- 1 Department of Microbial Ecology, Centre for Life Sciences, University of Groningen, Groningen, The Netherlands
- 2 Microbial Ecology Centre, UMR 5557, CNRS, USC 1193 INRA, Université Lyon 1, Lyon, France
The milieu in soil in which microorganisms dwell is never constant. Conditions such as temperature, water availability, pH and nutrients frequently change, impacting the overall functioning of the soil system. To understand the effects of such factors on soil functioning, proxies (indicators) of soil function are needed that, in a sensitive manner, reveal normal amplitude of variation. Thus, the so-called normal operating range (NOR) of soil can be defined. In this study we determined different components of nitrification by analyzing, in eight agricultural soils, how the community structures and sizes of ammonia oxidizing bacteria and archaea (AOB and AOA, respectively), and their activity, fluctuate over spatial and temporal scales. The results indicated that soil pH and soil type are the main factors that influence the size and structure of the AOA and AOB, as well as their function. The nitrification rates varied between 0.11 ± 0.03 μgN h−1 gdw−1 and 1.68 ± 0.11 μgN h−1 gdw−1, being higher in soils with higher clay content (1.09 ± 0.12 μgN h−1 gdw−1) and lower in soils with lower clay percentages (0.27 ± 0.04 μgN h−1 gdw−1). Nitrifying activity was driven by soil pH, mostly related to its effect on AOA but not on AOB abundance. Regarding the influence of soil parameters, clay content was the main soil factor shaping the structure of both the AOA and AOB communities. Overall, the potential nitrifying activities were higher and more variable over time in the clayey than in the sandy soils. Whereas the structure of AOB fluctuated more (62.7 ± 2.10%) the structure of AOA communities showed lower amplitude of variation (53.65 ± 3.37%). Similar trends were observed for the sizes of these communities. The present work represents a first step toward defining a NOR for soil nitrification. The sensitivity of the process and organisms to impacts from the milieu support their use as proxies in the NOR of agricultural soils. Moreover, the clear effect of soil texture established here suggests that the NOR should be defined in a soil type-specific manner.
Introduction
The diversity of microorganisms on Earth is astonishing. Torsvik et al. (1990) estimated the number of bacterial genomes in a mixed sample using DNA:DNA hybridization. The number of bacterial species in a gram of boreal forest soil was estimated to approximate 10,000. Recently, this number was reevaluated and estimations are that the number of bacterial types per gram of soil varies between 2000 in polluted soil and 8.3 million in pristine soil (Gans et al., 2005; Schloss and Handelsman, 2006). Microorganisms dominate soil communities and have a profound impact on ecosystem functioning, being drivers of key processes in the cycling of energy and nutrients. The environment in which these microorganisms dwell is, however, never constant. Abiotic and biotic conditions often change with time, leading to fluctuations in the soil microbial communities and in the overall functioning of the soil ecosystem. The natural variation of soil processes over time, in response to fluctuations in ecological factors can be depicted as the highs and lows in soil process rates. These high and lows comprise the upper and lower borders of what has been coined the normal operating range (NOR). The NOR thus represents the amplitude of variation of a given process/parameter under natural (field) conditions, over time. The manner in which the NOR is defined will depend on the spatial and temporal scales at which measurements are taken. For instance, the accumulation of organic matter (OM) in a forest is a slow process. Thus, in order to capture the natural amplitude of variation in OM in forest soils, measurement should be taken over a large timespan, on the order of decades. Local conditions are also likely to influence OM deposition, and therefore, measurements may be taken in similar forests across a region of interest. For processes that respond fast to changes caused by weather and/or anthropogenic activities, such as nitrification, a NOR might be defined on the basis of a shorter (one to a few year) study. Thus, due to its sensitivity to external drivers, processes like nitrification are considered to represent good indicators of soil quality (Doran and Zeiss, 2000; Bruinsma et al., 2003).
The NOR of soil functioning is of key relevance when evaluating the impact of disturbances on soil-borne ecosystem services and processes. Examples of such potential soil perturbations are extreme abiotic events (drought, flooding, fire), changes in agricultural management or land use, and/or the planting of genetically modified (GM) crops. Defining a NOR is important, as it provides a background against which to compare the extent of the effects of such, and other, disturbances (van Straalen, 2002; Bruinsma et al., 2003; Kowalchuk et al., 2003). For instance, by determining the fluctuations in the bacterial diversity associated with a suite of potato plants, Inceoglu et al. (2011) showed that the physiological changes associated with a GM potato did not affect the bacterial community in its rhizosphere differently from the effects of five other cultivars. Moreover, by incorporating perturbance-sensitive processes (and their proxies) into a mathematical model, an overall NOR of soil may be determined, which may be of use as a parameter that indicates the overall soil quality (Pereira e Silva et al., submitted). This overall NOR will represent a statistical tool that provides a score for soil functioning. Once the NOR is defined, it can be used to detect statistically significant changes in soil functioning, in response to disturbances at a specific time point.
Agricultural systems annually receive approximately 25% of global nitrogen input, mostly in the form of ammonium (Gruber and Galloway, 2008). The added ammonium can be oxidized to nitrate in a two-step process called nitrification. Besides its ecological relevance, nitrification is considered to represent a perturbation-sensitive process, and as such it has been advocated as a potentially suitable indicator of soil quality, e.g., in the risk assessment of GM plants (Bruinsma et al., 2003; Kowalchuk et al., 2003; Ritz et al., 2009; Wessén and Hallin, 2011). The oxidation of ammonia, the first (and rate-limiting) step in the nitrification process (performed by ammonia monooxygenase, which is encoded by amo genes), until recently was considered to be largely performed by just two monophyletic groups within the gamma- and beta-proteobacteria (AOB). AOB have been frequently used as indicators of perturbations, to measure the effects of pollution in fish farm sediments (McCaig et al., 1999), contamination of soil with toxic metals (Stephen et al., 1999), effect of effluent irrigation (Oved et al., 2001) and organic waste residues (Horz et al., 2004; Nyberg et al., 2006). However, ammonia oxidizing archaea belonging to the recently described thaumarchaea (AOA; Spang et al., 2010) have been identified several years ago (Schleper et al., 2005; Treusch et al., 2005) and these organisms were found to respond to environmental factors (Ying et al., 2010). They often revealed a remarkable numerical dominance in soils (Leininger et al., 2006).
Both AOA and AOB play roles in nitrification, although the exact contribution of each one of the two communities to the process remains unclear. There is evidence that ammonia oxidation by archaea may exceed that performed by bacteria in some soils (Prosser and Nicol, 2008; Tourna et al., 2008; Offre et al., 2009). In contrast, Jia and Conrad (2009) found that, after ammonium addition, the changes in nitrification activity were paralleled by changes in the abundances of AOB but not of AOA. Thus, the likely involvement of the AOA in the process (Caffrey et al., 2007; Zhang et al., 2009; Wessén et al., 2011; Yao et al., 2011) suggested that AOA, in conjunction with AOB, should be used as proxies to monitor nitrification. Accordingly, both AOA and AOB have been recently suggested as good indicators of soil quality (Wessén and Hallin, 2011).
Considering the great importance of nitrification and the usefulness of nitrifiers as bioindicators of soil quality, the aim of this work was to determine the NOR of nitrification across agricultural soils. For that purpose, we assessed nitrification across eight soils over 2 years. The NOR that was thus obtained represents a descriptive measure which illustrates the amplitude of variation of nitrification and/or its proxies under prevailing conditions in the soils, over eight locations and time. In particular, we determined the size, structure and diversity of both AOA and AOB communities across the soils. Community sizes were studied by quantifying the archaeal and bacterial amoA genes, whereas community structures were determined by PCR-DGGE of archaeal amoA and (betaproteo) bacterial 16S rRNA genes. Moreover, we constructed bacterial and archaeal amoA clone libraries to identify the dominant types. Finally, we also measured relevant chemical soil parameters. We hypothesized that the ammonia oxidizing communities would be mainly driven by soil type and pH, suggesting that a NOR should be defined per soil (textural) type.
Materials and Methods
Experimental Sites and Soil Sampling
Eight soils from different sites in the Netherlands were sampled seven times between April 2009 and October 2010, after seedling (April 2009 and 2010), before flowering (June 2009 and 2010), and in senescence stage (September 2009 and October 2010). In November 2009 there were no plants in the fields anymore. The fields are used for potato cropping and were under agricultural rotation regime. Information on land use and location is available (Table 1). The soils were chosen to represent different soil types (clay versus sand) and present different chemical properties (Table A1 in Appendix). Bulk soil samples (4 replicates per soil; 0.5 kg per replicate) were collected in plastic bags and thoroughly homogenized before further processing in the lab. A 100-g subsample was used for measuring ammonia oxidizing enzyme activity, molecular biology and soil chemical properties.
Soil Chemical Analysis and Ammonia Oxidizing Activity
Soil pH was defined in 0.01 M CaCl2 (1:4.5). Water content was determined by drying for 48 h at 65°C. OM content was calculated on dried soil as the difference between the initial and final sample weights measured after 4 h at 550°C. Nitrate and ammonium
were determined colorimetrically in a solution of 0.01 M CaCl2 with an Autoanalyzer II (Technicon Instrument Corporation, Tarrytown, New York; samples from 2009) and using the commercial kits Nanocolor Nitrat50 (detection limit, 0.3 mg N kg−1 dry weight, Macherey-Nagel, Germany) and Ammonium3 (detection limit, 0.04 mg N kg−1 dry weight; Macherey-Nagel, Germany; samples from 2010) according to Töwe et al. (2010). Potential nitrifying enzyme activity (NEA) was measured in soil suspensions in the presence of non-limiting ammonium and ambient atmospheric O2 concentration according to Dassonville et al. (2011), using a modified version of the method of Hart et al. (1994) with an ionic chromatography (DX120, Dionex, Salt Lake City, USA) equipped with a 4-mm × 250-mm column (IonPac AS9 HC).
Nucleic Acid Extraction
DNA was extracted from 0.5 g of soil using Power Soil MoBio kit (Mo Bio Laboratories Inc., NY, USA), according to the manufacturer’s instructions, after the addition of glass beads (diameter 0.1 mm; 0.25 g) to the soil slurries. The cells were disrupted by bead beating (mini-bead beater; BioSpec Products, United States) three times for 60 s. The quantity of extracted DNA was estimated by comparison to a 1-kb DNA ladder (Promega, Leiden, Netherlands) and quality was determined based on the degree of DNA shearing (average molecular size) as well as the amounts of co-extracted compounds.
Real Time Quantitative PCR
The abundance of archaeal and bacterial ammonia oxidizers was quantified by quantitative PCR (qPCR) targeting the amoA gene. For AOA primers amo23F (Tourna et al., 2008) and crenamo616r (Nicol et al., 2008) were used obtaining fragments of 624 bp. AOB amoA quantification was performed using primers amoA-1F (Stephen et al., 1999) and amoA-2R (Rotthauwe et al., 1997), according to Nicol et al. (2008), generating fragments of 491 bp. Cycling programs and primer sequences are detailed in Table A2 (in Appendix). Quantification was carried out twice from each of the four soil replicates on the ABI Prism 7300 Cycler (Applied Biosystems, Germany). The specificity of the amplification products was confirmed by melting-curve analysis, and the expected sizes of the amplified fragments were checked in a 1.5% agarose gel stained with ethidium bromide. Standard curves were obtained using serial dilutions of plasmid containing cloned archaeal or bacterial amoA gene, from 107 to 102 gene copy numbers/microliter. Possible inhibitory effects of co-extracted humid compounds were checked by spiking standard concentrations with samples. No apparent inhibition was observed.
Standard PCR Amplification and DGGE Analysis
PCR was performed targeting 16S rRNA and amoA genes of ammonia oxidizing bacteria (AOB) or AOA, respectively. Amplification of 16S rRNA gene fragments from extracted soil DNA was achieved by primary amplification with CTO189f and CTO654r primers (Kowalchuk et al., 1997) and with a secondary nested amplification using bacterial 357f-GC and 518r primers (Muyzer et al., 1993). CTO and bacterial primers amplified 465 and 161 bp fragments, respectively. A detailed procotol is described in Freitag et al. (2006). AOA amoA was amplified using primers crenamA23f/crenamoA616r (Tourna et al., 2008). Cycling conditions are described in Table A2 (in Appendix). DGGE profiles were generated with the Ingeny Phor-U system (Ingeny International, Goes, The Netherlands). The PCR products (120 ng/lane) were loaded onto 6% (w/v) polyacrylamide gels, with a 15–55 and 35–70% denaturant gradient (100% denaturant corresponded to 7 M urea and 40% (v/v) deionized formamide) for archaeal amoA and 16S rRNA gene, respectively, as described previously by Nicol et al. (2008). Electrophoresis was performed at a constant voltage of 100 V for 16 h at 60°C. The gels were stained for 60 min in 0.5× TAE buffer with SYBR Gold (final concentration 0.5 μg/l; Invitrogen, Breda, The Netherlands). Images of the gels were obtained with Imagemaster VDS (Amersham Biosciences, Buckinghamshire, United Kingdom) and normalized in the GelCompar II software (Applied Maths, Sint-Martens Latem, Belgium), using the unweighted-pair group method with arithmetic mean, rolling-disk background subtraction, and no optimization (Kropf, 2004; Rademaker and de Bruijn, 2004). Patterns were compared by clustering the different lanes by Pearson’s correlation coefficient implemented in GelCompar.
