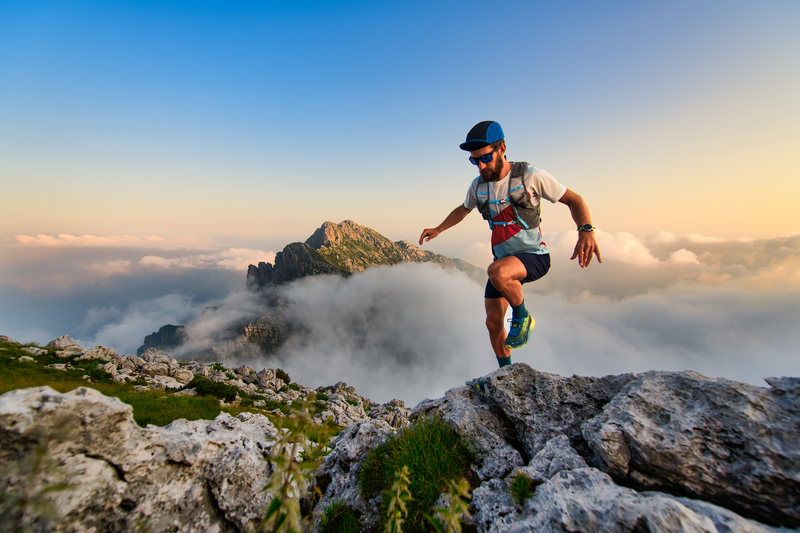
95% of researchers rate our articles as excellent or good
Learn more about the work of our research integrity team to safeguard the quality of each article we publish.
Find out more
PERSPECTIVE article
Front. Microbiol. , 17 October 2011
Sec. Cellular and Infection Microbiology - closed section
Volume 1 - 2011 | https://doi.org/10.3389/fmicb.2011.00188
This article is part of the Research Topic Human health and disease in a microbial world View all 13 articles
The human intestinal microbiota encode multiple critical functions impacting human health, including metabolism of dietary substrate, prevention of pathogen invasion, immune system modulation, and provision of a reservoir of antibiotic resistance genes accessible to pathogens. The complexity of this microbial community, its recalcitrance to standard cultivation, and the immense diversity of its encoded genes has necessitated the development of novel molecular, microbiological, and genomic tools. Functional metagenomics is one such culture-independent technique, used for decades to study environmental microorganisms, but relatively recently applied to the study of the human commensal microbiota. Metagenomic functional screens characterize the functional capacity of a microbial community, independent of identity to known genes, by subjecting the metagenome to functional assays in a genetically tractable host. Here we highlight recent work applying this technique to study the functional diversity of the intestinal microbiota, and discuss how an approach combining high-throughput sequencing, cultivation, and metagenomic functional screens can improve our understanding of interactions between this complex community and its human host.
A growing body of evidence indicates that human microbial communities play a role in the pathogenesis of diseases as diverse as neonatal necrotizing enterocolitis, asthma, eczema, inflammatory bowel disease, obesity, atherosclerosis, insulin resistance, and neoplasia. Because the composition of the intestinal microbiota is highly variable in early infancy and largely stabilizes by the end of the first year of life, understanding the determinants of the composition of the infant enteric microbial community is of particular interest (Vael and Desager, 2009). The decreased rates of early childhood infections, atopic disease, diabetes, and obesity in breastfed infants have been well-documented (Oddy, 2004; Bartok and Ventura, 2009; Duijts et al., 2009; Le Huerou-Luron et al., 2010; Gouveri et al., 2011), as have the differences in the composition of the intestinal microbiota between breast- and formula-fed infants. In breastfed infants, Bifidobacterium spp. rapidly become the predominant group of organisms (Harmsen et al., 2000), while formula-fed infants develop a different microbial community comprised of some Bifidobacteria and large proportions of other potentially pathogenic organisms, including Bacteroides, Staphylococcus, Enterobacteria, Clostridia, and Enterococcus spp. (Yoshioka et al., 1983; Rubaltelli et al., 1998). Fermentative metabolites generated by Bifidobacterium and other saccharolytic species decrease stool pH, inhibiting the growth of potential pathogens in breastfed infants (Bullen et al., 1976). Relative decreases in the proportion of Bifidobacteria and concomitant increases in other enteric flora in infancy have been linked to disease states later in life: increased numbers of Escherichia coli and Clostridium difficile are associated with the development of atopic disease such as asthma and eczema (Penders et al., 2007), while lower Bifidobacterial counts and greater numbers of Staphylococcus aureus are associated both with overweight mothers (Collado et al., 2010) and an increased risk of the infant becoming overweight in early childhood (Kalliomaki et al., 2008). Bifidobacteria may also enhance intestinal barrier function, decreasing the likelihood of bacterial translocation during periods of metabolic stress (Wang et al., 2006; Ruan et al., 2007). The gastrointestinal microbiota appear essential to the development of the immune system (Round and Mazmanian, 2009), can act as a reservoir for antibiotic resistance genes (van der Waaij and Nord, 2000), and may contribute to chronic inflammatory states (Erridge et al., 2007; Ghanim et al., 2009). Together, these data suggest that understanding the interactions between microbial communities and their human hosts may illuminate the pathogenesis of complex human diseases such as obesity and the metabolic syndrome, atopic disease, and autoimmune disorders, and thereby provide a rich source for mining novel therapeutic approaches.
To understand microbial community effects on human health, both the phylogenetic profile of human microbial communities and the functional capacity of their members must be characterized. Much progress has been made toward these ends using direct bacterial culture, 16S sequencing, shotgun metagenomic sequencing, PCR probing for specific genes, and chemical profiling of microbial metabolites. These approaches have yielded incredible insights ranging from shifts in prevalent bacterial phylotypes and altered metabolic profiles in human subjects with inflammatory bowel disease, variations in the composition of the intestinal microbiota with human diet and functional differences in the gut microbiota related to host body habitus, developmental changes in the composition of the gastrointestinal microbiota during infancy and childhood, and the genetic epidemiology of antibiotic resistance in the intestinal microbiota. (Rimbara et al., 2005; Qin et al., 2006; Turnbaugh et al., 2006; Bezabeh et al., 2009; Jansson et al., 2009; Paliy et al., 2009; Gillevet et al., 2010; Kang et al., 2010; Koenig et al., 2011; Rigsbee et al., 2011). In this perspective, we will focus on the emerging application of functional metagenomic screens, a technique developed for investigating unculturable environmental microbes that neatly complements the aforementioned techniques currently used to characterize the human microbiota.
