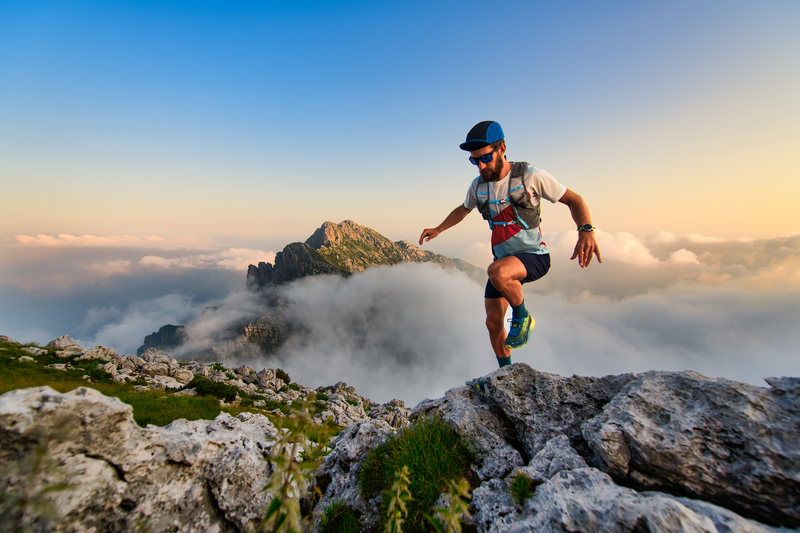
94% of researchers rate our articles as excellent or good
Learn more about the work of our research integrity team to safeguard the quality of each article we publish.
Find out more
ORIGINAL RESEARCH article
Front. Med. Technol. , 07 July 2021
Sec. Diagnostic and Therapeutic Devices
Volume 3 - 2021 | https://doi.org/10.3389/fmedt.2021.690442
This article is part of the Research Topic Highlights in Diagnostic and Therapeutic Devices 2021/22 View all 9 articles
Background: Patient-ventilator synchronization during non-invasive ventilation (NIV) can be assessed by visual inspection of flow and pressure waveforms but it remains time consuming and there is a large inter-rater variability, even among expert physicians. SYNCSMART™ software developed by Breas Medical (Mölnycke, Sweden) provides an automatic detection and scoring of patient-ventilator asynchrony to help physicians in their daily clinical practice. This study was designed to assess performance of the automatic scoring by the SYNCSMART software using expert clinicians as a reference in patient with chronic respiratory failure receiving NIV.
Methods: From nine patients, 20 min data sets were analyzed automatically by SYNCSMART software and reviewed by nine expert physicians who were asked to score auto-triggering (AT), double-triggering (DT), and ineffective efforts (IE). The study procedure was similar to the one commonly used for validating the automatic sleep scoring technique. For each patient, the asynchrony index was computed by automatic scoring and each expert, respectively. Considering successively each expert scoring as a reference, sensitivity, specificity, positive predictive value (PPV), κ-coefficients, and agreement were calculated.
Results: The asynchrony index assessed by SYNSMART was not significantly different from the one assessed by the experts (18.9 ± 17.7 vs. 12.8 ± 9.4, p = 0.19). When compared to an expert, the sensitivity and specificity provided by SYNCSMART for DT, AT, and IE were significantly greater than those provided by an expert when compared to another expert.
Conclusions: SYNCSMART software is able to score asynchrony events within the inter-rater variability. When the breathing frequency is not too high (<24), it therefore provides a reliable assessment of patient-ventilator asynchrony; AT is over detected otherwise.
Nocturnal non-invasive ventilation (NIV) is recognized as an effective treatment for chronic hypercapnic respiratory failure. Monitoring NIV during sleep is a necessary adjunct to daytime assessment of the treatment, which may affect prognosis, quality of sleep, or morning dyspnea (1–4). A systematic approach for determining undesired events such as leaks, upper airway obstruction (UAO) with or without a decrease in respiratory drive, and patient-ventilator asynchrony (PVA) from polygraphy performed under NIV was provided by the SomnoNIV group (5–7). An asynchrony index (AI) greater than 10% is quite often observed (8–12) and may be sleep-dependent (13). PVA is often the source of discomfort (11, 14). During long-term home NIV, a high prevalence of ineffective efforts (IEs) in patients with obstructive and restrictive diseases using polygraphic assessment was reported by Fanfulla et al. (13) and Guo et al. (10) and was later confirmed by Ramsay et al. with a parasternal EMG (12).