Construction of AOA and AOB Libraries and Phylogenetic Analysis
Clones libraries of archaeal and bacterial amoA genes for the eight soils were constructed using DNA extracted from soil collected in June of 2010. Primers Crenam23f/Crenamo616r for archaeal amoA, and primers amoA-1R/amoA-2R for bacterial amoA were used, as described for real time PCR. The products from replicates were pooled per soil, ligated into PGEM-T-Easy vector (Promega, Madison, WI, USA, EUA) in accordance with the manufacturer’s instructions and white colonies were subject to a colony PCR with vector specific primers M13-F and M13-R to check for the presence of amoA inserts. DNA sequencing was performed using an Applied Biosystems 3730 XL DNA Analyzer at LGC Genomics GmbH (Berlin, Germany). Short sequences or sequences of chimeric origin were checked by analyzing alignments using Bellerophon (Huber et al., 2004) and excluded from the analysis. Sequences obtained were processed in Mega (version 5, Mega, Biodesign Institute), translated, and the deduced amino acid sequences were aligned using Clustal W (Jeanmougin, 1998). Sequences at 1% cut-off were used to construct phylogenetic trees in which representative sequences from GenBank were included. Distance analysis of derived archaeal and bacterial amoA protein sequences and bootstrap support (neighbor-joining and parsimony analysis; 1000 replicates each) were constructed in Mega, using Jones-Taylor-Thornton (JTT) substitution model with site variation (invariable sites and eight variable gamma rates; Olsen et al., 1994). Differences in the community structures of AOA and AOB clone libraries were analyzed with UniFrac (Lozupone et al., 2006), and the program DOTUR (Distance-based OTU and richness; Schloss and Handelsman, 2006) was used to create rarefaction curves and to determine the Shannon diversity index.
Data Analysis
Physico-chemical variables were checked for normality and were log-transformed, except for soil pH. Differences in these variables between sandy and clay soils, among all eight soils, and over time were assessed with Student’s t-tests.
To test the influence of soil physico-chemical parameters (environmental factors) on community structure, forward selection was used on CCA, to select a combination of environmental variables that explained most of the variation observed in the AOA and AOB species matrix. For that, a series of constrained CCA permutations was performed in Canoco (version 4.0 for Windows, PRI Wageningen, The Netherlands,) to determine which variables best explained the assemblage variation, using automatic forward selection and Monte Carlo permutations tests (permutations = 999). The length of the corresponding arrows indicated the relative importance of the chemical factor explaining variation in the two microbial communities.
To study the dynamics of AOA and AOB communities over time, a matrix of similarities based on Pearson’s correlation was used to perform moving window analysis – MWA (Marzorati et al., 2008), by calculating the rate of change in community structure, as dissimilarity = 100 − similarity % (represented by Δt). The rate of change parameter (Δt) averages the degree of change between consecutive DGGE profiles of the same community over a fixed time interval (Marzorati et al., 2008), giving an indication of community turnover for that time period.
Correlations between NEA and community structure were tested using the RELATE analysis, a non-parametric form of Mantel test, implemented in PRIMER-E software package (version 6, PRIMER-E Ltd., Plymouth, UK; Clarke and Gorley, 2006). More specifically, for each functional group (AOA or AOB), a rank correlation coefficient (here Spearman coefficient) and significance level (obtained by a permutation test using 5000 permutations) were computed to quantify the correlation between the rank similarity matrices obtained for activity and genetic structure (Clarke and Ainsworth, 1993). For each functional group, ANOSIM statistics (Primer-E software) was performed to test for an effect of soil type on AOA and AOB community structure.
The NOR of NEA and the abundance of ammonia oxidizers were determined by subtracting lower values from higher values (also represented by Δt) of activity or amoA gene copy numbers, respectively. The influence of soil parameters or abundance of ammonia oxidizers on NEA was determined by using Pearson’s linear correlation coefficient implemented in SPSS 16.0 (SPSS, Inc., IL, USA).
We also conducted multiple regression analyses on log-normalized data (SAS® system for Windows version 8.02, SAS Institute Inc., Cary, NC, USA, 2001) to identify the main drivers of nitrification. The following parameters were included in the analysis: diversity (Shannon diversity index H′ based on DGGE profiles) and abundance (A) of archaeal (AOA) and bacterial (AOB) ammonia oxidizers, potential NEA, nitrate (NO3), ammonium (NH4), pH, OM in %, clay content in % (clay) and soil moisture in % (humidity).
Data Accessibility
The amoA sequences for the AOA and AOB have been deposited in the GenBank under accession number JF935450 – JF936076 and JF936077 – JF936667 for AOA and AOB, respectively.
Results
Seasonal Variations of Soil Chemical Properties
Soil pH, nitrate, ammonium and OM levels and water content were determined in triplicate across all soil samples. Overall, considering all soils, soil pH was significantly higher (P < 0.05) in soils K, G and L (7.32 ± 0.06, n = 57) than in soils B, V, D, W, and S (4.88 ± 0.04, n = 99) during the whole experimental period and no significant variation over time was observed. Significant changes were observed in levels of nitrate at all times, with lower values in the end of the season (September 2009: 32.8 mg kg−1 ± 7.08; October 2010: 24.2 mg kg−1 ± 2.98) and higher at the beginning (April 2009: 75.6 mg kg−1 ± 12.5; April 2010: 56.4 mg kg−1 ± 5.63). Levels of ammonium also varied over the whole period, lower values being observed at the end of the season (September 2009: 1.92 mg kg−1 ± 0.16; October 2010: 5.86 mg kg−1 ± 0.63), and higher ones at the start (April 2009: 13.3 mg kg−1 ± 1.13; April 2010: 15.3 mg kg−1 ± 1.01). Significant fluctuations (P < 0.05) in water contents in the soils were detected at all times in 2009, but not 2010. The most humid sampling time was November (23.64 ± 2.15%), and the driest was September (12.25 ± 1.68%). Variations in OM content were observed from September (5.63 ± 1.20%) to November (7.34 ± 1.45%) 2009, and from April (6.28 ± 0.85%) to June (5.04 ± 0.89%) 2010. Individual values for each soil at each sampling time can be found in Table A1 (in Appendix). Concerning differences between sandy and clayey soils, soil pH was significantly higher (P < 0.05) in the clayey (6.9 ± 0.17, n = 78) than in the sandy soils (4.71 ± 0.06, n = 78). Levels of ammonium and nitrate were significantly higher (P < 0.05) in the sandy soils in June and September of 2009 ( : 13.6 ± 0.97 and 2.3 ± 0.15 mg kg−1, respectively;
: 103.3 ± 11.20 and 52.4 ± 8.41 mg kg−1, respectively), and no significant difference in the OM content was found between sandy and clayey soils at any of the sampling times.
Seasonal Variation of Nitrifying Enzyme Activity in Relation to Soil Parameters
Variations in NEA over time were observed in all soils. On average per time, lower rates were observed in November 2009 (0.59 μgN h−1 gdw−1 ± 0.09) and June 2010 (0.59 μgN h−1 gdw−1 ± 0.07), whereas higher rates were detected in April 2010 (0.76 μgN h−1 gdw−1 ± 0.10) and October 2010 (0.79 μgN h−1 gdw−1 ± 0.11). More specifically, significantly higher values were observed for soils V, K, S, G, and L (on average 1.00 μgN h−1 gdw−1 ± 0.04, n = 100) compared to soils B, D, and W (on average 0.15 μgN h−1 gdw−1 ± 0.00, n = 60; Figure 1A). The former ones also showed higher variability across the sampling times. Higher rates were observed in soil G (1.68 μgN h−1 gdw−1 ± 0.12), and lower ones in soil W (0.11 μgN h−1 gdw−1 ± 0.03). On average per soil type, clayey soils had significantly higher values than sandy ones (1.055 μgN h−1 gdw−1 and 0.261 μgN h−1 gdw−1, respectively; P < 0.05; Figure 1B).
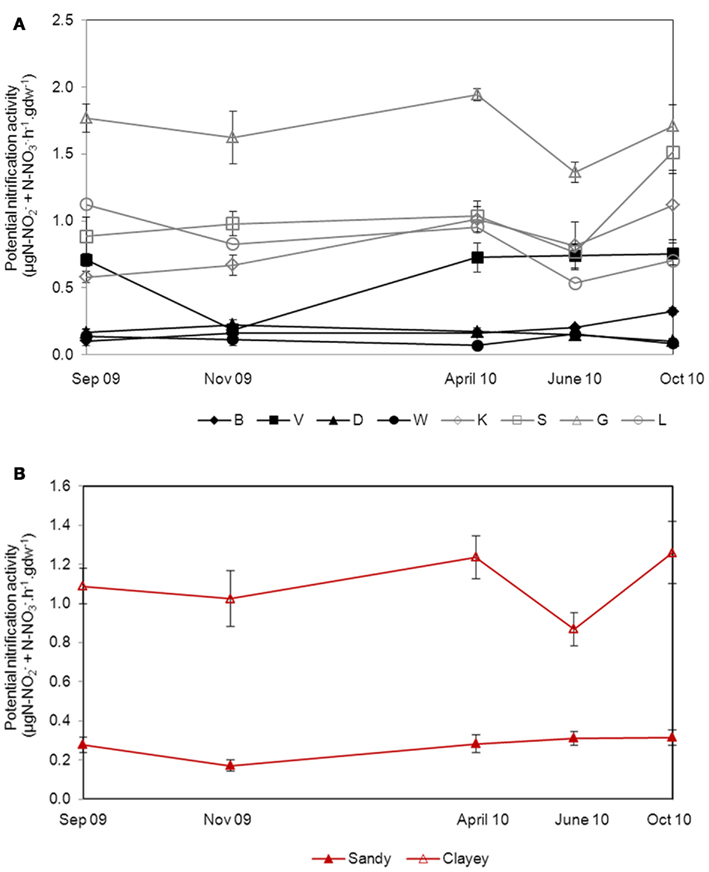
Figure 1. Potential nitrifying enzyme activity (NEA) measured in the eight soils from September 2009 to October 2010 (A), and the difference observed in the amplitude of variation in sandy (Δtsandy = 0.14 μgN h− 1 gdw− 1 ± 0.04), and in clayey soils (Δtclayey = 0.39 μgN h− 1 gdw− 1 ± 0.08) over time (B), calculated by subtracting lower from higher values. Soil names are: B, Buinen; V, Valthermond; D, Droevendaal; W, Wildekamp; K, Kollumerwaard; S, Steenharst; G, Grebbedijk; and L, Lelystad. Bars are SE (n = 4). Black symbols: sandy soils; gray symbols, clayey soil.
Pearson’s correlation analysis between NEA and soil physico-chemical parameters over time revealed that the enzyme activities correlated positively only with soil pH (r = 0.70, P ≤ 0.05; Table 2), but a small yet significant effect of clay content was also identified by multiple regression analysis (Table 3). When the same analysis was repeated for sandy and clayey soils separately, we observed that nitrate and OM were also important explanatory variables (Table 3).
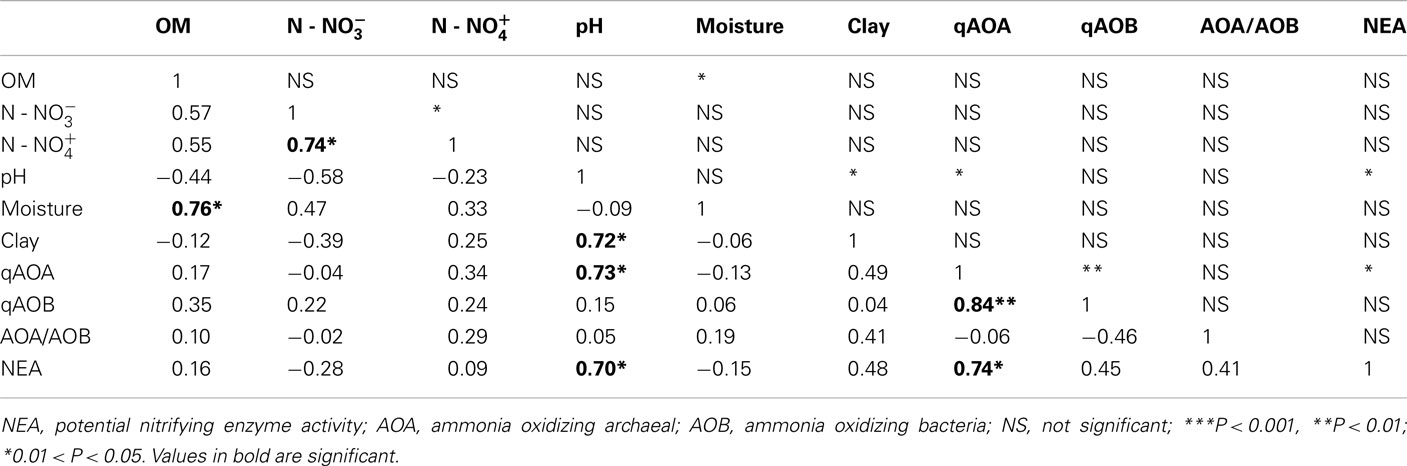
Table 2. Pearson’s correlations (r) between community size (qAOA, qAOB, and AOA/AOB), nitrifying activity (NEA), and soil properties (OM, pH, moisture and clay), calculated as average values per soil between September 2009 and October 2010.
