- Department of Food Science, University of Foggia, Foggia, Italy
The selection of yeasts intended as starters for table olives is a complex process, including a characterization step at laboratory level and a validation at lab level and factory-scale. The characterization at lab level deals with the assessment of some technological traits (growth under different temperatures and at alkaline pHs, effect of salt, and for probiotic strains the resistance to preservatives), enzymatic activities, and some new functional properties (probiotic traits, production of vitamin B-complex, biological debittering). The paper reports on these traits, focusing both on their theoretical implications and lab protocols; moreover, there are some details on predictive microbiology for yeasts of table olives and on the use of multivariate approaches to select suitable starters.
Yeasts as Starter Cultures in Table Olives
Olive fermentation relies on a complex microflora, including lactic acid bacteria (LAB), Gram Negative bacteria, and yeasts (Garrido-Fernández et al., 1997; Arroyo-López et al., 2008b); however, the fermentation of treated olives (e.g., olives where oleuropein had been removed through a treatment with NaOH) has been attributed for a long period to LAB, while yeasts have been referred as spoiling microorganisms, due to their pectolytic and xylanolytic activities or to the production of off-flavors and off-odors, clouding of brines, gas-pocket formation, and package bulging (Garrido-Fernández et al., 1997; Bevilacqua et al., 2009; Tofalo et al., 2012). Recently, the role of yeasts has been reviewed and many authors have reported that they could exert a fundamental role for olive fermentation, both for green-treated olives and black-naturally fermented ones, with a focus on both their technological implications and functional properties (Arroyo-López et al., 2008b; Bevilacqua et al., 2009; Bautista-Gallego et al., 2011; Silva et al., 2011; Rodríguez-Gómez et al., 2012). Although it is not possible to point out a general yeast evolution throughout olive fermentation, due to different olive varieties and flow-sheets of production, literature analysis allows the definition of the most common species; yeasts belong to the genera Aureobasidium, Candida, Cryptococcus, Issatchenkia, Pichia, Rhodotorula, Saccharomyces, Zygotorulaspora; Table 1 represents a short overview of the literature and reports the most important species, for different kinds of olives (green and alkali-treated, black and naturally fermented ones), from different cultivars and Countries (Italy, Greece, Spain, and some other Countries of Mediterranean area).
Starter cultures are preparations of live microorganisms or their resting forms, whose metabolic activity has desired effects in the fermentation substrate (DFG-Senate Commission of Food Safety, 2010).
Keeping in mind the idea that yeasts can contribute to improve olive quality, its obvious that yeast use as starters in this kind of fermented foods could have strong benefits. The basic question is “which strain? what are the characteristics required for a potential starter? how select a starter?”
Starter selection is a complex process; although there is not a general flow-sheet for starters of table olives, we can use the procedure proposed by Carnevali et al. (2007) for other microorganisms and other foods and modify it. Generally, the selection involves three steps:
1. isolation and “in vitro” selection;
2. validation on a lab-scale;
3. validation at factory-scale.
Figure 1 reports a possible general flow-sheet for the selection of suitable starter cultures. Focusing on yeasts of table olives, the criteria to be used at laboratory level (step 1) includes technological traits, enzymatic activities, and some new criteria (Table 2); the technological traits deal with the ability to grow and persist under the conditions usually encountered in olive fermentation, i.e., moderate-to-high salt amounts, temperature profile (an import tool to assure starter ability to conduct fermentation from September to November), growth, and survival at alkaline pHs (for green alkali-treated olives-Spanish style). Apart from these primary technological traits, the use of probiotic strains requires their ability to survive throughout storage and resist to the common preservatives used for olive stabilization.
Another important field for yeast selection is their ability to improve olive quality through production of volatile and flavor compounds (esterase and lipolytic activity). Finally a new trend for fermented foods is the use of functional starter cultures, i.e., starter cultures showing an added value (probiotic properties, or the production of vitamin B-complex, as well as the ability to degrade oleuropein-biological debittering of olives).
In the following sections of this chapter, these characteristics will be discussed, reporting on the practical protocols to be used, as well as on their theoretical implications; finally, some details on starter validation in laboratory and at industrial level will be reported.