In general, waveforms of pressure and flow contain all the necessary information required for identifying the type of asynchrony events (AEs) (6, 7, 9, 11, 15–19). Pressure waveforms provide a direct access to ventilator cycles. The flow results from a combination between ventilator cycles and patient breathing cycles. Interpreting these waveforms is not always simple, primarily because the interplay between these two cycles is not trivial. Indeed, the ability of intensive care unit (ICU) physicians to score AEs is quite low (20, 21) and scarcely implemented due to a lack of skills (22). Nevertheless, IEs can be reliably detected by visual inspection (19). Specific training in mechanical ventilation increases the ability to identify AEs from waveforms, but the years of experience are not necessarily associated with a better ability to recognize the three main AEs [IE, double-triggering (DT), and auto-triggering (AT), the latter one being less often well-recognized] (21).
An automatic analysis of the pressure and flow waveforms is required to substantially shorten the long duration spent performing visual scoring (23). It was shown that detection of IEs by the means of an algorithm applied to the flow and pressure in the ventilation circuit was possible (24–29). All studies, but two, were performed in invasive ventilation where the leak is not relevant. Moreover, either only IEs were often considered (24, 25, 27) or only AEs without any further specifications (26, 28, 30). DT has only been considered in two studies (29, 31). ATs were never investigated with an automatic detection. It is therefore important to develop an algorithm to automatically detect the three main AEs (IE, DT, and AT) for NIV. The SyncSmart™ software was developed for such purpose, which works from the pressure and airflow waveforms sampled at the device rate (here 64 Hz). This study aimed to is to assess the ability of this algorithm to detect the three main PVA events during pressure support ventilation (PSV) by only processing flow and pressure waveforms.
Nine stable patients monitored under NIV (PSV mode with a backup frequency) in the Pneumology Unit Care of the Corporació Parc Tauli (Sabadell, Spain) were included in this study. The data were randomly selected from a study focused on the prevalence of AEs. This study was approved by the local ethics committee of Corporació Parc Tauli (CIR2010/015). Written informed consent was obtained from patients. Nine expert physicians from France, Germany, Italy, The Netherlands, Spain, Turkey, and the United Kingdom reviewed the automatic scorings.
Two restrictive patients, one obstructive patient, three patients with chronic obstructive pulmonary disease (COPD), and three patients with amyotrophic lateral sclerosis (ALS) were selected. They were ventilated with different devices: three with a Vivo 40 (Beas Medical, Mölnlycke, Sweden), four with a Lumis 150, one with an Astral 150 (ResMed, North Ryde, Australia), and one with a Trilogy (Philips Respironics, Murrysville, USA). Each patient used a full-face mask. The waveforms were recorded in napping patients in their most comfortable position during the initiation of mechanical ventilation or during routine controls in a quiet room. Flow Q, airway pressure Paw, and belt waveforms Bthorax and Babdom were measured. The data were acquired using an external polygraph (Powerlab 16Sp, ADInstruments, Sydney, Australia), equipped with a pressure transducer (model 1050, ADInstruments, Sydney, Australia) and a pneumotachograph (model S300, instrumental dead space 70 ml, resistance rp = 0.0018 cmH2O/l/s, ADInstruments, Sydney, Australia), both inserted in the ventilation circuit close to the mask and with respiratory inductance plethysmography belts (Pro-Tech, Canada). The polygraph was connected to a computer equipped with data acquisition software (Chart 7.0, ADInstruments, Sydney, Australia). The sampling frequency of measurements was fs = 1, 000 Hz, but the data were then resampled at the frequency fV = 64 Hz, as used by SyncSmart software. The acquired data were read by the SyncSmart software in a text format.
For each patient, we recorded a long session of NIV. One 20-min window of data was extracted from each of the recordings: The selected data window starts at least 5 min after the beginning of the session to avoid transient patient-ventilator interactions as commonly observed. No other criterion was used to select these windows. The belt signals, only used by the expert physicians to review the automatic scoring produced by the SyncSmart software, were filtered to remove the long-term drift and to improve their readability. A typical excerpt of the four measured waveforms with the total (intentional and non-intentional) leakage computed by SyncSmart is shown in Figure 1.
Figure 1. Excerpt from the 20-min times series recorded in patient 1. The leakage Φ is averaged over each ventilatory cycle. The belt signals Bthorax and Babdom were provided to the physicians for reviewing the automatic scoring. The ventilatory cycles at t = 713 s and t = 728 s (marked with red cross) were automatically discarded by SyncSmart due to its excessively turbulent aspect and because the leakage Φ exceeds the threshold Φt, here equal to 18 l min−1.
The SyncSmart software is analyzes the pressure Paw and the flow Q, either measured by the ventilator or by the external sensors as in the present protocol. The SyncSmart software considers three ventilator settings: expiratory positive airway pressure (EPAP), inspiratory positive airway pressure (IPAP), and the backup frequency (fbck) at which the ventilator delivers the pressure cycles. The software computes a leakage
where tinit is the time at which the inspiratory, effort is initiated and tend is the time at the end of expiration (tend is also the time at which the next inspiratory effort is initiated). According to this equation, the leakage Φ has a constant value over each cycle. This leakage is used to discard the excessively turbulent parts of the waveforms. As exemplified in Figure 1, it is impossible to reliably score these cycles, neither by visual inspection by expert physicians nor by an automatic algorithm. The corresponding ventilatory cycles are thus marked with a red cross and are discarded from the statistical analysis. The events scored by SyncSmart software are shown in Figure 2 and are defined as follows.