Seasonal Variation in the Abundance of AOA and AOB Communities in Relation to Soil Parameters
Considering all eight soils, both the AOA and AOB abundances varied within 1–2 orders of magnitude across the sampling times. The numbers of archaeal amoA genes were in the range of 5.94 × 105–2.53 × 107 gene copies per gram of dry soil, whereas the bacterial amoA gene numbers varied between 2.95 × 105 and 8.32 × 107 gene copies per gram of dry soil. The AOA abundance was significantly higher in June 2010 and lower in April 2009 (P < 0.05), whereas the AOB abundance was the highest in October 2010, with comparable numbers at the other sampling times (Figures 2A–C). In general, AOA and AOB population sizes tended to increase from April to June in both years, decreasing in November (Figure 2A). Abundances of archaeal amoA genes varied from 4.76 × 105 to 3.58 × 106 gene copies gdw−1 in the sandy soils and from 1.40 × 106 to 1.54 × 107 gene copies gdw−1 in the clayey soils (Figure 2B). The bacterial amoA gene numbers varied from 1.74 × 106 to 2.30 × 107 gene copies gdw−1 in the sandy soils and from 1.60 × 106 to 4.53 × 107 gene copies gdw−1 in the clayey soils (Figure 2C). The observed amplitude of variation in community size between AOA and AOB was significantly different in April and June 2009 and in October 2010 and was larger for AOB (ΔtAOB = 1.35 ± 0.14), especially in the sandy soils (Δtclay = 1.45 ± 0.3 and Δtsandy = 1.26 ± 0.08), than for AOA (ΔtAOA = 1.21 ± 0.08) abundances, which was higher in the clayey soils (Δtclay = 1.25 ± 0.02 and Δtsandy = 1.18 ± 0.02; especially in the clayey soils (Figures 2B,C).
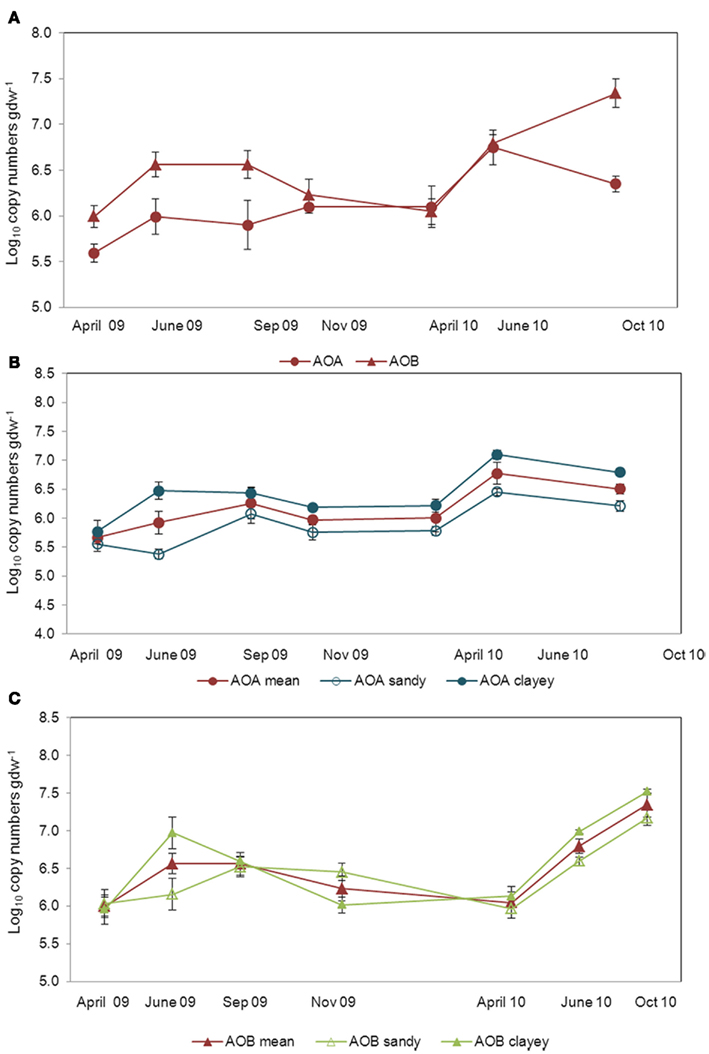
Figure 2. Amplitude of variation in the community size of AOA and AOB. Fluctuations in the community size were determined by real time quantification (qPCR) of amoA gene as a mean of all soils [(A); ΔtAOA = 1.21 ± 0.08; ΔtAOB = 1.35 ± 0.14] and separated in sandy and clayey for both AOA [(B); Δtsandy = 1.18 ± 0.02; Δtclayey = 1.25 ± 0.02] and AOB [(C); Δtsandy = 1.45 ± 0.30; Δtclayey = 1.26 ± 0.08]. Bars are SE.
Pearson’s correlations between the archaeal and bacterial amoA gene copy numbers and the soil chemical parameters measured revealed that only soil pH significantly affected the abundance of AOA (r = 0.73, P ≤ 0.05), but showed no influence on the abundance of AOB (Table 2).
Analysis of AOA and AOB Community Structure and Diversity in Relation to Soil Parameters
Two-way analysis of similarities (ANOSIM) showed an overall effect of soil type on AOA and AOB community structures at all times, but to a lesser extent on AOB (Table A3 in Appendix). Based on R values, the greatest community differentiations became measurable during early fall for AOA, but during spring and summer for AOB. The dynamics of the AOA and AOB communities were addressed by moving window analysis (MWA), whose concept can be interpreted as the number of species that on average come to significant dominance at a given habitat, during a defined time interval. Our results showed that AOA and AOB had different patterns of variation. On average, the variability of AOB was higher than that of AOA populations (ΔtAOB = 62.76 ± 2.10% and ΔtAOA = 53.65 ± 3.37%; Figure 3A). Whereas for AOA higher variations were detected in the sandy soils (Δtsandy = 60.27 ± 2.97%) compared to the clayey ones (Δtclayey = 41.09 ± 3.92%; Figure 3B), for AOB communities the amplitude of variation was higher in the clayey soils (Δtclayey = 66.55 ± 1.18%) compared to the sandy ones (Δtsandy = 58.98 ± 3.01%; Figure 3C).
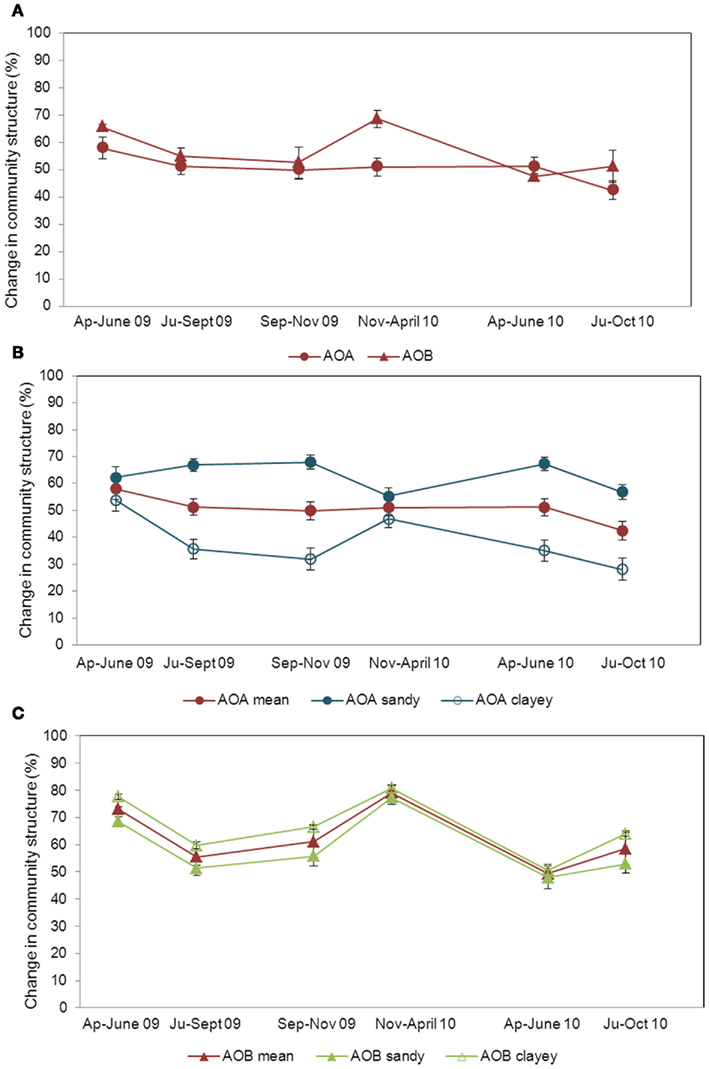
Figure 3. Amplitude of variation in the community structure of AOA and AOB. The fluctuations were determined by moving window analysis (MWA; Marzorati et al., 2008) based on DGGE profiles as a mean of all soils [(A); ΔtAOA = 53.65 ± 3.37%; ΔtAOB = 62.76 ± 2.10%], and separated in sandy and clayey for both AOA [(B); Δtsandy = 60.27 ± 2.97%; Δtclayey = 41.09 ± 3.92%] and AOB [(C); Δtsandy = 58.98 ± 3.01%; Δtclayey = 66.55 ± 1.18%]. Bars are SE. In the MWA each data point is in itself a comparison between two consecutive sampling times. Bars are SE.
Canonical correspondence analysis was used to investigate possible trends in the temporal changes in the community structures of AOA and AOB, and to test the significance of the influence of soil parameters on those changes. Although seasonality seemed to play a role in the distribution of both communities, no clear trend could be observed. In general, communities at the start of the growth season tended to cluster together (Figures A1 and A2 in Appendix). This was true especially for the AOB, in both sandy and clayey soils. The community structures of the AOA seemed to be more variable across the sampling times. Moreover, all soil variables measured apparently exerted significant effects on the AOA and AOB community structures. In order to determine the relative contribution of each soil parameter, we used variance partitioning to control for the effect of each individual parameter, when all others are defined as covariables in the constrained analyses (Leps and Smilauer, 2003). Considering the whole data set, soil parameters explained 33.4 and 49% of the variability in AOA and AOB community structures, respectively. In both cases, the percentage of clay, OM and soil pH were the most important parameters, explaining 18% (AOA) and 5.9% (AOB) of these variation.
Separating the soil in two groups, i.e., sandy and clayey ones, led to an increase in the overall percentage of variation explained by soil parameters for the AOA communities (76.6 and 79.4% for clayey and sandy soils, respectively). In clayey soils, the percentage of clay, OM, and soil pH explained 45.5%, whereas for sandy soils, these three parameters explained 44.9% of the variation in community structure. Although the separation per soil type diminished the total percentage of variation explained by the soil parameters for AOB communities (15.8 and 17.1% for clayey and sand soil, respectively), it increased the percentage of variation explained by the percentage of clay, OM and soil pH to 10.7–10.8%.
To gain larger fine-scale taxonomic resolution of the archaeal and bacterial communities, we constructed 16 clone libraries based on the amoA gene, from the eight soils. Phylogenetic analyses of the archaeal amoA fragments revealed that all clones were related to sequences of uncultured crenarchaeota obtained in earlier environmental studies. All sequences were found to cluster in a few groups denoted soil/sediment, sediment/soil and, to a lesser percentage, marine lineages (Figure 4A), with some site-dependent variability. Sequences from sandy soil sites were dispersed among sequences from soil/sediment, sediment/soil and marine clusters, whereas sequences from the clayey sites were mainly related to the soil/sediment and marine clusters (Figure A3 in Appendix). The soil type effect detected by multivariate analyses on DGGE data was also observed for clone libraries, as determined by UniFrac analysis of the archaeal amoA sequences (Figure 5A).
