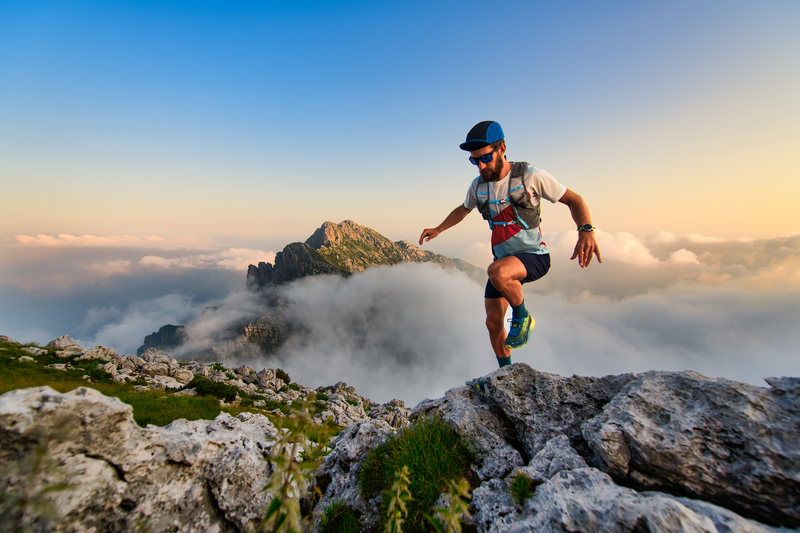
94% of researchers rate our articles as excellent or good
Learn more about the work of our research integrity team to safeguard the quality of each article we publish.
Find out more
REVIEW article
Front. Microbiol. , 02 June 2011
Sec. Cellular and Infection Microbiology - closed section
volume 2 - 2011 | https://doi.org/10.3389/fmicb.2011.00121
This article is part of the Research Topic Salmonella host-pathogen interactions View all 10 articles
Salmonella is a primary cause of enteric diseases in a variety of animals. During its evolution into a pathogenic bacterium, Salmonella acquired an elaborate regulatory network that responds to multiple environmental stimuli within host animals and integrates them resulting in fine regulation of the virulence program. The coordinated action by this regulatory network involves numerous virulence regulators, necessitating genome-wide profiling analysis to assess and combine efforts from multiple regulons. In this review we discuss recent high-throughput analytic approaches used to understand the regulatory network of Salmonella that controls virulence processes. Application of high-throughput analyses have generated large amounts of data and necessitated the development of computational approaches for data integration. Therefore, we also cover computer-aided network analyses to infer regulatory networks, and demonstrate how genome-scale data can be used to construct regulatory and metabolic systems models of Salmonella pathogenesis. Genes that are coordinately controlled by multiple virulence regulators under infectious conditions are more likely to be important for pathogenesis. Thus, reconstructing the global regulatory network during infection or, at the very least, under conditions that mimic the host cellular environment not only provides a bird’s eye view of Salmonella survival strategy in response to hostile host environments but also serves as an efficient means to identify novel virulence factors that are essential for Salmonella to accomplish systemic infection in the host.
Salmonellae are Gram-negative facultative pathogens that live in diverse environments and infect a wide variety of animals. In addition they can use a great variety of compounds as carbon and energy sources. Gastrointestinal infections are the second most common cause of childhood mortality in the developing world and systemic disease typhoid alone (caused by serovar Typhi) is estimated to result in 500,000 deaths per year (Graham, 2002). In addition to fluid and electrolyte loss, non-typhoidal Salmonella often results in septicemia in children and in HIV infected adults in developing countries with a fatality rate of 25% or greater (Graham, 2010). Salmonella diverged from E. coli more than 100 million years ago (Vernikos et al., 2007), but the evolution within the Salmonella genus is more recent. Although there are now only two species of Salmonella; S. enterica and S. bongori, S. enterica has diverged into numerous subspecies and serovars that show a great variation in their infectivity for different cold blooded and warm blooded hosts but share greater than 95% DNA sequence homology. In fact, there are over 2500 serovars for S. enterica with close genetic homology and yet highly diverse regulatory and virulence expression patterns one can assume based on host preference.
Most of the human pathogenic Salmonella serovars, the general subject of virulence regulation in this article, belong to the S. enterica enterica subspecies. Often serovars are written with a capital letter and are not italicized, with only the genus name preceding. Thus Salmonella enterica subspecies enterica serovar Typhimurium is often written as Salmonella Typhimurium and we will use this convention throughout. Salmonella Pullorum and Gallinarum are primarily avian pathogens whereas many serotypes such as Rubislaw, Agona, Infantis, and Panama are found in reptiles and amphibians (Pedersen et al., 2009). S. enterica Typhi and Paratyphi are host restricted to humans, but Typhimurium can infect a variety of animals including cattle. Possibly because of the diversity of potential hosts and environmental conditions it encounters, the regulatory network for virulence in Salmonella is complicated and involves many regulators and a large number of virulence proteins.
One of the earliest genetic-screening approaches to identify the Salmonella genes required for virulence was carried out in the Heffron laboratory by infecting primary peritoneal macrophages with Salmonella transposon mutants (Fields et al., 1986). After incubation the host cells were lysed and plated on selective bacteriological plates to determine if a specific mutant strain was lost. Over 9500 independently derived transposon insertions were screened one by one in elicited peritoneal macrophages. The most important mutation derived in this first screen was located in phoP/phoQ, a two-component regulator, that controls resistance to defensins (Groisman et al., 1989). Many of the genes regulated by phoP/phoQ are required for virulence. Other mutations showed increased sensitivity to oxidizing agents that are found within macrophages. When virulence genes are often present within a contiguous stretch of DNA and are missing in closely related and often non-pathogenic bacteria, it suggests that they were acquired horizontally. These so called pathogenicity islands are about the size that would be carried by a transducing bacteriophage and are often flanked by sequences such as transposons or t-RNA that could permit homologous recombination events. In Salmonella two of these regions important in virulence are called Salmonella pathogenicity island (SPI)-1 and 2.
Both SPI-1 and SPI-2 encode the structural components of their respective type III secretion system (T3SS) apparatus and translocate effectors for distinct purposes. SPI-1-secreted effectors play essential roles for invasion into epithelial cells and promote intestinal inflammation and gastroenteritis. SPI-1, is required for persistent infections in mice (Monack et al., 2004) and for cell invasion (Steele-Mortimer, 2008; McGhie et al., 2009), but is not required following intraperitoneal infection (i.e., systemic infection models) in BALB/c or C57/BL6 mice (rev. in Zhou and Galan, 2001). SPI-2 is induced during intracellular Salmonella infection of a variety of cell types (Geddes et al., 2007) and secretes dozens of distinct effector proteins (Niemann et al., 2011). Mutation of this T3SS does not have a huge effect on intramacrophage survival, however it does result in completely abrogating infection in mice (Buchmeier and Heffron, 1991; Poh et al., 2008). Following internalization into host cells, the SPI-2 T3SS secretes effectors into the cytoplasmic space of epithelial cells or professional phagocytes such as macrophages. SPI-2-secreted effectors prevent maturation of the phagocytic vesicle thereby blocking phago-lysosome fusions and exposure of Salmonella to oxidative and non-oxidative microbicidal mechanisms (Buchmeier and Heffron, 1991; Ramsden et al., 2007; Poh et al., 2008). While most known Salmonella effectors have been found to be secreted specifically by the SPI-2 or SPI-1 T3SS (Steele-Mortimer, 2008; McGhie et al., 2009; Niemann et al., 2011), some have been shown to utilize both the SPI-1 and SPI-2 T3SSs for efficient secretion (Haraga et al., 2008). This complex mixture of secretion processes for Salmonella effectors suggests that there are multiple levels of regulation from transcription to translation to the secretion apparatus each level is critical for the virulence program of these pathogens.
Besides the complex T3SS, Salmonella possesses alternative mechanisms to translocate virulence factors into the extracellular milieu. These include outer membrane vesicles (OMV), the two-partner secretion system ZirT/ZirS, and a type VI secretion system. OMV are observed in a variety of Gram-negative bacteria including pathogenic bacteria and deliver specific periplasmic proteins as well as some outer membrane components to the host cells, promoting proinflammatory responses (Ellis et al., 2010). The ZirT/ZirS system, conserved throughout the Salmonella genus, secretes ZirS via a hydrophilic β-barrel pore formed by ZirT (Gal-Mor et al., 2008). Salmonella lacking this system was hypervirulent, implying a unique function of ZirT/ZirS as an antivirulence modulator during systemic infection. Salmonella also horizontally acquired a type VI secretion system (Bingle et al., 2008). SciS, a component homologous to IcmF in Legionella, appeared to attenuate virulence to prevent acute infections for a long-term dissemination (Parsons and Heffron, 2005). Due to the variety of secretion mechanisms in Salmonella, the virulence program is in many ways surprisingly robust and often challenging to dissect.
Salmonella dwells a variety of environments, thus being able to quickly recognize and respond to the environmental stimuli is essential for its survival. During the course of systemic infection in mice, bacteria are found within neutrophils, monocytes, dendritic cells, and B and T cells but are not found extracellularly until the last 1 or 2 days immediately before death of the host (Dunlap et al., 1994; Yrlid and Wick, 2002; Geddes et al., 2007). The mechanisms of how Salmonella survives and replicates within the host and how it regulates virulence genes at the appropriate time during systemic infection is imperfectly understood and the subject of this work. In fact, Salmonella encodes more than 300 annotated transcriptional and translational regulators that govern expression of Salmonella genes under a myriad of parasitic and free-living conditions (McClelland et al., 2001). By comparison the eukaryote Saccharomyces cerevisiae has nearer 100 (Goffeau et al., 1996).
The fact that Salmonella can successfully survive in these disparate environments even within a single host is likely one reason for the large number of regulators it encodes. Recently, we have deleted all genes annotated as transcriptional or translational regulators in Salmonella and have tested all of them in an acute mouse infection model. There are many regulators that are involved in chemotaxis and cell motility. These have not been examined in detail except for FliA, the flagellar sigma factor (Lockman and Curtiss, 1990). Previous work suggests that with the exception of one or two genes none of the genes required for chemotaxis and motility are necessary for virulence in a BALB/c mouse. In all we have identified 26 regulators that are required for potent virulence in our mouse infection model. This is a surprisingly small number given that 330 were tested and many might have been assumed to result in less fit Salmonella generally. In fact, only in-frame deletions in 20 regulator genes conferred a strong virulence deficient phenotype following intraperitoneal (i.p.) infection (Table 1), while the remaining deletions affected virulence only in intragastric (i.g.) infection or long-term persistence (l.p.) test, likely suggesting the requirement of those regulators for adaptation to the intestinal environment (i.g. attenuated strains) or for evading the adaptive immune system (l.p. attenuated strains). About 90 of the 330 regulators have been tested by i.g. infection and thus there are likely to be several more than the three listed that are unable to effect by this route.
In this review we discuss recent efforts to understand the regulatory network of Salmonella that controls a robust set of virulence processes and mechanisms. We focus here on the application of high-throughput and/or global methodologies to measure virulence and regulatory aspects of Salmonella, the computational approaches used to determine regulatory networks, and how this information can be used to construct systems models/simulations of Salmonella pathogenesis (Figure 1).
Figure 1. Overview of high-throughput and computational methods to elucidation of the regulatory networks governing Salmonella virulence.