Technological Traits: Growth Assays
The positive technological traits for yeasts intended as starters for table olives include both growth assays and resistance profile to some common preservatives (this trait only for probiotic strains).
Concerning growth assays, they focus on the most important variables acting on yeast evolution in brines, i.e., salt concentration, temperature, and pH; thus Bevilacqua et al. (2009) proposed a quali-quantitative approach, based on yeast growth in lab media, supplemented with different amounts of salt, adjusted to different pHs, or stored under different temperatures. The growth was evaluated through absorbance measurement at 600 nm and compared to yeast growth in a control (i.e., not-modified lab medium at pH 6.0–6.5 and incubated at 25°C); Bevilacqua et al. (2009) proposed a low level of inoculum (3 log cfu/ml) and modified the equation of Growth Index (GI) reported by Blaszyk and Holley (1998), as follows:
where Abssample is the absorbance in the samples with salt, at different pHs, or incubated under various temperatures, while Abscontrol is the absorbance of the control; this index is time-dependent, i.e., it represents yeast growth for a particular time-point.
Based upon GI, Bevilacqua et al. (2009) used the following approach to highlight inhibitory effects:
(a) GI < 25%, strong inhibition,
(b) 25 < GI < 75%, partial inhibition,
(c) GI > 75%, growth similar to that recovered in the control.
Predictive Microbiology
The evaluation of growth/resistance of yeasts under different technological conditions is one of the most important field to use Predictive Microbiology (PM) in table olives; as reported by Arroyo-López et al. (2010), the final result of PM is obtaining an useful and safe tool for the implementation and evaluation of corrective actions throughout olive processing and packaging.
Focusing on the use of PM for yeast selection, basically it can be applied at two different levels, i.e., modeling growth/survival under different conditions (primary model) and building a second-level model.
Data for primary models are usually collected by means of plate count and OD measurement and then fitted through some common sigmoid equations (Gompertz, Logistic, Baranyi) to obtain the physiological parameters of yeast population (lag phase duration, maximum specific growth rate and maximum population level reached; Arroyo-López et al., 2010).
Another trait of great concern is modeling death kinetic and yeast survival under different conditions; for this purpose a shoulder/tail equations could be of interest (Arroyo-López et al., 2010), as well as the Weibull-type model proposed by Van Boekel (2002).
Recently, some primary models were proposed to fit simultaneously both the growth and decay phases of microorganisms, such as the quasi-chemical primary model, the Peleg model, or the Churchill and two-term Gompertz equations (Arroyo-López et al., 2010).
Fitting parameters of primary models represent the input values to build second-level model, able to predict yeast growth as a function of technological and environmental conditions. Secondary models can be some simple regression functions or more complex probabilistic models, depending on the initial approach and experimental design used to combine the different environmental variables (Arroyo-López et al., 2010).
Why use PM in table olives and for the selection of a suitable yeast starter cultures? Arroyo-López et al. (2010) reports some interesting ideas:
1. Reduce the length of the fermentation period, finding the combination of factors that favor growth and imposition of a “starter” culture.
2. Study the interactions between microorganisms during table olive fermentation or storage.
3. Optimize the concentration of preservatives during packaging that guarantee the inhibition of microorganisms.
4. Estimate the shelf life of packed table olives.
5. Optimize the production of desirable metabolites.
Resistance to Preservatives
A trait of great concern for yeast selection is the resistance to kaolin and copper based products, ZnCl2, sorbate, benzoate and metabisulfite, natamycin, and citric acid (Arroyo-López et al., 2008b; Echevarria et al., 2010; Bautista-Gallego et al., 2011, 2012; Muccilli et al., 2011); this trait could be not a primary tool to be assessed, as preservatives are used to stabilize olives throughout packaging and storage and yeasts should not be able to grow under these conditions. An important exception to this generalized statement is the use of probiotic yeasts which are required to survive and persist throughout storage.
Apart from the role of these compounds, extensively reviewed by the literature, the evaluation of yeast resistance is based upon the use of PM and mathematical tools in lab media, later confirmed in brines. Briefly, three innovative and interesting approaches were proposed for yeasts of table olives (Quasi-chemical model, fractional area, and growth/no growth interface); growth/no growth interface is more focused on product stabilization and on the inhibition of spoiling yeasts, thus it will be not discussed in the following sections.