Figure 2. (A) Double-triggering. (B) Auto-triggering. (C) Ineffective effort. Examples of double-triggering (DT), auto-triggering (AT), and ineffective efforts (IEs) in a patient ventilated with a pressure support ventilation (PSV) mode. Waveforms of pressure P, flow Q, thoracic belt Bthorax, and total leakage Φ.
DT Double-triggered ventilatory cycles for which there are two pressure rises during one inspiratory effort;
AT Auto-triggered ventilatory cycles when the pressure rise is not triggered by an inspiratory effort and thus occurs during the expiratory phase;
IE Ineffective efforts when there is a patient inspiratory effort, which is not followed by a pressure cycle delivered by the ventilator;
Bck Backup cycles when the pressure rise is triggered by the ventilator according to the backup frequency fbck;
N Ventilatory cycles are considered normal when there is absence of one of the events described above.
The asynchrony index (AI) is defined as
where Ntot is the number of ventilatory cycles (N, Bck, DT, AT, IE, and discarded cycles). AI is expressed as a percentage.
All the physicians have been working with NIV for 21.5 ± 7 years and, consequently, are considered as experts with NIV. All of them were asked to independently review the automatic scoring of PVA produced by SyncSmart according to their skills and knowledge. Expert physicians were reviewing the scoring by visual inspection of the pressure and flow as used by the SyncSmart software in addition to the belt signals as recommended by Gonzales-Bermejo et al. (6) and Longhini et al. (32), providing a reliable gold standard scoring. Since there is a long experience in sleep scoring for reviewing automatic scoring, we chose to reproduce the corresponding methodology: starting from the automatic scoring, we asked each expert physicians to individually correct it, and then, we compared the corrected scoring among each other and with the automatic scoring (33–35). The software contains a functionality that allows the physician to review the automatic scoring and to correct the type of ventilatory cycle. The physician may also add or remove a ventilatory cycle. Since the expert physicians have the belt signals, they can identify UAO which may be defined by the decrease in patient flow during a pressure cycle delivered by the ventilator (6). By definition, there is no effective IE and the pressure rises are triggered by the backup frequency fbck: The SyncSmart software thus scored these ventilatory cycles as Bck cycles. Consequently, backup cycles scored as obstruction by physicians are retained as Bck cycles for the statistical analysis.
Physicians were thus asked to focus on the main PVA events, that is, on IE, AT, and DT. Physicians were moderately under “time pressure” since they had approximately 2 min to review 1 min of tracing. Each physician reviewed at least 110 min of tracing; consequently, each patient recording was reviewed by at least five expert physicians. The automatic scoring was then compared to the reviewed scoring of each expert, which was considered as a reference. Inter-rater comparisons were also computed by selecting successively each scorer as a reference and then by computing the mean values. The number of each type of AE and the was computed. We computed the sensitivity (Se), specificity (Sp) PPV, κ-coefficients, and agreement when SyncSmart scorings were compared with the scorings of the experts. These quantities were also computed to compare experts with each other as commonly carried out for assessing inter-rater agreement in sleep scoring (33–35). Student's t-test is used with a significance level at 0.05.
Characteristics of the patient at enrollment and ventilator settings are reported in Table 1. Five patients were well-established on mechanical ventilation, and four were recently initiated. A total of 4,201 ventilatory cycles were analyzed.
The nine physicians performed the analysis of the flow, pressure, and belt waveforms using the SyncSmart graphical interface. The number of AEs as scored by SyncSmart and as scored by the experts is reported for each patient in Table 2. On average, the SyncSmart software reported the same smaller numbers of DT (4.1 ± 3.6vs. 6.6 ± 13.0, p = 0.31) and IE (24.1±21.7vs. 22.0±21.5, p = 0.39) as the by experts. Conversely, the automatic scoring reported significantly more AT than the experts (71.7±88.0 vs. 25.5±35.3, p = 0.0036). The Se, Sp, PPV, κ-coefficient, and agreement are reported in Table 3 in which the results provided by SyncSmart software are successively compared with each expert, and where each expert is compared to one another. The AI assessed by SyncSmart is not significantly different from the at assessed by the experts (18.9 ± 17.7% vs. 12.8 ± 9.4%, p = 0.19) as shown in Figure 3. Se and Sp provided by SyncSmart for N, Bck, DT, and IE are significantly greater than those provided by experts when compared to one another. The PPV for IE is significantly greater for the automatic scoring than for experts. The inter-scorer variability for DT and AT is nearly twice that of IE. AT is clearly the event that leads to the most important discrepancies between the automatic scoring and the experts (Table 2), particularly for patients 5, 7, and 8 (Table 4).