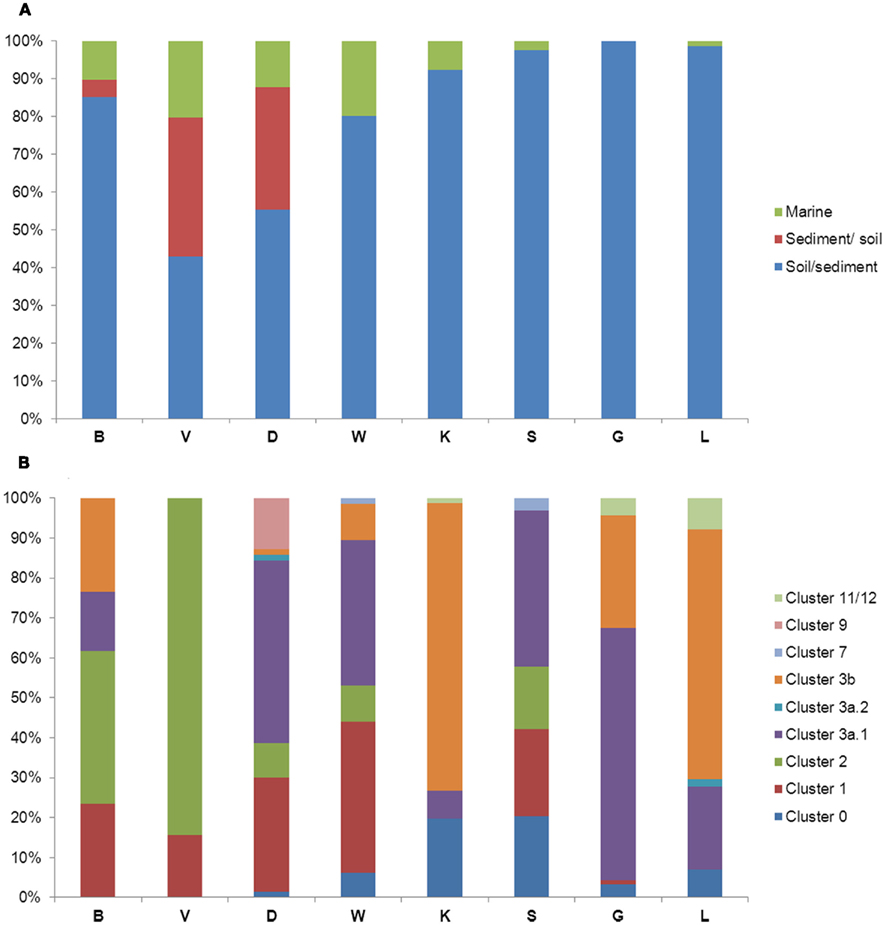
Figure 4. Diversity of archaeal (A) and bacterial (B) amoA sequences retrieved in this study from the eight soils. Archaeal and bacterial amoA clusters were classified according to Nicol et al. (2008) and Zhang et al. (2009), respectively. See Figure 1 for soil names.
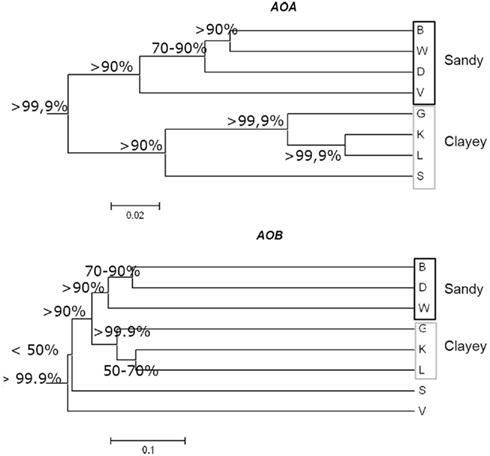
Figure 5. Dendrogram based on AOA (A) and AOB (B) amoA clone libraries, showing the differences in the community structure from eight soils. Distance matrices generated with UniFrac were used to cluster the soils using UPGMA; and jackknife analysis was used to evaluate how robust each environment cluster is to sample size and evenness. Numbers indicate the frequency with which nodes were supported by jackknife analysis.
Regarding the analysis of bacterial amoA gene fragments, almost all bacterial clone sequences represented amoA-like sequences that grouped with Nitrosospira clusters, one grouping with Nitrosomonas, although some of them showed no similarity with any known cluster (Figure A4 in Appendix). Most of the bacterial sequences were found spread over eight clusters and a high site-dependent variability was observed (Figure 4B). For instance, Nitrosospira cluster 3b was predominant in soils K and L, whereas cluster 11/12 comprised 40 and 90% of the sequences in soils B and V, respectively. We further found that the sequences tended to cluster according to soil type, being Nitrosospira clusters 1 and 2 mainly represented in the sandy soils and Nitrosospira cluster 0 mostly in the clayey soils. This was also confirmed by UniFrac analysis of the sequences (Figure 5B).
Seasonal Variation of NEA in Relation to Biological Parameters
Nitrifying enzyme activity was positively correlated with the abundance of AOA (r = 0.74, P ≤ 0.05), but not with that of AOB (Table 2). Changes in nitrification rates were also significantly correlated with changes in the community structures of the AOA and AOB, which were observed to vary with season and soil type (Table 4; Table A3 in Appendix). The analyses over time revealed high correlation values between NEA and AOA community structures at all times (except June 2010). These were season dependent, as correlations with the AOA communities were higher at the end of the season (September and November) and lower at the start (April and June). Correlations between activity and AOB community were higher at the start (June 2010) and lower at the end (September 2009 and October 2010).
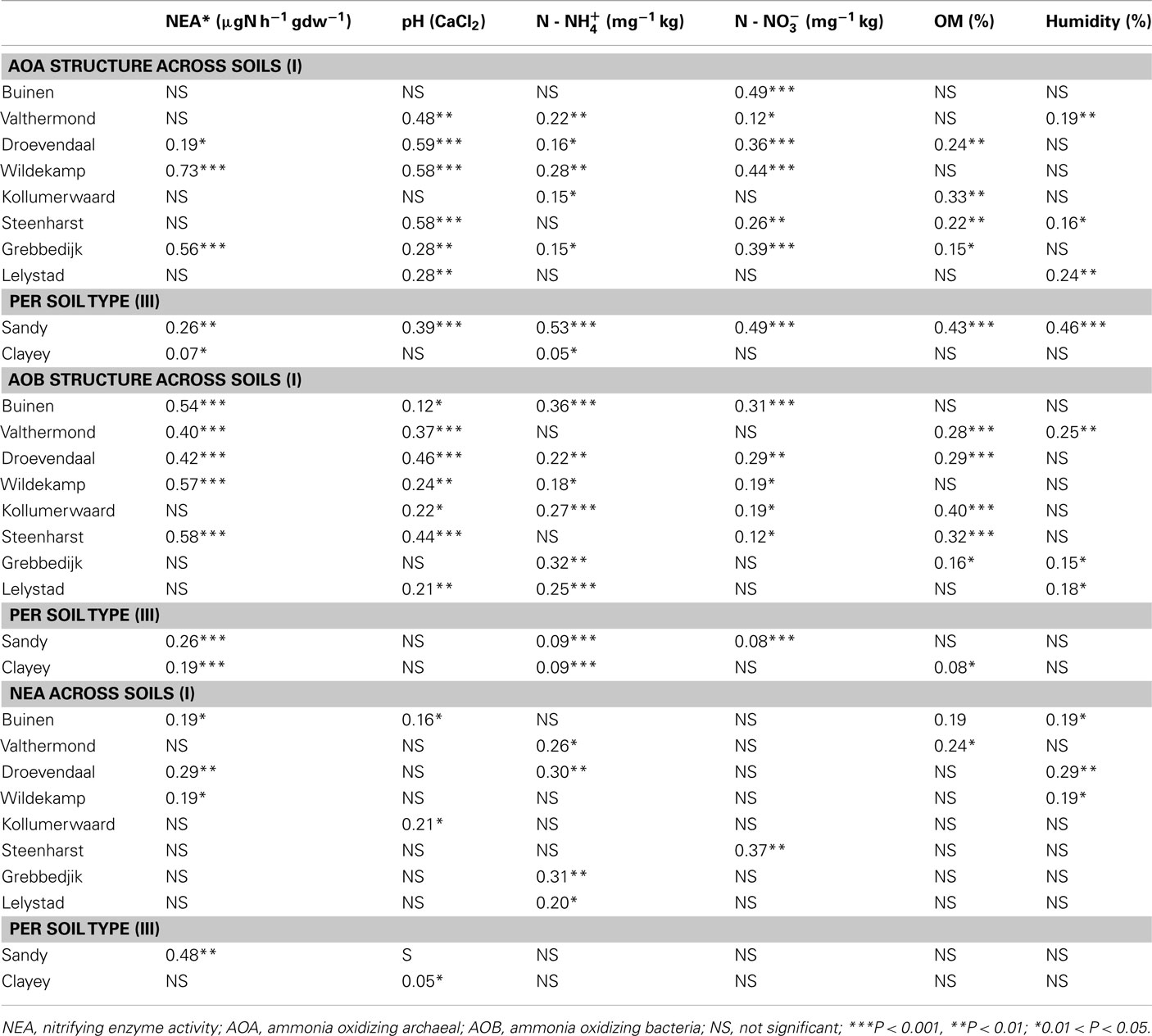
Table 4. Correlations between the community structure AOA and AOB, soil chemical parameters (pH, OM %, clay content % and water content %) and NEA (Relate Analysis) obtained with Primer-E (BEST test), for all sampling times per soil and per soil type.
Overall, NEA was affect mainly by the abundance and diversity (Shannon index from DGGE profiles) of AOA, which together explained 17% of the variation in nitrifying activities (Table 3). The results observed were similar for the sandy soils; however in the clayey soils only diversity, but not abundance, of AOB seemed to play a significant – although small – role (Table 3).
Discussion
Temporal and Spatial Variation in Potential Nitrification Rates in Relation to Chemical Parameters
In general, the rates of potential nitrification in soil have been found to vary greatly, whether in an agricultural field, natural grassland or a forest soil, with observed amplitudes of variation being soil-dependent. Rates of 40–132 μg have been observed in acid agricultural soils in China (Yao et al., 2011) and of 20–120 μg
in a forest soil in the UK (Wheatley et al., 2003). In a field under intensive cultivation in a wheat–barley–potato rotation, rates were found to vary from around 5 to 127 μg
(March to August 1998), and from 120 and 180 to 20 μg
(June to January 2000). In the soils analyzed by us, the nitrification rates varied significantly over time, although the values were much lower than the abovementioned ones, from 0.59 to 0.79 μgN h−1. This variation could be mainly attributed to two soil parameters, soil pH and soil texture. Interestingly, positive correlations between pH and NEA were consistently found, which might be explained by the fact that at lower pH values an increasingly higher number of ammonia oxidizers is inhibited (Webster et al., 2002).
This indicates that patterns of NEA become even more complex when including the perspective of time. Nevertheless, such overall process parameters are important, as they constitute the “normal” amplitude of variation found across soil systems. Regarding the influence of soil parameters, several soil factors are known to influence the potential nitrification rates. For instance, the rates of potential nitrification were found to increase with decreasing salinity (Caffrey et al., 2007) and with increasing temperature up to 30°C (Tourna et al., 2008). The rates are known to be significantly reduced in acid soils (de Boer and Kowalchuk, 2001), although only a slightly significant negative relationship between nitrification and pH was observed in organic soils (Booth et al., 2005).
Abundance and Structure of Ammonia Oxidizing Communities as Affected by Soil Chemical Parameters
It is important to understand how the nitrification process is impacted by soil conditions, and also how and to what extent the structure, composition and abundance of the ammonia oxidizing communities are affected, as the latter may coincide with altered rates. The population sizes of the AOA and AOB across soils and times were found to be within the range observed in other soil systems (Shen et al., 2008; Hallin et al., 2009; Wessén et al., 2011). However, the AOA/AOB ratio’s observed in our study were lower than those previously reported (Leininger et al., 2006). In recent work, levels of 106–107 amoA gene copy numbers per g dry soil have been observed for the AOA and AOB in agricultural soils (Gubry-Rangin et al., 2010; Wessén et al., 2011), although AOA numbers of up to 108 and AOB numbers of 107 have been observed in different agricultural soils (Leininger et al., 2006). Moreover, amoA gene numbers as low as 104 have been found for AOB in flooded paddy soils (Chen et al., 2010) and non-fertilized agricultural soil (Leininger et al., 2006). We found significant seasonal variation in the abundances of AOA and AOB. Temporal variations in the abundances of AOB and AOB were also observed in a 2-year study of the influence of different soil management techniques (Le Roux et al., 2008). Moreover, such variations were already hypothesized to occur in grassland and cropping systems (Berg and Rosswall, 1987).