Direct culture, historically the sine qua non of microbiology, readily provides information on the functional characteristics of the species being investigated. The majority of gastrointestinal microbiota, however, are obligate anaerobes recalcitrant to culture. Traditional estimates are that only 15–20% of the gastrointestinal microbiota are culturable, precluding direct characterization of the majority of bacterial species (Langendijk et al., 1995; Eckburg et al., 2005; Gill et al., 2006). A recent report by Goodman et al. (2011) showed, using high-throughput 16S sequencing in combination with extensive anaerobic culturing, that up to 56% of gastrointestinal microbial species are culturable. Although this represents a dramatic improvement over standard culturing techniques, there remains a significant proportion of unculturable organisms that must be characterized using complementary techniques. Different approaches have been employed to overcome this problem ranging from simple PCR-based screens to large metagenomic sequencing analyses and functional metagenomic screens. Together, these methods have expanded our knowledge about the fraction of the GI tract microbiota that cannot be characterized by culture-based approaches.
Functional metagenomic screens, originally proposed as a method to characterize the unculturable fraction of soil microbiota (Handelsman et al., 1998; Rondon et al., 2000) and successfully used for years to characterize the functional diversity of microbes in a variety of environments (Warnecke et al., 2007; Allen et al., 2009b; Berlemont et al., 2009; Torres-Cortes et al., 2011), has relatively recently been adapted to characterize the functions of human microbial communities, representing an interesting cross-pollination between environmental microbiology and biomedical science. The functional metagenomic screening method, based on clone libraries containing genomic DNA from a microbial community, does not require direct culture of fastidious organisms. Instead, clone libraries are constructed by first extracting and shearing DNA from a sample of a microbial community, then cloning the fragmented DNA into a relevant vector, and subsequently transforming this vector into a suitable host strain (Figure 1). Once a library is constructed, it can be functionally screened by cultivation on selective media or by employing a reporter system. Using this approach, it is possible to identify genes encoding a variety of functions such as antibiotic resistance, metabolism of complex compounds, and modulation of eukaryotic cells. Subsequent sequencing and in silico analysis of the DNA inserts from isolated clones provides information about the source of the genes and the putative mechanisms of action of their products.
Figure 1. Schematic presentation of the processes leading from fecal microbial sample to functional selection of antibiotic resistance genes. Metagenomic DNA is directly extracted from any microbial community (e.g., from a fecal sample) and cloned into an expression system in a cultivable, genetically tractable host strain (e.g., E. coli). Metagenomic transformants harboring DNA fragments that encode antibiotic resistance genes are selected by subjecting the library of clones to specific antibiotics at concentrations which inhibit the growth of the untransformed indicator strain. Selected DNA fragments can then be sequenced to identify the specific resistance genes.
One area of early success for functional metagenomic screens is in the discovery of new antibiotic resistance genes in the human gastrointestinal microbiota. Multidrug resistant bacteria are increasingly prevalent in both hospitals and the community, and pose a growing threat to human health (Boucher et al., 2009; Högberg et al., 2010). Infections with antibiotic resistant organisms are associated with increased mortality and cost of treatment (Maragakis et al., 2008), and novel antibiotic discovery has not kept pace with the emergence of microbial resistance to existing agents (Högberg et al., 2010). In order to develop a rational approach to curtail the emergence of antibiotic resistance in human pathogens, a deeper understanding of the flow of resistance genes within microbial communities is required. Pathogenic organisms present in the environment may acquire resistance genes from soil or water microbes, while commensal gastrointestinal organisms that are continuously exposed to the outside environment via host ingestion of food, may also come in contact with pathogenic bacteria during the course of an infection. Although great strides have been made in recent years documenting genetic resistance reservoirs and patterns of gene flow within and between environmental and human commensal microbiota, fully characterizing the diversity and mobility of the environmental resistome will be crucial to control the emergence of ever more resistant organisms (Aminov and Mackie, 2007; Martinez, 2008; Aminov, 2009; Allen et al., 2010).
Multiple studies demonstrate the efficacy of simple PCR screens in the detection and quantification of antibiotic resistance genes present in the gastrointestinal microbiota. PCR assays have been used to detect the presence of known tetracycline resistance genes (tet) in fecal samples from antibiotic-naive infants (Gueimonde et al., 2006). Similarly, qPCR has been used to quantify the levels of tet and erm genes, which confer resistance to tetracycline and macrolide, lincosamide, and streptogramin B antibiotics respectively, in animal and human waste water (Smith et al., 2004; Auerbach et al., 2007; Chen et al., 2010). The extraordinary specificity of PCR-based studies is also an important limitation of the technique: because PCR can only be used to interrogate a sample for known genes, it is an ineffective method for identifying novel resistance genes.
Functional metagenomic screens obviate this problem by identifying genes by their function in an expression vector rather than by a specific sequence used for PCR probing. Using this approach, novel antibiotic resistance genes have been identified in different environments including oral microbiota, soil microbiota, and moth gut flora (Diaz-Torres et al., 2003; Riesenfeld et al., 2004; Allen et al., 2009a). Sommer et al. (2009) demonstrated the power of metagenomic functional screens to identify novel antibiotic resistance genes in fecal samples from two healthy adults. Metagenomic libraries with a total size of 9.3 Gb (gigabases) and an average insert size of 1.8 kb (kilobases) were screened for resistance against 13 different antibiotics, revealing 95 unique inserts representing a variety of known resistance genes as well as 10 novel beta-lactamase gene families (Sommer et al., 2009). Genes identified using metagenomic functional screens were, on average, 61% identical to known resistance genes from pathogenic organisms, while genes identified via aerobic culturing of isolates from the same individuals had greater than 90% sequence identity to previously described resistance genes. One of the novel resistance genes identified with the functional metagenomic screen had 100% sequence identity to a protein of unknown function, demonstrating the power of metagenomic functional screens to identify novel resistance genes even in fully sequenced and apparently well-annotated organisms. Antibiotic resistance with high sequence identity to known genes were more likely than novel genes to be flanked by mobile genetic elements such as transposases, possibly indicating that the novel genes represent a potential resistance reservoir that has not yet become widely disseminated. Recent work by Goodman et al. (2011) demonstrated that interindividual differences in gastrointestinal antibiotic resistance genes can be detected by subjecting both uncultured fecal samples as well as pools of phylogenetically representative fecal culture collections to metagenomic functional screens. Notably, the presence or absence of specific resistance genes (e.g., those encoding amikacin resistance) in uncultured samples, as determined by functional metagenomics, correlated with the fraction of cultured isolates phenotypically resistant to those compounds, and the presence of the exact genes identified by functional metagenomic screens was reconfirmed by PCR assay in phenotypically resistant cultured strains. These authors also found that the nearest genome-sequenced phylogenetic neighbors of the resistant strains isolated from the the gastrointestinal microbiota of the sampled individuals lacked similar resistance genes, further highlighting the diversity and individualized nature of antibiotic resistomes. Together, these studies indicate that the gastrointestinal microbiota are likely to harbor many more resistance genes that will continue to be revealed by further investigation.
