1E−15, minimum percent identity 50, and minimum alignment length 100. Using the set of sequences with a clear taxonomic identification we further processed the data to obtain the percentage contribution of each taxonomic group in the metagenomic groupings (Table 5). The Fe-metabolism protein frequency matrix was analyzed for differences in Fe-metabolism components across the GOS metagenomic groupings. The NMDS plots labeled by Ocean Basin – Niche categories as well as the Temperature – Niche groups are shown in Figure 3D. The ANOSIM tests for all these groups were significant (Table 2), suggesting that the distribution of Fe uptake system components was different. However, based on the environmental characterization (see the section above), we selected only the samples from Tropical vs. Temperate and Atlantic Open Ocean vs. Pacific for further analysis with SIMPER along with the previously defined Open Ocean vs. Coastal samples (Toulza et al., 2012). Differences in gene abundances between these groups are given in Table 3. The average abundance of the heme uptake machinery HmuTUV was higher in the Pacific, Tropical, and Coastal groups whereas the Fe3+ uptake (IdiA, HitB) and IsiA had a higher representation in the Atlantic Open Ocean (compared to the Pacific), Tropical (compared to Temperate), and Open Ocean (compared to Coastal) groups. The Fe2+ uptake components (FeoAB) and Ferric reductase were more abundant in Atlantic Open Ocean, Temperate, and Coastal groups. The Flavodoxin protein IsiB and heme oxygenase HO1 had a higher average abundance in the Pacific, Tropical, and Open Ocean groups. While ZupT (zinc uptake protein) was more abundant in Pacific, Temperate, and Coastal groups, the storage protein ferritin had a higher abundance in Atlantic Open Ocean, Temperate, and Open Ocean groups.
Phylogenetic Spread and Non-Synonymous Mutation Rate of Selected Genes
The nucleotide sequences of some of the abundant genes, extracted from the genomes, were analyzed for the rate of non-synonymous mutations. Because non-synonymous mutations result in amino acid replacement, they are often eliminated by purifying selection, a form of natural selection that selectively removes deleterious mutations. Under certain selection pressures, non-synonymous mutations might be retained when they are advantageous (known as positive selection). The dN/dS ratio therefore provides a measure of the selection pressure operating on a gene. The dN/dS ratio for some of the genes was plotted (Figure 7) along with their average phylogenetic spread (the average phylogenetic distance among the genomes possessing the gene, calculated from a Maximum Likelihood tree of 16S rRNA gene sequences of the genomes). Ferric reductase, feoA, feoB, idiA, and the zinc uptake gene zupT, tonB, and ferritin genes all had a dN/dS value >1, indicating that non-synonymous mutations were possibly beneficial for these genes and that they were evolving rapidly under positive selection. With the exception of the idiA gene, all of these genes also had a wide phylogenetic spread indicating that they were present in a wide range of taxonomic groups (Figure 7). The remaining genes analyzed had a dN/dS ratio <1 (28 out of 35), indicating that they were under purifying selection pressure. All proteins involved in siderophore biosynthesis or high-affinity uptake systems for hydroxamate or catecholate siderophore/heme or vitamin B12 were undergoing purifying selection. The regulatory element fur, fbp-family gene fbpA, hitB, isiA, isiB, and the Mg2+ transporter mgtE were also included in the category of purifying selection and, with the exception of isiA, retained a wide phylogenetic spread among the marine genomes.
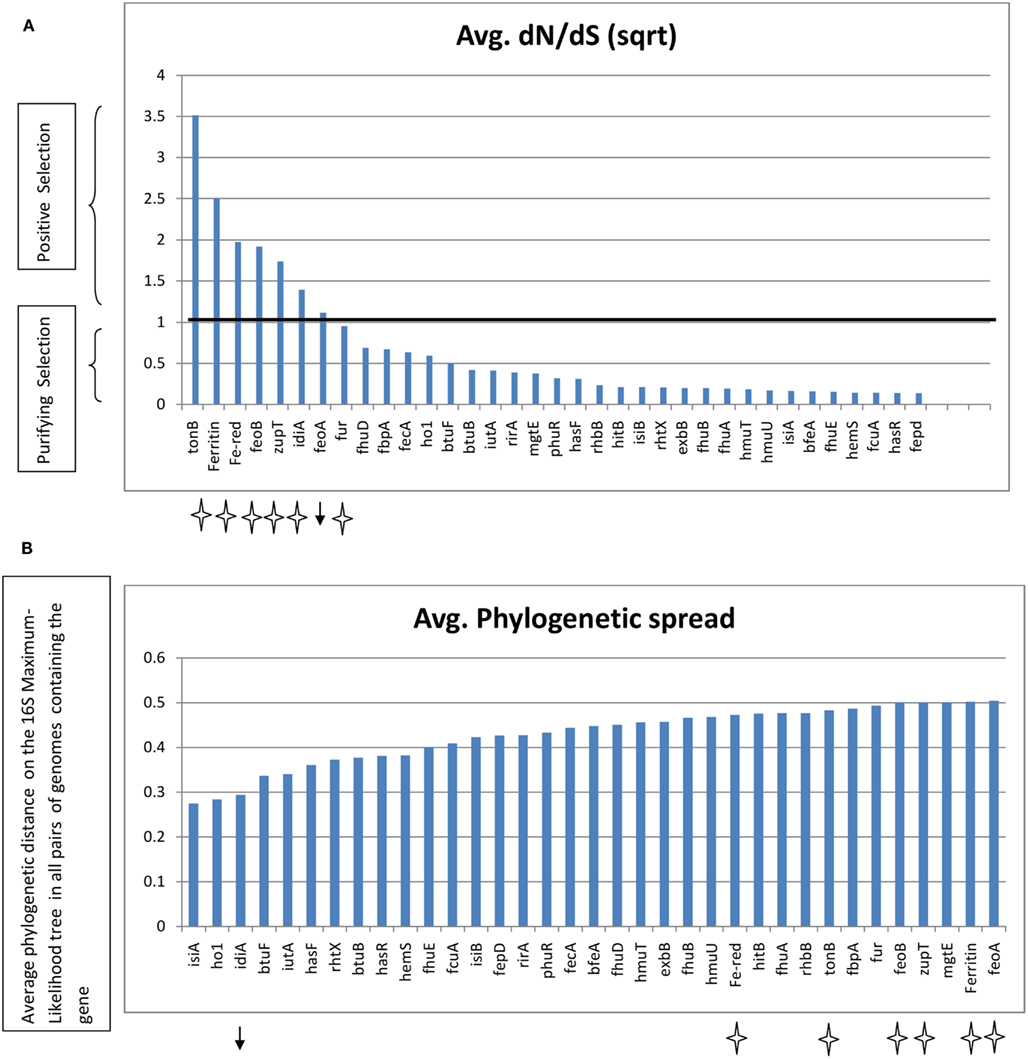
Figure 7. (A) Average dN/dS ratios of selected genes. The genes are sorted by highest dN/dS value. (B) Phylogenetic spread as defined by maximum phylogenetic distance among the genomes possessing the gene. The black line marks the dN/dS value = 1. Genes with dN/dS >1 and a wide phylogenetic spread are marked with stars. Genes with dN/dS >1 and a narrow phylogenetic spread are marked by an arrow. Fe-red – Ferric reductase.