In the first part of this review we cover some of the experimental methods that have been employed to determine virulence patterns and regulatory networks in Salmonella, or are currently being used to develop these networks, focusing on high-throughput techniques. We begin with a short description of the types of approaches used to characterize virulence in Salmonella. We then discuss transcriptomic and chromatin immunoprecipitation (ChIP) approaches, proteomic, and protein–protein interactions (PPIs).
Regulatory interactions in Salmonella are studied in three principal ways. The first is based on using in vitro media conditions that simulate infectious and non-infectious conditions to elicit changes that can be studied and perturbed (Beuzon et al., 1999; Coombes et al., 2005; Adkins et al., 2006; Shi et al., 2009a). The second is in infected cultured macrophages (depending on perspective this is referred to as in vivo or ex vivo; Eriksson et al., 2003; Shi et al., 2009b). And the third is using model organisms such as mice or cattle, though this is generally aimed at answering relatively narrow questions of virulence (Chan et al., 2005; Lawley et al., 2006). The choice of experimental system used to investigate regulatory networks is driven by the need for coverage and depth of measurements versus the correctness of the biological environment. That is, in vitro culture conditions, while biologically imperfect, are capable of generating large quantities of samples required for many of the assays described in this section. Advances in methodology are allowing more work to be done ex vivo and in vivo, such as in infected macrophages or by direct measurement within the mouse. These advances include those related to next-generation sequencing for expression profiling, improvements in the sensitivity of proteomics, and ChIP-based methods. As more ex vivo data becomes available it will be possible to compare responses in in vitro conditions with those in macrophages to refine regulatory networks and to refine the culture conditions to better reflect the intracellular milieu.
Changes in gene expression are attributed to the DNA-binding activity of regulators responding to environmental stimuli. Transcriptional regulation is often a complex process composed of multiple regulatory factors and thus systems biology approaches are necessary to integrate the activities of multiple regulators. With the development of the microarray where thousands of sequences are spotted on a chip and the expression of numerous genes is simultaneously compared, the inference of regulatory networks could be accomplished in a high-throughput manner (Faith et al., 2007; Bonneau, 2008). Furthermore, related approaches including ChIP–microarray (ChIP–chip) and ChIP-sequencing (ChIP-seq) methods, discussed below, have accelerated defining the transcriptional regulatory network (Macquarrie et al., 2011). We have used a transcriptomic approach to decipher the regulatory network governed by virulence regulators during Salmonella systemic infection. Regulators sensing the multiple environmental cues execute defense programs and coordinately tune the expression of genes involved in virulence. By deleting Salmonella regulators across the chromosome and its virulence-related plasmid, we defined 20 regulators that were required for Salmonella systemic infection in mice (avirulent strains in i.p. infection in Table 1). These virulence regulators were varied, including two-component regulators (PhoP/PhoQ, SsrA/SsrB, and OmpR/EnvZ), alternative sigma factors (RpoE, RpoS, and FliA), post-transcriptional/post-translational regulators (SmpB, CsrA, Hfq, and RseA), a response regulator for which the signal sensor is unknown (Hnr), a bending protein essential for some types of recombination (IHF), and an assortment of other transcriptional/putative transcriptional regulators (SlyA, Crp, FruR, RelA/SpoT, STM1547, STM3121, SpvR, and RcsA). We chose 14 Salmonella regulators whose absence caused severe survival defect in mice (see strains in bold in Table 1) and determined the global transcriptional changes by each virulence regulator in intracellular-mimicking conditions. Collective transcriptomic data revealed an interaction network among virulence regulators and furthermore suggested a group of genes that were coordinately controlled by virulence regulators and are likely to be important for Salmonella virulence (Yoon et al., 2009). We show a simplified version of the inferred regulatory network that was obtained in this study in Figure 2. This network shows that the primary regulator responsible for SPI-2 virulence expression, SsrB, integrates signals from many regulators and two-component regulatory systems. Interestingly, this network showed that SlyA is directly upstream of SsrB, but that it also seemed to be directly regulating SPI-2 expression. Both these predictions were validated in the study, elaborating the role that SlyA plays in virulence in Salmonella.
Figure 2. Regulatory network of selected transcription factors essential for virulence. Regulators essential in systemic infection were deleted and microarray expression data under SPI-2 inducing conditions were used to construct a regulatory network. The figure shown represents a selected subset of all the regulators examined (see Yoon et al., 2009 for the complete network). The nodes indicate regulators, with the red node indicating the SPI-2 genes. Edges indicate activation (red) or repression (blue). Predictions made by this model were validated experimentally (Yoon et al., 2009).
There have been many studies that characterize the global expression of Salmonella under different conditions that are relevant to virulence (Clements et al., 2002; Eriksson et al., 2003; Lawhon et al., 2003; Kelly et al., 2004; Mangan et al., 2006; Sheng et al., 2009). While these studies do not directly address the global regulatory network driving virulence, they have been used to elucidate novel virulence factors, functional pathways involved in pathogenesis and regulons activated by virulence conditions.
Recent advances in high-throughput DNA sequencing technology have also been applied to the studies on bacterial transcriptome (Passalacqua et al., 2009; Perkins et al., 2009) as well as the eukaryotic transcriptome (Nagalakshmi et al., 2008; Wilhelm et al., 2008). Using an RNA-sequencing (RNA-seq) method, it became possible to define the composition of a whole mRNA population and evaluate gene regulation in detail at the transcriptional level. By converting RNA to DNA, Perkins et al. (2009) analyzed the transcription profile of S. Typhi in a strand-specific manner and identified small RNA and RNA transcripts originating from overlapping DNA sequences as well, which is not possible using low-density microarrays. This analysis revealed a number of novel non-coding RNAs and was used to refine the regulon of the OmpR regulator, which plays important roles in virulence.
Although transcriptomics is more commonly applied to determine gene regulatory networks, global profiling of protein abundance by proteomic analysis can also be very useful in this regard. A large-scale view of protein abundances from regulatory mutants combined with different stimuli can help identify co-regulated groups, and be used to link these groups to specific regulators. The reasons that transcriptomics are more often used to study gene regulatory networks are (i) microarray analysis has more complete coverage; and (ii) protein abundances are complex being regulated by gene expression, post-transcriptional modifications, and degradation. Recent advances in nanoflow liquid chromatography and mass spectrometry (LC–MS) have tremendously improved proteome coverage (Nilsson et al., 2010). For instance, with current technology and applying extensive protein or peptide fraction previous to LC–MS analysis (two-dimensional LC–MS or 2D-LC–MS) it is possible to routinely obtain proteome coverage of better than ∼50% of the predicted ORFs for prokaryotic organisms (Adkins et al., 2006; Malmstrom et al., 2009). Recent analysis of the S. Typhi and S. Typhimurium proteomes led to the identification of 47 and 51% of all predicted ORFs, respectively (Adkins et al., 2006; Ansong et al., 2008). It is also worth noting that a number of genes are expressed only under specific conditions and some of the predicted ORFs may not even be functional, thus the coverage of the actual expressible proteome is likely higher.
Relative to traditional transcriptomic studies, increasing depth of proteome coverage is frequently associated with labor-intensive, low-throughput analysis, thus limiting the throughput and number of samples to be studied. More commonly applied multi-dimensional LC–MS/MS experiments can detect a great number of peptides; however, the approach relies on decisions made automatically by the MS instrument on which peptides will be selected and fragmented for identification. To circumvent this issue, our team utilizes a methodology that separates the peptide identifications from the quantitative analysis, in a process called accurate mass and time (AMT) tag approach (Zimmer et al., 2006). The AMT tag approach reduces labor-intensive experimental steps and enables relatively comprehensive analysis of large number of samples with deep proteome coverage.
The AMT tag approach has enabled a number of proteomic analyses of Salmonella that would have been difficult and highly labor-intensive otherwise. This includes analyses of the combined Salmonella and host proteomes during time course analyses (Shi et al., 2006, 2009b) and analysis of post-transcriptional regulatory mutants lacking Hfq and SmpB under multiple conditions (Ansong et al., 2009). In a more recent study, we have utilized the AMT tag approach to revisit the 14 virulence regulators studied by transcriptomic analysis (Yoon et al., 2011). All 14 mutant strains lacking virulence regulators were grown in conditions that mimic intracellular environments and global proteomics measurements were made. We then inferred networks from proteomics data and used the structure and topology of these networks to find virulence effector candidates that were co-regulated with known virulence factors, such as the SPI-2 T3SS. Five of these candidates were found to be virulence proteins secreted through a non-T3SS mechanism, validating our approach and demonstrating the utility of regulatory networks. These examples show that high dimensional proteomic datasets can be useful to help elucidate gene regulatory networks or determine more complex mechanisms of protein abundance controls.
Chromatin immunoprecipitation is a popular methodology to study gene regulation. ChIP–chip and ChIP-seq enable the determination of the genes regulated by certain known regulator. In these procedures DNA is cross-linked with interacting proteins and the regulator of interest is pulled down by immunoprecipitation (Gilchrist et al., 2009). The interacting regions of the genome are determined by either microarray (ChIP–chip) or by next-generation sequencing (ChIP-seq; Gilchrist et al., 2009). An elegant example of a ChIP–chip experiment has been demonstrated by Tomljenovic-Berube et al. (2010) in which they combine microarray analysis of ssrB-mutant bacteria with ChIP–chip experiments to determine the set of genes under regulatory control of SsrA–SsrB, which are major regulators of SPI-2 genes. In another example, the regulatory network of the transcription silencer H-NS was determined by ChIP–chip analysis (Dillon et al., 2010).
Although transcription factors (TFs) are key elements of expression control, they are often associated with large protein complexes. In this context, a valuable approach consists of analyzing the immunoprecipitated protein complex-DNA by mass spectrometry (ChIP–MS) leading to the identification of gene regulator partners (Lambert et al., 2009). An approach was recently introduced that enables the determination of loci-specific regulatory machinery. This method is based on the cross-linking of DNA–protein complexes; then the DNA is hybridized with region-specific probes and pull-down by affinity purifications (AP). This method became known as reverse-ChIP since rather than immunoprecipitating the regulators to discover their interacting loci in the genome, probes are designed to pull-down proteins bound to specific DNA regions (Dejardin and Kingston, 2009).
Protein–protein interactions play an essential role in cells because many proteins are part of larger complexes (Nooren and Thornton, 2003). There are many approaches to study PPIs including in silico predictions, yeast two-hybrid (Y2H) systems and AP (Perkins et al., 2010). Although in silico predictions are very useful, experimental validation is always required to ensure data accuracy (Perkins et al., 2010). Y2H screening is a very popular methodology to study PPIs, but may present high rates of false-positive and false-negative interactions (Perkins et al., 2010). Chowdhury et al. (2009) studied PPIs for three S. Typhimurium proteins (HimD, PduB, and PhoP), by expressing them fused with a histidine–biotin–histidine tag, chemical cross-link, and two-steps AP followed by proteomic analysis. With this approach several proteins were found, including previously characterized partners for the targeted proteins (Chowdhury et al., 2009). The advantage of cross-linking proteins is that depending on the type of AP, the purification can be performed in very stringent denaturing conditions, thus decreasing undesired unspecific interactions of proteins to the affinity beads (Guerrero et al., 2006, 2008). Another AP approach to study PPIs is by performing the purifications of non-cross-linked samples in much milder conditions. However, this method can lead to more “noisy” data by having proteins binding non-specifically to beads. Recently, progress has been made to distinguish the specific interacting proteins from the background. Based on quantitative proteomic analysis and statistical analysis, Choi et al. designed a computational tool to determine the proteins that are significantly enriched compared to the background (Breitkreutz et al., 2010; Choi et al., 2011).