Functional metagenomic screens have also been used to mine the resistance reservoir in the intestinal microbiota of farm animals. Livestock are frequently dosed with antibiotics to treat infections and promote growth, and mounting evidence indicates that these practices lead to increased antibiotic resistance not only in the microbiota of the treated animals but also in their human caregivers (Sorum et al., 2006). The scope of this problem is highlighted by the findings of Kazimierczak et al. (2009), who employed metagenomic functional screens to identify both known and novel tetracycline resistance genes in fecal samples from organically farmed pigs that had not been exposed to antibiotics. Most of these genes were associated with mobile genetic elements, possibly explaining their persistence in an environment without any obvious selection pressure. The clinical and epidemiologic relevance of resistance genes present in the intestinal microbiota must be further defined by examining secondary effects of these genes, such as fitness costs or benefits associated with their expression, as well as by demonstrating the potential for direct transfer of the resistance gene to pathogenic organisms.
As previously noted, specific variations in the composition of the gastrointestinal microbial community have been linked to important states of human health and disease. Recent advances in understanding the interactions between bacterial metabolites and the host cellular machinery have begun to illuminate the physiologic basis of microbial contributions to human pathology. Metabolites generated either directly or indirectly by saccharolytic species may provide a mechanistic explanation for the observed human health outcomes associated with different compositions of the enteric microbial community. Conjugated linoleic acids, which are generated by some Bifidobacterial species (Rosberg-Cody et al., 2004), modulate tumorigenesis in animal models (Kelley et al., 2007), and are being investigated for a role in modulating inflammation and risk for neoplasia in humans (Bhattacharya et al., 2006; Coakley et al., 2009). Short-chain fatty acids (SCFAs) are bacterial metabolites that have wide-ranging effects on human physiology. In animal models of prematurity, some SCFAs (acetic and butyric acid) directly injure colonic mucosa in a dose-dependent fashion in the most immature age groups (Lin et al., 2002), an effect that disappears with increasing postnatal age (Nafday et al., 2005). This suggests a possible role for bacterial metabolites in the complex pathogenesis of necrotizing enterocolitis, a necroinflammatory disease commonly seen in preterm infants but non existent in older age groups. Butyrate, a SCFA that is produced by fermentation of dietary fiber, has a variety of effects modulating inflammation and risk for neoplasia (Hamer et al., 2008). Butyrate is taken up by colonocytes via the MCT1 and SMCT1 transporters (downregulated in cancer cells), and is protective against colon cancer in animal models. It also inhibits histone deacetylase and inhibits TNF-κB activation, which may explain its role in modulating inflammation. Acetate and propionate, two other SCFAs, have opposing effects on cholesterol biosynthesis (Wong et al., 2006). Microbe-generated SCFAs also may contribute to host obesity via interaction with fasting-induced adipocyte factor (Fiaf), AMPK, and Gpr41, which modulate pathways regulating fatty acid storage in adipocytes, fatty acid oxidation, gastrointestinal motility, and nutrient absorption (Backhed et al., 2007; Samuel et al., 2008).
Functional metagenomic screens offer a powerful means for detecting the genetic determinants of microbial metabolism. Jones et al. (2008) employed a functional screen using a large-insert metagenomic library to identify bile salt hydrolases within the human gastrointestinal microbiota. End-sequencing of clones displaying bile salt hydrolase activity revealed a broad phylogenetic distribution of bile salt hydrolase enzymes suggesting that this metabolic capacity is a conserved trait among bacteria adapted to life in the human gastrointestinal tract. Since bile salts play important roles in the processing and uptake of dietary fats in the intestines, microbial catabolism of these compounds may affect the amount of energy extracted from the diet.
Catabolism of fibers indigestible by the human host, another significant activity of the human intestinal microbiota, has been investigated using successive rounds of functional screens to enrich the metagenomic library with carbohydrate-metabolizing enzymes followed by high-throughput sequencing to identify genetic determinants of carbohydrate metabolism within the human gastrointestinal microbiota (Tasse et al., 2010). They identified 73 carbohydrate-metabolizing enzymes from the enriched library, representing a fivefold increase in active genes identified compared to metagenomic sequencing without enrichment. This highlights the strong potential of serial functional screens combined with high-throughput sequencing to identify novel genes and yield increasingly comprehensive information on the metabolic potential of a given microbial community.
The advent of convenient applications for metagenomic data analysis such as MG-RAST and MEGAN have simplified annotation and comparative analysis of functionally selected genes, which together with the declining cost of high-throughput sequencing, offer an efficient complement to functional screens (Huson et al., 2007; Meyer et al., 2008). Several studies have used this approach to connect functional genes with metabolic capacities and to identify pathways, such as metabolism of sugars, amino acids, and nucleotides, that are enriched in the gastrointestinal microbiota relative to representative genome-sequenced strains (Gill et al., 2006; Kurokawa et al., 2007; Turnbaugh et al., 2009; Arumugam et al., 2011). Moreover, by ranking functional gene clusters according to their frequencies, a minimal gut genome and a minimal gut metagenome have been described (Qin et al., 2010). The former reflects the minimal set of genes required by a single member of the gastrointestinal microbiota, while the latter indicates the minimal set of genes required to sustain the aggregate gastrointestinal microbiota. The minimal gut genome includes genes essential to all bacteria (e.g., replication, transcription, translation) as well as gut-specific genes such as those facilitating adhesion to epithelium. In contrast, the minimal gut metagenome includes genes necessary for metabolism of complex sugars, underscoring the importance of coupled metabolism in sustaining the GI tract microbiota. The importance of confirming gene function in vitro and in vivo to ensure reliable annotation is illustrated by Hess et al. (2011), who used metagenomic sequencing to identify >20,000 carbohydrate active genes from the cow rumen microbiota. From this gene set, they selected 90 in silico predicted carbohydrate-metabolizing genes, expressed them, subjected them to functional assays, and found that 51 genes were enzymatically active in vitro (Hess et al., 2011). These studies exemplify how metagenomic sequencing, automated annotation of large data sets, and functional screening comprise a powerful toolkit capable of characterizing functional networks in highly complex environments such as the GI tract microbiota.
Functional metagenomic screens may also illuminate the genetic determinants of microbial interactions with host cells. The intestinal microbiota have long been known to modulate intestinal epithelia, for instance, by stimulating intestinal cell differentiation (Bry et al., 1996). In order to identify specific bacterial gene products that directly influence the fate of human cells, Gloux et al. (2007) used cell lysate from individual clones in a gastrointestinal metagenomic library to screen for modulation of cell growth in CV-1 kidney fibroblast and HT-29 human colonic tumor cells. Using this approach, they identified 30 growth-stimulating and 20 growth-inhibiting clones, with Bacteroidetes as the dominant phylum among both sets. Using transposon mutagenesis on these sets of clones, they identified seven candidate genes with putative growth modulation effects.