Discussion
Multivariate Approach Using Functional Gene Specific HMMs
Hidden Markov models of protein families or folds have been routinely used in genome wide studies of protein functions such as metal binding capabilities (Dupont et al., 2006, 2010) or Fe transport (Hopkinson and Barbeau, 2012). Some Fe uptake proteins have multiple domains, which could be shared among different functional classes. For example, the TonB-box is a conserved motif which is common to all TBD receptors binding to different substrates (hydroxamate, catecholate, heme, or citrate). The GTP-binding domain of FeoB which is conserved and involved in various other functions could also lead to increased FP, especially in the metagenomic sequences (Hopkinson and Barbeau, 2012). It has been shown earlier that the specificity of the identification of function at the substrate binding level can be increased by modifying the HMM using information from the negative training sequences (i.e., sequences of the same fold or family but having different substrate binding function) (Srivastava et al., 2007; Desai et al., 2011). We constructed profile HMMs of Fe-metabolism proteins covering most known Fe uptake systems (Table 1), using the HMM-ModE protocol (Srivastava et al., 2007) to increase the specificity at the substrate binding level. Our finding that TBD hydroxamate uptake components are relatively abundant in the metagenomes (Figure 1B) was in agreement with the fact that hydroxamate siderophores are abundant in seawater and constitute upto 5% of the dFe concentration in the Atlantic Ocean (Macrellis et al., 2001; Mawji et al., 2008; Velasquez et al., 2011; Gledhill and Buck, 2012).
Our method also provided a wider range of components to search for in the genomes and metagenomes as demonstrated by the hits obtained for the proteins RhbA (diaminobutyrate-2-oxoglutarate aminotransferase) and RhbB (L-2,4 diaminobutyrate decarboxylase) that suggest the presence of some of the components for rhizobactin siderophore biosynthesis in Cyanobacteria and eukaryotic phytoplankton genomes (Table S1 in Supplementary Material). However, the confirmation of siderophore biosynthesis pathways in these organisms will depend in part on the positive identification of the remaining genes for the many necessary components that could not be detected in these genomes using the HMMs and the STRING database as input for the search. Also, the homologs of RhtX detected in the eukaryotic genomes from the STRING database, were mostly annotated as Acetyl CoA transporters and had only a weak similarity with the S. meliloti RhtX protein (Tables S3–S5 in Supplementary Material), making their identification tenuous without supportive evidence. Siderophore biosynthesis has been reported in some Synechococcus species (Wilhelm and Trick, 1994; Ito and Butler, 2005; Hopkinson and Morel, 2009) and predicted to be present in the prasinophyte O. lucimarinus (Palenik et al., 2007) suggesting that they may be more widespread than originally thought. Recent reports have shown the production of Fe-binding ligands by microbial communities dominated by diatoms under Fe-depleted conditions with a distinct correlation between Fe-binding ligand concentration and diatom growth (Buck et al., 2010; King et al., 2012).
The increased specificity (and the related sensitivity drop) of the HMM search implies that we might miss identifying Fe-metabolism components in the genomes and metagenomes and our bottom-up approach (starting from known sequences) would prevent us from discovering novel or highly diverged forms of these Fe uptake systems. Also, for the metagenomes, since we sampled equal numbers of sequences, the reduced search space led to the under-representation of some Fe-metabolism systems. For example, even though the hydroxamate TBD uptake systems turned up as discriminating between the genome groups, they were not detected in sufficient numbers or discriminating between metagenome groupings (Table 3). To see if this under-representation was a result of the method and the reduced search space, we scanned all the sequences in the metagenomes with our profiles (Figure 1B). We did find components of hydroxamate, catecholate, and citrate siderophore uptake along with heme degrading oxygenases in all the groups of samples. However, since our aim was to compare samples from different locations, it was important to minimize the effect of sequencing effort (Gilbert et al., 2010), and so, all our comparisons were performed with the sampled metagenome sequence data.
Multivariate analysis is increasingly being applied in microbial ecology studies, for example, to trace the seasonal variation in bacterial communities (Gilbert et al., 2010), compare communities from different niches (Dinsdale et al., 2008) or investigate the correlation of environmental factors with the observed community structure and function in the GOS metagenomes (Gianoulis et al., 2009; Raes et al., 2011). The traditional concept of the species as a fundamental unit of biological diversity does not apply to prokaryotes. A new bacterial equivalent of a species (an ecotype) arises when a bacterial lineage starts utilizing a different set of resources for occupying a new ecological niche or microhabitat (Cohan, 2002). The bacterial genome has the capability to re-organize itself according to environmental cues in a given niche by mechanisms such as horizontal gene transfer (Thompson et al., 2011; Hopkinson and Barbeau, 2012) and hence, could be viewed as an assemblage of genes. In our analysis, we therefore used the profiles of abundances of the Fe-metabolism components (as variables or species that are subject to change) in the genomes or metagenomes (the ecological equivalent of samples) to calculate the similarities between pairs of samples, and further, between a priori groupings of the samples. Our multivariate analysis detected distinct patterns of co-occurrence in the groups (both genomes and metagenomes) including the co-occurrence of multiple components of the same system. For example, in most of the comparisons of the groups that we performed, all components of the heme uptake machinery HmuTUV, Fe3+ transporters, or the FeoAB system were reported as significant. Concomitantly, the characteristic occurrence patterns for each genome group (Figure 1) were also captured accurately. The reliance of picocyanobacteria and Alphaproteobacteria on Fe3+ transporters and their absence from Flavobacteria, or the widespread use of FeoAB based Fe2+ uptake by Flavobacteria and its absence from picocyanobacteria and Alphaproteobacteria are facts borne out by recent studies (Hopkinson and Barbeau, 2012) which were also apparent in our analysis. Thus, the multivariate analysis of uptake systems in genomes, using the genome as a unit of ecological treatment, validated our approach and demonstrated that it could be used to determine similar differences between the niche groups of genomes and metagenomes.