Several soil factors, such as water content, seasonality and fertilizer type, are thought to affect the population sizes and community structures of ammonia oxidizers in soil (Nugroho et al., 2006; Schmidt et al., 2007; Hansel et al., 2008). In this study, a highly positive correlation of soil pH with the abundance of AOA, but not with the abundance of AOB, was observed. Although soil pH is known to drive changes in the AOA and AOB communities (Nicol et al., 2008; Erguder et al., 2009), its effects are still controversial, as decreases in AOA abundances have been observed both with decreasing (Hallin et al., 2009), and increasing soil pH (Nicol et al., 2008). Other factors, such as soil moisture and nitrogen availability, are also known to influence the ammonia oxidizing communities (He et al., 2007; Hallin et al., 2009). It has previously been shown that AOA are more abundant in soils with lower levels of available nitrogen, whereas AOB become more abundant in soils under higher levels (Jia and Conrad, 2009). However, in the current study, none of these factors (moisture and N availability) determined the AOA/AOB community sizes. This may indicate either that the abundances are not affected by these drivers or, most probably, that the drivers are not the same across soils. Several previous studies proposed soil type to be the primary determinant of the bacterial composition in arable soils (Gelsomino et al., 1999; Girvan et al., 2003), but only few studies have addressed the effect of soil type on AOA and AOB abundance. Wessén et al. (2011) found that the abundance of AOA was negatively affected by clay content, which could be indicative of the AOA being less abundant in the supposedly nutrient-rich environments. However, in our study we did not find significant correlations between AOA abundance and clay content.
The community structure analyses by MWA indicated higher changes for the AOB, whereas the observed changes were lower for AOA. MWA describes the stability and species turnover over time; hence, a 65% change in AOB community structure between April and June means that from April to June the AOB community was very dynamic. In fact, the two communities shared only 35% of phylotypes and 65% changed over this time period. The AOA community was less dynamic than AOB over this time period, as shown by the lower percentage of change. Multivariate analysis revealed that the variables that contributed the most to changes in the system were soil clay content, OM, and pH. In fact, taking the differences in soil texture into account, we increased the resolving power of the method, allowing the detection of significant differences in the pattern.
An effect of soil texture on the phylogenetic make-up of the AOA was also observed, as sequences from the sandy soils formed a cluster that was separate from those from the clayey ones (supported by UniFrac). Analyses of the bacterial amoA genes showed a dominance of Nitrosospira clusters 3a.1 and 3b, which was mainly due to their dominance in the clayey soils. Conversely, in the sandy soils, Nitrosospira clusters 1 and 2 were dominant. This is consistent with findings by Stephen et al. (1996), who detected a dominance of Nitrosospira cluster 3 in pH-neutral agricultural soils versus Nitrosospira cluster 2 in more acidic soils; this followed a classification of AOB clusters defined in other studies (Avrahami and Conrad, 2003).
Exploring the Effect of Different Aspects of Ammonia Oxidizing Communities on Nitrification Rates
Strong correlations were found between NEA and AOA abundances (Table 2). Moreover, when studying AOA and AOB community structures, higher correlations of NEA were found with AOA than with AOB at all times (Table A3 in Appendix). In contrast, Morimoto et al. (2011) found that nitrification rates in a low-humic Andosol soil correlated more with the abundance of AOB, suggesting that the relative importance of AOA or AOB to nitrification is site-dependent. The composition of the AOA and AOB communities, and their potential niches, also play roles in soil nitrification rates. Phylogenetic analyses of archaeal amoA genes showed that the sequences retrieved were quite similar to sequences found in previous studies (Figures A3 and A4 in Appendix). Moreover, the diversity was low, as indicated from rarefaction analysis. Although no study has been able to clearly link the rate of nitrification with the presence of distinct AOA or AOB groups, it was recently reported that higher nitrification rates were observed in sediments dominated by phylogenetically more diverse archaeal amoA sequences (Wankel et al., 2010). This goes against our findings, which revealed that higher nitrification rates occurred in less diverse soil assemblages, indicating that just a few dominant types maybe be responsible for the nitrification process in these soils.
Establishing the NOR of Nitrification in Agricultural Soils
In the past decades, a lot of attention has been given to the effect of external disturbances on soil microbial communities (Mendum, 2002; Wertz et al., 2007; Bardgett et al., 2008; Drenovsky et al., 2010; Pereira e Silva et al., 2011). For instance, the effects of alien plant species or GM plants on the sizes, structures and compositions of microbial communities (Inceoglu et al., 2011) have been addressed. This applies also to the introduction of new plant cultivars (GM or non GM), and any concomitant changes in agricultural practices, such as mechanization, different plowing regimen, planting times and pest controls, which might cause disturbances in soil microbial processes. An elegant approach to determine the effects of such factors on field soil is to evaluate whether their strength is enough to affect processes in such a way that these fall outside of what would be considered normal. In order to do so, we need to know the NOR of the process under “normal” or natural conditions. In the context of our work, we propose the definition of a NOR for agricultural soil by determining the highs and lows in selected processes relevant for ecosystem functioning. Thus, a range of soil attributes or properties (indicators) are selected, which are representative of a process that is sensitive to external drivers and easily measured and whose changes can be monitored through time, as previously suggested (Bruinsma et al., 2003; Kowalchuk et al., 2003). Second, it is crucial to take measurements from long-term datasets, possibly across several sites, to enable the capturing of environmental fluctuations that are independent of spatial and temporal scales. Third, only after implementing the appropriate NOR in a model can the concept of an overall soil NOR be fully operational.
We considered the oxidation of ammonia as such a sensitive process, and established the natural fluctuations by analyzing community structure, abundance and activity of ammonia oxidizers in eight soils over 2 year. We took into account seasonal influences, management practices, addition of fertilizers and crop rotation, representing the “normal” conditions. We observed that the drivers of the changes in structure, abundance and activity were mainly clay content and soil pH, although other soil parameters were also found to affect the structures of these communities, e.g., nitrate and ammonium. All analyses performed indicated a strong effect of soil type, roughly defined in sandy and clayey. Although this division is somewhat loose, and other factors such as pH co-vary with soil type, it enabled us to detect significant differences in the NOR. The relevance of soil type has also been found in studies focusing on macroorganisms, in which gene expression of the soil-dwelling collembolan Folsomia candida was differentially regulated in clayey versus sandy soil (de Boer et al., 2011). These results suggest that differences in chemical composition observed between sandy and clay soils are of great relevance when studying soil organisms in general, suggesting that a soil type-dependent NOR should be envisaged. One can argue that the timeframe used in our study is relatively short for definitive conclusions. However, the soils used in this study are subjected to the same agricultural practice for many years already, and thus a 2-year study was considered a sufficiently sound first step allowing the definition of the variation that might be considered normal for these sites. Moreover, from the data we may already glean a basis for a possible NOR.
Overall, the NOR of potential nitrification was also different between sandy and clayey soils, being lower and less variable over time in the sandy than in the clayey soils. Moreover, the biological and chemical parameters measured were better able to predict nitrification rates in sandy soils. Both ammonia oxidizing communities were sensitive to the parameters associated with soil type, and fluctuated differently among each other, as well as within soils with contrasting texture and pH. MWA showed the AOB communities to fluctuate more, indicating a more dynamic community with higher species turnover than AOA. Furthermore, the diversity of both communities differed greatly between sandy and clayey soils. This soil type-specific response indicated that different aspects of the bacterial and archaeal ammonia oxidizers should be taken into account when evaluating the effect of external disturbances on nitrification. Understanding the normal fluctuations of these soil communities and determining how environmental variations structure them will allow the provision of a key monitoring tool (Magurran et al., 2010). In this context, the NOR of soil functioning will allow us to define normality and to grasp the mechanisms responsible for variation, enabling us to describe the impact of perturbations on the process measured.
Conclusion
To be able to assess the impact of disturbances on soil microbial community structure and function, it is imperative to obtain complete knowledge of the “normal” sources of variation, the extent to which they influence soil microorganisms and the possible outcomes of this interaction. Accordingly, the collection of a large data set, like the current one, should be encouraged for both natural ecosystems and agricultural areas. These types of data will be of key relevance when evaluating the impact of GM plants or global change on soil ecosystem services. We propose here that, to establish the NOR of nitrification in agricultural soils, both the AOA and AOB abundances and community structures should be considered in addition to the nitrifying activities. The conspicuous differences regarding soil type dictate the establishment of NORs per soil type. However, field studies comparable to the current one, performed across time, remain necessary to evaluate the extent and direction of the variations that underpin the NOR of nitrification.
Conflict of Interest Statement
The authors declare that the research was conducted in the absence of any commercial or financial relationships that could be construed as a potential conflict of interest.
Acknowledgments
This work was supported by the NWO-ERGO Program and was part of a collaborative project with Utrecht University, Utrecht, The Netherlands. We would like to thank our colleagues Alexander V. Semenov and Jolanda Brons, and our Utrecht partners, Heike Schmitt and Agnieszka Szturc, for their help with sampling, data analysis and interesting discussions. The authors thank Stefanie Töwe, from the Institute of Soil Ecology, German Research Center for Environmental Health (GmbH), Neuherberg, for support and help with real time PCR data analysis.
References
Avrahami, S., and Conrad, R. (2003). Patterns of community change among ammonia oxidizers in meadow soils upon long-term incubation at different temperatures. Appl. Environ. Microbiol. 69, 6152–6164.
Bardgett, R. D. F., Ostle, C., and Nicholas, J. (2008). Microbial contributions to climate change through carbon cycle feedbacks. ISME J. 8, 805–814.
Berg, P., and Rosswall, T. (1987). Seasonal variations in abundance and activity of nitrifiers in four arable cropping systems. Microb. Ecol. 13, 75–87.
Booth, M. S., Stark, J. M., and Rastetter, E. (2005). Controls on nitrogen cycling in terrestrial ecosystems: a synthetic analysis of literature data. Ecol. Monogr. 75, 139–157.
Bruinsma, M., Kowalchuk, G. A., and van Veen, J. A. (2003). Effects of genetically modified plants on microbial communities and processes in soil. Biol. Fertil. Soils 37, 329–337.
Caffrey, J. M., Bano, N., Kalanetra, K., and Hollibaugh, J. T. (2007). Ammonia oxidation and ammonia-oxidizing bacteria and archaea from estuaries with differing histories of hypoxia. ISME J. 1, 660–662.
Chen, X., Zhang, L., Shen, J., Xu, Z., and He, J. (2010). Soil type determines the abundance and community structure of ammonia-oxidizing bacteria and archaea in flooded paddy soils. J. Soils Sediments 10, 1510–1516.
Clarke, K., and Ainsworth, M. (1993). A method of linking multivariate community structure to environmental variables. Mar. Ecol. Prog. Ser. 92, 205–219.
Dassonville, N., Guillaumaud, N., Piola, F., Meerts, P., and Poly, F. (2011). Niche construction by the invasive Asian knotweeds (species complex Fallopia): impact on activity, abundance and community structure of denitrifiers and nitrifiers. Biol. Invasions 13, 1115–1133.
de Boer, T. E., Birlutiu, A., Bochdanovits, Z., Timmermans, M. J. T., Dijkstra, T. M. H., van Straalen, N. M., Ylstra, B., and Roelofs, D. (2011). Transcriptional plasticity of a soil arthropod across different ecological conditions. Mol. Ecol. 20, 1144–1154.
de Boer, W., and Kowalchuk, G. A. (2001). Nitrification in acid soils: micro-organisms and mechanisms. Soil Biol. Biochem. 33, 853–866.
Doran, J. W., and Zeiss, M. R. (2000). Soil health and sustainability: managing the bioticcomponent of soil quality. Appl. Soil Ecol. 15, 3–11.
Drenovsky, R. E., Steenwerth, K. L., Jackson, L. E., and Scow, K. M. (2010). Land use and climatic factors structure regional patterns in soil microbial communities. Glob. Ecol. Biogeogr. 19, 27–39.
Erguder, T. H., Boon, N., Wittebolle, L., Marzorate, M., and Verstraete, W. (2009). Environmental factors shapping the ecological niches of ammonia oxidizing archaea. FEMS Microbiol. Ecol. Rev. 33, 855–869.
Freitag, T. E., Chang, L., and Prosser, J. I. (2006). Changes in the community structure and activity of betaproteobacterial ammonia-oxidizing sediment bacteria along a freshwater – marine gradient. Environ. Microbiol. 8, 684–696.
Gans, J., Wolinsk, M., and Dunbar, J. (2005). Computacional improvements reveal great bacterial diversity and high metal toxicity in soil. Science 309, 1387–1390.