Functional metagenomic screens have also been designed to investigate the immune-modulatory capacity of the gastrointestinal microbiota. To identify clones modifying the host immune response, Lakhdari et al. (2010) constructed an NF-κB activated reporter system from a human colorectal carcinoma cell line. By screening metagenomic libraries of GI tract microbiota from patients with Crohn’s disease, in which NF-κB activity is frequently elevated (Ellis et al., 1998), they identified several clones either inducing or inhibiting NF-κB activity. Together, these studies demonstrate the potential for functional metagenomic screens to illuminate the genetic mechanisms for microbial community contribution to the development of the human immune system and the pathogenesis of atopic, autoimmune, and neoplastic disease, which may provide novel therapeutic targets for these conditions.
In addition to interacting with human cells, commensal bacteria can also use quorum-sensing to convey signals over distances and thereby coordinate community gene expression. Guan et al. (2007) used a metabolite regulated expression(METREX) screen based on a quorum-sensing inducible promoter fused to gfp to identify genes encoding a new class of quorum-sensing inducing molecules in moth gut microbiota, demonstrating the power of functional metagenomics for characterizing the determinants of community behavior in uncultured organisms.
Increased understanding of the effects of gastrointestinal microbiota on human health has generated interest in targeting these communities for therapeutic intervention (Cani and Delzenne, 2011). Short-chain carbohydrates that are indigestible by humans but are fermentable by some microbes have demonstrable efficacy in increasing the populations of Lactobacilli and Bifidobacteria in the human gastrointestinal tract (Wang and Gibson, 1993). Investigations of galactose oligosaccharides (GOS) and fructose oligosaccharides (FOS) as additives to infant formula have demonstrated increased Bifidobacterial populations, decreased stool pH, generation of a stool fatty acid profile more similar to that found in breastfed infants, and reduced populations of potential pathogens such as Clostridia spp., Bacteroides spp., and E. coli (Fanaro et al., 2005; Knol et al., 2005; Costalos et al., 2008; Magne et al., 2008; Rao et al., 2009). Prebiotic supplementation with oligosaccharides may promote blooms of beneficial bacteria more effectively than direct administration of pro-biotic organisms: a study directly comparing infant formula containing Bifidobacterium animalis with GOS/FOS-supplemented formula revealed a significantly greater proportion of Bifidobacterial species in the infants fed oligosaccharide-containing formula but no difference between the Bifidobacterial supplemented formula and control formula groups (Bakker-Zierikzee et al., 2005). Administration of prebiotics such as inulin and oligosaccharides in adult humans have shown some effect on hunger and satiety mechanisms (Whelan et al., 2006; Cani et al., 2009) but inconsistent results when applied to pathologies such as atopy and inflammatory bowel disease (Guarner, 2005; Roberfroid et al., 2010). Functional metagenomics has the potential to refine current prebiotic therapies by more completely defining the genetic determinants of metabolism for given constituents of a microbial community, providing a rational basis for more precise design of prebiotic agents intended to promote blooming of a specific subset of organisms.
Functional metagenomic screens have been successful in elucidating novel genes encoding microbial antibiotic resistance, metabolic machinery, and immune-modulatory elements. Despite their demonstrable utility, functional metagenomic screens have several important limitations. First, the DNA insert must be compatible with the host’s expression machinery and the gene product must be non-toxic and functional in the host (for an in-depth review, see Uchiyama and Miyazaki, 2009). Second, the host must be suited for the screen: when screening for antibiotic resistance genes, a host sensitive to the antibiotic of interest must be chosen. Third, the insert size may restrict the diversity of functions portrayed in a screen; a small insert library cannot reveal the function of genes organized in large operons such as many metabolic pathways or some efflux pumps associated with antibiotic resistance. Finally, the expression level of the insert can significantly affect the result of a functional screen. Using a high-copy plasmid as vector or a strong synthetic promoter can result in an overestimation of functionality. Conversely, overexpression of potentially lethal proteins may cause underestimation of functional genes, (e.g., cell lysis due to overexpression of efflux pumps). Despite these limitations, multiple studies demonstrate the potential for functional metagenomic screens to powerfully complement direct culture, 16S sequencing, shotgun metagenomic sequencing, and metabolomic analysis to offer new insight into the complex interactions between microbial communities and their human hosts. Used in concert, these techniques promise to expand our understanding of microbial community function, its impact on human health, and to provide novel targets for therapeutic development in the coming years.
The authors declare that the research was conducted in the absence of any commercial or financial relationships that could be construed as a potential conflict of interest.
Allen, H. K., Cloud-Hansen, K. A., Wolinski, J. M., Guan, C., Greene, S., Lu, S., Boeyink, M., Broderick, N. A., Raffa, K. F., and Handelsman, J. (2009a). Resident microbiota of the gypsy moth midgut harbors antibiotic resistance determinants. DNA Cell Biol. 28, 109–117.
Allen, H. K., Moe, L. A., Rodbumrer, J., Gaarder, A., and Handelsman, J. (2009b). Functional metagenomics reveals diverse beta-lactamases in a remote Alaskan soil. ISME J. 3, 243–251.
Allen, H. K., Donato, J., Wang, H. H., Cloud-Hansen, K. A., Davies, J., and Handelsman, J. (2010). Call of the wild: antibiotic resistance genes in natural environments. Nat. Rev. Microbiol. 8, 251–259.
Aminov, R. I. (2009). The role of antibiotics and antibiotic resistance in nature. Environ. Microbiol. 11, 2970–2988.
Aminov, R. I., and Mackie, R. I. (2007). Evolution and ecology of antibiotic resistance genes. FEMS Microbiol. Lett. 271, 147–161.
Arumugam, M., Raes, J., Pelletier, E., Le Paslier, D., Yamada, T., Mende, D. R., Fernandes, G. R., Tap, J., Bruls, T., Batto, J.-M., Bertalan, M., Borruel, N., Casellas, F., Fernandez, L., Gautier, L., Hansen, T., Hattori, M., Hayashi, T., Kleerebezem, M., Kurokawa, K., Leclerc, M., Levenez, F., Manichanh, C., Nielsen, H. B., Nielsen, T., Pons, N., Poulain, J., Qin, J., Sicheritz-Ponten, T., Tims, S., Torrents, D., Ugarte, E., Zoetendal, E. G., Wang, J., Guarner, F., Pedersen, O., de Vos, W. M., Brunak, S., Doré, J.; MetaHIT Consortium, Antolín, M., Artiguenave, F., Blottiere, H. M., Almeida, M., Brechot, C., Cara, C., Chervaux, C., Cultrone, A., Delorme, C., Denariaz, G., Dervyn, R., Foerstner, K. U., Friss, C., van de Guchte, M., Guedon, E., Haimet, F., Huber, W., van Hylckama-Vlieg, J., Jamet, A., Juste, C., Kaci, G., Knol, J., Lakhdari, O., Layec, S., Le Roux, K., Maguin, E., Mérieux, A., Melo Minardi, R., M’rini, C., Muller, J., Oozeer, R., Parkhill, J., Renault, P., Rescigno, M., Sanchez, N., Sunagawa, S., Torrejon, A., Turner, K., Vandemeulebrouck, G., Varela, E., Winogradsky, Y., Zeller, G., Weissenbach, J., Ehrlich, S. D., and Bork, P. (2011). Enterotypes of the human gut microbiome. Nature 473, 174–180.