Environmental Enrichment of Fe Uptake System Components Correlates with the Distribution of Dominant Taxonomic Groups
The classification of a microbial genome as either Open Ocean or Coastal in our study was based on the location where they were isolated and does not preclude the possibility of it being present in other niches. However, the abundance of a plethora of Fe uptake systems along with both NIS and NRPS siderophore biosynthesis components in Freshwater and Coastal phototrophs potentially reflected the diversity of Fe-binding ligands in these niches as compared to the oligotrophic Open Ocean organisms where only the homeostasis proteins IsiA, IsiB, and Ferric reductase were abundant (Table 3). The GOS metagenomes provided an opportunity for the same comparison at the community level and also afforded a correlation of these differences to temperature, dFe concentration, and a distinction between Open Ocean and Coastal niches which were set apart from each other by water column depth (Figure 5). The SIMPER analysis demonstrated that certain genes were enriched in a particular niche, i.e., they had a distinct environmental signature.
A detailed analysis of the occurrence patterns of major microbial taxa in the same GOS metagenomic groupings (Table 5) was instructive of how the distribution of Fe-metabolism systems was effected by the environmental parameters. The Tropical metagenomes were dominated by SAR11 cluster and other Alphaproteobacteria (36.18% of total identified taxa) and picocyanobacteria (11.55%). These taxa were notably under-represented in the Temperate metagenomes (16.6 and 0.28% respectively). On the other hand the Temperate metagenomes were mainly composed of Flavobacteria (12% as compared to 6% in Tropical) and Gammaproteobacteria (39%; Table 5). The major differences in the Fe uptake systems between these groups, i.e., more Fe3+ transporters, IsiA, and IsiB in Tropical vs. more Fe2+ uptake, ferritin and Ferric reductase in Temperate were in accordance with the differences between the picocyanobacteria and Alphaproteobacteria on one hand and the Flavobacteria on the other (Table 3). The HmuTUV system, though decidedly more widespread in Gammaproteobacteria, is nonetheless present in Alphaproteobacteria genomes (Table 3). The combined contribution of Alphaproteobacteria and Gammaproteobacteria sequences is roughly the same in both groups (58.17 and 55.80% of all Tropical and Temperate sequences respectively; Table 5). We also compared just the coastal samples from the Tropical and Temperate zones (Table S2 in Supplementary Material) to remove the effect of Open ocean and Coastal locations and the results were unchanged. The impact of temperature on the taxonomic and functional diversity of the GOS samples is well established (Rusch et al., 2007; Raes et al., 2011). Here, we showed that temperature, potentially, also has an impact on the Fe uptake system distribution.
In the metagenomic groups separated by dFe concentrations, picocyanobacteria were well represented in the Atlantic Open Ocean (Fe-replete) samples whereas Alphaproteobacteria clades dominated the Fe-depleted Pacific samples. Again in this case, Fe uptake system distribution between the two groups (Table 3), i.e., more Fe3+ transporters, Fe2+ uptake, and IsiA in the Atlantic vs. more HmuTUV, FbpA, ZupT, and IsiB in the Pacific, was a reflection of the differences between corresponding dominant taxonomic groups (Table 5).
Niche specific adaptations and diversity in Fe uptake mechanisms among Cyanobacteria are well documented. For example, siderophore production and its associated receptor mediated uptake is more common in freshwater and coastal cyanobacteria but is not the preferred iron acquisition strategy in open ocean Cyanobacteria (Webb et al., 2001; Palenik et al., 2006; Hopkinson and Morel, 2009). Our results of the niche analysis of phototrophic genomes also showed this distinction (Table 4). Recent sequencing of various Synechococcus genomes points to the presence of Fe2+ uptake in coastal strains (Palenik et al., 2006) as an adaptation to the higher concentrations of bioavailable Fe2+ in coastal regions (Kuma et al., 1992). The upregulation of IdiA as a response to Fe limitation both in culture as well as in the Fe-limited open ocean is well known in Cyanobacteria (Rusch et al., 2010; Thompson et al., 2011). Additionally, presence of Fe3+ transporters, and lack of TBD Fe uptake systems in Candidatus Pelagibacter ubique, an open ocean alphaproteobacterium might also be a niche specific adaptation (Smith et al., 2010). In light of the above facts and because of possible differences in Fe-speciation (Boye et al., 2003; Buck and Bruland, 2007), niche specific adaptive differences in Fe-metabolism gene profiles were expected between the Open Ocean and Coastal groups. However since all the Temperate samples were also Coastal, some of the differences between Temperate and Tropical groups might, in essence, be reflected in the differences between the Coastal and Open Ocean niche groups. For example, the FeoAB system and Ferric reductase (over-represented in Temperate) were also more abundant in the Coastal group. Additionally, the Coastal group also had higher abundance of the HmuTUV. The Open Ocean group on the other hand had higher occurrence of Fe3+ transporters, IsiA, and IsiB (Table 3). Taxonomically, the Coastal group was again dominated by Alphaproteobacteria and Gammaproteobacteria together constituting around 55% of all identifiable sequences in the group. The Open Ocean group also had a similar proportion of the proteobacteria (roughly 52% of total). The Flavobacteria and picocyanobacteria were both over-represented in the Coastal group (Table 5). But within the picocyanobacteria the pelagic Prochlorococcus was more abundant in Open Ocean while the Chroococcales (Synechococcus spp., Synechocystis spp. etc) were almost six times more abundant in the Coastal group than in Open Ocean (data not shown). The over-representation of Fe3+transporters, IsiA, and IsiB in the Open Ocean could be explained by the abundance of Prochlorococcus while the FeoAB and Ferric reductase in the Coastal group could be due to the abundant Flavobacteria. In conclusion for each metagenomic group the Fe-metabolism component was representative of the taxonomic groups dominant in those metagenomic groups. The environmental niche defined the taxonomic dominance which in turn led to the enrichment of particular Fe uptake components in that particular niche.