Quasi-Chemical Primary Model
Echevarria et al. (2010) studied the effects of ascorbic acid and sodium metabisulfite, combined with NaCl, through a Quasi-chemical primary model; the main idea of this approach is to integrate the individual phases of microbial life cycle into a series of chemical equation at constant rate (Taub et al., 2003; Echevarria et al., 2010). Basically, life cycle is divided into four steps, each of them associated to a differential equation (ODE, ordinary differential equation); the set of equation can be summarized as follows (Ross et al., 2005).
Activation
Multiplication
Sensitized death/decay
Natural death/decayed
In this set of equations, M is the concentration of cells in the lag phase (inoculum); M*, growth phase cells; A, antagonistic metabolite; D, dead cells; k1–k4, rate constants; G, net growth rate.
The numerical solving of these equations results in the estimation of the growth rate (μ), lag phase (λ) and maximum population size (called ratio of growth and denoted as Rg).
After parameters evaluation, Echevarria et al. (2010) put them in a polynomial equation of cubic order to highlight the individual and interactive effects of the different antimicrobials (theory of Design of Experiments, DoE).
Fractional Area
Another approach to highlight the antimicrobial effect of a preservative toward yeasts of table olives is the evaluation of the Minimal Inhibitory Concentration (MIC) along with Non-Inhibitory Concentration (NIC; Arroyo-López et al., 2008a).
This approach is based on a spectro-photometric assay and compares the area under OD/time curve corresponding to a positive control (inoculated sample without preservative; areacont) with the areas of the test samples (areatest) through the following equation:
where “fa” is the fractional area; areauni is the area under OD/time curve corresponding to uninoculated samples, but containing the antimicrobial compound, and areaunicot is the area under OD/time curve of negative control (samples without microorganisms and preservative).
It is possible to estimate the “fa” for each concentration of the antimicrobial compound; then, the values of “fa” versus log10 concentration can be fitted through the equation of Lambert et al. (2001):
where x is the concentration of the antimicrobial; P1 the concentration of preservative when fa is 1/e and P2 the slope parameter.
Re-arranging the equations and using the fitting parameters P1 and P2, MIC and NIC can be evaluated as follows:
For two antimicrobials (A and B) used in a combination, Arroyo-López et al. (2008a) proposed the use of the fractional inhibitory concentrations (FIC). Briefly, FIC values can be estimated as follows:
Calculation of a combined FIXindex yields a single number able to highlight additive, synergistic, or antagonistic effects. Theoretically, FICindex near 1 indicates an additive effect, while FICindex < 1 and FICindex > 1 stand for synergic and antagonistic effects, respectively.
Enzymatic Activities
The study of enzymatic activities represents a critical point for yeast selection, as a starter could enhance, increase, or decrease the quality of raw material through the production of some metabolites and secondary compounds; the enzymatic activities for yeasts intended for table olives include both desired and undesired characteristics.
The desired characters include the following ones:
1. Esterase and lipolysis: Esterase and lipase enzymes (generally recognized as lipolytic activity) are desirable characters, because they can improve the flavor of olives through the formation of volatile compounds, generated by the catabolism of free fatty acids (Hernándèz et al., 2007; Rodríguez-Gómez et al., 2012); the compounds related to the improvement of flavor include ethanol, glycerol, higher alcohols, esters, and other volatile compounds (Garrido-Fernández et al., 1997). Lipolytic activity can be assessed through a qualitative test, by streaking yeasts on Tributyrin Agar Base, acidified to pH 4.0; a positive lipolytic activity can be evidenced by a clear halo (Hernándèz et al., 2007). This activity can be evaluated also through the quantitative assay suggested by Rodríguez-Gómez et al. (2012), using a chromogenic substrate, containing p-nitrophenyl stearate and 4-nitrophenyl palmitate (for lipase enzymes) and p-nitrophenyl butyrate for esterase determination.
2. β-Glucosidase: This character is probably related to the ability to degrade oleuropein, a new functional trend for yeast selection, thus it will be discussed in the following section.