Table 3. Sensitivity (Se), specificity (Sp), positive predictive value (PPV), κ-coefficient, and agreement for the automatic scoring by SyncSmart successively compared to each expert and for each expert successively compared to one another.
Figure 3. Asynchrony index (AI) computed from the SyncSmart scoring and the mean AI (with the standard deviation) computed from the expert scorings for each patient and for all patients, respectively.
Table 4. Sensitivity (Se), specitify (Sp), PPV, κ-coefficient, and agreement between (i) the experts and the automatic scoring by SyncSmart and (ii) between experts, successively computed for each patient.
This study confirms that the inter-rater variability between physicians to detect PVA events during NIV by visual inspection is pretty large for the three main AEs (DT, AT, and IE). This was already shown by Longhini et al. (32). Most often physicians recognize correctly the presence of an AE but they had difficulties to discriminate them; for instance, it is rather difficult to distinguish DT from a combination of AT-N. Our results show that the events detected by automatic scoring were well recognized by expert physicians but scored as a different AE. This explains why the inter-rater variability is greater than the disagreement with the automatic scoring. This partly explains the large inter-rater variability for determining the type of PVA although the inter-rater variability in the AI is rather low (the variance is equal to 9.9%). For six of the nine patients, there is a slight trend in SyncSmart to miss some AEs (about 10% as detailed in Table 2) as exemplified with the AT shown in Figure 1 (t = 738 s) that is considered as a normal cycle. It is also known that IE may be missed from a simple waveform analysis (36). Conversely, in three patients (5, 7, and 8), there was an over detection of AT. Over- detection of AT occurred in two patients with ALS and one patient with COPD whose breathing frequencies were greater than 24 breath per min (see Table 2). Indeed, the other six patients had a breathing frequency that is lower and AT as correctly detected. Even with this overdetection, the main result of this study is that automatic scoring is within the inter-rater variability and provides, in general, a lower bound for the AI.
To our knowledge, this pilot study is the first attempt to the assess performance of software to automatically detect DT, AT, and IE from the pressure and flow waveforms. In most of the previous studies, only IEs were investigated (24, 25, 27, 31). These different algorithms were detecting IEs with Se and Sp greater than 0.90 as observed with SyncSmart. One study investigated DT in 67 patients but there is no information on the validation of the algorithm (29). Two studies investigated AE without distinguishing them. One reported the AI with a Se and a Sp at about 0.90 (28). The second one investigated the asynchrony between patient and ventilator (30). The latter study was, in fact, devoted to neurally adjusted non-invasive ventilator (NAVA), and the algorithm uses the diaphragm electrical activity, pressure, and flow waveforms. So, its purpose was different from our pilot study. None of these studies, whose main characteristics are reported in Table 5, investigated AT, which is, as revealed in this study, the most difficult AE to determine, even when the belt signal is provided.
Table 5. Brief overview of the studies devoted to automatic scoring of AE in mechanical ventilation.
In the study of Mulqueeny et al. (31), DTs were defined as two pressure cycles separated by less than 500 ms. This definition does not allow to distinguish a DT from a combination of AT-N. Distinguishing these two types of AEs is indeed sometimes very difficult, thus explaining the low Se found for these two types of AE, from the SyncSmart software as well as from the experts. In DT, the first oscillation of the flow has an amplitude that is larger than the second one (7, 37). During AT, the patient is still expiring: the inspired volume should be therefore smaller than for that normal cycle, thus inducing lower amplitude flow waveforms (7, 37). This is a possible way to discriminate DT from AT-N (37). It seems that when the breathing frequency is too high, such feature is no longer reliable: this could explain the over detection of AT observed in our study.
Some studies show that more than 30% of patients in NIV have more than 10% of AEs (11, 38, 39). In our cohort, 44% of patients had AI >30%. Omitting the over detected AT, 349 AEs were actually detected. This is not sufficient for robust results and investigating a larger cohort is required. Nevertheless, previous study showed that the detection of AEs is inversely related to their prevalence, indicating that the ability to recognize PVA is reduced when their occurrence increases (20, 32). For these reasons, asynchrony events were not too numerous during our ventilation sessions for optimizing the ability of physicians to correctly recognize the asynchrony events. The Se, PPV, κ-coefficients, and agreement are the lowest for the three patients, for which AT was over detected and, consequently, with AI>34%. Clearly, this drawback of SyncSmart should be corrected. As physicians are commonly doing for sleep scoring (40), in our study, experts start also from the automatic scoring.