Differential selection pressures on Fe responsive genes
The genes for some of the proteins which were discriminatory between the niches also showed evidence of positive selection pressure at the sequence level (Figure 7). Most of these fast evolving genes perform generalized functions, which could involve interacting with multiple ligands. Both idiA and fbpA bind Fe3+, but only idiA was under positive selection pressure while fbpA was under purifying selection pressure. However the phylogenetic spread of FbpA was high and occurrence of idiA was more or less confined to the Cyanobacteria. It has been postulated that Fe3+ transporters such as idiA interact with Fe bound organic complexes rather than with free Fe3+(Hopkinson and Barbeau, 2012). Hydroxamate siderophores have been detected throughout the Atlantic Ocean (Mawji et al., 2008), and it could be believed that by virtue of broadly specific Fe3+ transporter idiA or fbpA the Ópen Ocean cyanobacteria could make a variety of siderophores available to themselves. Similarly the non-specific nature of Ferric reductase mechanism (Schroder et al., 2003) might provide an edge to organisms by catering Fe bound to diverse ligands in an organically rich environment, as evident by our finding that Ferric reductase mechanism was relatively abundant in North Atlantic Open Ocean and Temperate (Coastal) groups of metagenomes. An exception is the abundance of TBD mechanisms in South Pacific group which is known to be oligotrophic. The components of the specialized TBD siderophore/heme uptake systems or the enzymes involved in siderophore biosynthesis, heme oxygenase etc were undergoing purifying selection and the phylogenetic spread was also low for many of them. This suggested that both, the design of the siderophore and the uptake machinery employed are species specific and not much variation at the amino acid sequence level is allowed in the proteins involved. In addition, because of the cost involved in the production of siderophore and the uptake machinery, when present they should confer a definite competitive advantage to the organism. The open ocean environment is highly diffusive and an organism in such a niche cannot benefit from the possession of Fe uptake systems specific for a particular Fe-binding ligand or production of siderophores (Hopkinson and Morel, 2009). This could explain our observation that the specialized TBD uptake systems (e.g., HmuTUV) were more represented in the nutrient rich Coastal niche than in the Open Ocean. These dN/dS calculations were performed using only the genomic sequences. Thus there is a possibility that the results obtained were biased by the high specificity of the HMMs and that the actual rates of evolution in environmental sequences may differ. However, the HMM-ModE protocol has a sensitivity of ∼90 and ∼96% specificity for annotating complete gene sequences in bacterial genomes (Desai et al., 2011). A recent study of evolutionary rates of genes from environmental populations of coastal Synechococcus spp. reported that around 98% of the genes evolved under purifying selection (Tai et al., 2011). Also, the relationship between selection pressure and dN/dS ratio is only valid over long evolutionary time scales when comparing the sequences of divergent species (Kryazhimskiy and Plotkin, 2008).
In this time of rapid change in global oceanic conditions, the selection mechanisms operating on the evolution of genes conferring adaptation to a particular oceanic habitat are continuously shaping the genetic composition of microbial communities. We defined aquatic ecological niches for the GOS metagenomes in terms of dFe concentrations and temperature and investigated the differences in distribution of the taxonomic groups as well as the Fe-metabolism systems between these niches. The distribution of the Fe uptake proteins correlated with the taxonomic distribution of the organisms that possessed these systems, suggesting a role for temperature and Fe in shaping the microbial community in these niches. The biological availability of Fe is complicated by the presence of diverse organic ligands that bind to it. The high demand and low bioavailability of Fe mean that it is an abiotic stressor driving the evolution of microbial Fe-metabolism. We calculated rates of non-synonymous mutations for a set of genes that were discriminating between the above mentioned niches which were distinct with respect to temperature, dFe concentrations, or Coastal and Open Ocean location, and inferred that genes that exhibited higher rates of non-synonymous mutations were the ones involved in non-specific uptake of Fe bound to diverse ligands. This indicated that in the highly diffusive, oligotrophic open ocean marine environment possession of Fe uptake strategies with broad specificities provides a competitive edge to the microorganisms.
Conflict of Interest Statement
The authors declare that the research was conducted in the absence of any commercial or financial relationships that could be construed as a potential conflict of interest.
Supplementary Material
The Supplementary Material for this article can be found online at http://www.frontiersin.org/Microbiological_Chemistry/10.3389/fmicb.2012.00362/abstract
Footnotes
References
Adhikari, P., Kirby, S. D., Nowalk, A. J., Veraldi, K. L., Schryvers, A. B., and Mietzner, T. A. (1995). Biochemical characterization of a Haemophilus influenzae periplasmic iron transport operon. J. Biol. Chem. 270, 25142–25149.
Benson, H. P., Boncompagni, E., and Guerinot, M. L. (2005). An iron uptake operon required for proper nodule development in the Bradyrhizobium japonicum-soybean symbiosis. Mol. Plant Microbe Interact. 18, 950–959.
Boyd, P. W., Watson, A. J., Law, C. S., Abraham, E. R., Trull, T., Murdoch, R., et al. (2000). A mesoscale phytoplankton bloom in the polar Southern Ocean stimulated by iron fertilization. Nature 407, 695–702.
Boye, M., Aldrich, A. P., Van Den Berg, C. M. G., De Jong, J. T. M., Veldhuis, M., and De Baar, H. J. W. (2003). Horizontal gradient of the chemical speciation of iron in surface waters of the northeast Atlantic Ocean. Mar. Chem. 80, 129–143.