Gelsomino, A., Keijzer-wolters, A. C., Cacco, G., and van Elsas, J. D. (1999). Assessment of bacterial community structure in soil by polymerase chain reaction and denaturing gradient gel electrophoresis. J. Microbiol. Methods 38, 1–15.
Girvan, M. S., Bullimore, J., Pretty, J. N., Osborn, A. M., and Ballet, A. S. (2003). Soil type is the primary determinant of the composition of the total and active bacterial communities in arable soils. Appl. Environ. Microbiol. 69, 1800–1809.
Gruber, N., and Galloway, J. N. (2008). An earth-system perspective of the global nitrogen cycle. Nature 451, 293–296.
Gubry-Rangin, C., Nicol, G. W., and Prosser, J. I. (2010). Archaea rather than bacteria control nitrification in two agricultural acidic soils. FEMS Microbiol. Ecol. 74, 566–574.
Hallin, S., Jones, C. M., Schloter, M., and Philippot, L. (2009). Relationship between N-cycling communities and ecosystem functioning in a 50-year-old fertilization experiment. ISME J. 3, 597–605.
Hansel, C. M., Fendorf, S., Jardine, P. M., and Francis, C. A. (2008). Changes in bacterial and archaeal community structure and functional diversity along a geochemically variable soil profile. Appl. Environ. Microbiol. 74, 1620–1633.
Hart, S., Nason, G., Myrold, D., and Perry, D. (1994). Dynamics of gross nitrogen transformations in an old-growth forest: the carbon connection. Ecology 75, 880–891.
He, J., Shen, J., Zhang, L., Zhu, Y., Zheng, Y., Xu, M., and Di, H. J. (2007). Quantitative analyses of the abundance and composition of ammonia oxidizing bacteria and ammonia oxidizing archaea of a Chinese upland red soil under long-term fertilization practices. Environ. Microbiol. 9, 2364–2374.
Horz, H.-P., Barbrook, A., Fields, C. B., and Bohannan, B. J. M. (2004). Ammonia-oxidizing bacteria respond to multifactorial global change. Proc. Natl. Acad. Sci. U.S.A. 101, 15136–15141.
Huber, T., Faulkner, G., and Hugenholtz, P. (2004). Bellerophon: a program to detect chimeric sequences in multiple sequence alignments. Bioinformatics 20, 2317–2319.
Inceoglu, O., Al-Soud, W. A., Salles, J. F., Semenov, A. V., and van Elsas, J. D. (2011). Comparative analysis of bacterial communities in a potato field as determined by pyrosequencing. PLoS ONE 6, e23321. doi: 10.1371/journal.pone.0023321
Jeanmougin, F. (1998). Multiple sequence alignment with Clustal X. Trends Biochem. Sci. 23, 403–405.
Jia, Z., and Conrad, R. (2009). Bacteria rather than archaea dominate microbial ammonia oxidation in an agricultural soil. Environ. Microbiol. 11, 1658–1671.
Kowalchuk, G. A., Bruinsma, M., and van Veen, J. A. (2003). Assessing responses of soil microorganisms to GM plants. Trends Ecol. Evol. (Amst.) 18, 403–410.
Kowalchuk, G. A., Stephen, J. R., de Boer, W., Prosser, J. I., Embley, T. M., and Wolderdorp, J. W. (1997). Analysis of ammonia-oxidizing bacteria of the β subdivision of the class proteobacteria in coastal sand dunes by denaturing gradient gel lectrophoresis and sequencing of PCR-amplified 16S Ribosomal DNA fragments. Appl. Environ. Microbiol. 63, 1489–1497.
Kropf, S. (2004). Nonparametric multiple test procedures with data-driven order of hypotheses and with weighted hypotheses. J. Stat. Plan Inference 125, 31–47.
Le Roux, X., Poly, F., Currey, P., Commeaux, C., Hai, B., Nicol, G. W., Prosser, J. I., Schloter, M., Attard, E., and Klumpp, K. (2008). Effects of aboveground grazing on coupling among nitrifier activity, abundance and community structure. ISME J. 2, 221–232.
Leininger, S., Urich, T., Schloter, M., Schwark, L., Qi, L., Nicol, G. W., Prosser, J. I., Schuster, S. C., and Schleper, C. (2006). Archaea predominate among ammonia-oxidizing prokaryotes in soils. Nature 442, 806–809.
Leps, J., and Smilauer, P. (2003). Multivariate Analysis of Ecologic Data Using CANOCO. Cambridge: Cambridge University Press.
Lozupone, C., Hamady, M., and Knight, R. (2006). UniFrac – an online tool for comparing microbial community diversity in a phylogenetic context. BMC Bioinformatics 7, 371. doi: 10.1186/1471-2105-7-371
Magurran, A. E., Baillie, S. R., Buckland, S. T., Dick, J. M., Elston, D. A., Scott, E. M., Smith, R. I., Somerfield, P. J., and Watt, A. D. (2010). Long-term datasets in biodiversity research and monitoring: assessing change in ecological communities through time. Trends Ecol. Evol. (Amst.) 25, 574–582.
Marzorati, M., Wittebolle, L., Boon, B., Daffonchio, D., and Verstraete, W. (2008). How to get more out of molecular fingerprints: practical tools for microbial ecology. Environ. Microbiol. 10, 1571–1581.
McCaig, A. E., Phillips, P. J., Stephen, J. R., Kowalchuk, G. A., Harvey, S. M., Herbert, R. A., Embley, T. M., and Prosser, J. I. (1999). Nitrogen cycling and community structure of proteobacterial b-subgroup ammonia-oxidizing bacteria within polluted marine fish farm sediments. Appl. Environ. Microbiol. 65, 213–220.
Mendum, T. (2002). Changes in the population structure of β-group autotrophic ammonia oxidising bacteria in arable soils in response to agricultural practice. Soil Biol. Biochem. 34, 1479–1485.
Morimoto, S., Hauatsu, M., Hoshino, Y. T., Nagaoka, K., Yamazaki, M., Karasawa, T., Takenaka, M., and Akiyama, H. (2011). Quantitative analyses of ammonia-oxidizing archaea (AOA) and ammonia-oxidizing bacteria (AOB) in fields with different soil types. Microbes Environ. 26, 248–253.
Muyzer, G., de Waal, E. C., and Uitierlinden, A. G. (1993). Profiling of complex microbial populations by denaturing gradient gel electrophoresis analysis of polymerase chain reaction-amplified genes coding for 16S rRNA. Appl. Environ. Microbiol. 59, 695–700.
Nicol, G. W., Leininger, S., Schleper, C., and Prosser, J. I. (2008). The influence of soil pH on the diversity, abundance and transcriptional activity of ammonia oxidizing archaea and bacteria. Environ. Microbiol. 10, 2966–2978.
Nugroho, R., Roling, W., Laverman, A., and Verhoef, H. (2006). Net nitrification rate and presence of Nitrosospira cluster 2 in acid coniferous forest soils appear to be tree species specific. Soil Biol. Biochem. 38, 1166–1171.
Nyberg, K., Schnürer, A., Sundh, I., Jarvis, Å., and Hallin, S. (2006). Ammonia-oxidizing communities in agricultural soil incubated with organic waste residues. Biol. Fertil. Soils 42, 315–323.
Offre, P., Prosser, J. I., and Nicol, G. W. (2009). Growth of ammonia-oxidizing archaea in soil microcosms is inhibited by acetylene. FEMS Microbiol. Ecol. 70, 99–108.
Olsen, G. J., Matsuda, H., and Hagstrom, R. (1994). FASTDNAML – a tool for construction of phylogenetic trees of DNA-sequences using maximum-likehood. Comput. Appl. Biosci. 10, 41–48.
Oved, T., Shaviv, A., Goldrath, T., Mandelbaum, R. T., and Minz, D. (2001). Influence of effluent irrigation on community composition and function of ammonia-oxidizing bacteria in soil. Appl. Environ. Microbiol. 67, 3426–3433.
Pereira e Silva, M. C., Semenov, A. V., van Elsas, J. D., and Salles, J. F. (2011). Seasonal variations in diversity and abundance of diazotrophic communities across soils. FEMS Microbiol. Ecol. 77, 57–68.
Prosser, J. I., and Nicol, G. W. (2008). Relative contributions of archaea and bacteria to aerobic ammonia oxidation in the environment. Environ. Microbiol. 10, 2931–2941.
Rademaker, J. L. W., and de Bruijn, J. (2004). “Computer-assissted analysis of molecular fingerprint profiles and database construction,” in Molecular Microbial Ecology Manual, 2nd Edn, eds G. A. Kowalchuk, F. J. de Bruijn, I. M. Head, A. D. L. Akkermans, and J. D. van Elsas (Dordrecht: Kluwer Academic Publishers), 1397–1446.
Ritz, K., Black, H. I. J., Campbell, C. D., Harris, J. A., and Wood, C. (2009). Selecting biological indicators for monitoring soils: a framework for balancing scientific and technical opinion to assist policy development. Ecol. Indic. 9, 1212–1221.
Rotthauwe, J.-H., Witzel, K.-P., and Liesack, W. (1997). The ammonia monooxygenase structural gene amoA as a functional marker: molecular fine-scale analysis of natural ammonia-oxidizing populations. Appl. Environ. Microbiol. 63, 4704–4712.
Schleper, C., Jurgens, G., and Jonuscheit, M. (2005). Genomic studies of uncultivated archaea. Nat. Rev. Microbiol. 3, 479–488.
Schloss, P. D., and Handelsman, J. (2006). Toward a Census of bacteria in soil. Plos Comp. Biol. 2, e92.
Schmidt, C. S., Hultman, K. A., Robinson, D., Killham, K., and Prosser, J. I. (2007). PCR profiling of ammonia-oxidizer communities in acidic soils subjected to nitrogen and sulphur deposition. FEMS Microbiol. Ecol. 61, 305–316.
Shen, J.-P., Zhang, L.-M., Zhu, Y.-G., Zhang, J.-B., and He, J.-Z. (2008). Abundance and composition of ammonia-oxidizing bacteria and ammonia-oxidizing archaea communities of an alkaline sandy loam. Environ. Microbiol. 10, 1601–1611.
Spang, A., Hatzenpichler, R., Brochier-Armanet, C., Rattei, T., Tischler, P., Spieck, E., Streit, W., Stahl, D. A., Wagner, M., and Schleper, C. (2010). Distinct gene set in two different lineages of ammonia-oxidizing archaea supports the phylum Thaumarchaeota. Trends Microbiol. 18, 331–340.
Stephen, J. R., Chang, Y.-J., Macnaughton, S. J., Kowalchuk, G. A., Leung, K. T., Flemming, C. A., and White, D. C. (1999). Effect of toxic metals on indigenous soil b-subgroup proteobacterium ammonia oxidizer community structure and protection against toxicity by inoculated metal-resistant bacteria. Appl. Environ. Microbiol. 65, 95–101.
Stephen, J. R., McCaig, A. E., Smith, Z., Prosser, J. I., and Embley, T. M. (1996). Molecular diversity of soil and marine 16S rRNA gene sequences related to beta-subgroup ammonia-oxidizing bacteria. Appl. Environ. Microbiol. 62, 4147–4154.
Torsvik, V., Goksøyr, J., and Daae, F. L. (1990). High diversity in DNA of soil bacteria. Appl. Environ. Microbiol. 56, 782–787.
Tourna, M., Freitag, T. E., Nicol, G. W., and Prosser, J. I. (2008). Growth, activity and temperature responses of ammonia-oxidizing archaea and bacteria in soil microcosms. Environ. Microbiol. 10, 1357–1364.
Töwe, S., Albert, A., Kleneidam, K., Brankatschk, R., Dumig, A., Welzl, G., Much, J. C., Zeyer, J., and Schloter, M. (2010). Abundance of microbes involved in nitrogen transformation in the rhizosphere of Leucanthemopsis alpina (L.) Heywood grown in soils from different sites of the Damma glacier forefield. Microb. Ecol. 60, 762–770.
Treusch, A. H., Leininger, S., Kletzin, A., Schuster, S. C., Klenk, H.-P., and Schleper, C. (2005). Novel genes for nitrite reductase and Amo-related proteins indicate a role of uncultivated mesophilic crenarchaeota in nitrogen cycling. Environ. Microbiol. 7, 1985–1995.
van Straalen, N. M. (2002). Assessment of soil contamination – a functional perspective. Biodegradation 13, 41–52.
Wankel, S. D., Mosier, A. C., Hansel, C. M., Paytan, A., and Francis, C. A. (2010). Spatial variability in nitrification rates and ammonia-oxidizing microbial communities in the agriculturally-impacted Elkhorn Slough estuary. Appl. Environ. Microbiol. 77, 269–280.
Webster, G., Embley, T. M., and Prosser, J. I. (2002). Grassland management regimens reduce small-scale heterogeneity and species diversity of b-proteobacterial ammonia oxidizer populations. Appl. Environ. Microbiol. 68, 20–30.