There are possible limitations in this study. The first being that the experts were not blind to automatic scores and had, in fact, to reviewed them. This may create a bias by reducing the inter-rater variability compared to scoring from raw tracings. Nevertheless, even with this bias, the statistics (κ-coefficients and agreement as reported in Table 3) are still in favor of automatic scoring since the expert scoring better matches with the automatic scoring than with each other. Indeed, the modified scores very often differ from one expert to the other. With blind scoring, the results becomes intractable as we observed in a preliminary study (non-published), leading us to adopt the procedure commonly used to assess the performance of automatic sleep scoring. As the aim of the present pilot study is to evaluate the SyncSmart software as a proof of concept, further study is warranted to evaluate it for longer NIV sessions and for a larger cohort of patients.
There is not yet a standardization for coding AEs for patients using NIV. There are few contributions in that direction which are based on visual inspection (6, 7, 41) and, consequently, which use qualitative arguments that may sometimes lead to subjective interpretation. Another problem is related to obstructions that are not considered in the present study. Two of the patients have a noticeable number of obstructions. These events were merged with backup cycles. In this study, as it is considered by the SyncSmart software, the ventilatory cycles are discarded (<5% of the number of breaths) from the analysis when leaks are too large: This avoids inappropriate tracings that could prevent physicians from correctly detecting PVA. The presence of high leakage is to be considered as a primary event during NIV, which needs to be corrected before any other action, adjustment of treatment, or settings that can be considered or recommended.
It should be noted that, in the daily clinical practice, physicians use “visual” signs as chest movement or signs of discomfort and possibly feedback provided by the patient. In addition to the waveforms (pressure, flow, and belt signal), the SyncSmart software provides the patient and ventilator frequencies, which are useful to identify PVA. These last two limitations are assumed to be a source for increasing the inter-rater variability (32). Since “minor” AEs as advanced and delayed cycling (pressure release) (42, 43) are not detected by the SyncSmart software, they were not considered in this study. As they are suspected to be important for inducing major AEs, detecting them would lead to a better understanding of the causes of the main events.
When the AI is typically greater than 10%, an adjustment of the ventilator settings is required (9, 11, 20). Since these adjustments are event type dependent, it is relevant to discriminate DT, AT, and IE. DT occurs to restrictive patient with a low breathing frequency (37). AT may contribute for 40% of all AEs (32). As pointed out by Longhini et al., “because of the poor performance of visual inspection of ventilator waveforms, algorithms able to recognize patient-ventilator asynchrony might indeed represent an important advance for the management of patient underlying NIV” (32). SyncSmart overcomes the lack of a tool for helping physicians to identify the source for poor mechanical coupling between patient and ventilator.
To our knowledge, this is the first study to show that it is possible to automatically detect AEs from solely pressure and flow waveforms and that the results are within the inter-rater variability. This pilot study shows that such a procedure can be used to validate automatic scoring of AEs. Most of the events from more than 4,200 ventilatory cycles were well-detected by the SyncSmart software. When the breathing frequency is lower than 24 breath per min, SyncSmart returns an AI slightly less than the at assessed by expert physicians. A validation with a larger cohort is required to evaluate whether the AI provided by automatic scoring could be considered as a lower bound.
The datasets analyzed for this study can be found in the web page http://www.atomosyd.net/spip.php?article222
The studies involving human participants were reviewed and approved by Corporacio Parc Tauli (CIR2010/015). The patients/participants provided their written informed consent to participate in this study.
All the authors contributed to the literature search and to the study design. ML collected the data measured in the patients in the Pneumology Unit Care of the Corporacio Parc Tauli (Sabadell, Spain). All the authors, but CL, reviewed the automatic scoring provided by the software. CL processed the data provided by the software and by the manual review of the physician. The analysis of the data was performed by all the authors. The manuscript was prepared by CL and reviewed by all the other authors.
This study received funding from Breas Medical. The funder was not involved in study design, collection, analysis, interpretation of data, the writing of this article, or the decision to submit it for publication.
ML reports personal fees for lectures from Philips and Resmed. JA is part time employee at Hamilton Medical. He has received advisory honorarium from Breas Medical and ResMed. He has received honorarium for lecturing from Philips. AC has received fees for lecturing and roundtable meetings from Philips, Breas and Resmed. MC has received advisory honorarium from Breas Medical and ResMed. She has received honorarium for lecturing from, Philips, ResMed, Breas and MPR. CL has received fees for consulting from Breas Medical. JS reports grants and personal fees for lectures from Heinen und Löwenstein and VitalAire, grants, personal fees for lectures and non-financial support for meeting attendance from Vivisol GmbH, grants from Weinmann Deutschland, personal fees for consultancy/advisory board work from Breas Medical, during the conduct of the study; personal fees for consultancy and lectures, and non-financial support for meeting attendance from Boehringer Ingelheim Pharma, personal fees for consultancy and lectures from SenTec AG, Keller Medical GmbH, Linde Deutschland and Santis GmbH, outside the submitted work. NH has received unrestricted research grants from Philips, Philips-Respironics, B&D Electromedical, Breas and Fisher-Paykel. He has has received fees for lecturing and roundtable meetings from Philips, Philips-Respironics, B&D Electromedical, Breas and Fisher-Paykel. SN has received fees for lecturing and roundtable meetings from Philips, Breas and Resmed. He is on the advisory board for Breas and Philips.