Buck, K. N., and Bruland, K. W. (2007). The physicochemical speciation of dissolved iron in the Bering Sea, Alaska. Limnol. Oceanogr. 52, 1800–1808.
Buck, K. N., Selph, K. E., and Barbeau, K. A. (2010). Iron-binding ligand production and copper speciation in an incubation experiment of Antarctic Peninsula shelf waters from the Bransfield Strait, Southern Ocean. Mar. Chem. 122, 148–159.
Burnap, R. L., Troyan, T., and Sherman, L. A. (1993). The highly abundant chlorophyll-protein complex of iron-deficient Synechococcus sp. PCC7942 (CP43’) is encoded by the isiA gene. Plant Physiol. 103, 893–902.
Butterton, J. R., Stoebner, J. A., Payne, S. M., and Calderwood, S. B. (1992). Cloning, sequencing, and transcriptional regulation of viuA, the gene encoding the ferric-vibriobactin receptor of Vibrio cholerae. J. Bacteriol. 174, 3729–3738.
Cadieux, N., Bradbeer, C., Reeger-Schneider, E., Koster, W., Mohanty, A. K., Wiener, M. C., et al. (2002). Identification of the periplasmic cobalamin-binding protein BtuF of Escherichia coli. J. Bacteriol. 184, 706–717.
Challis, G. L. (2005). A widely distributed bacterial pathway for siderophore biosynthesis independent of non-ribosomal peptide synthetases. Chembiochem 6, 601–611.
Clarke, K. R. (1993). Non-parametric multivariate analyses of changes in community structure. Aust. J. Ecol. 18, 117–143.
Coale, K. H., Johnson, K. S., Fitzwater, S. E., Gordon, R. M., Tanner, S., Chavez, F. P., et al. (1996). A massive phytoplankton bloom induced by an ecosystem-scale iron fertilization experiment in the equatorial Pacific Ocean. Nature 383, 495–501.
Cope, L. D., Yogev, R., Muller-Eberhard, U., and Hansen, E. J. (1995). A gene cluster involved in the utilization of both free heme and heme:hemopexin by Haemophilus influenzae type b. J. Bacteriol. 177, 2644–2653.
Cornelis, P., and Bodilis, J. (2009). A survey of TonB-dependent receptors in fluorescent pseudomonads. Environ. Microbiol. Rep. 1, 256–262.
de Baar, H. J. W., Boyd, P. W., Coale, K. H., Landry, M. R., Tsuda, A., Assmy, P., et al. (2005). Synthesis of iron fertilization experiments: from the iron age in the age of enlightenment. J. Geophys. Res. Oceans 110, C09S16. doi: 10.1029/2004JC002601
Desai, D. K., Nandi, S., Srivastava, P. K., and Lynn, A. M. (2011). ModEnzA: accurate identification of metabolic enzymes using function specific profile HMMs with optimised discrimination threshold and modified emission probabilities. Adv. Bioinformatics. doi: 10.1155/2011/743782
Dinsdale, E. A., Edwards, R. A., Hall, D., Angly, F., Breitbart, M., Brulc, J. M., et al. (2008). Functional metagenomic profiling of nine biomes. Nature 452, U628–U629.
Duce, R. A., and Tindale, N. W. (1991). Atmospheric transport of iron and its deposition in the ocean. Limnol. Oceanogr. 36, 1715–1726.
Dupont, C. L., Butcher, A., Valas, R. E., Bourne, P. E., and Caetano-Anolles, G. (2010). History of biological metal utilization inferred through phylogenomic analysis of protein structures. Proc. Natl. Acad. Sci. U.S.A. 107, 10567–10572.
Dupont, C. L., Yang, S., Palenik, B., and Bourne, P. E. (2006). Modern proteomes contain putative imprints of ancient shifts in trace metal geochemistry. Proc. Natl. Acad. Sci. U.S.A. 103, 17822–17827.
Eddy, S. (1998). HMMER: Biological Sequence Analysis Using Profile Hidden Markov Models. Available at: http://hmmer.org
Edgar, R. C. (2004). MUSCLE: a multiple sequence alignment method with reduced time and space complexity. BMC Bioinformatics 5, 113. doi:10.1186/1471-2105-5-113
Enright, A. J., Van Dongen, S., and Ouzounis, C. A. (2002). An efficient algorithm for large-scale detection of protein families. Nucleic Acids Res. 30, 1575–1584.
Fecker, L., and Braun, V. (1983). Cloning and expression of the fhu genes involved in the iron(III)-hydroxamate uptake by Escherichia coli. J. Bacteriol. 156, 1301–1314.
Ferreiros, C., Criado, M. T., and Gomez, J. A. (1999). The neisserial 37 kDa ferric binding protein (FbpA). Comp. Biochem. Physiol. B Biochem. Mol. Biol. 123, 1–7.
Gall, M. P., Boyd, P. W., Hall, J., Safi, K. A., and Chang, H. (2001). Phytoplankton processes. Part 1: community structure during the Southern Ocean Iron RElease Experiment (SOIREE). Deep Sea Res. Part II Top. Stud. Oceanogr. 48, 2551–2570.
Gao, Y., Kaufman, Y. J., Tanre, D., Kolber, D., and Falkowski, P. G. (2001). Seasonal distributions of aeolian iron fluxes to the global ocean. Geophys. Res. Lett. 28, 29–32.
Gianoulis, T. A., Raes, J., Patel, P. V., Bjornson, R., Korbel, J. O., Letunic, I., et al. (2009). Quantifying environmental adaptation of metabolic pathways in metagenomics. Proc. Natl. Acad. Sci. U.S.A. 106, 1374–1379.
Gilbert, J. A., Field, D., Swift, P., Thomas, S., Cummings, D., Temperton, B., et al. (2010). The taxonomic and functional diversity of microbes at a temperate coastal site: a ‘multi-omic’ study of seasonal and diel temporal variation. PLoS ONE 5, e15545. doi:10.1371/journal.pone.0015545
Gledhill, M., and Buck, K. N. (2012). The organic complexation of iron in the marine environment: a review. Front. Microbiol. 3:69. doi:10.3389/fmicb.2012.00069
Gledhill, M., and van den Berg, C. M. G. (1994). Determination of complexation of iron(III) with natural organic complexing ligands in seawater using cathodic stripping voltammetry. Mar. Chem. 47, 41–54.