Wertz, S., Degrange, V., Prosser, J. I., Poly, F., Commeaux, C., Guillaumaud, N., and Le Roux, X. (2007). Decline of soil microbial diversity does not influence the resistance and resilience of key soil microbial functional groups following a model disturbance. Environ. Microbiol. 9, 2211–2219.
Wessén, E., and Hallin, S. (2011). Abundance of archaeal and bacterial ammonia oxidizers – possible bioindicator for soil monitoring. Ecol. Indic. 11, 1696–1698.
Wessén, E., Söderström, M., Stenberg, M., Bru, D., Hellman, M., Welsh, A., Thomsen, F., Klemedtson, L., Philippot, L., and Hallin, S. (2011). Spatial distribution of ammonia-oxidizing bacteria and archaea across a 44-hectare farm related to ecosystem functioning. ISME J. 5, 1213–1225.
Wheatley, R. E. W., Caul, S., Crabb, D., Daniell, T. J., Griffiths, B. S., and Ritz, K. (2003). Microbial population dynamics related to temporal variations in nitrification in three arable fields. Eur. J. Soil Sci. 54, 707–714.
Yao, H., Gao, Y., Nicol, G. W., Campbell, C. D., Prosser, J. I., Zhang, L., Han, W., and Singh, B. K. (2011). Links between ammonia oxidizer community structure, abundance, and nitrification potential in acidic soils. Appl. Environ. Microbiol. 77, 4618–4625.
Ying, J.-P., Zhang, L.-M., and He, J.-Z. (2010). Putative ammonia-oxidizing bacteria and archaea in an acidic red soil with different land utilization patterns. Environ. Microbiol. Rep. 2, 304–312.
Zhang, L.-M., Wang, M., Prosser, J. I., Zheng, Y.-M., and He, J.-Z. (2009). Altitude ammonia-oxidizing bacteria and archaea in soils of Mount Everest. FEMS Microbiol. Ecol. 70, 52–61.
Appendix
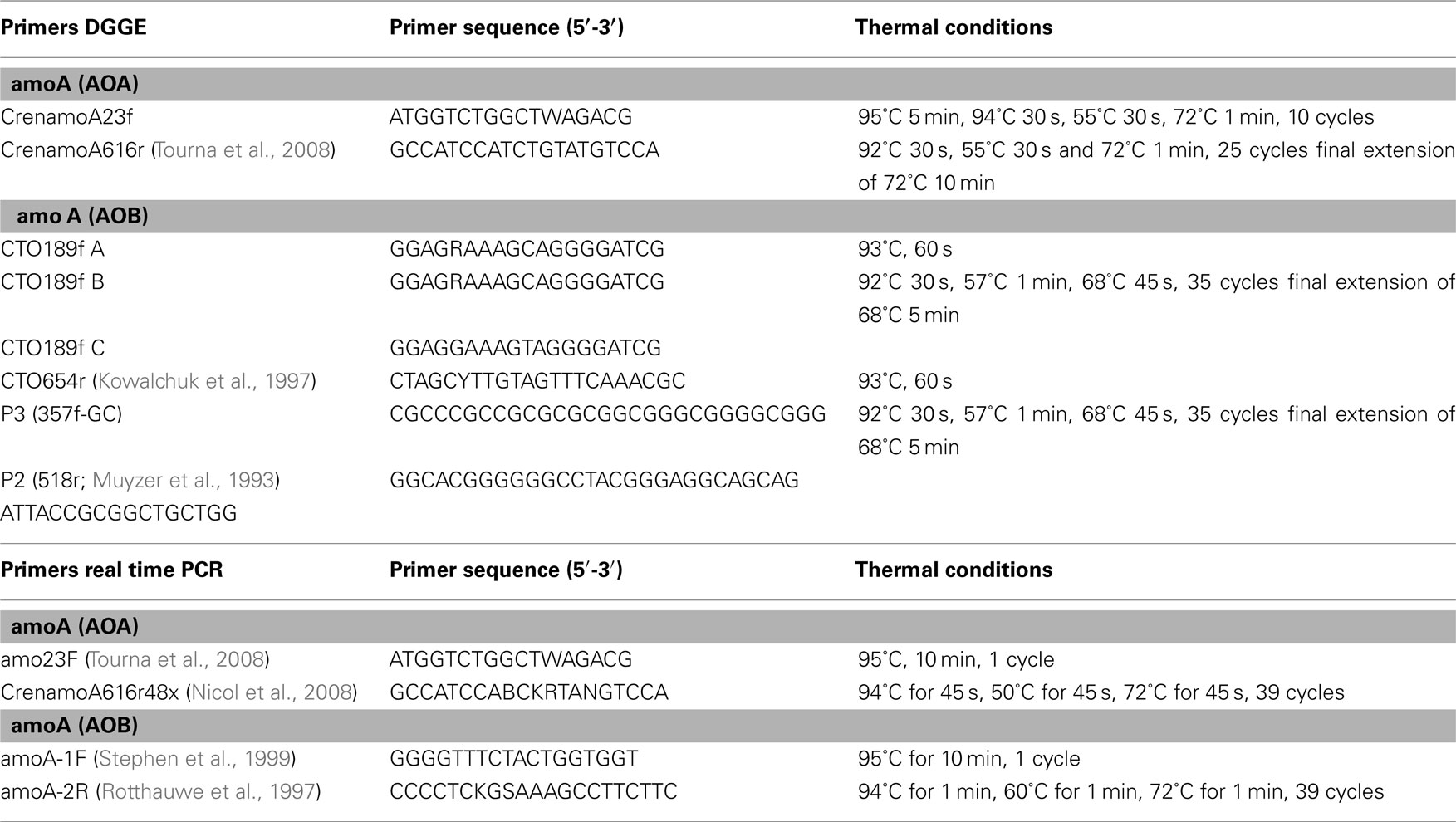
Table A2. PCR and cycling conditions for PCR-DGGE analysis and real time quantification of AOA and AOB genes.
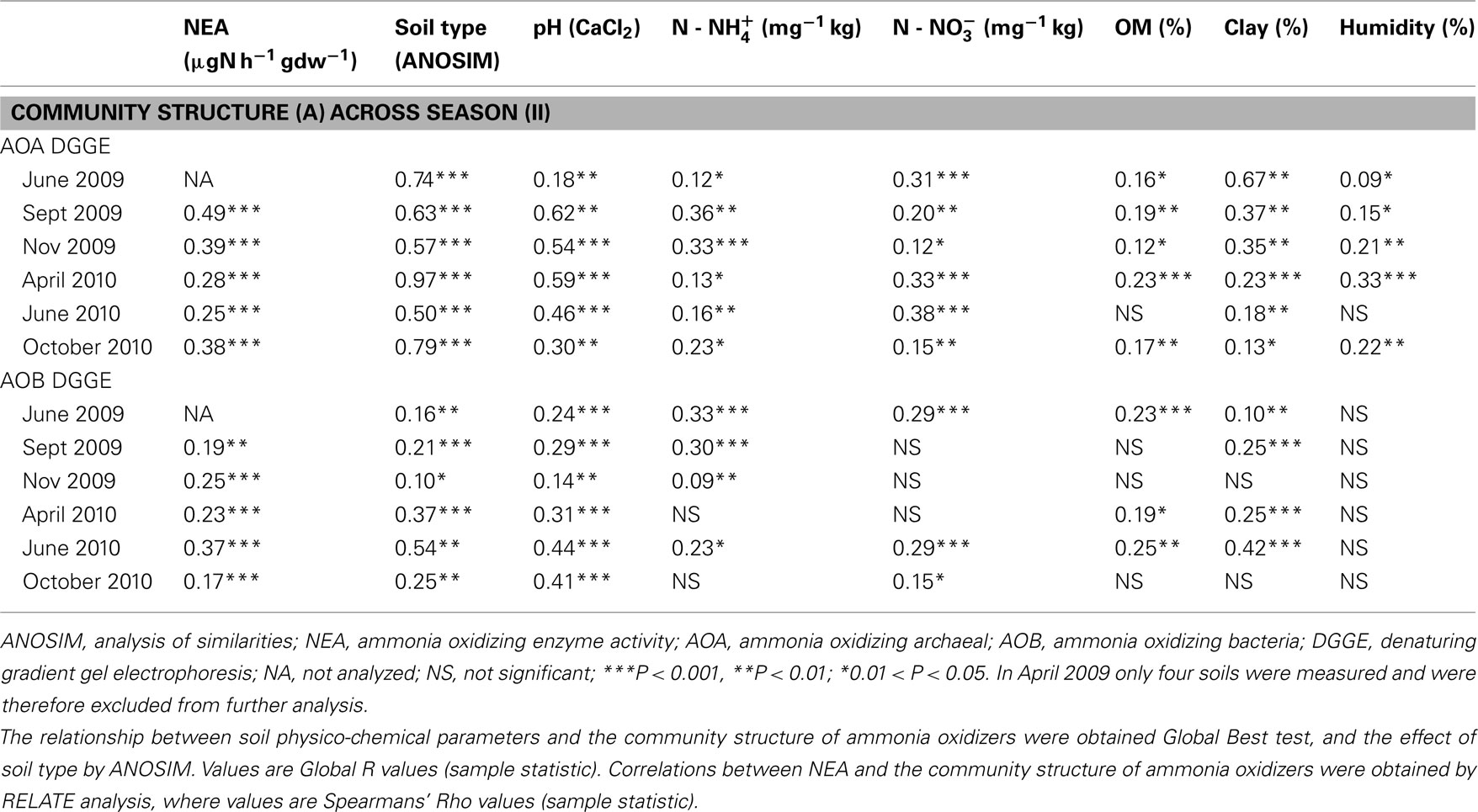
Table A3. Correlations between the similarity matrices of community structure AOA and AOB, soil chemical parameters (pH, OM %, clay content % and water content %) and NEA (relate analysis; NEA from September 2009 to October 2010) obtained with Primer-E (BEST test), for all sampling times.
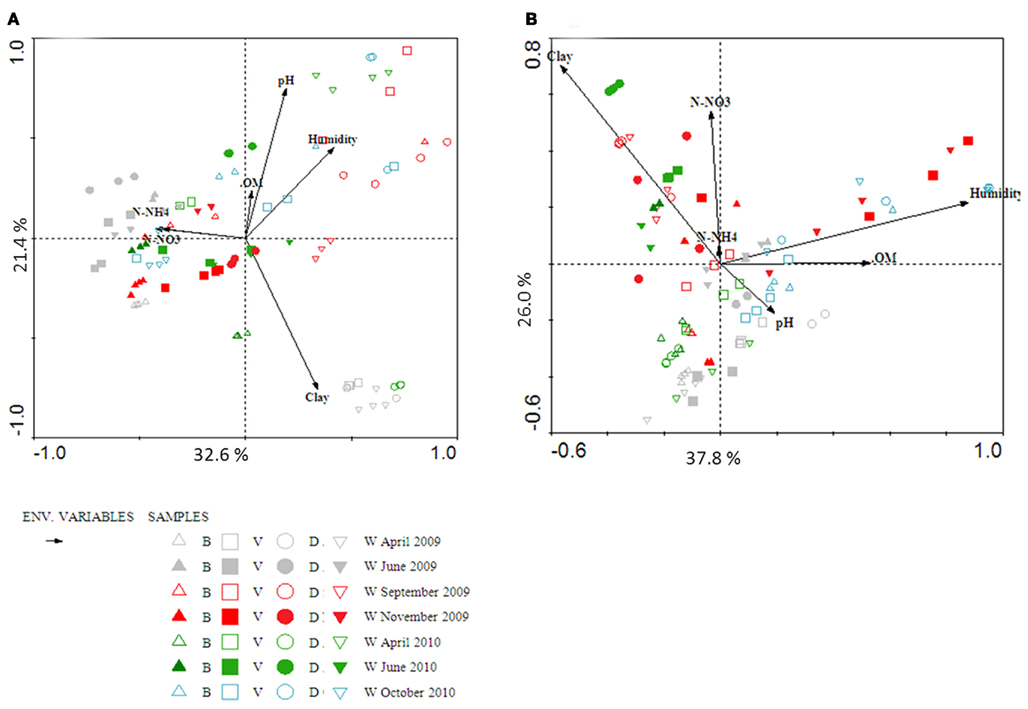
Figure A1. Biplots of canonical correspondence analysis of archaeal amoA gene (A) and beta-proteobacterial 16S rRNA gene (B) from DGGE data obtained from four sandy soils over 2 years. Physico-chemical data, soil moisture (Humidity), soil nitrate (N-NO3), soil ammonium (N-NH4), organic matter (OM), clay content (clay), soil pH (pH) are presented with black arrows.