3. Catalase: The catalase activity of yeasts can be evaluated by adding 3% (v/v) of hydrogen peroxide onto the cultured colonies, according to the Whittenbury method (Whittenbury, 1964). The role of this enzymatic activity is uncertain; some authors reported in the past that it could be related to the ability to prevent radical formation and lipid oxidation (Bevilacqua et al., 2009).
Apart from the positive enzymatic activities, yeasts should be evaluated in relation to their production of toxic compounds and for their effects on olive texture and quality. The assessment of amine (BA) production (spermine, spermidine, agmatine) was proposed in the past as a new criterion for the selection of yeasts as starter cultures for wine (Caruso et al., 2002; Landete et al., 2007); many yeast species responsible of BA synthesis can be recovered in olives, thus this trait should be evaluated carefully also for microflora of table olives. In laboratory this assay can be performed on a chromogenic medium and then confirmed through HPLC.
Other traits for yeast selection include some possible enzymatic activities with a negative impact on olive quality, i.e., pectolytic and xylanolytic activities and protease enzymes; hydrolysis of pectins and xylans, as well as proteolytic activity, are related to olive softening and to the production of pellicles (Rhodotorula spp., P. anomala, S. rosei, C. krusei; Arroyo-López et al., 2008b).
Many authors suggested some qualitative assays, i.e., yeast streaking on lab media containing pectins and xylans as only carbon source or casein to assess proteolytic activity (Hernándèz et al., 2007; Bevilacqua et al., 2009; Bautista-Gallego et al., 2011).
Functional Properties
The use of functional traits for the selection of yeasts for table olives is one of the most recent trend; for these microorganisms the term “functional” could highlight probiotic properties (Silva et al., 2011) or other properties health- or process-focused (Restuccia et al., 2011; Silva et al., 2011).
Concerning the probiotic properties, the classical traits includes the survival and/or resistance to gastric pH (1.5–2.5) and to bile salts, as well as the ability to adhere to intestinal mucosa and the antimicrobial activity toward foodborne and intestinal pathogens (van der Aa Kühle et al., 2005; Silva et al., 2011). Some authors proposed in the past that the survival to gastro-intestinal conditions could be assessed in YM broth (Yeast Medium, a universal medium for yeasts, medium n. 186, www.dsmz.de/catalogues/details/culture/DSM70854.html, accessed April 7, 2012) or in YNB (Yeast Nutrient Broth), acidified at pH 2.5 or supplemented with 0.3% of Oxgall, a commercial mix miming the composition of bile salts of intestine.
Another trait of great concern is the ability to adhere to intestinal mucosa, that could be evaluated directly by assessing yeast adhesion to IPEC-J2 cells (van der Aa Kühle et al., 2005) or indirectly, through the measurement of aggregation, auto-aggregation, and hydrophobicity (i.e., adhesion to hydrocarbons; Vinderola and Reinheimer, 2003). The last classic probiotic feature is the antimicrobial activity toward foodborne and intestinal pathogens (Escherichia coli, Listeria monocytogenes, Salmonella sp., Staphylococcus aureus), generally assessed through a modified agar-diffusion test (Silva et al., 2011).
Apart from the classic probiotic features, the functional properties of yeasts includes some traits health- or process-oriented; amongst the health-oriented traits, the most important ones are the production of B vitamins and cytokine assays, whereas two process-oriented properties are the assimilation of oleuropein and β-glucosidase activity. Vitamin production can be evaluated through the method of Pearson et al. (1986) in a vitamin free medium and using a spectro-photometric measurement. Cytokine assay is another way to evaluate an antagonistic effect of yeasts or probiotic bacteria toward pathogen; a probiotic could either have an antagonistic adhesion to intestinal mucosa, thus reducing the adhesion of pathogens, or exert a benefit, reducing the intestinal pro-inflammatory response, mediated through the excretion of both pro- and anti-inflammatory cytokines (van der Aa Kühle et al., 2005).
A recent trait suggested by Restuccia et al. (2011) is the β-glucosidase activity and the ability to degrade oleuropein; this property could be evaluated through a colorimetric method on an solid medium, containing arbutin, and then confirmed by qualitative assays (Restuccia et al., 2011; Silva et al., 2011). The impact of this property is really strong as it is related to the possibility to replace, or combine, chemical debittering with a biological approach.