Although, PPI and ChIP approaches are similar in many aspects, we believe that they are complementary. Thus, the combination of both PPI and ChIP may in the future lead to a more comprehensive view of the regulatory processes in Salmonella, which will lead to the ability to predict environmental responses by Salmonella.
High-throughput data acquisition methods have been effectively used to define regulons and regulatory networks in Salmonella as discussed above. However, these approaches require sophisticated computational analysis methods to provide reliable regulatory network predictions. Additionally, analysis of other kinds of data, for example genomic sequences, can provide corroborating or supporting information about virulence regulation in Salmonella. In this section we describe some of the computational approaches that have been applied to supply supporting information about virulence regulation, to derive regulatory networks from high-throughput data, and to develop predictive models of regulatory processes in Salmonella virulence. This kind of analysis is essential to complete a systems biology approach and to make sense of the complicated high-throughput data being generated (Aderem et al., 2011).
Mining genomic sequence information has proven to be very informative about virulence in Salmonella, and this information directly supports virulence-related regulatory network analysis. Spatial organization of genes on the chromosome has been used to identify the Salmonella pathogenicity islands, which are essential for virulence and largely co-regulated (Salama and Falkow, 1999; Vernikos and Parkhill, 2006; Hensel, 2007; Chatterjee et al., 2008). An additional approach to identifying virulence-related genes from sequence information is by using the nucleotide composition of the gene, relying on the observation that higher G + C content is associated with horizontally transferred virulence genes (Collmer et al., 2002; Srividhya et al., 2007). Analysis of the promoter sequences for several TFs involved in virulence has further expanded their regulons (Wozniak and Hughes, 2008; Harari et al., 2009). Finally, we (Samudrala et al., 2009; McDermott et al., 2011) and others (Arnold et al., 2009; Lower and Schneider, 2009) have recently described methods to integrate this kind of genomic data to provide accurate prediction of type III secreted effectors, which do not share easily recognizable signal sequences that direct their secretion. These approaches have led to the definition of a number of novel effectors (Niemann et al., 2011) and have provided insights about the conservation and regulation of T3SSs across organisms (Arnold et al., 2009; Buchko et al., 2010; McDermott et al., 2011). Since type III effectors are a primary means by which Salmonella promotes virulence in the host, better definition of the secreted effector repertoire is expected to shed light on virulence regulation and evolutionary patterns associated with virulence.
Prediction of regulatory interactions between genes based on high-throughput data is called network inference. Network inference is a data-driven process that relies on the association of genes based on similar expression patterns over a collection of different experimental conditions (De Smet and Marchal, 2010). Regulatory networks can be inferred from high-throughput data with good accuracy, if sufficient data is available. As discussed above we have used network inference approaches to identify interactions between regulators essential to virulence (Yoon et al., 2009). In that study we applied an inference method that relies on the similarity between gene profiles as well as a network inference method that assesses the impact of regulator deletions on the expression levels of other regulators to derive a network of regulatory interactions (Yoon et al., 2009; Prill et al., 2010) that was validated using RT-PCR. This regulatory network highlighted the redundancy and interdependency present in the virulence regulatory network in Salmonella (Figure 2), defined points of control for the regulatory network (McDermott et al., 2009), and identified groups of important virulence genes with conserved regulatory patterns (Yoon et al., 2011). Network inference is useful as a relatively unbiased approach to determining regulatory networks, but does not make use of the considerable amount of information available about regulation in bacterial systems.
A variety of approaches to modeling transcriptional regulation have been developed over the years, many of which are reviewed by Karlebach and Shamir (2008). When it comes to analyzing transcriptional output or developing predictive integrated models of regulation and metabolism on the genome-scale, constraint-based approaches such as network component analysis (NCA; Liao et al., 2003) and Boolean networks (Kauffman, 1969; Covert et al., 2001, 2008; Gianchandani et al., 2006; Klamt et al., 2006; Shlomi et al., 2007; Graudenzi et al., 2011) and more recently probabilistic models (Chandrasekaran and Price, 2010) have been used. Figure 3 provides an overview of these methods. These methods all require a regulatory network structure as an input that may be assembled from manual curation of the literature (Gama-Castro et al., 2008), ChIP studies (Cho et al., 2009), or inference methods (Sabatti et al., 2002, 2005; Gardner et al., 2003; Margolin et al., 2006; Faith et al., 2007) discussed above and in a variety of other reviews (Chou and Voit, 2009; De Smet and Marchal, 2010). The regulatory network structure is often referred to as the connectivity matrix as it is modeled as a matrix where all of the TFs are on one axis, all of the genes on the other axis, and a non-zero element in the matrix indicates that a TF is known to influence the expression of the corresponding gene. A second common feature for these methods is that they were designed to only approximate the interactions between a TF and its target genes: NCA employs a Hill-type equation for each TF-gene interaction, Boolean models represent each gene as on or off based on the sum of Boolean interactions for all TFs that interact with the gene, and probabilistic regulation of metabolism (PROM) uses linear weights derived from prior transcriptome data to constrain flux through a gene’s associated enzymatic activities based on current TF expression profiles.
Figure 3. Systems modeling approaches. (A) Network component analysis uses transcriptome data to deduce transcription factor (TF) activities given a TF/gene (g) connectivity network. (B) Genome-scale metabolic models are constructed with all enzymes. With Boolean regulatory constraints, enzymes are either expressed or not expressed. With probabilistic regulatory constraints, the enzyme expression levels are modulated based on expression level of the regulators.
It is possible to integrate a variety of data types, such as transcriptome measurements, motifs, promoter regions, ChIP data, and growth phenotypes, with these modeling tools to update the connectivity matrix for a specific condition or during analysis to uncover additional hypothetical interactions (Foat et al., 2005; Sabatti and James, 2006; Hyduke et al., 2007; Wang et al., 2008; Barua et al., 2010; Tran et al., 2010). These methods have been predominantly used with E. coli due to the availability of a global regulatory network, but as Salmonella’s regulatory network is delineated through the methods described above it will be possible to apply these methods to the massive amounts of Salmonella high-throughput data. Since classical virulence genes such as adhesins, toxins, and secreted effectors are not included in standard metabolic models the integration of metabolic models with more descriptive data-driven models will be of paramount importance to fully model virulence in Salmonella.
There have been several studies in Salmonella that have modeled regulation of the SPI-1 T3SS, which is important for virulence as described above. Two studies (Temme et al., 2008; Saini et al., 2010) described experimental approaches to define parameters for computational models of the TFs (HilA, HilC, HilD, and RtsA) and their downstream targets (components of the T3SS and effector proteins). These studies allowed determination of how individual components in the SPI-1 regulatory network coordinate its virulence program. Though these studies are not aimed at providing a comprehensive, global view of the virulence regulatory network in Salmonella they provide valuable insight into the details of the regulatory network driving SPI-1 expression.
Computational models can be used to predict behaviors of the system under conditions not previously considered. Integrated models of regulation and metabolism may be used to simulate the growth phenotypes of microbes in a variety of conditions, including those relevant to infection (Bumann, 2009). Metabolic network reconstructions aim to be comprehensive biochemically, genetically, and genomically (BiGG) consistent knowledge bases for an organism (Reed et al., 2006; Schellenberger et al., 2010). The recent publication of a BiGG metabolic knowledgebase for Salmonella Typhimurium LT2 represents a resource that may be used as a platform for integrated modeling of metabolic activities during infection (Bumann, 2009; Thiele et al., 2011).
Mathematical models derived from BiGG knowledge bases will often predict capabilities that are not biologically realizable; this is due to the lack of regulatory constraints. Integration of Boolean regulatory constraints (Kauffman, 1969; Covert et al., 2001; Covert and Palsson, 2002) with the metabolic models increases their accuracy (Covert et al., 2004). In Covert et al. (2004), Boolean regulatory constraints were integrated with a metabolic network model for E. coli and used to simulate growth phenotypes for over 13,000 conditions described in the ASAP database (Glasner et al., 2006). The integrated model accurately simulated growth for ∼79% of the conditions; when the regulatory constraints were removed accuracy dropped to ∼65%. Amongst the set of failures, Covert et al. (2004) noticed that low oxygen (O2) concentration was a common theme. Pathogens will likely encounter low O2 levels when interacting with the innate immune response. This highlights the need to improve our knowledge of regulatory interactions in microaerobic and anaerobic conditions.
Recently, Barua et al. (2010) developed an automated approach for using global phenotypic (phenomic) data in conjunction with BiGG metabolic models to update Boolean regulatory rules. Additionally, they used their approach along with sequence homology data to construct and refine a putative regulatory network for S. Typhimurium LT2. Integrating this putative regulatory network with experimental and inference methods described above may result in an initial global regulatory network for Salmonella that can be used with NCA to interpret transcriptome data or with BiGG metabolic models to develop predictive models.
Chandrasekaran and Price (Covert and Palsson, 2002) devised another promising method to incorporate regulation with metabolism at the genome-scale. This method “PROM” integrates omics data with a priori regulatory network information to model regulation in a continuous fashion. The two attractive features of PROM are that it does not model gene expression as Boolean and that it can be used with an inferred regulatory network to make qualitative phenotypic predictions. While direct measurements of TF/target interactions are preferred it is often not possible to acquire the information for organisms of interest.
Finally, it is possible to use networks inferred from global gene expression profiling to generate models capable of predicting gene expression under novel conditions (Bonneau et al., 2007). In this approach the relationships between regulators and their target regulons are represented as mathematical equations. This set of equations can then be used to generate predictions of system behavior when growth conditions have changed or when individual regulators have been inactivated.
In addition to the explicit effectors of the virulence program (T3SS effectors, etc.), Salmonella must tightly regulate its metabolism to allow survival in the host environment. Changes in Salmonella metabolism can be addressed using metabolomics approaches, which can provide untargeted, quantitative measurements of perturbations in the metabolite complement of an integrated biological system in response to stimuli (Nicholson et al., 1999). In this respect, metabolomics analyses have not yet been widely implemented in the study of pathogenic bacteria (Bundy et al., 2005; Boroujerdi et al., 2009; Henderson et al., 2009; Himmelreich et al., 2009; de Carvalho et al., 2010; Eylert et al., 2010; Raghunathan et al., 2010; Simon et al., 2010). Indeed, only two recent papers report the application of metabolomics in the study of Salmonella: Xu et al. (2011) used a volatile organic compound (VOC)-based metabolic profiling approach to differentiate between natural spoiled pork and pork contaminated with Salmonella, and White et al. (2010) employed gas chromatography–mass spectrometry and nuclear magnetic resonance spectroscopy to identify metabolite profiles specific for Salmonella cells in radar (red, dry, and rough morphotype) colony biofilms and for cells that do not produce an extracellular matrix due to a csgD deletion mutant. Neither study addressed the Salmonella virulence regulatory network.