Auerbach, E. A., Seyfried, E. E., and McMahon, K. D. (2007). Tetracycline resistance genes in activated sludge wastewater treatment plants. Water Res. 41, 1143–1151.
Backhed, F., Manchester, J. K., Semenkovich, C. F., and Gordon, J. I. (2007). Mechanisms underlying the resistance to diet-induced obesity in germ-free mice. Proc. Natl. Acad. Sci. U.S.A. 104, 979–984.
Bakker-Zierikzee, A. M., Alles, M. S., Knol, J., Kok, F. J., Tolboom, J. J., and Bindels, J. G. (2005). Effects of infant formula containing a mixture of galacto- and fructo-oligosaccharides or viable Bifidobacterium animalis on the intestinal microflora during the first 4 months of life. Br. J. Nutr. 94, 783–790.
Bartok, C. J., and Ventura, A. K. (2009). Mechanisms underlying the association between breastfeeding and obesity. Int. J. Pediatr. Obes. 4, 196–204.
Berlemont, R., Delsaute, M., Pipers, D., D’amico, S., Feller, G., Galleni, M., and Power, P. (2009). Insights into bacterial cellulose biosynthesis by functional metagenomics on Antarctic soil samples. ISME J. 3, 1070–1081.
Bezabeh, T., Somorjai, R. L., and Smith, I. C. (2009). MR metabolomics of fecal extracts: applications in the study of bowel diseases. Magn. Reson. Chem. 47(Suppl. 1), S54–S61.
Bhattacharya, A., Banu, J., Rahman, M., Causey, J., and Fernandes, G. (2006). Biological effects of conjugated linoleic acids in health and disease. J. Nutr. Biochem. 17, 789–810.
Boucher, H. W., Talbot, G. H., Bradley, J. S., Edwards, J. E., Gilbert, D., Rice, L. B., Scheld, M., Spellberg, B., and Bartlett, J. (2009). Bad bugs, no drugs: no ESKAPE! An update from the Infectious Diseases Society of America. Clin. Infect. Dis. 48, 1–12.
Bry, L., Falk, P. G., Midtvedt, T., and Gordon, J. I. (1996). A model of host-microbial interactions in an open mammalian ecosystem. Science 273, 1380–1383.
Bullen, C. L., Tearle, P. V., and Willis, A. T. (1976). Bifidobacteria in the intestinal tract of infants: an in-vivo study. J. Med. Microbiol. 9, 325–333.
Cani, P. D., and Delzenne, N. M. (2011). The gut microbiome as therapeutic target. Pharmacol. Ther. 130, 202–212.
Cani, P. D., Lecourt, E., Dewulf, E. M., Sohet, F. M., Pachikian, B. D., Naslain, D., De Backer, F., Neyrinck, A. M., and Delzenne, N. M. (2009). Gut microbiota fermentation of prebiotics increases satietogenic and incretin gut peptide production with consequences for appetite sensation and glucose response after a meal. Am. J. Clin. Nutr. 90, 1236–1243.
Chen, J., Michel, F. C. Jr., Sreevatsan, S., Morrison, M., and Yu, Z. (2010). Occurrence and persistence of erythromycin resistance genes (erm) and tetracycline resistance genes (tet) in waste treatment systems on swine farms. Microb. Ecol. 60, 479–486.
Coakley, M., Banni, S., Johnson, M. C., Mills, S., Devery, R., Fitzgerald, G., Paul Ross, R., and Stanton, C. (2009). Inhibitory effect of conjugated alpha-linolenic acid from Bifidobacteria of intestinal origin on SW480 cancer cells. Lipids 44, 249–256.
Collado, M. C., Isolauri, E., Laitinen, K., and Salminen, S. (2010). Effect of mother’s weight on infant’s microbiota acquisition, composition, and activity during early infancy: a prospective follow-up study initiated in early pregnancy. Am. J. Clin. Nutr. 92, 1023–1030.
Costalos, C., Kapiki, A., Apostolou, M., and Papathoma, E. (2008). The effect of a prebiotic supplemented formula on growth and stool microbiology of term infants. Early Hum. Dev. 84, 45–49.
Diaz-Torres, M. L., McNab, R., Spratt, D. A., Villedieu, A., Hunt, N., Wilson, M., and Mullany, P. (2003). Novel tetracycline resistance determinant from the oral metagenome. Antimicrob. Agents Chemother. 47, 1430–1432.
Duijts, L., Ramadhani, M. K., and Moll, H. A. (2009). Breastfeeding protects against infectious diseases during infancy in industrialized countries. A systematic review. Matern. Child Nutr. 5, 199–210.
Eckburg, P. B., Bik, E. M., Bernstein, C. N., Purdom, E., Dethlefsen, L., Sargent, M., Gill, S. R., Nelson, K. E., and Relman, D. A. (2005). Diversity of the human intestinal microbial flora. Science 308, 1635–1638.
Ellis, R. D., Goodlad, J. R., Limb, G. A., Powell, J. J., Thompson, R. P., and Punchard, N. A. (1998). Activation of nuclear factor kappa B in Crohn’s disease. Inflamm. Res. 47, 440–445.
Erridge, C., Attina, T., Spickett, C. M., and Webb, D. J. (2007). A high-fat meal induces low-grade endotoxemia: evidence of a novel mechanism of postprandial inflammation. Am. J. Clin. Nutr. 86, 1286–1292.
Fanaro, S., Boehm, G., Garssen, J., Knol, J., Mosca, F., Stahl, B., and Vigi, V. (2005). Galacto-oligosaccharides and long-chain fructo-oligosaccharides as prebiotics in infant formulas: a review. Acta Paediatr. Suppl. 94, 22–26.
Ghanim, H., Abuaysheh, S., Sia, C. L., Korzeniewski, K., Chaudhuri, A., Fernandez-Real, J. M., and Dandona, P. (2009). Increase in plasma endotoxin concentrations and the expression of Toll-like receptors and suppressor of cytokine signaling-3 in mononuclear cells after a high-fat, high-carbohydrate meal: implications for insulin resistance. Diabetes Care 32, 2281–2287.
Gill, S. R., Pop, M., Deboy, R. T., Eckburg, P. B., Turnbaugh, P. J., Samuel, B. S., Gordon, J. I., Relman, D. A., Fraser-Liggett, C. M., and Nelson, K. E. (2006). Metagenomic analysis of the human distal gut microbiome. Science 312, 1355–1359.
Gillevet, P., Sikaroodi, M., Keshavarzian, A., and Mutlu, E. A. (2010). Quantitative assessment of the human gut microbiome using multitagpyrosequencing. Chem. Biodivers. 7, 1065–1075.