This possibility has been suggested some years ago for some strains of Lactobacillus plantarum (Ciafardini et al., 1994); recently, Restuccia et al. (2011) characterized a β-glucosidase produced by a strain of Wickerhamomyces anomalus, showing the maximum activity at alkaline pHs and at 35°C. The contemporary presence of esterase and β-glucosidase activities in this strain suggested a potential application in olive debittering.
Selection of Promising Strains
The selection of promising strains poses an important problem, i.e., how manage a large amount of data? Multivariate analysis offers an interesting approach to solve this setback as suggested by Rodríguez-Gómez et al. (2012) and Bevilacqua et al. (2009).
The starting situation is a large amount of data, with several biochemical activities and/or the results of different assays (variables) from a considerable number of strains (cases). Which approach use? Rodríguez-Gómez et al. (2012) and Bevilacqua et al. (2009) suggested three kinds of ways: Cluster analysis (Cl), Principal Component Analysis (PCA), and Correspondence Analysis (CA); the choice of an approach relies upon the aim of the research. Mainly, Cl uses different algorithms to put all the samples (e.g., the strains) in different groups or clusters. Rodríguez-Gómez et al. (2012) reported that clustering should be used when researcher does not possess a “priori” hypothesis and is still in an exploratory phase of the research; this approach is convenient as it shows in a simple way the strains with similar characteristics. Its main drawback is that researcher can say if two strains are similar or not, but he does not know the reasons (i.e., the variables) acting on their similarities/dissimilarities. An approach to by-pass this limit is the use of PCA and CA. PCA implies a mathematical procedure that transforms the overall set of original variables into a smaller number of mathematical constructs, called factors; thereby, a new set of axes, called factor axes, is obtained in a lower dimensional space, where the cases (e.g., the strains) can be projected and classified into categories. The benefit of this kind of approach is the possibility to group strains in categories and see what are the variables acting on similarities/dissimilarities.
A similar result can be obtained through CA; CA is a descriptive/exploratory technique, designed to analyze simple two-way and multi-way tables, containing some measures of correspondence between the rows and columns; it can be considered a weighted principal component analysis method, based on the chi-square distance between categories of each variable, where the relationship between the variables is represented by a contingency table and low-dimensional graphs (Sanz et al., 2004). Graphically, in the CA strains and variables are represented as points in a factorial space; the more a strain (e.g., a point) is near to a variable, the more that strain shows the variable at high level.
Before running a multivariate approach, it is important to pay attention that all the variables are expressed with the same or similar scales (e.g., all quantitative or all qualitative); when the dataset comprises some quantitative variables and qualitative ones, it is important to use the same scale and standardize data.
A convenient approach was proposed by Bevilacqua et al. (2009), who grouped yeasts from Bella di Cerignola table olives using some quantitative (growth under different pHs, temperatures and salt concentrations, as GI value) and qualitative assays (catalase hydrolysis of pectins and xylans); thus, they used a 3-digit scale (0, 1, and 2).
As a final step we propose a simple case-study. Yeasts (C. famata, C. guilliermondii, and C. pelliculosa) were isolated from Bella di Cerignola table olives and belong to the Culture Collection of the Laboratory of Applied Microbiology (University of Foggia); the strains were studied for their ability to grow at pH 9.5, under different temperatures (from 15 to 37°C), in a medium supplemented with salt and for their pectolytic and lipolytic activities. Data were converted into codes (0, 1, and 2; Table 3).
Using the qualitative codes, Cl, PCA, and CA were run; the outputs of these approaches are reported in Figure 2. Cl grouped the strains into three statistical classes: A (strains 6, 7, 8, and 9-C. guilliermondii); B (strains 5 and 11, identified as C. famata and C. pelliculosa); C (strains, 1, 2, 3, 4-C. famata- and 10-C. pelliculosa).