Grass, G., Franke, S., Taudte, N., Nies, D. H., Kucharski, L. M., Maguire, M. E., et al. (2005). The metal permease ZupT from Escherichia coli is a transporter with a broad substrate spectrum. J. Bacteriol. 187, 1604–1611.
Hannenhalli, S. S., and Russell, R. B. (2000). Analysis and prediction of functional sub-types from protein sequence alignments. J. Mol. Biol. 303, 61–76.
Homann, V. V., Edwards, K. J., Webb, E. A., and Butler, A. (2009a). Siderophores of Marinobacter aquaeolei: petrobactin and its sulfonated derivatives. Biometals 22, 565–571.
Homann, V. V., Sandy, M., Tincu, J. A., Templeton, A. S., Tebo, B. M., and Butler, A. (2009b). Loihichelins A-F, a suite of amphiphilic siderophores produced by the marine bacterium Halomonas LOB-5. J. Nat. Prod. 72, 884–888.
Hopkinson, B. M., and Barbeau, K. A. (2012). Iron transporters in marine prokaryotic genomes and metagenomes. Environ. Microbiol. 14, 114–128.
Hopkinson, B. M., and Morel, F. M. M. (2009). The role of siderophores in iron acquisition by photosynthetic marine microorganisms. Biometals 22, 659–669.
Hopkinson, B. M., Roe, K. L., and Barbeau, K. A. (2008). Heme uptake by Microscilla marina and evidence for heme uptake systems in the genomes of diverse marine bacteria. Appl. Environ. Microbiol. 74, 6263–6270.
Hunter, K. A., and Boyd, P. W. (2007). Iron-binding ligands and their role in the ocean biogeochemistry of iron. Environ. Chem. 4, 221–232.
Ito, Y., and Butler, A. (2005). Structure of synechobactins, new siderophores of the marine cyanobacterium Synechococcus sp. PCC 7002. Limnol. Oceanogr. 50, 1918–1923.
Ito, Y., Ishida, K., Okada, S., and Murakami, M. (2004). The absolute stereochemistry of anachelins, siderophores from the cyanobacterium Anabaena cylindrica. Tetrahedron 60, 9075–9080.
Jeanjean, R., Talla, E., Latifi, A., Havaux, M., Janicki, A., and Zhang, C. C. (2008). A large gene cluster encoding peptide synthetases and polyketide synthases is involved in production of siderophores and oxidative stress response in the cyanobacterium Anabaena sp. strain PCC 7120. Environ. Microbiol. 10, 2574–2585.
Jickells, T. D., An, Z. S., Andersen, K. K., Baker, A. R., Bergametti, G., Brooks, N., et al. (2005). Global iron connections between desert dust, ocean biogeochemistry, and climate. Science 308, 67–71.
Johnson, K. S., Gordon, R. M., and Coale, K. H. (1997). What controls dissolved iron concentrations in the world ocean? Mar. Chem. 57, 137–161.
Johnston, A. W. B., Todd, J. D., Curson, A. R., Lei, S., Nikolaidou-Katsaridou, N., Gelfand, M. S., et al. (2007). Living without Fur: the subtlety and complexity of iron-responsive gene regulation in the symbiotic bacterium Rhizobium and other alpha-proteobacteria. Biometals 20, 501–511.
Joshi, F. R., Kholiya, S. P., Archana, G., and Desai, A. J. (2008). Siderophore cross-utilization amongst nodule isolates of the cowpea miscellany group and its effect on plant growth in the presence of antagonistic organisms. Microbiol. Res. 163, 564–570.
Kammler, M., Schön, C., and Hantke, K. (1993). Characterization of the ferrous iron uptake system of Escherichia coli. J. Bacteriol. 175, 6212–6219.
Katoh, H., Hagino, N., Grossman, A. R., and Ogawa, T. (2001). Genes essential to iron transport in the cyanobacterium Synechocystis sp. strain PCC 6803. J. Bacteriol. 183, 2779–2784.
King, A. L., Buck, K. N., and Barbeau, K. A. (2012). Quasi-Lagrangian drifter studies of iron speciation and cycling off point conception, California. Mar. Chem. 128, 1–12.
Koebnik, R., Bäumler, A. J., Heesemann, J., Braun, V., and Hantke, K. (1993a). The TonB protein of Yersinia enterocolitica and its interactions with TonB-box proteins. Mol. Gen. Genet. 237, 152–160.
Koebnik, R., Hantke, K., and Braun, V. (1993b). The TonB-dependent ferrichrome receptor FcuA of Yersinia enterocolitica: evidence against a strict co-evolution of receptor structure and substrate specificity. Mol. Microbiol. 7, 383–393.
Koster, M., Ovaa, W., Bitter, W., and Weisbeek, P. (1995). Multiple outer membrane receptors for uptake of ferric pseudobactins in Pseudomonas putida WCS358. Mol. Gen. Genet. 248, 735–743.
Kryazhimskiy, S., and Plotkin, J. B. (2008). The population genetics of dN/dS. PLoS Genet. 4, e1000304. doi:10.1371/journal.pgen.1000304
Kuma, K., Nakabayashi, S., Suzuki, Y., Kudo, I., and Matsunaga, K. (1992). Photo-reduction of Fe (III) by dissolved organic substances and existence of Fe(II) in seawater during spring blooms. Mar. Chem. 37, 15–27.
LaRoche, J., Boyd, P. W., McKay, R. M. L., and Geider, R. J. (1996). Flavodoxin as an in situ marker for iron stress in phytoplankton. Nature 382, 802–805.
Lewis, L. A., Gray, E., Wang, Y. P., Roe, B. A., and Dyer, D. W. (1997). Molecular characterization of hpuAB, the haemoglobin-haptoglobin-utilization operon of Neisseria meningitidis. Mol. Microbiol. 23, 737–749.