Gloux, K., Leclerc, M., Iliozer, H., L’Haridon, R., Manichanh, C., Corthier, G., Nalin, R., Blottiere, H. M., and Dore, J. (2007). Development of high-throughput phenotyping of metagenomic clones from the human gut microbiome for modulation of eukaryotic cell growth. Appl. Environ. Microbiol. 73, 3734–3737.
Goodman, A. L., Kallstrom, G., Faith, J. J., Reyes, A., Moore, A., Dantas, G., and Gordon, J. I. (2011). Extensive personal human gut microbiota culture collections characterized and manipulated in gnotobiotic mice. Proc. Natl. Acad. Sci. U.S.A. 108, 6252–6257.
Gouveri, E., Papanas, N., Hatzitolios, A. I., and Maltezos, E. (2011). Breastfeeding and diabetes. Curr. Diabetes Rev. 7, 135–142.
Guan, C., Ju, J., Borlee, B. R., Williamson, L. L., Shen, B., Raffa, K. F., and Handelsman, J. (2007). Signal mimics derived from a metagenomic analysis of the gypsy moth gut microbiota. Appl. Environ. Microbiol. 73, 3669–3676.
Guarner, F. (2005). Inulin and oligofructose: impact on intestinal diseases and disorders. Br. J. Nutr. 93(Suppl. 1), S61–S65.
Gueimonde, M., Salminen, S., and Isolauri, E. (2006). Presence of specific antibiotic (tet) resistance genes in infant faecal microbiota. FEMS Immunol. Med. Microbiol. 48, 21–25.
Hamer, H. M., Jonkers, D., Venema, K., Vanhoutvin, S., Troost, F. J., and Brummer, R. J. (2008). Review article: the role of butyrate on colonic function. Aliment. Pharmacol. Ther. 27, 104–119.
Handelsman, J., Rondon, M. R., Brady, S. F., Clardy, J., and Goodman, R. M. (1998). Molecular biological access to the chemistry of unknown soil microbes: a new frontier for natural products. Chem. Biol. 5, R245–R249.
Harmsen, H. J., Wildeboer-Veloo, A. C., Raangs, G. C., Wagendorp, A. A., Klijn, N., Bindels, J. G., and Welling, G. W. (2000). Analysis of intestinal flora development in breast-fed and formula-fed infants by using molecular identification and detection methods. J. Pediatr. Gastroenterol. Nutr. 30, 61–67.
Hess, M., Sczyrba, A., Egan, R., Kim, T. W., Chokhawala, H., Schroth, G., Luo, S., Clark, D. S., Chen, F., Zhang, T., Mackie, R. I., Pennacchio, L. A., Tringe, S. G., Visel, A., Woyke, T., Wang, Z., and Rubin, E. M. (2011). Metagenomic discovery of biomass-degrading genes and genomes from cow rumen. Science 331, 463–467.
Högberg, L. D., Heddini, A., and Cars, O. (2010). The global need for effective antibiotics: challenges and recent advances. Trends Pharmacol. Sci. 31, 509–515.
Huson, D. H., Auch, A. F., Qi, J., and Schuster, S. C. (2007). MEGAN analysis of metagenomic data. Genome Res. 17, 377–386. doi: 10.1371/journal.pone.0006386
Jansson, J., Willing, B., Lucio, M., Fekete, A., Dicksved, J., Halfvarson, J., Tysk, C., and Schmitt-Kopplin, P. (2009). Metabolomics reveals metabolic biomarkers of Crohn’s disease. PLoS ONE 4, e6386.
Jones, B. V., Begley, M., Hill, C., Gahan, C. G., and Marchesi, J. R. (2008). Functional and comparative metagenomic analysis of bile salt hydrolase activity in the human gut microbiome. Proc. Natl. Acad. Sci. U.S.A. 105, 13580–13585.
Kalliomaki, M., Collado, M. C., Salminen, S., and Isolauri, E. (2008). Early differences in fecal microbiota composition in children may predict overweight. Am. J. Clin. Nutr. 87, 534–538.
Kang, S., Denman, S. E., Morrison, M., Yu, Z., Dore, J., Leclerc, M., and Mcsweeney, C. S. (2010). Dysbiosis of fecal microbiota in Crohn’s disease patients as revealed by a custom phylogenetic microarray. Inflamm. Bowel Dis. 16, 2034–2042.
Kazimierczak, K. A., Scott, K. P., Kelly, D., and Aminov, R. I. (2009). Tetracycline resistome of the organic pig gut. Appl. Environ. Microbiol. 75, 1717–1722.
Kelley, N. S., Hubbard, N. E., and Erickson, K. L. (2007). Conjugated linoleic acid isomers and cancer. J. Nutr. 137, 2599–2607.
Knol, J., Scholtens, P., Kafka, C., Steenbakkers, J., Gro, S., Helm, K., Klarczyk, M., Schopfer, H., Bockler, H. M., and Wells, J. (2005). Colon microflora in infants fed formula with galacto- and fructo-oligosaccharides: more like breast-fed infants. J. Pediatr. Gastroenterol. Nutr. 40, 36–42.
Koenig, J. E., Spor, A., Scalfone, N., Fricker, A. D., Stombaugh, J., Knight, R., Angenent, L. T., and Ley, R. E. (2011). Succession of microbial consortia in the developing infant gut microbiome. Proc. Natl. Acad. Sci. U.S.A. 108, 4578–4585.
Kurokawa, K., Itoh, T., Kuwahara, T., Oshima, K., Toh, H., Toyoda, A., Takami, H., Morita, H., Sharma, V. K., Srivastava, T. P., Taylor, T. D., Noguchi, H., Mori, H., Ogura, Y., Ehrlich, D. S., Itoh, K., Takagi, T., Sakaki, Y., Hayashi, T., and Hattori, M. (2007). Comparative metagenomics revealed commonly enriched gene sets in human gut microbiomes. DNA Res. 14, 169–181.
Lakhdari, O., Cultrone, A., Tap, J., Gloux, K., Bernard, F., Ehrlich, S. D., Lefevre, F., Dore, J., and Blottiere, H. M. (2010). Functional metagenomics: a high throughput screening method to decipher microbiota-driven NF-kappaB modulation in the human gut. PLoS ONE 5, e13092. doi: 10.1371/journal.pone.0013092
Langendijk, P. S., Schut, F., Jansen, G. J., Raangs, G. C., Kamphuis, G. R., Wilkinson, M. H., and Welling, G. W. (1995). Quantitative fluorescence in situ hybridization of Bifidobacterium spp. with genus-specific 16S rRNA-targeted probes and its application in fecal samples. Appl. Environ. Microbiol. 61, 3069–3075.
Le Huerou-Luron, I., Blat, S., and Boudry, G. (2010). Breast-v. formula-feeding: impacts on the digestive tract and immediate and long-term health effects. Nutr. Res. Rev. 23, 23–36.