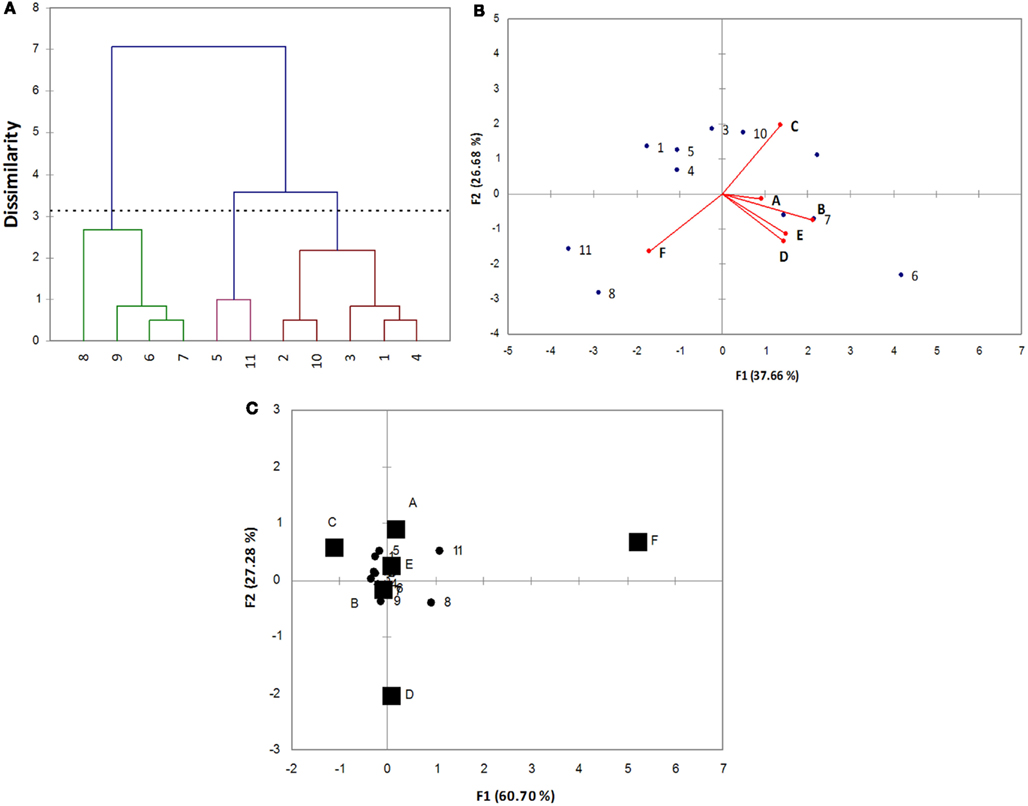
Figure 2. Multivariate analyses for the data reported in Table 3. (A) Cluster analysis; (B) principal component analysis; (C) correspondence analysis.
This approach is useful to highlight similar strains and could be of great concern for the formulation of a multiple strain starter, as I can choose similar or dissimilar strains, depending on the desired characteristics of the starter. As reported elsewhere, Cl does not highlight which variable acts on the differences, thus different multivariate approaches should be used.
Although the statistical backgrounds of CA and PCA are quite different, the results are very similar (2B and 2C), as both the picture highlight the different strains and the variables acting on this difference. The choice of CA or PCA relies upon the aims of statistical treatment of data: if I want to select some strains with a desired character, CA could be more useful, on the other hand if the aim is the selection of the best strain/strains, taking into account all the technological and functional traits, PCA is more versatile.
Apart from the statistical approach used, the main output of this step is the selection of some promising strains for the following validation. It is important to select at least 5–10 strains and perform the laboratory validation with this number, as some strains could show different traits in “in vivo” conditions.
Validation
The last step for starter selection and optimization is the validation of selected strains in foods under laboratory conditions and at industrial level; to the best of our knowledge, no paper focused on this topic. Keeping in mind the general traits for the validation of a starter, as well as the characteristics required and assessed for yeasts in other foods, the validation of yeasts for table olives should focus on some primary tools, i.e.:
1. course of fermentation,
2. effects on sensory attributes,
3. production of biomass,
4. yeast storage.
Table 4 reports the desired traits for each primary tool; these properties could represent a future direction to guide research in the selection of suitable yeasts intended for table olives. The research is at the beginning; however, it is important to re-direct assays and experiments following an holistic way and focusing on the practical aspects involved in olive fermentation.