The remaining authors declare that the research was conducted in the absence of any commercial or financial relationships that could be construed as a potential conflict of interest.
This study would not have been possible without the great assistance of Sally Cozens, Carl van Loey, Didier Menguy, and Sebastian Mommers.
1. Adler D, Perrig S, Takahashi H, Espa F, Rodenstein D, Pépin JL, et al. Polysomnography in stable COPD under non-invasive ventilation to reduce patient-ventilator asynchrony and morning breathlessness. Sleep Breath. (2012) 16:1081–90. doi: 10.1007/s11325-011-0605-y
2. Gonzalez-Bermejo J, Morelot-Panzini C, Arnol N, Meininger V, Kraoua S, Salachas F, et al. Prognostic value of efficiently correcting nocturnal desaturations after one month of non-invasive ventilation in amyotrophic lateral sclerosis: a retrospective monocentre observational cohort study. Amyotroph Lateral Scler Frontotemporal Degener. (2013) 14:373–9. doi: 10.3109/21678421.2013.776086
3. Vrijsen B, Testelmans D, Belge C, Robberecht W, Damme PV, Buyse B. Non-invasive ventilation in amyotrophic lateral sclerosis. Amyotroph Lateral Scler Frontotemporal Degener. (2013) 14:85–95. doi: 10.3109/21678421.2012.745568
4. Georges M, Attali V, Golmard JL, Morélot-Panzini C, Crevier-Buchman L, Collet JM, et al. Reduced survival in patients with ALS with upper airway obstructive events on non-invasive ventilation. J Neurol Neurosurg Psychiatry. (2016) 87:1045–50. doi: 10.1136/jnnp-2015-312606
5. Rabec C, Rodenstein D, Léger P, Rouault S, Perrin C, Gonzalez-Bermejo J, et al. Ventilator modes and settings during non-invasive ventilation: effects on respiratory events and implications for their identification. Thorax. (2011) 66:170–8. doi: 10.1136/thx.2010.142661
6. Gonzalez-Bermejo J, Perrin C, Janssens JP, Pepin JL, Mroue G, Léger P, et al. Proposal for a systematic analysis of polygraphy or polysomnography for identifying and scoring abnormal events occurring during non-invasive ventilation. Thorax. (2012) 67:546–52. doi: 10.1136/thx.2010.142653
7. Gonzalez-Bermejo J, Janssens JP, Rabec C, Perrin C, Lofaso F, Langevin B, et al. Framework for patient-ventilator asynchrony during long-term non-invasive ventilation. Thorax. (2019) 74:715–7. doi: 10.1136/thoraxjnl-2018-213022
8. Chao DC, Scheinhorn DJ, Stearn-Hassenpflug M. Patient-ventilator trigger asynchrony in prolonged mechanical ventilation. Chest. (1997) 112:1592–9. doi: 10.1378/chest.112.6.1592
9. Thille AW, Rodriguez P, Cabello B, Lellouche F, Brochard L. Patient-ventilator asynchrony during assisted mechanical ventilation. Intens Care Med. (2006) 32:1515–22. doi: 10.1007/s00134-006-0301-8
10. Guo YF, Sforza E, Janssens JP. Respiratory patterns during sleep in obesity-hypoventilation patients treated with nocturnal pressure support: a preliminary report. Chest. (2007) 131:1090–9. doi: 10.1378/chest.06-1705
11. Vignaux L, Vargas F, Roeseler J, Tassaux D, Thille AW, Kossowsky MP, et al. Patient-ventilator asynchrony during non-invasive ventilation for acute respiratory failure: a multicenter study. Intens Care Med. (2009) 35:840–6. doi: 10.1007/s00134-009-1416-5
12. Ramsay M, Mandal S, Suh ES, Steier J, Douiri A, Polkey MI, et al. Parasternal electromyography to determine the relationship between patient-ventilator asynchrony and nocturnal gas exchange during home mechanical ventilation set up. Thorax. (2015) 70:946–52. doi: 10.1136/thoraxjnl-2015-206944
13. Fanfulla F, Taurino AE, Lupo ND, Trentin R, D'Ambrosio C, Nava S. Effect of sleep on patient/ventilator asynchrony in patients undergoing chronic non-invasive mechanical ventilation. Respir Med. (2007) 101:1702–7. doi: 10.1016/j.rmed.2007.02.026
14. Vitacca M, Bianchi L, Zanotti E, Vianello A, Barbano L, Porta R, et al. Assessment of physiologic variables and subjective comfort under different levels of pressure support ventilation. Chest. (2004) 126:851–9. doi: 10.1378/chest.126.3.851
15. Dhand R. Ventilator graphics and respiratory mechanics in the patient with obstructive lung disease. Respir Care. (2005) 50:246–61.