Lin, J., Nafday, S. M., Chauvin, S. N., Magid, M. S., Pabbatireddy, S., Holzman, I. R., and Babyatsky, M. W. (2002). Variable effects of short chain fatty acids and lactic acid in inducing intestinal mucosal injury in newborn rats. J. Pediatr. Gastroenterol. Nutr. 35, 545–550.
Magne, F., Hachelaf, W., Suau, A., Boudraa, G., Bouziane-Nedjadi, K., Rigottier-Gois, L., Touhami, M., Desjeux, J. F., and Pochart, P. (2008). Effects on faecal microbiota of dietary and acidic oligosaccharides in children during partial formula feeding. J. Pediatr. Gastroenterol. Nutr. 46, 580–588.
Maragakis, L. L., Perencevich, E. N., and Cosgrove, S. E. (2008). Clinical and economic burden of antimicrobial resistance. Expert Rev. Anti. Infect. Ther. 6, 751–763.
Martinez, J. L. (2008). Antibiotics and antibiotic resistance genes in natural environments. Science 321, 365–367.
Meyer, F., Paarmann, D., D’Souza, M., Olson, R., Glass, E. M., Kubal, M., Paczian, T., Rodriguez, A., Stevens, R., Wilke, A., Wilkening, J., and Edwards, R. A. (2008). The metagenomics RAST server – a public resource for the automatic phylogenetic and functional analysis of metagenomes. BMC Bioinformatics 9, 386. doi: 10.1186/1471-2105-9-386
Nafday, S. M., Chen, W., Peng, L., Babyatsky, M. W., Holzman, I. R., and Lin, J. (2005). Short-chain fatty acids induce colonic mucosal injury in rats with various postnatal ages. Pediatr. Res. 57, 201–204.
Oddy, W. H. (2004). A review of the effects of breastfeeding on respiratory infections, atopy, and childhood asthma. J. Asthma 41, 605–621.
Paliy, O., Kenche, H., Abernathy, F., and Michael, S. (2009). High-throughput quantitative analysis of the human intestinal microbiota with a phylogenetic microarray. Appl. Environ. Microbiol. 75, 3572–3579.
Penders, J., Thijs, C., van den Brandt, P. A., Kummeling, I., Snijders, B., Stelma, F., Adams, H., van Ree, R., and Stobberingh, E. E. (2007). Gut microbiota composition and development of atopic manifestations in infancy: the KOALA Birth Cohort Study. Gut 56, 661–667.
Qin, J., Li, R., Raes, J., Arumugam, M., Burgdorf, K. S., Manichanh, C., Nielsen, T., Pons, N., Levenez, F., Yamada, T., Mende, D. R., Li, J., Xu, J., Li, S., Li, D., Cao, J., Wang, B., Liang, H., Zheng, H., Xie, Y., Tap, J., Lepage, P., Bertalan, M., Batto, J. M., Hansen, T., Le Paslier, D., Linneberg, A., Nielsen, H. B., Pelletier, E., Renault, P., Sicheritz-Ponten, T., Turner, K., Zhu, H., Yu, C., Li, S., Jian, M., Zhou, Y., Li, Y., Zhang, X., Li, S., Qin, N., Yang, H., Wang, J., Brunak, S., Doré, J., Guarner, F., Kristiansen, K., Pedersen, O., Parkhill, J., Weissenbach, J.; MetaHIT Consortium., Bork, P., Ehrlich, S. D., and Wang, J. (2010). A human gut microbial gene catalogue established by metagenomic sequencing. Nature 464, 59–65.
Qin, X., Razia, Y., Johnson, J. R., Stapp, J. R., Boster, D. R., Tsosie, T., Smith, D. L., Braden, C. R., Gay, K., Angulo, F. J., and Tarr, P. I. (2006). Ciprofloxacin-resistant gram-negative bacilli in the fecal microflora of children. Antimicrob. Agents Chemother. 50, 3325–3329.
Rao, S., Srinivasjois, R., and Patole, S. (2009). Prebiotic supplementation in full-term neonates: a systematic review of randomized controlled trials. Arch. Pediatr. Adolesc. Med. 163, 755–764.
Riesenfeld, C. S., Goodman, R. M., and Handelsman, J. (2004). Uncultured soil bacteria are a reservoir of new antibiotic resistance genes. Environ. Microbiol. 6, 981–989.
Rigsbee, L., Agans, R., Foy, B. D., and Paliy, O. (2011). Optimizing the analysis of human intestinal microbiota with phylogenetic microarray. FEMS Microbiol. Ecol. 75, 332–342.
Rimbara, E., Noguchi, N., Yamaguchi, T., Narui, K., Kawai, T., and Sasatsu, M. (2005). Development of a highly sensitive method for detection of clarithromycin-resistant Helicobacter pylori from human feces. Curr. Microbiol. 51, 1–5.
Roberfroid, M., Gibson, G. R., Hoyles, L., McCartney, A. L., Rastall, R., Rowland, I., Wolvers, D., Watzl, B., Szajewska, H., Stahl, B., Guarner, F., Respondek, F., Whelan, K., Coxam, V., Davicco, M. J., Léotoing, L., Wittrant, Y., Delzenne, N. M., Cani, P. D., Neyrinck, A. M., and Meheust, A. (2010). Prebiotic effects: metabolic and health benefits. Br. J. Nutr. 104(Suppl. 2), S1–S63.
Rondon, M. R., August, P. R., Bettermann, A. D., Brady, S. F., Grossman, T. H., Liles, M. R., Loiacono, K. A., Lynch, B. A., MacNeil, I. A., Minor, C., Tiong, C. L., Gilman, M., Osburne, M. S., Clardy, J., Handelsman, J., and Goodman, R. M. (2000). Cloning the soil metagenome: a strategy for accessing the genetic and functional diversity of uncultured microorganisms. Appl. Environ. Microbiol. 66, 2541–2547.
Rosberg-Cody, E., Ross, R. P., Hussey, S., Ryan, C. A., Murphy, B. P., Fitzgerald, G. F., Devery, R., and Stanton, C. (2004). Mining the microbiota of the neonatal gastrointestinal tract for conjugated linoleic acid-producing Bifidobacteria. Appl. Environ. Microbiol. 70, 4635–4641.
Round, J. L., and Mazmanian, S. K. (2009). The gut microbiota shapes intestinal immune responses during health and disease. Nat. Rev. Immunol. 9, 313–323.
Ruan, X., Shi, H., Xia, G., Xiao, Y., Dong, J., Ming, F., and Wang, S. (2007). Encapsulated Bifidobacteria reduced bacterial translocation in rats following hemorrhagic shock and resuscitation. Nutrition 23, 754–761.
Rubaltelli, F. F., Biadaioli, R., Pecile, P., and Nicoletti, P. (1998). Intestinal flora in breast- and bottle-fed infants. J. Perinat. Med. 26, 186–191.