The role of metabolites in Salmonella virulence is two-fold. First and foremost, they represent nutrients necessary to sustain cellular growth and division and to provide the basic building blocks of virulence effectors. Studies using metabolic mutants have revealed insights into the nutritional requirements of Salmonella both in vitro and in vivo. For example, glucose is required for efficient intracellular growth in the Salmonella-containing vacuole (Tchawa Yimga et al., 2006; Gotz et al., 2010); however, a mutant unable to uptake glucose was still able to replicate within cells at a reduced rate, indicating that other carbon sources can be utilized when necessary (Bowden et al., 2009). Similar studies revealed various degrees of attenuation in mice infected by mutants lacking a complete TCA cycle (Tchawa Yimga et al., 2006), defective in converting malate to pyruvate, or lacking isocitrate lyase (Mercado-Lubo et al., 2009). The second role of metabolites in Salmonella virulence is as signaling molecules. Quorum sensing, or the recognition of bacterial cell populations based on concentrations of key molecules, is the small-molecule signaling mechanism in bacteria (Vendeville et al., 2005; Boyen et al., 2009). While the majority of Gram-negative bacteria utilize N-acylhomoserine lactones (AHLs), or autoinducer-1, as the signaling molecules (Boyen et al., 2009), Salmonella does not produce AHLs (Soares and Ahmer, 2011). Instead, Salmonella relies on autoinducer-2 molecules, such as 4,5-dihydroxy-2,3-pentanedione (DPD; Choi et al., 2007). Alternatively, the nucleotide alarmones guanosine 5′-diphosphate-3′-diphosphate and guanosine 5′-triphosphate-3′-diphosphate (collectively referred to as ppGpp) play a comprehensive role in Salmonella virulence (reviewed in Dalebroux et al., 2010). An as yet relatively unexplored area of research is the role played by host cell signaling molecules in virulence. In this respect, epinephrine was recently reported to play an important role in modulating Salmonella virulence (Spencer et al., 2010). Thus, comprehensive metabolomics analyses should be able to provide additional insights into the mechanisms by which Salmonella receives nutrition during infection and the role of small signaling molecules in virulence.
Given their ability to make predictions about the outputs of a network (e.g., virulence processes, metabolic function) given inputs (e.g., growth conditions, carbon source, environmental cures) genome-scale reconstructions will be able to provide a more complete model of Salmonella virulence. One goal of constraint-based regulatory network analysis is to be able to deduce the activity of all of an organism’s TFs in a given condition. The TF activities (TFAs) will provide insight into how the TF network responds to a given perturbation. Once the TFA changes are known in response to a specific perturbation, it may be possible to determine the underlying factors that drive these changes. One perturbation that is essential to pathogenesis is exposure to reactive nitrogen species, such as nitric oxide (NO) and nitrosothiols (RSNOs), which are produced by the innate immune in response to the presence of a pathogen and induce cytolysis of the invading pathogen (MacMicking et al., 1997; Fang, 2004). In an effort to unravel the full effects of RNS on E. coli, a variety of transcriptomic surveys were made (Mukhopadhyay et al., 2004; Flatley et al., 2005; Justino et al., 2005) and with ad hoc analysis important interactions were implicated. However, the precise targets and regulatory response have not yet been elucidated.
Based on the hypothesis that it would be possible to trace a shift in regulatory network activity in response to a perturbation back to its originating source, we designed a series of experiments where we measured the transcriptomic response of E. coli to NO and S-nitrosoglutathione and used NCA to identify the TFA perturbations (Hyduke et al., 2007). A number of the significantly perturbed TFs (IscR, NorR, NsrR) have been associated with the NO-response, however, they had not be examined as an integrated system (D’Autreaux et al., 2005; Rodionov et al., 2005; Pullan et al., 2007). Next, for the significantly perturbed TFs we constructed deletion mutants to validate the NCA calculations and observe the changes in phenotypes. From the NCA-deduced perturbations in TFAs and a series of phenotyping studies we were able to identify the iron–sulfur cluster of dihydroxyacid dehydratase as a crucial enzyme that innate immune system targets to inhibit bacterial growth. Salmonella may exhibit similar responses due to its relatively recent divergence from E. coli, however, the larger number of regulators and greater variety of pathogenic strains indicate that there will be key differences. After Salmonella’s global regulatory network is further delineated, it will be possible to use NCA to identify the crucial differences.
Salmonella systemic infection is accomplished by a sophisticated regulatory network orchestrating activities of multiple regulators. Virulence regulators process and integrate a variety of hostile environmental cues including acidic pH, antimicrobial peptides, and reactive oxygen species within macrophages, and trigger induction of specific subsets of genes required for successful replication and evading lysosomal fusion. The coordinated action by this regulatory network involves numerous virulence factors including SPI-1 and -2 T3SSs. Genes that are coordinately controlled by multiple virulence regulators under infectious conditions and show a similar expression profile to that of well-known virulence genes are more likely to be important for pathogenesis. Thus, reconstructing the global regulatory network during infection or, at the very least, under conditions that mimic the host cellular environment can serve as an efficient strategy for the identification of genes that are required for Salmonella virulence (Yoon et al., 2011).
Impairing microbial virulence could be an interesting strategy to fight infections, so we propose that these secreted effectors among others could be excellent targets for the development of new antimicrobial therapies. Drugs could be designed to interfere in their binding to host proteins or even to disrupt their enzymatic activities. In this context, small compound libraries can be screened to select the drug candidates. Rasko et al. (2008) used a library of small organic compounds to screen for molecules that bind and inhibit QseC. QseC is a sensor histidine kinase that recognizes host adrenergic signals by binding to epinephrine and norepinephrine (Rasko et al., 2008). After binding to any of these signaling molecules, QseC autophosphorylates and subsequently phosphorylates the TF QseB leading to a cascade that results in the expression of virulence factors. After screening the library, one compound named LED209 was found to bind with high affinity and to strongly inhibit QseC autophosphorylation disrupting the expression of known virulence factors. Furthermore, LED209 was also shown to decrease bacterial virulence in murine infections. Understanding regulatory networks and virulence processes at the system level will allow development of more sophisticated and nuanced approaches to target specific proteins and entire pathways. Information about the regulatory and metabolic networks controlling virulence can be used to identify better targets for drug development. Small-molecule inhibitors that can target interactions between proteins, enzymes, effector, and host proteins, or transcriptional regulatory interactions, will allow finer control of virulence processes and thus better antibiotics.
Another approach to inhibiting virulence in Salmonella is to target the T3SS apparatus itself with inhibitors. Several small-molecule inhibitors have been developed that target the T3SS and impair secretion (Nordfelth et al., 2005; Muschiol et al., 2006; Bailey et al., 2007; Negrea et al., 2007). Additionally, we have undertaken preliminary structural studies of the disordered N-terminal secretion signal of T3SS effectors in Salmonella (Buchko et al., 2010) and this information could potentially be used for future drug discovery efforts. The ability of Salmonella to introduce proteins directly into host cells has raised the possibility of targeted therapeutic approaches for other diseases using Salmonella as the delivery vehicle (Chamekh, 2010). Finally, there have been efforts to use Salmonella for biotechnology applications, such as exporting spider silk monomers (Widmaier et al., 2009). Clearly, the complicated regulatory control of virulence proteins will need to be better understood in order for progress to be made in these efforts.
In this review we have discussed various systems biology approaches to determination of regulatory networks involved in virulence in Salmonella. With the increasing body of knowledge and data arising from the high-throughput approaches discussed, and those that are currently being developed, it is very important that more sophisticated computational approaches to use this information be developed. Comprehensive systems models of Salmonella pathogenesis will have applications for antibiotic development, new strategies for therapeutic treatments, and further understanding of the complex interplay between pathogen and host during infection. Improved understanding of virulence regulation will be essential to fully realize the potential of each of these applications.
The authors declare that the research was conducted in the absence of any commercial or financial relationships that could be construed as a potential conflict of interest.
This work was supported by the National Institute of Allergy and Infectious Diseases, NIH/DHHS, through interagency agreement Y1-AI-8401-01 and NIH RO1 AI022933.
Aderem, A., Adkins, J. N., Ansong, C., Galagan, J., Kaiser, S., Korth, M. J., Law, G. L., McDermott, J. G., Proll, S. C., Rosenberger, C., Schoolnik, G., and Katze, M. G. (2011). A systems biology approach to infectious disease research: innovating the pathogen-host research paradigm. MBio 2, e00325–e00410.
Adkins, J. N., Mottaz, H. M., Norbeck, A. D., Gustin, J. K., Rue, J., Clauss, T. R., Purvine, S. O., Rodland, K. D., Heffron, F., and Smith, R. D. (2006). Analysis of the Salmonella typhimurium proteome through environmental response toward infectious conditions. Mol. Cell Proteomics 5, 1450–1461.
Alba, B. M., and Gross, C. A. (2004). Regulation of the Escherichia coli sigma-dependent envelope stress response. Mol. Microbiol. 52, 613–619.
Ansong, C., Yoon, H., Norbeck, A. D., Gustin, J. K., McDermott, J. E., Mottaz, H. M., Rue, J., Adkins, J. N., Heffron, F., and Smith, R. D. (2008). Proteomics analysis of the causative agent of typhoid fever. J. Proteome Res. 7, 546–557.
Ansong, C., Yoon, H., Porwollik, S., Mottaz-Brewer, H., Petritis, B. O., Jaitly, N., Adkins, J. N., McClelland, M., Heffron, F., and Smith, R. D. (2009). Global systems-level analysis of Hfq and SmpB deletion mutants in Salmonella : implications for virulence and global protein translation. PLoS ONE 4, e4809. doi: 10.1371/journal.pone.0004809
Arnold, R., Brandmaier, S., Kleine, F., Tischler, P., Heinz, E., Behrens, S., Niinikoski, A., Mewes, H. W., Horn, M., and Rattei, T. (2009). Sequence-based prediction of type III secreted proteins. PLoS Pathog. 5, e1000376. doi: 10.1371/journal.ppat.1000376
Bailey, L., Gylfe, A., Sundin, C., Muschiol, S., Elofsson, M., Nordström, P., Henriques-Normark, B., Lugert, R., Waldenström, A., Wolf-Watz, H., and Bergström, S. (2007). Small molecule inhibitors of type III secretion in Yersinia block the Chlamydia pneumoniae infection cycle. FEBS Lett. 581, 587–595.
Barua, D., Kim, J., and Reed, J. (2010). An automated phenotype-driven approach (GeneForce) for refining metabolic and regulatory models. PLoS Comput. Biol. 6, e1000970. doi: 10.1371/journal.pcbi.1000970
Bearson, S. M. D., Benjamin, W. H. Jr., Swords, W. E., and Foster, J. W. (1996). Acid shock induction of RpoS is mediated by the mouse virulence gene mviA of Salmonella typhimurium. J. Bacteriol. 178, 2572–2579.
Beuzon, C. R., Banks, G., Deiwick, J., Hensel, M., and Holden, D. W. (1999). pH-dependent secretion of SseB, a product of the SPI-2 type III secretion system of Salmonella typhimurium. Mol. Microbiol. 33, 806–816.
Bingle, L. E., Bailey, C. M., and Pallen, M. J. (2008). Type VI secretion: a beginner’s guide. Curr. Opin. Microbiol. 11, 3–8.
Bonneau, R. (2008). Learning biological networks: from modules to dynamics. Nat. Chem. Biol. 4, 658–664.
Bonneau, R., Facciotti, M. T., Reiss, D. J., Schmid, A. K., Pan, M., Kaur, A., Thorsson, V., Shannon, P., Johnson, M. H., Bare, J. C., Longabaugh, W., Vuthoori, M., Whitehead, K., Madar, A., Suzuki, L., Mori, T., Chang, D. E., Diruggiero, J., Johnson, C. H., Hood, L., and Baliga, N. S. (2007). A predictive model for transcriptional control of physiology in a free living cell. Cell 131, 1354–1365.