Liu, H. B., Landry, M. R., Vaulot, D., and Campbell, L. (1999). Prochlorococcus growth rates in the central equatorial Pacific: an application of the fmax approach. J. Geophys. Res. Oceans 104, 3391–3399.
Liu, H. B., Nolla, H. A., and Campbell, L. (1997). Prochlorococcus growth rate and contribution to primary production in the equatorial and subtropical North Pacific Ocean. Aquat. Microb. Ecol. 12, 39–47.
Ludwig, W., Strunk, O., Westram, R., Richter, L., Meier, H., Yadhukumar, et al. (2004). ARB: a software environment for sequence data. Nucleic Acids Res. 32, 1363–1371.
Lynch, D., O’brien, J., Welch, T., Clarke, P., Cuiv, P. O., Crosa, J. H., et al. (2001). Genetic organization of the region encoding regulation, biosynthesis, and transport of rhizobactin 1021, a siderophore produced by Sinorhizobium meliloti. J. Bacteriol. 183, 2576–2585.
Macrellis, H. M., Trick, C. G., Rue, E. L., Smith, G., and Bruland, K. W. (2001). Collection and detection of natural iron-binding ligands from seawater. Mar. Chem. 76, 175–187.
Martin, J. H., Coale, K. H., Johnson, K. S., Fitzwater, S. E., Gordon, R. M., Tanner, S. J., et al. (1994). Testing the iron hypothesis in ecosystems of the equatorial Pacific Ocean. Nature 371, 123–129.
Martinez, J. S., and Butler, A. (2007). Marine amphiphilic siderophores: Marinobactin structure, uptake, and microbial partitioning. J. Inorg. Biochem. 101, 1692–1698.
Martinez, J. S., Zhang, G. P., Holt, P. D., Jung, H. T., Carrano, C. J., Haygood, M. G., et al. (2000). Self-assembling amphiphilic siderophores from marine bacteria. Science 287, 1245–1247.
Mawji, E., Gledhill, M., Milton, J. A., Tarran, G. A., Ussher, S., Thompson, A., et al. (2008). Hydroxamate siderophores: occurrence and importance in the Atlantic Ocean. Environ. Sci. Technol. 42, 8675–8680.
Mey, A. R., and Payne, S. M. (2001). Haem utilization in Vibrio cholerae involves multiple TonB-dependent haem receptors. Mol. Microbiol. 42, 835–849.
Meyer, F., Paarmann, D., D’souza, M., Olson, R., Glass, E. M., Kubal, M., et al. (2008). The metagenomics RAST server – a public resource for the automatic phylogenetic and functional analysis of metagenomes. BMC Bioinformatics 9, 386. doi:10.1186/1471-2105-9-386
Mochizuki, N., Tanaka, R., Grimm, B., Masuda, T., Moulin, M., Smith, A. G., et al. (2010). The cell biology of tetrapyrroles: a life and death struggle. Trends Plant Sci. 15, 488–498.
Ochsner, U. A., Johnson, Z., and Vasil, M. L. (2000). Genetics and regulation of two distinct haem-uptake systems, phu and has, in Pseudomonas aeruginosa. Microbiology 146, 185–198.
Olsen, G. J., Matsuda, H., Hagstrom, R., and Overbeek, R. (1994). fastDNAml: a tool for construction of phylogenetic trees of DNA sequences using maximum likelihood. Comput. Appl. Biosci. 10, 41–48.
Palenik, B., Grimwood, J., Aerts, A., Rouze, P., Salamov, A., Putnam, N., et al. (2007). The tiny eukaryote Ostreococcus provides genomic insights into the paradox of plankton speciation. Proc. Natl. Acad. Sci. U.S.A. 104, 7705–7710.
Palenik, B., Ren, Q. H., Dupont, C. L., Myers, G. S., Heidelberg, J. F., Badger, J. H., et al. (2006). Genome sequence of Synechococcus CC9311: insights into adaptation to a coastal environment. Proc. Natl. Acad. Sci. U.S.A. 103, 13555–13559.
Plessner, O., Klapatch, T., and Guerinot, M. L. (1993). Siderophore utilization by Bradyrhizobium japonicum. Appl. Environ. Microbiol. 59, 1688–1690.
Poole, K., and McKay, G. A. (2003). Iron acquisition and its control in Pseudomonas aeruginosa: many roads lead to Rome. Front. Biosci. 8, D661–D686.
Raes, J., Letunic, I., Yamada, T., Jensen, L. J., and Bork, P. (2011). Toward molecular trait-based ecology through integration of biogeochemical, geographical and metagenomic data. Mol. Syst. Biol. 7, 473.
Rakin, A., Saken, E., Harmsen, D., and Heesemann, J. (1994). The pesticin receptor of Yersinia enterocolitica: a novel virulence factor with dual function. Mol. Microbiol. 13, 253–263.
Reid, R. T., Live, D. H., Faulkner, D. J., and Butler, A. (1993). A siderophore from a marine bacterium with an exceptional ferric ion affinity constant. Nature 366, 455–458.
Rue, E. L., and Bruland, K. W. (1995). Complexation of iron(III) by natural organic ligands in the Central North Pacific as determined by a new competitive ligand equilibration/adsorptive cathodic stripping voltammetric method. Mar. Chem. 50, 117–138.
Rusch, D. B., Halpern, A. L., Sutton, G., Heidelberg, K. B., Williamson, S., Yooseph, S., et al. (2007). The sorcerer II global ocean sampling expedition: Northwest Atlantic through Eastern Tropical Pacific. PLoS Biol. 5, e77. doi:10.1371/journal.pbio.0050077
Rusch, D. B., Martiny, A. C., Dupont, C. L., Halpern, A. L., and Venter, J. C. (2010). Characterization of Prochlorococcus clades from iron-depleted oceanic regions. Proc. Natl. Acad. Sci. U.S.A. 107, 16184–16189.
Sanders, J. D., Cope, L. D., Muller-Eberhard, U., and Hansen, E. J. (1994). Identification of a locus involved in the utilization of iron by Haemophilus influenzae. Infect. Immun. 62, 4515–4525.
