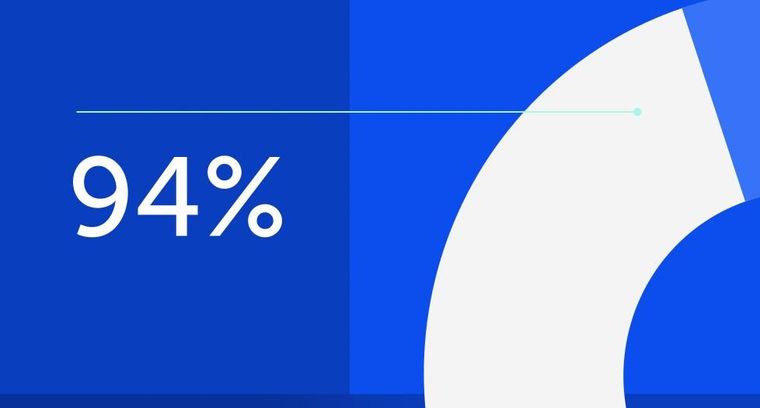
94% of researchers rate our articles as excellent or good
Learn more about the work of our research integrity team to safeguard the quality of each article we publish.
Find out more
EDITORIAL article
Front. Med., 11 April 2025
Sec. Intensive Care Medicine and Anesthesiology
Volume 12 - 2025 | https://doi.org/10.3389/fmed.2025.1593416
This article is part of the Research TopicClinical Application of Artificial Intelligence in Emergency and Critical Care Medicine, Volume VView all 15 articles
Editorial on the Research Topic
Clinical application of artificial intelligence in emergency and critical care medicine, volume V
This Research Topic explores the clinical applications of artificial intelligence (AI) in emergency and critical care medicine through 13 research articles. These studies systematically investigate how advanced data mining and AI techniques enhance risk assessment, diagnostic assistance, prognosis prediction, and treatment decision-making. Leveraging large-scale data resources and machine learning (ML) algorithms, these studies provide detailed analyses of complex conditions such as sepsis, acute pancreatitis, gastroparesis, acute kidney injury, disseminated intravascular coagulation (DIC), and heart failure with sepsis. From a data-driven perspective, this research offers a robust theoretical foundation and practical support for precision medicine.
AI is expected to significantly improve the prognosis of critically ill patients by assisting in disease identification, predicting disease progression, and supporting clinical decision-making (1). One key area of focus is the early prediction of sepsis, Yadgarov et al. conducted a systematic review of studies published from database inception to October 2023, searching Medline, PubMed, Google Scholar, and CENTRAL. Out of 3,953 studies, they analyzed 73 articles encompassing 457,932 sepsis patients and 256 models. Their findings demonstrated that ML models significantly outperformed traditional scoring systems in early sepsis prediction, with neural networks and decision tree models achieving the highest performance. Kim et al. developed a Transformer-based deep learning model for predicting ICU length of stay in sepsis patients, achieving a mean absolute error of only 2.05 days, demonstrating high accuracy and reliability. Beyond sepsis detection, Zhang et al. constructed a 28-day mortality prediction model specifically for sepsis patients with concurrent heart failure. Using the eICU-CRD database for model development and validating it externally on the MIMIC-IV database, they found that a logistic regression-based model achieved an AUC of 0.746 on the validation set, outperforming more complex algorithms such as XGBoost. The final model identified 10 key predictive features and employed the SHAP method to enhance interpretability, aiding clinicians in early identification of high-risk patients and optimizing resource allocation.
Traditional early warning systems primarily rely on vital signs and basic laboratory tests, whereas AI models integrating multi-source data allow for the incorporation of molecular and biological information into clinical decision-making. Hu et al. explored the combination of metagenomics, radiomics, and ML for sepsis diagnosis. By performing metagenomic sequencing on blood samples from sepsis patients and extracting radiomic features, they developed a fusion model that achieved an AUC close to 0.88 in its best-performing version. The integration of multimodal data helps overcome the limitations of single-indicator approaches, providing a novel strategy for the early and precise diagnosis of sepsis.
Furthermore, interpretable AI-based risk prediction models offer refined and individualized decision support in critical care medicine (2). Zhai et al. developed an interpretable XGBoost model for predicting in-hospital mortality risk in patients with severe pulmonary infections, achieving an AUC of 0.956. More importantly, the model incorporated SHAP and LIME methodologies to enhance interpretability. Such personalized risk assessment tools assist in mortality risk stratification and provide valuable clinical decision support. However, for AI models to truly assist in ICU decision-making, they must evolve from purely “predictive AI” to “actionable AI” capable of comparing the effects of different interventions. This transition necessitates the integration of causal inference to guide optimal treatment selection (3).
Beyond sepsis, AI technologies also hold significant clinical potential in other critical conditions. Tan et al. reviewed advancements in ML applications for predicting disease severity and complications in acute pancreatitis. Liu et al. utilized explainable ML to construct a postoperative gastroparesis risk prediction model, providing empirical evidence for early intervention. In another study, Wei et al. developed a nomogram for predicting early acute kidney injury (AKI) in patients with acute non-variceal upper gastrointestinal bleeding (NVUGIB) using data from the MIMIC-IV database, subsequently implementing it as a web-based clinical calculator.