Boroujerdi, A. F., Vizcaino, M. I., Meyers, A., Pollock, E. C., Huynh, S. L., Schock, T. B., Morris, P. J., and Bearden, D. W. (2009). NMR-based microbial metabolomics and the temperature-dependent coral pathogen Vibrio coralliilyticus. Environ. Sci. Technol. 43, 7658–7664.
Bowden, S. D., Rowley, G., Hinton, J. C., and Thompson, A. (2009). Glucose and glycolysis are required for the successful infection of macrophages and mice by Salmonella enterica serovar typhimurium. Infect. Immun. 77, 3117–3126.
Boyen, F., Eeckhaut, V., Van Immerseel, F., Pasmans, F., Ducatelle, R., and Haesebrouck, F. (2009). Quorum sensing in veterinary pathogens: mechanisms, clinical importance and future perspectives. Vet. Microbiol. 135, 187–195.
Breitkreutz, A., Choi, H., Sharom, J. R., Boucher, L., Neduva, V., Larsen, B., Lin, Z. Y., Breitkreutz, B. J., Stark, C., Liu, G., Ahn, J., Dewar-Darch, D., Reguly, T., Tang, X., Almeida, R., Qin, Z. S., Pawson, T., Gingras, A. C., Nesvizhskii, A. I., and Tyers, M. (2010). A global protein kinase and phosphatase interaction network in yeast. Science 328, 1043–1046.
Buchko, G. W., Niemann, G., Baker, E. S., Belov, M. E., Smith, R. D., Heffron, F., Adkins, J. N., and McDermott, J. E. (2010). A multi-pronged search for a common structural motif in the secretion signal of Salmonella enterica serovar Typhimurium type III effector proteins. Mol Biosyst. 6, 2448–2458.
Buchmeier, N., Bossie, S., Chen, C. Y., Fang, F. C., Guiney, D. G., and Libby, S. J. (1997). SlyA, a transcriptional regulator of Salmonella typhimurium, is required for resistance to oxidative stress and is expressed in the intracellular environment of macrophages. Infect. Immun. 65, 3725–3730.
Buchmeier, N. A., and Heffron, F. (1991). Inhibition of macrophage phagosome-lysosome fusion by Salmonella typhimurium. Infect. Immun. 59, 2232–2238.
Bumann, D. (2009). System-level analysis of Salmonella metabolism during infection. Curr. Opin. Microbiol. 12, 559–567.
Bundy, J. G., Willey, T. L., Castell, R. S., Ellar, D. J., and Brindle, K. M. (2005). Discrimination of pathogenic clinical isolates and laboratory strains of Bacillus cereus by NMR-based metabolomic profiling. FEMS Microbiol. Lett. 242, 127–136.
Chamekh, M. (2010). Immunomodulation using genetically engineered bacteria for type III-mediated delivery of heterologous antigens and cytokines: potential application in vaccine and therapeutical developments. Immunopharmacol. Immunotoxicol. 32, 1–4.
Chan, K., Kim, C. C., and Falkow, S. (2005). Microarray-based detection of Salmonella enterica serovar Typhimurium transposon mutants that cannot survive in macrophages and mice. Infect. Immun. 73, 5438–5449.
Chandrasekaran, S., and Price, N. (2010). Probabilistic integrative modeling of genome-scale metabolic and regulatory networks in Escherichia coli and Mycobacterium tuberculosis. Proc. Natl. Acad. Sci. U.S.A. 107, 17845–17850.
Chatterjee, R., Chaudhuri, K., and Chaudhuri, P. (2008). On detection and assessment of statistical significance of genomic islands. BMC Genomics 9, 150. doi: 10.1186/1471-2164-9-150
Chin, A., Feucht, B., and Saier, M. J. (1987). Evidence for regulation of gluconeogenesis by the fructose phosphotransferase system in Salmonella typhimurium. J. Bacteriol. 169, 897–899.
Cho, B., Zengler, K., Qiu, Y., Park, Y. S., Knight, E. M., Barrett, C. L., Gao, Y., and Palsson, B. Ø. (2009). The transcription unit architecture of the Escherichia coli genome. Nat. Biotechnol. 27, 1043–1049.
Choi, H., Larsen, B., Lin, Z. Y., Breitkreutz, A., Mellacheruvu, D., Fermin, D., Qin, Z. S., Tyers, M., Gingras, A. C., and Nesvizhskii, A. I. (2011). SAINT: probabilistic scoring of affinity purification-mass spectrometry data. Nat. Methods 8, 70–73.
Choi, J., Shin, D., and Ryu, S. (2007). Implication of quorum sensing in Salmonella enterica serovar typhimurium virulence: the luxS gene is necessary for expression of genes in pathogenicity island 1. Infect. Immun. 75, 4885–4890.
Chou, I., and Voit, E. (2009). Recent developments in parameter estimation and structure identification of biochemical and genomic systems. Math. Biosci. 219, 57–83.
Chowdhury, S. M., Shi, L., Yoon, H., Ansong, C., Rommereim, L. M., Norbeck, A. D., Auberry, K. J., Moore, R. J., Adkins, J. N., Heffron, F., and Smith, R. D. (2009). A method for investigating protein-protein interactions related to Salmonella typhimurium pathogenesis. J. Proteome Res. 8, 1504–1514.
Clements, M. O., Eriksson, S., Thompson, A., Lucchini, S., Hinton, J. C., Normark, S., and Rhen, M. (2002). Polynucleotide phosphorylase is a global regulator of virulence and persistency in Salmonella enterica. Proc. Natl. Acad. Sci. U.S.A. 99, 8784–8789.
Collmer, A., Lindeberg, M., Petnicki-Ocwieja, T., Schneider, D. J., and Alfano, J. R. (2002). Genomic mining type III secretion system effectors in Pseudomonas syringae yields new picks for all TTSS prospectors. Trends Microbiol. 10, 462–469.
Coombes, B. K., Wickham, M. E., Lowden, M. J., Brown, N. F., and Finlay, B. B. (2005). Negative regulation of Salmonella pathogenicity island 2 is required for contextual control of virulence during typhoid. Proc. Natl. Acad. Sci. U.S.A. 102, 17460–17465.
Covert, M., Knight, E. M., Reed, J. L., Herrgard, M. J., and Palsson, B. O. (2004). Integrating high-throughput and computational data elucidates bacterial networks. Nature 429, 92–96.
Covert, M., and Palsson, B. (2002). Transcriptional regulation in constraints-based metabolic models of Escherichia coli. J. Biol. Chem. 277, 28058–28064.
Covert, M., Schilling, C., and Palsson, B. (2001). Regulation of gene expression in flux balance models of metabolism. J. Theor. Biol. 213, 73–88.
Covert, M., Xiao, N., Chen, T. J., and Karr, J. R. (2008). Integrating metabolic, transcriptional regulatory and signal transduction models in Escherichia coli. Bioinformatics 24, 2044–2050.
Crouch, M., Becker, L. A., Bang, I. S., Tanabe, H., Ouellette, A. J., and Fang, F. C. (2005). The alternative sigma factor sigma is required for resistance of Salmonella enterica serovar Typhimurium to anti-microbial peptides. Mol. Microbiol. 56, 789–799.
Curtiss, R. R., and Kelly, S. (1987). Salmonella typhimurium deletion mutants lacking adenylate cyclase and cyclic AMP receptor protein are avirulent and immunogenic. Infect. Immun. 55, 3035–3043.
Dalebroux, Z. D., Svensson, S. L., Gaynor, E. C., and Swanson, M. S. (2010). ppGpp conjures bacterial virulence. Microbiol. Mol. Biol. Rev. 74, 171–199.
D’Autreaux, B., Tucker, N. P., Dixon, R., and Spiro, S. (2005). A non-haem iron centre in the transcription factor NorR senses nitric oxide. Nature 437, 769–772.
de Carvalho, L. P., Fischer, S. M., Marrero, J., Nathan, C., Ehrt, S., and Rhee, K. Y. (2010). Metabolomics of Mycobacterium tuberculosis reveals compartmentalized co-catabolism of carbon substrates. Chem. Biol. 17, 1122–1131.
De Smet, R., and Marchal, K. (2010). Advantages and limitations of current network inference methods. Nat. Rev. Microbiol. 8, 717–729.
Dejardin, J., and Kingston, R. E. (2009). Purification of proteins associated with specific genomic loci. Cell 136, 175–186.
Dillon, S. C., Cameron, A. D., Hokamp, K., Lucchini, S., Hinton, J. C., and Dorman, C. J. (2010). Genome-wide analysis of the H-NS and Sfh regulatory networks in Salmonella typhimurium identifies a plasmid-encoded transcription silencing mechanism. Mol. Microbiol. 76, 1250–1265.
Dorman, C. J., Chatfield, S., Higgins, C. F., Hayward, C., and Dougan, G. (1989). Characterization of porin and ompR mutants of a virulent strain of Salmonella typhimurium : ompR mutants are attenuated in vivo. Infect. Immun. 57, 2136–2140.
Dunlap, N., Benjamin, W. J., and Briles, D. (1994). The intracellular nature of Salmonella infection during the early stages of mouse typhoid. Immunol. Ser. 60, 303–312.
Ellis, T. N., Leiman, S. A., and Kuehn, M. J. (2010). Naturally produced outer membrane vesicles from Pseudomonas aeruginosa elicit a potent innate immune response via combined sensing of both lipopolysaccharide and protein components. Infect. Immun. 78, 3822–3831.
Eriksson, S., Lucchini, S., Thompson, A., Rhen, M., and Hinton, J. C. (2003). Unravelling the biology of macrophage infection by gene expression profiling of intracellular Salmonella enterica. Mol. Microbiol. 47, 103–118.
Eylert, E., Herrmann, V., Jules, M., Gillmaier, N., Lautner, M., Buchrieser, C., Eisenreich, W., and Heuner, K. (2010). Isotopologue profiling of Legionella pneumophila : role of serine and glucose as carbon substrates. J. Biol. Chem. 285, 22232–22243.
Faith, J. J., Hayete, B., Thaden, J. T., Mogno, I., Wierzbowski, J., Cottarel, G., Kasif, S., Collins, J. J., and Gardner, T. S. (2007). Large-scale mapping and validation of Escherichia coli transcriptional regulation from a compendium of expression profiles. PLoS Biol. 5, e8. doi: 10.1371/journal.pbio.0050008
Fang, F. (2004). Antimicrobial reactive oxygen and nitrogen species: concepts and controversies. Nat. Rev. Microbiol. 2, 820–832.
Fang, F., Chen, C. Y., Guiney, D. G., and Xu, Y. (1996). Identification of sigma S-regulated genes in Salmonella typhimurium : complementary regulatory interactions between sigma S and cyclic AMP receptor protein. J. Bacteriol. 178, 5112–5120.
Fields, P., Swanson, R. V., Haidaris, C. G., and Heffron, F. (1986). Mutants of Salmonella typhimurium that cannot survive within the macrophage are avirulent. Proc. Natl. Acad. Sci. U.S.A. 83, 5189–5193.
Figueroa-Bossi, N., Lemire, S., Maloriol, D., Balbontín, R., Casadesús, J., and Bossi, L. (2006). Loss of Hfq activates the sigmaE-dependent envelope stress response in Salmonella enterica. Mol. Microbiol. 62, 838–852.