Samuel, B. S., Shaito, A., Motoike, T., Rey, F. E., Backhed, F., Manchester, J. K., Hammer, R. E., Williams, S. C., Crowley, J., Yanagisawa, M., and Gordon, J. I. (2008). Effects of the gut microbiota on host adiposity are modulated by the short-chain fatty-acid binding G protein-coupled receptor, Gpr41. Proc. Natl. Acad. Sci. U.S.A. 105, 16767–16772.
Smith, M. S., Yang, R. K., Knapp, C. W., Niu, Y., Peak, N., Hanfelt, M. M., Galland, J. C., and Graham, D. W. (2004). Quantification of tetracycline resistance genes in feedlot lagoons by real-time PCR. Appl. Environ. Microbiol. 70, 7372–7377.
Sommer, M. O., Dantas, G., and Church, G. M. (2009). Functional characterization of the antibiotic resistance reservoir in the human microflora. Science 325, 1128–1131.
Sorum, M., Johnsen, P. J., Aasnes, B., Rosvoll, T., Kruse, H., Sundsfjord, A., and Simonsen, G. S. (2006). Prevalence, persistence, and molecular characterization of glycopeptide-resistant enterococci in Norwegian poultry and poultry farmers 3 to 8 years after the ban on avoparcin. Appl. Environ. Microbiol. 72, 516–521.
Tasse, L., Bercovici, J., Pizzut-Serin, S., Robe, P., Tap, J., Klopp, C., Cantarel, B. L., Coutinho, P. M., Henrissat, B., Leclerc, M., Doré, J., Monsan, P., Remaud-Simeon, M., and Potocki-Veronese, G. (2010). Functional metagenomics to mine the human gut microbiome for dietary fiber catabolic enzymes. Genome Res. 20, 1605–1612.
Torres-Cortes, G., Millan, V., Ramirez-Saad, H. C., Nisa-Martinez, R., Toro, N., and Martinez-Abarca, F. (2011). Characterization of novel antibiotic resistance genes identified by functional metagenomics on soil samples. Environ. Microbiol. 13, 1101–1114.
Turnbaugh, P. J., Hamady, M., Yatsunenko, T., Cantarel, B. L., Duncan, A., Ley, R. E., Sogin, M. L., Jones, W. J., Roe, B. A., Affourtit, J. P., Egholm, M., Henrissat, B., Heath, A. C., Knight, R., and Gordon, J. I. (2009). A core gut microbiome in obese and lean twins. Nature 457, 480–484.
Turnbaugh, P. J., Ley, R. E., Mahowald, M. A., Magrini, V., Mardis, E. R., and Gordon, J. I. (2006). An obesity-associated gut microbiome with increased capacity for energy harvest. Nature 444, 1027–1031.
Uchiyama, T., and Miyazaki, K. (2009). Functional metagenomics for enzyme discovery: challenges to efficient screening. Curr. Opin. Biotechnol. 20, 616–622.
Vael, C., and Desager, K. (2009). The importance of the development of the intestinal microbiota in infancy. Curr. Opin. Pediatr. 21, 794–800.
van der Waaij, D., and Nord, C. E. (2000). Development and persistence of multi-resistance to antibiotics in bacteria; an analysis and a new approach to this urgent problem. Int. J. Antimicrob. Agents 16, 191–197.
Wang, X., and Gibson, G. R. (1993). Effects of the in vitro fermentation of oligofructose and inulin by bacteria growing in the human large intestine. J. Appl. Bacteriol. 75, 373–380.
Wang, Z., Xiao, G., Yao, Y., Guo, S., Lu, K., and Sheng, Z. (2006). The role of Bifidobacteria in gut barrier function after thermal injury in rats. J. Trauma 61, 650–657.
Warnecke, F., Luginbuhl, P., Ivanova, N., Ghassemian, M., Richardson, T. H., Stege, J. T., Cayouette, M., Mchardy, A. C., Djordjevic, G., Aboushadi, N., Sorek, R., Tringe, S. G., Podar, M., Martin, H. G., Kunin, V., Dalevi, D., Madejska, J., Kirton, E., Platt, D., Szeto, E., Salamov, A., Barry, K., Mikhailova, N., Kyrpides, N. C., Matson, E. G., Ottesen, E. A., Zhang, X., Hernandez, M., Murillo, C., Acosta, L. G., Rigoutsos, I., Tamayo, G., Green, B. D., Chang, C., Rubin, E. M., Mathur, E. J., Robertson, D. E., Hugenholtz, P., and Leadbetter, J. R. (2007). Metagenomic and functional analysis of hindgut microbiota of a wood-feeding higher termite. Nature 450, 560–565.
Whelan, K., Efthymiou, L., Judd, P. A., Preedy, V. R., and Taylor, M. A. (2006). Appetite during consumption of enteral formula as a sole source of nutrition: the effect of supplementing pea-fibre and fructo-oligosaccharides. Br. J. Nutr. 96, 350–356.
Wong, J. M., de Souza, R., Kendall, C. W., Emam, A., and Jenkins, D. J. (2006). Colonic health: fermentation and short chain fatty acids. J. Clin. Gastroenterol. 40, 235–243.
Keywords: functional metagenomics, human intestinal microbiota, antibiotic resistome
Citation: Moore AM, Munck C, Sommer MOA and Dantas G (2011) Functional metagenomic investigations of the human intestinal microbiota. Front. Microbio. 2:188. doi: 10.3389/fmicb.2011.00188
Received: 01 April 2011;
Paper pending published: 31 May 2011;
Accepted: 23 August 2011;
Published online: 17 October 2011.
Edited by:
Peter J. Turnbaugh, Harvard University, USAReviewed by:
Alain Stintzi, Ottawa Institute of Systems Biology, CanadaCopyright: © 2011 Moore, Munck, Sommer and Dantas. This is an open-access article subject to a non-exclusive license between the authors and Frontiers Media SA, which permits use, distribution and reproduction in other forums, provided the original authors and source are credited and other Frontiers conditions are complied with.
*Correspondence: Gautam Dantas, Department of Pathology and Immunology, Center for Genome Science and Systems Biology, Washington University School of Medicine, 4444 Forest Park Avenue, Room 6215, Campus Box 8510, St. Louis, MO63108, USA. e-mail:ZGFudGFzQHd1c3RsLmVkdQ==; Morten O.A. Sommer, Department of Systems Biology, Technical University of Denmark, Matematik Torvet Building 301, DK-2800 Lyngby, Denmark. e-mail:bXNvbUBiaW8uZHR1LmRr
Disclaimer: All claims expressed in this article are solely those of the authors and do not necessarily represent those of their affiliated organizations, or those of the publisher, the editors and the reviewers. Any product that may be evaluated in this article or claim that may be made by its manufacturer is not guaranteed or endorsed by the publisher.
Research integrity at Frontiers
Learn more about the work of our research integrity team to safeguard the quality of each article we publish.