Conflict of Interest Statement
The authors declare that the research was conducted in the absence of any commercial or financial relationships that could be construed as a potential conflict of interest.
References
Arroyo-López, F. N., Bautista-Gallego, J., Durán-Quintana, M. C., and Garrido-Fernández, A. (2008a). Modelling the inhibition of sorbic and benzoic acids on a native yeast cocktail. Food Microbiol. 25, 566–574.
Arroyo-López, F. N., Querol, A., Bautista-Gallego, A., and Garrido-Fernández, A. (2008b). Role of yeasts in table olive production. Int. J. Food. Microbiol. 128, 189–196.
Arroyo-López, F. N., Bautista-Gallego, J., Rodríguez-Gómez, F., and Garrido-Fernández, A. (2010). “Predictive microbiology and table olives,” in Current Research, Technology and Education Topics in Applied Microbiology and Microbial Biotechnology, ed. A. Mèndez-Vilas (Bajadoz: Formatex Resaerch Center), 1452–1461.
Bautista-Gallego, J., Rodríguez-Gómez, F., Barrio, E., Querol, A., Garrido-Fernández, A., and Arroyo-López, F. N. (2011). Exploring the yeast biodiversity of green table olive industrial fermentations for technological applications. Int. J. Food Microbiol. 147, 89–96.
Bautista-Gallego, J., Romero-Gil, V., Garrido-Fernández, A., and Arroyo-López, F. N. (2012). Modeling the inhibitory effects of zinc chloride on table olive related yeasts. Food Control 23, 499–505.
Bevilacqua, A., Perricone, M., Cannarsi, M., Corbo, M. R., and Sinigaglia, M. (2009). Technological and spoiling characteristics of the yeast microflora isolated from Bella di Cerignola table olives. Int. J. Food Sci. Technol. 44, 2198–2207.
Blaszyk, M., and Holley, R. A. (1998). Interaction of monolaurin, eugenol and sodium citrate on growth of common meat spoilage and pathogenic organisms. Int. J. Food Microbiol. 39, 175–183.
Carnevali, P., Ciati, R., Leporati, A., and Paese, A. (2007). Liquid sourdough fermentation: industrial application perspectives. Food Microbiol. 24, 150–154.
Caruso, P., Fiore, C., Contursi, M., Salzano, G., Paparella, A., and Romano, P. (2002). Formation of biogenic amines as criteria for the selection of wine yeasts. World J. Microbiol. Biotechnol. 18, 159–163.
Ciafardini, G., Marsilio, V., Lanza, B., and Pozzi, N. (1994). Hyrolysis of oleuropein by Lactobacillus plantarum strains associated with olive fermentation. Appl. Environ. Microbiol. 60, 4142–4147.
DFG-Senate Commission of Food Safety. (2010). Microbial Food Cultures. University of Kaiserslautern, Kauserslautern.
Echevarria, R., Bautista-Gallego, J., Arroyo-López, F. N., and Garrido-Fernández, A. (2010). Modelling the effect of ascorbic acid, sodium metabisulphite and sodium chloride on the kinetic response of lactic acid bacteria and yeasts in table olive storage using a specifically implemented Quasi-chemical primary model. Int. J. Food Microbiol. 138, 212–222.
Garrido-Fernández, A., Fernández-Díez, M. J., and Adams, M. R. (1997). Table Olives: Production and Processing. London: Chapman & Hall.
Hernándèz, A., Martin, A., Aranda, E., Pèrez-Nevado, F., and Còrdoba, M. G. (2007). Identification and characterization of yeast isolated from the elaboration of seasoned green table olives. Food Microbiol. 24, 346–351.
Lambert, R. J. W., Skandamis, P. N., Coote, P. J., and Nychas, G. J. E. (2001). A study of the minimum inhibitory concentration and mode of action of oregano essential oil, thymol and carvacrol. J. Appl. Microbiol. 91, 453–462.
Landete, J. M., Ferrer, S., and Pardo, I. (2007). Biogenic amine production by lactic acid bacteria, acetic acid bacteria and yeasts isolated from wine. Food Control 18, 1569–1574.