Flatley, J., Barrett, J., Pullan, S. T., Hughes, M. N., Green, J., and Poole, R. K. (2005). Transcriptional responses of Escherichia coli to S-nitrosoglutathione under defined chemostat conditions reveal major changes in methionine biosynthesis. J. Biol. Chem. 280, 10065–10072.
Foat, B., Houshmandi, S. S., Olivas, W. M., and Bussemaker, H. J. (2005). Profiling condition-specific, genome-wide regulation of mRNA stability in yeast. Proc. Natl. Acad. Sci. U.S.A. 102, 17675–17680.
Gal-Mor, O., Gibson, D. L., Baluta, D., Vallance, B. A., and Finlay, B. B. (2008). A novel secretion pathway of Salmonella enterica acts as an antivirulence modulator during salmonellosis. PLoS Pathog. 4, e1000036. doi: 10.1371/journal.ppat.1000036
Gama-Castro, S., Jiménez-Jacinto, V., Peralta-Gil, M., Santos-Zavaleta, A., Peñaloza-Spinola, M. I., Contreras-Moreira, B., Segura-Salazar, J., Muñiz-Rascado, L., Martínez-Flores, I., Salgado, H., Bonavides-Martínez, C., Abreu-Goodger, C., Rodríguez-Penagos, C., Miranda-Ríos, J., Morett, E., Merino, E., Huerta, A. M., Treviño-Quintanilla, L., and Collado-Vides, J. (2008). RegulonDB (version 6.0): gene regulation model of Escherichia coli K-12 beyond transcription, active (experimental) annotated promoters and Textpresso navigation. Nucleic Acids Res. 36, D120–D124.
Gardner, T., di Bernardo, D., Lorenz, D., and Collins, J. J. (2003). Inferring genetic networks and identifying compound mode of action via expression profiling. Science 301, 102–105.
Geddes, K., Cruz, F., and Heffron, F. (2007). Analysis of cells targeted by Salmonella type III secretion in vivo. PLoS Pathog. 3, e196. doi: 10.1371/journal.ppat.0030196
Gianchandani, E., Papin, J. A., Price, N. D., Joyce, A. R., and Palsson, B. O. (2006). Matrix formalism to describe functional states of transcriptional regulatory systems. PLoS Comput. Biol. 2, e101. doi: 10.1371/journal.pcbi.0020101
Gilchrist, D. A., Fargo, D. C., and Adelman, K. (2009). Using ChIP-chip and ChIP-seq to study the regulation of gene expression: genome-wide localization studies reveal widespread regulation of transcription elongation. Methods 48, 398–408.
Glasner, J., Rusch, M., Liss, P., Plunkett, G. III, Cabot, E. L., Darling, A., Anderson, B. D., Infield-Harm, P., Gilson, M. C., and Perna, N. T. (2006). ASAP: a resource for annotating, curating, comparing, and disseminating genomic data. Nucleic Acids Res. 34, D41–D45.
Goffeau, A., Barrell, B. G., Bussey, H., Davis, R. W., Dujon, B., Feldmann, H., Galibert, F., Hoheisel, J. D., Jacq, C., Johnston, M., Louis, E. J., Mewes, H. W., Murakami, Y., Philippsen, P., Tettelin, H., and Oliver, S. G. (1996). Life with 6000 genes. Science 274, 563–567.
Gotz, A., Eylert, E., Eisenreich, W., and Goebel, W. (2010). Carbon metabolism of enterobacterial human pathogens growing in epithelial colorectal adenocarcinoma (Caco-2) cells. PLoS ONE 5, e10586. doi: 10.1371/journal.pone.0010586
Graham, S. (2002). Salmonellosis in children in developing and developed countries and populations. Curr. Opin. Infect. Dis. 15, 507–512.
Graudenzi, A., Serra, R., Villani, M., Damiani, C., Colacci, A., and Kauffman, S. A. (2011). Dynamical properties of a Boolean model of gene regulatory network with memory. J. Comput. Biol. doi: 10.1089/cmb.2010.0069. [Epub ahead of print].
Groisman, E., Chiao, E., Lipps, C. J., and Heffron, F. (1989). Salmonella typhimurium phoP virulence gene is a transcriptional regulator. Proc. Natl. Acad. Sci. U.S.A. 86, 7077–7081.
Guerrero, C., Milenkovic, T., Przulj, N., Kaiser, P., and Huang, L. (2008). Characterization of the proteasome interaction network using a QTAX-based tag-team strategy and protein interaction network analysis. Proc. Natl. Acad. Sci. U.S.A. 105, 13333–13338.
Guerrero, C., Tagwerker, C., Kaiser, P., and Huang, L. (2006). An integrated mass spectrometry-based proteomic approach: quantitative analysis of tandem affinity-purified in vivo cross-linked protein complexes (QTAX) to decipher the 26 S proteasome-interacting network. Mol. Cell Proteomics 5, 366–378.
Haraga, A., Ohlson, M. B., and Miller, S. I. (2008). Salmonellae interplay with host cells. Nat. Rev. Microbiol. 6, 53–66.
Harari, O., del Val, C., Romero-Zaliz, R., Shin, D., Huang, H., Groisman, E. A., and Zwir, I. (2009). Identifying promoter features of co-regulated genes with similar network motifs. BMC Bioinformatics 10(Suppl. 4), S1. doi: 10.1186/1471-2105-10-S4-S1
Henderson, J. P., Crowley, J. R., Pinkner, J. S., Walker, J. N., Tsukayama, P., Stamm, W. E., Hooton, T. M., and Hultgren, S. J. (2009). Quantitative metabolomics reveals an epigenetic blueprint for iron acquisition in uropathogenic Escherichia coli. PLoS Pathog. 5, e1000305. doi: 10.1371/journal.ppat.1000305
Hensel, M. (2007). Genome-based identification and molecular analyses of pathogenicity islands and genomic islands in Salmonella enterica. Methods Mol. Biol. 394, 77–88.
Hensel, M., Shea, J. E., Waterman, S. R., Mundy, R., Nikolaus, T., Banks, G., Vazquez-Torres, A., Gleeson, C., Fang, F. C., and Holden, D. W. (1998). Genes encoding putative effector proteins of the type III secretion system of Salmonella pathogenicity island 2 are required for bacterial virulence and proliferation in macrophages. Mol. Microbiol. 30, 163–174.
Himmelreich, U., Malik, R., Kühn, T., Daniel, H. M., Somorjai, R. L., Dolenko, B., and Sorrell, T. C. (2009). Rapid etiological classification of meningitis by NMR spectroscopy based on metabolite profiles and host response. PLoS ONE 4, e5328. doi: 10.1371/journal.pone.0005328
Hyduke, D., Jarboe, L. R., Tran, L. M., Chou, K. J., and Liao, J. C. (2007). Integrated network analysis identifies nitric oxide response networks and dihydroxyacid dehydratase as a crucial target in Escherichia coli. Proc. Natl. Acad. Sci. U.S.A. 104, 8484–8489.
Justino, M., Vicente, J. B., Teixeira, M., and Saraiva, L. M. (2005). New genes implicated in the protection of anaerobically grown Escherichia coli against nitric oxide. J. Biol. Chem. 280, 2636–2643.
Karlebach, G., and Shamir, R. (2008). Modelling and analysis of gene regulatory networks. Nat. Rev. Mol. Cell Biol. 9, 770–780.
Kauffman, S. (1969). Metabolic stability and epigenesis in randomly constructed genetic nets. J. Theor. Biol. 22, 437–467.
Kelly, A., Goldberg, M. D., Carroll, R. K., Danino, V., Hinton, J. C., and Dorman, C. J. (2004). A global role for Fis in the transcriptional control of metabolism and type III secretion in Salmonella enterica serovar Typhimurium. Microbiology 150(Pt 7), 2037–2053.
Klamt, S., Saez-Rodriguez, J., Lindquist, J. A., Simeoni, L., and Gilles, E. D. (2006). A methodology for the structural and functional analysis of signaling and regulatory networks. BMC Bioinformatics 7, 56. doi: 10.1186/1471-2105-7-56
Kowarz, L., Coynault, C., Robbe-Saule, V., and Norel, F. (1994). The Salmonella typhimurium katF (rpoS) gene: cloning, nucleotide sequence, and regulation of spvR and spvABCD virulence plasmid genes. J. Bacteriol. 176, 6852–6860.
Krause, M., Fang, F., and Guiney, D. (1992). Regulation of plasmid virulence gene expression in Salmonella Dublin involves an unusual operon structure. J. Bacteriol. 174, 4482–4489.
Lambert, J. P., Mitchell, L., Rudner, A., Baetz, K., and Figeys, D. (2009). A novel proteomics approach for the discovery of chromatin-associated protein networks. Mol. Cell Proteomics 8, 870–882.
Lawhon, S., Frye, J. G., Suyemoto, M., Porwollik, S., McClelland, M., and Altier, C. (2003). Global regulation by CsrA in Salmonella typhimurium. Mol. Microbiol. 48, 1633–1645.
Lawley, T. D., Chan, K., Thompson, L. J., Kim, C. C., Govoni, G. R., and Monack, D. M. (2006). Genome-wide screen for Salmonella genes required for long-term systemic infection of the mouse. PLoS Pathog. 2, e11. doi: 10.1371/journal.ppat.0020011
Liao, J., Boscolo, R., Yang, Y. L., Tran, L. M., Sabatti, C., and Roychowdhury, V. P. (2003). Network component analysis: reconstruction of regulatory signals in biological systems. Proc. Natl. Acad. Sci. U.S.A. 100, 15522–15527.
Lockman, H., and Curtiss, R. R. (1990). Salmonella typhimurium mutants lacking flagella or motility remain virulent in BALB/c mice. Infect. Immun. 58, 137–143.
Lower, M., and Schneider, G. (2009). Prediction of type III secretion signals in genomes of gram-negative bacteria. PLoS ONE 4, e5917. doi: 10.1371/journal.pone.0005917
Lucas, R., and Lee, C. (2001). Roles of hilC and hilD in regulation of hilA expression in Salmonella enterica serovar Typhimurium. J. Bacteriol. 183, 2733–2745.
MacMicking, J., Xie, Q., and Nathan, C. (1997). Nitric oxide and macrophage function. Annu. Rev. Immunol. 15, 323–350.
Macquarrie, K. L., Fong, A. P., Morse, R. H., and Tapscott, S. J. (2011). Genome-wide transcription factor binding: beyond direct target regulation. Trends Genet. 27, 141–148.
Malmstrom, J., Beck, M., Schmidt, A., Lange, V., Deutsch, E. W., and Aebersold, R. (2009). Proteome-wide cellular protein concentrations of the human pathogen Leptospira interrogans. Nature 460, 762–765.
Mangan, M., Lucchini, S., Danino, V., Cróinín, T. O., Hinton, J. C., and Dorman, C. J. (2006). The integration host factor (IHF) integrates stationary-phase and virulence gene expression in Salmonella enterica serovar Typhimurium. Mol. Microbiol. 59, 1831–1847.
Margolin, A. A., Nemenman, I., Basso, K., Wiggins, C., Stolovitzky, G., Dalla Favera, R., and Califano, A. (2006). ARACNE: an algorithm for the reconstruction of gene regulatory networks in a mammalian cellular context. BMC Bioinformatics 7(Suppl. 1), S7. doi: 10.1186/1471-2105-7-S1-S7
McClelland, M., Sanderson, K. E., Spieth, J., Clifton, S. W., Latreille, P., Courtney, L., Porwollik, S., Ali, J., Dante, M., Du, F., Hou, S., Layman, D., Leonard, S., Nguyen, C., Scott, K., Holmes, A., Grewal, N., Mulvaney, E., Ryan, E., Sun, H., Florea, L., Miller, W., Stoneking, T., Nhan, M., Waterston, R., and Wilson, R. K. (2001). Complete genome sequence of Salmonella enterica serovar Typhimurium LT2. Nature 413, 852–856.