16. Georgopoulos D, Prinianakis G, Kondili E. Bedside waveforms interpretation as a tool to identify patient-ventilator asynchronies. Intens Care Med. (2006) 32:34–47. doi: 10.1007/s00134-005-2828-5
17. Camporota L. Bedside detection of patient-ventilation asynchrony. Signa Vitae. (2016) 11(Suppl. 2):31–4. doi: 10.22514/SV112.062016.5
18. Blanch L, Arnal JM, Mojoli F. Optimising patient-ventilator synchronisation. ICU Manage Pract. (2018) 18:supplements.
19. Ciorba C, Gonzalez-Bermejo J, Salva MAQ, Annane D, Orlikowski D, Lofaso F, et al. Flow and airway pressure analysis for detecting ineffective effort during mechanical ventilation in neuromuscular patients. Chronic Respir Dis. (2018) 16:1–9. doi: 10.1177/1479972318790267
20. Colombo D, Cammarota G, Alemani M, Carenzo L, Barra FL, Vaschetto R, et al. Efficacy of ventilator waveforms observation in detecting patient-ventilator asynchrony. Crit Care Med. (2011) 39:2452–7. doi: 10.1097/CCM.0b013e318225753c
21. Ramirez II, Arellano DH, Adasme RS, Landeros JM, Salinas FA, Vargas AG, et al. Ability of ICU health-care professionals to identify patient-ventilator asynchrony using waveform analysis. Respir Care. (2017) 62:144–9. doi: 10.4187/respcare.04750
22. Enrico B, Cristian F, Stefano B, Luigi P. Patient-ventilator asynchronies: types, outcomes and nursing detection skills. Acta Bio Med Atenei Parmensis. (2018) 89:6–18. doi: 10.23750/abm.v89i7-S.7737
23. Luján M, Sogo A, Monsó E. Home mechanical ventilation monitoring software: measure more or measure better? Arch Bronconeumol. (2012) 48:141–86. doi: 10.1016/j.arbr.2011.10.008
24. Chen CW, Lin WC, Hsu CH, Cheng KS, Lo CS. Detecting ineffective triggering in the expiratory phase in mechanically ventilated patients based on airway flow and pressure deflection: feasibility of using a computer algorithm. Crit Care Med. (2008) 36:455–61. doi: 10.1097/01.CCM.0000299734.34469.D9
25. Cuvelier A, Achour L, Rabarimanantsoa H, Letellier C, Muir JF, Fauroux B. A noninvasive method to identify ineffective triggering in patients with noninvasive pressure support ventilation. Respiration. (2010) 80:198–206. doi: 10.1159/000264606
26. Gutierrez G, Ballarino GJ, Turkan H, Abril J, Cruz LDL, Edsall C, et al. Automatic detection of patient-ventilator asynchrony by spectral analysis of airway flow. Crit Care. (2011) 15:R167. doi: 10.1186/cc10309
27. Blanch L, Sales B, Montanya J, Lucangelo U, Garcia-Esquirol O, Villagra A, et al. Validation of the Better Care® system to detect ineffective efforts during expiration in mechanically ventilated patients: a pilot study. Intens Care Med. (2012) 38:772–80. doi: 10.1007/s00134-012-2493-4
28. Chiew YS, Pretty CG, Beatson A, Glassenbury D, Major V, Corbett S, et al. Automated logging of inspiratory and expiratory non-synchronized breathing (ALIEN) for mechanical ventilation. In: 2015 37th Annual International Conference of the IEEE Engineering in Medicine and Biology Society (EMBC). Milan (2015). p. 5315–8.