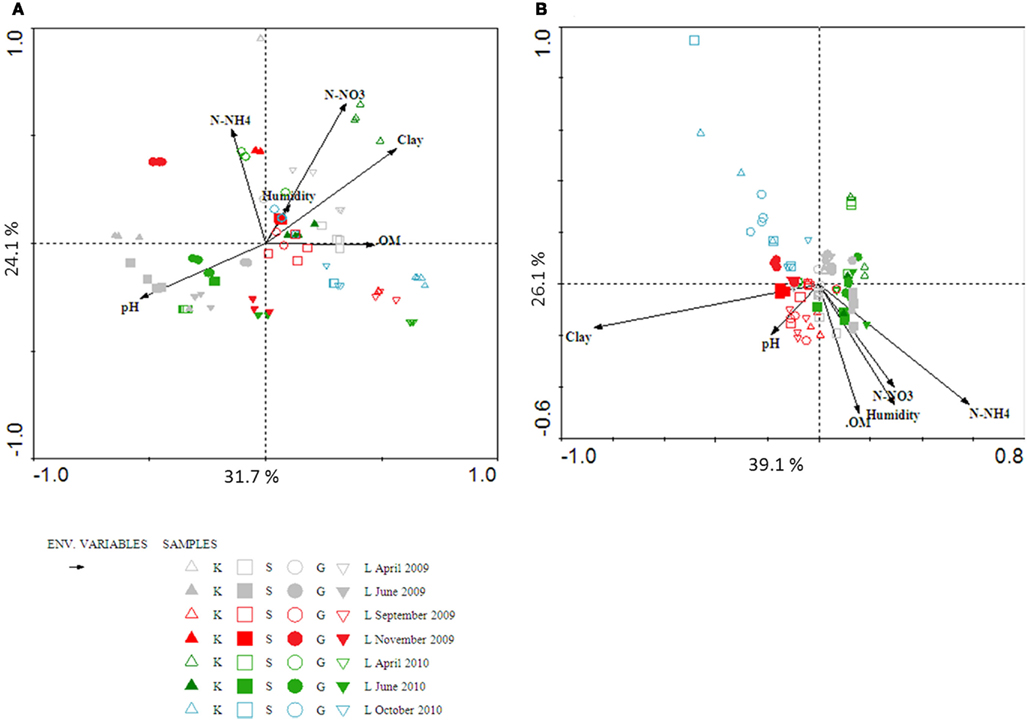
Figure A2. Biplots of canonical correspondence analysis of archaeal amoA gene (A) and beta-proteobacterial 16S rRNA gen (B) from DGGE data obtained from four clayey soils over 2 years. Physico-chemical data, soil moisture (Humidity), soil nitrate (N-NO3), soil ammonium (N-NH4), organic matter (OM), clay content (clay), soil pH (pH) are presented with black arrows.
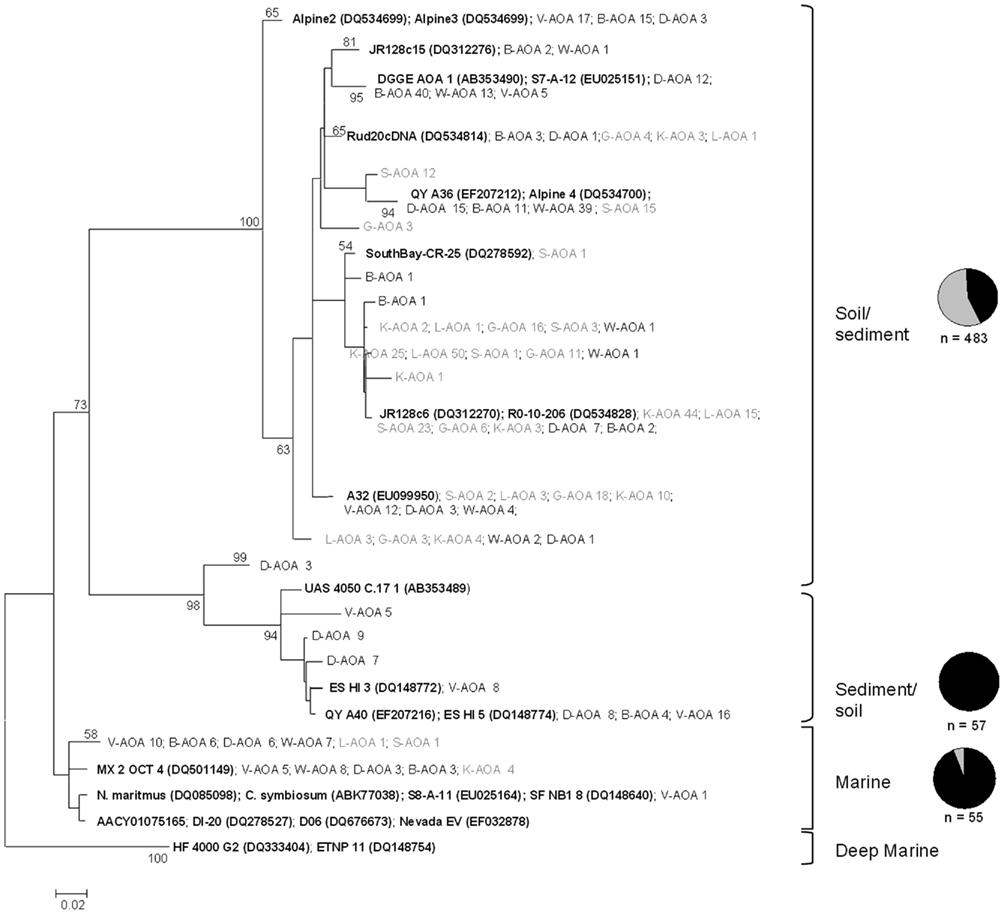
Figure A3. Phylogenetic analysis of 625 archaeal amoA partial amino acid sequences retrieved in this study from the eight soils. Sequences from sandy soils are represented in black and sequences from clayey soils in gray. Clades were classified according to Nicol et al. (2008). Pie charts represent the percentage of sequences found in sandy and clayey soil and N values are total number of sequences found in the clade. Bootstrap support (>50) represent values from Neighbor-joining, using JTT substitution model (1000 replicates and 8 gamma rates; expressed as percentage). First letters represent the soil of origin followed by the number of clones found in that specific soil. Reference sequences are in bold described as “Name (accession number).” The tree was rooted with two sequences within the deep marine water clade.
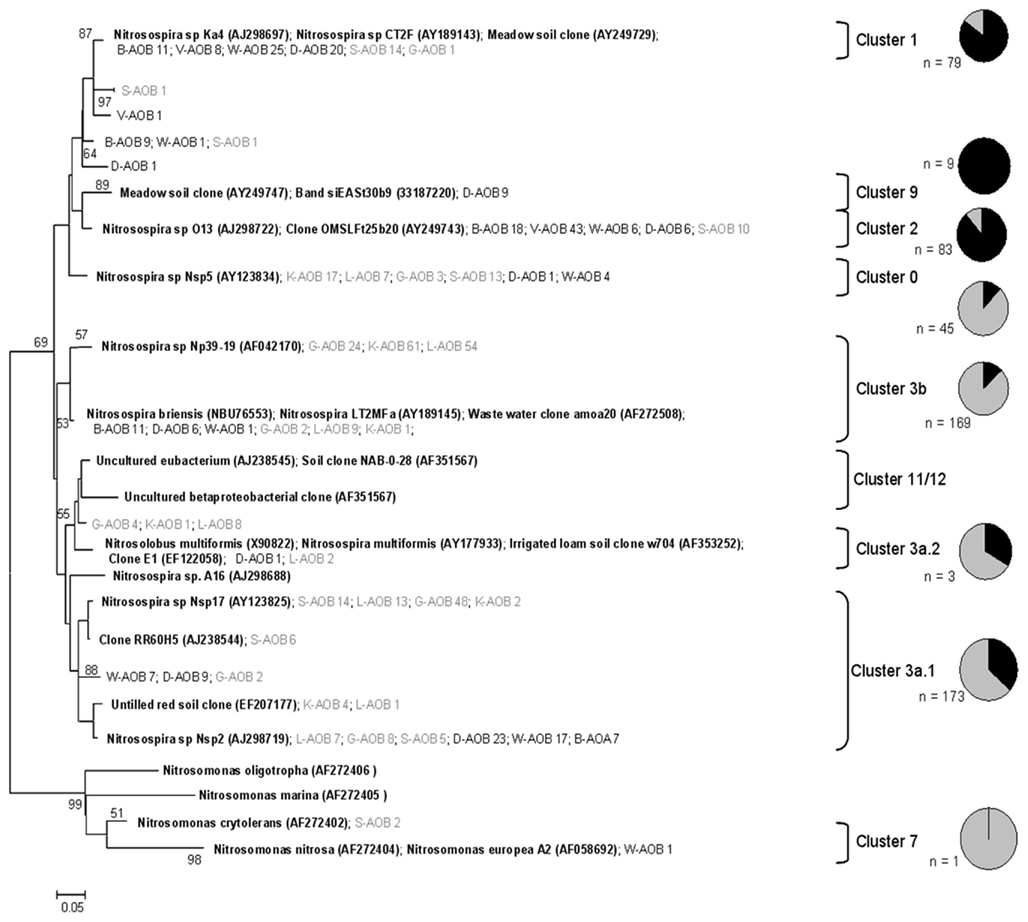
Figure A4. Phylogenetic analysis of 583 bacterial amoA partial amino acid sequences retrieved in this from the eight soils. Sequences from sandy soils are represented in black and sequences from clayey soils in gray. Clades were classified according to Zhang et al. (2009). Pie charts represent the percentage of sequences found in sandy and clayey soil and N values are total number of sequences found in the clade. Bootstrap support (>50) represent values from Neighbor-joining, using JTT substitution model (1000 replicates and 8 gamma rates; expressed as percentage). First letters represent the soil of origin followed by the number of clones found in that specific soil. Reference sequences are in bold described as “Name (accession number).” The tree was rooted with four sequences within the Nitrosomonas clade.
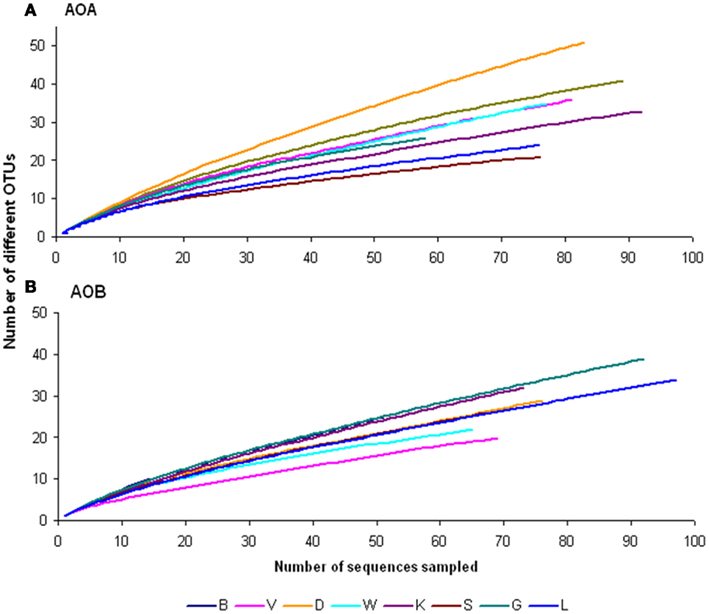
Figure A5. Rarefaction curves of observed operational taxonomic units (OTU) based on archaeal (A) and bacterial (B) amoA sequences retrieved from the eight soils, determined by DOTUR (Schloss and Handelsman, 2006). See legend from Figure 1 for soil names.
Keywords: nitrification, NOR, AOA, AOB, soil type, pH
Citation: Pereira e Silva MC, Poly F, Guillaumaud N, van Elsas JD and Salles JF (2012) Fluctuations in ammonia oxidizing communities across agricultural soils are driven by soil structure and pH. Front. Microbio. 3:77. doi: 10.3389/fmicb.2012.00077
Received: 13 December 2011; Accepted: 14 February 2012;
Published online: 02 March 2012.
Edited by:
Lisa Y. Stein, University of Alberta, CanadaReviewed by:
Christopher Blackwood, Kent State University, USALevente Bodrossy, CSIRO Marine and Atmospheric Research, Australia
Copyright: © 2012 Pereira e Silva, Poly, Guillaumaud, van Elsas and Salles. This is an open-access article distributed under the terms of the Creative Commons Attribution Non Commercial License, which permits non-commercial use, distribution, and reproduction in other forums, provided the original authors and source are credited.
*Correspondence: Joana Falcão Salles, Department of Microbial Ecology, Centre for Life Sciences, University of Groningen, P.O. BOX 11103, 9700 CC Groningen, The Netherlands. e-mail: j.falcao.salles@rug.nl