McDermott, J. E., Corrigan, A., Peterson, E., Oehmen, C., Niemann, G., Cambronne, E. D., Sharp, D., Adkins, J. N., Samudrala, R., and Heffron, F. (2011). Computational prediction of type III and IV secreted effectors in gram-negative bacteria. Infect. Immun. 79, 23–32.
McDermott, J. E., Taylor, R. C., Yoon, H., and Heffron, F. (2009). Bottlenecks and hubs in inferred networks are important for virulence in Salmonella typhimurium. J. Comput. Biol. 16, 169–180.
McGhie, E. J., Brawn, L. C., Hume, P. J., Humphreys, D., and Koronakis, V. (2009). Salmonella takes control: effector-driven manipulation of the host. Curr. Opin. Microbiol. 12, 117–124.
Mercado-Lubo, R., Leatham, M. P., Conway, T., and Cohen, P. S. (2009). Salmonella enterica serovar Typhimurium mutants unable to convert malate to pyruvate and oxaloacetate are avirulent and immunogenic in BALB/c mice. Infect. Immun. 77, 1397–1405.
Monack, D., Bouley, D., and Falkow, S. (2004). Salmonella typhimurium persists within macrophages in the mesenteric lymph nodes of chronically infected Nramp1+/+ mice and can be reactivated by IFNgamma neutralization. J. Exp. Med. 199, 231–241.
Mukhopadhyay, P., Zheng, M., Bedzyk, L. A., LaRossa, R. A., and Storz, G. (2004). Prominent roles of the NorR and Fur regulators in the Escherichia coli transcriptional response to reactive nitrogen species. Proc. Natl. Acad. Sci. U.S.A. 101, 745–750.
Munro, P., Flatau, G. N., Clément, R. L., and Gauthier, M. J. (1995). Influence of the RpoS (KatF) sigma factor on maintenance of viability and culturability of Escherichia coli and Salmonella typhimurium in seawater. Appl. Environ. Microbiol. 61, 1853–1858.
Muschiol, S., Bailey, L., Gylfe, A., Sundin, C., Hultenby, K., Bergström, S., Elofsson, M., Wolf-Watz, H., Normark, S., and Henriques-Normark, B. (2006). A small-molecule inhibitor of type III secretion inhibits different stages of the infectious cycle of Chlamydia trachomatis. Proc. Natl. Acad. Sci. U.S.A. 103, 14566–14571.
Nagalakshmi, U., Wang, Z., Waern, K., Shou, C., Raha, D., Gerstein, M., and Snyder, M. (2008). The transcriptional landscape of the yeast genome defined by RNA sequencing. Science 320, 1344–1349.
Negrea, A., Bjur, E., Ygberg, S. E., Elofsson, M., Wolf-Watz, H., and Rhen, M. (2007). Salicylidene acylhydrazides that affect type III protein secretion in Salmonella enterica serovar typhimurium. Antimicrob. Agents Chemother. 51, 2867–2876.
Nicholson, J. K., Lindon, J. C., and Holmes, E. (1999). “Metabonomics”: understanding the metabolic responses of living systems to pathophysiological stimuli via multivariate statistical analysis of biological NMR spectroscopic data. Xenobiotica 29, 1181–1189.
Niemann, G., Brown, R. N., Gustin, J. K., Stufkens, A., Shaikh-Kidwai, A. S., Li, J., McDermott, J. E., Brewer, H. M., Schepmoes, A., Smith, R. D., Adkins, J. N., and Heffron, F. (2011). Discovery of novel secreted virulence factors from Salmonella enterica serovar Typhimurium by proteomic analysis of culture supernatants. Infect. Immun. 79, 33–43.
Nilsson, T., Mann, M., Aebersold, R., Yates, J. R. III, Bairoch, A., and Bergeron, J. J. (2010). Mass spectrometry in high-throughput proteomics: ready for the big time. Nat. Methods 7, 681–685.
Nooren, I. M., and Thornton, J. M. (2003). Diversity of protein-protein interactions. EMBO J. 22, 3486–3492.
Nordfelth, R., Kauppi, A. M., Norberg, H. A., Wolf-Watz, H., and Elofsson, M. (2005). Small-molecule inhibitors specifically targeting type III secretion. Infect. Immun. 73, 3104–3114.
Ochman, H., Soncini, F. C., Solomon, F., and Groisman, E. A. (1996). Identification of a pathogenicity island required for Salmonella survival in host cells. Proc. Natl. Acad. Sci. U.S.A. 93, 7800–7804.
Ohnishi, K., Kutsukake, K., Suzuki, H., and Iino, T. (1990). Gene fliA encodes an alternative sigma factor specific for flagellar operons in Salmonella typhimurium. Mol. Gen. Genet. 221, 139–147.
Osborne, S., and Coombes, B. (2009). RpoE fine tunes expression of a subset of SsrB-regulated virulence factors in Salmonella enterica serovar Typhimurium. BMC Microbiol. 9, 45. doi: 10.1186/1471-2180-9-45
Parsons, D. A., and Heffron, F. (2005). sciS, an icmF homolog in Salmonella enterica serovar Typhimurium, limits intracellular replication and decreases virulence. Infect. Immun. 73, 4338–4345.
Passalacqua, K. D., Varadarajan, A., Ondov, B. D., Okou, D. T., Zwick, M. E., and Bergman, N. H. (2009). Structure and complexity of a bacterial transcriptome. J. Bacteriol. 191, 3203–3211.
Pedersen, K., Lassen-Nielsen, A. M., Nordentoft, S., and Hammer, A. S. (2009). Serovars of Salmonella from captive reptiles. Zoonoses Public Health 56, 238–242.
Perkins, J. R., Diboun, I., Dessailly, B. H., Lees, J. G., and Orengo, C. (2010). Transient protein-protein interactions: structural, functional, and network properties. Structure 18, 1233–1243.
Perkins, T. T., Kingsley, R. A., Fookes, M. C., Gardner, P. P., James, K. D., Yu, L., Assefa, S. A., He, M., Croucher, N. J., Pickard, D. J., Maskell, D. J., Parkhill, J., Choudhary, J., Thomson, N. R., and Dougan, G. (2009). A strand-specific RNA-Seq analysis of the transcriptome of the typhoid bacillus Salmonella typhi. PLoS Genet. 5, e1000569. doi: 10.1371/journal.pgen.1000569
Poh, J., Odendall, C., Spanos, A., Boyle, C., Liu, M., Freemont, P., and Holden, D. W. (2008). SteC is a Salmonella kinase required for SPI-2-dependent F-actin remodelling. Cell. Microbiol. 10, 20–30.
Prill, R. J., Marbach, D., Saez-Rodriguez, J., Sorger, P. K., Alexopoulos, L. G., Xue, X., Clarke, N. D., Altan-Bonnet, G., and Stolovitzky, G. (2010). Towards a rigorous assessment of systems biology models: the DREAM3 challenges. PLoS ONE 5, e9202. doi: 10.1371/journal.pone.0009202
Pullan, S., Gidley, M. D., Jones, R. A., Barrett, J., Stevanin, T. M., Read, R. C., Green, J., and Poole, R. K. (2007). Nitric oxide in chemostat-cultured Escherichia coli is sensed by Fnr and other global regulators: unaltered methionine biosynthesis indicates lack of S nitrosation. J. Bacteriol. 189, 1845–1855.
Raghunathan, A., Shin, S., and Daefler, S. (2010). Systems approach to investigating host-pathogen interactions in infections with the biothreat agent Francisella. Constraints-based model of Francisella tularensis. BMC Syst. Biol. 4, 118. doi: 10.1186/1752-0509-4-118
Ramsden, A. E., Mota, L. J., Münter, S., Shorte, S. L., and Holden, D. W. (2007). The SPI-2 type III secretion system restricts motility of Salmonella -containing vacuoles. Cell. Microbiol. 9, 2517–2529.
Rasko, D. A., Moreira, C. G., Li de, R., Reading, N. C., Ritchie, J. M., Waldor, M. K., Williams, N., Taussig, R., Wei, S., Roth, M., Hughes, D. T., Huntley, J. F., Fina, M. W., Falck, J. R., and Sperandio, V. (2008). Targeting QseC signaling and virulence for antibiotic development. Science 321, 1078–1080.
Reed, J., Patel, T. R., Chen, K. H., Joyce, A. R., Applebee, M. K., Herring, C. D., Bui, O. T., Knight, E. M., Fong, S. S., and Palsson, B. O. (2006). Systems approach to refining genome annotation. Proc. Natl. Acad. Sci. U.S.A. 103, 17480–17484.
Rodionov, D., Dubchak, I. L., Arkin, A. P., Alm, E. J., and Gelfand, M. S. (2005). Dissimilatory metabolism of nitrogen oxides in bacteria: comparative reconstruction of transcriptional networks. PLoS Comput. Biol. 1, e55. doi: 10.1371/journal.pcbi.0010055
Sabatti, C., and James, G. (2006). Bayesian sparse hidden components analysis for transcription regulation networks. Bioinformatics 22, 739–746.
Sabatti, C., Rohlin, L., Lange, K., and Liao, J. C. (2005). Vocabulon: a dictionary model approach for reconstruction and localization of transcription factor binding sites. Bioinformatics 21, 922–931.
Sabatti, C., Rohlin, L., Oh, M. K., and Liao, J. C. (2002). Co-expression pattern from DNA microarray experiments as a tool for operon prediction. Nucleic Acids Res. 30, 2886–2893.
Saini, S., Ellermeier, J. R., Slauch, J. M., and Rao, C. V. (2010). The role of coupled positive feedback in the expression of the SPI1 type three secretion system in Salmonella. PLoS Pathog. 6, e1001025. doi: 10.1371/journal.ppat.1001025
Salama, N. R., and Falkow, S. (1999). Genomic clues for defining bacterial pathogenicity. Microbes Infect. 1, 615–619.
Samudrala, R., Heffron, F., and McDermott, J. E. (2009). Accurate prediction of secreted substrates and identification of a conserved putative secretion signal for type III secretion systems. PLoS Pathog. 5, e1000375. doi: 10.1371/journal.ppat.1000375
Schechter, L., and Lee, C. (2001). AraC/XylS family members, HilC and HilD, directly bind and derepress the Salmonella typhimurium hilA promoter. Mol. Microbiol. 40, 1289–1299.
Schellenberger, J., Park, J. O., Conrad, T. M., and Palsson, B. Ø. (2010). BiGG: a biochemical genetic and genomic knowledgebase of large scale metabolic reconstructions. BMC Bioinformatics 11, 213. doi: 10.1186/1471-2105-11-213
Sheng, X., Huang, X., Li, J., Xie, X., Xu, S., Zhang, H., and Xu, H. (2009). Regulation of sulfur assimilation pathways in Salmonella enterica serovar Typhi upon up-shift high osmotic treatment: the role of UhpA revealed through transcriptome profiling. Curr. Microbiol. 59, 628–635.