29. de Haro C, López-Aguilar J, Magrans R, Montanya J, Fernández-Gonzalo S, Turon M, et al. Double cycling during mechanical ventilation: frequency, mechanisms, and physiologic implications. Crit Care Med. (2018) 46:1385–92. doi: 10.1097/CCM.0000000000003256
30. Doorduin J, Sinderby CA, Beck J, van der Hoeven JG, Heunks LM. Automated patient-ventilator interaction analysis during neurally adjusted non-invasive ventilation and pressure support ventilation in chronic obstructive pulmonary disease. Crit Care. (2014) 18:550. doi: 10.1186/cc13489
31. Mulqueeny Q, Ceriana P, Carlucci A, Fanfulla F, Delmastro M, Nava S. Automatic detection of ineffective triggering and double triggering during mechanical ventilation. Intens Care Med. (2007) 33:2014–8. doi: 10.1007/s00134-007-0767-z
32. Longhini F, Colombo D, Pisani L, Idone F, Chun P, Doorduin J, et al. Efficacy of ventilator waveform observation for detection of patient—ventilator asynchrony during NIV: a multicentre study. ERJ Open Res. (2017) 3:00075. doi: 10.1183/23120541.00075-2017
33. Norman RG, Pal I, Stewart C, Walsleben JA, Rapoport DM. Interobserver agreement among sleep scorers from different centers in a large dataset. Sleep. (2000) 23:901–8. doi: 10.1093/sleep/23.7.1e
34. Berthomier C, Drouot X, Herman-Stoïca M, Berthomier P, Prado J, Bokar-Thire D, et al. Automatic analysis of single-channel sleep EEG: validation in healthy individuals. Sleep. (2007) 30:1587–95. doi: 10.1093/sleep/30.11.1587
35. Stepnowsky C, Levendowski D, Popovic D, Ayappad I, Rapoport DM. Scoring accuracy of automated sleep staging from a bipolar electroocular recording compared to manual scoring by multiple raters. Sleep Med. (2013) 14:1199–207. doi: 10.1016/j.sleep.2013.04.022
36. Younes M, Brochard L, Grasso S, Kun J, Mancebo J, Ranieri M, et al. A method for monitoring and improving patient: ventilator interaction. Intens Care Med. (2007) 33:1337–46. doi: 10.1007/s00134-007-0681-4
37. Fresnel E. Étude comparative des performances des ventilateurs de domicile et analyse des interactions patient-ventilateur en ventilation non invasive. Normandie University. Rouen, France (2016).
38. Vignaux L, Tassaux D, Carteaux G, Roeseler J, Piquilloud L, Brochard L, et al. Performance of noninvasive ventilation algorithms on ICU ventilators during pressure support: a clinical study. Intens Care Med. (2010) 36:2053–9. doi: 10.1007/s00134-010-1994-2
39. Carlucci A, Pisani L, Ceriana P, Malovini A, Nava S. Patient-ventilator asynchronies: may the respiratory mechanics play a role? Crit Care. (2013) 17:R54. doi: 10.1186/cc12580
40. Rotem AY, Sperber AD, Krugliak P, Freidman B, Tal A, Tarasiuk A. Polysomnographic and actigraphic evidence of sleep fragmentation in patients with irritable bowel syndrome. Sleep. (2003) 26:747–52. doi: 10.1093/sleep/26.6.747
41. Janssens JP, Borel JC, Pépin JL, Group S. Nocturnal monitoring of home non-invasive ventilation: the contribution of simple tools such as pulse oximetry, capnography, built-in ventilator software and autonomic markers of sleep fragmentation. Thorax. (2011) 66:438–45. doi: 10.1016/j.rmr.2013.08.003
42. Calderini E, Confalonieri M, Puccio PG, Francavilla N, Stella L, Gregoretti C. Patient-ventilator asynchrony during noninvasive ventilation: the role of expiratory trigger. Intensive Care Med. (1999) 25:662–7. doi: 10.1007/s001340050927
Keywords: non-invasive ventilation, patient ventilator asynchrony, chronic obstructive pulmonary disease, ineffective triggering, monitoring, automatic scoring
Citation: Letellier C, Lujan M, Arnal J-M, Carlucci A, Chatwin M, Ergan B, Kampelmacher M, Storre JH, Hart N, Gonzalez-Bermejo J and Nava S (2021) Patient-Ventilator Synchronization During Non-invasive Ventilation: A Pilot Study of an Automated Analysis System. Front. Med. Technol. 3:690442. doi: 10.3389/fmedt.2021.690442
Received: 02 April 2021; Accepted: 28 May 2021;
Published: 07 July 2021.
Edited by:
Dinesh Kumar, RMIT University, AustraliaReviewed by:
Behzad Aliahmad, RMIT University, AustraliaCopyright © 2021 Letellier, Lujan, Arnal, Carlucci, Chatwin, Ergan, Kampelmacher, Storre, Hart, Gonzalez-Bermejo and Nava. This is an open-access article distributed under the terms of the Creative Commons Attribution License (CC BY). The use, distribution or reproduction in other forums is permitted, provided the original author(s) and the copyright owner(s) are credited and that the original publication in this journal is cited, in accordance with accepted academic practice. No use, distribution or reproduction is permitted which does not comply with these terms.
*Correspondence: Christophe Letellier, Y2hyaXN0b3BoZS5sZXRlbGxpZXJAdW5pdi1yb3Vlbi5mcg==
Disclaimer: All claims expressed in this article are solely those of the authors and do not necessarily represent those of their affiliated organizations, or those of the publisher, the editors and the reviewers. Any product that may be evaluated in this article or claim that may be made by its manufacturer is not guaranteed or endorsed by the publisher.
Research integrity at Frontiers
Learn more about the work of our research integrity team to safeguard the quality of each article we publish.