AI also demonstrates immense potential in personalized treatment strategies. Traditional clinical guidelines often struggle to account for the unique physiological characteristics of individual patients, whereas AI-driven approaches offer the ability to support clinicians in devising more precise and tailored treatment plans (4). Nevertheless, while AI has demonstrated outstanding performance, it is crucial to recognize its limitations and reinforce validation and regulatory oversight in real-world applications to ensure tangible patient benefits (5).
Overall, Clinical application of artificial intelligence in emergency and critical care medicine, Volume V presents a comprehensive workflow from data acquisition and feature extraction to model construction and result interpretation. With advancements in multimodal data integration, causal inference modeling, and improvements in AI interpretability, AI is poised to become a powerful tool in sepsis management. We anticipate the deep integration of AI technologies into clinical medicine, fostering more precise and efficient workflows from early disease identification to treatment decision-making, ultimately contributing to lower mortality rates worldwide.
YW: Writing – original draft. RK: Writing – review & editing. PZ: Writing – review & editing. QM: Writing – review & editing. ZZ: Writing – review & editing.
The author(s) declare that financial support was received for the research and/or publication of this article. Zhongheng Zhang received funding from the China National Key Research and Development Program (2022YFC2504500), the Huadong Medicine Joint Funds of the Zhejiang Provincial Natural Science Foundation of China (LHDMD24H150001), the National Natural Science Foundation of China (82272180, 82472243), the China National Key Research and Development Program (2023YFC3603104), a collaborative scientific project co-established by the Science and Technology Department of the National Administration of Traditional Chinese Medicine and the Zhejiang Provincial Administration of Traditional Chinese Medicine (GZY-ZJ-KJ-24082), the General Health Science and Technology Program of Zhejiang Province (2024KY1099), and Project of Zhejiang University Longquan Innovation Center (ZJDXLQCXZCJBGS2024016).
The authors declare that the research was conducted in the absence of any commercial or financial relationships that could be construed as a potential conflict of interest.
The author(s) declared that they were an editorial board member of Frontiers, at the time of submission. This had no impact on the peer review process and the final decision.
All claims expressed in this article are solely those of the authors and do not necessarily represent those of their affiliated organizations, or those of the publisher, the editors and the reviewers. Any product that may be evaluated in this article, or claim that may be made by its manufacturer, is not guaranteed or endorsed by the publisher.
1. Saqib M, Iftikhar M, Neha F, Karishma F, Mumtaz H. Artificial intelligence in critical illness and its impact on patient care: a comprehensive review. Front Med. (2023) 10:1176192. doi: 10.3389/fmed.2023.1176192
2. Yang M, Chen H, Hu W, Mischi M, Shan C, Li J et al. Development and validation of an interpretable conformal predictor to predict sepsis mortality risk: retrospective cohort study. J Med Internet Res. (2024) 26:e50369. doi: 10.2196/50369
3. Smit JM, Krijthe JH, van Bommel J. The future of artificial intelligence in intensive care: moving from predictive to actionable AI. Intensive Care Med. (2023) 49:1114–6. doi: 10.1007/s00134-023-07102-y
4. Li F, Wang S, Gao Z, Qing M, Pan S, Liu Y, et al. Harnessing artificial intelligence in sepsis care: advances in early detection, personalized treatment, and real-time monitoring. Front Med. (2025) 11:1510792. doi: 10.3389/fmed.2024.1510792
Keywords: artificial intelligence, prediction, machine learning, critical care, treatment decision-making
Citation: Wang Y, Kashyap R, Zhang P, Meng Q and Zhang Z (2025) Editorial: Clinical application of artificial intelligence in emergency and critical care medicine, volume V. Front. Med. 12:1593416. doi: 10.3389/fmed.2025.1593416
Received: 14 March 2025; Accepted: 31 March 2025;
Published: 11 April 2025.
Edited and reviewed by: Ata Murat Kaynar, University of Pittsburgh, United States
Copyright © 2025 Wang, Kashyap, Zhang, Meng and Zhang. This is an open-access article distributed under the terms of the Creative Commons Attribution License (CC BY). The use, distribution or reproduction in other forums is permitted, provided the original author(s) and the copyright owner(s) are credited and that the original publication in this journal is cited, in accordance with accepted academic practice. No use, distribution or reproduction is permitted which does not comply with these terms.
*Correspondence: Zhongheng Zhang, emhfemhhbmcxOTg0QHpqdS5lZHUuY24=
Disclaimer: All claims expressed in this article are solely those of the authors and do not necessarily represent those of their affiliated organizations, or those of the publisher, the editors and the reviewers. Any product that may be evaluated in this article or claim that may be made by its manufacturer is not guaranteed or endorsed by the publisher.
Research integrity at Frontiers
Learn more about the work of our research integrity team to safeguard the quality of each article we publish.