Schroder, I., Johnson, E., and De Vries, S. (2003). Microbial ferric iron reductases. FEMS Microbiol. Rev. 27, 427–447.
Seshadri, R., Kravitz, S. A., Smarr, L., Gilna, P., and Frazier, M. (2007). CAMERA: a community resource for metagenomics. PLoS Biol. 5, e75. doi:10.1371/journal.pbio.0050075
Smith, D. P., Kitner, J. B., Norbeck, A. D., Clauss, T. R., Lipton, M. S., Schwalbach, M. S., et al. (2010). Transcriptional and translational regulatory responses to iron limitation in the globally distributed marine bacterium Candidatus Pelagibacter ubique. PLoS ONE 5, e10487. doi:10.1371/journal.pone.0010487
Srivastava, P. K., Desai, D. K., Nandi, S., and Lynn, A. M. (2007). HMM-ModE – improved classification using profile hidden Markov models by optimising the discrimination threshold and modifying emission probabilities with negative training sequences. BMC Bioinformatics 8, 104. doi:10.1186/1471-2105-8-104
Stojiljkovic, I., and Hantke, K. (1994). Transport of haemin across the cytoplasmic membrane through a haemin-specific periplasmic binding-protein-dependent transport system in Yersinia enterocolitica. Mol. Microbiol. 13, 719–732.
Szklarczyk, D., Franceschini, A., Kuhn, M., Simonovic, M., Roth, A., Minguez, P., et al. (2011). The STRING database in 2011: functional interaction networks of proteins, globally integrated and scored. Nucleic Acids Res. 39, D561–D568.
Tai, V., Poon, A. F. Y., Paulsen, I. T., and Palenik, B. (2011). Selection in coastal Synechococcus (Cyanobacteria) populations evaluated from environmental metagenomes. PLoS ONE 6, e24249. doi:10.1371/journal.pone.0024249
Thompson, A. W., Huang, K., Saito, M. A., and Chisholm, S. W. (2011). Transcriptome response of high- and low-light-adapted Prochlorococcus strains to changing iron availability. ISME J. 5, 1580–1594.
Thompson, J. M., Jones, H. A., and Perry, R. D. (1999). Molecular characterization of the hemin uptake locus (hmu) from Yersinia pestis and analysis of hmu mutants for hemin and hemoprotein utilization. Infect. Immun. 67, 3879–3892.
Toulza, E., Tagliabue, A., Blain, S., and Piganeau, G. (2012). Analysis of the Global Ocean Sampling (GOS) Project for trends in iron uptake by surface ocean microbes. PLoS ONE 7, e30931. doi:10.1371/journal.pone.0030931
Tsuda, A., Takeda, S., Saito, H., Nishioka, J., Nojiri, Y., Kudo, I., et al. (2003). A mesoscale iron enrichment in the Western Subarctic Pacific induces a large centric diatom bloom. Science 300, 958–961.
Vanderpool, C. K., and Armstrong, S. K. (2003). Heme-responsive transcriptional activation of Bordetella bhu genes. J. Bacteriol. 185, 909–917.
Velasquez, I., Nunn, B. L., Ibisanmi, E., Goodlett, D. R., Hunter, K. A., and Sander, S. G. (2011). Detection of hydroxamate siderophores in coastal and Sub-Antarctic waters off the South Eastern Coast of New Zealand. Mar. Chem. 126, 97–107.
Vichi, M., Masina, S., and Navarra, A. (2007a). A generalized model of pelagic biogeochemistry for the global ocean ecosystem. Part II: numerical simulations. J. Mar. Syst. 64, 110–134.
Vichi, M., Pinardi, N., and Masina, S. (2007b). A generalized model of pelagic biogeochemistry for the global ocean ecosystem. Part I: theory. J. Mar. Syst. 64, 89–109.
Waterbury, J. B., Watson, S. W., Guillard, R. R. L., and Brand, L. E. (1979). Widespread occurrence of a unicellular, marine, planktonic cyanobacterium. Nature 277, 293–294.
Webb, E. A., Moffett, J. W., and Waterbury, J. B. (2001). Iron stress in open-ocean cyanobacteria (Synechococcus, Trichodesmium, and Crocosphaera spp.): identification of the IdiA protein. Appl. Environ. Microbiol. 67, 5444–5452.
Wexler, M., Todd, J. D., Kolade, O., Bellini, D., Hemmings, A. M., Sawers, G., et al. (2003). Fur is not the global regulator of iron uptake genes in Rhizobium leguminosarum. Microbiology 149, 1357–1365.
Wilhelm, S. W., and Trick, C. G. (1994). Iron-limited growth of cyanobacteria: multiple siderophore production is a common response. Limnol. Oceanogr. 39, 1979–1984.
Worsham, P. L., and Konisky, J. (1985). Locus affecting regulation of the colicin I receptor by iron. J. Bacteriol. 161, 428–431.
Keywords: marine microbes, eukaryotic phytoplankton, Fe limitation, Fe- binding ligands, multivariate statistics, metagenomes, dN/dS ratio, aquatic niches
Citation: Desai DK, Desai FD and LaRoche J (2012) Factors influencing the diversity of iron uptake systems in aquatic microorganisms. Front. Microbio. 3:362. doi: 10.3389/fmicb.2012.00362
Received: 16 January 2012; Accepted: 24 September 2012;
Published online: 18 October 2012.
Edited by:
Martha Gledhill, University of Southampton, UKReviewed by:
Amy M. Grunden, North Carolina State University, USAKatherine Barbeau, University of California San Diego, USA
Sylvia McDevitt, Skidmore College, USA
Copyright: © 2012 Desai, Desai and LaRoche. This is an open-access article distributed under the terms of the Creative Commons Attribution License, which permits use, distribution and reproduction in other forums, provided the original authors and source are credited and subject to any copyright notices concerning any third-party graphics etc.
*Correspondence: Dhwani K. Desai, Biological Oceanography Division, Helmholtz-Zentrum für Ozeanforschung Kiel (GEOMAR), DusternbrookerWeg 20, 24015 Kiel, Germany. e-mail: dhwanidesai@gmail.com
†Dhwani K. Desai and Falguni D. Desai have contributed equally to this work.