Shi, L., Adkins, J. N., Coleman, J. R., Schepmoes, A. A., Dohnkova, A., Mottaz, H. M., Norbeck, A. D., Purvine, S. O., Manes, N. P., Smallwood, H. S., Wang, H., Forbes, J., Gros, P., Uzzau, S., Rodland, K. D., Heffron, F., Smith, R. D., and Squier, T. C. (2006). Proteomic analysis of Salmonella enterica serovar typhimurium isolated from RAW 264.7 macrophages: identification of a novel protein that contributes to the replication of serovar typhimurium inside macrophages. J. Biol. Chem. 281, 29131–29140.
Shi, L., Ansong, C., Smallwood, H., Rommereim, L., McDermott, J. E., Brewer, H. M., Norbeck, A. D., Taylor, R. C., Gustin, J. K., Heffron, F., Smith, R. D., and Adkins, J. N. (2009a). Proteome of Salmonella enterica serotype Typhimurium grown in a low Mg2+/pH medium. J. Proteomics Bioinform. 2, 388–397.
Shi, L., Chowdhury, S. M., Smallwood, H. S., Yoon, H., Mottaz-Brewer, H. M., Norbeck, A. D., McDermott, J. E., Clauss, T. R., Heffron, F., Smith, R. D., and Adkins, J. N. (2009b). Proteomic investigation of the time course responses of RAW 264.7 macrophages to infection with Salmonella enterica. Infect. Immun. 77, 3227–3233.
Shlomi, T., Eisenberg, Y., Sharan, R., and Ruppin, E. (2007). A genome-scale computational study of the interplay between transcriptional regulation and metabolism. Mol. Syst. Biol. 3, 101.
Simon, C., Langlois-Meurinne, M., Bellvert, F., Garmier, M., Didierlaurent, L., Massoud, K., Chaouch, S., Marie, A., Bodo, B., Kauffmann, S., Noctor, G., and Saindrenan, P. (2010). The differential spatial distribution of secondary metabolites in Arabidopsis leaves reacting hypersensitively to Pseudomonas syringae pv. tomato is dependent on the oxidative burst. J. Exp. Bot. 61, 3355–3370.
Sittka, A., Sharma, C. M., Rolle, K., and Vogel, J. (2009). Deep sequencing of Salmonella RNA associated with heterologous Hfq proteins in vivo reveals small RNAs as a major target class and identifies RNA processing phenotypes. RNA Biol. 6, 266–275.
Soares, J. A., and Ahmer, B. M. (2011). Detection of acyl-homoserine lactones by Escherichia and Salmonella. Curr. Opin. Microbiol. 14, 188–193.
Song, M., Kim, H. J., Kim, E. Y., Shin, M., Lee, H. C., Hong, Y., Rhee, J. H., Yoon, H., Ryu, S., Lim, S., and Choy, H. E. (2004). ppGpp-dependent stationary phase induction of genes on Salmonella pathogenicity island 1. J. Biol. Chem. 279, 34183–34190.
Spencer, H., Karavolos, M. H., Bulmer, D. M., Aldridge, P., Chhabra, S. R., Winzer, K., Williams, P., and Khan, C. M. (2010). Genome-wide transposon mutagenesis identifies a role for host neuroendocrine stress hormones in regulating the expression of virulence genes in Salmonella. J. Bacteriol. 192, 714–724.
Srividhya, K. V., Alaguraj, V., Poornima, G., Kumar, D., Singh, G. P., Raghavenderan, L., Katta, A. V., Mehta, P., and Krishnaswamy, S. (2007). Identification of prophages in bacterial genomes by dinucleotide relative abundance difference. PLoS ONE 2, e1193. doi: 10.1371/journal.pone.0001193
Steele-Mortimer, O. (2008). The Salmonella -containing vacuole: moving with the times. Curr. Opin. Microbiol. 11, 38–45.
Tchawa Yimga, M., Leatham, M. P., Allen, J. H., Laux, D. C., Conway, T., and Cohen, P. S. (2006). Role of gluconeogenesis and the tricarboxylic acid cycle in the virulence of Salmonella enterica serovar Typhimurium in BALB/c mice. Infect. Immun. 74, 1130–1140.
Temme, K., Salis, H., Tullman-Ercek, D., Levskaya, A., Hong, S. H., and Voigt, C. A. (2008). Induction and relaxation dynamics of the regulatory network controlling the type III secretion system encoded within Salmonella pathogenicity island 1. J. Mol. Biol. 377, 47–61.
Teplitski, M., Goodier, R., and Ahmer, B. (2003). Pathways leading from BarA/SirA to motility and virulence gene expression in Salmonella. J. Bacteriol. 185, 7257–7265.
Teplitski, M., Goodier, R., and Ahmer, B. (2006). Catabolite repression of the SirA regulatory cascade in Salmonella enterica. Int. J. Med. Microbiol. 296, 449–466.
Thiele, I., Hyduke, D. R., Steeb, B., Fankam, G., Allen, D. K., Bazzani, S., Charusanti, P., Chen, F. C., Fleming, R. M., Hsiung, C. A., De Keersmaecker, S. C., Liao, Y. C., Marchal, K., Mo, M. L., özdemir, E., Raghunathan, A., Reed, J. L., Shin, S. I., Sigurbjörnsdóttir, S., Steinmann, J., Sudarsan, S., Swainston, N., Thijs, I. M., Zengler, K., Palsson, B. O., Adkins, J. N., and Bumann, D. (2011). A community effort towards a knowledge-base and mathematical model of the human pathogen Salmonella typhimurium LT2. BMC Syst. Biol. 5, 8. doi: 10.1186/1752-0509-5-8
Tomljenovic-Berube, A. M., Mulder, D. T., Whiteside, M. D., Brinkman, F. S., and Coombes, B. K. (2010). Identification of the regulatory logic controlling Salmonella pathoadaptation by the SsrA-SsrB two-component system. PLoS Genet. 6, e1000875. doi: 10.1371/journal.pgen.1000875
Tran, L., Hyduke, D., and Liao, J. (2010). Trimming of mammalian transcriptional networks using network component analysis. BMC Bioinformatics 11, 511. doi: 10.1186/1471-2105-11-511
Vendeville, A., Winzer, K., Heurlier, K., Tang, C. M., and Hardie, K. R. (2005). Making “sense” of metabolism: autoinducer-2, LuxS and pathogenic bacteria. Nat. Rev. Microbiol. 3, 383–396.
Vernikos, G., Thomson, N., and Parkhill, J. (2007). Genetic flux over time in the Salmonella lineage. Genome Biol. 8, R100.
Vernikos, G. S., and Parkhill, J. (2006). Interpolated variable order motifs for identification of horizontally acquired DNA: revisiting the Salmonella pathogenicity islands. Bioinformatics 22, 2196–2203.
Virlogeux, I., Waxin, H., Ecobichon, C., Lee, J. O., and Popoff, M. Y. (1996). Characterization of the rcsA and rcsB genes from Salmonella typhi : rcsB through tviA is involved in regulation of Vi antigen synthesis. J. Bacteriol. 178, 1691–1698.
Wang, C., Xuan, J., Chen, L., Zhao, P., Wang, Y., Clarke, R., and Hoffman, E. (2008). Motif-directed network component analysis for regulatory network inference. BMC Bioinformatics 9(Suppl. 1), S21. doi: 10.1186/1471-2105-9-S1-S21
White, A. P., Weljie, A. M., Apel, D., Zhang, P., Shaykhutdinov, R., Vogel, H. J., and Surette, M. G. (2010). A global metabolic shift is linked to Salmonella multicellular development. PLoS ONE 5, e11814. doi: 10.1371/journal.pone.0011814
Widmaier, D. M., Tullman-Ercek, D., Mirsky, E. A., Hill, R., Govindarajan, S., Minshull, J., and Voigt, C. A. (2009). Engineering the Salmonella type III secretion system to export spider silk monomers. Mol. Syst. Biol. 5, 309.
Wilhelm, B. T., Marguerat, S., Watt, S., Schubert, F., Wood, V., Goodhead, I., Penkett, C. J., Rogers, J., and Bähler, J. (2008). Dynamic repertoire of a eukaryotic transcriptome surveyed at single-nucleotide resolution. Nature 453, 1239–1243.
Wilson, R., Libby, S. J., Freet, A. M., Boddicker, J. D., Fahlen, T. F., and Jones, B. D. (2001). Fis, a DNA nucleoid-associated protein, is involved in Salmonella typhimurium SPI-1 invasion gene expression. Mol. Microbiol. 39, 79–88.
Wozniak, C. E., and Hughes, K. T. (2008). Genetic dissection of the consensus sequence for the class 2 and class 3 flagellar promoters. J. Mol. Biol. 379, 936–952.
Xu, Y., Cheung, W., Winder, C. L., Dunn, W. B., and Goodacre, R. (2011). Metabolic profiling of meat: assessment of pork hygiene and contamination with Salmonella typhimurium. Analyst 136, 508–514.
Yoon, H., Ansong, C., Adkins, J. N., and Heffron, F. (2011). Secretion of Salmonella virulence factors into host cytoplasm via outer membrane vesicles. BMC Syst. Biol. (in press).
Yoon, H., Gros, and Heffron, F. (2011). Quantitative PCR-based competitive index for high-throughput screening of Salmonella virulence factors. Infect. Immun. 79, 360–368.
Yoon, H., McDermott, J. E., Porwollik, S., McClelland, M., and Heffron, F. (2009). Coordinated regulation of virulence during systemic infection of Salmonella enterica serovar Typhimurium. PLoS Pathog. 5, e1000306. doi: 10.1371/journal.ppat.1000306
Yrlid, U., and Wick, M. (2002). Antigen presentation capacity and cytokine production by murine splenic dendritic cell subsets upon Salmonella encounter. J. Immunol. 169, 108–116.
Zhou, D., and Galan, J. (2001). Salmonella entry into host cells: the work in concert of type III secreted effector proteins. Microbes Infect. 3, 1293–1298.
Keywords: Salmonella, virulence, regulatory network, regulators, transcriptomics, proteomics, computational modeling
Citation: McDermott JE, Yoon H, Nakayasu ES, Metz TO, Hyduke DR, Kidwai AS, Palsson BO, Adkins JN and Heffron F (2011) Technologies and approaches to elucidate and model the virulence program of Salmonella. Front. Microbio. 2:121. doi: 10.3389/fmicb.2011.00121
Received: 01 March 2011; Accepted: 15 May 2011;
Published online: 02 June 2011.
Edited by:
John S. Gunn, The Ohio State University, USAReviewed by:
Brian Ahmer, The Ohio State University, USACopyright: © 2011 McDermott, Yoon, Nakayasu, Metz, Hyduke, Kidwai, Palsson, Adkins and Heffron. This is an open-access article subject to a non-exclusive license between the authors and Frontiers Media SA, which permits use, distribution and reproduction in other forums, provided the original authors and source are credited and other Frontiers conditions are complied with.
*Correspondence: Jason E. McDermott, Computational Biology and Bioinformatics Group, Pacific Northwest National Laboratory, 902 Battelle Boulevard, Richland, WA, USA. e-mail:amFzb24ubWNkZXJtb3R0QHBubC5nb3Y=
Disclaimer: All claims expressed in this article are solely those of the authors and do not necessarily represent those of their affiliated organizations, or those of the publisher, the editors and the reviewers. Any product that may be evaluated in this article or claim that may be made by its manufacturer is not guaranteed or endorsed by the publisher.
Research integrity at Frontiers
Learn more about the work of our research integrity team to safeguard the quality of each article we publish.