Muccilli, S., Caggia, C., Randazzo, C. L., and Restuccia, C. (2011). Yeast dynamics during the fermentation of brined green olives treated in the field with kaolin and Bordeaux mixture to control the olive fruit fly. Int. J. Food Microbiol. 148, 15–22.
Pearson, B. M., MacKenzie, D. A., and Keenan, M. H. J. (1986). Production of biotin by yeasts. Lett. Appl. Microbiol. 2, 25–28.
Restuccia, C., Muccilli, S., Palmeri, R., Randazzo, C. L., Caggia, C., and Spagna, G. (2011). An alkaline (β-glucosidase isolated from an olive brine strain of Wickerhamomyces anomalus. FEMS Yeast Res. 11, 487–493.
Rodríguez-Gómez, F., Romero-Gil, V., Bautista-Gallego, J., Garrido-Fernández, A., and Arroyo-López, F. N. (2012). Multivariate analysis to discriminate yeast strains with technological applications in table olive processing. World J. Microbiol. Biotechnol. 28, 1761–1770.
Ross, E. W., Taub, I. A., Doona, C. J., Feeherry, F. E., and Kustin, K. (2005). The mathematical properties of Quasi-chemical model for microorganism growth-death kinetics in foods. Int. J. Food Microbiol. 99, 157–171.
Sanz, A., Bernuès, A., Villalba, D., Casasùs, I., and Revilla, R. (2004). Influence of management and nutritionon postpartum interval in Brown Swiss and Pirenaica cows. Livest. Prod. Sci. 86, 179–191.
Silva, T., Reto, M., Sol, M., Peito, A., Peres, C. M., Peres, C., and Xavier Malcata, F. (2011). Characterization of yeasts from Portuguese brined olives, with a focus on their potentially probiotic behavior. LWT Food Sci. Technol. 44, 1349–1354.
Taub, I. A., Feeherry, F. E., Ross, E. W., Kustin, K., and Doona, C. J. (2003). A Quasi-chemical kinetic model for the growth and death of Staphylococcus aureus in intermediate moisture bread. J. Food Sci. 68, 2530–2537.
Tofalo, R., Schirone, M., Perpetuini, G., Angelozzi, G., Suzzi, G., and Corsetti, A. (2012). Microbiological and chemical profiles of naturally fermented table olives and brines from different Italian cultivars. Antonie Van Leeuwenhoek 102, 121–131.
Van Boekel, M. A. J. S. (2002). On the use of the Weibull model to describe the thermal inactivation of microbial vegetative cells. Int. J. Food Microbiol. 74, 139–159.
van der Aa Kühle, A., Skovgaard, K., and Jespersen, L. (2005). In vitro screening of probiotic properties of Saccharomyces cerevisiae var. boulardii and foodborne Saccharomyces cerevisiae strains. Int. J. Food Microbiol. 101, 29–39.
Vinderola, C. G., and Reinheimer, J. A. (2003). Lactic acid starter and probitoic bacteria: a comparative “in vitro” study of probiotic characteristics and biological barrier resistance. Food Res. Int. 36, 895–904.
Keywords: yeasts, table olives, starter selection, validation
Citation: Bevilacqua A, Corbo MR and Sinigaglia M (2012) Selection of yeasts as starter cultures for table olives: a step-by-step procedure. Front. Microbio. 3:194. doi: 10.3389/fmicb.2012.00194
Received: 11 April 2012; Paper pending published: 02 May 2012;
Accepted: 13 May 2012; Published online: 31 May 2012.
Edited by:
Aldo Corsetti, University of Teramo, ItalyReviewed by:
Francisco Noé Arroyo López, Instituto de la Grasa (CSIC), SpainRosanna Tofalo, University of Teramo, Italy
Copyright: © 2012 Bevilacqua, Corbo and Sinigaglia. This is an open-access article distributed under the terms of the Creative Commons Attribution Non Commercial License, which permits non-commercial use, distribution, and reproduction in other forums, provided the original authors and source are credited.
*Correspondence: Antonio Bevilacqua, Department of Food Science, University of Foggia, Via Napoli 25, 71122 Foggia, Italy. e-mail: a.bevilacqua@unifg.it; abevi@libero.it